《视觉 SLAM 十四讲》第 7 讲 视觉里程计1 【如何根据图像 估计 相机运动】【特征点法】
github源码链接V2
文章目录
- 第 7 讲 视觉里程计1
- 7.1 特征点法
- 7.1.1 特征点
- 7.1.2 ORB 特征
- 7.1.3 特征匹配
- 7.2 实践 【Code】
- 本讲 CMakeLists.txt
- 7.2.1 使用 OpenCV 进行 ORB 的特征匹配 【Code】
- 7.2.2 手写 ORB 特征
- 估计 相机运动【相机位姿 估计】 3种情形 【对极几何、ICP、PnP】
- 7.3 2D-2D: 对极几何 单目相机(无距离信息)
- 7.3.2 本质矩阵 E \bm{E} E
- 7.3.3 单应矩阵(Homography)【墙、地面】
- 7.4 实践:对极约束 求解相机运动 【Code】
- 讨论!!!
- 7.5 三角测量
- 7.6 实践: 已知相机位姿,通过三角测量求特征点的空间位置 【Code】
- 7.6.2 三角测量的矛盾 : 增加平移 Yes or No
- 7.7 3D-2D: PnP (Perspective-n-Point) 【最重要】
- 7.7.1 直接线性变换(DLT)
- 7.7.2 P3P 【3对点 估计位姿】
- 7.7.3 最小化 重投影误差 求解PnP
- 7.8 实践: 求解 PnP 【Code】
- 7.9 3D-3D: ICP(Iterative Closest Point, ICP,迭代最近点) 已知两个图的深度
- 7.9.1 SVD 方法
- 7.9.2 非线性优化方法
- 7.10 使用 SVD 及 非线性优化 来求解 ICP 【Code】
- 其它
- 查看opencv 版本命令
第 7 讲 视觉里程计1
图像特征点
在单幅 图像中 提取特征点 及 多幅图像 中 匹配特征点 的方法
对极几何 恢复图像之间 的 摄像机 的三维运动
PNP ICP
后续内容: 4 个模块 (视觉里程计、后端优化、回环检测、地图构建)
什么是特征点、如何提取和匹配特征点、如何根据配对的特征点估计相机运动。
——————————
7.1 特征点法
前端【视觉里程计】
: 根据相邻图像的信息 估计 相机运动,给后端提供初值基础。
基于特征点法的前端: 对光照、动态物体不敏感。
视觉里程计 的两大类 算法: 特征点法 和 直接法。
- 如何提取、匹配图像特征点,然后估计两帧之间的相机运动和场景结构。【两帧间视觉里程计】
- 也称为
两视图几何 (Two-view geometry)
7.1.1 特征点
视觉里程计的核心: 如何根据图像 估计相机运动
有代表性的点
经典 SLAM 模型:路标
视觉SLAM:图像特征 ⟺ \iff ⟺ 路标
灰度值: 受光照、形变、物体材质影响严重,不稳定。
角点、边缘、区块
角点: 在不同图像之间 的 辨识度 更强。
2000年以前提出的特征:
更加稳定的局部图像特征:
可重复:相同的特征 可在不同图像中找到
可区别: 不同特征 表达不同
高效率: 同一图像,特征点的数量 远小于 像素数量。
本地性: 特征 仅与 一小片 图像区域相关。 【局部性?】
SIFT(尺度不变特征变换, Scale-Invariant Feature Transform)
计算量大
在一张图像中计算SIFT特征点 ⟺ \iff ⟺ 提取SIFT关键点, 并计算SIFT描述子。
关键点: 特征点的位置、朝向、大小等
描述子: 描述 该关键点 周围像素的信息。
两个特征点
的描述子
在向量空间上的距离相近
⟺ \iff ⟺ 同样的特征点
ORB(Oriented FAST and Rotated BRIEF)
: 特征子具有旋转、尺度不变性,速度提升。
质量和性能之间的折中 成本、速度、匹配效果
7.1.2 ORB 特征
ORB贡献:
FAST角点提取 计算了 特征点的方向, 为后续 BRIEF描述子 增加了旋转不变性。
FAST 关键点 ⟹ \Longrightarrow ⟹ Oriented FAST
FAST 一种角点 检测 局部像素 灰度变化 明显的地方。 速度快
只比较 像素亮度 大小
预测试: 排除绝大多数不是角点 的像素。 加速 角点 检测
因为 一般要求 16个点里 N = 12 且连续, 因此 根据 这个间隔 4 要是超过两个点,就无法满足条件了。
避免 角点集中:在一定区域内 仅保留对应极大值的角点。非极大值抑制(Non-maximal suppression)
- Code 非极大值抑制 算法
优点:速度快【仅比较像素间亮度的差异】
不足:
1、重复性不强, 分布不均匀。
2、不具有 方向信息。 ⟹ \Longrightarrow ⟹ 灰度质心法(Intensity Centroid)
3、固定取半径为 3 的圆, 存在尺度问题 远看是角点,近看不是 ⟹ \Longrightarrow ⟹ 构建图像金字塔
FAST ⟹ \Longrightarrow ⟹ ORB 中的 Oriented FAST 【尺度+旋转】
质心: 以 图像块 灰度值 作为权重的中心
BRIEF 描述子
原始的BRIEF 描述子 不具有旋转不变性,在图像发生旋转时容易丢失。
7.1.3 特征匹配
特征匹配 数据关联 当前看到的路标与之前看到的路标之间的对应关系。
- 匹配 描述子
场景中 经常存在 大量重复纹理,特征描述相似 ⟶ \longrightarrow ⟶ 误匹配
两个二进制串之间的汉明距离—— 不同位数 的个数。
快速近似最近邻 (FLANN)
适用场景:匹配点数量极多
7.2 实践 【Code】
本讲 CMakeLists.txt
CMakeLists.txt
cmake_minimum_required(VERSION 2.8)
project(vo1)set(CMAKE_CXX_STANDARD 17)
set(CMAKE_BUILD_TYPE "Release")
add_definitions("-DENABLE_SSE")
set(CMAKE_CXX_FLAGS "-std=c++14 -O2 ${SSE_FLAGS} -msse4")
list(APPEND CMAKE_MODULE_PATH ${PROJECT_SOURCE_DIR}/cmake)find_package(OpenCV 4.2.0 REQUIRED)
find_package(G2O REQUIRED)
find_package(Sophus REQUIRED)include_directories(${OpenCV_INCLUDE_DIRS}${G2O_INCLUDE_DIRS}${Sophus_INCLUDE_DIRS}"/usr/include/eigen3/"
)add_executable(orb_cv orb_cv.cpp)
target_link_libraries(orb_cv ${OpenCV_LIBS})#[[ # 块注释,用于选择 只运行 某个.cpp #[[]]
add_executable(orb_self orb_self.cpp)
target_link_libraries(orb_self ${OpenCV_LIBS})# add_executable( pose_estimation_2d2d pose_estimation_2d2d.cpp extra.cpp ) # use this if in OpenCV2
add_executable(pose_estimation_2d2d pose_estimation_2d2d.cpp)
target_link_libraries(pose_estimation_2d2d ${OpenCV_LIBS})# # add_executable( triangulation triangulation.cpp extra.cpp) # use this if in opencv2
add_executable(triangulation triangulation.cpp)
target_link_libraries(triangulation ${OpenCV_LIBS})add_executable(pose_estimation_3d2d pose_estimation_3d2d.cpp)
target_link_libraries(pose_estimation_3d2dg2o_core g2o_stuff${OpenCV_LIBS}${Sophus_LIBRARIES})add_executable(pose_estimation_3d3d pose_estimation_3d3d.cpp)
target_link_libraries(pose_estimation_3d3dg2o_core g2o_stuff${OpenCV_LIBS}${Sophus_LIBRARIES})]]
7.2.1 使用 OpenCV 进行 ORB 的特征匹配 【Code】
报错:
/home/xixi/Downloads/slambook2-master/ch7/orb_cv.cpp:16:31: error: ‘CV_LOAD_IMAGE_COLOR’ was not declared in this scope16 | Mat img_1 = imread(argv[1], CV_LOAD_IMAGE_COLOR);
mkdir build && cd build
cmake ..
make
./orb_cv ../1.png ../2.png
orb_cv.cpp
#include <iostream>
#include <opencv4/opencv2/core/core.hpp>
#include <opencv4/opencv2/features2d/features2d.hpp>
#include <opencv4/opencv2/highgui/highgui.hpp>
#include <chrono>using namespace std;
using namespace cv;int main(int argc, char **argv) {if (argc != 3) {cout << "usage: feature_extraction img1 img2" << endl;return 1;}//-- 读取图像Mat img_1 = imread(argv[1], cv::IMREAD_COLOR); //OpenCV4 需要 改这里Mat img_2 = imread(argv[2], cv::IMREAD_COLOR);assert(img_1.data != nullptr && img_2.data != nullptr);//-- 初始化std::vector<KeyPoint> keypoints_1, keypoints_2;Mat descriptors_1, descriptors_2;Ptr<FeatureDetector> detector = ORB::create();Ptr<DescriptorExtractor> descriptor = ORB::create();Ptr<DescriptorMatcher> matcher = DescriptorMatcher::create("BruteForce-Hamming");//-- 第一步:检测 Oriented FAST 角点位置chrono::steady_clock::time_point t1 = chrono::steady_clock::now();detector->detect(img_1, keypoints_1);detector->detect(img_2, keypoints_2);//-- 第二步:根据角点位置计算 BRIEF 描述子descriptor->compute(img_1, keypoints_1, descriptors_1);descriptor->compute(img_2, keypoints_2, descriptors_2);chrono::steady_clock::time_point t2 = chrono::steady_clock::now();chrono::duration<double> time_used = chrono::duration_cast<chrono::duration<double>>(t2 - t1);cout << "extract ORB cost = " << time_used.count() << " seconds. " << endl;Mat outimg1;drawKeypoints(img_1, keypoints_1, outimg1, Scalar::all(-1), DrawMatchesFlags::DEFAULT);imshow("ORB features", outimg1);//-- 第三步:对两幅图像中的BRIEF描述子进行匹配,使用 Hamming 距离vector<DMatch> matches;t1 = chrono::steady_clock::now();matcher->match(descriptors_1, descriptors_2, matches);t2 = chrono::steady_clock::now();time_used = chrono::duration_cast<chrono::duration<double>>(t2 - t1);cout << "match ORB cost = " << time_used.count() << " seconds. " << endl;//-- 第四步:匹配点对筛选// 计算最小距离和最大距离auto min_max = minmax_element(matches.begin(), matches.end(),[](const DMatch &m1, const DMatch &m2) { return m1.distance < m2.distance; });double min_dist = min_max.first->distance;double max_dist = min_max.second->distance;printf("-- Max dist : %f \n", max_dist);printf("-- Min dist : %f \n", min_dist);//当描述子之间的距离大于两倍的最小距离时,即认为匹配有误.但有时候最小距离会非常小,设置一个经验值30作为下限.std::vector<DMatch> good_matches;for (int i = 0; i < descriptors_1.rows; i++) {if (matches[i].distance <= max(2 * min_dist, 30.0)) {good_matches.push_back(matches[i]);}}//-- 第五步:绘制匹配结果Mat img_match;Mat img_goodmatch;drawMatches(img_1, keypoints_1, img_2, keypoints_2, matches, img_match);drawMatches(img_1, keypoints_1, img_2, keypoints_2, good_matches, img_goodmatch);imshow("all matches", img_match);imshow("good matches", img_goodmatch);waitKey(0);return 0;
}
去除误匹配: 汉明距离小于最小距离的 2 倍
7.2.2 手写 ORB 特征
改图片 路径
cd build
cmake ..
make
./orb_self
orb_self.cpp
//
// Created by xiang on 18-11-25.
//#include <opencv4/opencv2/opencv.hpp>
#include <string>
#include <nmmintrin.h>
#include <chrono>using namespace std;// global variables
string first_file = "../1.png"; // 要 改路径 如果 cd build 的话
string second_file = "../2.png";// 32 bit unsigned int, will have 8, 8x32=256
typedef vector<uint32_t> DescType; // Descriptor type/*** compute descriptor of orb keypoints* @param img input image* @param keypoints detected fast keypoints* @param descriptors descriptors** NOTE: if a keypoint goes outside the image boundary (8 pixels), descriptors will not be computed and will be left as* empty*/
void ComputeORB(const cv::Mat &img, vector<cv::KeyPoint> &keypoints, vector<DescType> &descriptors);/*** brute-force match two sets of descriptors* @param desc1 the first descriptor* @param desc2 the second descriptor* @param matches matches of two images*/
void BfMatch(const vector<DescType> &desc1, const vector<DescType> &desc2, vector<cv::DMatch> &matches);int main(int argc, char **argv) {// load imagecv::Mat first_image = cv::imread(first_file, 0);cv::Mat second_image = cv::imread(second_file, 0);assert(first_image.data != nullptr && second_image.data != nullptr);// detect FAST keypoints1 using threshold=40chrono::steady_clock::time_point t1 = chrono::steady_clock::now();vector<cv::KeyPoint> keypoints1;cv::FAST(first_image, keypoints1, 40);vector<DescType> descriptor1;ComputeORB(first_image, keypoints1, descriptor1);// same for the secondvector<cv::KeyPoint> keypoints2;vector<DescType> descriptor2;cv::FAST(second_image, keypoints2, 40);ComputeORB(second_image, keypoints2, descriptor2);chrono::steady_clock::time_point t2 = chrono::steady_clock::now();chrono::duration<double> time_used = chrono::duration_cast<chrono::duration<double>>(t2 - t1);cout << "extract ORB cost = " << time_used.count() << " seconds. " << endl;// find matchesvector<cv::DMatch> matches;t1 = chrono::steady_clock::now();BfMatch(descriptor1, descriptor2, matches);t2 = chrono::steady_clock::now();time_used = chrono::duration_cast<chrono::duration<double>>(t2 - t1);cout << "match ORB cost = " << time_used.count() << " seconds. " << endl;cout << "matches: " << matches.size() << endl;// plot the matchescv::Mat image_show;cv::drawMatches(first_image, keypoints1, second_image, keypoints2, matches, image_show);cv::imshow("matches", image_show);cv::imwrite("matches.png", image_show);cv::waitKey(0);cout << "done." << endl;return 0;
}// -------------------------------------------------------------------------------------------------- //
// ORB pattern
int ORB_pattern[256 * 4] = {8, -3, 9, 5/*mean (0), correlation (0)*/,4, 2, 7, -12/*mean (1.12461e-05), correlation (0.0437584)*/,-11, 9, -8, 2/*mean (3.37382e-05), correlation (0.0617409)*/,7, -12, 12, -13/*mean (5.62303e-05), correlation (0.0636977)*/,2, -13, 2, 12/*mean (0.000134953), correlation (0.085099)*/,1, -7, 1, 6/*mean (0.000528565), correlation (0.0857175)*/,-2, -10, -2, -4/*mean (0.0188821), correlation (0.0985774)*/,-13, -13, -11, -8/*mean (0.0363135), correlation (0.0899616)*/,-13, -3, -12, -9/*mean (0.121806), correlation (0.099849)*/,10, 4, 11, 9/*mean (0.122065), correlation (0.093285)*/,-13, -8, -8, -9/*mean (0.162787), correlation (0.0942748)*/,-11, 7, -9, 12/*mean (0.21561), correlation (0.0974438)*/,7, 7, 12, 6/*mean (0.160583), correlation (0.130064)*/,-4, -5, -3, 0/*mean (0.228171), correlation (0.132998)*/,-13, 2, -12, -3/*mean (0.00997526), correlation (0.145926)*/,-9, 0, -7, 5/*mean (0.198234), correlation (0.143636)*/,12, -6, 12, -1/*mean (0.0676226), correlation (0.16689)*/,-3, 6, -2, 12/*mean (0.166847), correlation (0.171682)*/,-6, -13, -4, -8/*mean (0.101215), correlation (0.179716)*/,11, -13, 12, -8/*mean (0.200641), correlation (0.192279)*/,4, 7, 5, 1/*mean (0.205106), correlation (0.186848)*/,5, -3, 10, -3/*mean (0.234908), correlation (0.192319)*/,3, -7, 6, 12/*mean (0.0709964), correlation (0.210872)*/,-8, -7, -6, -2/*mean (0.0939834), correlation (0.212589)*/,-2, 11, -1, -10/*mean (0.127778), correlation (0.20866)*/,-13, 12, -8, 10/*mean (0.14783), correlation (0.206356)*/,-7, 3, -5, -3/*mean (0.182141), correlation (0.198942)*/,-4, 2, -3, 7/*mean (0.188237), correlation (0.21384)*/,-10, -12, -6, 11/*mean (0.14865), correlation (0.23571)*/,5, -12, 6, -7/*mean (0.222312), correlation (0.23324)*/,5, -6, 7, -1/*mean (0.229082), correlation (0.23389)*/,1, 0, 4, -5/*mean (0.241577), correlation (0.215286)*/,9, 11, 11, -13/*mean (0.00338507), correlation (0.251373)*/,4, 7, 4, 12/*mean (0.131005), correlation (0.257622)*/,2, -1, 4, 4/*mean (0.152755), correlation (0.255205)*/,-4, -12, -2, 7/*mean (0.182771), correlation (0.244867)*/,-8, -5, -7, -10/*mean (0.186898), correlation (0.23901)*/,4, 11, 9, 12/*mean (0.226226), correlation (0.258255)*/,0, -8, 1, -13/*mean (0.0897886), correlation (0.274827)*/,-13, -2, -8, 2/*mean (0.148774), correlation (0.28065)*/,-3, -2, -2, 3/*mean (0.153048), correlation (0.283063)*/,-6, 9, -4, -9/*mean (0.169523), correlation (0.278248)*/,8, 12, 10, 7/*mean (0.225337), correlation (0.282851)*/,0, 9, 1, 3/*mean (0.226687), correlation (0.278734)*/,7, -5, 11, -10/*mean (0.00693882), correlation (0.305161)*/,-13, -6, -11, 0/*mean (0.0227283), correlation (0.300181)*/,10, 7, 12, 1/*mean (0.125517), correlation (0.31089)*/,-6, -3, -6, 12/*mean (0.131748), correlation (0.312779)*/,10, -9, 12, -4/*mean (0.144827), correlation (0.292797)*/,-13, 8, -8, -12/*mean (0.149202), correlation (0.308918)*/,-13, 0, -8, -4/*mean (0.160909), correlation (0.310013)*/,3, 3, 7, 8/*mean (0.177755), correlation (0.309394)*/,5, 7, 10, -7/*mean (0.212337), correlation (0.310315)*/,-1, 7, 1, -12/*mean (0.214429), correlation (0.311933)*/,3, -10, 5, 6/*mean (0.235807), correlation (0.313104)*/,2, -4, 3, -10/*mean (0.00494827), correlation (0.344948)*/,-13, 0, -13, 5/*mean (0.0549145), correlation (0.344675)*/,-13, -7, -12, 12/*mean (0.103385), correlation (0.342715)*/,-13, 3, -11, 8/*mean (0.134222), correlation (0.322922)*/,-7, 12, -4, 7/*mean (0.153284), correlation (0.337061)*/,6, -10, 12, 8/*mean (0.154881), correlation (0.329257)*/,-9, -1, -7, -6/*mean (0.200967), correlation (0.33312)*/,-2, -5, 0, 12/*mean (0.201518), correlation (0.340635)*/,-12, 5, -7, 5/*mean (0.207805), correlation (0.335631)*/,3, -10, 8, -13/*mean (0.224438), correlation (0.34504)*/,-7, -7, -4, 5/*mean (0.239361), correlation (0.338053)*/,-3, -2, -1, -7/*mean (0.240744), correlation (0.344322)*/,2, 9, 5, -11/*mean (0.242949), correlation (0.34145)*/,-11, -13, -5, -13/*mean (0.244028), correlation (0.336861)*/,-1, 6, 0, -1/*mean (0.247571), correlation (0.343684)*/,5, -3, 5, 2/*mean (0.000697256), correlation (0.357265)*/,-4, -13, -4, 12/*mean (0.00213675), correlation (0.373827)*/,-9, -6, -9, 6/*mean (0.0126856), correlation (0.373938)*/,-12, -10, -8, -4/*mean (0.0152497), correlation (0.364237)*/,10, 2, 12, -3/*mean (0.0299933), correlation (0.345292)*/,7, 12, 12, 12/*mean (0.0307242), correlation (0.366299)*/,-7, -13, -6, 5/*mean (0.0534975), correlation (0.368357)*/,-4, 9, -3, 4/*mean (0.099865), correlation (0.372276)*/,7, -1, 12, 2/*mean (0.117083), correlation (0.364529)*/,-7, 6, -5, 1/*mean (0.126125), correlation (0.369606)*/,-13, 11, -12, 5/*mean (0.130364), correlation (0.358502)*/,-3, 7, -2, -6/*mean (0.131691), correlation (0.375531)*/,7, -8, 12, -7/*mean (0.160166), correlation (0.379508)*/,-13, -7, -11, -12/*mean (0.167848), correlation (0.353343)*/,1, -3, 12, 12/*mean (0.183378), correlation (0.371916)*/,2, -6, 3, 0/*mean (0.228711), correlation (0.371761)*/,-4, 3, -2, -13/*mean (0.247211), correlation (0.364063)*/,-1, -13, 1, 9/*mean (0.249325), correlation (0.378139)*/,7, 1, 8, -6/*mean (0.000652272), correlation (0.411682)*/,1, -1, 3, 12/*mean (0.00248538), correlation (0.392988)*/,9, 1, 12, 6/*mean (0.0206815), correlation (0.386106)*/,-1, -9, -1, 3/*mean (0.0364485), correlation (0.410752)*/,-13, -13, -10, 5/*mean (0.0376068), correlation (0.398374)*/,7, 7, 10, 12/*mean (0.0424202), correlation (0.405663)*/,12, -5, 12, 9/*mean (0.0942645), correlation (0.410422)*/,6, 3, 7, 11/*mean (0.1074), correlation (0.413224)*/,5, -13, 6, 10/*mean (0.109256), correlation (0.408646)*/,2, -12, 2, 3/*mean (0.131691), correlation (0.416076)*/,3, 8, 4, -6/*mean (0.165081), correlation (0.417569)*/,2, 6, 12, -13/*mean (0.171874), correlation (0.408471)*/,9, -12, 10, 3/*mean (0.175146), correlation (0.41296)*/,-8, 4, -7, 9/*mean (0.183682), correlation (0.402956)*/,-11, 12, -4, -6/*mean (0.184672), correlation (0.416125)*/,1, 12, 2, -8/*mean (0.191487), correlation (0.386696)*/,6, -9, 7, -4/*mean (0.192668), correlation (0.394771)*/,2, 3, 3, -2/*mean (0.200157), correlation (0.408303)*/,6, 3, 11, 0/*mean (0.204588), correlation (0.411762)*/,3, -3, 8, -8/*mean (0.205904), correlation (0.416294)*/,7, 8, 9, 3/*mean (0.213237), correlation (0.409306)*/,-11, -5, -6, -4/*mean (0.243444), correlation (0.395069)*/,-10, 11, -5, 10/*mean (0.247672), correlation (0.413392)*/,-5, -8, -3, 12/*mean (0.24774), correlation (0.411416)*/,-10, 5, -9, 0/*mean (0.00213675), correlation (0.454003)*/,8, -1, 12, -6/*mean (0.0293635), correlation (0.455368)*/,4, -6, 6, -11/*mean (0.0404971), correlation (0.457393)*/,-10, 12, -8, 7/*mean (0.0481107), correlation (0.448364)*/,4, -2, 6, 7/*mean (0.050641), correlation (0.455019)*/,-2, 0, -2, 12/*mean (0.0525978), correlation (0.44338)*/,-5, -8, -5, 2/*mean (0.0629667), correlation (0.457096)*/,7, -6, 10, 12/*mean (0.0653846), correlation (0.445623)*/,-9, -13, -8, -8/*mean (0.0858749), correlation (0.449789)*/,-5, -13, -5, -2/*mean (0.122402), correlation (0.450201)*/,8, -8, 9, -13/*mean (0.125416), correlation (0.453224)*/,-9, -11, -9, 0/*mean (0.130128), correlation (0.458724)*/,1, -8, 1, -2/*mean (0.132467), correlation (0.440133)*/,7, -4, 9, 1/*mean (0.132692), correlation (0.454)*/,-2, 1, -1, -4/*mean (0.135695), correlation (0.455739)*/,11, -6, 12, -11/*mean (0.142904), correlation (0.446114)*/,-12, -9, -6, 4/*mean (0.146165), correlation (0.451473)*/,3, 7, 7, 12/*mean (0.147627), correlation (0.456643)*/,5, 5, 10, 8/*mean (0.152901), correlation (0.455036)*/,0, -4, 2, 8/*mean (0.167083), correlation (0.459315)*/,-9, 12, -5, -13/*mean (0.173234), correlation (0.454706)*/,0, 7, 2, 12/*mean (0.18312), correlation (0.433855)*/,-1, 2, 1, 7/*mean (0.185504), correlation (0.443838)*/,5, 11, 7, -9/*mean (0.185706), correlation (0.451123)*/,3, 5, 6, -8/*mean (0.188968), correlation (0.455808)*/,-13, -4, -8, 9/*mean (0.191667), correlation (0.459128)*/,-5, 9, -3, -3/*mean (0.193196), correlation (0.458364)*/,-4, -7, -3, -12/*mean (0.196536), correlation (0.455782)*/,6, 5, 8, 0/*mean (0.1972), correlation (0.450481)*/,-7, 6, -6, 12/*mean (0.199438), correlation (0.458156)*/,-13, 6, -5, -2/*mean (0.211224), correlation (0.449548)*/,1, -10, 3, 10/*mean (0.211718), correlation (0.440606)*/,4, 1, 8, -4/*mean (0.213034), correlation (0.443177)*/,-2, -2, 2, -13/*mean (0.234334), correlation (0.455304)*/,2, -12, 12, 12/*mean (0.235684), correlation (0.443436)*/,-2, -13, 0, -6/*mean (0.237674), correlation (0.452525)*/,4, 1, 9, 3/*mean (0.23962), correlation (0.444824)*/,-6, -10, -3, -5/*mean (0.248459), correlation (0.439621)*/,-3, -13, -1, 1/*mean (0.249505), correlation (0.456666)*/,7, 5, 12, -11/*mean (0.00119208), correlation (0.495466)*/,4, -2, 5, -7/*mean (0.00372245), correlation (0.484214)*/,-13, 9, -9, -5/*mean (0.00741116), correlation (0.499854)*/,7, 1, 8, 6/*mean (0.0208952), correlation (0.499773)*/,7, -8, 7, 6/*mean (0.0220085), correlation (0.501609)*/,-7, -4, -7, 1/*mean (0.0233806), correlation (0.496568)*/,-8, 11, -7, -8/*mean (0.0236505), correlation (0.489719)*/,-13, 6, -12, -8/*mean (0.0268781), correlation (0.503487)*/,2, 4, 3, 9/*mean (0.0323324), correlation (0.501938)*/,10, -5, 12, 3/*mean (0.0399235), correlation (0.494029)*/,-6, -5, -6, 7/*mean (0.0420153), correlation (0.486579)*/,8, -3, 9, -8/*mean (0.0548021), correlation (0.484237)*/,2, -12, 2, 8/*mean (0.0616622), correlation (0.496642)*/,-11, -2, -10, 3/*mean (0.0627755), correlation (0.498563)*/,-12, -13, -7, -9/*mean (0.0829622), correlation (0.495491)*/,-11, 0, -10, -5/*mean (0.0843342), correlation (0.487146)*/,5, -3, 11, 8/*mean (0.0929937), correlation (0.502315)*/,-2, -13, -1, 12/*mean (0.113327), correlation (0.48941)*/,-1, -8, 0, 9/*mean (0.132119), correlation (0.467268)*/,-13, -11, -12, -5/*mean (0.136269), correlation (0.498771)*/,-10, -2, -10, 11/*mean (0.142173), correlation (0.498714)*/,-3, 9, -2, -13/*mean (0.144141), correlation (0.491973)*/,2, -3, 3, 2/*mean (0.14892), correlation (0.500782)*/,-9, -13, -4, 0/*mean (0.150371), correlation (0.498211)*/,-4, 6, -3, -10/*mean (0.152159), correlation (0.495547)*/,-4, 12, -2, -7/*mean (0.156152), correlation (0.496925)*/,-6, -11, -4, 9/*mean (0.15749), correlation (0.499222)*/,6, -3, 6, 11/*mean (0.159211), correlation (0.503821)*/,-13, 11, -5, 5/*mean (0.162427), correlation (0.501907)*/,11, 11, 12, 6/*mean (0.16652), correlation (0.497632)*/,7, -5, 12, -2/*mean (0.169141), correlation (0.484474)*/,-1, 12, 0, 7/*mean (0.169456), correlation (0.495339)*/,-4, -8, -3, -2/*mean (0.171457), correlation (0.487251)*/,-7, 1, -6, 7/*mean (0.175), correlation (0.500024)*/,-13, -12, -8, -13/*mean (0.175866), correlation (0.497523)*/,-7, -2, -6, -8/*mean (0.178273), correlation (0.501854)*/,-8, 5, -6, -9/*mean (0.181107), correlation (0.494888)*/,-5, -1, -4, 5/*mean (0.190227), correlation (0.482557)*/,-13, 7, -8, 10/*mean (0.196739), correlation (0.496503)*/,1, 5, 5, -13/*mean (0.19973), correlation (0.499759)*/,1, 0, 10, -13/*mean (0.204465), correlation (0.49873)*/,9, 12, 10, -1/*mean (0.209334), correlation (0.49063)*/,5, -8, 10, -9/*mean (0.211134), correlation (0.503011)*/,-1, 11, 1, -13/*mean (0.212), correlation (0.499414)*/,-9, -3, -6, 2/*mean (0.212168), correlation (0.480739)*/,-1, -10, 1, 12/*mean (0.212731), correlation (0.502523)*/,-13, 1, -8, -10/*mean (0.21327), correlation (0.489786)*/,8, -11, 10, -6/*mean (0.214159), correlation (0.488246)*/,2, -13, 3, -6/*mean (0.216993), correlation (0.50287)*/,7, -13, 12, -9/*mean (0.223639), correlation (0.470502)*/,-10, -10, -5, -7/*mean (0.224089), correlation (0.500852)*/,-10, -8, -8, -13/*mean (0.228666), correlation (0.502629)*/,4, -6, 8, 5/*mean (0.22906), correlation (0.498305)*/,3, 12, 8, -13/*mean (0.233378), correlation (0.503825)*/,-4, 2, -3, -3/*mean (0.234323), correlation (0.476692)*/,5, -13, 10, -12/*mean (0.236392), correlation (0.475462)*/,4, -13, 5, -1/*mean (0.236842), correlation (0.504132)*/,-9, 9, -4, 3/*mean (0.236977), correlation (0.497739)*/,0, 3, 3, -9/*mean (0.24314), correlation (0.499398)*/,-12, 1, -6, 1/*mean (0.243297), correlation (0.489447)*/,3, 2, 4, -8/*mean (0.00155196), correlation (0.553496)*/,-10, -10, -10, 9/*mean (0.00239541), correlation (0.54297)*/,8, -13, 12, 12/*mean (0.0034413), correlation (0.544361)*/,-8, -12, -6, -5/*mean (0.003565), correlation (0.551225)*/,2, 2, 3, 7/*mean (0.00835583), correlation (0.55285)*/,10, 6, 11, -8/*mean (0.00885065), correlation (0.540913)*/,6, 8, 8, -12/*mean (0.0101552), correlation (0.551085)*/,-7, 10, -6, 5/*mean (0.0102227), correlation (0.533635)*/,-3, -9, -3, 9/*mean (0.0110211), correlation (0.543121)*/,-1, -13, -1, 5/*mean (0.0113473), correlation (0.550173)*/,-3, -7, -3, 4/*mean (0.0140913), correlation (0.554774)*/,-8, -2, -8, 3/*mean (0.017049), correlation (0.55461)*/,4, 2, 12, 12/*mean (0.01778), correlation (0.546921)*/,2, -5, 3, 11/*mean (0.0224022), correlation (0.549667)*/,6, -9, 11, -13/*mean (0.029161), correlation (0.546295)*/,3, -1, 7, 12/*mean (0.0303081), correlation (0.548599)*/,11, -1, 12, 4/*mean (0.0355151), correlation (0.523943)*/,-3, 0, -3, 6/*mean (0.0417904), correlation (0.543395)*/,4, -11, 4, 12/*mean (0.0487292), correlation (0.542818)*/,2, -4, 2, 1/*mean (0.0575124), correlation (0.554888)*/,-10, -6, -8, 1/*mean (0.0594242), correlation (0.544026)*/,-13, 7, -11, 1/*mean (0.0597391), correlation (0.550524)*/,-13, 12, -11, -13/*mean (0.0608974), correlation (0.55383)*/,6, 0, 11, -13/*mean (0.065126), correlation (0.552006)*/,0, -1, 1, 4/*mean (0.074224), correlation (0.546372)*/,-13, 3, -9, -2/*mean (0.0808592), correlation (0.554875)*/,-9, 8, -6, -3/*mean (0.0883378), correlation (0.551178)*/,-13, -6, -8, -2/*mean (0.0901035), correlation (0.548446)*/,5, -9, 8, 10/*mean (0.0949843), correlation (0.554694)*/,2, 7, 3, -9/*mean (0.0994152), correlation (0.550979)*/,-1, -6, -1, -1/*mean (0.10045), correlation (0.552714)*/,9, 5, 11, -2/*mean (0.100686), correlation (0.552594)*/,11, -3, 12, -8/*mean (0.101091), correlation (0.532394)*/,3, 0, 3, 5/*mean (0.101147), correlation (0.525576)*/,-1, 4, 0, 10/*mean (0.105263), correlation (0.531498)*/,3, -6, 4, 5/*mean (0.110785), correlation (0.540491)*/,-13, 0, -10, 5/*mean (0.112798), correlation (0.536582)*/,5, 8, 12, 11/*mean (0.114181), correlation (0.555793)*/,8, 9, 9, -6/*mean (0.117431), correlation (0.553763)*/,7, -4, 8, -12/*mean (0.118522), correlation (0.553452)*/,-10, 4, -10, 9/*mean (0.12094), correlation (0.554785)*/,7, 3, 12, 4/*mean (0.122582), correlation (0.555825)*/,9, -7, 10, -2/*mean (0.124978), correlation (0.549846)*/,7, 0, 12, -2/*mean (0.127002), correlation (0.537452)*/,-1, -6, 0, -11/*mean (0.127148), correlation (0.547401)*/
};// compute the descriptor
void ComputeORB(const cv::Mat &img, vector<cv::KeyPoint> &keypoints, vector<DescType> &descriptors) {const int half_patch_size = 8;const int half_boundary = 16;int bad_points = 0;for (auto &kp: keypoints) {if (kp.pt.x < half_boundary || kp.pt.y < half_boundary ||kp.pt.x >= img.cols - half_boundary || kp.pt.y >= img.rows - half_boundary) {// outsidebad_points++;descriptors.push_back({});continue;}float m01 = 0, m10 = 0;for (int dx = -half_patch_size; dx < half_patch_size; ++dx) {for (int dy = -half_patch_size; dy < half_patch_size; ++dy) {uchar pixel = img.at<uchar>(kp.pt.y + dy, kp.pt.x + dx);m10 += dx * pixel;m01 += dy * pixel;}}// angle should be arc tan(m01/m10);float m_sqrt = sqrt(m01 * m01 + m10 * m10) + 1e-18; // avoid divide by zerofloat sin_theta = m01 / m_sqrt;float cos_theta = m10 / m_sqrt;// compute the angle of this pointDescType desc(8, 0);for (int i = 0; i < 8; i++) {uint32_t d = 0;for (int k = 0; k < 32; k++) {int idx_pq = i * 32 + k;cv::Point2f p(ORB_pattern[idx_pq * 4], ORB_pattern[idx_pq * 4 + 1]);cv::Point2f q(ORB_pattern[idx_pq * 4 + 2], ORB_pattern[idx_pq * 4 + 3]);// rotate with thetacv::Point2f pp = cv::Point2f(cos_theta * p.x - sin_theta * p.y, sin_theta * p.x + cos_theta * p.y)+ kp.pt;cv::Point2f qq = cv::Point2f(cos_theta * q.x - sin_theta * q.y, sin_theta * q.x + cos_theta * q.y)+ kp.pt;if (img.at<uchar>(pp.y, pp.x) < img.at<uchar>(qq.y, qq.x)) {d |= 1 << k;}}desc[i] = d;}descriptors.push_back(desc);}cout << "bad/total: " << bad_points << "/" << keypoints.size() << endl;
}// brute-force matching
void BfMatch(const vector<DescType> &desc1, const vector<DescType> &desc2, vector<cv::DMatch> &matches) {const int d_max = 40;for (size_t i1 = 0; i1 < desc1.size(); ++i1) {if (desc1[i1].empty()) continue;cv::DMatch m{i1, 0, 256};for (size_t i2 = 0; i2 < desc2.size(); ++i2) {if (desc2[i2].empty()) continue;int distance = 0;for (int k = 0; k < 8; k++) {distance += _mm_popcnt_u32(desc1[i1][k] ^ desc2[i2][k]);}if (distance < d_max && distance < m.distance) {m.distance = distance;m.trainIdx = i2;}}if (m.distance < d_max) {matches.push_back(m);}}
}
估计 相机运动【相机位姿 估计】 3种情形 【对极几何、ICP、PnP】
1、相机为单目 : 根据两组2D点 估计运动 对极几何
2、相机可获得距离信息(双目、RGB-D等):两组3D点 估计运动 ICP
3、一组 3D + 一组 2D : PnP
7.3 2D-2D: 对极几何 单目相机(无距离信息)
通过 二维图像点的对应关系, 恢复两帧之间摄像机的运动。
极平面(Epipolar plane)
: O 1 , O 2 , P 三点形成的平面 O_1, O_2, P三点形成的平面 O1,O2,P三点形成的平面
- 注意 点 P P P 是 O 1 p 1 O_1p_1 O1p1 延长线 和 O 2 p 2 O_2p_2 O2p2 延长线 的交点
极点(Epipoles)
: e 1 , e 2 e_1, e_2 e1,e2 【 O 1 O 2 O_1O_2 O1O2 连线 与 像平面 I 1 , I 2 I_1,I_2 I1,I2的交点】
极线(Epipolar line)
: p 1 e 1 ( l 1 ) 、 p 2 e 2 ( l 2 ) p_1e_1(l_1)、p_2e_2(l_2) p1e1(l1)、p2e2(l2)
基线
: O 1 O 2 O_1O_2 O1O2
像平面: I 1 , I 2 I_1,I_2 I1,I2
假设 I 1 I_1 I1 中特征点 p 1 p_1 p1 匹配到 I 2 I_2 I2 中特征点 p 2 p_2 p2
本质矩阵(Essential Matrix)
E = t ∧ R \bm{E} =\bm{t}^{\land}\bm{R} E=t∧R
基础矩阵(Fundamental Matrix)
F = K − T E K − 1 \bm{F}=\bm{K}^{-T}\bm{E}\bm{K}^{-1} F=K−TEK−1
- E \bm{E} E 和 F \bm{F} F 只差了相机内参 K \bm{K} K 部分
对于归一化坐标 x 1 , x 2 \bm{x}_1, \bm{x}_2 x1,x2 : x 2 T E x 1 = 0 \bm{x}_2^T\bm{E}\bm{x}_1=0 x2TEx1=0 【本质矩阵】
对于匹配的像素坐标 p 1 , p 2 \bm{p}_1, \bm{p}_2 p1,p2 : p 2 T F p 1 = 0 \bm{p}_2^T\bm{F}\bm{p}_1=0 p2TFp1=0 【基础矩阵】
对极约束作用:
给出了两个匹配点的空间位置关系,将相机位姿估计问题变为以下两步:
1、根据配对点的像素位置 求出 E \bm{E} E 或 F \bm{F} F
2、根据 E \bm{E} E 或 F \bm{F} F 求出 R , t \bm{R,t} R,t
以 E \bm{E} E 为例,如何求解这两个问题
7.3.2 本质矩阵 E \bm{E} E
求解 E \bm{E} E:
根据已经估得的本质矩阵 E \bm{E} E, 恢复相机的运动 R , t \bm{R,t} R,t
7.3.3 单应矩阵(Homography)【墙、地面】
单应矩阵(Homography) H \bm{H} H:描述两个平面之间的映射关系。
-
运动估计 适用场景:场景中的特征点都落在同一平面上(墙、地面等)
-
无人机携带的俯视相机 或 扫地机携带的顶视相机
求解单应矩阵 H \bm{H} H:
直接线性变换法(Direct Linear Transform, DLT)
7.4 实践:对极约束 求解相机运动 【Code】
OpenCV官网相关API
报错:
/home/xixi/Downloads/slambook2-master/ch7/pose_estimation_2d2d.cpp:36:31: error: ‘CV_LOAD_IMAGE_COLOR’ was not declared in this scope36 | Mat img_1 = imread(argv[1], CV_LOAD_IMAGE_COLOR);| ^~~~~~~~~~~~~~~~~~~
/home/xixi/Downloads/slambook2-master/ch7/pose_estimation_2d2d.cpp: In function ‘void pose_estimation_2d2d(std::vector<cv::KeyPoint>, std::vector<cv::KeyPoint>, std::vector<cv::DMatch>, cv::Mat&, cv::Mat&)’:
/home/xixi/Downloads/slambook2-master/ch7/pose_estimation_2d2d.cpp:143:61: error: ‘CV_FM_8POINT’ was not declared in this scope143 | fundamental_matrix = findFundamentalMat(points1, points2, CV_FM_8POINT);| ^~~~~~~~~~~~
解决方案链接
之前 遇到了问题,改了CmakeLists.txt 很多地方,遇到了别的问题【Segmentation fault (core dumped)】,卡了挺久。重新复制原版CmakeLists.txt ,只改了OpenCV版本,CMAKE标准改成14。
cd build
cmake ..
make
./pose_estimation_2d2d ../1.png ../2.png
pose_estimation_2d2d.cpp
#include <iostream>
#include <opencv2/core/core.hpp>
#include <opencv2/features2d/features2d.hpp>
#include <opencv2/highgui/highgui.hpp>
#include <opencv2/calib3d/calib3d.hpp>
// #include "extra.h" // use this if in OpenCV2using namespace std;
using namespace cv;/***************************************************** 本程序演示了如何使用2D-2D的特征匹配估计相机运动* **************************************************/void find_feature_matches(const Mat &img_1, const Mat &img_2,std::vector<KeyPoint> &keypoints_1,std::vector<KeyPoint> &keypoints_2,std::vector<DMatch> &matches);void pose_estimation_2d2d(std::vector<KeyPoint> keypoints_1,std::vector<KeyPoint> keypoints_2,std::vector<DMatch> matches,Mat &R, Mat &t);// 像素坐标转相机归一化坐标
Point2d pixel2cam(const Point2d &p, const Mat &K);int main(int argc, char **argv) {if (argc != 3) {cout << "usage: pose_estimation_2d2d img1 img2" << endl;return 1;}//-- 读取图像Mat img_1 = imread(argv[1], IMREAD_COLOR); // OpenCV4 要改这里Mat img_2 = imread(argv[2], IMREAD_COLOR);assert(img_1.data && img_2.data && "Can not load images!");vector<KeyPoint> keypoints_1, keypoints_2;vector<DMatch> matches;find_feature_matches(img_1, img_2, keypoints_1, keypoints_2, matches);cout << "一共找到了" << matches.size() << "组匹配点" << endl;//-- 估计两张图像间运动Mat R, t;pose_estimation_2d2d(keypoints_1, keypoints_2, matches, R, t);//-- 验证E=t^R*scaleMat t_x =(Mat_<double>(3, 3) << 0, -t.at<double>(2, 0), t.at<double>(1, 0),t.at<double>(2, 0), 0, -t.at<double>(0, 0),-t.at<double>(1, 0), t.at<double>(0, 0), 0);cout << "t^R=" << endl << t_x * R << endl;//-- 验证对极约束Mat K = (Mat_<double>(3, 3) << 520.9, 0, 325.1, 0, 521.0, 249.7, 0, 0, 1);for (DMatch m: matches) {Point2d pt1 = pixel2cam(keypoints_1[m.queryIdx].pt, K);Mat y1 = (Mat_<double>(3, 1) << pt1.x, pt1.y, 1);Point2d pt2 = pixel2cam(keypoints_2[m.trainIdx].pt, K);Mat y2 = (Mat_<double>(3, 1) << pt2.x, pt2.y, 1);Mat d = y2.t() * t_x * R * y1;cout << "epipolar constraint = " << d << endl;}return 0;
}void find_feature_matches(const Mat &img_1, const Mat &img_2,std::vector<KeyPoint> &keypoints_1,std::vector<KeyPoint> &keypoints_2,std::vector<DMatch> &matches) {//-- 初始化Mat descriptors_1, descriptors_2;// used in OpenCV3Ptr<FeatureDetector> detector = ORB::create();Ptr<DescriptorExtractor> descriptor = ORB::create();// use this if you are in OpenCV2// Ptr<FeatureDetector> detector = FeatureDetector::create ( "ORB" );// Ptr<DescriptorExtractor> descriptor = DescriptorExtractor::create ( "ORB" );Ptr<DescriptorMatcher> matcher = DescriptorMatcher::create("BruteForce-Hamming");//-- 第一步:检测 Oriented FAST 角点位置detector->detect(img_1, keypoints_1);detector->detect(img_2, keypoints_2);//-- 第二步:根据角点位置计算 BRIEF 描述子descriptor->compute(img_1, keypoints_1, descriptors_1);descriptor->compute(img_2, keypoints_2, descriptors_2);//-- 第三步:对两幅图像中的BRIEF描述子进行匹配,使用 Hamming 距离vector<DMatch> match;//BFMatcher matcher ( NORM_HAMMING );matcher->match(descriptors_1, descriptors_2, match);//-- 第四步:匹配点对筛选double min_dist = 10000, max_dist = 0;//找出所有匹配之间的最小距离和最大距离, 即是最相似的和最不相似的两组点之间的距离for (int i = 0; i < descriptors_1.rows; i++) {double dist = match[i].distance;if (dist < min_dist) min_dist = dist;if (dist > max_dist) max_dist = dist;}printf("-- Max dist : %f \n", max_dist);printf("-- Min dist : %f \n", min_dist);//当描述子之间的距离大于两倍的最小距离时,即认为匹配有误.但有时候最小距离会非常小,设置一个经验值30作为下限.for (int i = 0; i < descriptors_1.rows; i++) {if (match[i].distance <= max(2 * min_dist, 30.0)) {matches.push_back(match[i]);}}
}Point2d pixel2cam(const Point2d &p, const Mat &K) {return Point2d((p.x - K.at<double>(0, 2)) / K.at<double>(0, 0),(p.y - K.at<double>(1, 2)) / K.at<double>(1, 1));
}void pose_estimation_2d2d(std::vector<KeyPoint> keypoints_1,std::vector<KeyPoint> keypoints_2,std::vector<DMatch> matches,Mat &R, Mat &t) {// 相机内参,TUM Freiburg2Mat K = (Mat_<double>(3, 3) << 520.9, 0, 325.1, 0, 521.0, 249.7, 0, 0, 1);//-- 把匹配点转换为vector<Point2f>的形式vector<Point2f> points1; vector<Point2f> points2;for (int i = 0; i < (int) matches.size(); i++) {points1.push_back(keypoints_1[matches[i].queryIdx].pt);points2.push_back(keypoints_2[matches[i].trainIdx].pt);}//-- 计算基础矩阵Mat fundamental_matrix;fundamental_matrix = findFundamentalMat(points1, points2, FM_8POINT); // OpenCV4 修改cout << "fundamental_matrix is " << endl << fundamental_matrix << endl;//-- 计算本质矩阵Point2d principal_point(325.1, 249.7); //相机光心, TUM dataset标定值double focal_length = 521; //相机焦距, TUM dataset标定值Mat essential_matrix;essential_matrix = findEssentialMat(points1, points2, focal_length, principal_point);cout << "essential_matrix is " << endl << essential_matrix << endl;//-- 计算单应矩阵//-- 但是本例中场景不是平面,单应矩阵意义不大Mat homography_matrix;homography_matrix = findHomography(points1, points2, RANSAC, 3);cout << "homography_matrix is " << endl << homography_matrix << endl;//-- 从本质矩阵中恢复旋转和平移信息.// 此函数仅在Opencv3中提供recoverPose(essential_matrix, points1, points2, R, t, focal_length, principal_point);cout << "R is " << endl << R << endl;cout << "t is " << endl << t << endl;}
讨论!!!
7.5 三角测量
在单目 SLAM 中,仅通过 单张图像 无法获得像素的深度信息,需要通过三角测量(Triangulation)(或三角化) 估计地图点的深度
三角测量
: 通过不同位置对同一路标点进行观察,从观察到的位置判断路标点的距离。
- 通过不同季节观察到的星星的角度,估计它与我们的距离。
7.6 实践: 已知相机位姿,通过三角测量求特征点的空间位置 【Code】
cd build
cmake ..
make
./triangulation ../1.png ../2.png
triangulation.cpp
#include <iostream>
#include <opencv4/opencv2/opencv.hpp>
// #include "extra.h" // used in opencv2
using namespace std;
using namespace cv;void find_feature_matches(const Mat &img_1, const Mat &img_2,std::vector<KeyPoint> &keypoints_1,std::vector<KeyPoint> &keypoints_2,std::vector<DMatch> &matches);void pose_estimation_2d2d(const std::vector<KeyPoint> &keypoints_1,const std::vector<KeyPoint> &keypoints_2,const std::vector<DMatch> &matches,Mat &R, Mat &t);void triangulation(const vector<KeyPoint> &keypoint_1,const vector<KeyPoint> &keypoint_2,const std::vector<DMatch> &matches,const Mat &R, const Mat &t,vector<Point3d> &points
);/// 作图用
inline cv::Scalar get_color(float depth) {float up_th = 50, low_th = 10, th_range = up_th - low_th;if (depth > up_th) depth = up_th;if (depth < low_th) depth = low_th;return cv::Scalar(255 * depth / th_range, 0, 255 * (1 - depth / th_range));
}// 像素坐标转相机归一化坐标
Point2f pixel2cam(const Point2d &p, const Mat &K);int main(int argc, char **argv) {if (argc != 3) {cout << "usage: triangulation img1 img2" << endl;return 1;}//-- 读取图像Mat img_1 = imread(argv[1], cv::IMREAD_COLOR); // OpenCV4 要修改 IMREAD_COLORMat img_2 = imread(argv[2], cv::IMREAD_COLOR);vector<KeyPoint> keypoints_1, keypoints_2;vector<DMatch> matches;find_feature_matches(img_1, img_2, keypoints_1, keypoints_2, matches);cout << "一共找到了" << matches.size() << "组匹配点" << endl;//-- 估计两张图像间运动Mat R, t;pose_estimation_2d2d(keypoints_1, keypoints_2, matches, R, t);//-- 三角化vector<Point3d> points;triangulation(keypoints_1, keypoints_2, matches, R, t, points);//-- 验证三角化点与特征点的重投影关系Mat K = (Mat_<double>(3, 3) << 520.9, 0, 325.1, 0, 521.0, 249.7, 0, 0, 1);Mat img1_plot = img_1.clone();Mat img2_plot = img_2.clone();for (int i = 0; i < matches.size(); i++) {// 第一个图float depth1 = points[i].z;cout << "depth: " << depth1 << endl;Point2d pt1_cam = pixel2cam(keypoints_1[matches[i].queryIdx].pt, K);cv::circle(img1_plot, keypoints_1[matches[i].queryIdx].pt, 2, get_color(depth1), 2);// 第二个图Mat pt2_trans = R * (Mat_<double>(3, 1) << points[i].x, points[i].y, points[i].z) + t;float depth2 = pt2_trans.at<double>(2, 0);cv::circle(img2_plot, keypoints_2[matches[i].trainIdx].pt, 2, get_color(depth2), 2);}cv::imshow("img 1", img1_plot);cv::imshow("img 2", img2_plot);cv::waitKey();return 0;
}void find_feature_matches(const Mat &img_1, const Mat &img_2,std::vector<KeyPoint> &keypoints_1,std::vector<KeyPoint> &keypoints_2,std::vector<DMatch> &matches) {//-- 初始化Mat descriptors_1, descriptors_2;// used in OpenCV3Ptr<FeatureDetector> detector = ORB::create();Ptr<DescriptorExtractor> descriptor = ORB::create();// use this if you are in OpenCV2// Ptr<FeatureDetector> detector = FeatureDetector::create ( "ORB" );// Ptr<DescriptorExtractor> descriptor = DescriptorExtractor::create ( "ORB" );Ptr<DescriptorMatcher> matcher = DescriptorMatcher::create("BruteForce-Hamming");//-- 第一步:检测 Oriented FAST 角点位置detector->detect(img_1, keypoints_1);detector->detect(img_2, keypoints_2);//-- 第二步:根据角点位置计算 BRIEF 描述子descriptor->compute(img_1, keypoints_1, descriptors_1);descriptor->compute(img_2, keypoints_2, descriptors_2);//-- 第三步:对两幅图像中的BRIEF描述子进行匹配,使用 Hamming 距离vector<DMatch> match;// BFMatcher matcher ( NORM_HAMMING );matcher->match(descriptors_1, descriptors_2, match);//-- 第四步:匹配点对筛选double min_dist = 10000, max_dist = 0;//找出所有匹配之间的最小距离和最大距离, 即是最相似的和最不相似的两组点之间的距离for (int i = 0; i < descriptors_1.rows; i++) {double dist = match[i].distance;if (dist < min_dist) min_dist = dist;if (dist > max_dist) max_dist = dist;}printf("-- Max dist : %f \n", max_dist);printf("-- Min dist : %f \n", min_dist);//当描述子之间的距离大于两倍的最小距离时,即认为匹配有误.但有时候最小距离会非常小,设置一个经验值30作为下限.for (int i = 0; i < descriptors_1.rows; i++) {if (match[i].distance <= max(2 * min_dist, 30.0)) {matches.push_back(match[i]);}}
}void pose_estimation_2d2d(const std::vector<KeyPoint> &keypoints_1,const std::vector<KeyPoint> &keypoints_2,const std::vector<DMatch> &matches,Mat &R, Mat &t) {// 相机内参,TUM Freiburg2Mat K = (Mat_<double>(3, 3) << 520.9, 0, 325.1, 0, 521.0, 249.7, 0, 0, 1);//-- 把匹配点转换为vector<Point2f>的形式vector<Point2f> points1;vector<Point2f> points2;for (int i = 0; i < (int) matches.size(); i++) {points1.push_back(keypoints_1[matches[i].queryIdx].pt);points2.push_back(keypoints_2[matches[i].trainIdx].pt);}//-- 计算本质矩阵Point2d principal_point(325.1, 249.7); //相机主点, TUM dataset标定值int focal_length = 521; //相机焦距, TUM dataset标定值Mat essential_matrix;essential_matrix = findEssentialMat(points1, points2, focal_length, principal_point);//-- 从本质矩阵中恢复旋转和平移信息.recoverPose(essential_matrix, points1, points2, R, t, focal_length, principal_point);
}void triangulation(const vector<KeyPoint> &keypoint_1,const vector<KeyPoint> &keypoint_2,const std::vector<DMatch> &matches,const Mat &R, const Mat &t,vector<Point3d> &points) {Mat T1 = (Mat_<float>(3, 4) <<1, 0, 0, 0,0, 1, 0, 0,0, 0, 1, 0);Mat T2 = (Mat_<float>(3, 4) <<R.at<double>(0, 0), R.at<double>(0, 1), R.at<double>(0, 2), t.at<double>(0, 0),R.at<double>(1, 0), R.at<double>(1, 1), R.at<double>(1, 2), t.at<double>(1, 0),R.at<double>(2, 0), R.at<double>(2, 1), R.at<double>(2, 2), t.at<double>(2, 0));Mat K = (Mat_<double>(3, 3) << 520.9, 0, 325.1, 0, 521.0, 249.7, 0, 0, 1);vector<Point2f> pts_1, pts_2;for (DMatch m:matches) {// 将像素坐标转换至相机坐标pts_1.push_back(pixel2cam(keypoint_1[m.queryIdx].pt, K));pts_2.push_back(pixel2cam(keypoint_2[m.trainIdx].pt, K));}Mat pts_4d;cv::triangulatePoints(T1, T2, pts_1, pts_2, pts_4d);// 转换成非齐次坐标for (int i = 0; i < pts_4d.cols; i++) {Mat x = pts_4d.col(i);x /= x.at<float>(3, 0); // 归一化Point3d p(x.at<float>(0, 0),x.at<float>(1, 0),x.at<float>(2, 0));points.push_back(p);}
}Point2f pixel2cam(const Point2d &p, const Mat &K) {return Point2f((p.x - K.at<double>(0, 2)) / K.at<double>(0, 0),(p.y - K.at<double>(1, 2)) / K.at<double>(1, 1));
}
7.6.2 三角测量的矛盾 : 增加平移 Yes or No
1、平移很小时, 像素上的不确定性 将导致 较大的深度不确定性。
- 特征点 运动一个 像素 Δ x \Delta x Δx ⟶ \longrightarrow ⟶ 视线角 变换一个角度 Δ θ \Delta \theta Δθ ⟶ \longrightarrow ⟶ 将测量到 深度值变化 Δ d \Delta d Δd
- 当 t \bm{t} t 较大时, Δ d \Delta d Δd 将明显变小。说明平移较大时,在同样的相机分辨率下,三角化测量将会更精确。
提高三角化精度的 2 种方法:
1、提高特征点的提取精度,也就是提高图像分辨率 ⟶ \longrightarrow ⟶ 图像变大,增加计算成本
2、增大平移量 ⟶ \longrightarrow ⟶ 图像外观发生明显变化,使得特征提取与匹配变得困难
三角化的矛盾 【视差(parallax)】: 增大平移,可能导致匹配失效;而平移太小,则三角化精度不够。
——————————
2D-2D 的 对极几何法 的 不足
1、需要8个或8个以上的点对
2、存在初始化、纯旋转和尺度的问题
7.7 3D-2D: PnP (Perspective-n-Point) 【最重要】
当知道 n 个 3D 空间点及其投影位置时,如何估计相机的位姿。
如果两张图像中的一张特征点的 3D 位置已知,最少需要 3 个点对(以及至少一个额外点验证结果) 即可估计相机运动。
特征点的3D位置获取方法: 三角化 或 RGB-D相机的深度图
3D-2D 方法的优点:
不需要使用对极约束,又可以在很少的匹配点获得较好的运动估计。
7.7.1 直接线性变换(DLT)
适用场景:
1、已知一组3D点的位置,以及它们在某个相机中的投影位置,求该相机的位姿。
2、给定地图和图像,求解相机状态。
3、把 3D 点看成在另一个相机坐标系中的点, 用来求解两个相机的相对运动。
7.7.2 P3P 【3对点 估计位姿】
P3P 不足:
1、只用了 3个点的信息,浪费了其它信息
2、如果3D 点 或 2D 点 受噪声影响,或存在 误匹配 ,则算法失效。
——> EPnP、UPnP
- 利用更多的信息,用迭代的方式对相机位姿进行优化,尽可能消除噪声的影响。
SLAM中的通常做法: 先使用 P3P/EPnP 等方法估计相机位姿,再构建最小二乘优化问题对估计值进行调整。
7.7.3 最小化 重投影误差 求解PnP
线性方法: 先求相机位姿,再求空间点位置
非线性优化: 把相机和三维点放在一起优化 【Bundle Adjustment】
3D 点的投影位置 与 观测位置 作差 【重投影误差】
优化特征点的空间位置:
7.8 实践: 求解 PnP 【Code】
7.8.1 使用 PnP 求解位姿
要修改的报错:
报错1:
/home/xixi/Downloads/slambook2-master/ch7/pose_estimation_3d2d.cpp:37:11: error: ‘Sophus::SE3d’ has not been declared37 | Sophus::SE3d &pose| ^~~~
/home/xixi/Downloads/slambook2-master/ch7/pose_estimation_3d2d.cpp:45:11: error: ‘Sophus::SE3d’ has not been declared45 | Sophus::SE3d &pose
代码里所有的 SE3d
去掉d
报错2:
/home/xixi/Downloads/slambook2-master/ch7/pose_estimation_3d2d.cpp:54:31: error: ‘CV_LOAD_IMAGE_COLOR’ was not declared in this scope54 | Mat img_1 = imread(argv[1], CV_LOAD_IMAGE_COLOR);| ^~~~~~~~~~~~~~~~~~~
/home/xixi/Downloads/slambook2-master/ch7/pose_estimation_3d2d.cpp:64:28: error: ‘CV_LOAD_IMAGE_UNCHANGED’ was not declared in this scope64 | Mat d1 = imread(argv[3], CV_LOAD_IMAGE_UNCHANGED); // 深度图为16位无符号数,单通道图像
opencv3 opencv4
CV_LOAD_IMAGE_UNCHANGED IMREAD_UNCHANGED
CV_LOAD_IMAGE_GRAYSCALE IMREAD_GRAYSCALE
CV_LOAD_IMAGE_COLOR IMREAD_COLOR
CV_LOAD_IMAGE_ANYDEPTH IMREAD_ANYDEPTH
报错3:
/usr/local/include/g2o/stuff/tuple_tools.h:41:46: error: ‘tuple_size_v’ is not a member of ‘std’; did you mean ‘tuple_size’?41 | f, t, i, std::make_index_sequence<std::tuple_size_v<std::decay_t<T>>>());
解决办法:
在 CMakeLists.txt
中添加 set(CMAKE_CXX_STANDARD 17)
报错4:
/home/xixi/Downloads/slambook2-master/ch7/pose_estimation_3d2d.cpp:318:10: error: ‘make_unique’ is not a member of ‘g2o’; did you mean ‘std::make_unique’?318 | g2o::make_unique<BlockSolverType>(g2o::make_unique<LinearSolverType>()));
类似第6讲,直接替换 代码块
// 构建图优化,先设定g2o typedef 别名替换/*typedef g2o::BlockSolver<g2o::BlockSolverTraits<3, 1>> BlockSolverType; // 每个误差项优化变量维度为3,误差值维度为1typedef g2o::LinearSolverDense<BlockSolverType::PoseMatrixType> LinearSolverType; // 线性求解器类型*/std::unique_ptr<g2o::BlockSolverX::LinearSolverType> linearSolver (new g2o::LinearSolverDense<g2o::BlockSolverX::PoseMatrixType>());// 梯度下降方法,可以从GN, LM, DogLeg 中选/*auto solver = new g2o::OptimizationAlgorithmGaussNewton(g2o::make_unique<BlockSolverType>(g2o::make_unique<LinearSolverType>()));*/std::unique_ptr<g2o::BlockSolverX> solver_ptr (new g2o::BlockSolverX(std::move(linearSolver)));g2o::OptimizationAlgorithmGaussNewton* solver = new g2o::OptimizationAlgorithmGaussNewton(std::move(solver_ptr));g2o::SparseOptimizer optimizer; // 图模型optimizer.setAlgorithm(solver); // 设置求解器optimizer.setVerbose(true); // 打开调试输出
报错5:
/usr/bin/ld: CMakeFiles/pose_estimation_3d2d.dir/pose_estimation_3d2d.cpp.o: in function `bundleAdjustmentGaussNewton(std::vector<Eigen::Matrix<double, 3, 1, 0, 3, 1>, Eigen::aligned_allocator<Eigen::Matrix<double, 3, 1, 0, 3, 1> > > const&, std::vector<Eigen::Matrix<double, 2, 1, 0, 2, 1>, Eigen::aligned_allocator<Eigen::Matrix<double, 2, 1, 0, 2, 1> > > const&, cv::Mat const&, Sophus::SE3&)':
pose_estimation_3d2d.cpp:(.text+0x2a4f): undefined reference to `Sophus::SE3::operator*(Eigen::Matrix<double, 3, 1, 0, 3, 1> const&) const'
/usr/bin/ld: pose_estimation_3d2d.cpp:(.text+0x3254): undefined reference to `Sophus::SE3::exp(Eigen::Matrix<double, 6, 1, 0, 6, 1> const&)'
是CMakeLists.txt 里没链接到 Sophus,加上即可
add_executable(pose_estimation_3d2d pose_estimation_3d2d.cpp)
target_link_libraries(pose_estimation_3d2dg2o_core g2o_stuff${OpenCV_LIBS}${Sophus_LIBRARIES})
cd build
cmake ..
make
./pose_estimation_3d2d ../1.png ../2.png ../1_depth.png ../2_depth.png
pose_estimation_3d2d.cpp
#include <iostream>
#include <opencv4/opencv2/core/core.hpp>
#include <opencv4/opencv2/features2d/features2d.hpp>
#include <opencv4/opencv2/highgui/highgui.hpp>
#include <opencv4/opencv2/calib3d/calib3d.hpp>
#include <Eigen/Core>
#include <g2o/core/base_vertex.h>
#include <g2o/core/base_unary_edge.h>
#include <g2o/core/sparse_optimizer.h>
#include <g2o/core/block_solver.h>
#include <g2o/core/solver.h>
#include <g2o/core/optimization_algorithm_gauss_newton.h>
#include <g2o/solvers/dense/linear_solver_dense.h>
#include <sophus/se3.h>
#include <chrono>using namespace std;
using namespace cv;void find_feature_matches(const Mat &img_1, const Mat &img_2,std::vector<KeyPoint> &keypoints_1,std::vector<KeyPoint> &keypoints_2,std::vector<DMatch> &matches);// 像素坐标转相机归一化坐标
Point2d pixel2cam(const Point2d &p, const Mat &K);// BA by g2o
typedef vector<Eigen::Vector2d, Eigen::aligned_allocator<Eigen::Vector2d>> VecVector2d;
typedef vector<Eigen::Vector3d, Eigen::aligned_allocator<Eigen::Vector3d>> VecVector3d;void bundleAdjustmentG2O(const VecVector3d &points_3d,const VecVector2d &points_2d,const Mat &K,Sophus::SE3 &pose
);// BA by gauss-newton
void bundleAdjustmentGaussNewton(const VecVector3d &points_3d,const VecVector2d &points_2d,const Mat &K,Sophus::SE3 &pose
);int main(int argc, char **argv) {if (argc != 5) {cout << "usage: pose_estimation_3d2d img1 img2 depth1 depth2" << endl;return 1;}//-- 读取图像Mat img_1 = imread(argv[1], IMREAD_COLOR);Mat img_2 = imread(argv[2], IMREAD_COLOR);assert(img_1.data && img_2.data && "Can not load images!");vector<KeyPoint> keypoints_1, keypoints_2;vector<DMatch> matches;find_feature_matches(img_1, img_2, keypoints_1, keypoints_2, matches);cout << "一共找到了" << matches.size() << "组匹配点" << endl;// 建立3D点Mat d1 = imread(argv[3], IMREAD_UNCHANGED); // 深度图为16位无符号数,单通道图像Mat K = (Mat_<double>(3, 3) << 520.9, 0, 325.1, 0, 521.0, 249.7, 0, 0, 1);vector<Point3f> pts_3d;vector<Point2f> pts_2d;for (DMatch m:matches) {ushort d = d1.ptr<unsigned short>(int(keypoints_1[m.queryIdx].pt.y))[int(keypoints_1[m.queryIdx].pt.x)];if (d == 0) // bad depthcontinue;float dd = d / 5000.0;Point2d p1 = pixel2cam(keypoints_1[m.queryIdx].pt, K);pts_3d.push_back(Point3f(p1.x * dd, p1.y * dd, dd));pts_2d.push_back(keypoints_2[m.trainIdx].pt);}cout << "3d-2d pairs: " << pts_3d.size() << endl;chrono::steady_clock::time_point t1 = chrono::steady_clock::now();Mat r, t;solvePnP(pts_3d, pts_2d, K, Mat(), r, t, false); // 调用OpenCV 的 PnP 求解,可选择EPNP,DLS等方法Mat R;cv::Rodrigues(r, R); // r为旋转向量形式,用Rodrigues公式转换为矩阵chrono::steady_clock::time_point t2 = chrono::steady_clock::now();chrono::duration<double> time_used = chrono::duration_cast<chrono::duration<double>>(t2 - t1);cout << "solve pnp in opencv cost time: " << time_used.count() << " seconds." << endl;cout << "R=" << endl << R << endl;cout << "t=" << endl << t << endl;VecVector3d pts_3d_eigen;VecVector2d pts_2d_eigen;for (size_t i = 0; i < pts_3d.size(); ++i) {pts_3d_eigen.push_back(Eigen::Vector3d(pts_3d[i].x, pts_3d[i].y, pts_3d[i].z));pts_2d_eigen.push_back(Eigen::Vector2d(pts_2d[i].x, pts_2d[i].y));}cout << "calling bundle adjustment by gauss newton" << endl;Sophus::SE3 pose_gn;t1 = chrono::steady_clock::now();bundleAdjustmentGaussNewton(pts_3d_eigen, pts_2d_eigen, K, pose_gn);t2 = chrono::steady_clock::now();time_used = chrono::duration_cast<chrono::duration<double>>(t2 - t1);cout << "solve pnp by gauss newton cost time: " << time_used.count() << " seconds." << endl;cout << "calling bundle adjustment by g2o" << endl;Sophus::SE3 pose_g2o;t1 = chrono::steady_clock::now();bundleAdjustmentG2O(pts_3d_eigen, pts_2d_eigen, K, pose_g2o);t2 = chrono::steady_clock::now();time_used = chrono::duration_cast<chrono::duration<double>>(t2 - t1);cout << "solve pnp by g2o cost time: " << time_used.count() << " seconds." << endl;return 0;
}void find_feature_matches(const Mat &img_1, const Mat &img_2,std::vector<KeyPoint> &keypoints_1,std::vector<KeyPoint> &keypoints_2,std::vector<DMatch> &matches) {//-- 初始化Mat descriptors_1, descriptors_2;// used in OpenCV3Ptr<FeatureDetector> detector = ORB::create();Ptr<DescriptorExtractor> descriptor = ORB::create();// use this if you are in OpenCV2// Ptr<FeatureDetector> detector = FeatureDetector::create ( "ORB" );// Ptr<DescriptorExtractor> descriptor = DescriptorExtractor::create ( "ORB" );Ptr<DescriptorMatcher> matcher = DescriptorMatcher::create("BruteForce-Hamming");//-- 第一步:检测 Oriented FAST 角点位置detector->detect(img_1, keypoints_1);detector->detect(img_2, keypoints_2);//-- 第二步:根据角点位置计算 BRIEF 描述子descriptor->compute(img_1, keypoints_1, descriptors_1);descriptor->compute(img_2, keypoints_2, descriptors_2);//-- 第三步:对两幅图像中的BRIEF描述子进行匹配,使用 Hamming 距离vector<DMatch> match;// BFMatcher matcher ( NORM_HAMMING );matcher->match(descriptors_1, descriptors_2, match);//-- 第四步:匹配点对筛选double min_dist = 10000, max_dist = 0;//找出所有匹配之间的最小距离和最大距离, 即是最相似的和最不相似的两组点之间的距离for (int i = 0; i < descriptors_1.rows; i++) {double dist = match[i].distance;if (dist < min_dist) min_dist = dist;if (dist > max_dist) max_dist = dist;}printf("-- Max dist : %f \n", max_dist);printf("-- Min dist : %f \n", min_dist);//当描述子之间的距离大于两倍的最小距离时,即认为匹配有误.但有时候最小距离会非常小,设置一个经验值30作为下限.for (int i = 0; i < descriptors_1.rows; i++) {if (match[i].distance <= max(2 * min_dist, 30.0)) {matches.push_back(match[i]);}}
}Point2d pixel2cam(const Point2d &p, const Mat &K) {return Point2d((p.x - K.at<double>(0, 2)) / K.at<double>(0, 0),(p.y - K.at<double>(1, 2)) / K.at<double>(1, 1));
}void bundleAdjustmentGaussNewton(const VecVector3d &points_3d,const VecVector2d &points_2d,const Mat &K,Sophus::SE3 &pose) {typedef Eigen::Matrix<double, 6, 1> Vector6d;const int iterations = 10;double cost = 0, lastCost = 0;double fx = K.at<double>(0, 0);double fy = K.at<double>(1, 1);double cx = K.at<double>(0, 2);double cy = K.at<double>(1, 2);for (int iter = 0; iter < iterations; iter++) {Eigen::Matrix<double, 6, 6> H = Eigen::Matrix<double, 6, 6>::Zero();Vector6d b = Vector6d::Zero();cost = 0;// compute costfor (int i = 0; i < points_3d.size(); i++) {Eigen::Vector3d pc = pose * points_3d[i];double inv_z = 1.0 / pc[2];double inv_z2 = inv_z * inv_z;Eigen::Vector2d proj(fx * pc[0] / pc[2] + cx, fy * pc[1] / pc[2] + cy);Eigen::Vector2d e = points_2d[i] - proj;cost += e.squaredNorm();Eigen::Matrix<double, 2, 6> J;J << -fx * inv_z,0,fx * pc[0] * inv_z2,fx * pc[0] * pc[1] * inv_z2,-fx - fx * pc[0] * pc[0] * inv_z2,fx * pc[1] * inv_z,0,-fy * inv_z,fy * pc[1] * inv_z2,fy + fy * pc[1] * pc[1] * inv_z2,-fy * pc[0] * pc[1] * inv_z2,-fy * pc[0] * inv_z;H += J.transpose() * J;b += -J.transpose() * e;}Vector6d dx;dx = H.ldlt().solve(b);if (isnan(dx[0])) {cout << "result is nan!" << endl;break;}if (iter > 0 && cost >= lastCost) {// cost increase, update is not goodcout << "cost: " << cost << ", last cost: " << lastCost << endl;break;}// update your estimationpose = Sophus::SE3::exp(dx) * pose;lastCost = cost;cout << "iteration " << iter << " cost=" << std::setprecision(12) << cost << endl;if (dx.norm() < 1e-6) {// convergebreak;}}cout << "pose by g-n: \n" << pose.matrix() << endl;
}/// vertex and edges used in g2o ba
class VertexPose : public g2o::BaseVertex<6, Sophus::SE3> {
public:EIGEN_MAKE_ALIGNED_OPERATOR_NEW;virtual void setToOriginImpl() override {_estimate = Sophus::SE3();}/// left multiplication on SE3virtual void oplusImpl(const double *update) override {Eigen::Matrix<double, 6, 1> update_eigen;update_eigen << update[0], update[1], update[2], update[3], update[4], update[5];_estimate = Sophus::SE3::exp(update_eigen) * _estimate;}virtual bool read(istream &in) override {}virtual bool write(ostream &out) const override {}
};class EdgeProjection : public g2o::BaseUnaryEdge<2, Eigen::Vector2d, VertexPose> {
public:EIGEN_MAKE_ALIGNED_OPERATOR_NEW;EdgeProjection(const Eigen::Vector3d &pos, const Eigen::Matrix3d &K) : _pos3d(pos), _K(K) {}virtual void computeError() override {const VertexPose *v = static_cast<VertexPose *> (_vertices[0]);Sophus::SE3 T = v->estimate();Eigen::Vector3d pos_pixel = _K * (T * _pos3d);pos_pixel /= pos_pixel[2];_error = _measurement - pos_pixel.head<2>();}virtual void linearizeOplus() override {const VertexPose *v = static_cast<VertexPose *> (_vertices[0]);Sophus::SE3 T = v->estimate();Eigen::Vector3d pos_cam = T * _pos3d;double fx = _K(0, 0);double fy = _K(1, 1);double cx = _K(0, 2);double cy = _K(1, 2);double X = pos_cam[0];double Y = pos_cam[1];double Z = pos_cam[2];double Z2 = Z * Z;_jacobianOplusXi<< -fx / Z, 0, fx * X / Z2, fx * X * Y / Z2, -fx - fx * X * X / Z2, fx * Y / Z,0, -fy / Z, fy * Y / (Z * Z), fy + fy * Y * Y / Z2, -fy * X * Y / Z2, -fy * X / Z;}virtual bool read(istream &in) override {}virtual bool write(ostream &out) const override {}private:Eigen::Vector3d _pos3d;Eigen::Matrix3d _K;
};void bundleAdjustmentG2O(const VecVector3d &points_3d,const VecVector2d &points_2d,const Mat &K,Sophus::SE3 &pose) {// 构建图优化,先设定g2o typedef 别名替换/*typedef g2o::BlockSolver<g2o::BlockSolverTraits<3, 1>> BlockSolverType; // 每个误差项优化变量维度为3,误差值维度为1typedef g2o::LinearSolverDense<BlockSolverType::PoseMatrixType> LinearSolverType; // 线性求解器类型*/std::unique_ptr<g2o::BlockSolverX::LinearSolverType> linearSolver (new g2o::LinearSolverDense<g2o::BlockSolverX::PoseMatrixType>());// 梯度下降方法,可以从GN, LM, DogLeg 中选/*auto solver = new g2o::OptimizationAlgorithmGaussNewton(g2o::make_unique<BlockSolverType>(g2o::make_unique<LinearSolverType>()));*/std::unique_ptr<g2o::BlockSolverX> solver_ptr (new g2o::BlockSolverX(std::move(linearSolver)));g2o::OptimizationAlgorithmGaussNewton* solver = new g2o::OptimizationAlgorithmGaussNewton(std::move(solver_ptr));g2o::SparseOptimizer optimizer; // 图模型optimizer.setAlgorithm(solver); // 设置求解器optimizer.setVerbose(true); // 打开调试输出// vertexVertexPose *vertex_pose = new VertexPose(); // camera vertex_posevertex_pose->setId(0);vertex_pose->setEstimate(Sophus::SE3());optimizer.addVertex(vertex_pose);// KEigen::Matrix3d K_eigen;K_eigen <<K.at<double>(0, 0), K.at<double>(0, 1), K.at<double>(0, 2),K.at<double>(1, 0), K.at<double>(1, 1), K.at<double>(1, 2),K.at<double>(2, 0), K.at<double>(2, 1), K.at<double>(2, 2);// edgesint index = 1;for (size_t i = 0; i < points_2d.size(); ++i) {auto p2d = points_2d[i];auto p3d = points_3d[i];EdgeProjection *edge = new EdgeProjection(p3d, K_eigen);edge->setId(index);edge->setVertex(0, vertex_pose);edge->setMeasurement(p2d);edge->setInformation(Eigen::Matrix2d::Identity());optimizer.addEdge(edge);index++;}chrono::steady_clock::time_point t1 = chrono::steady_clock::now();optimizer.setVerbose(true);optimizer.initializeOptimization();optimizer.optimize(10);chrono::steady_clock::time_point t2 = chrono::steady_clock::now();chrono::duration<double> time_used = chrono::duration_cast<chrono::duration<double>>(t2 - t1);cout << "optimization costs time: " << time_used.count() << " seconds." << endl;cout << "pose estimated by g2o =\n" << vertex_pose->estimate().matrix() << endl;pose = vertex_pose->estimate();
}
7.8.3 使用 g2o 进行 BA 优化
7.9 3D-3D: ICP(Iterative Closest Point, ICP,迭代最近点) 已知两个图的深度
迭代最近点
: 认为距离最近的两个点为同一个。
7.9.1 SVD 方法
7.9.2 非线性优化方法
7.10 使用 SVD 及 非线性优化 来求解 ICP 【Code】
7.10.1 SVD方法
通过特征匹配 获取两组 3D 点,最后用 ICP 计算 位姿变换
7.10.2 非线性优化方法
根据 7.8 节改一遍
新的报错:
/home/xixi/Downloads/slambook2-master/ch7/pose_estimation_3d3d.cpp:81:50: error: ‘Sophus::SO3d’ has not been declared81 | _jacobianOplusXi.block<3, 3>(0, 3) = Sophus::SO3d::hat(xyz_trans);
去掉d,改成 SO3
/home/xixi/Downloads/slambook2-master/ch7/pose_estimation_3d3d.cpp: In function ‘void bundleAdjustment(const std::vector<cv::Point3_<float> >&, const std::vector<cv::Point3_<float> >&, cv::Mat&, cv::Mat&)’:
/home/xixi/Downloads/slambook2-master/ch7/pose_estimation_3d3d.cpp:298:41: error: ‘const EstimateType’ {aka ‘const class Sophus::SE3’} has no member named ‘rotationMatrix’; did you mean ‘rotation_matrix’?298 | Eigen::Matrix3d R_ = pose->estimate().rotationMatrix();| ^~~~~~~~~~~~~~| rotation_matrix
按照提示改成
Eigen::Matrix3d R_ = pose->estimate().rotation_matrix();
cd build
cmake ..
make
./pose_estimation_3d3d ../1.png ../2.png ../1_depth.png ../2_depth.png
pose_estimation_3d3d.cpp
#include <iostream>
#include <opencv4/opencv2/core/core.hpp>
#include <opencv4/opencv2/features2d/features2d.hpp>
#include <opencv4/opencv2/highgui/highgui.hpp>
#include <opencv4/opencv2/calib3d/calib3d.hpp>
#include <Eigen/Core>
#include <Eigen/Dense>
#include <Eigen/Geometry>
#include <Eigen/SVD>
#include <g2o/core/base_vertex.h>
#include <g2o/core/base_unary_edge.h>
#include <g2o/core/block_solver.h>
#include <g2o/core/optimization_algorithm_gauss_newton.h>
#include <g2o/core/optimization_algorithm_levenberg.h>
#include <g2o/solvers/dense/linear_solver_dense.h>
#include <chrono>
#include <sophus/se3.h>using namespace std;
using namespace cv;void find_feature_matches(const Mat &img_1, const Mat &img_2,std::vector<KeyPoint> &keypoints_1,std::vector<KeyPoint> &keypoints_2,std::vector<DMatch> &matches);// 像素坐标转相机归一化坐标
Point2d pixel2cam(const Point2d &p, const Mat &K);void pose_estimation_3d3d(const vector<Point3f> &pts1,const vector<Point3f> &pts2,Mat &R, Mat &t
);void bundleAdjustment(const vector<Point3f> &points_3d,const vector<Point3f> &points_2d,Mat &R, Mat &t
);/// vertex and edges used in g2o ba
class VertexPose : public g2o::BaseVertex<6, Sophus::SE3> {
public:EIGEN_MAKE_ALIGNED_OPERATOR_NEW;virtual void setToOriginImpl() override {_estimate = Sophus::SE3();}/// left multiplication on SE3virtual void oplusImpl(const double *update) override {Eigen::Matrix<double, 6, 1> update_eigen;update_eigen << update[0], update[1], update[2], update[3], update[4], update[5];_estimate = Sophus::SE3::exp(update_eigen) * _estimate;}virtual bool read(istream &in) override {}virtual bool write(ostream &out) const override {}
};/// g2o edge
class EdgeProjectXYZRGBDPoseOnly : public g2o::BaseUnaryEdge<3, Eigen::Vector3d, VertexPose> {
public:EIGEN_MAKE_ALIGNED_OPERATOR_NEW;EdgeProjectXYZRGBDPoseOnly(const Eigen::Vector3d &point) : _point(point) {}virtual void computeError() override {const VertexPose *pose = static_cast<const VertexPose *> ( _vertices[0] );_error = _measurement - pose->estimate() * _point;}virtual void linearizeOplus() override {VertexPose *pose = static_cast<VertexPose *>(_vertices[0]);Sophus::SE3 T = pose->estimate();Eigen::Vector3d xyz_trans = T * _point;_jacobianOplusXi.block<3, 3>(0, 0) = -Eigen::Matrix3d::Identity();_jacobianOplusXi.block<3, 3>(0, 3) = Sophus::SO3::hat(xyz_trans);}bool read(istream &in) {}bool write(ostream &out) const {}protected:Eigen::Vector3d _point;
};int main(int argc, char **argv) {if (argc != 5) {cout << "usage: pose_estimation_3d3d img1 img2 depth1 depth2" << endl;return 1;}//-- 读取图像Mat img_1 = imread(argv[1], IMREAD_COLOR);Mat img_2 = imread(argv[2], IMREAD_COLOR);vector<KeyPoint> keypoints_1, keypoints_2;vector<DMatch> matches;find_feature_matches(img_1, img_2, keypoints_1, keypoints_2, matches);cout << "一共找到了" << matches.size() << "组匹配点" << endl;// 建立3D点Mat depth1 = imread(argv[3], IMREAD_UNCHANGED); // 深度图为16位无符号数,单通道图像Mat depth2 = imread(argv[4], IMREAD_UNCHANGED); // 深度图为16位无符号数,单通道图像Mat K = (Mat_<double>(3, 3) << 520.9, 0, 325.1, 0, 521.0, 249.7, 0, 0, 1);vector<Point3f> pts1, pts2;for (DMatch m:matches) {ushort d1 = depth1.ptr<unsigned short>(int(keypoints_1[m.queryIdx].pt.y))[int(keypoints_1[m.queryIdx].pt.x)];ushort d2 = depth2.ptr<unsigned short>(int(keypoints_2[m.trainIdx].pt.y))[int(keypoints_2[m.trainIdx].pt.x)];if (d1 == 0 || d2 == 0) // bad depthcontinue;Point2d p1 = pixel2cam(keypoints_1[m.queryIdx].pt, K);Point2d p2 = pixel2cam(keypoints_2[m.trainIdx].pt, K);float dd1 = float(d1) / 5000.0;float dd2 = float(d2) / 5000.0;pts1.push_back(Point3f(p1.x * dd1, p1.y * dd1, dd1));pts2.push_back(Point3f(p2.x * dd2, p2.y * dd2, dd2));}cout << "3d-3d pairs: " << pts1.size() << endl;Mat R, t;pose_estimation_3d3d(pts1, pts2, R, t);cout << "ICP via SVD results: " << endl;cout << "R = " << R << endl;cout << "t = " << t << endl;cout << "R_inv = " << R.t() << endl;cout << "t_inv = " << -R.t() * t << endl;cout << "calling bundle adjustment" << endl;bundleAdjustment(pts1, pts2, R, t);// verify p1 = R * p2 + tfor (int i = 0; i < 5; i++) {cout << "p1 = " << pts1[i] << endl;cout << "p2 = " << pts2[i] << endl;cout << "(R*p2+t) = " <<R * (Mat_<double>(3, 1) << pts2[i].x, pts2[i].y, pts2[i].z) + t<< endl;cout << endl;}
}void find_feature_matches(const Mat &img_1, const Mat &img_2,std::vector<KeyPoint> &keypoints_1,std::vector<KeyPoint> &keypoints_2,std::vector<DMatch> &matches) {//-- 初始化Mat descriptors_1, descriptors_2;// used in OpenCV3Ptr<FeatureDetector> detector = ORB::create();Ptr<DescriptorExtractor> descriptor = ORB::create();// use this if you are in OpenCV2// Ptr<FeatureDetector> detector = FeatureDetector::create ( "ORB" );// Ptr<DescriptorExtractor> descriptor = DescriptorExtractor::create ( "ORB" );Ptr<DescriptorMatcher> matcher = DescriptorMatcher::create("BruteForce-Hamming");//-- 第一步:检测 Oriented FAST 角点位置detector->detect(img_1, keypoints_1);detector->detect(img_2, keypoints_2);//-- 第二步:根据角点位置计算 BRIEF 描述子descriptor->compute(img_1, keypoints_1, descriptors_1);descriptor->compute(img_2, keypoints_2, descriptors_2);//-- 第三步:对两幅图像中的BRIEF描述子进行匹配,使用 Hamming 距离vector<DMatch> match;// BFMatcher matcher ( NORM_HAMMING );matcher->match(descriptors_1, descriptors_2, match);//-- 第四步:匹配点对筛选double min_dist = 10000, max_dist = 0;//找出所有匹配之间的最小距离和最大距离, 即是最相似的和最不相似的两组点之间的距离for (int i = 0; i < descriptors_1.rows; i++) {double dist = match[i].distance;if (dist < min_dist) min_dist = dist;if (dist > max_dist) max_dist = dist;}printf("-- Max dist : %f \n", max_dist);printf("-- Min dist : %f \n", min_dist);//当描述子之间的距离大于两倍的最小距离时,即认为匹配有误.但有时候最小距离会非常小,设置一个经验值30作为下限.for (int i = 0; i < descriptors_1.rows; i++) {if (match[i].distance <= max(2 * min_dist, 30.0)) {matches.push_back(match[i]);}}
}Point2d pixel2cam(const Point2d &p, const Mat &K) {return Point2d((p.x - K.at<double>(0, 2)) / K.at<double>(0, 0),(p.y - K.at<double>(1, 2)) / K.at<double>(1, 1));
}void pose_estimation_3d3d(const vector<Point3f> &pts1,const vector<Point3f> &pts2,Mat &R, Mat &t) {Point3f p1, p2; // center of massint N = pts1.size();for (int i = 0; i < N; i++) {p1 += pts1[i];p2 += pts2[i];}p1 = Point3f(Vec3f(p1) / N);p2 = Point3f(Vec3f(p2) / N);vector<Point3f> q1(N), q2(N); // remove the centerfor (int i = 0; i < N; i++) {q1[i] = pts1[i] - p1;q2[i] = pts2[i] - p2;}// compute q1*q2^TEigen::Matrix3d W = Eigen::Matrix3d::Zero();for (int i = 0; i < N; i++) {W += Eigen::Vector3d(q1[i].x, q1[i].y, q1[i].z) * Eigen::Vector3d(q2[i].x, q2[i].y, q2[i].z).transpose();}cout << "W=" << W << endl;// SVD on WEigen::JacobiSVD<Eigen::Matrix3d> svd(W, Eigen::ComputeFullU | Eigen::ComputeFullV);Eigen::Matrix3d U = svd.matrixU();Eigen::Matrix3d V = svd.matrixV();cout << "U=" << U << endl;cout << "V=" << V << endl;Eigen::Matrix3d R_ = U * (V.transpose());if (R_.determinant() < 0) {R_ = -R_;}Eigen::Vector3d t_ = Eigen::Vector3d(p1.x, p1.y, p1.z) - R_ * Eigen::Vector3d(p2.x, p2.y, p2.z);// convert to cv::MatR = (Mat_<double>(3, 3) <<R_(0, 0), R_(0, 1), R_(0, 2),R_(1, 0), R_(1, 1), R_(1, 2),R_(2, 0), R_(2, 1), R_(2, 2));t = (Mat_<double>(3, 1) << t_(0, 0), t_(1, 0), t_(2, 0));
}void bundleAdjustment(const vector<Point3f> &pts1,const vector<Point3f> &pts2,Mat &R, Mat &t) {
// 构建图优化,先设定g2o typedef 别名替换/*typedef g2o::BlockSolver<g2o::BlockSolverTraits<3, 1>> BlockSolverType; // 每个误差项优化变量维度为3,误差值维度为1typedef g2o::LinearSolverDense<BlockSolverType::PoseMatrixType> LinearSolverType; // 线性求解器类型*/std::unique_ptr<g2o::BlockSolverX::LinearSolverType> linearSolver (new g2o::LinearSolverDense<g2o::BlockSolverX::PoseMatrixType>());// 梯度下降方法,可以从GN, LM, DogLeg 中选/*auto solver = new g2o::OptimizationAlgorithmGaussNewton(g2o::make_unique<BlockSolverType>(g2o::make_unique<LinearSolverType>()));*/std::unique_ptr<g2o::BlockSolverX> solver_ptr (new g2o::BlockSolverX(std::move(linearSolver)));g2o::OptimizationAlgorithmGaussNewton* solver = new g2o::OptimizationAlgorithmGaussNewton(std::move(solver_ptr));g2o::SparseOptimizer optimizer; // 图模型optimizer.setAlgorithm(solver); // 设置求解器optimizer.setVerbose(true); // 打开调试输出// vertexVertexPose *pose = new VertexPose(); // camera posepose->setId(0);pose->setEstimate(Sophus::SE3());optimizer.addVertex(pose);// edgesfor (size_t i = 0; i < pts1.size(); i++) {EdgeProjectXYZRGBDPoseOnly *edge = new EdgeProjectXYZRGBDPoseOnly(Eigen::Vector3d(pts2[i].x, pts2[i].y, pts2[i].z));edge->setVertex(0, pose);edge->setMeasurement(Eigen::Vector3d(pts1[i].x, pts1[i].y, pts1[i].z));edge->setInformation(Eigen::Matrix3d::Identity());optimizer.addEdge(edge);}chrono::steady_clock::time_point t1 = chrono::steady_clock::now();optimizer.initializeOptimization();optimizer.optimize(10);chrono::steady_clock::time_point t2 = chrono::steady_clock::now();chrono::duration<double> time_used = chrono::duration_cast<chrono::duration<double>>(t2 - t1);cout << "optimization costs time: " << time_used.count() << " seconds." << endl;cout << endl << "after optimization:" << endl;cout << "T=\n" << pose->estimate().matrix() << endl;// convert to cv::MatEigen::Matrix3d R_ = pose->estimate().rotation_matrix();Eigen::Vector3d t_ = pose->estimate().translation();R = (Mat_<double>(3, 3) <<R_(0, 0), R_(0, 1), R_(0, 2),R_(1, 0), R_(1, 1), R_(1, 2),R_(2, 0), R_(2, 1), R_(2, 2));t = (Mat_<double>(3, 1) << t_(0, 0), t_(1, 0), t_(2, 0));
}
使用了越来越多的信息:
对极几何 | PnP | ICP |
---|---|---|
没有深度 | 一个图的深度 | 两个图的深度 |
7.11 小结
其它
查看opencv 版本命令
sudo apt update
sudo apt install libopencv-dev python3-opencv
python3 -c "import cv2; print(cv2.__version__)"
sophus安装
Ubuntu20.04安装Ceres和g2o
相关文章:
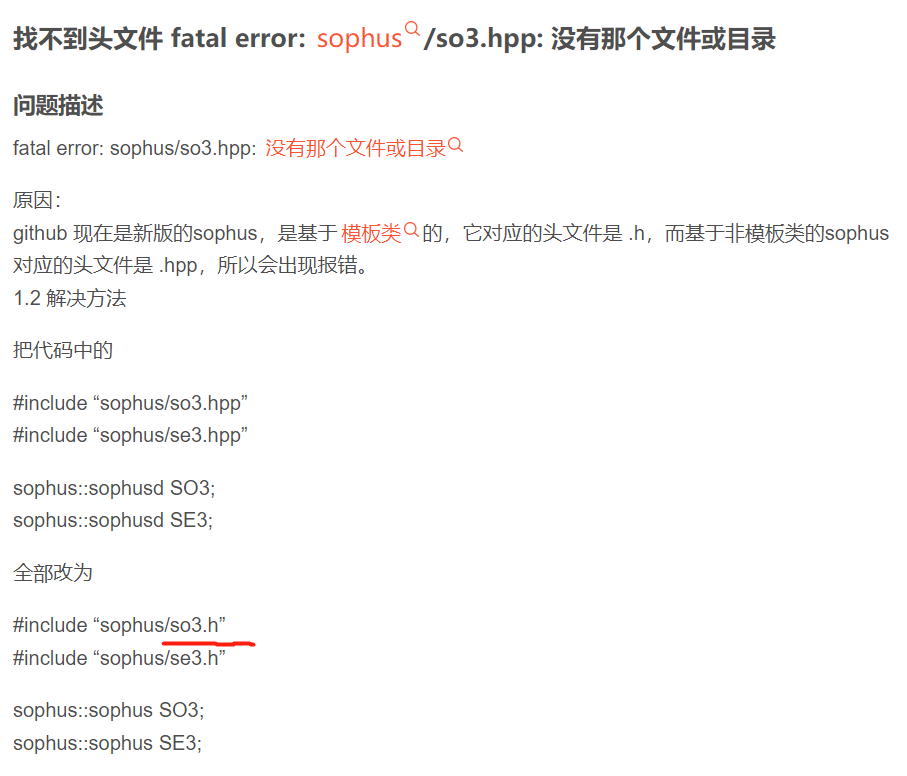
《视觉 SLAM 十四讲》第 7 讲 视觉里程计1 【如何根据图像 估计 相机运动】【特征点法】
github源码链接V2 文章目录 第 7 讲 视觉里程计17.1 特征点法7.1.1 特征点7.1.2 ORB 特征FAST 关键点 ⟹ \Longrightarrow ⟹ Oriented FASTBRIEF 描述子 7.1.3 特征匹配 7.2 实践 【Code】本讲 CMakeLists.txt 7.2.1 使用 OpenCV 进行 ORB 的特征匹配 【Code】7.2.2 手写 O…...
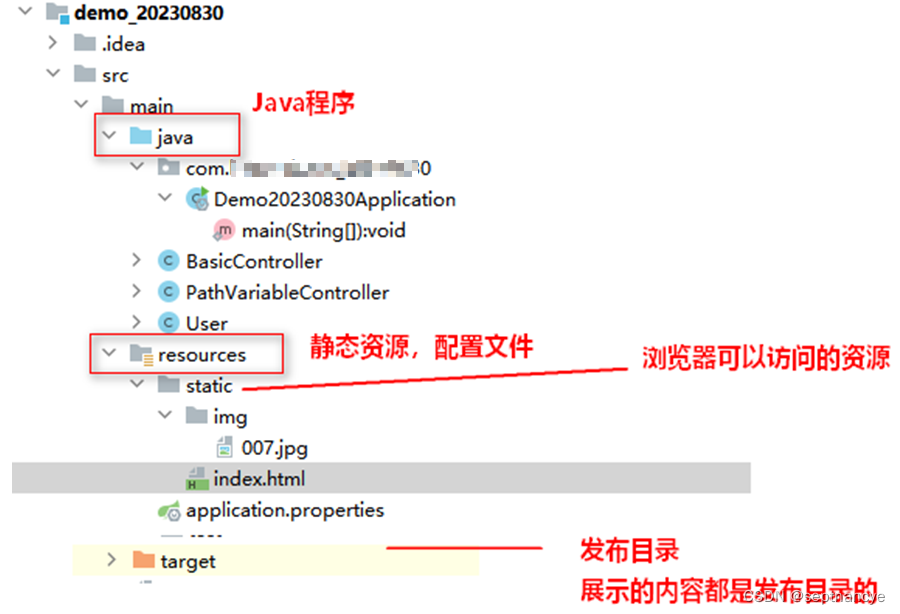
9. 一个SpringBoot项目运行
新手如何运行一个SpringBoot项目 1.SpringBoot项目运行 新创建的SpringBoot项目如何运行 2.启动lombok注解 点击该按钮,启动lombok注解支持 3.展示说明...
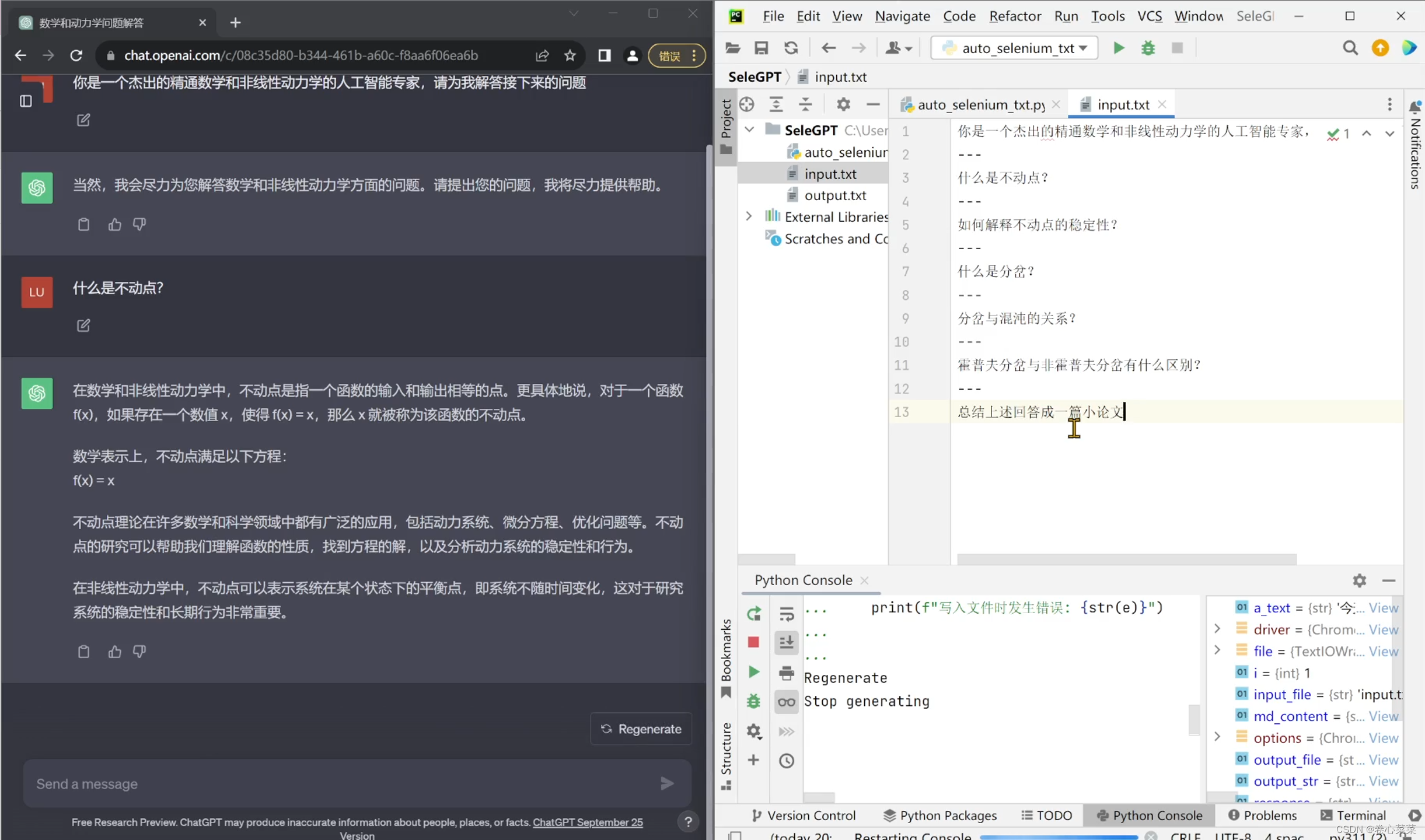
如何实现chatGPT批量问答,不用token
一、背景 因为需要批量提取一本教材里的概念做成知识图谱,想用chatGPT做概念提取。 调用api?别想了… 免费帐户的api慢得一批于是想用模仿人类交互的方法来调用,本来想用pyautogui的,但是主要是与浏览器交互,还是用s…...

Arduino驱动LIS2DH三轴加速度传感器(惯性测量传感器篇)
目录 1、传感器特性 2、硬件原理图 3、控制器和传感器连线图 4、驱动程序 LIS2DH加速度计相对传统的ADXL345在稳定性以及功耗上都有一定的优化,低功耗模式下仅为2μA(普通模式11μA),并且最高支持5.3KHz输出频率,拥有2g/4g/8g/16g四档可选量程&...

B 开组会(可持久线段树+树剖) 武汉大学2023年新生程序设计竞赛(同步赛)
其实题目就是每次询问一个节点 在这个节点的基础上往下继续遍历t的深度,在这个遍历的过程中找一个最大值就行了 其实这个题目数据非常水,直接暴力就可以过了 下面是别人过的代码 #include<bits/stdc.h> using namespace std; const int mxn5e…...

vue的axios方法
Axios是Vue.js推荐使用的一个基于Promise的HTTP库,用于浏览器和Node.js中发送HTTP请求。它可以让我们更容易地与后端进行数据交互。 以下是Axios的基本用法: 安装Axios 在Vue项目中,可以使用npm来安装Axios: npm install axio…...
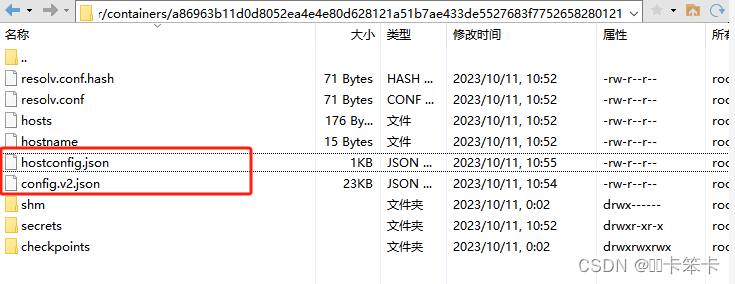
gitlab docker部署,备份,恢复。附踩坑记录
本次安装在CentOS7下进行 1、安装yum 检查是否已经安装yum yum --version如果未安装 sudo yum install -y yum-utils添加镜像源: 国外镜像源:yum-config-manager --add-repo https://download.docker.com/linux/centos/docker-ce.repo阿里镜像源&am…...
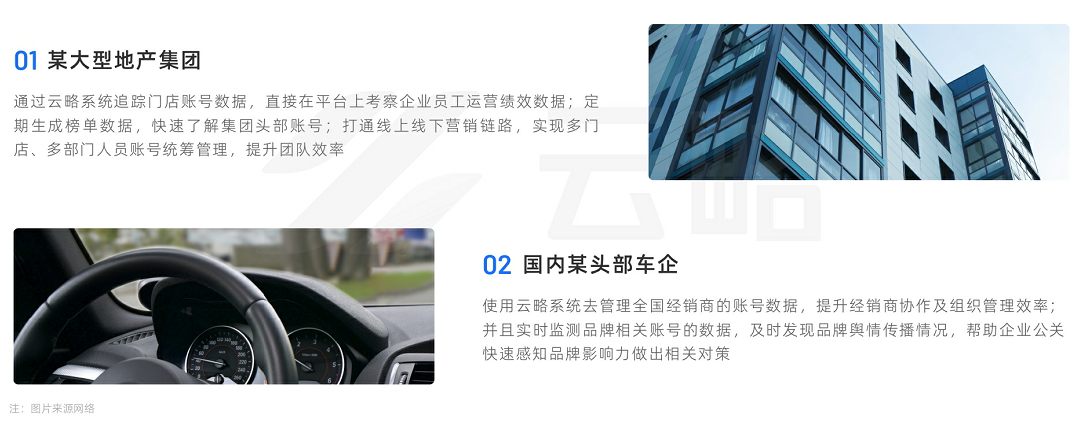
2023品牌新媒体矩阵营销洞察报告:流量内卷下,如何寻找增长新引擎?
近年来,随着移动互联网的发展渗透,短视频、直播的兴起,新消费/新零售、兴趣电商/社交电商等的驱动下,布局线上渠道已成为绝大多数品牌的必然选择。 2022年,越来越多的品牌加入到自运营、自播的行列中,并且从…...
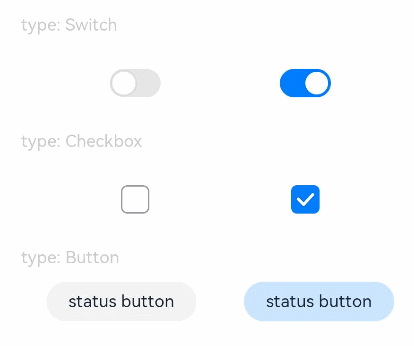
HarmonyOS/OpenHarmony原生应用-ArkTS万能卡片组件Toggle
组件提供勾选框样式、状态按钮样式及开关样式。该组件从API Version 8开始支持。 仅当ToggleType为Button时可包含子组件。 一、接口 Toggle(options: { type: ToggleType, isOn?: boolean }) 从API version 9开始,该接口支持在ArkTS卡片中使用。 参数: Toggle…...
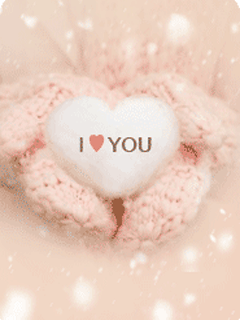
redis,mongoDB,mysql,Elasticsearch区别
Redis: Redis是一种高性能键值存储数据库,基于内存操作,支持数据持久化,支持数据类型丰富灵活,如字符串、哈希、列表、集合、有序集合等。Redis还提供了订阅/发布、事务、Lua脚本、主从同步等功能,适用于访…...

什么是软件测试架构师?
软件测试架构师是一个新职位,但确实是一个非常必要的职位,主要有几点: 1. 根据V模型、广义测试概念等,(静态)测试的越早,发现缺陷越早,越有利于产品的质量、加快产品开发周期、降低企业的成本。更重要预防…...
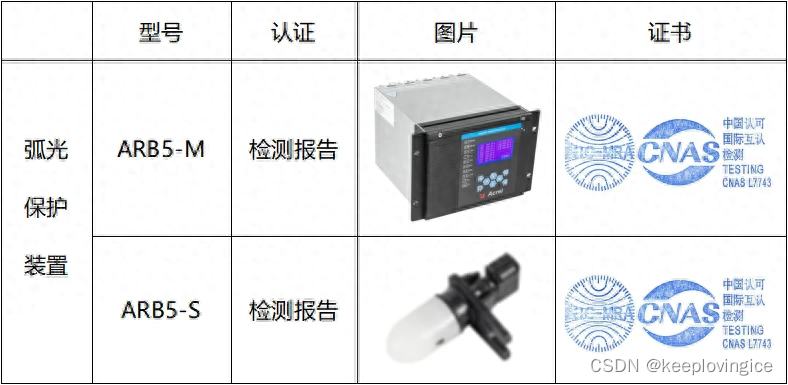
安科瑞ARB5系列弧光保护装置,智能电弧光保护,保障用电安全
安科瑞虞佳豪壹捌柒陆壹伍玖玖零玖叁 什么是弧光 电弧是放电过程中发生的一种现象,当两点之间的电压超过其工频绝缘强度极限时就会发生。当适当的条件出现时,一个携带着电流的等离子产生,直到电源侧的保护设备断开才会消失。空气在通常条件…...
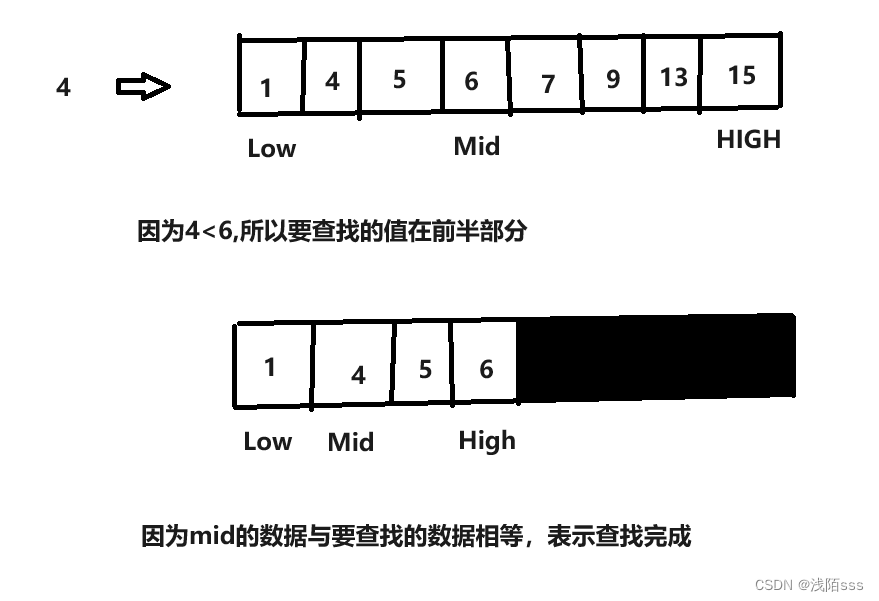
查找算法——二分查找法
一、介绍 首先需要将查找的数据排好序,再进行二分查找法来进行查找,二分查找是将数据范围不断分割为两份,不断比较中间值与待查找值的大小来确定其在哪个区间范围的一种方法。例如:在一组数据(1,4ÿ…...
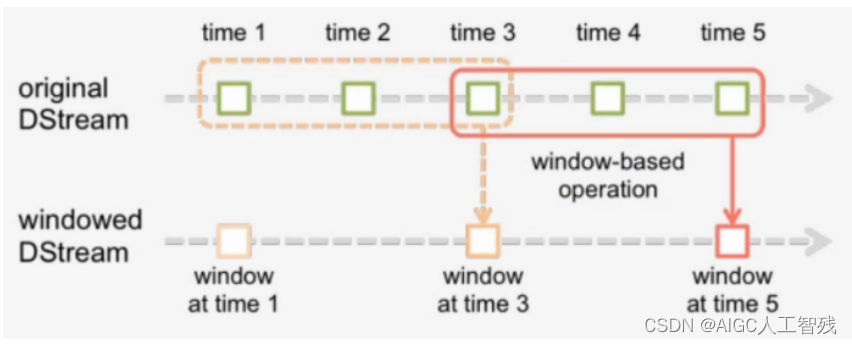
大数据——Spark Streaming
是什么 Spark Streaming是一个可扩展、高吞吐、具有容错性的流式计算框架。 之前我们接触的spark-core和spark-sql都是离线批处理任务,每天定时处理数据,对于数据的实时性要求不高,一般都是T1的。但在企业任务中存在很多的实时性的任务需求&…...
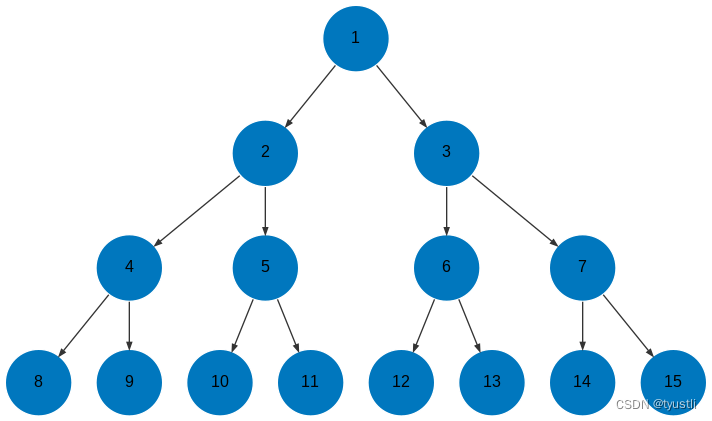
graphviz 绘制二叉树
代码 digraph BalancedBinaryTree {node [fontname"Arial", shapecircle, stylefilled, color"#ffffff", fillcolor"#0077be", fontsize12, width0.7, height0.7];edge [fontname"Arial", fontsize10, color"#333333", arr…...
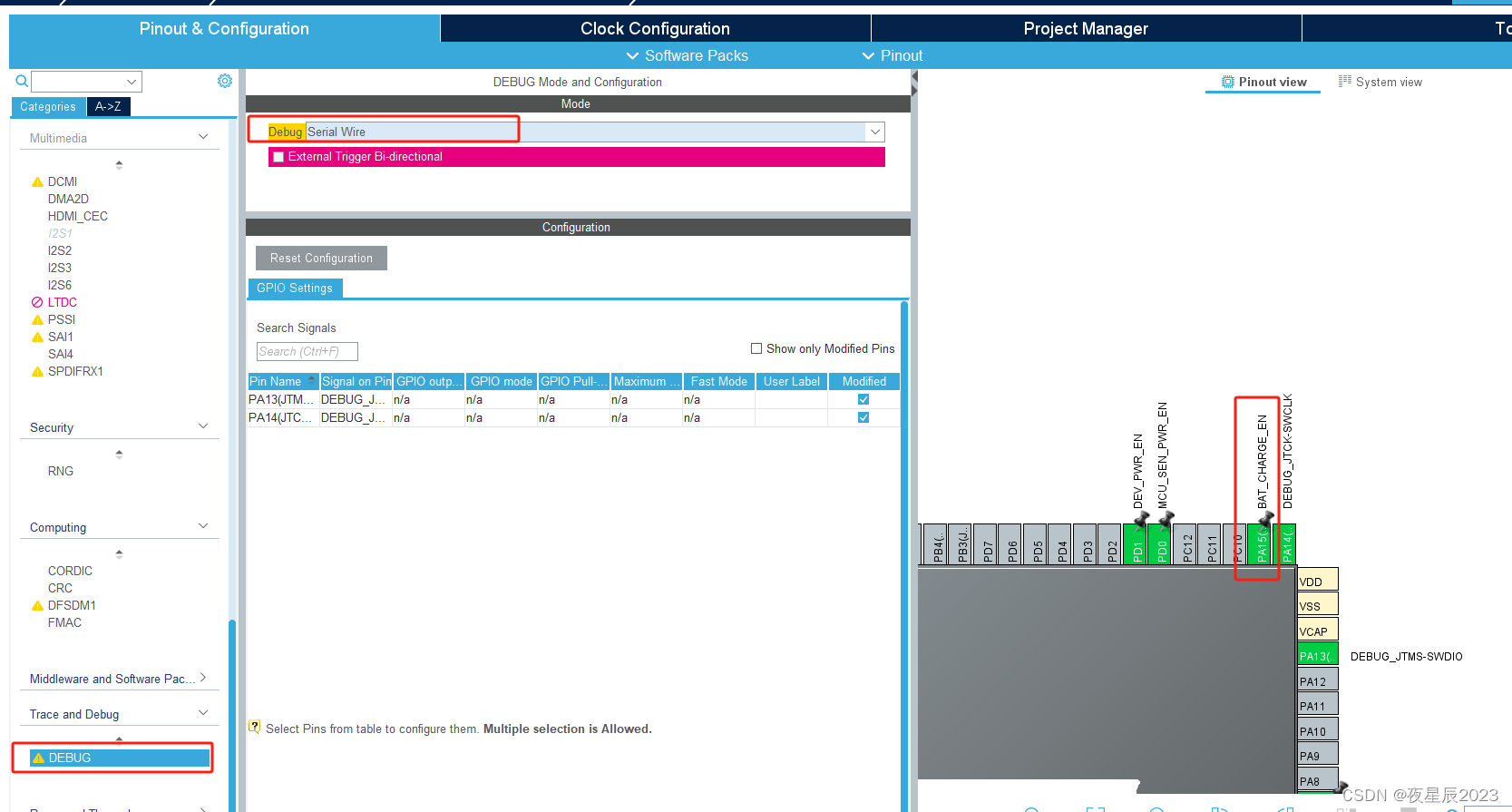
STM32 PA15/JTDI 用作普通IO,烧录口不能使用问题解决
我们一般用SW调试接口 所以DEBUG选择Serial Wire 这样PA15可以用作普通IO使用。 工程中默认加上: PA13(JTMS/SWDIO).ModeSerial_Wire PA13(JTMS/SWDIO).SignalDEBUG_JTMS-SWDIO PA14(JTCK/SWCLK).ModeSerial_Wire PA14(JTCK/SWCLK).SignalDEBUG_JTCK-SWCLK...

【ARM Coresight 系列文章 9 -- ETM 介绍 1】
文章目录 ARM Coresight ETM 介绍1.1.1 ARM Coresight ETM 版本介绍1.1.2 ARM Coresight 常见术语1.2 ARM Coresight ETM 常用寄存器介绍1.2.1 TRCVIIECTLR(ViewInst Include-Exclude Control Register)1.2.2 TRCVISSCTLR(ViewInst Start/Stop Processing Element Comparator C…...
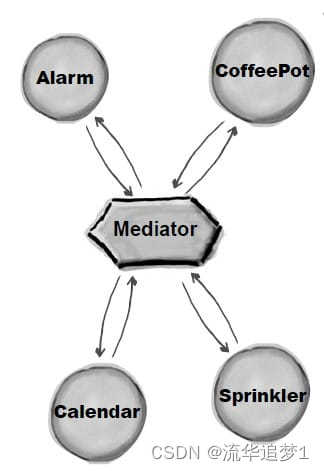
设计模式 - 中介者模式
目录 一. 前言 二. 实现 三. 优缺点 一. 前言 中介者模式又叫调停模式,定义一个中介角色来封装一系列对象之间的交互,使原有对象之间的耦合松散,且可以独立地改变它们之间的交互。 中介者模式可以使对象之间的关系数量急剧减少࿰…...
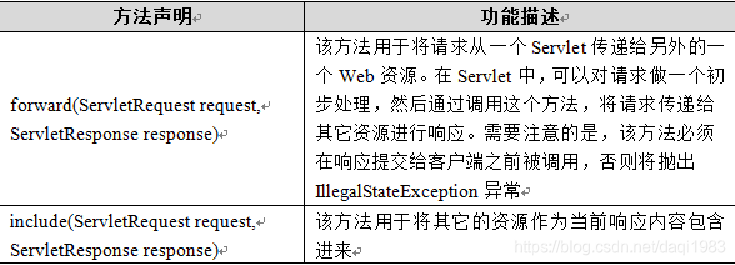
HttpServletRequest对象与RequestDispatcher对象
一、HttpServletRequest对象 1.介绍 在Servlet API中,定义了一个HttpServletRequest接口,它继承自ServletRequest接口,专门用来封装HTTP请求消息。由于HTTP请求消息分为请求行、请求消息头和请求消息体三部分,因此,在…...

Spring Boot启动流程
加载启动类:加了SpringBootApplication的启动类的main 方法中,通过运行SpringApplication.run()方法启动 【SpringBootApplication是由EnableAutoConfiguration(导入自动配置AutoConfigurationSelector类从而加载加了Configuration的配置&am…...
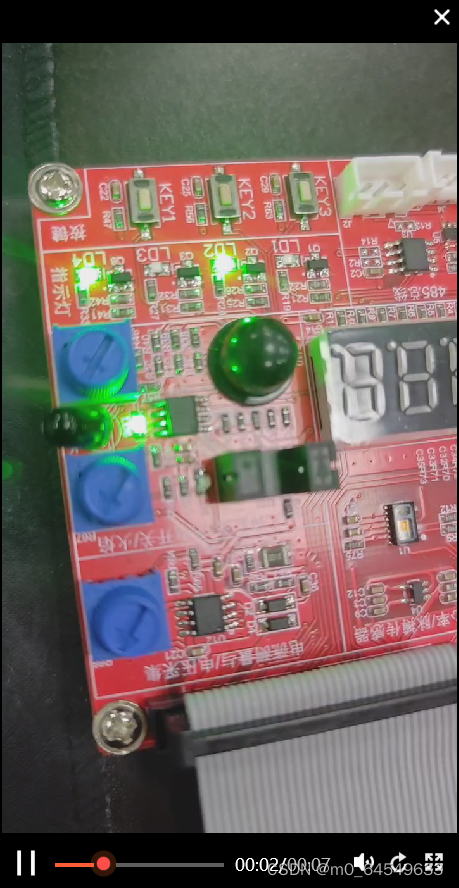
ARM day5
三盏灯流水 .text .global _start _start: 1.LDR R0,0X50000A28LDR R1,[R0]ORR R1,R1,#(0X1<<4)STR R1,[R0] 1.LDR R0,0X50000A28LDR R1,[R0]ORR R1,R1,#(0X1<<5)STR R1,[R0] 2.LDR R0,0X50006000LDR R1,[R0]BIC R1,R1,#(0X3<<20)ORR R1,R1,#(0X1<<…...
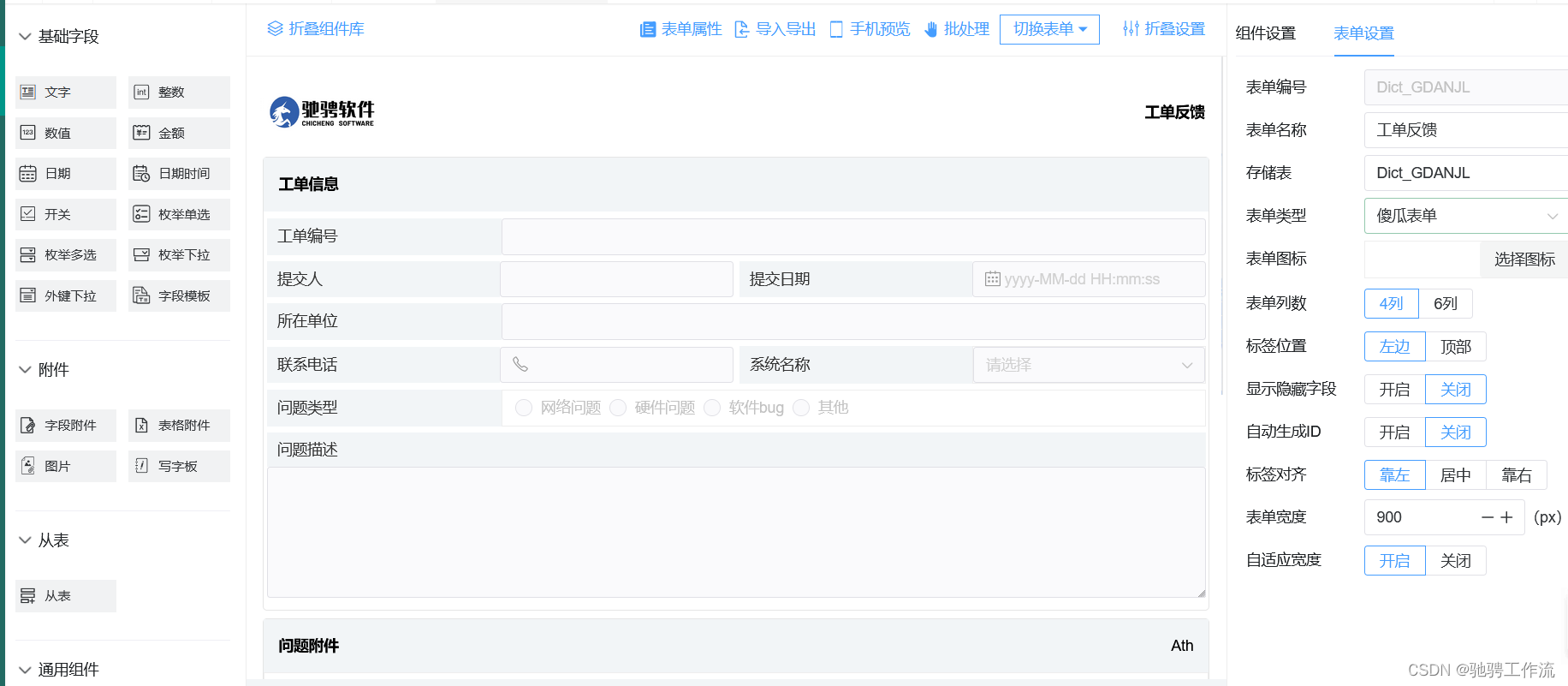
流程引擎概述及组成
流程引擎概述及组成 一、流程引擎概述 流程,可以理解为步骤,一个有序的活动或动作; 引擎,可以理解为驱动,是一个程序或者一套系统。 所以,字面意思可以理解为,流程引擎是一套(或…...

定时任务Apscheduler实践案例
定时任务Apscheduler实践案例 参考文章 https://blog.csdn.net/weixin_44799217/article/details/127353134 实现案例 本案例是使用定时任务apscheduler实现的每个三分钟发送一次邮件的任务 实现代码 import time from apscheduler.schedulers.blocking import BlockingSched…...

C#学习系列相关之多线程(五)----线程池ThreadPool用法
一、线程池的作用 线程池是一种多线程处理形式,处理过程中将任务添加到队列,然后在创建线程后自动启动这些任务。线程池线程都是后台线程。每个线程都使用默认堆栈大小,以默认的优先级运行,并处于多线程单元中。如果某个线程在托管…...
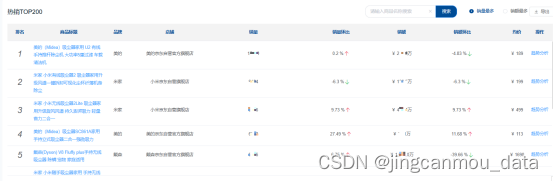
京东数据接口|电商运营中数据分析的重要性
在电商运营中,数据分析是非常重要的一环,它可以帮助电商企业更好地了解市场、了解消费者、了解产品、了解销售渠道等各种信息,从而制定更为科学有效的运营策略,提高销售效益。 数据方面用户可以直接选择使用数据接口来获取&#…...

C++入门(1)
目录 1.C关键字2.命名空间(namespace)2.1是什么2.2为什么2.3怎么用 3.C输入&输出4.缺省函数概念分类 5.函数重载6.引用6.1概念6.2特性6.3使用场景6.4引用和指针的不同点 1.C关键字 C总共有63个关键字 这里入门不多说,有需要的自行去了解 2.命名空间(namespac…...

redis-6.2.7 集群安装3主3从
因为资源有限准备了3 台 服务器,先查看防火墙的端口是否开放,如果没有开放先开放端口我使用的 6379 和 6380 这两个端口 所以将这两个端口放开。去redis 官网下载redis 安装包。下载地址 : redis 安装包下载 3. 安装redis 上传上去之后 3 台…...

【动态库】Ubuntu 添加动态库的搜索路径
在运行程序时,经常遇到下面这种动态库加载失败的情况,这时往往是系统在动态库的搜索路径下没有找到对应的库文件导致的。 目录 一、使用 LD_LIBRARY_PATH 二、修改 /etc/ld.so.conf 一、使用 LD_LIBRARY_PATH 环境变量 LD_LIBRARY_PATH是动态库的搜索…...
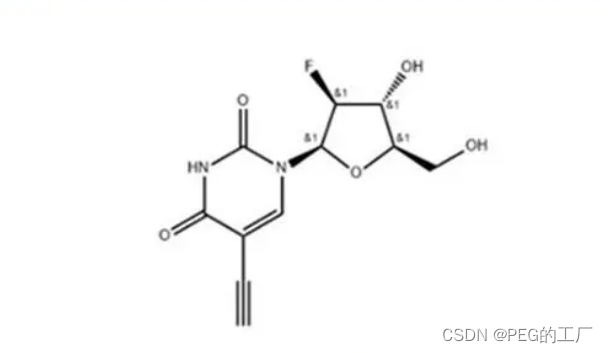
95740-26-4|用于体内DNA合成的探针F-ara-EdU
产品简介:(2S)-2-Deoxy-2-fluoro-5-ethynyluridine,一种用于体内DNA合成的探针,其毒性比EdU和BrdU都小。当需要延长细胞存活时间和不受干扰的细胞周期进展时,非常适合进行代谢DNA标记。 CAS号:95740-26-4 分子式&…...
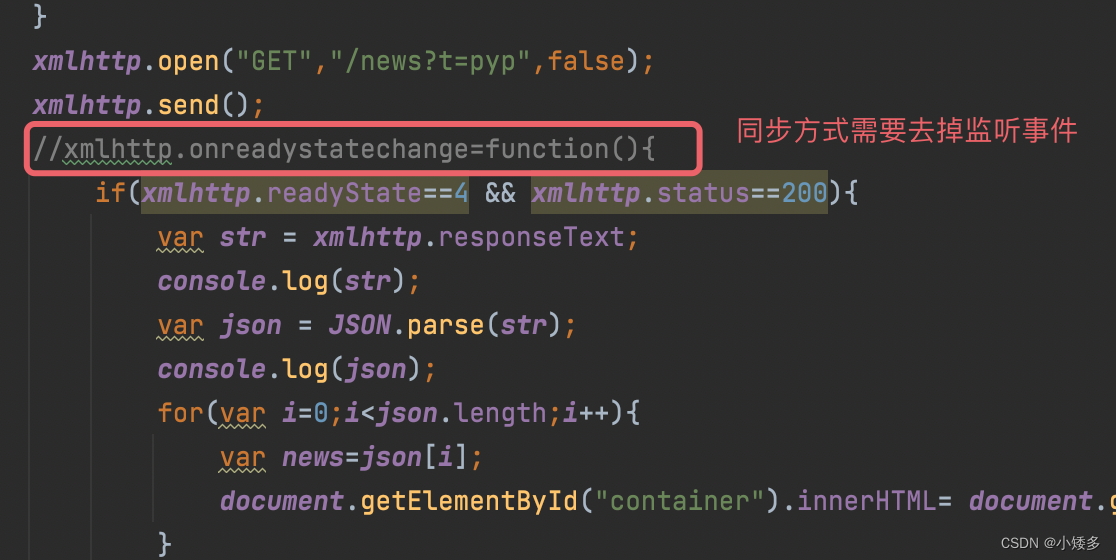
Ajax使用流程
Ajax在不刷新页面的情况下,进行页面局部更新。 Ajax使用流程: 创建XmlHttpReqeust对象发送Ajax请求处理服务器响应 1. 创建XmlHttpReqeust对象 XmlHttpReqeust对象是Ajax的核心,使用该对象发起请求,接收响应 不同的浏览器创建…...