ESIM实战文本匹配
引言
今天我们来实现ESIM文本匹配,这是一个典型的交互型文本匹配方式,也是近期第一个测试集准确率超过80%的模型。
我们来看下是如何实现的。
模型架构
我们主要实现左边的ESIM网络。
从下往上看,分别是
- 输入编码层(Input Ecoding)
对前提和假设进行编码
把语句中的单词转换为词向量,得到一个向量序列
把两句话的向量序列分别送入各自的Bi-LSTM网络进行语义特征抽取 - 局部推理建模层(Local Inference Modeling)
就是注意力层
通过注意力层捕获LSTM输出向量间的局部特征
然后通过元素级方法构造了一些特征 - 推理组合层(Inference Composition)
和输入编码层一样,也是Bi-LSTM
在捕获了文本间的注意力特征后,进一步做的融合/提取语义特征工作 - 预测层(Prediction)
拼接平均池化和最大池化后得到的向量
接Softmax进行分类
模型实现
class ESIM(nn.Module):def __init__(self,vocab_size: int,embedding_size: int,hidden_size: int,num_classes: int,lstm_dropout: float = 0.1,dropout: float = 0.5,) -> None:"""_summary_Args:vocab_size (int): the size of the Vocabularyembedding_size (int): the size of each embedding vectorhidden_size (int): the size of the hidden layernum_classes (int): the output sizelstm_dropout (float, optional): dropout ratio in lstm layer. Defaults to 0.1.dropout (float, optional): dropout ratio in linear layer. Defaults to 0.5."""super().__init__()self.embedding = nn.Embedding(vocab_size, embedding_size)# lstm for input embeddingself.lstm_a = nn.LSTM(hidden_size,hidden_size,batch_first=True,bidirectional=True,dropout=lstm_dropout,)self.lstm_b = nn.LSTM(hidden_size,hidden_size,batch_first=True,bidirectional=True,dropout=lstm_dropout,)
首先有一个嵌入层,然后对于输入的两个句子分别有一个Bi-LSTM。
然后定义推理组合层:
# lstm for augment inference vectorself.lstm_v_a = nn.LSTM(8 * hidden_size,hidden_size,batch_first=True,bidirectional=True,dropout=lstm_dropout,)self.lstm_v_b = nn.LSTM(8 * hidden_size,hidden_size,batch_first=True,bidirectional=True,dropout=lstm_dropout,)
完了就是最后的预测层,这里是一个多层前馈网络:
self.predict = nn.Sequential(nn.Linear(8 * hidden_size, 2 * hidden_size),nn.ReLU(),nn.Dropout(dropout),nn.Linear(2 * hidden_size, hidden_size),nn.ReLU(),nn.Dropout(dropout),nn.Linear(hidden_size, num_classes),)
初始化函数的完整实现为:
class ESIM(nn.Module):def __init__(self,vocab_size: int,embedding_size: int,hidden_size: int,num_classes: int,lstm_dropout: float = 0.1,dropout: float = 0.5,) -> None:"""_summary_Args:vocab_size (int): the size of the Vocabularyembedding_size (int): the size of each embedding vectorhidden_size (int): the size of the hidden layernum_classes (int): the output sizelstm_dropout (float, optional): dropout ratio in lstm layer. Defaults to 0.1.dropout (float, optional): dropout ratio in linear layer. Defaults to 0.5."""super().__init__()self.embedding = nn.Embedding(vocab_size, embedding_size)# lstm for input embeddingself.lstm_a = nn.LSTM(hidden_size,hidden_size,batch_first=True,bidirectional=True,dropout=lstm_dropout,)self.lstm_b = nn.LSTM(hidden_size,hidden_size,batch_first=True,bidirectional=True,dropout=lstm_dropout,)# lstm for augment inference vectorself.lstm_v_a = nn.LSTM(8 * hidden_size,hidden_size,batch_first=True,bidirectional=True,dropout=lstm_dropout,)self.lstm_v_b = nn.LSTM(8 * hidden_size,hidden_size,batch_first=True,bidirectional=True,dropout=lstm_dropout,)self.predict = nn.Sequential(nn.Linear(8 * hidden_size, 2 * hidden_size),nn.ReLU(),nn.Dropout(dropout),nn.Linear(2 * hidden_size, hidden_size),nn.ReLU(),nn.Dropout(dropout),nn.Linear(hidden_size, num_classes),)
使用ReLU激活函数,在激活函数后都有一个Dropout。
重点是forward方法:
def forward(self, a: torch.Tensor, b: torch.Tensor) -> torch.Tensor:"""Args:a (torch.Tensor): input sequence a with shape (batch_size, a_seq_len)b (torch.Tensor): input sequence b with shape (batch_size, b_seq_len)Returns:torch.Tensor:"""# a (batch_size, a_seq_len, embedding_size)a_embed = self.embedding(a)# b (batch_size, b_seq_len, embedding_size)b_embed = self.embedding(b)# a_bar (batch_size, a_seq_len, 2 * hidden_size)a_bar, _ = self.lstm_a(a_embed)# b_bar (batch_size, b_seq_len, 2 * hidden_size)b_bar, _ = self.lstm_b(b_embed)# score (batch_size, a_seq_len, b_seq_len)score = torch.matmul(a_bar, b_bar.permute(0, 2, 1))# softmax (batch_size, a_seq_len, b_seq_len) x (batch_size, b_seq_len, 2 * hidden_size)# a_tilde (batch_size, a_seq_len, 2 * hidden_size)a_tilde = torch.matmul(torch.softmax(score, dim=2), b_bar)# permute (batch_size, b_seq_len, a_seq_len) x (batch_size, a_seq_len, 2 * hidden_size)# b_tilde (batch_size, b_seq_len, 2 * hidden_size)b_tilde = torch.matmul(torch.softmax(score, dim=1).permute(0, 2, 1), a_bar)# m_a (batch_size, a_seq_len, 8 * hidden_size)m_a = torch.cat([a_bar, a_tilde, a_bar - a_tilde, a_bar * a_tilde], dim=-1)# m_b (batch_size, b_seq_len, 8 * hidden_size)m_b = torch.cat([b_bar, b_tilde, b_bar - b_tilde, b_bar * b_tilde], dim=-1)# v_a (batch_size, a_seq_len, 2 * hidden_size)v_a, _ = self.lstm_v_a(m_a)# v_b (batch_size, b_seq_len, 2 * hidden_size)v_b, _ = self.lstm_v_b(m_b)# (batch_size, 2 * hidden_size)avg_a = torch.mean(v_a, dim=1)avg_b = torch.mean(v_b, dim=1)max_a, _ = torch.max(v_a, dim=1)max_b, _ = torch.max(v_b, dim=1)# (batch_size, 8 * hidden_size)v = torch.cat([avg_a, max_a, avg_b, max_b], dim=-1)return self.predict(v)
标注出每个向量的维度就挺好理解的。
首先分别为两个输入得到嵌入向量;
其次喂给各自的LSTM层,得到每个时间步的输出,注意由于是双向LSTM,因此最后的维度是2 * hidden_size
;
接着对它两计算注意力分数score (batch_size, a_seq_len, b_seq_len)
;
然后分别计算a对b和b对a的注意力,这里要注意softmax
中dim
的维度。在计算a的注意力输出时,是利用b每个输入的加权和,所以softmax(score, dim=2)
在b_seq_len
维度上间求和;
然后是增强的局部推理,差、乘各种拼接,得到一个8 * hidden_size
的维度,所以lstm_v_a
的输入维度是8 * hidden_size
;
接下来就是对得到的a和b进行平均池化和最大池化,然后把它们拼接起来,也得到了8 * hidden_size
的一个维度;
最后经过我们的预测层,多层前馈网络,进行了一些非线性变换后变成了输出维度大小,比较最后一个维度的值哪个大当成对哪个类的一个预测;
数据准备
和前面几篇几乎一样,这里直接贴代码:
from collections import defaultdict
from tqdm import tqdm
import numpy as np
import json
from torch.utils.data import Dataset
import pandas as pd
from typing import TupleUNK_TOKEN = "<UNK>"
PAD_TOKEN = "<PAD>"class Vocabulary:"""Class to process text and extract vocabulary for mapping"""def __init__(self, token_to_idx: dict = None, tokens: list[str] = None) -> None:"""Args:token_to_idx (dict, optional): a pre-existing map of tokens to indices. Defaults to None.tokens (list[str], optional): a list of unique tokens with no duplicates. Defaults to None."""assert any([tokens, token_to_idx]), "At least one of these parameters should be set as not None."if token_to_idx:self._token_to_idx = token_to_idxelse:self._token_to_idx = {}if PAD_TOKEN not in tokens:tokens = [PAD_TOKEN] + tokensfor idx, token in enumerate(tokens):self._token_to_idx[token] = idxself._idx_to_token = {idx: token for token, idx in self._token_to_idx.items()}self.unk_index = self._token_to_idx[UNK_TOKEN]self.pad_index = self._token_to_idx[PAD_TOKEN]@classmethoddef build(cls,sentences: list[list[str]],min_freq: int = 2,reserved_tokens: list[str] = None,) -> "Vocabulary":"""Construct the Vocabulary from sentencesArgs:sentences (list[list[str]]): a list of tokenized sequencesmin_freq (int, optional): the minimum word frequency to be saved. Defaults to 2.reserved_tokens (list[str], optional): the reserved tokens to add into the Vocabulary. Defaults to None.Returns:Vocabulary: a Vocubulary instane"""token_freqs = defaultdict(int)for sentence in tqdm(sentences):for token in sentence:token_freqs[token] += 1unique_tokens = (reserved_tokens if reserved_tokens else []) + [UNK_TOKEN]unique_tokens += [tokenfor token, freq in token_freqs.items()if freq >= min_freq and token != UNK_TOKEN]return cls(tokens=unique_tokens)def __len__(self) -> int:return len(self._idx_to_token)def __getitem__(self, tokens: list[str] | str) -> list[int] | int:"""Retrieve the indices associated with the tokens or the index with the single tokenArgs:tokens (list[str] | str): a list of tokens or single tokenReturns:list[int] | int: the indices or the single index"""if not isinstance(tokens, (list, tuple)):return self._token_to_idx.get(tokens, self.unk_index)return [self.__getitem__(token) for token in tokens]def lookup_token(self, indices: list[int] | int) -> list[str] | str:"""Retrive the tokens associated with the indices or the token with the single indexArgs:indices (list[int] | int): a list of index or single indexReturns:list[str] | str: the corresponding tokens (or token)"""if not isinstance(indices, (list, tuple)):return self._idx_to_token[indices]return [self._idx_to_token[index] for index in indices]def to_serializable(self) -> dict:"""Returns a dictionary that can be serialized"""return {"token_to_idx": self._token_to_idx}@classmethoddef from_serializable(cls, contents: dict) -> "Vocabulary":"""Instantiates the Vocabulary from a serialized dictionaryArgs:contents (dict): a dictionary generated by `to_serializable`Returns:Vocabulary: the Vocabulary instance"""return cls(**contents)def __repr__(self):return f"<Vocabulary(size={len(self)})>"class TMVectorizer:"""The Vectorizer which vectorizes the Vocabulary"""def __init__(self, vocab: Vocabulary, max_len: int) -> None:"""Args:vocab (Vocabulary): maps characters to integersmax_len (int): the max length of the sequence in the dataset"""self.vocab = vocabself.max_len = max_lendef _vectorize(self, indices: list[int], vector_length: int = -1, padding_index: int = 0) -> np.ndarray:"""Vectorize the provided indicesArgs:indices (list[int]): a list of integers that represent a sequencevector_length (int, optional): an arugment for forcing the length of index vector. Defaults to -1.padding_index (int, optional): the padding index to use. Defaults to 0.Returns:np.ndarray: the vectorized index array"""if vector_length <= 0:vector_length = len(indices)vector = np.zeros(vector_length, dtype=np.int64)if len(indices) > vector_length:vector[:] = indices[:vector_length]else:vector[: len(indices)] = indicesvector[len(indices) :] = padding_indexreturn vectordef _get_indices(self, sentence: list[str]) -> list[int]:"""Return the vectorized sentenceArgs:sentence (list[str]): list of tokensReturns:indices (list[int]): list of integers representing the sentence"""return [self.vocab[token] for token in sentence]def vectorize(self, sentence: list[str], use_dataset_max_length: bool = True) -> np.ndarray:"""Return the vectorized sequenceArgs:sentence (list[str]): raw sentence from the datasetuse_dataset_max_length (bool): whether to use the global max vector lengthReturns:the vectorized sequence with padding"""vector_length = -1if use_dataset_max_length:vector_length = self.max_lenindices = self._get_indices(sentence)vector = self._vectorize(indices, vector_length=vector_length, padding_index=self.vocab.pad_index)return vector@classmethoddef from_serializable(cls, contents: dict) -> "TMVectorizer":"""Instantiates the TMVectorizer from a serialized dictionaryArgs:contents (dict): a dictionary generated by `to_serializable`Returns:TMVectorizer:"""vocab = Vocabulary.from_serializable(contents["vocab"])max_len = contents["max_len"]return cls(vocab=vocab, max_len=max_len)def to_serializable(self) -> dict:"""Returns a dictionary that can be serializedReturns:dict: a dict contains Vocabulary instance and max_len attribute"""return {"vocab": self.vocab.to_serializable(), "max_len": self.max_len}def save_vectorizer(self, filepath: str) -> None:"""Dump this TMVectorizer instance to fileArgs:filepath (str): the path to store the file"""with open(filepath, "w") as f:json.dump(self.to_serializable(), f)@classmethoddef load_vectorizer(cls, filepath: str) -> "TMVectorizer":"""Load TMVectorizer from a fileArgs:filepath (str): the path stored the fileReturns:TMVectorizer:"""with open(filepath) as f:return TMVectorizer.from_serializable(json.load(f))class TMDataset(Dataset):"""Dataset for text matching"""def __init__(self, text_df: pd.DataFrame, vectorizer: TMVectorizer) -> None:"""Args:text_df (pd.DataFrame): a DataFrame which contains the processed data examplesvectorizer (TMVectorizer): a TMVectorizer instance"""self.text_df = text_dfself._vectorizer = vectorizerdef __getitem__(self, index: int) -> Tuple[np.ndarray, np.ndarray, int]:row = self.text_df.iloc[index]return (self._vectorizer.vectorize(row.sentence1),self._vectorizer.vectorize(row.sentence2),row.label,)def get_vectorizer(self) -> TMVectorizer:return self._vectorizerdef __len__(self) -> int:return len(self.text_df)
模型训练
定义辅助函数和指标:
def make_dirs(dirpath):if not os.path.exists(dirpath):os.makedirs(dirpath)def tokenize(sentence: str):return list(jieba.cut(sentence))def build_dataframe_from_csv(dataset_csv: str) -> pd.DataFrame:df = pd.read_csv(dataset_csv,sep="\t",header=None,names=["sentence1", "sentence2", "label"],)df.sentence1 = df.sentence1.apply(tokenize)df.sentence2 = df.sentence2.apply(tokenize)return dfdef metrics(y: torch.Tensor, y_pred: torch.Tensor) -> Tuple[float, float, float, float]:TP = ((y_pred == 1) & (y == 1)).sum().float() # True PositiveTN = ((y_pred == 0) & (y == 0)).sum().float() # True NegativeFN = ((y_pred == 0) & (y == 1)).sum().float() # False NegatvieFP = ((y_pred == 1) & (y == 0)).sum().float() # False Positivep = TP / (TP + FP).clamp(min=1e-8) # Precisionr = TP / (TP + FN).clamp(min=1e-8) # RecallF1 = 2 * r * p / (r + p).clamp(min=1e-8) # F1 scoreacc = (TP + TN) / (TP + TN + FP + FN).clamp(min=1e-8) # Accuraryreturn acc, p, r, F1
定义评估函数和训练函数:
def evaluate(data_iter: DataLoader, model: nn.Module
) -> Tuple[float, float, float, float]:y_list, y_pred_list = [], []model.eval()for x1, x2, y in tqdm(data_iter):x1 = x1.to(device).long()x2 = x2.to(device).long()y = torch.LongTensor(y).to(device)output = model(x1, x2)pred = torch.argmax(output, dim=1).long()y_pred_list.append(pred)y_list.append(y)y_pred = torch.cat(y_pred_list, 0)y = torch.cat(y_list, 0)acc, p, r, f1 = metrics(y, y_pred)return acc, p, r, f1def train(data_iter: DataLoader,model: nn.Module,criterion: nn.CrossEntropyLoss,optimizer: torch.optim.Optimizer,print_every: int = 500,verbose=True,
) -> None:model.train()for step, (x1, x2, y) in enumerate(tqdm(data_iter)):x1 = x1.to(device).long()x2 = x2.to(device).long()y = torch.LongTensor(y).to(device)output = model(x1, x2)loss = criterion(output, y)optimizer.zero_grad()loss.backward()optimizer.step()if verbose and (step + 1) % print_every == 0:pred = torch.argmax(output, dim=1).long()acc, p, r, f1 = metrics(y, pred)print(f" TRAIN iter={step+1} loss={loss.item():.6f} accuracy={acc:.3f} precision={p:.3f} recal={r:.3f} f1 score={f1:.4f}")
参数定义:
args = Namespace(dataset_csv="text_matching/data/lcqmc/{}.txt",vectorizer_file="vectorizer.json",model_state_file="model.pth",save_dir=f"{os.path.dirname(__file__)}/model_storage",reload_model=False,cuda=True,learning_rate=4e-4,batch_size=128,num_epochs=10,max_len=50,embedding_dim=300,hidden_size=300,num_classes=2,lstm_dropout=0.8,dropout=0.5,min_freq=2,print_every=500,verbose=True,)
这个模型非常简单,但效果是非常不错的,可以作为一个很好的baseline。在训练过程中发现对训练集的准确率达到了100%,有点过拟合了,因此最后把lstm的dropout加大到0.8,最后看一下训练效果如何:
make_dirs(args.save_dir)if args.cuda:device = torch.device("cuda:0" if torch.cuda.is_available() else "cpu")else:device = torch.device("cpu")print(f"Using device: {device}.")vectorizer_path = os.path.join(args.save_dir, args.vectorizer_file)train_df = build_dataframe_from_csv(args.dataset_csv.format("train"))test_df = build_dataframe_from_csv(args.dataset_csv.format("test"))dev_df = build_dataframe_from_csv(args.dataset_csv.format("dev"))if os.path.exists(vectorizer_path):print("Loading vectorizer file.")vectorizer = TMVectorizer.load_vectorizer(vectorizer_path)args.vocab_size = len(vectorizer.vocab)else:print("Creating a new Vectorizer.")train_sentences = train_df.sentence1.to_list() + train_df.sentence2.to_list()vocab = Vocabulary.build(train_sentences, args.min_freq)args.vocab_size = len(vocab)print(f"Builds vocabulary : {vocab}")vectorizer = TMVectorizer(vocab, args.max_len)vectorizer.save_vectorizer(vectorizer_path)train_dataset = TMDataset(train_df, vectorizer)test_dataset = TMDataset(test_df, vectorizer)dev_dataset = TMDataset(dev_df, vectorizer)train_data_loader = DataLoader(train_dataset, batch_size=args.batch_size, shuffle=True)dev_data_loader = DataLoader(dev_dataset, batch_size=args.batch_size)test_data_loader = DataLoader(test_dataset, batch_size=args.batch_size)print(f"Arguments : {args}")model = ESIM(args.vocab_size,args.embedding_dim,args.hidden_size,args.num_classes,args.lstm_dropout,args.dropout,)print(f"Model: {model}")model_saved_path = os.path.join(args.save_dir, args.model_state_file)if args.reload_model and os.path.exists(model_saved_path):model.load_state_dict(torch.load(args.model_saved_path))print("Reloaded model")else:print("New model")model = model.to(device)optimizer = torch.optim.Adam(model.parameters(), lr=args.learning_rate)criterion = nn.CrossEntropyLoss()for epoch in range(args.num_epochs):train(train_data_loader,model,criterion,optimizer,print_every=args.print_every,verbose=args.verbose,)print("Begin evalute on dev set.")with torch.no_grad():acc, p, r, f1 = evaluate(dev_data_loader, model)print(f"EVALUATE [{epoch+1}/{args.num_epochs}] accuracy={acc:.3f} precision={p:.3f} recal={r:.3f} f1 score={f1:.4f}")model.eval()acc, p, r, f1 = evaluate(test_data_loader, model)print(f"TEST accuracy={acc:.3f} precision={p:.3f} recal={r:.3f} f1 score={f1:.4f}")
python .\text_matching\esim\train.py
Using device: cuda:0.
Building prefix dict from the default dictionary ...
Loading model from cache C:\Users\ADMINI~1\AppData\Local\Temp\jieba.cache
Loading model cost 0.563 seconds.
Prefix dict has been built successfully.
Loading vectorizer file.
Arguments : Namespace(dataset_csv='text_matching/data/lcqmc/{}.txt', vectorizer_file='vectorizer.json', model_state_file='model.pth', save_dir='D:\\workspace\\nlp-in-action\\text_matching\\esim/model_storage', reload_model=False, cuda=True, learning_rate=0.0004, batch_size=128, num_epochs=10, max_len=50, embedding_dim=300, hidden_size=300, num_classes=2, lstm_dropout=0.8, dropout=0.5, min_freq=2, print_every=500, verbose=True, vocab_size=35925)
D:\workspace\nlp-in-action\.venv\lib\site-packages\torch\nn\modules\rnn.py:67: UserWarning: dropout option adds dropout after all but last recurrent layer, so non-zero dropout expects num_layers greater than 1, but got dropout=0.8 and num_layers=1warnings.warn("dropout option adds dropout after all but last "
Model: ESIM((embedding): Embedding(35925, 300)(lstm_a): LSTM(300, 300, batch_first=True, dropout=0.8, bidirectional=True)(lstm_b): LSTM(300, 300, batch_first=True, dropout=0.8, bidirectional=True)(lstm_v_a): LSTM(2400, 300, batch_first=True, dropout=0.8, bidirectional=True)(lstm_v_b): LSTM(2400, 300, batch_first=True, dropout=0.8, bidirectional=True)(predict): Sequential((0): Linear(in_features=2400, out_features=600, bias=True)(1): ReLU()(2): Dropout(p=0.5, inplace=False)(3): Linear(in_features=600, out_features=300, bias=True)(4): ReLU()(5): Dropout(p=0.5, inplace=False)(6): Linear(in_features=300, out_features=2, bias=True))
)
New model27%|█████████████████████████████████████████████████▋ | 499/1866 [01:33<04:13, 5.40it/s]
TRAIN iter=500 loss=0.500601 accuracy=0.750 precision=0.714 recal=0.846 f1 score=0.774654%|███████████████████████████████████████████████████████████████████████████████████████████████████▌ | 999/1866 [03:04<02:40, 5.41it/s]
TRAIN iter=1000 loss=0.250350 accuracy=0.883 precision=0.907 recal=0.895 f1 score=0.900780%|████████████████████████████████████████████████████████████████████████████████████████████████████████████████████████████████████████████████████▌ | 1499/1866 [04:35<01:07, 5.43it/s]
TRAIN iter=1500 loss=0.311494 accuracy=0.844 precision=0.868 recal=0.868 f1 score=0.8684
100%|█████████████████████████████████████████████████████████████████████████████████████████████████████████████████████████████████████████████████████████████████████████████████████████| 1866/1866 [05:42<00:00, 5.45it/s]
Begin evalute on dev set.
100%|█████████████████████████████████████████████████████████████████████████████████████████████████████████████████████████████████████████████████████████████████████████████████████████████| 69/69 [00:04<00:00, 17.10it/s]
EVALUATE [1/10] accuracy=0.771 precision=0.788 recal=0.741 f1 score=0.7637
...
TRAIN iter=500 loss=0.005086 accuracy=1.000 precision=1.000 recal=1.000 f1 score=1.0000
...
EVALUATE [10/10] accuracy=0.815 precision=0.807 recal=0.829 f1 score=0.8178
100%|█████████████████████████████████████████████████████████████████████████████████████████████████████████████████████████████████████████████████████████████████████████████████████████████| 98/98 [00:05<00:00, 17.38it/s]
TEST accuracy=0.817 precision=0.771 recal=0.903 f1 score=0.8318
可以看到,训练集上的损失第一次降到了0.005
级别,准确率甚至达到了100%,但最终在测试集上的准确率相比训练集还是逊色了一些,只有81.7%,不过相比前面几个模型已经很不错了。
相关文章:
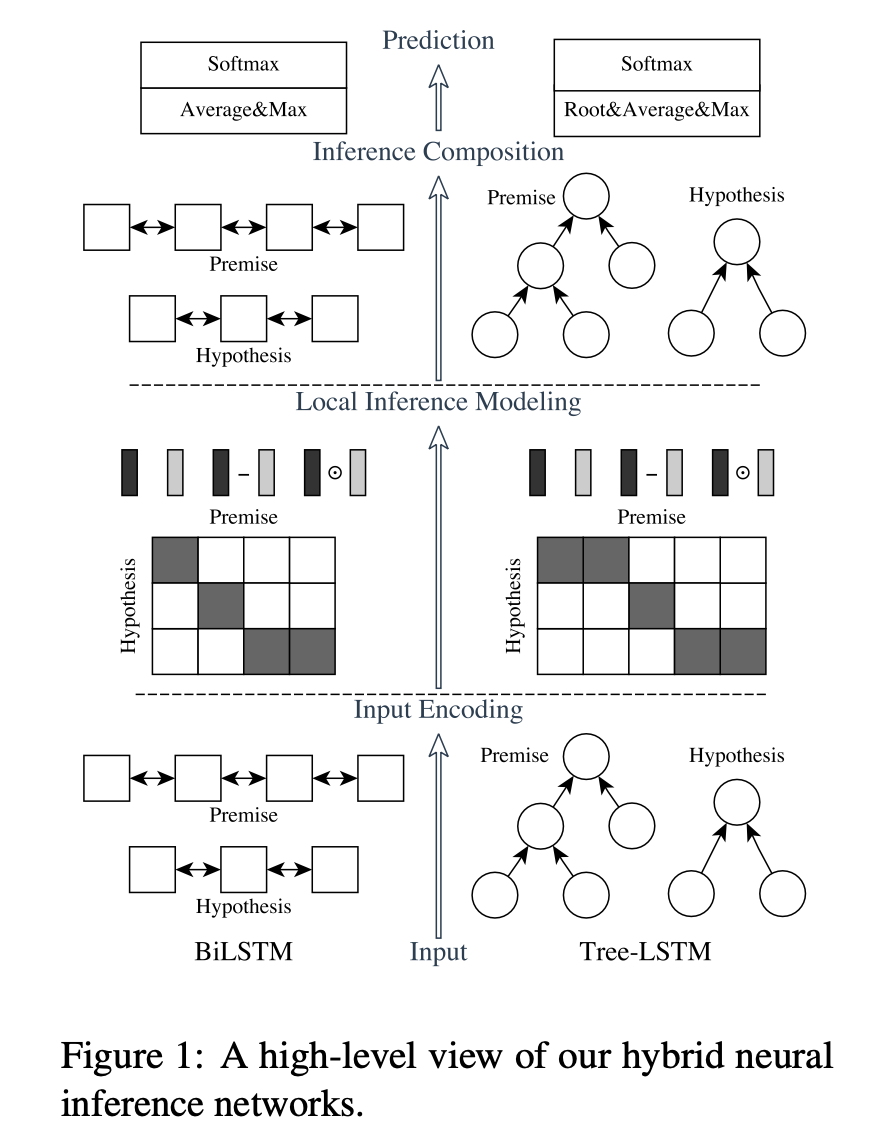
ESIM实战文本匹配
引言 今天我们来实现ESIM文本匹配,这是一个典型的交互型文本匹配方式,也是近期第一个测试集准确率超过80%的模型。 我们来看下是如何实现的。 模型架构 我们主要实现左边的ESIM网络。 从下往上看,分别是 输入编码层(Input Ecoding) 对前…...
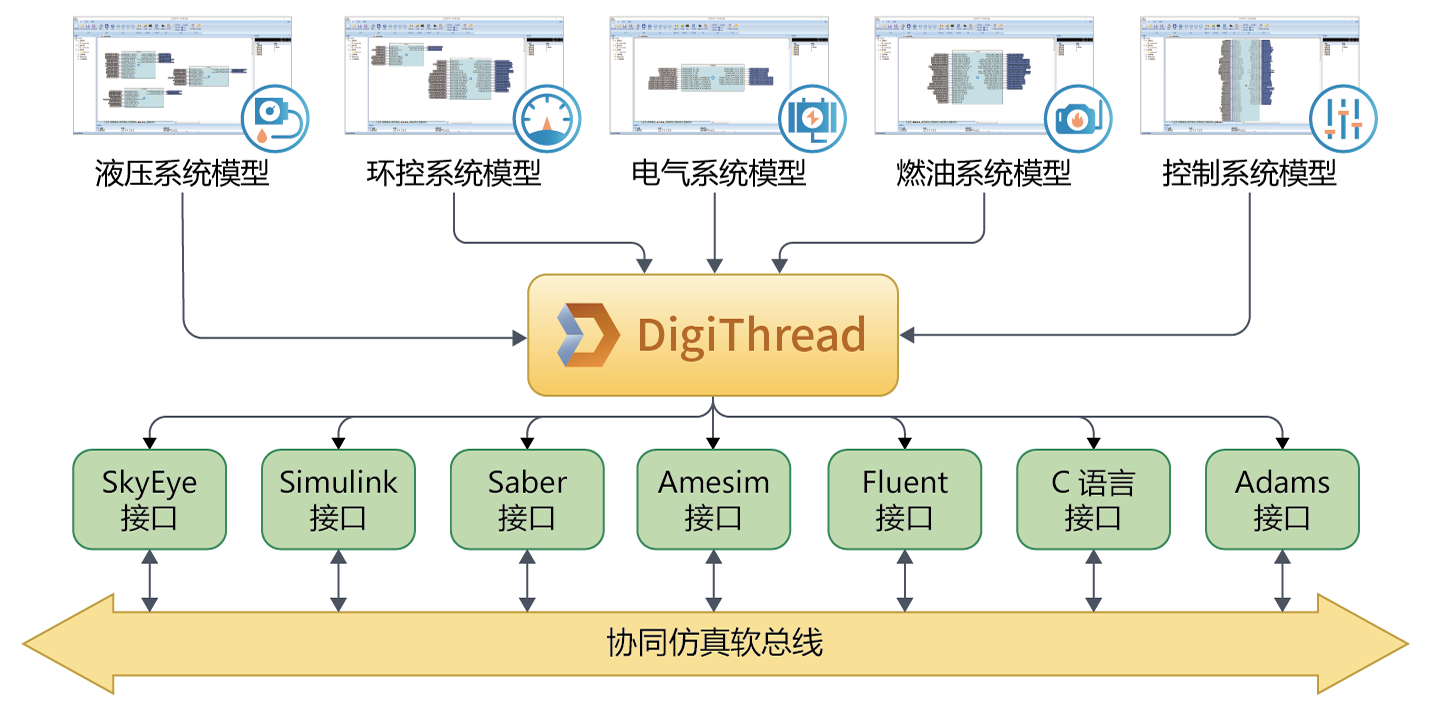
基于虚拟仿真技术的汽车燃油泵控制
在当前激烈的竞争环境下,汽车行业正在加速产业和技术更迭,整车厂对大型ECU嵌入式控制系统和软件的需求迫在眉睫。 然而,复杂而庞大的汽车系统往往由多个物理系统组成,系统所对应的模型都需要在不同的领域实现:发动机、…...

angular:HtmlElement的子节点有Shadow dom时奇怪的现象
描述: 这样写时,会自动跳过shadow dom节点的遍历 const cloneElement this.contentElement.cloneNode(true) as HTMLElement; for(let childNodeIndex 0; childNodeIndex < cloneElement.childNodes.length; childNodeIndex) {element.appendChild…...

栈与队列--删除字符串中的所有相邻重复项
给出由小写字母组成的字符串 S,重复项删除操作会选择两个相邻且相同的字母,并删除它们。 在 S 上反复执行重复项删除操作,直到无法继续删除。 在完成所有重复项删除操作后返回最终的字符串。答案保证唯一。 示例: 输入&#x…...
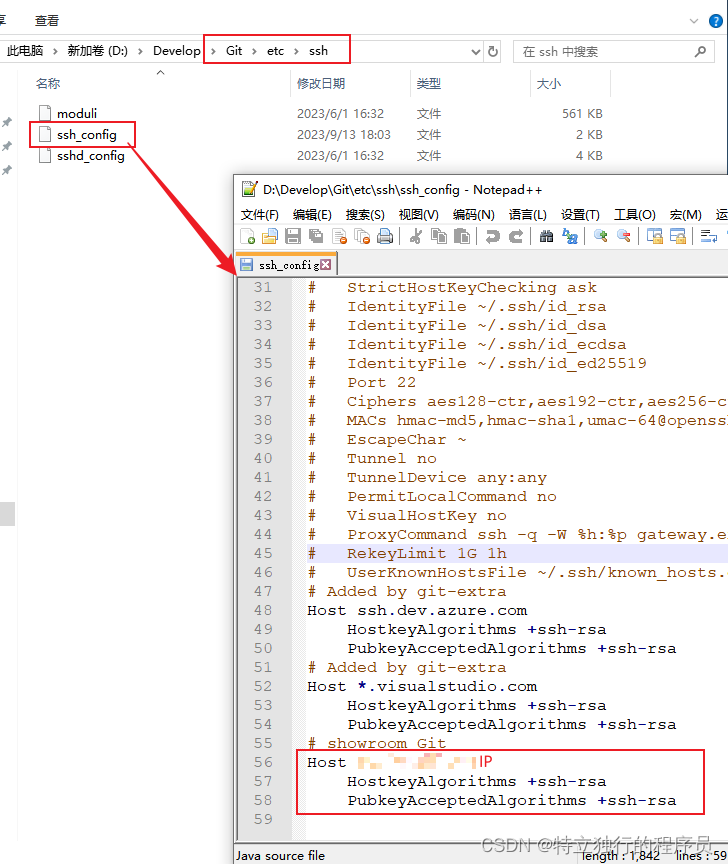
使用SSH地址拉取远程仓库代码报下面的错误
说明:配置了SSH秘钥后,使用SSH地址克隆代码,依旧无法拉取代码,提示下面这个信息。 Their offer:ssh-rsa,ssh-dss fatal:Could not read from remote repository. Please make sure you have the…...
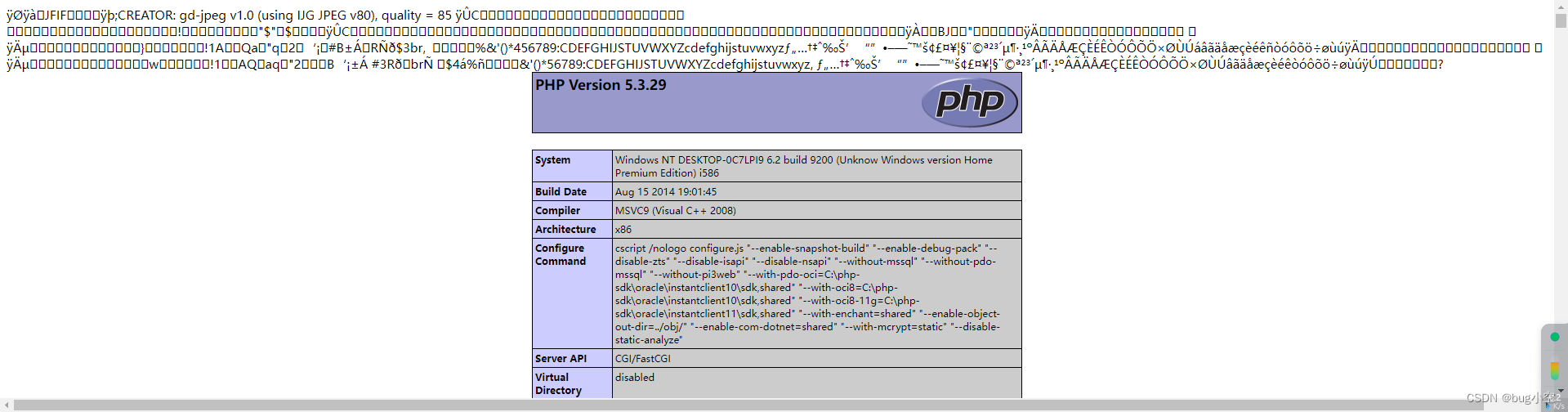
easycms v5.5 分析 | Bugku S3 AWD排位赛
前言 这个awd打的悲,后台默认用户名密码为admin:admin,但是几乎所有人都改了 而且一进去看到这个cms就有点懵逼,都不知道这个cms是干嘛的(没用过相似的cms) 虽然网上找出了很多相关的漏洞,但是不知道为什…...
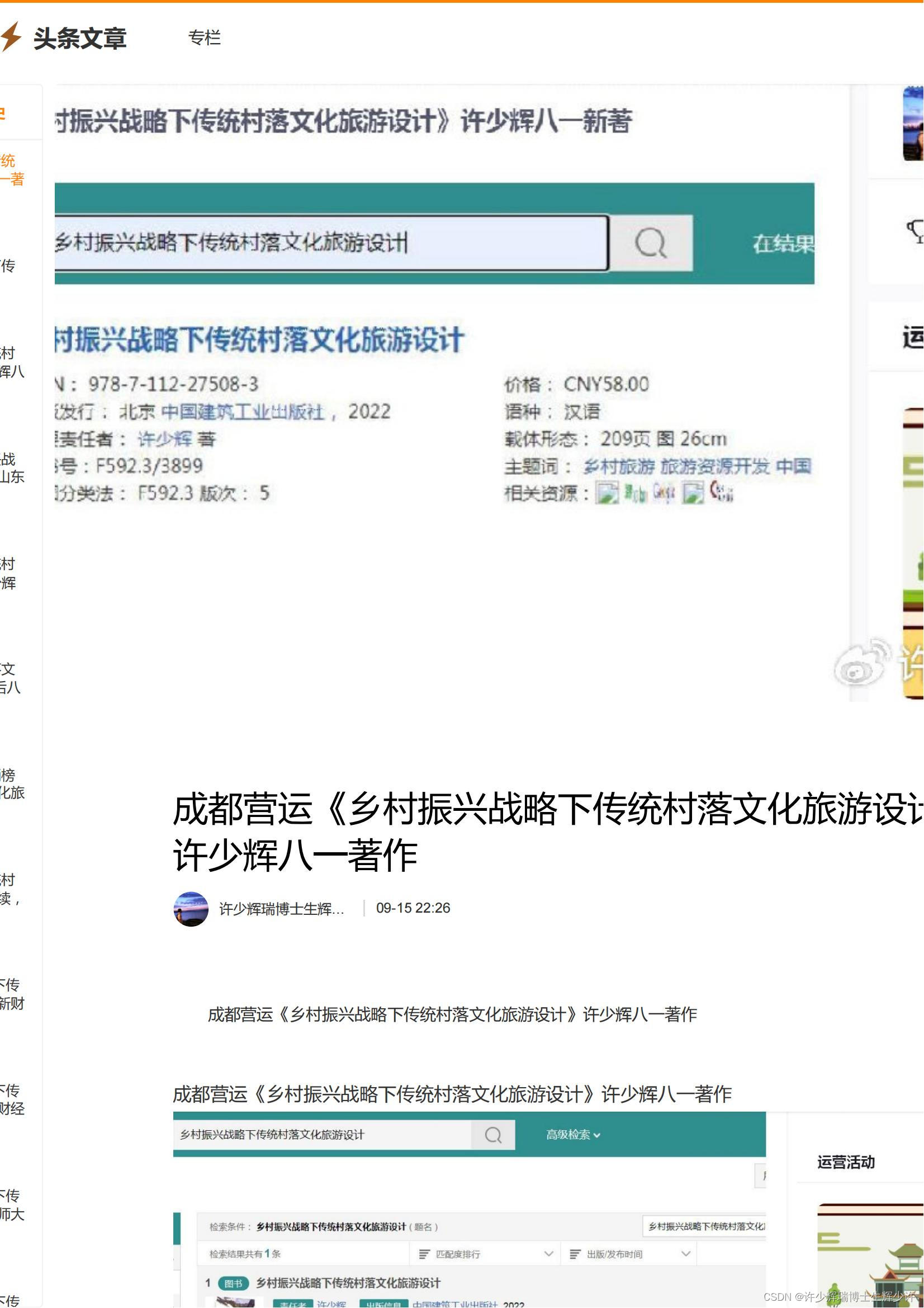
成都营运《乡村振兴战略下传统村落文化旅游设计》许少辉八一著作
成都营运《乡村振兴战略下传统村落文化旅游设计》许少辉八一著作...
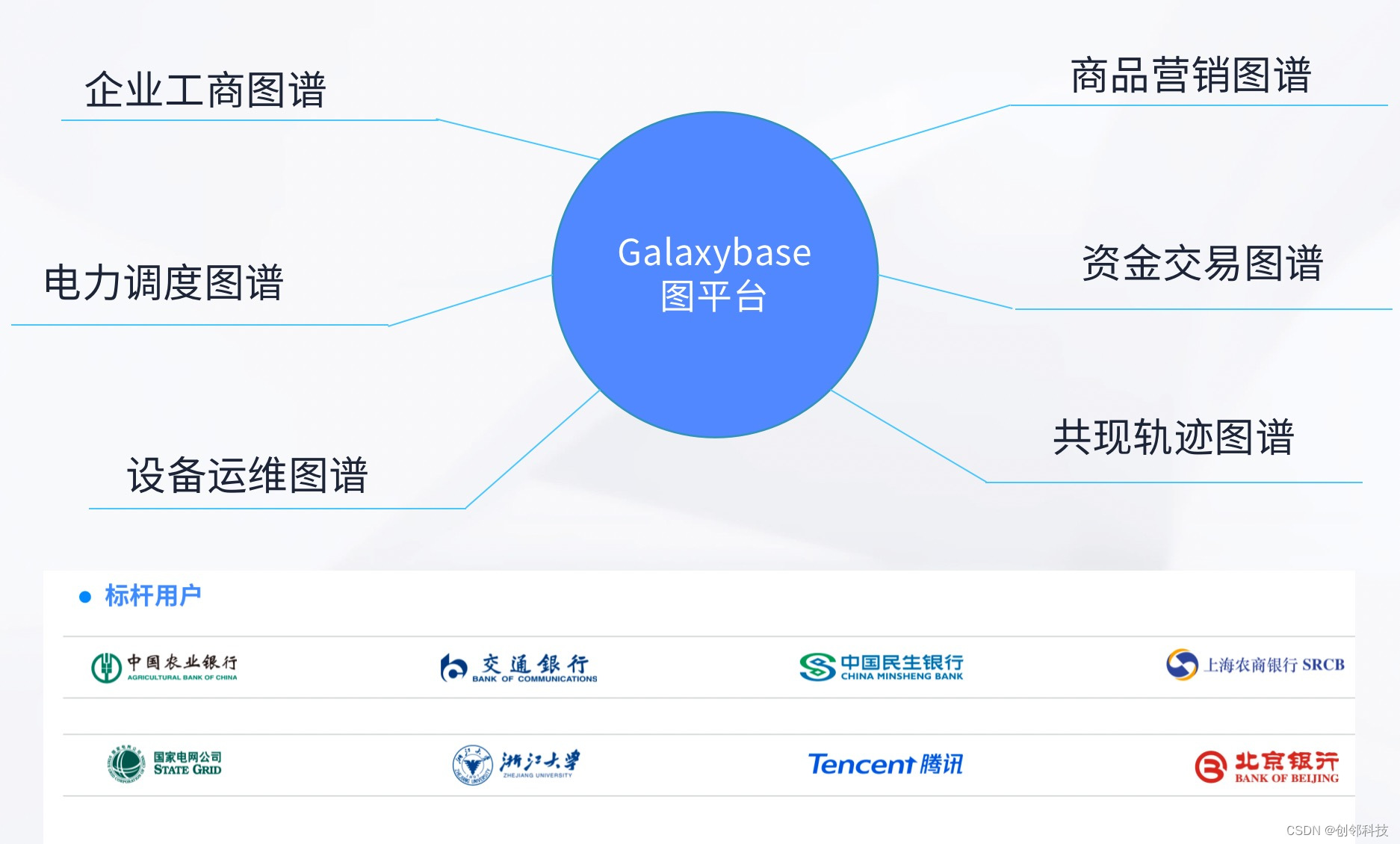
创邻科技Galaxybase助力SPG推动知识图谱应用落地
1. 知识图谱实践应用:从理论到落地的全景视角 知识图谱,作为一种先进的数据模型和信息表示策略,极大地提升了信息检索与分析的能力。该模型利用图结构,将不同领域、层次和类别的信息有机整合,令复杂的数据关系变得清晰…...

《TCP/IP网络编程》阅读笔记--域名及网络地址
目录 1--域名系统 2--域名与 IP 地址的转换 2-1--利用域名来获取 IP 地址 2-2--利用 IP 地址获取域名 3--代码实例 3-1--gethostbyname() 3-2--gethostbyaddr() 1--域名系统 域名系统(Domain Name System,DNS)是对 IP 地址和域名进行相…...
我的C#基础
using System; namespace HelloWorldApplication }TOC 欢迎使用Markdown编辑器 你好! 这是你第一次使用 Markdown编辑器 所展示的欢迎页。 为帮助您在CSDN创作的文章获得更多曝光和关注,我们为您提供了专属福利: 已注册且未在CSDN平台发布过…...
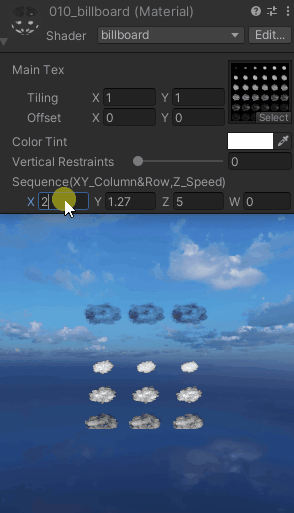
【UnityShaderLab实现“Billboard“始终面向相机_播放序列图的效果_案例分享(内附源码)】
"Billboard"始终面向相机 Shader "billboard" {Properties{_MainTex ("Main Tex", 2D) = "white" {}_Color (...
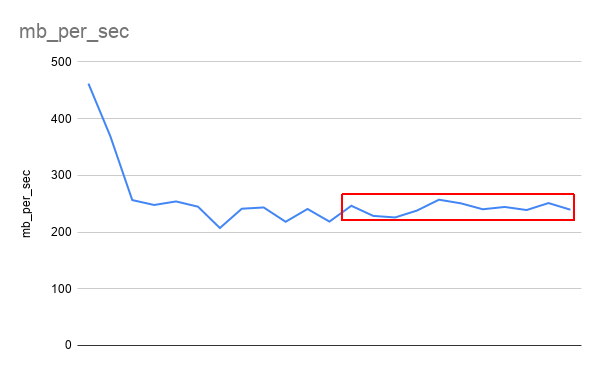
Ceph入门到精通-S3 基准测试工具warp使用入门
S3 基准测试工具。 下载 下载适用于各种平台的二进制版本。 配置 可以使用命令行参数或环境变量配置 Warp。 可以使用 、 在命令行上指定要使用的 S3 服务器,也可以选择指定 TLS 和自定义区域。--host--access-key--secret-key--tls--region 也可以使用 、、 和…...
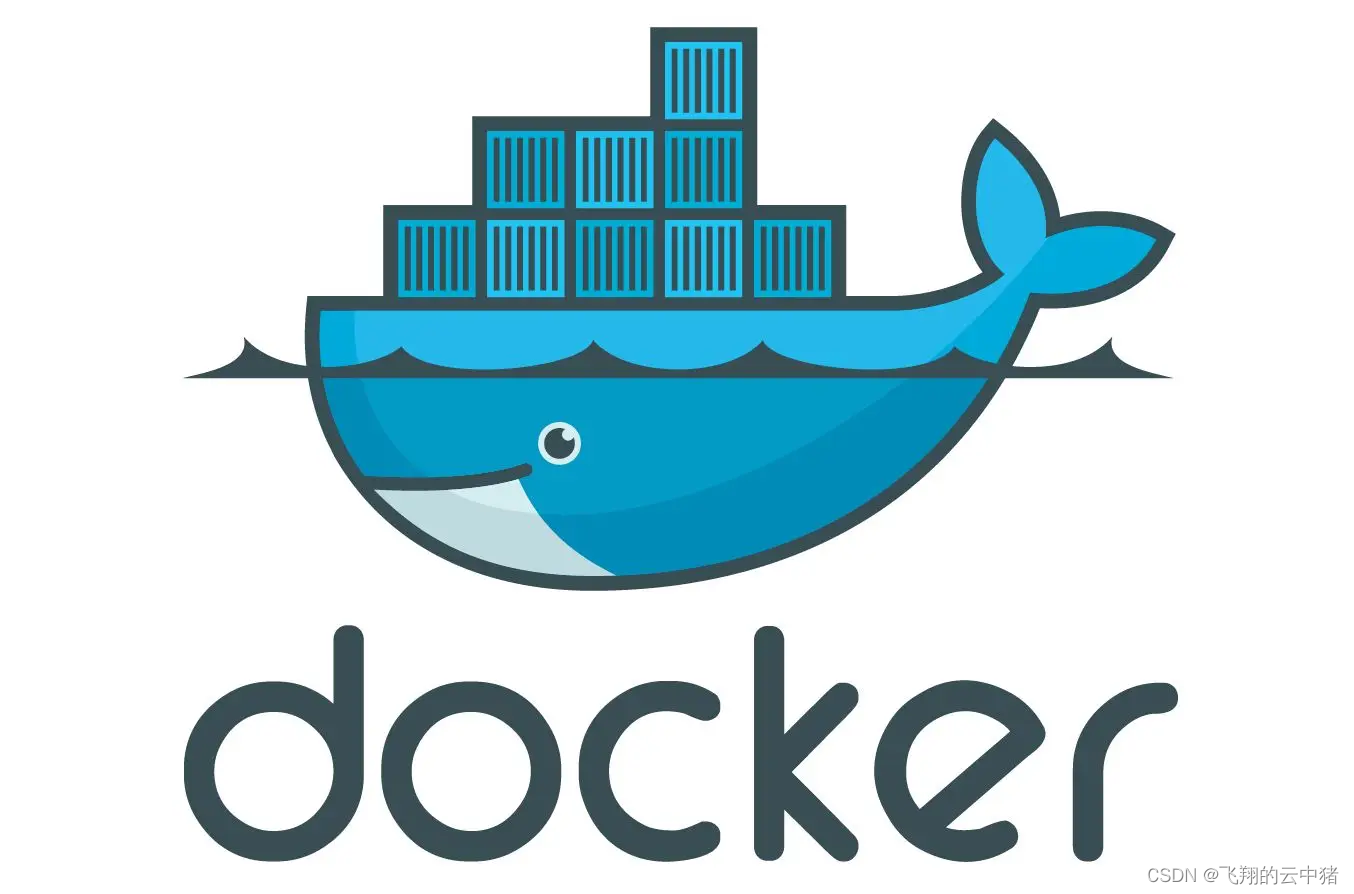
Docker--未完结
一.Docker是干什么的 在没亲自使用过之前,再多的术语也仅仅是抽象,只有写的人或者使用过的人能看懂。 所以,作为新手来说,只要知道Docker是用于部署项目就够了,下面展示如何用Docker部署项目及Docker常用命令。 二、…...

string的使用和模拟实现
💓博主个人主页:不是笨小孩👀 ⏩专栏分类:数据结构与算法👀 C👀 刷题专栏👀 C语言👀 🚚代码仓库:笨小孩的代码库👀 ⏩社区:不是笨小孩👀 🌹欢迎大…...
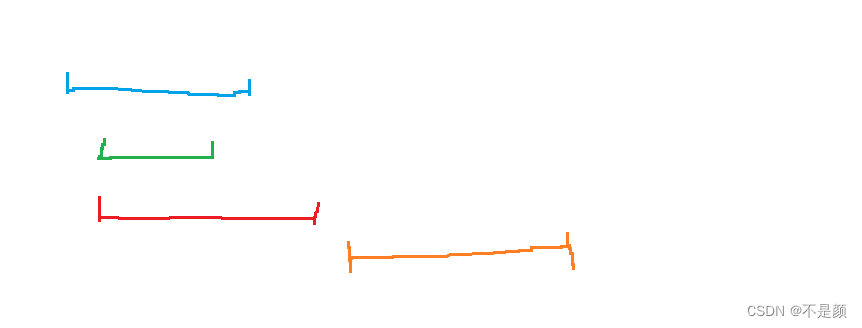
基础算法---区间合并
直接上题目,不废话! 题目 给定 n 个区间 [l,r],要求合并所有有交集的区间。 注意如果在端点处相交,也算有交集。 输出合并完成后的区间个数。 例如:[1,3] 和 [2,6] 可以合并为一个区间 [1,6]。 输入格式 第一行包含整数 n。 接下来 n 行&am…...
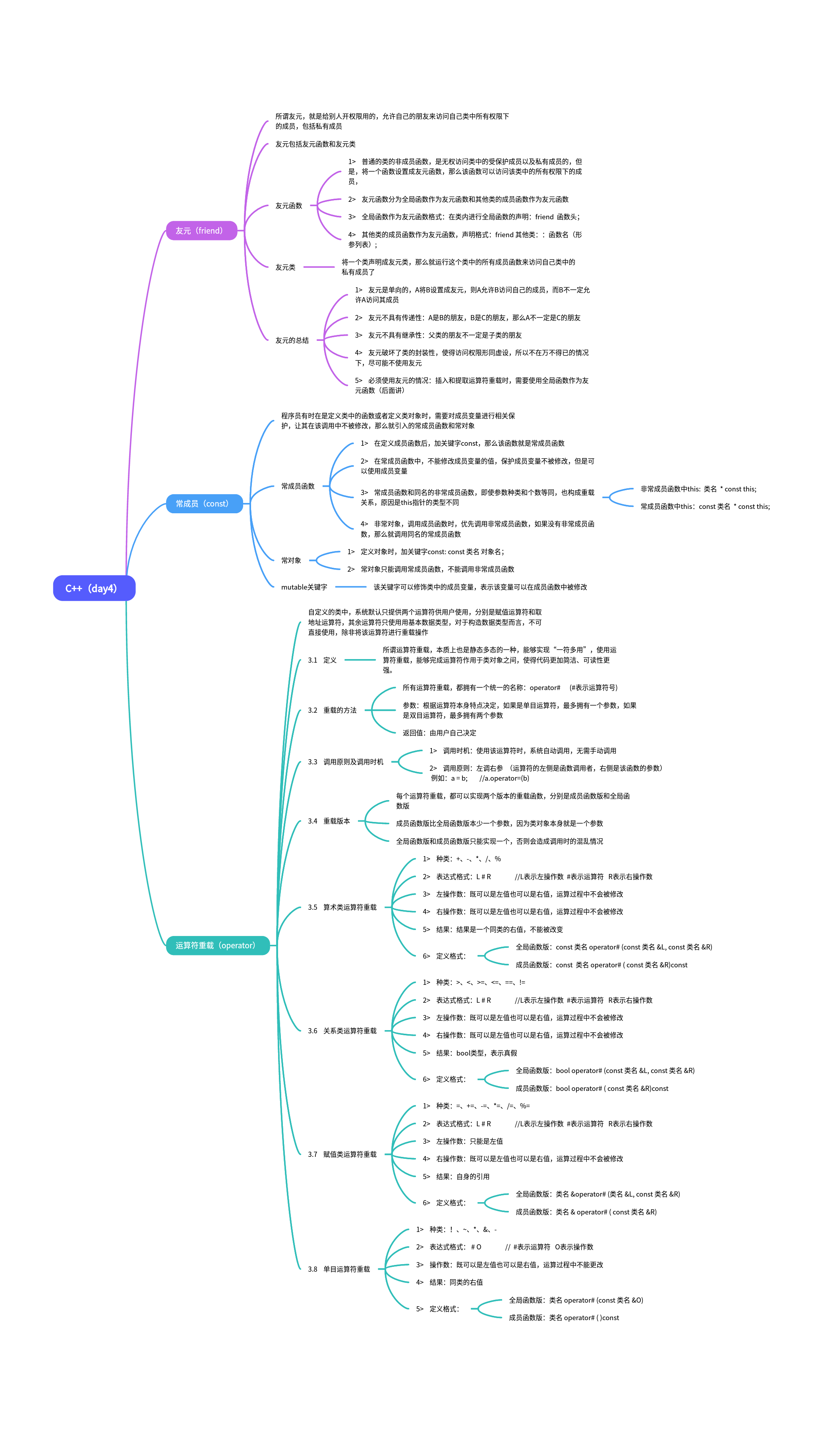
C++(day4)
思维导图 封装Mystring #include <iostream> #include<cstring>using namespace std;class Mystring{ public://无参构造函数Mystring():size(10){strnew char[size];strcpy(str,"");cout<<"无参构造函数"<<endl;}//有参构造函数…...

docker 部署 node.js(express) 服务
1、在 express 项目根目录下新增 Dockerfile 文件,内容如下: 创建服务容器的方法,可以根据自己的情况选择: 1、以下示例为宿主机没有安装 node 环境的写法; 2、先在本地构建包含 node 和 express 的基础镜像࿰…...
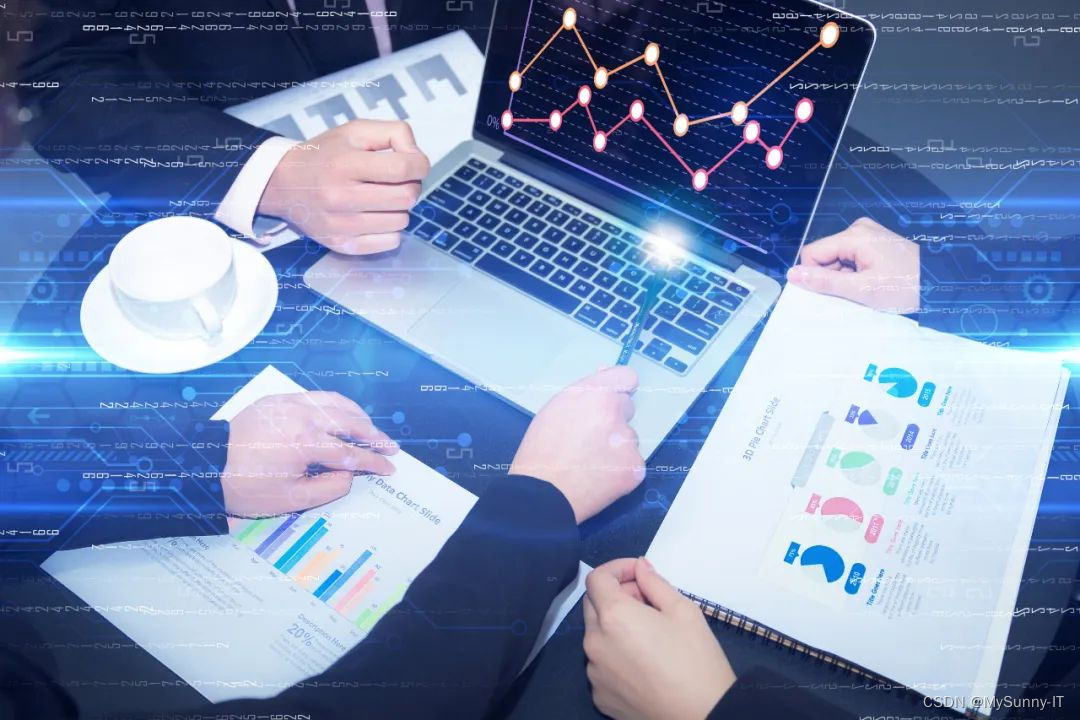
商城系统开发,如何确保用户数据的安全性?
确保用户数据的安全性是商城系统开发中至关重要的一项任务。随着数字化时代的到来,用户的个人信息和交易数据已成为黑客和不法分子的重要目标,因此保护用户数据的安全性对于商城系统的成功运营至关重要。在开发商城系统时,以下几个方面是确保…...
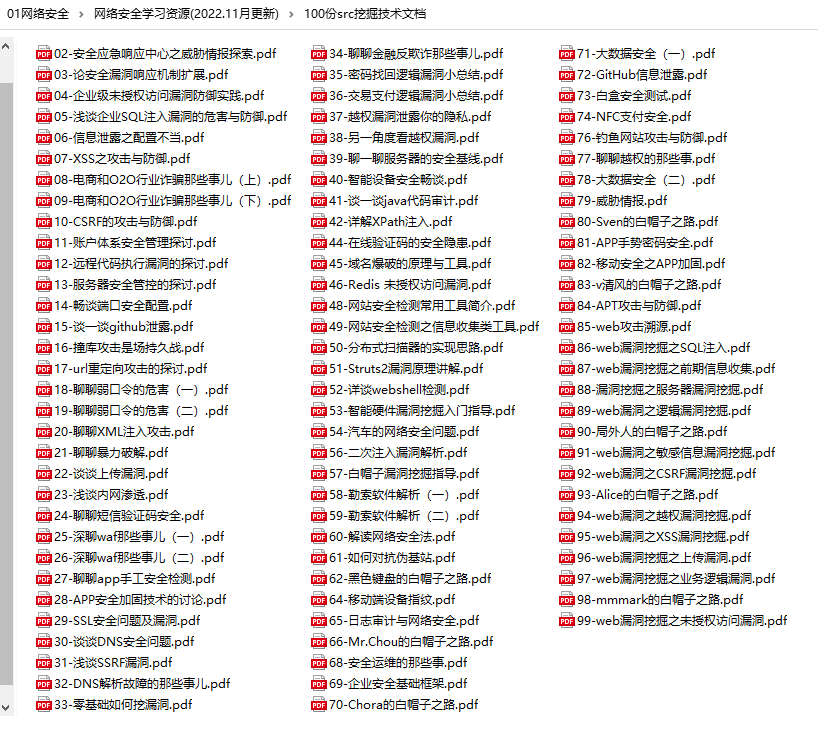
黑客必备工具Kali Linux,安装与使用教程全包含,从入门到精通,全网最详细全面的Kali Linux教程
Kali Linux是一个高级渗透测试和安全审计Linux发行版,目前可以说是网络安全人员的专用系统。 Kali Linux功能非常强大,能够进行信息取证、渗透测试、攻击WPA / WPA2保护的无线网络、离线破解哈希密码、将android、Java、C编写的程序反编译成代码等等&am…...

2024滴滴校招面试真题汇总及其讲解(二)
4.【基础题】HashMap了解吗?介绍一下它对应的线程安全版本。 HashMap 是 Java 中一种键值对映射的集合,它使用哈希表来存储键值对。HashMap 具有插入和删除元素效率高的优势,但不是线程安全的。 ConcurrentHashMap 是 Java 中一种线程安全的 HashMap,它使用分段锁来保证线…...

嵌入式-C语言中的if语句
目录 一.if语句介绍 二.案例实操 2.1C语言运行模板代码 2.2运行方法 2.3案例 一.if语句介绍 if判断语句是一种用于根据条件来进行条件分支的控制流语句。通过判断一个条件的真假来决定执行不同的代码块。if语句的基本语法如下:if (条件表达式) {// 如果条件为…...

组合数 rust解法
组合数。 编写函数,参数是两个非负整数n和m,返回组合数 C n m C_n^m Cnm,其中m≤n≤25。 例如,n25,m12时答案为5200300。 解法: fn c(n: u32, m: u32)->u64 {let m if m > n-m {n-m}else{m};le…...
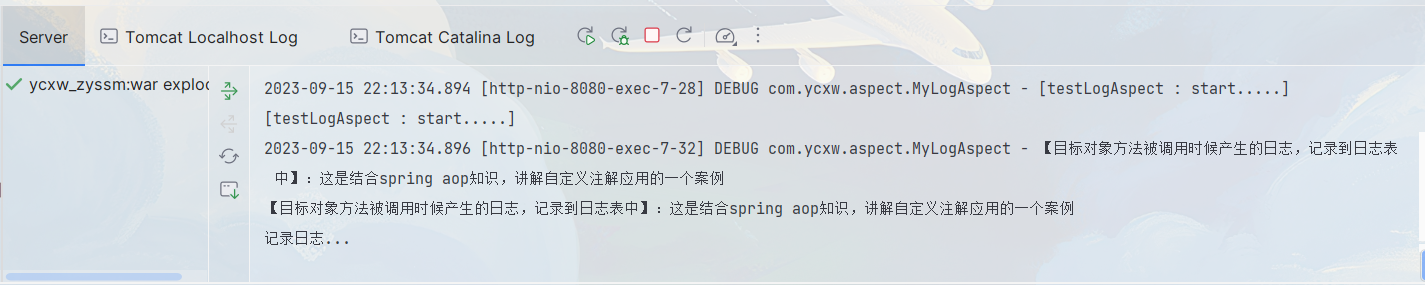
【SpringMVC】自定义注解与AOP结合使用
目录 一、SpringMVC之自定义注解 1.1 Java注解简介 1.2 为什么要用注解 1.3 注解的分类 ⭐ 1.3.1 JDK基本注解 1.3.2 JDK元注解 1.3.3 自定义注解 1.4 自定义注解三种使用案例 1.4.1 案例一(获取类与方法上的注解值) 1.4.2 案例二࿰…...
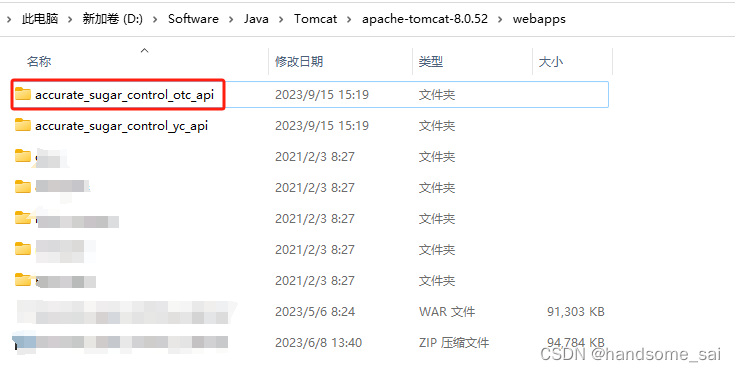
MyEclipse 用tomcat部署SSM项目后,项目名称和当前项目不一致
MyEclipse 用tomcat部署SSM项目后,项目成功启动,但是访问所有接口报404 从这里可以看到,部署的项目名为accurate_sugar_control_yc_api,但实际我们项目名字应该为accurate_sugar_control_otc_api 解决办法 在本地找到项目的根目…...
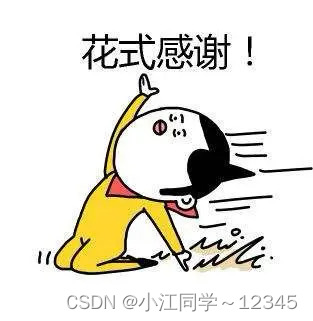
来喽!!炒鸡详细的“数据在内存中的存储”真的来喽!
目录 1. 整数在内存中的存储 1.1 ⼆进制介绍 1.1.1 2进制转10进制 1.1.2 10进制转2进制 1.1.3 2进制转8进制 1.1.4 2进制转16进制 1.2 原码、反码、补码 2. ⼤⼩端字节序和字节序判断 2.1 什么是⼤⼩端? 2.2 为什么有⼤⼩端? 2.3 练习 …...
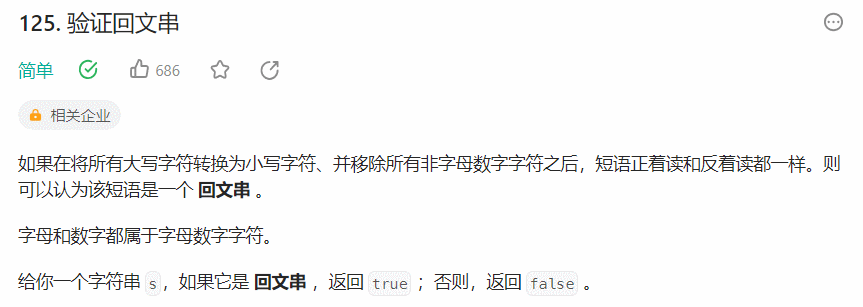
【面试经典150 | 双指针】验证回文串
文章目录 写在前面Tag题目来源题目解读解题思路方法一:筛选判断方法二:原地判断 知识回顾回文串双指针字符串操作 写在最后 写在前面 本专栏专注于分析与讲解【面试经典150】算法,两到三天更新一篇文章,欢迎催更…… 专栏内容以分…...
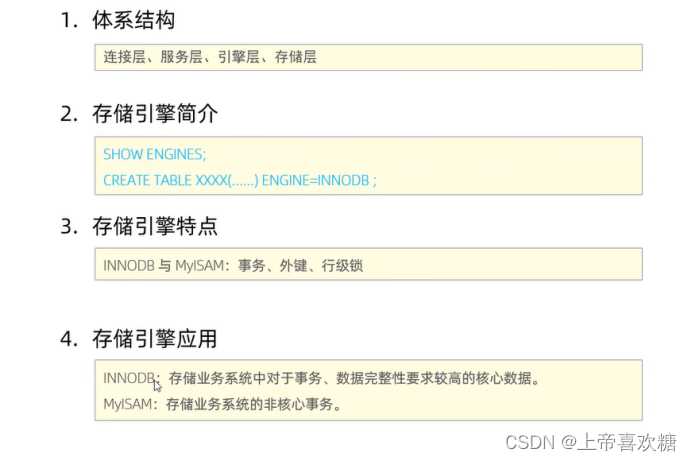
sql存储引擎
-- 查询建表语句 --可以查看引擎 show create table account; -- 可以看到默认引擎 InnoDB ENGINEInnoDB -- 查看当前数据库支持得存储引擎 show engines ; # InnoDB 默认 存储引擎 # MyISAM sql早期默认 存储引擎 # MEMORY 存储在内存中 用来做临时表和缓存 存储引擎 …...
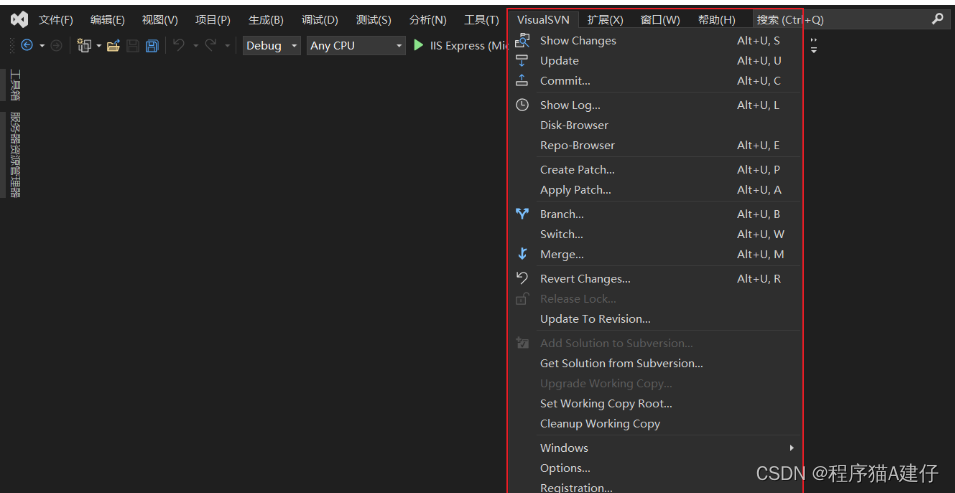
Visual Studio 2022安装SVN插件教程
1. 第一步:避免踩坑,超级重要!!!关闭Visual Studio 2022应用程序;(不然插件装不上,一直转圈!) 2.第二步:下载Visual Studio 2022版本对应的SVN插件…...

【PyCharm Community Edition】:串口开发
串口开发 安装模块:pyserial端口检查:uartDevice自定义文件:SerialMonitor.py导入自定义文件:SerialMonitor.py延伸阅读 安装模块:pyserial Pyserial 是 Python 中使用串口通信的一个第三方库,使用它可以方…...

亲测可用!!!Centos7安装chrome+chromedriver以便实现selenium自动化详细教程
网上很多教程都是在线安装chrome,这样安装了最新稳定的chrome,可惜我遇到chromdriver的版本跟上 chrome,为了早日实现在centos服务selenium自动化,不可能去等待 chromdriver 更新,只能 chrome进行降版本来离线安装。花…...