CUDA C编程权威指南:1.2-CUDA基础知识点梳理
主要整理了N多年前(2013年)学习CUDA的时候开始总结的知识点,好长时间不写CUDA代码了,现在LLM推理需要重新学习CUDA编程,看来出来混迟早要还的。
1.闭扫描和开扫描
对于一个二元运算符 ⊕ \oplus ⊕和一个 n n n元输入数组 [ x 0 , x 1 , . . . , x n − 1 ] \left[ {{x_0},{x_1},...,{x_{n - 1}}} \right] [x0,x1,...,xn−1]。如果返回输出数组为 [ x 0 , ( x 0 ⊕ x 1 ) , . . . , ( x 0 ⊕ x 1 ⊕ … ⊕ x n − 1 ) ] \left[ {{x_0},\left( {{x_0} \oplus {x_1}} \right),...,\left( {{x_0} \oplus {x_1} \oplus \ldots \oplus {x_{n - 1}}} \right)} \right] [x0,(x0⊕x1),...,(x0⊕x1⊕…⊕xn−1)],那么是闭扫描;如果返回输出数组为 [ 0 , x 0 , ( x 0 ⊕ x 1 ) , . . . , ( x 0 ⊕ x 1 ⊕ … ⊕ x n − 2 ) ] \left[ {0,{x_0},\left( {{x_0} \oplus {x_1}} \right),...,\left( {{x_0} \oplus {x_1} \oplus \ldots \oplus {x_{n - 2}}} \right)} \right] [0,x0,(x0⊕x1),...,(x0⊕x1⊕…⊕xn−2)],那么是开扫描。串行闭扫描算法,如下所示:
/*** x: input array* y: output array*/
void sequential_scan(float* x, float* y, int Max_i) {y[0] = x[0];for (int i=1; i<Max_i; i++) {y[i] = y[i-1] + x[i];}
}
说明:在闭扫描的输出和开扫描的输出间互相转换还是比较简单的,只需要移一次位并填上一个元素即可。
(1)从闭扫描转换到开扫描,只需把所有元素右移,0号元素填0值。
(2)从开扫描转换到闭扫描,需要把所有元素向左移动一位,最后一个元素填充原来最后一个元素和输入数组的最后一个元素之和。
说明:假设输入数组为[3, 1, 7, 0, 4, 1, 6, 3],闭操作输出数组为[3, 4, 11, 11, 15, 16, 22, 25],开操作输出数组为[0, 3, 4, 11, 11, 15, 16, 22],可以验证。
2.简单并行扫描
在实践中,并行扫描常常作为一些并行算法的原始操作,比如基数排序、快速排序、字符串比较、多项式求值、递归求解、树操作和直方图等。
解析:
(1)__syncthreads()
确保所有线程在开始下一次迭代之前完成归约树中当前这次迭代的加法。
(2)inclusive scan表示闭扫描部分,而exclusive scan表示开扫描部分。
说明:除了简单并行扫描外,还有工作高效的并行扫描,任意输入长度的并行扫描。
3.Thrust与CUDA的互操作性
解析:Thrust与CUDA的互操作性有利于迭代开发策略,比如使用Thrust库快速开发出并行应用的原型,确定程序瓶颈,使用CUDA C实现特定算法并作必要优化。When a Thrust function is called, it inspects the type of the iterator to determine whether to use a host or a device implementation. This process is known as static dispatching since the host/device dispatch is resolved at compile time. Note that this implies that there is no runtime overhead to the dispatch process.
(1)Thrust到CUDA的互操作性
size_t N = 1024;
device_vector<int> d_vec(N);
int raw_ptr = raw_pointer_cast(&d_vec[0]);
cudaMemset(raw_ptr, 0, N*sizeof(int));
my_kernel << <N / 128, 128 >> >(N, raw_ptr);
说明:通过raw_pointer_cast()将设备地址转换为原始C指针,原始C指针可以调用CUDA C API函数,或者作为参数传递到CUDA C kernel函数中。
(2)CUDA到Thrust的互操作性
size_t N = 1024;
int raw_ptr;
cudaMalloc(&raw_ptr, N*sizeof(int));
device_ptr<int> dev_ptr = device_pointer_cast(raw_ptr);
sort(dev_ptr, dev_ptr+N);
dev_ptr[0] = 1;
cudaFree(raw_ptr);
说明:通过device_pointer_cast()将原始C指针转换为设备向量的设备指针,以便访问Thrust库中的算法。
4.GPU,SM,SP与Grid,Block,Thread之间的映射关系
解析:GPU的任务分配单元将Grid分配到GPU芯片上。任务分配单元使用轮询策略将Block分配到SM上,决定能否分配的因素包括每个Block使用的共享存储器数量,每个Block使用的寄存器数量,以及其它的一些限制条件。SM中的线程调度单元又将分配到的Block进行细分,将其中的线程组织成线程束(Warp),Block中的每一个Thread被发射到一个SP上。一个SM可以同时处理多个Block,比如现在有16个SM、64个Block、每个SM可以同时处理3个Block,那么设备刚开始的时候就会同时处理48个Block,剩下的16个Block等待SM。一个SM一次只会执行一个Block中的一个Warp,但是SM遇到正在执行的Warp需要等待的时候(比如存取Global Memory等),就切换到别的Warp继续做运算。
5.固定内存(pinned memory)
解析:malloc()分配的是可分页的主机内存,而cudaHostAlloc()分配的是页锁定的主机内存,也称固定内存(pinned memory),它的一个重要特点是操作系统不会对这块内存分页并交换到磁盘上,从而保证了这块内存不会被破坏或者重新定位。
6.CUDA 7.5和cuDNN 5.0安装
解析:
(1)解压缩会生成cuda/include、cuda/lib、cuda/bin三个目录;
(2)分别将cuda/include、cuda/lib、cuda/bin三个目录中的内容拷贝到C:\Program Files\NVIDIA GPU Computing Toolkit\CUDA\v7.5对应的include、lib、bin目录下。
说明:CUDA 8.0对应的cuDNN 5.0和CUDA 7.5对应的cuDNN 5.0是不一样的。
7.NVIDIA Deep Learning SDK
解析:
(1)Deep Learning Primitives (cuDNN): High-performance building blocks for deep neural network applications including convolutions, activation functions, and tensor transformations.
(2)Deep Learning Inference Engine (TensorRT): High-performance deep learning inference runtime for production deployment.
(3)Deep Learning for Video Analytics (DeepStream SDK): High-level C++ API and runtime for GPU-accelerated transcoding and deep learning inference.
(4)Linear Algebra (cuBLAS): GPU-accelerated BLAS functionality that delivers 6x to 17x faster performance than CPU-only BLAS libraries.
(5)Sparse Matrix Operations (cuSPARSE): GPU-accelerated linear algebra subroutines for sparse matrices that deliver up to 8x faster performance than CPU BLAS (MKL), ideal for applications such as natural language processing.
(6)Multi-GPU Communication (NCCL): Collective communication routines, such as all-gather, reduce, and broadcast that accelerate multi-GPU deep learning training on up to eight GPUs.
(7)NVIDIA DIGITS:Interactively manage data and train deep learning models for image classification, object detection, and image segmentation without the need to write code.
说明:Fast Fourier Transforms (cuFFT);Dense and Sparse Direct Solvers (cuSOLVER);Random Number Generation (cuRAND);Image & Video Processing Primitives (NPP);NVIDIA Graph Analytics Library (nvGRAPH);Templated Parallel Algorithms & Data Structures (Thrust);CUDA Math Library.
8.istream_iterator和ostream_iterator
解析:
(1)template <class T, class charT=char, class traits=char_traits > class ostream_iterator;
#include <iostream> // std::cout
#include <iterator> // std::ostream_iterator
#include <vector> // std::vector
#include <algorithm> // std::copyint main () {std::vector<int> myvector;for (int i=1; i<10; ++i) myvector.push_back(i*10);std::ostream_iterator<int> out_it (std::cout, ", ");std::copy ( myvector.begin(), myvector.end(), out_it );return 0;
}
(2)template <class T, class charT=char, class traits=char_traits, class Distance = ptrdiff_t> class istream_iterator;
#include <iostream> // std::cin, std::cout
#include <iterator> // std::istream_iterator
using namespace std;int main() {double value1, value2;std::cout << "Please, insert two values: ";std::istream_iterator<double> eos; // end-of-stream iteratorstd::istream_iterator<double> iit(std::cin); // stdin iteratorif (iit != eos){cout << *eos << endl;cout << *iit << endl;cout << "test1" << endl;value1 = *iit;}++iit;if (iit != eos){cout << *eos << endl;cout << *iit << endl;cout << "test2" << endl;value2 = *iit;}std::cout << value1 << "*" << value2 << "=" << (value1*value2) << '\n';return 0;
}
9.__host__ __device__ int foo(int a){}
解析:host int foo(int a){}表示由CPU调用的函数。device int foo(int a){}表示由GPU调用的函数。host__和__device__关键字可以连用,比如__host __device__
int foo(int a){}会被编译成两个版本,分别可以由CPU和GPU调用。
10.SAXPY
解析:SAXPY(Scalar Alpha X Plus Y)是一个在Basic Linear Algebra Subprograms(BLAS)数据包中的函数,并且是一个并行向量处理机(vector processor)中常用的计算操作指令。SAXPY是标量乘法和矢量加法的组合:y=ax+y,其中a是标量,x和y矢量。
struct saxpy_functor
{ const float a; saxpy_functor(float _a) : a(_a) {} __host__ __device__ float operator()(const float& x, const float& y) const { return a * x + y; }
}; void saxpy_fast(float A, thrust::device_vector<float>& X, thrust::device_vector<float>& Y)
{ // Y <- A * X + Y thrust::transform(X.begin(), X.end(), Y.begin(), Y.begin(), saxpy_functor(A));
} void saxpy_slow(float A, thrust::device_vector<float>& X, thrust::device_vector<float>& Y
{ thrust::device_vector<float> temp(X.size()); // temp <- A thrust::fill(temp.begin(), temp.end(), A); // temp <- A * X thrust::transform(X.begin(), X.end(), temp.begin(), temp.begin(), thrust::multiplies<float>()); // Y <- A * X + Y thrust::transform(temp.begin(), temp.end(), Y.begin(), Y.begin(), thrust::plus<float>());
}
说用:仿函数(functor),就是使一个类的使用看上去像一个函数。其实现就是类中实现一个operator(),这个类就有了类似函数的行为,就是一个仿函数类了。
11.Thrust中的Transformations(转换)
解析:
(1)thrust::fill
(2)thrust::sequence
(3)thrust::replace
(4)thrust::transform
(5)thrust::negate
(6)thrust::modulus
(7)thrust::zip_iterator
(8)thrust::for_each
12.Thrust中的Reductions(规约)
(1)thrust::reduce
(2)thrust::count
(3)thrust::count_if
(4)thrust::min_element
(5)thrust::max_element
(6)thrust::is_sorted
(7)thrust::inner_product
(8)thrust::transform_reduce
(9)transform_inclusive_scan
(10)transform_exclusive_scan
13.初始化thrust::device_vector
解析:
float x[4] = { 1.0, 2.0, 3.0, 4.0 };
thrust::device_vector<float> d_x(x, x + 4);
for (int i = 0; i < d_x.size(); i++)cout << d_x[i] << endl;
14.template<typename T> struct thrust::plus< T >
解析:
#include <thrust/functional.h>。
int sum = thrust::reduce(D.begin(), D.end(), (int) 0, thrust::plus<int>());
float norm = std::sqrt(thrust::transform_reduce(d_x.begin(), d_x.end(), unary_op, init, binary_op));
15.cudaDeviceReset
解析:重置当前线程所关联过的当前设备的所有资源。
16.CUDART_VERSION
解析:CUDA 7.5版本的CUDART_VERSION为7050,包含在头文件#include<cuda_runtime_api.h>中。
17.thrust::count
解析:thrust:count函数原型,如下所示:
template<typename InputIterator , typename EqualityComparable >
thrust::iterator_traits<InputIterator>::difference_type thrust::count (
InputIterator first,
InputIterator last,
const EqualityComparable & value
)
说明:count returns the number of iterators i in [first, last) such that *i == value.
18.transform_reduce
解析:transform_reduce函数原型,如下所示:
template<typename InputIterator , typename UnaryFunction , typename OutputType , typename BinaryFunction >
OutputType thrust::transform_reduce ( InputIterator first,
InputIterator last,
UnaryFunction unary_op,
OutputType init,
BinaryFunction binary_op
)
举个例子,如下所示:
#include <thrust\transform_reduce.h>
#include <thrust\functional.h>
#include <thrust\device_vector.h>
#include <thrust\host_vector.h>
#include <cmath>
using namespace std;
using namespace thrust;template <typename T>
struct square
{__host__ __device__T operator()(const T& x) const {return x*x;}
};int main(void)
{float x[4] = { 1.0, 2.0, 3.0, 4.0 };device_vector<float> d_x(x, x + 4);square<float> unary_op;thrust::plus<float> binary_op;float init = 10;float norm = thrust::transform_reduce(d_x.begin(), d_x.end(), unary_op, init, binary_op);cout << norm << endl;return 0;
}
19.Prefix-Sums:inclusive_scan和exclusive_scan
解析:
#include <thrust\scan.h>
#include <thrust\device_vector.h>
#include <thrust\host_vector.h>
using namespace std;
using namespace thrust;int main(void)
{int data[6] = { 1, 0, 2, 2, 1, 3 };// data is now {1, 1, 3, 5, 6, 9}// data[2] = data[0] + data[1] + data[2]// thrust::inclusive_scan(data, data + 6, data);// data is now {0, 1, 1, 3, 5, 6}// data[2] = data[0] + data[1]thrust::exclusive_scan(data, data + 6, data);for (int i = 0; i < 6; i++){cout << data[i] << endl;}return 0;
}
20.thrust::sort
和thrust::stable_sort
解析;thrust::stable_sort函数原型,如下所示:
template<typename DerivedPolicy , typename RandomAccessIterator , typename StrictWeakOrdering >
__host__ __device__ void thrust::stable_sort (
const thrust::detail::execution_policy_base< DerivedPolicy > & exec,
RandomAccessIterator first,
RandomAccessIterator last,
StrictWeakOrdering comp
)
(1)exec:The execution policy to use for parallelization.
(2)first:The beginning of the sequence.
(3)last:The end of the sequence.
(4)comp:Comparison operator.
举个例子,如下所示:
#include <thrust\sort.h>
using namespace std;
using namespace thrust;int main(void)
{const int N = 6;int A[N] = { 1, 4, 2, 8, 5, 7 };// A is now {1, 2, 4, 5, 7, 8}// thrust::sort(A, A + N);// A is now {1, 2, 4, 5, 7, 8}thrust::stable_sort(A, A + N);for (int i = 0; i < 6; i++){cout << A[i] << endl;}return 0;
}
(1)#include <thrust/functional.h>:操作的函数对象和工具。
(2)#include <thrust/execution_policy.h>:Thrust执行策略。
21.thrust::sort_by_key和thrust::stable_sort_by_key
解析:
#include <thrust\sort.h>
using namespace std;
using namespace thrust;int main(void)
{const int N = 6;int keys[N] = { 1, 4, 2, 8, 5, 7 };char values[N] = { 'a', 'b', 'c', 'd', 'e', 'f' };// keys is now { 1, 2, 4, 5, 7, 8} // values is now {'a', 'c', 'b', 'e', 'f', 'd'}// thrust::sort_by_key(keys, keys + N, values);// keys is now { 1, 2, 4, 5, 7, 8} // values is now {'a', 'c', 'b', 'e', 'f', 'd'}thrust::stable_sort_by_key(keys, keys + N, values);for (int i = 0; i < 6; i++){cout << values[i] << endl;}return 0;
}
22.Thrust中的Iterator
解析:
(1)constant_iterator
(2)counting_iterator
#include <thrust\iterator\constant_iterator.h>
#include <thrust/iterator/counting_iterator.h>
#include <thrust\reduce.h>
#include <iostream>
using namespace std;
using namespace thrust;int main(void)
{thrust::constant_iterator<int> first(10);thrust::constant_iterator<int> last = first + 3;// returns 30 (i.e. 3 * 10)// thrust::reduce(first, last);// returns 33 (i.e. 10 + 11 + 12) thrust::reduce(first, last); cout << thrust::reduce(first, last) << endl;return 0;
}
(3)transform_iterator
#include <thrust\iterator\transform_iterator.h>
#include <thrust\device_vector.h>
#include <iostream>
using namespace std;
using namespace thrust;int main(void)
{thrust::device_vector<int> vec(3);vec[0] = 10;vec[1] = 20;vec[2] = 30;// returns -60 (i.e. -10 + -20 + -30)cout << thrust::reduce(thrust::make_transform_iterator(vec.begin(), thrust::negate<int>()),thrust::make_transform_iterator(vec.end(), thrust::negate<int>())) << endl;return 0;
}
(4)permutation_iterator
#include <thrust\iterator\permutation_iterator.h>
#include <thrust\device_vector.h>
#include <iostream>
using namespace std;
using namespace thrust;int main(void)
{thrust::device_vector<int> map(4);map[0] = 3;map[1] = 1;map[2] = 0;map[3] = 5;thrust::device_vector<int> source(6);source[0] = 10;source[1] = 20;source[2] = 30;source[3] = 40;source[4] = 50;source[5] = 60;// sum = source[map[0]] + source[map[1]] + ...int sum = thrust::reduce(thrust::make_permutation_iterator(source.begin(), map.begin()),thrust::make_permutation_iterator(source.begin(), map.end()));cout << sum << endl;return 0;
}
(5)zip_iterator
#include <thrust\iterator\zip_iterator.h>
#include <thrust\device_vector.h>
#include <iostream>
using namespace std;
using namespace thrust;int main(void)
{thrust::device_vector<int> A(3);thrust::device_vector<char> B(3);A[0] = 10; A[1] = 20; A[2] = 30; B[0] = 'x'; B[1] = 'y'; B[2] = 'z';thrust::maximum< thrust::tuple<int, char> > binary_op;thrust::tuple<int, char> init = thrust::make_zip_iterator(thrust::make_tuple(A.begin(), B.begin()))[0];thrust::tuple<int, char> result = thrust::reduce(thrust::make_zip_iterator(thrust::make_tuple(A.begin(), B.begin())), thrust::make_zip_iterator(thrust::make_tuple(A.end(), B.end())), init, binary_op);cout << thrust::get<0>(result) << endl;cout << thrust::get<1>(result) << endl;return 0;
}
23.#include<stdlib.h>
解析:
(1)#define EXIT_SUCCESS 0
(2)#define EXIT_FAILURE 1
24.cuBLAS与CUBLASXT
解析:在CUDA 6的开发包中,提供了一个新的API——CUBLASXT,它是在cuBLAS API的上层封装了一个矩阵分块算法,解决了当数据量大时显存不足的问题。
25.cuRAND库
解析:cuRAND库提供了通过GPU生成随机数的接口,包含头文件#include <curand_kernel.h>。
26.CUDA同步方式
解析:在CUDA中,有两种方式实现同步,如下所示:
(1)System-level:等待所有host和device的工作完成。
(2)Block-level:等待device中block的所有thread执行到某个点。
27.CUDA中的on-chip和off-chip内存
解析:
(1)共享内存(share memory)是on-chip。局部内存(local memory)和全局内存(global memory)是off-chip。它为一个线程块(block)中所有线程共享。
(2)局部内存(local memory)是全局内存(global memory)中划出的一部分。它为一个线程网格(grid)中的所有线程共享。
28.CUDA内存管理
(1)cudaError_t cudaMalloc(void** devPtr, size_t count);(2)cudaError_t cudaMallocPitch(void** devPtr, size_t* pitch, size_t widthInBytes, size_t height);(3)cudaError_t cudaFree(void* devPtr);(4)cudaError_t cudaMallocArray(struct cudaArray** array, const struct cudaChannelFormatDesc* desc, size_t width, size_t height);(5)cudaError_t cudaFreeArray(struct cudaArray* array);(6)cudaError_t cudaMallocHost(void** hostPtr, size_t size);(page-locked)(7)cudaError_t cudaFreeHost(void* hostPtr);(8)cudaError_t cudaMemset(void* devPtr, int value, size_t count);(9)cudaError_t cudaMemset2D(void* dstPtr, size_t pitch, int value, size_t width, size_t height)(10)cudaError_t cudaMemcpy(void* dst, const void* src, size_t count, enum cudaMemcpyKind kind);
说明:kind可以是cudaMemcpyHostToHost,cudaMemcpyHostToDevice,cudaMemcpyDeviceToHost或cudaMemcpyDeviceToDevice。(11)cudaError_t cudaMemcpyAsync(void* dst,constvoid*src, size_t count, enum cudaMemcpyKind kind, cudaStream_t stream);
说明:它只能应用于page-locked的主机内存。(12)cudaError_t cudaMemcpy2D(void* dst, size_t dpitch, const void* src, size_t spitch, size_t width, size_t height, enum cudaMemcpyKind kind);(13)cudaError_t cudaMemcpy2DAsync(void* dst, size_t dpitch, const void* src, size_t spitch, size_t width, size_t height, enum cudaMemcpyKind kind, cudaStream_t stream);
说明:dpitch:Pitch of destination memory;spitch:Pitch of source memory。(14)cudaError_t cudaMemcpyToArray(struct cudaArray* dstArray, size_t dstX, size_t dstY, const void* src, size_t count, enum cudaMemcpyKind kind);(15)cudaError_t cudaMemcpyToArrayAsync(struct cudaArray* dstArray, size_t dstX, size_t dstY, const void* src, size_t count, enum cudaMemcpyKind kind, cudaStream_t stream);
说明:拷贝count字节,从src指向的内存区域到dstArray指向的CUDA数组,从数组的左上角(dstX, dstY)开始。(16)cudaError_t cudaMemcpy2DToArray(struct cudaArray* dstArray, size_t dstX, size_t dstY, const void* src, size_t spitch, size_t width, size_t height, enum cudaMemcpyKind kind);(17)cudaError_t cudaMemcpy2DToArrayAsync(struct cudaArray* dstArray, size_t dstX, size_t dstY, const void* src, size_t spitch, size_t width, size_t height, enum cudaMemcpyKind kind, cudaStream_t stream);
说明:拷贝一个矩阵,从src指向的内存区域到dstArray指向的CUDA数组,从数组的左上角(dstX, dstY)开始。spitch是由src指向的2D数组中的内存宽度字节,2D 数组中每行的最后包含自动填充的数值。(18)cudaError_t cudaMemcpyFromArray(void* dst, const struct cudaArray* srcArray, size_t srcX, size_t srcY, size_t count, enum cudaMemcpyKind kind);(19)cudaError_t cudaMemcpyFromArrayAsync(void* dst, const struct cudaArray* srcArray, size_t srcX, size_t srcY, size_t count, enum cudaMemcpyKind kind, cudaStream_t stream);
说明:拷贝count字节,从srcArray指向的CUDA数组,从数组的左上角(srcX, srcY)开始,到dst指向内存区域。(20)cudaError_t cudaMemcpy2DFromArray(void* dst, size_t dpitch, const struct cudaArray* srcArray, size_t srcX, size_t srcY, size_t width, size_t height, enum cudaMemcpyKind kind);(21)cudaError_t cudaMemcpy2DFromArrayAsync(void* dst, size_t dpitch, const struct cudaArray* srcArray, size_t srcX, size_t srcY, size_t width, size_t height, enum cudaMemcpyKind kind, cudaStream_t stream);
说明:拷贝一个矩阵,从srcArray指向的CUDA数组,从数组的左上角(srcX, srcY)开始,到dst指向的内存区域。dpitch是由dst指向的2D数组中的内存宽度字节,2D数组中每行的最后包含自动填充的数值。(22)cudaError_t cudaMemcpyArrayToArray(struct cudaArray* dstArray, size_t dstX, size_t dstY, const struct cudaArray* srcArray, size_t srcX, size_t srcY, size_t count, enum cudaMemcpyKind kind);
说明:拷贝count字节,从srcArray指向的CUDA数组,从数组的左上角(srcX, srcY)开始,到dstArray指向的CUDA数组,从数组的左上角(dstX, dstY)。(23)cudaError_t cudaMemcpyArrayToArray(struct cudaArray* dstArray, size_t dstX, size_t dstY, const struct cudaArray* srcArray, size_t srcX, size_t srcY, size_t width, size_t height, enum cudaMemcpyKind kind);
说明:拷贝一个矩阵,从srcArray指向的CUDA数组,从数组的左上角(srcX, srcY)开始,到dstArray指向的CUDA数组,从数组的左上角(dstX, dstY)。(24)template<class T> cudaError_t cudaMemcpyToSymbol(const T& symbol, const void* src, size_t count, size_t offset = 0, enum cudaMemcpyKind kind = cudaMemcpyHostToDevice);
说明:拷贝count字节,从src指向的内存区域到由符号symbol起始的offset字节指向的内存区域。symbol指向的是在device中的global或者constant memory。(25)template<class T> cudaError_t cudaMemcpyFromSymbol(void *dst, const T& symbol, size_t count, size_t offset = 0, enum cudaMemcpyKind kind = cudaMemcpyDeviceToHost);
说明:拷贝count字节,从由符号symbol起始的offset字节指向的内存区域到dst指向的内存区域。symbol指向的是在device中的global或者constant memory。(26)template<class T> cudaError_t cudaGetSymbolAddress(void** devPtr, const T& symbol);
说明:返回设备中符号symbol的地址*devPtr。(27)template<class T> cudaError_t cudaGetSymbolSize(size_t* size, const T& symbol );
说明:返回符号symbol大小的地址*size。
参考文献:
[1] CUDA并行算法系列之规约:http://blog.5long.me/2016/algorithms-on-cuda-reduction/
[2] 大规模并行处理器编程实战(第2版)
[3] CUDA之深入理解threadIdx:http://blog.csdn.net/canhui_wang/article/details/51730264
[4] Thrust:http://docs.nvidia.com/cuda/thrust/index.html#abstract
[5] GPU中的几个基本概念:http://blog.sina.com.cn/s/blog_80ce3a550101lntp.html
[6] Thrust:http://docs.nvidia.com/cuda/thrust/index.html#axzz4aFPI7CYb
[7] on-chip memory概念:http://bbs.csdn.net/topics/340269551
相关文章:

CUDA C编程权威指南:1.2-CUDA基础知识点梳理
主要整理了N多年前(2013年)学习CUDA的时候开始总结的知识点,好长时间不写CUDA代码了,现在LLM推理需要重新学习CUDA编程,看来出来混迟早要还的。 1.闭扫描和开扫描 对于一个二元运算符 ⊕ \oplus ⊕和一个 n n n元…...

C语言—位运算符
目录 &(位与,AND): |(位或,OR): 位取反(~): 左移(<<): 右移(>>): &(位与,AND)&…...
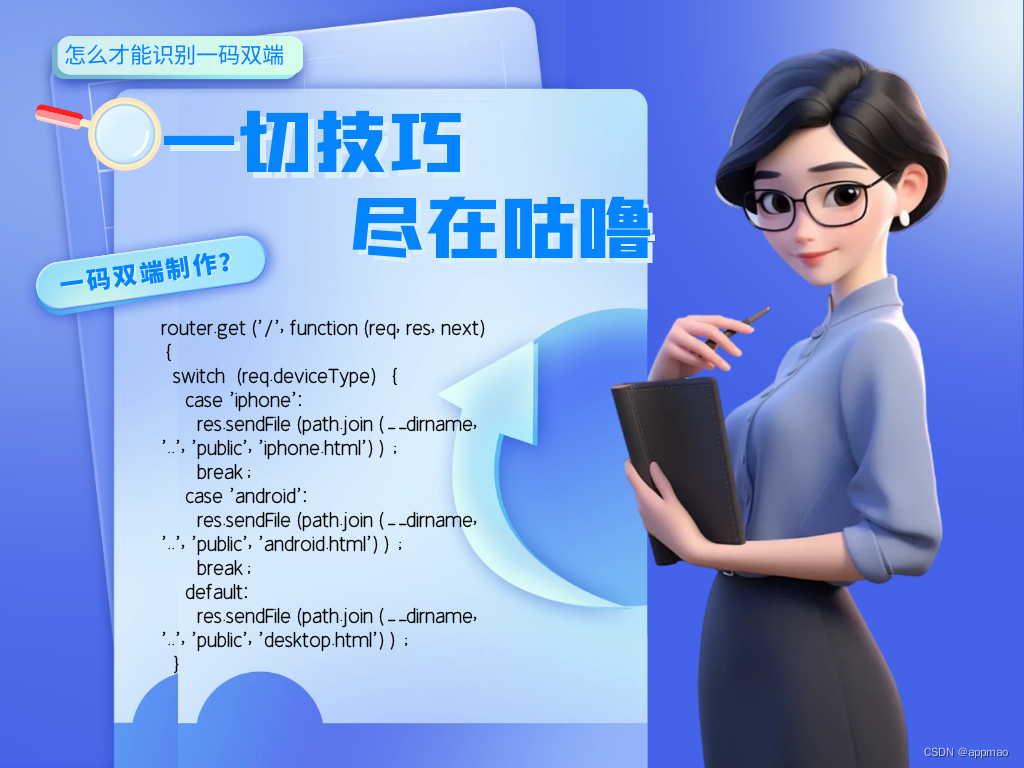
怎么才能实现一个链接自动识别安卓.apk苹果.ipa手机和win电脑wac电脑
您想要实现的功能是通过检测用户代理(User Agent)来识别访问设备类型并根据设备类型展示相应的页面。您可以根据以下步骤进行实现: 选择后端语言和框架,例如:Node.js、Express。 创建一个新的Express项目。 编写一个…...
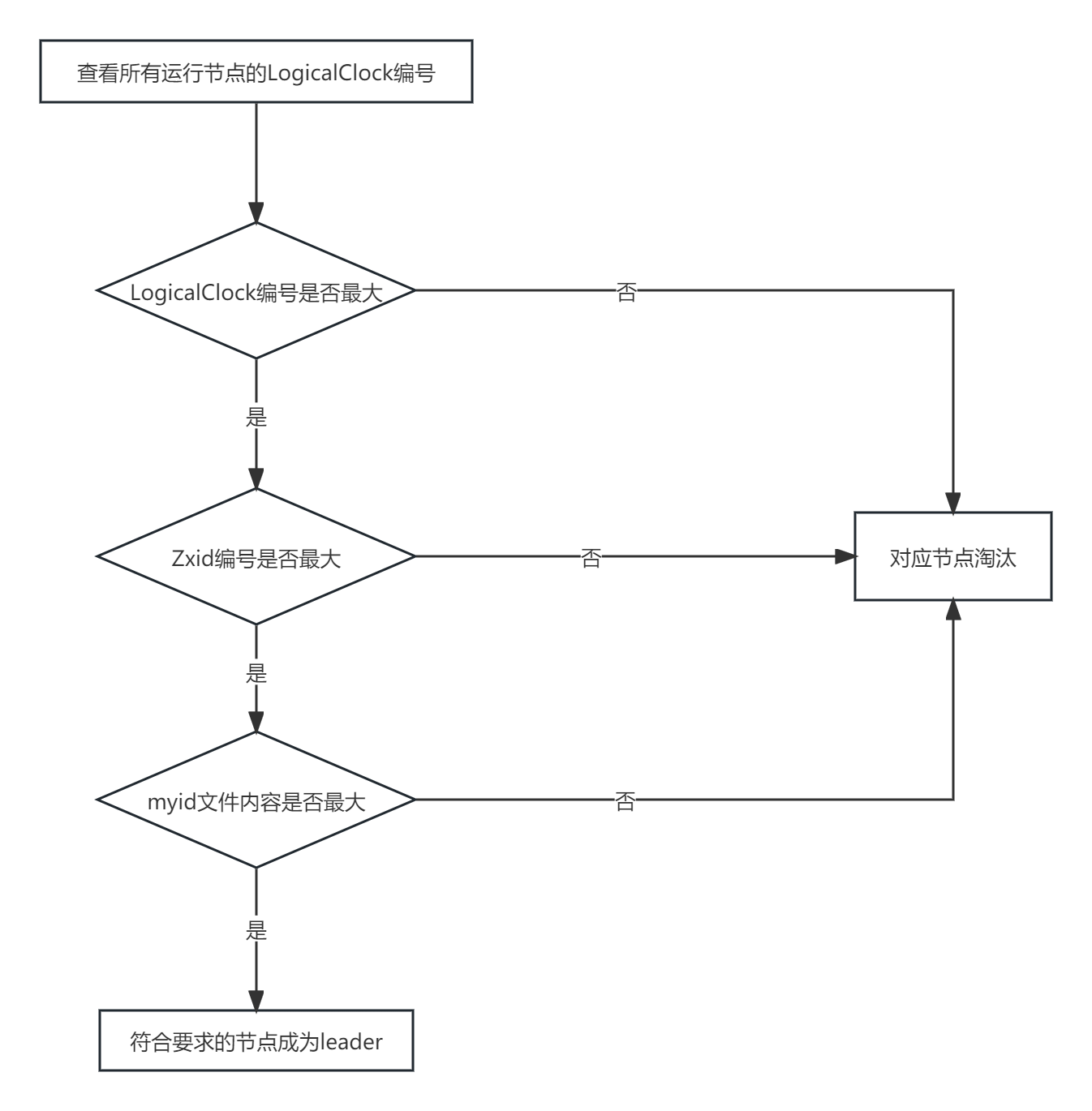
zookeeper选举机制
全新集群选举 zookeeper 全新集群选举机制网上资料很多说法很模糊,仔细思考了一下,应该是这样 得到票数最多的机器>机器总数半数 具体启动过程中的哪个节点成为 leader 与 zoo.cfg 中配置的节点数有关,下面以3个举例 选举过程如下 server…...

vcpkg切换 Visual Studio 版本
vcpkg切换 Visual Studio 版本 在使用vcpkg作为项目的包管理工具时,可能会遇到需要切换Visual Studio版本的情况。下面是一种简单的方法来实现这个目标,通过修改triplet文件来指定使用的Visual Studio版本。 步骤1: 创建或修改Triplet文件 首先&#…...
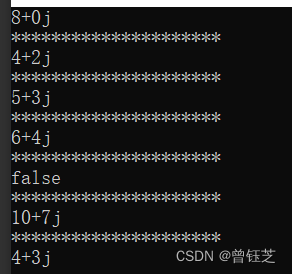
运算符重载
#include <iostream> using namespace std; class Num { private:int num1; //实部int num2; //虚部 public:Num(){}; //无参构造Num(int n1,int n2):num1(n1),num2(n2){}; //有参构造~Num(){}; //析构函数const Num operator(const Num &other)const //加号重载{Nu…...

Llama2-Chinese项目:7-外延能力LangChain集成
本文介绍了Llama2模型集成LangChain框架的具体实现,这样可更方便地基于Llama2开发文档检索、问答机器人和智能体应用等。 1.调用Llama2类 针对LangChain[1]框架封装的Llama2 LLM类见examples/llama2_for_langchain.py,调用代码如下所示:…...

ES6中数组的扩展
1. 扩展运算符 用三个点(...)表示,它如同rest参数的逆运算,将数组转为用逗号分隔的参数序列。扩展就是将一个集合分成一个个的。 console.log(...[1, 2, 3]); // 1, 2, 3可以用于函数调用 扩展运算符后还可以放置表达式 ...(x > 0 ? [a] : [])如…...
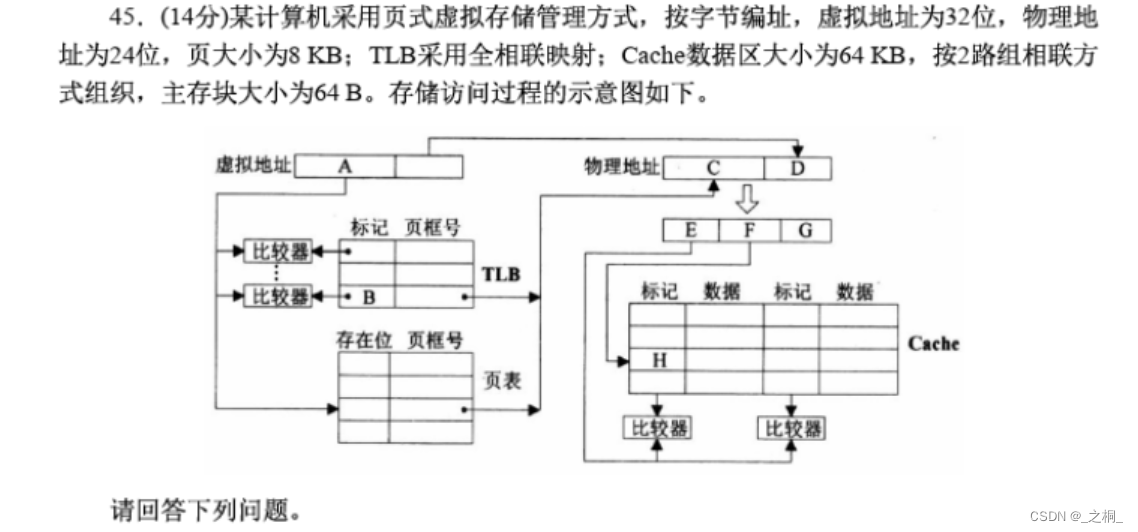
计算机考研 | 2016年 | 计算机组成原理真题
文章目录 【计算机组成原理2016年真题44题-9分】【第一步:信息提取】【第二步:具体解答】 【计算机组成原理2016年真题45题-14分】【第一步:信息提取】【第二步:具体解答】 【计算机组成原理2016年真题44题-9分】 假定CPU主频为5…...
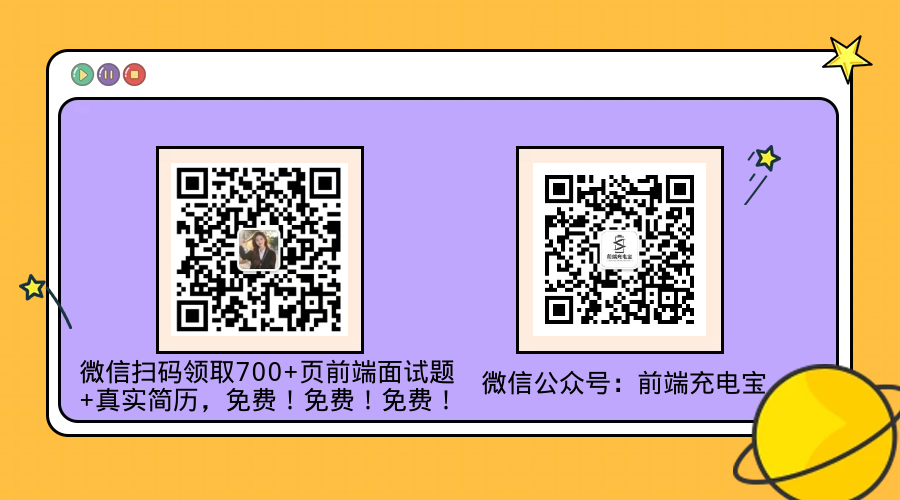
Web版Photoshop来了,用到了哪些前端技术?
经过 Adobe 工程师多年来的努力,并与 Chrome 等浏览器供应商密切合作,通过 WebAssembly Emscripten、Web Components Lit、Service Workers Workbox 和新的 Web API 的支持,终于在近期推出了 Web 版 Photoshop(photoshop.adobe…...
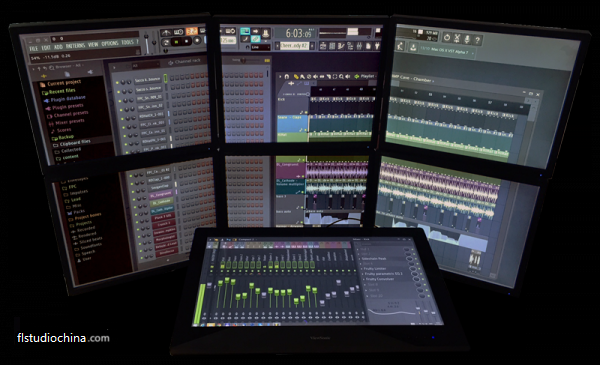
FL Studio21.1.0水果中文官方网站
FL Studio 21.1.0官方中文版重磅发布纯正简体中文支持,更快捷的音频剪辑及素材管理器,多样主题随心换!Mac版新增对苹果M2/1家族芯片原生支持。DAW界萌神!极富二次元造型的水果娘FL chan通过FL插件Fruity Dance登场,为其…...
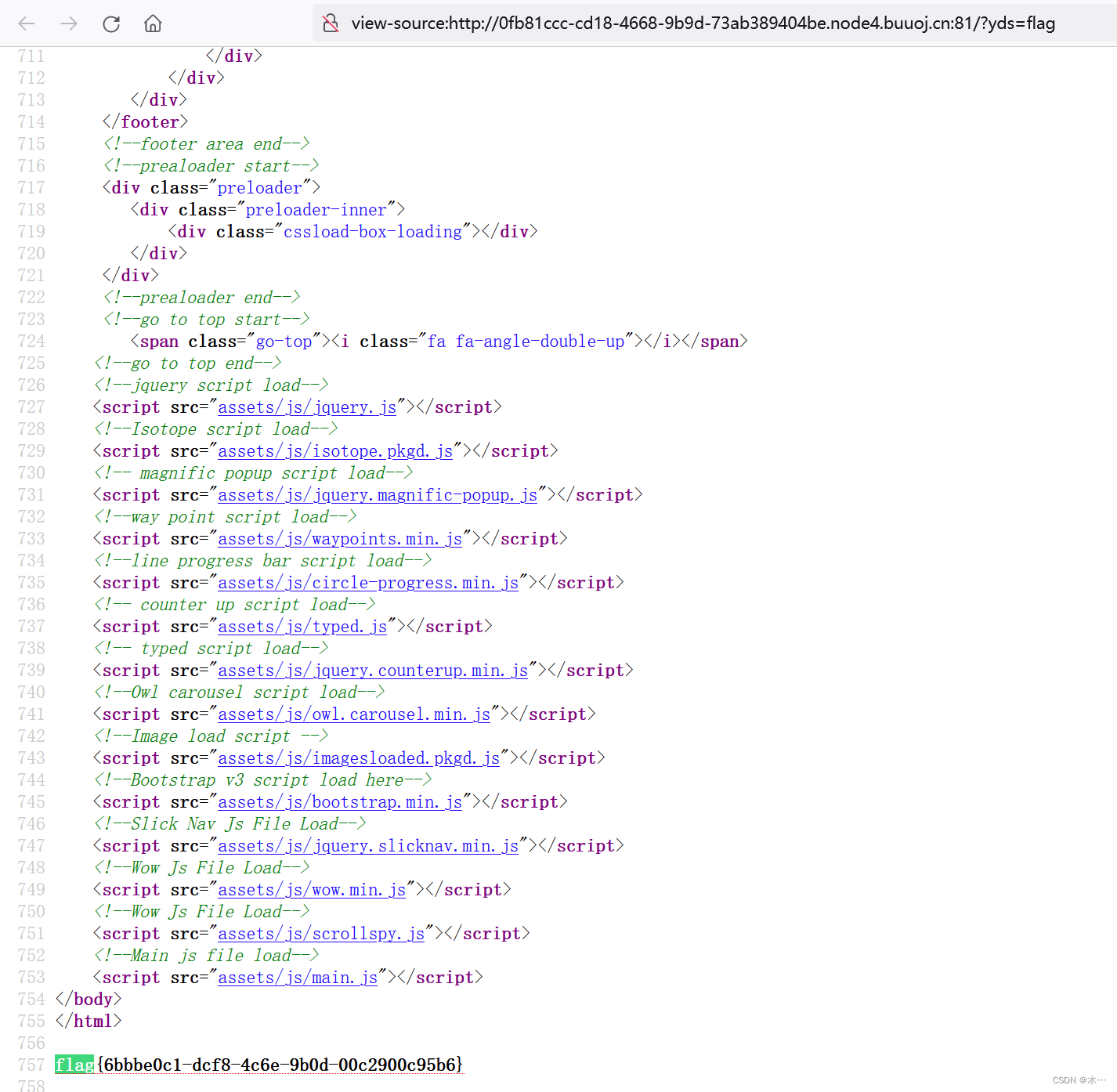
[BJDCTF2020]Mark loves cat
先用dirsearch扫一下,访问一下没有什么 需要设置线程 dirsearch -u http://8996e81f-a75c-4180-b0ad-226d97ba61b2.node4.buuoj.cn:81/ --timeout2 -t 1 -x 400,403,404,500,503,429使用githack python2 GitHack.py http://8996e81f-a75c-4180-b0ad-226d97ba61b2.…...
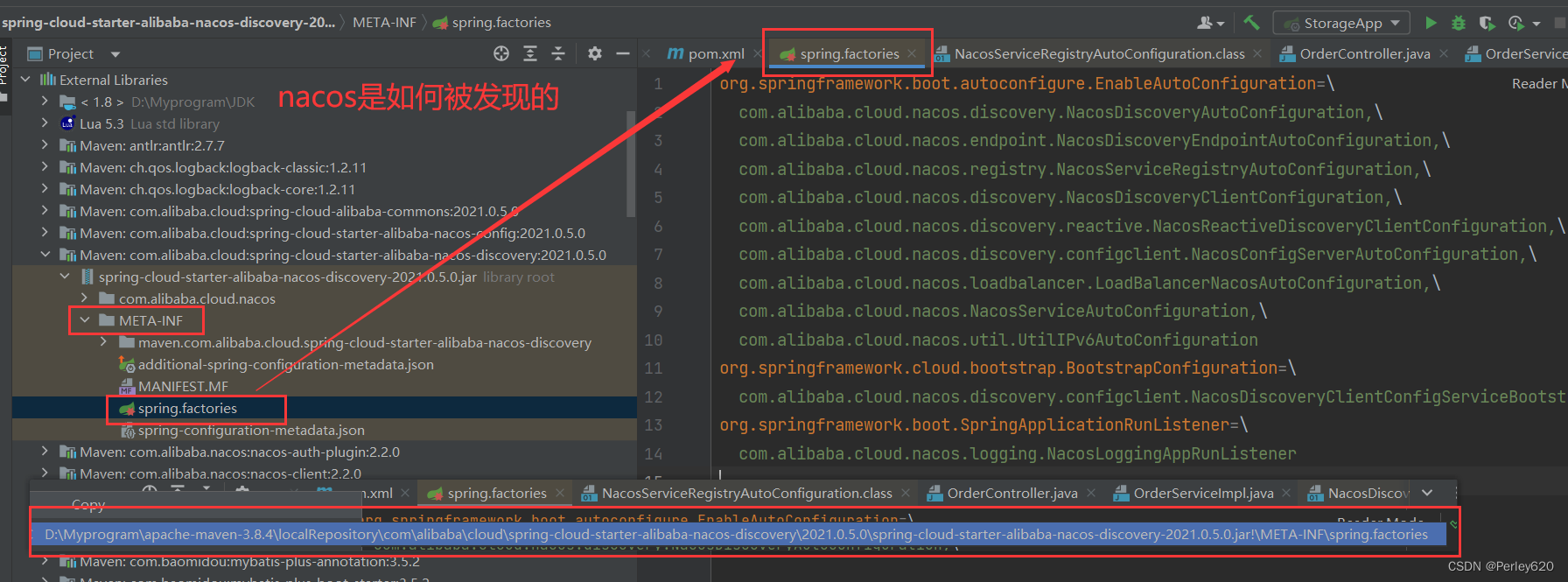
@SpringBootApplication注解的理解——如何排除自动装配 分布式情况下如何自动加载 nacos是怎么被发现的
前言 spring作为主流的 Java Web 开发的开源框架,是Java 世界最为成功的框架,持续不断深入认识spring框架是Java程序员不变的追求。 本篇博客介绍SpringBootApplicant注解的自动加载相关内容 其他相关的Spring博客文章列表如下: Spring基…...

HTTP的前世今生
史前时期 20 世纪 60 年代,美国国防部高等研究计划署(ARPA)建立了 ARPA 网,它有四个分布在各地的节点,被认为是如今互联网的“始祖”。 然后在 70 年代,基于对 ARPA 网的实践和思考,研究人员发…...

软件测试教程 自动化测试selenium篇(二)
掌握Selenium常用的API的使用 目录 一、webdriver API 1.1元素的定位 1.2 id定位 1.3name 定位 1.4tag name 定位和class name 定位 1.5CSS 定位 1.6XPath 定位 1.7link text定位 1.8Partial link text 定位 二、操作测试对象 2.1鼠标点击与键盘输入 2.2submit 提交…...
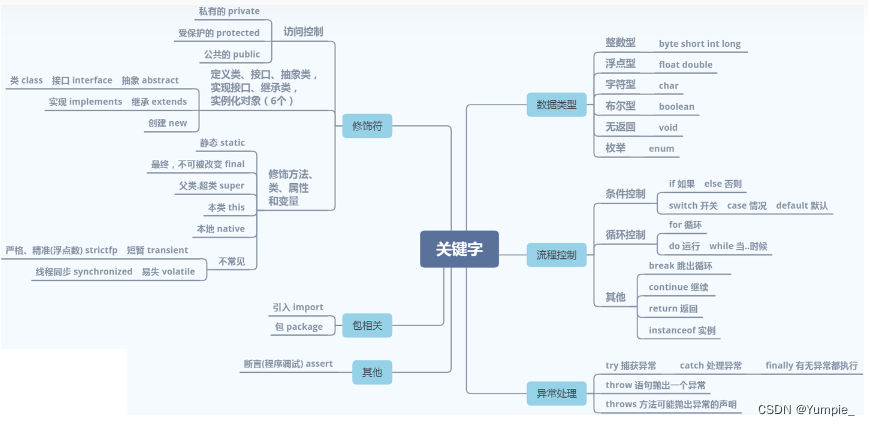
JavaSE入门--初始Java
文章目录 Java语言概述认识Java的main函数main函数示例运行Java程序认识注释认识标识符认识关键字 前言: 我从今天开始步入Java的学习,希望自己的博客可以带动小白学习,也能获得大佬的指点,日后能互相学习进步,都能如尝…...
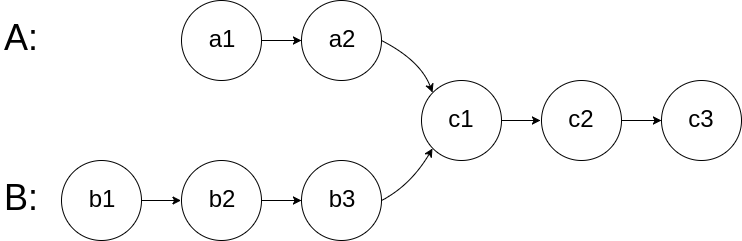
leetcode做题笔记160. 相交链表
给你两个单链表的头节点 headA 和 headB ,请你找出并返回两个单链表相交的起始节点。如果两个链表不存在相交节点,返回 null 。 图示两个链表在节点 c1 开始相交: 题目数据 保证 整个链式结构中不存在环。 注意,函数返回结果后&…...
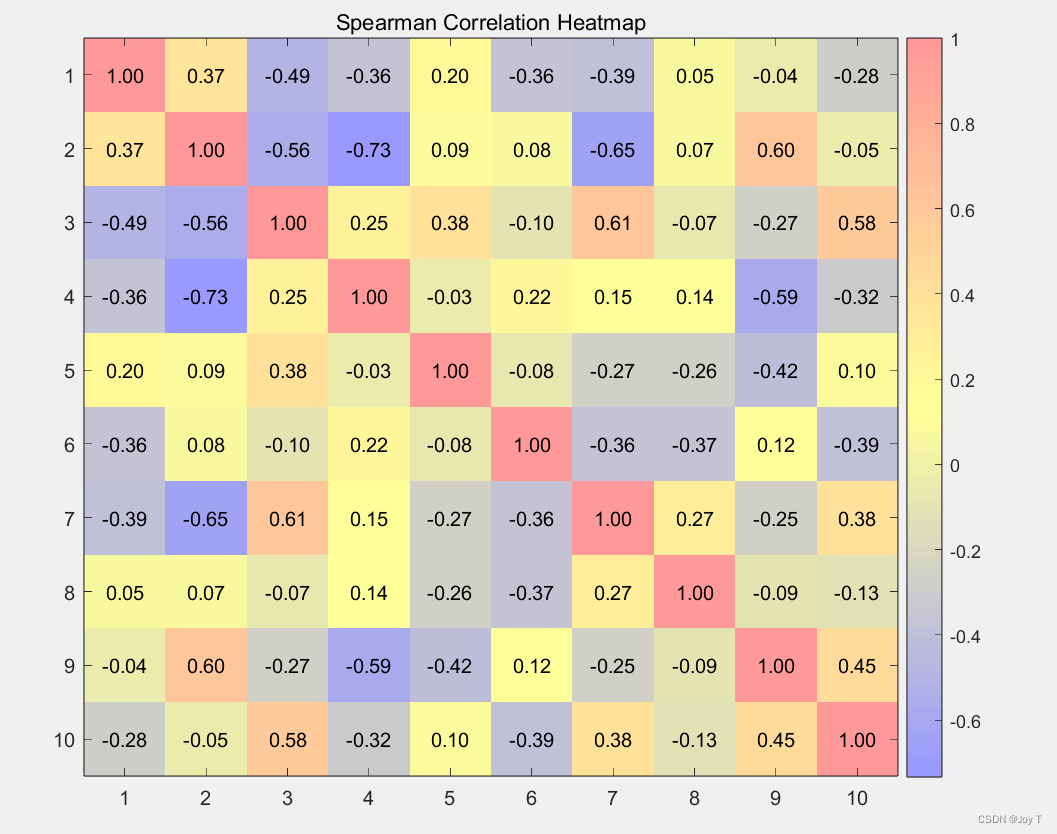
数学建模Matlab之检验与相关性分析
只要做C题基本上都会用到相关性分析、一般性检验等! 回归模型性能检验 下面讲一下回归模型的性能评估指标,用来衡量模型预测的准确性。下面是每个指标的简单解释以及它们的应用情境: 1. MAPE (平均绝对百分比误差) 描述: 衡量模型预测的相对…...

微服务网关:Spring Cloud Zuul 升级 Spring Cloud Gateway 的核心要点
1. 服务路由 1.1. Zuul 接收请求: 在routes路由规则中,根据path去匹配,如果匹配中,就使用对应的路由规则进行请求转发如果无法从routes中匹配,则根据path用“/”去截取第一段作为服务名进行请求转发,转发…...
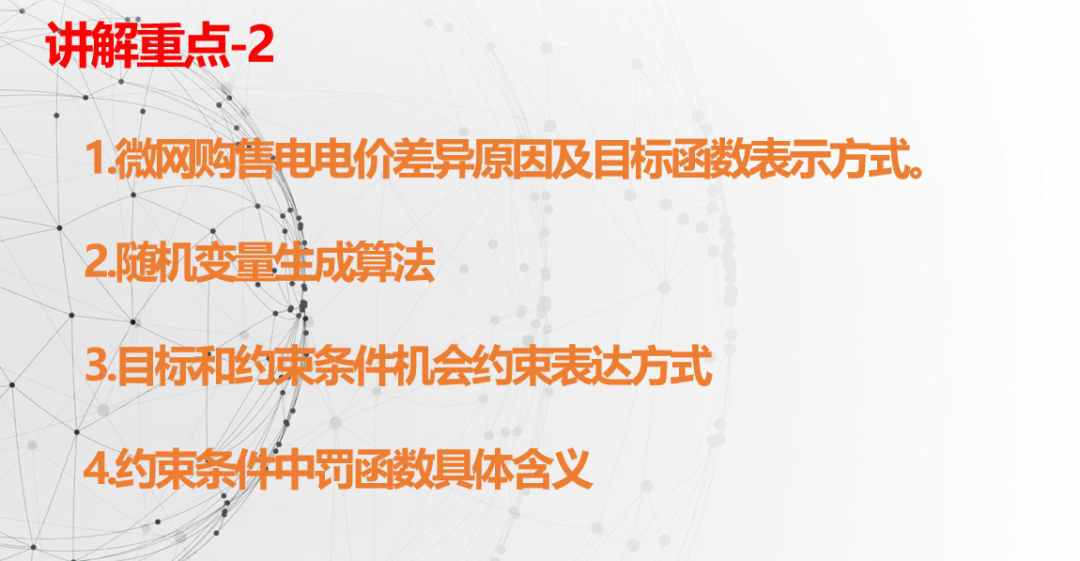
视频讲解|含可再生能源的热电联供型微网经济运行优化(含确定性和源荷随机两部分代码)
1 主要内容 该视频为《含可再生能源的热电联供型微网经济运行优化》代码讲解内容,对应的资源下载链接为考虑源荷不确定性的热电联供微网优化-王锐matlab(含视频讲解),对该程序进行了详尽的讲解,基本做到句句分析和讲解…...
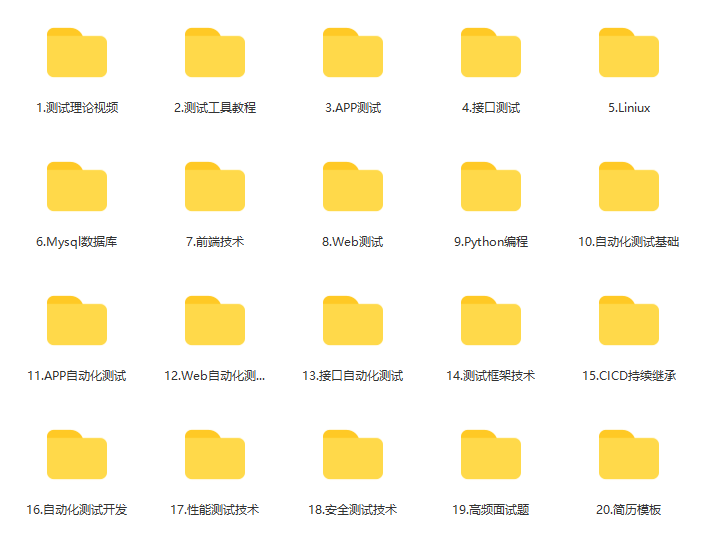
3种等待方式,让你学会Selenium设置自动化等待测试脚本!
一、Selenium脚本为什么要设置等待方式?——即他的应用背景到底是什么 应用Selenium时,浏览器加载过程中无法立即显示对应的页面元素从而无法进行元素操作,需设置一定的等待时间去等待元素的出现。(简单来说,就是设置…...
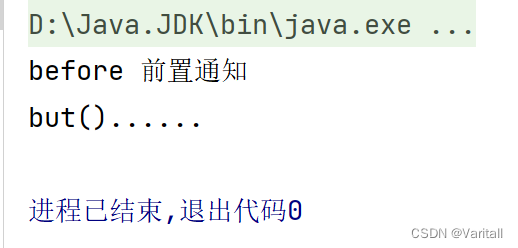
[Spring] Spring5——AOP 简介
目录 一、AOP 简介 1、什么是 AOP 二、AOP 底层原理 1、动态代理原理 2、基于接口的 JDK 动态代理 3、基于继承的 CGLib 动态代理 三、底层原理实现—— JDK 动态代理 1、使用 Proxy 类的方法创建代理对象 2、JDK 动态代理示例 四、AOP 操作术语 1、连接点 2、切入…...

C/C++ 动态规划面试算法题
1.买卖股票的最佳时机 https://blog.csdn.net/qq_41277628/article/details/113322136 输入:[7,1,5,3,6,4] 输出:5 解释:在第 2 天(股票价格 1)的时候买入,在第 5 天(股票价格 6ÿ…...

kafka伪集群部署,使用zookeeper模式
1:拉去管理kafka界面UI镜像 docker pull provectuslabs/kafka-ui2:拉去管理kafka镜像 docker pull bitnami/kafka3:docker-compose.yml version: 3.8 services:zookeeper-1:container_name: zookeeper1image: bitnami/zookeeperports:- "2181:2181"environment:- …...

Postgresql 主从复制+主从切换(流复制)
pgsql有多种主从复制方式,推荐的是流复制 一、前置条件 1.至少两个pgsql数据库(可以是一台设备上的两个) 可以参考下面的教程 pgsql编译安装:pgsql 编译安装(linux) pgsql单机多开:pgsql 单机…...

java获取字符串集合中每个字符并且组成一个新的集合实现
直接怼代码,刚好碰到了这种需求,也是想了可久,其实想想也还是挺简单的 public static void main(String[] args) { // 原始字符串集合 List<String> originalList new ArrayList<>(); originalList.add("Hello"); …...
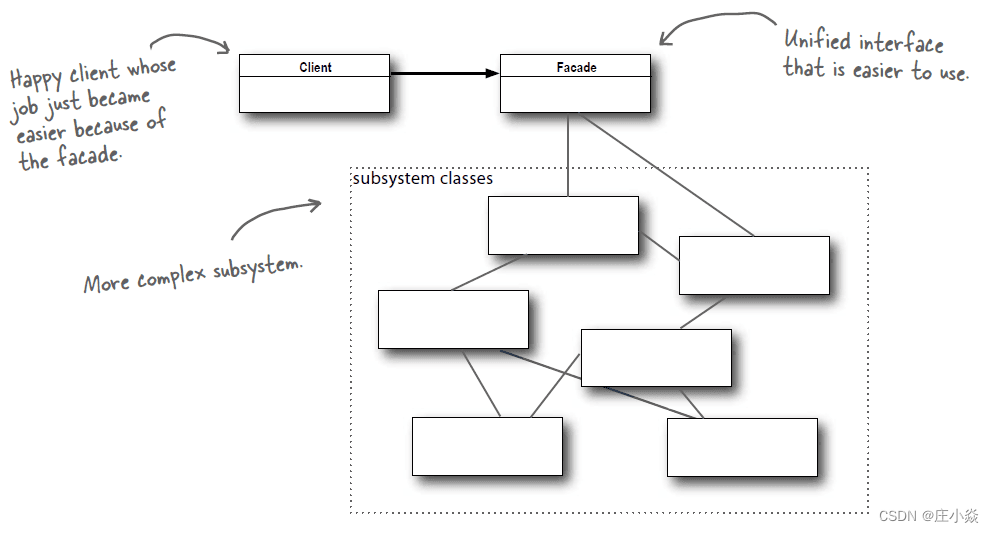
结构型设计模式——外观模式
摘要 本文主要分析设计模式 - 结构型 - 外观(Facade),它提供了一个统一的接口,用来访问子系统中的一群接口,从而让子系统更容易使用。 一、外观模式的意图 提供了一个统一的接口,用来访问子系统中的一群接口,从而让…...
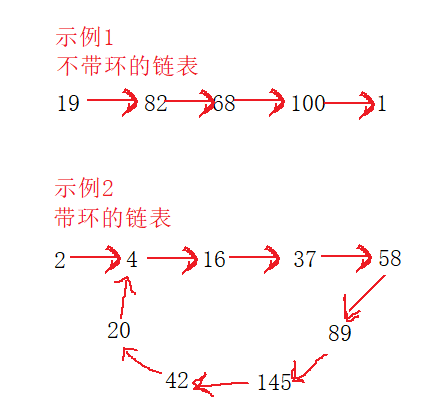
【算法学习】-【双指针】-【快乐数】
LeetCode原题链接:202. 快乐数 下面是题目描述: 「快乐数」 定义为: 对于一个正整数,每一次将该数替换为它每个位置上的数字的平方和。 然后重复这个过程直到这个数变为 1,也可能是 无限循环 但始终变不到 1。 如果…...

【Java-LangChain:使用 ChatGPT API 搭建系统-6】处理输入-链式 Prompt Chaining Prompts
第六章,处理输入-链式 Prompt Chaining Prompts 在本章中,我们将学习如何通过将复杂任务拆分为一系列简单的子任务来链接多个 Prompt。 您可能会想,为什么要将任务拆分为多个 Prompt,而不是像我们在上一个视频中学习的那样&…...
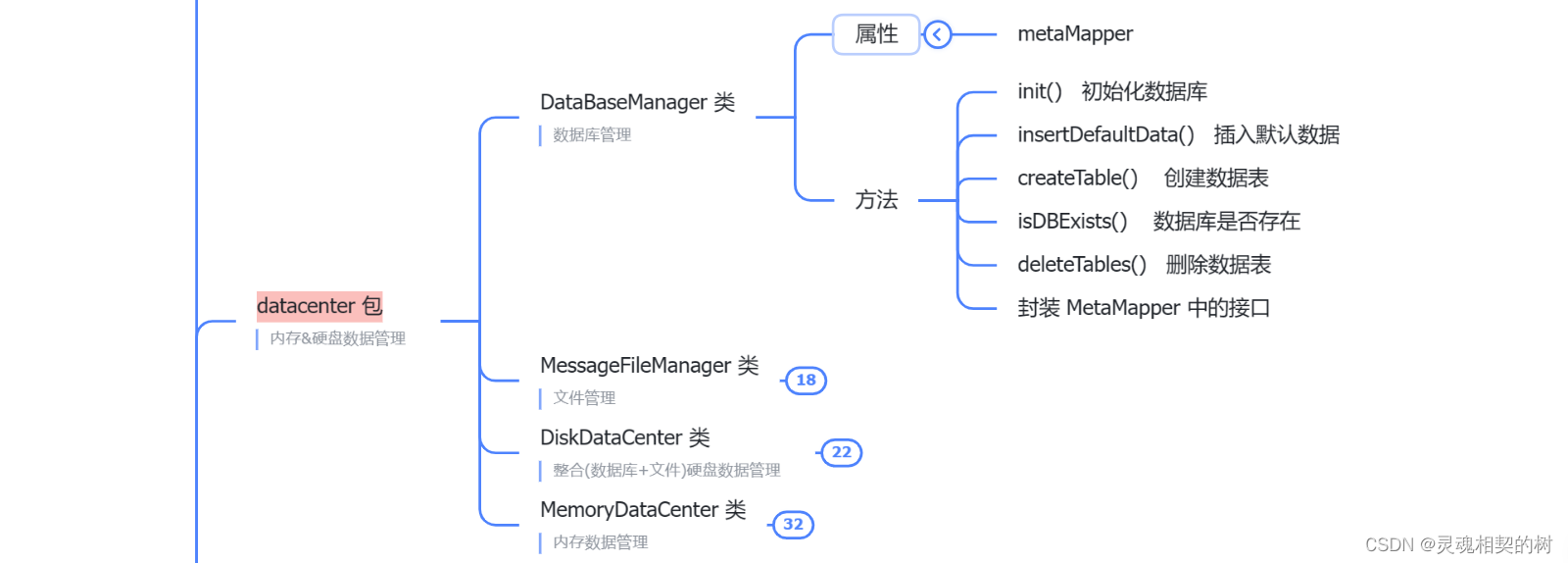
从零手搓一个【消息队列】创建核心类, 数据库设计与实现
文章目录 一、创建核心类1, 交换机2, 交换机类型3, 队列4, 绑定5, 交换机转发 & 绑定规则6, 消息7, 消息属性 二、数据库设计1, 使用 SQLite2, 使用 MyBatis2.1, 创建 Interface2.2, 创建 xml 文件 三、硬盘管理 -- 数据库1, 创建 DataBaseManager 类2, init() 初始化数据库…...