对极几何与三角化求3D空间坐标
一,使用对极几何约束求R,T
第一步:特征匹配。提取出有效的匹配点
void find_feature_matches(const Mat &img_1, const Mat &img_2,std::vector<KeyPoint> &keypoints_1,std::vector<KeyPoint> &keypoints_2,std::vector<DMatch> &matches) {//-- 初始化Mat descriptors_1, descriptors_2;// used in OpenCV3Ptr<FeatureDetector> detector = ORB::create();Ptr<DescriptorExtractor> descriptor = ORB::create();// use this if you are in OpenCV2// Ptr<FeatureDetector> detector = FeatureDetector::create ( "ORB" );// Ptr<DescriptorExtractor> descriptor = DescriptorExtractor::create ( "ORB" );Ptr<DescriptorMatcher> matcher = DescriptorMatcher::create("BruteForce-Hamming");//-- 第一步:检测 Oriented FAST 角点位置detector->detect(img_1, keypoints_1);detector->detect(img_2, keypoints_2);//-- 第二步:根据角点位置计算 BRIEF 描述子descriptor->compute(img_1, keypoints_1, descriptors_1);descriptor->compute(img_2, keypoints_2, descriptors_2);//-- 第三步:对两幅图像中的BRIEF描述子进行匹配,使用 Hamming 距离vector<DMatch> match;// BFMatcher matcher ( NORM_HAMMING );matcher->match(descriptors_1, descriptors_2, match);//-- 第四步:匹配点对筛选double min_dist = 10000, max_dist = 0;//找出所有匹配之间的最小距离和最大距离, 即是最相似的和最不相似的两组点之间的距离for (int i = 0; i < descriptors_1.rows; i++) {double dist = match[i].distance;if (dist < min_dist) min_dist = dist;if (dist > max_dist) max_dist = dist;}printf("-- Max dist : %f \n", max_dist);printf("-- Min dist : %f \n", min_dist);//当描述子之间的距离大于两倍的最小距离时,即认为匹配有误.但有时候最小距离会非常小,设置一个经验值30作为下限.for (int i = 0; i < descriptors_1.rows; i++) {if (match[i].distance <= max(2 * min_dist, 30.0)) {matches.push_back(match[i]);}}
}
二、使用本质矩阵求解R,T
第二步:根据匹配点对,依据对极几何约束原理,求相机运动的R,t
void pose_estimation_2d2d(const std::vector<KeyPoint> &keypoints_1,const std::vector<KeyPoint> &keypoints_2,const std::vector<DMatch> &matches,Mat &R, Mat &t) {// 相机内参,TUM Freiburg2Mat K = (Mat_<double>(3, 3) << 520.9, 0, 325.1, 0, 521.0, 249.7, 0, 0, 1);//-- 把匹配点转换为vector<Point2f>的形式vector<Point2f> points1;vector<Point2f> points2;for (int i = 0; i < (int) matches.size(); i++) {points1.push_back(keypoints_1[matches[i].queryIdx].pt);points2.push_back(keypoints_2[matches[i].trainIdx].pt);}//-- 计算本质矩阵Point2d principal_point(325.1, 249.7); //相机主点, TUM dataset标定值int focal_length = 521; //相机焦距, TUM dataset标定值Mat essential_matrix;essential_matrix = findEssentialMat(points1, points2, focal_length, principal_point);//-- 从本质矩阵中恢复旋转和平移信息.recoverPose(essential_matrix, points1, points2, R, t, focal_length, principal_point);
}
三、由R,T三角化空间坐标
第三步:根据针孔相机模型的公式,由 R,t估计特征点的空间坐标
//三角化,根据匹配点和求解到的三维点。存储在points中
void triangulation(const vector<KeyPoint> &keypoint_1,const vector<KeyPoint> &keypoint_2,const std::vector<DMatch> &matches,const Mat &R, const Mat &t,vector<Point3d> &points) {Mat T1 = (Mat_<float>(3, 4) <<1, 0, 0, 0,0, 1, 0, 0,0, 0, 1, 0);//根据求解到的RT构造T2矩阵Mat T2 = (Mat_<float>(3, 4) <<R.at<double>(0, 0), R.at<double>(0, 1), R.at<double>(0, 2), t.at<double>(0, 0),R.at<double>(1, 0), R.at<double>(1, 1), R.at<double>(1, 2), t.at<double>(1, 0),R.at<double>(2, 0), R.at<double>(2, 1), R.at<double>(2, 2), t.at<double>(2, 0));//相机内参Mat K = (Mat_<double>(3, 3) << 520.9, 0, 325.1, 0, 521.0, 249.7, 0, 0, 1);vector<Point2f> pts_1, pts_2;for (DMatch m:matches) {// 将像素坐标转换至相机坐标pts_1.push_back(pixel2cam(keypoint_1[m.queryIdx].pt, K));pts_2.push_back(pixel2cam(keypoint_2[m.trainIdx].pt, K));}Mat pts_4d;cv::triangulatePoints(T1, T2, pts_1, pts_2, pts_4d);// 转换成非齐次坐标for (int i = 0; i < pts_4d.cols; i++) {Mat x = pts_4d.col(i);x /= x.at<float>(3, 0); // 归一化Point3d p(x.at<float>(0, 0),x.at<float>(1, 0),x.at<float>(2, 0));points.push_back(p);}
}
其中 triangulatePoints()的具体用法为
triangulatePoints(T1, T2, left, right, points_final) ;Mat T1 = (Mat_<float>(3, 4) <<1, 0, 0, 0,0, 1, 0, 0,0, 0, 1, 0);
Mat T2 = (Mat_<float>(3, 4) <<R.at<double>(0, 0), R.at<double>(0, 1), R.at<double>(0, 2), T.at<double>(0, 0),R.at<double>(1, 0), R.at<double>(1, 1), R.at<double>(1, 2), T.at<double>(1, 0),R.at<double>(2, 0), R.at<double>(2, 1), R.at<double>(2, 2), T.at<double>(2, 0));`
triangulatePoints(T1, T2, left, right, points_final) ;其中T2为3x4的[R|T]矩阵,left、right为相机坐标系下的归一化坐标,
因此不能直接使用提取到的像素坐标。应首先将像素坐标通过相机内参转化到相机坐标系下。
所以通过函数pixel2cam可将像素坐标转换到归一化相机坐标系下
归一化坐标:X=(u-u0)/fx
//像素坐标到归一化平面相机坐标的转换
Point2f pixel2cam(const Point2f& p, const Mat& K)
{return Point2f((p.x - K.at<double>(0, 2)) / K.at<double>(0, 0),(p.y - K.at<double>(1, 2)) / K.at<double>(1, 1));
}
四、代码demo
总的代码为:
#include <iostream>
#include <opencv2/opencv.hpp>
// #include "extra.h" // used in opencv2
using namespace std;
using namespace cv;void find_feature_matches(const Mat &img_1, const Mat &img_2,std::vector<KeyPoint> &keypoints_1,std::vector<KeyPoint> &keypoints_2,std::vector<DMatch> &matches);void pose_estimation_2d2d(const std::vector<KeyPoint> &keypoints_1,const std::vector<KeyPoint> &keypoints_2,const std::vector<DMatch> &matches,Mat &R, Mat &t);void triangulation(const vector<KeyPoint> &keypoint_1,const vector<KeyPoint> &keypoint_2,const std::vector<DMatch> &matches,const Mat &R, const Mat &t,vector<Point3d> &points
);/// 作图用
inline cv::Scalar get_color(float depth) {float up_th = 50, low_th = 10, th_range = up_th - low_th;if (depth > up_th) depth = up_th;if (depth < low_th) depth = low_th;return cv::Scalar(255 * depth / th_range, 0, 255 * (1 - depth / th_range));
}// 像素坐标转相机归一化坐标
Point2f pixel2cam(const Point2d &p, const Mat &K);int main(int argc, char **argv) {if (argc != 3) {cout << "usage: triangulation img1 img2" << endl;return 1;}//-- 读取图像Mat img_1 = imread(argv[1], CV_LOAD_IMAGE_COLOR);Mat img_2 = imread(argv[2], CV_LOAD_IMAGE_COLOR);vector<KeyPoint> keypoints_1, keypoints_2;vector<DMatch> matches;find_feature_matches(img_1, img_2, keypoints_1, keypoints_2, matches);cout << "一共找到了" << matches.size() << "组匹配点" << endl;//-- 估计两张图像间运动Mat R, t;pose_estimation_2d2d(keypoints_1, keypoints_2, matches, R, t);//-- 三角化vector<Point3d> points;//tr是三维点triangulation(keypoints_1, keypoints_2, matches, R, t, tr);//-- 验证三角化点与特征点的重投影关系Mat K = (Mat_<double>(3, 3) << 520.9, 0, 325.1, 0, 521.0, 249.7, 0, 0, 1);Mat img1_plot = img_1.clone();Mat img2_plot = img_2.clone();for (int i = 0; i < matches.size(); i++) {// 第一个图float depth1 = points[i].z;cout << "depth: " << depth1 << endl;Point2d pt1_cam = pixel2cam(keypoints_1[matches[i].queryIdx].pt, K);cv::circle(img1_plot, keypoints_1[matches[i].queryIdx].pt, 2, get_color(depth1), 2);// 第二个图Mat pt2_trans = R * (Mat_<double>(3, 1) << points[i].x, points[i].y, points[i].z) + t;float depth2 = pt2_trans.at<double>(2, 0);cv::circle(img2_plot, keypoints_2[matches[i].trainIdx].pt, 2, get_color(depth2), 2);}cv::imshow("img 1", img1_plot);cv::imshow("img 2", img2_plot);cv::waitKey();return 0;
}void find_feature_matches(const Mat &img_1, const Mat &img_2,std::vector<KeyPoint> &keypoints_1,std::vector<KeyPoint> &keypoints_2,std::vector<DMatch> &matches) {//-- 初始化Mat descriptors_1, descriptors_2;// used in OpenCV3Ptr<FeatureDetector> detector = ORB::create();Ptr<DescriptorExtractor> descriptor = ORB::create();// use this if you are in OpenCV2// Ptr<FeatureDetector> detector = FeatureDetector::create ( "ORB" );// Ptr<DescriptorExtractor> descriptor = DescriptorExtractor::create ( "ORB" );Ptr<DescriptorMatcher> matcher = DescriptorMatcher::create("BruteForce-Hamming");//-- 第一步:检测 Oriented FAST 角点位置detector->detect(img_1, keypoints_1);detector->detect(img_2, keypoints_2);//-- 第二步:根据角点位置计算 BRIEF 描述子descriptor->compute(img_1, keypoints_1, descriptors_1);descriptor->compute(img_2, keypoints_2, descriptors_2);//-- 第三步:对两幅图像中的BRIEF描述子进行匹配,使用 Hamming 距离vector<DMatch> match;// BFMatcher matcher ( NORM_HAMMING );matcher->match(descriptors_1, descriptors_2, match);//-- 第四步:匹配点对筛选double min_dist = 10000, max_dist = 0;//找出所有匹配之间的最小距离和最大距离, 即是最相似的和最不相似的两组点之间的距离for (int i = 0; i < descriptors_1.rows; i++) {double dist = match[i].distance;if (dist < min_dist) min_dist = dist;if (dist > max_dist) max_dist = dist;}printf("-- Max dist : %f \n", max_dist);printf("-- Min dist : %f \n", min_dist);//当描述子之间的距离大于两倍的最小距离时,即认为匹配有误.但有时候最小距离会非常小,设置一个经验值30作为下限.for (int i = 0; i < descriptors_1.rows; i++) {if (match[i].distance <= max(2 * min_dist, 30.0)) {matches.push_back(match[i]);}}
}void pose_estimation_2d2d(const std::vector<KeyPoint> &keypoints_1,const std::vector<KeyPoint> &keypoints_2,const std::vector<DMatch> &matches,Mat &R, Mat &t) {// 相机内参,TUM Freiburg2Mat K = (Mat_<double>(3, 3) << 520.9, 0, 325.1, 0, 521.0, 249.7, 0, 0, 1);//-- 把匹配点转换为vector<Point2f>的形式vector<Point2f> points1;vector<Point2f> points2;for (int i = 0; i < (int) matches.size(); i++) {points1.push_back(keypoints_1[matches[i].queryIdx].pt);points2.push_back(keypoints_2[matches[i].trainIdx].pt);}//-- 计算本质矩阵Point2d principal_point(325.1, 249.7); //相机主点, TUM dataset标定值int focal_length = 521; //相机焦距, TUM dataset标定值Mat essential_matrix;essential_matrix = findEssentialMat(points1, points2, focal_length, principal_point);//-- 从本质矩阵中恢复旋转和平移信息.recoverPose(essential_matrix, points1, points2, R, t, focal_length, principal_point);
}//三角化,根据匹配点和求解到的三维点。存储在points中
void triangulation(const vector<KeyPoint> &keypoint_1,const vector<KeyPoint> &keypoint_2,const std::vector<DMatch> &matches,const Mat &R, const Mat &t,vector<Point3d> &points) {Mat T1 = (Mat_<float>(3, 4) <<1, 0, 0, 0,0, 1, 0, 0,0, 0, 1, 0);//根据求解到的RT构造T2矩阵Mat T2 = (Mat_<float>(3, 4) <<R.at<double>(0, 0), R.at<double>(0, 1), R.at<double>(0, 2), t.at<double>(0, 0),R.at<double>(1, 0), R.at<double>(1, 1), R.at<double>(1, 2), t.at<double>(1, 0),R.at<double>(2, 0), R.at<double>(2, 1), R.at<double>(2, 2), t.at<double>(2, 0));//相机内参Mat K = (Mat_<double>(3, 3) << 520.9, 0, 325.1, 0, 521.0, 249.7, 0, 0, 1);vector<Point2f> pts_1, pts_2;for (DMatch m:matches) {// 将像素坐标转换至相机坐标pts_1.push_back(pixel2cam(keypoint_1[m.queryIdx].pt, K));pts_2.push_back(pixel2cam(keypoint_2[m.trainIdx].pt, K));}Mat pts_4d;cv::triangulatePoints(T1, T2, pts_1, pts_2, pts_4d);// 转换成非齐次坐标for (int i = 0; i < pts_4d.cols; i++) {Mat x = pts_4d.col(i);x /= x.at<float>(3, 0); // 归一化Point3d p(x.at<float>(0, 0),x.at<float>(1, 0),x.at<float>(2, 0));points.push_back(p);}
}Point2f pixel2cam(const Point2d &p, const Mat &K) {return Point2f((p.x - K.at<double>(0, 2)) / K.at<double>(0, 0),(p.y - K.at<double>(1, 2)) / K.at<double>(1, 1));
}
相关文章:
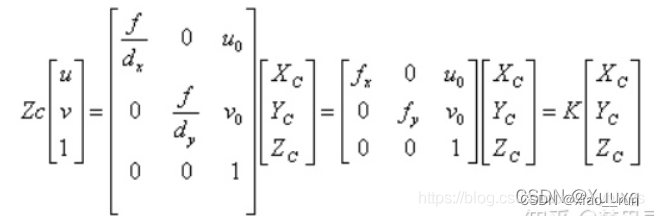
对极几何与三角化求3D空间坐标
一,使用对极几何约束求R,T 第一步:特征匹配。提取出有效的匹配点 void find_feature_matches(const Mat &img_1, const Mat &img_2,std::vector<KeyPoint> &keypoints_1,std::vector<KeyPoint> &keypoints_2,std::vector&l…...
英语语法笔记
1.英语五大句型 主谓(主语动词) 主谓宾(主语动词宾语) 主谓宾宾(主语动词简接宾语直接宾语) 主谓宾补(主语动词宾语宾语补语) 主系表(主语系动词主语补语) 1…...
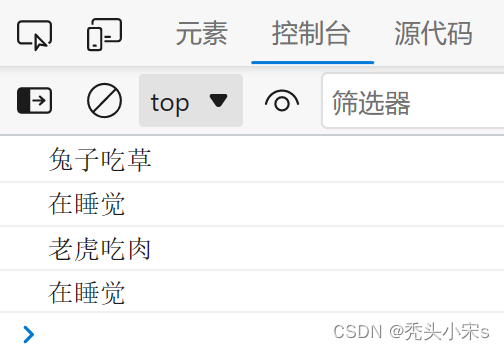
ES6的面向对象编程以及ES6中的类和对象
一、面向对象 1、面向对象 (1)是一种开发思想,并不是具体的一种技术 (2)一切事物均为对象,在项目中主要是对象的分工协作 2、对象的特征 (1)对象是属性和行为的结合体 &#x…...
ConfigMaps in K8s
摘要 ConfigMaps是Kubernetes(K8s)中用于存储应用程序配置信息的一种资源对象。它将key-value对存储为Kubernetes集群中的一个资源,并可以在Pod中以卷或环境变量的形式使用。 ConfigMaps的设计目的是将应用程序配置与应用程序本身解耦。它可…...

《机器人学一(Robotics(1))》_台大林沛群 第 6 周 【轨迹规划_直线转折处抛物线平滑】Quiz 6
步骤: 1、 编程 将PPT 的例子 跑一遍, 确保代码无误 2、根据题目 修改 相关参数 文章目录 求解代码_Python 解决的问题: 线段间转折点 的 速度 不连续 解决方法: 将直线段 两端 修正为 二次方程式 二次项圆滑 求解代码_Python …...
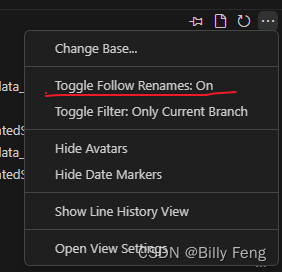
关于vscode的GitLens插件里的FILE HISTORY理解
最近在用vscode的GitLens插件开发项目遇到这个疑问,先看图: 每当我点击FILE HISTORY 一个commit时,正常来说显示器会自动将点击的提交版本和它上一个提交版本进行比较,如果单纯这么理解的话就错了,因为GitLens的File …...
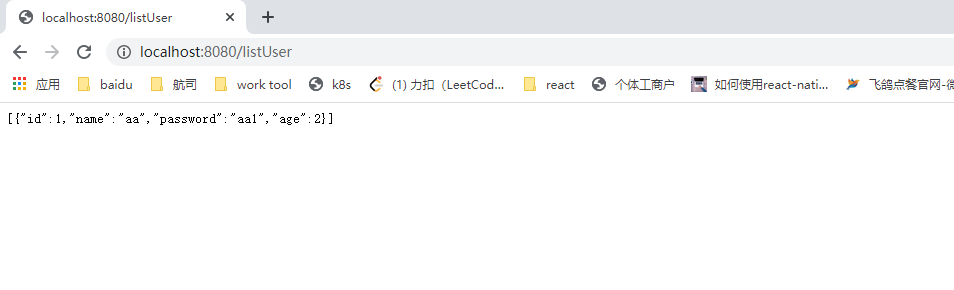
通过idea实现springboot集成mybatys
概述 使用springboot 集成 mybatys后,通过http请求接口,使得通过http请求可以直接直接操作数据库; 完成后端功能框架;前端是准备上小程序,调用https的请求接口用。简单实现后端框架; 详细 springboot 集…...
力扣(LeetCode)算法_C++——移位字符串分组
给定一个字符串,对该字符串可以进行 “移位” 的操作,也就是将字符串中每个字母都变为其在字母表中后续的字母,比如:“abc” -> “bcd”。这样,我们可以持续进行 “移位” 操作,从而生成如下移位序列&am…...
Vue2 与Vue3的区别?面试题
Vue 2和Vue 3是Vue.js框架的不同版本,在面试中经常涉及到它们之间的区别。以下是Vue 2和Vue 3的主要区别: 性能提升:Vue 3在性能方面进行了优化。Vue 3引入了更高效的Diff算法,提高了渲染性能。此外,Vue 3还进行了代码…...
java代码:Random和Scanner应用的小例子-猜数字小游戏
//java代码:Random和Scanner应用的小例子-猜数字小游戏 package com.test; import java.util.Random; import java.util.Scanner; /* * 需求:猜数字小游戏。 * 系统产生一个1-100之间的随机数,请猜出这个数据是多少? * * 分析…...
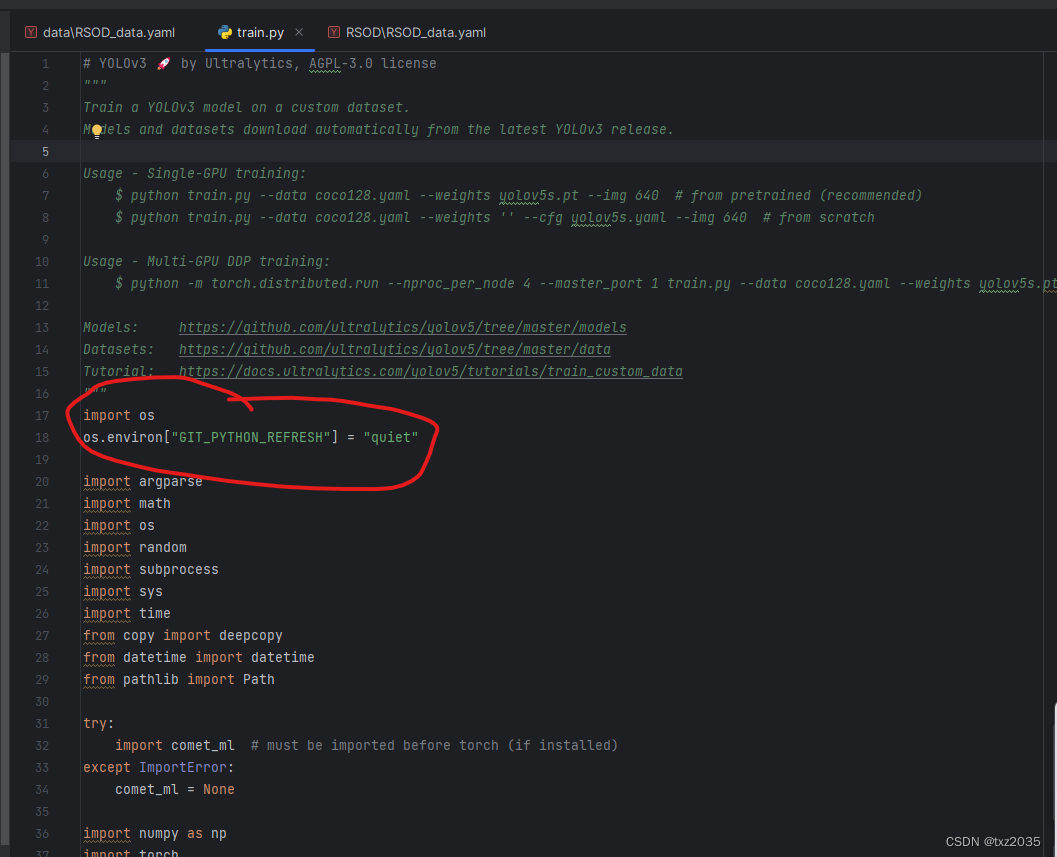
python调用git出错:ImportError: Failed to initialize: Bad git executable.
报错信息 #报错信息 Traceback (most recent call last): File “”, line 1, in File “C:\Python27\lib\site-packages\git_init_.py”, line 85, in raise ImportError(‘Failed to initialize: {0}’.format(exc)) ImportError: Failed to initialize: Bad git executab…...

【C语言】入门——指针
目录 编辑 1.指针是什么 2.指针类型和指针运算 2.1指针-整数 2.2指针-指针 2.3指针的关系运算 3.野指针 3.1野指针成因 👍指针未初始化: 👍指针越界访问: 👍指针指向空间释放: 3.2如何规避野指针 …...
C#_预处理指令
1. 预处理器指令指导编译器在实际编译开始之前对信息进行预处理。 所有的预处理器指令都是以 # 开始。且在一行上,只有空白字符可以出现在预处理器指令之前。预处理器指令不是语句,所以它们不以分号(;)结束。 C# 编译器没有一个单…...
容器命令(docker)
文章目录 前言一、docker容器命令0、准备工作1、新建容器并启动2、退出容器3、列出所有的运行的容器4、删除容器5、启动和停止容器的操作 总结 前言 本文主要介绍docker中与容器相关的一些命令,是对狂神课程的一些总结,作为一个手册帮助博主和使用docke…...
Vue3 ElementPlus el-cascader级联选择器动态加载数据
参考了这位的大佬的写法 element el-cascader动态加载数据 (多级联动,落地实现)_el-cascader 动态加载_林邵晨的博客-CSDN博客 <el-cascader style"width: 300px" :props"address" v-model"addressValue" …...
leetcode分类刷题:栈(Stack)(一、字符串相邻元素删除类型)
1、在leetcode分类刷题:基于数组的双指针(一、基于元素移除的O(1)类型)题目中,采用双指针之快慢指针的算法来解决。 2、字符串相邻元素的删除问题,用栈来进行管理,会非常有效;这种题型排在后面的…...
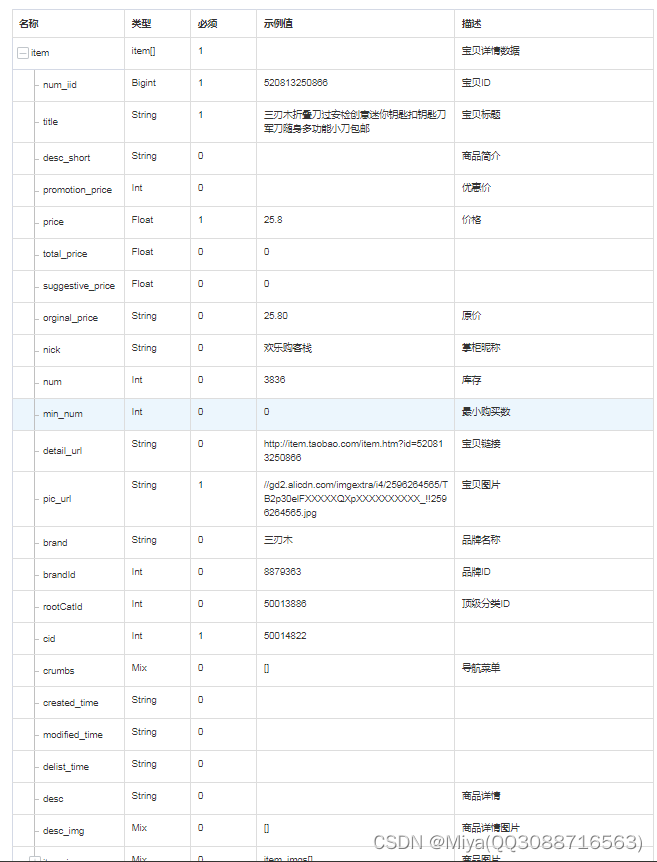
你还在找淘宝商品信息查询的接口吗?
你还在找淘宝商品信息查询的接口吗?,不用找了,我这有,免费测试 在很多行业,比如淘客、商品采集、刊登、数据分析行业都需要用到相关的商品接口,但是官方一般又没有开放这些接口,怎么办ÿ…...
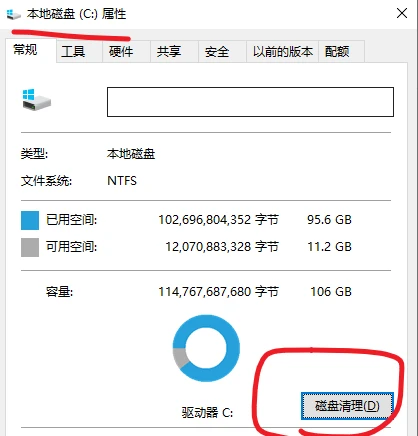
dll修复精灵,dll修复工具下载方法分享,mfc140u.dll缺失损坏一键修复
今天,我将为大家分享一个关于mfc140u.dll的问题。首先,我想问一下在座的网友们,有多少人知道mfc140u.dll是什么?又有多少人知道它的作用以及如何解决这个问题呢?在接下来的演讲中,我将详细介绍mfc140u.dll的…...
[LINUX使用] iptables tcpdump
iptables: 收到来自 10.10.10.10 的数据后都丢弃 iptables -I INPUT -s 10.10.10.10 -j DROP 直接 reject 来自 10.10.10.* 网段的数据 iptables -I INPUT -s 10.10.10.0/24 -j REJECT tcpdump: dump eth0的数据到本地 tcpdump -i eth0 -w dump.pcap 只抓 目的地址是 10…...
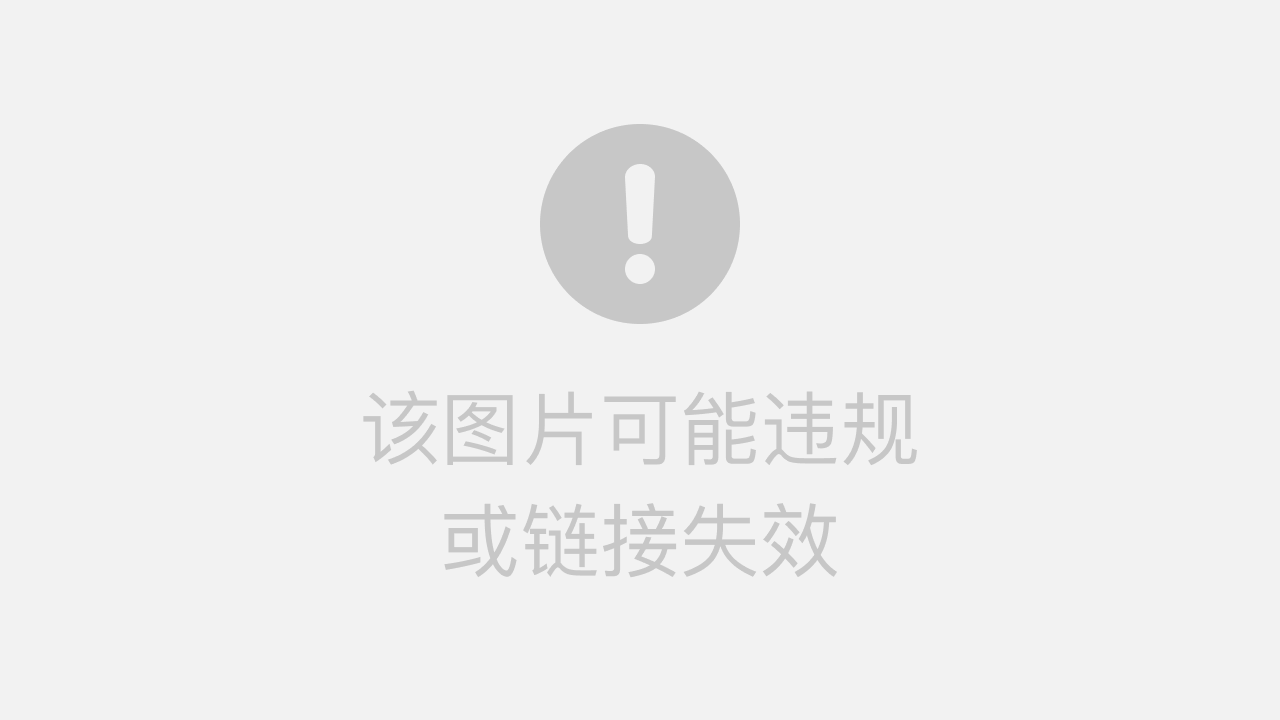
百度文心一率先言向全社会开放 应用商店搜“文心一言”可直接下载
8月31日,文心一言率先向全社会全面开放。广大用户可以在应用商店下载“文心一言APP”或登陆“文心一言官网”(https://yiyan.baidu.com) 体验。同时,企业用户可以直接登录百度智能云千帆大模型平台官网,调用文心一言能…...
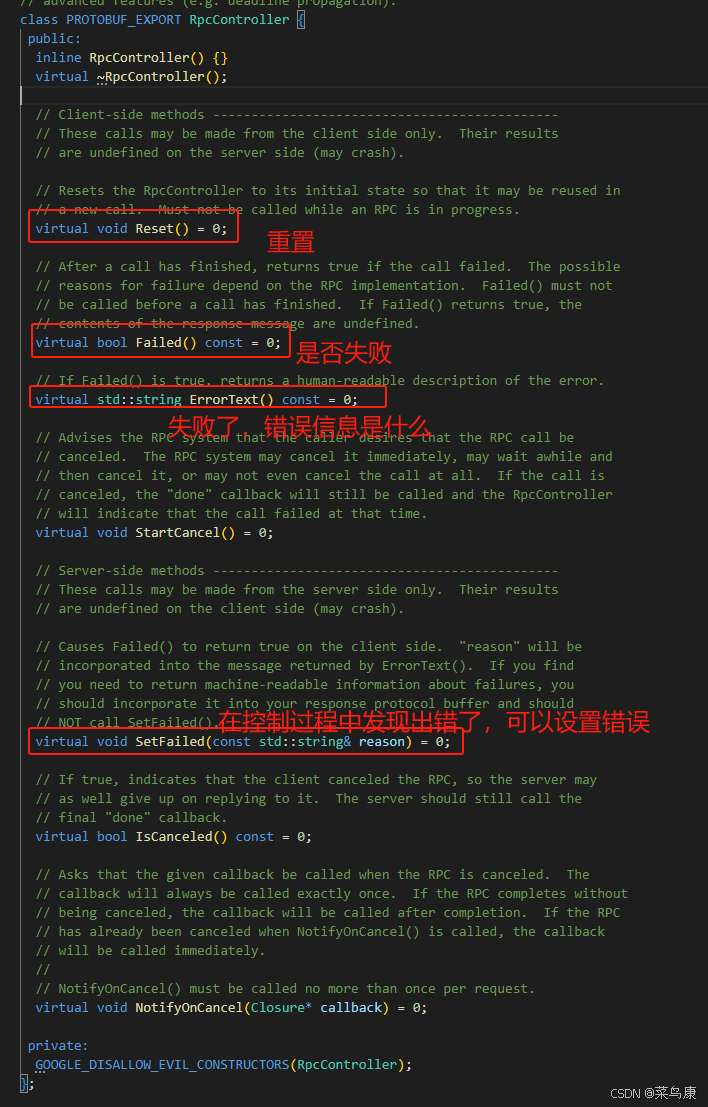
C++实现分布式网络通信框架RPC(3)--rpc调用端
目录 一、前言 二、UserServiceRpc_Stub 三、 CallMethod方法的重写 头文件 实现 四、rpc调用端的调用 实现 五、 google::protobuf::RpcController *controller 头文件 实现 六、总结 一、前言 在前边的文章中,我们已经大致实现了rpc服务端的各项功能代…...
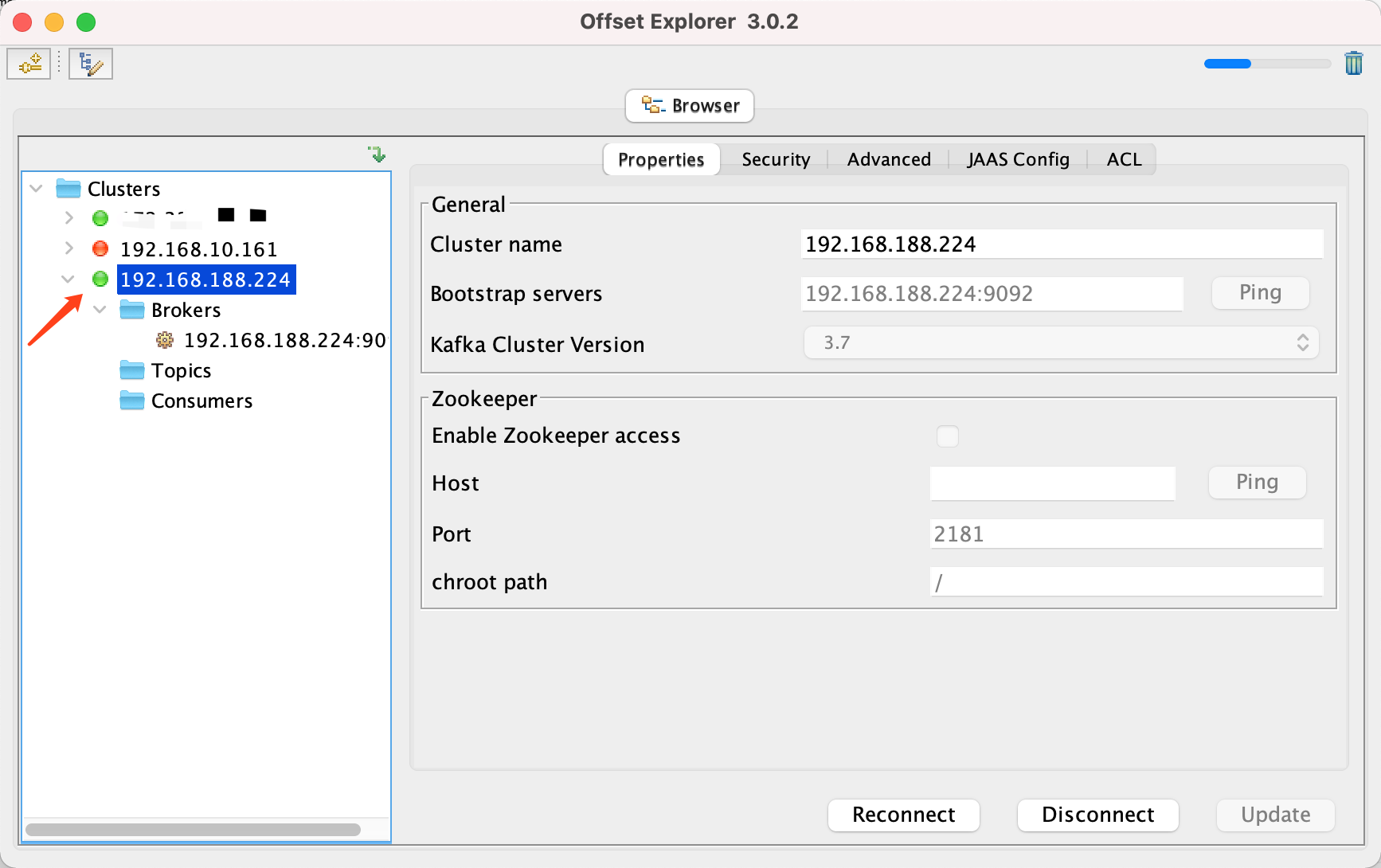
Docker 运行 Kafka 带 SASL 认证教程
Docker 运行 Kafka 带 SASL 认证教程 Docker 运行 Kafka 带 SASL 认证教程一、说明二、环境准备三、编写 Docker Compose 和 jaas文件docker-compose.yml代码说明:server_jaas.conf 四、启动服务五、验证服务六、连接kafka服务七、总结 Docker 运行 Kafka 带 SASL 认…...
oracle与MySQL数据库之间数据同步的技术要点
Oracle与MySQL数据库之间的数据同步是一个涉及多个技术要点的复杂任务。由于Oracle和MySQL的架构差异,它们的数据同步要求既要保持数据的准确性和一致性,又要处理好性能问题。以下是一些主要的技术要点: 数据结构差异 数据类型差异ÿ…...
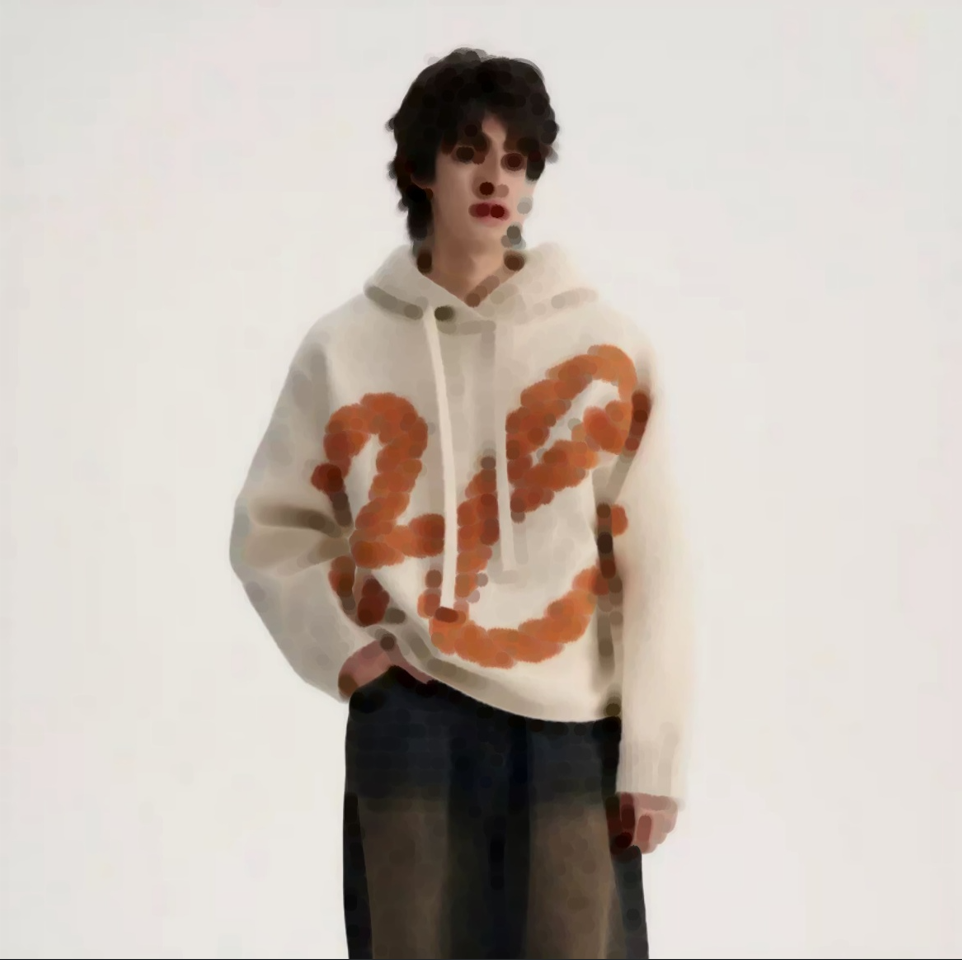
OPENCV形态学基础之二腐蚀
一.腐蚀的原理 (图1) 数学表达式:dst(x,y) erode(src(x,y)) min(x,y)src(xx,yy) 腐蚀也是图像形态学的基本功能之一,腐蚀跟膨胀属于反向操作,膨胀是把图像图像变大,而腐蚀就是把图像变小。腐蚀后的图像变小变暗淡。 腐蚀…...
#Uniapp篇:chrome调试unapp适配
chrome调试设备----使用Android模拟机开发调试移动端页面 Chrome://inspect/#devices MuMu模拟器Edge浏览器:Android原生APP嵌入的H5页面元素定位 chrome://inspect/#devices uniapp单位适配 根路径下 postcss.config.js 需要装这些插件 “postcss”: “^8.5.…...
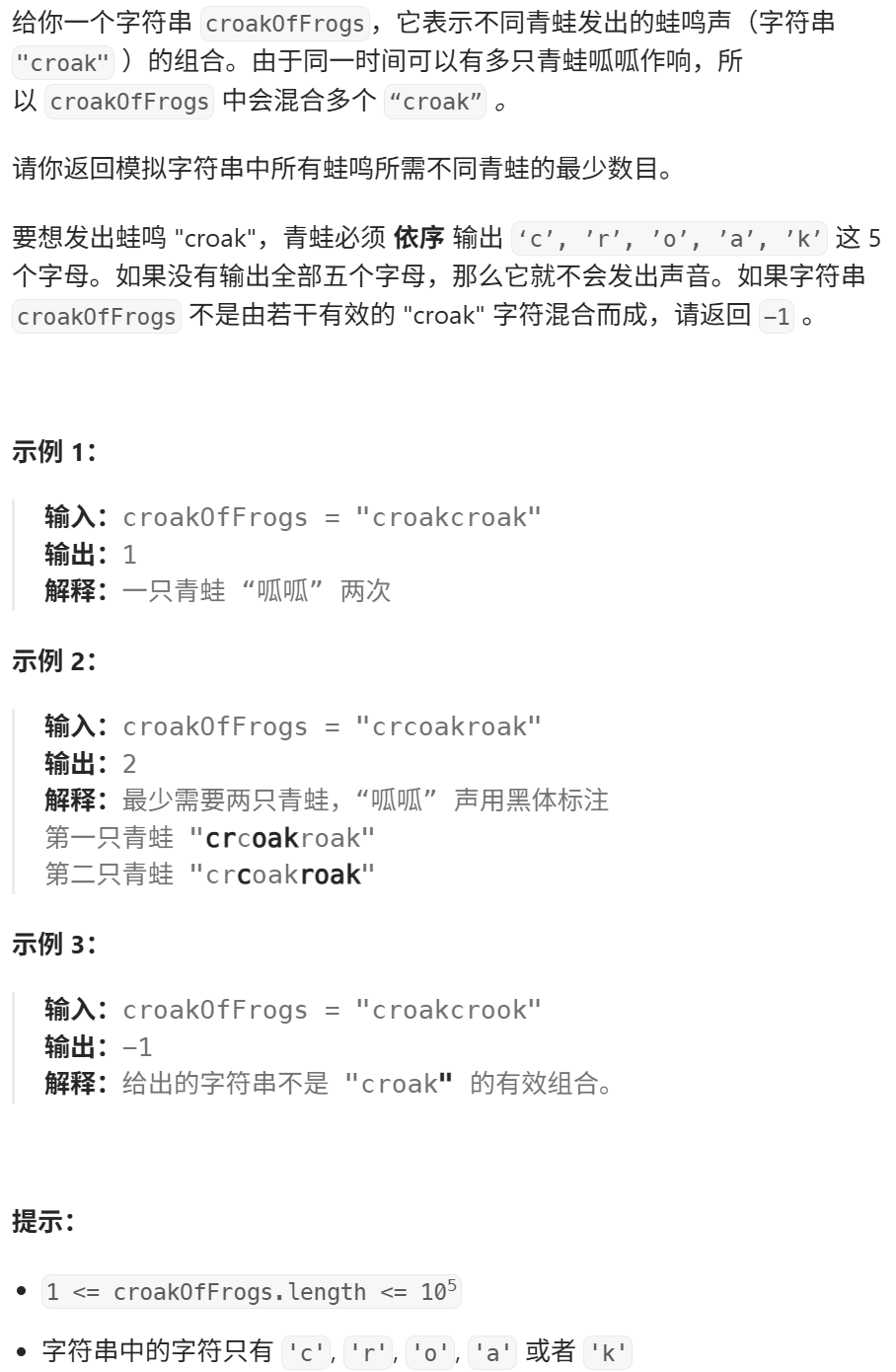
算法:模拟
1.替换所有的问号 1576. 替换所有的问号 - 力扣(LeetCode) 遍历字符串:通过外层循环逐一检查每个字符。遇到 ? 时处理: 内层循环遍历小写字母(a 到 z)。对每个字母检查是否满足: 与…...
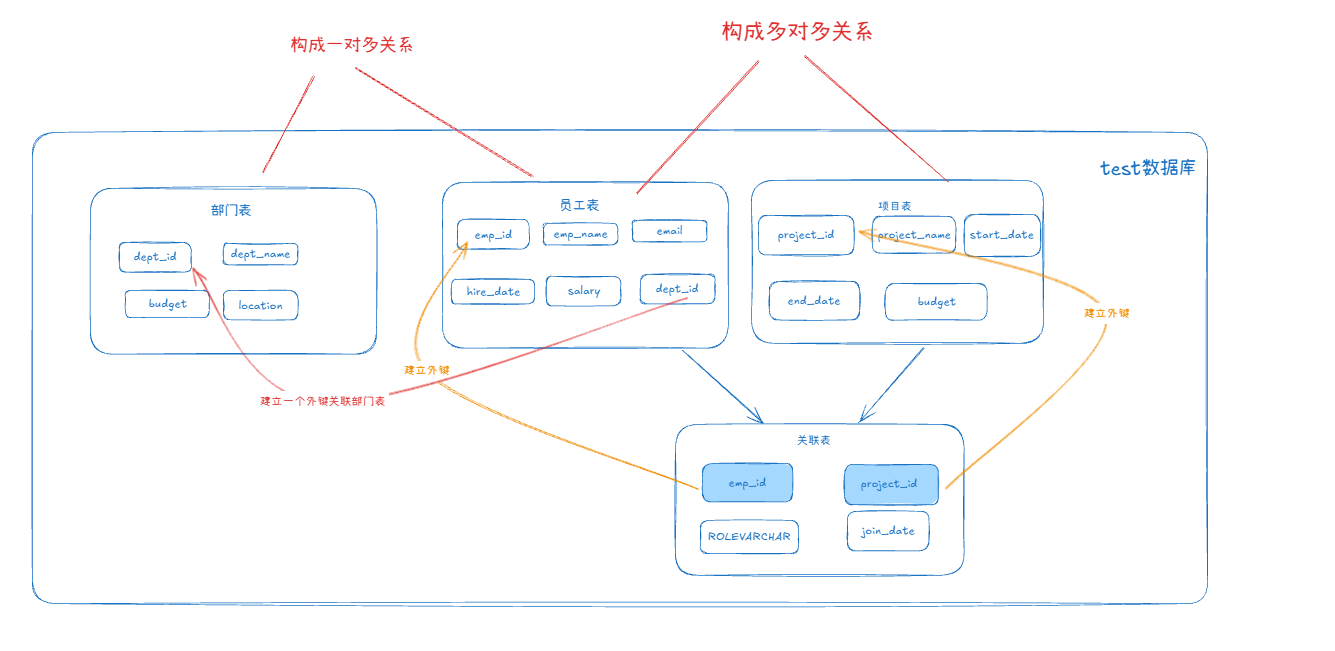
MySQL 知识小结(一)
一、my.cnf配置详解 我们知道安装MySQL有两种方式来安装咱们的MySQL数据库,分别是二进制安装编译数据库或者使用三方yum来进行安装,第三方yum的安装相对于二进制压缩包的安装更快捷,但是文件存放起来数据比较冗余,用二进制能够更好管理咱们M…...
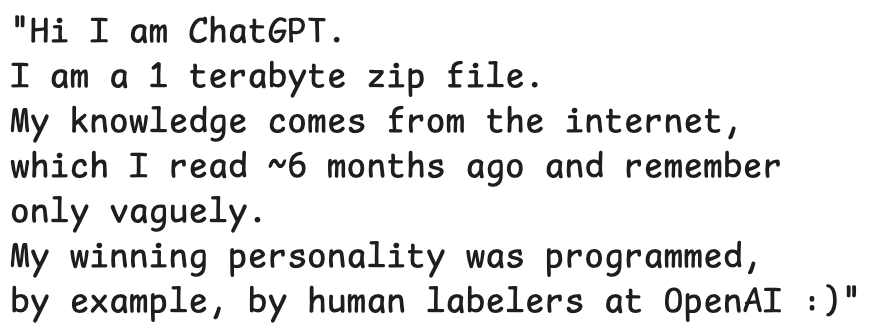
LLMs 系列实操科普(1)
写在前面: 本期内容我们继续 Andrej Karpathy 的《How I use LLMs》讲座内容,原视频时长 ~130 分钟,以实操演示主流的一些 LLMs 的使用,由于涉及到实操,实际上并不适合以文字整理,但还是决定尽量整理一份笔…...
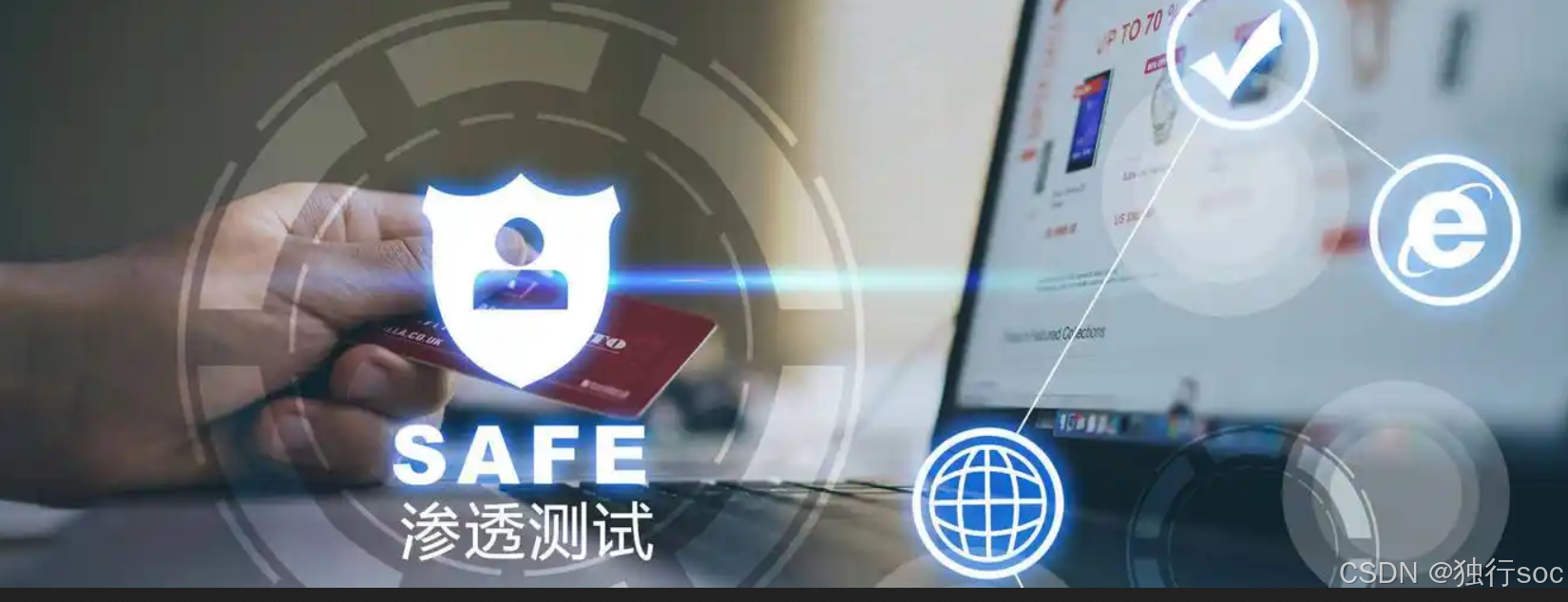
2025年渗透测试面试题总结-腾讯[实习]科恩实验室-安全工程师(题目+回答)
安全领域各种资源,学习文档,以及工具分享、前沿信息分享、POC、EXP分享。不定期分享各种好玩的项目及好用的工具,欢迎关注。 目录 腾讯[实习]科恩实验室-安全工程师 一、网络与协议 1. TCP三次握手 2. SYN扫描原理 3. HTTPS证书机制 二…...
从面试角度回答Android中ContentProvider启动原理
Android中ContentProvider原理的面试角度解析,分为已启动和未启动两种场景: 一、ContentProvider已启动的情况 1. 核心流程 触发条件:当其他组件(如Activity、Service)通过ContentR…...