webassembly003 TTS BARK.CPP
TTS task
- TTS(Text-to-Speech)任务是一种自然语言处理(NLP)任务,其中模型的目标是将输入的文本转换为声音,实现自动语音合成。具体来说,模型需要理解输入的文本并生成对应的语音输出,使得合成的语音听起来自然而流畅,类似于人类语音的表达方式。
Bark
- Bark(https://github.com/suno-ai/bark) 是由 Suno 创建的基于转换器的文本到音频模型。Bark 可以生成高度逼真的多语言语音以及其他音频,包括音乐、背景噪音和简单的音效。该模型还可以产生非语言交流,如大笑、叹息和哭泣。为了支持研究社区,我们提供了对预训练模型检查点的访问,这些检查点已准备好进行推理并可用于商业用途。
bark.cpp
- https://github.com/PABannier/bark.cpp
编译
$mkdir build
$cd build
$cmake ..
-- The C compiler identification is GNU 9.5.0
-- The CXX compiler identification is GNU 9.5.0
-- Detecting C compiler ABI info
-- Detecting C compiler ABI info - done
-- Check for working C compiler: /usr/bin/cc - skipped
-- Detecting C compile features
-- Detecting C compile features - done
-- Detecting CXX compiler ABI info
-- Detecting CXX compiler ABI info - done
-- Check for working CXX compiler: /usr/bin/c++ - skipped
-- Detecting CXX compile features
-- Detecting CXX compile features - done
-- Looking for pthread.h
-- Looking for pthread.h - found
-- Performing Test CMAKE_HAVE_LIBC_PTHREAD
-- Performing Test CMAKE_HAVE_LIBC_PTHREAD - Success
-- Found Threads: TRUE
-- CMAKE_SYSTEM_PROCESSOR: x86_64
-- x86 detected
-- Linux detected
-- Configuring done
-- Generating done
-- Build files have been written to: /home/pdd/le/bark.cpp/build
$cmake --build . --config Release
[ 7%] Building C object ggml/src/CMakeFiles/ggml.dir/ggml.c.o
[ 14%] Building C object ggml/src/CMakeFiles/ggml.dir/ggml-alloc.c.o
[ 21%] Linking C static library libggml.a
[ 21%] Built target ggml
[ 28%] Building CXX object CMakeFiles/bark.cpp.dir/bark.cpp.o
[ 42%] Linking CXX static library libbark.cpp.a
[ 42%] Built target bark.cpp
[ 50%] Building CXX object examples/main/CMakeFiles/main.dir/main.cpp.o
[ 57%] Linking CXX executable ../../bin/main
[ 57%] Built target main
[ 64%] Building CXX object examples/server/CMakeFiles/server.dir/server.cpp.o
[ 71%] Linking CXX executable ../../bin/server
[ 71%] Built target server
[ 78%] Building CXX object examples/quantize/CMakeFiles/quantize.dir/quantize.cpp.o
[ 85%] Linking CXX executable ../../bin/quantize
[ 85%] Built target quantize
[ 92%] Building CXX object tests/CMakeFiles/test-tokenizer.dir/test-tokenizer.cpp.o
[100%] Linking CXX executable ../bin/test-tokenizer
[100%] Built target test-tokenizer
权重下载与转换
$cd ../
# text_2.pt, coarse_2.pt, fine_2.pt,https://dl.fbaipublicfiles.com/encodec/v0/encodec_24khz-d7cc33bc.th
$python3 download_weights.py --download-dir ./models
# convert the model to ggml format
$python3 convert.py --dir-model ./models --codec-path ./models --vocab-path ./ggml_weights/ --out-dir ./ggml_weights/
$ ls -ahl ./models/
总用量 13G
drwxrwxr-x 2 pdd pdd 4.0K Jan 29 08:22 .
drwxrwxr-x 13 pdd pdd 4.0K Jan 29 06:50 ..
-rwxrwxrwx 1 pdd pdd 3.7G Jan 29 07:34 coarse_2.pt
-rw-rw-r-- 1 pdd pdd 89M Jan 29 07:29 encodec_24khz-d7cc33bc.th
-rwxrwxrwx 1 pdd pdd 3.5G Jan 29 07:53 fine_2.pt
-rwxrwxrwx 1 pdd pdd 5.0G Jan 29 07:22 text_2.pt
$ ls -ahl ./ggml_weights/
总用量 4.2G
drwxrwxr-x 2 pdd pdd 4.0K Jan 29 08:34 .
drwxrwxr-x 13 pdd pdd 4.0K Jan 29 06:50 ..
-rw-rw-r-- 1 pdd pdd 1.3M Jan 29 08:33 ggml_vocab.bin
-rw-rw-r-- 1 pdd pdd 1.3G Jan 29 08:34 ggml_weights_coarse.bin
-rw-rw-r-- 1 pdd pdd 45M Jan 29 08:34 ggml_weights_codec.bin
-rw-rw-r-- 1 pdd pdd 1.2G Jan 29 08:34 ggml_weights_fine.bin
-rw-rw-r-- 1 pdd pdd 1.7G Jan 29 08:33 ggml_weights_text.bin
-rw-rw-r-- 1 pdd pdd 973K Jan 29 05:23 vocab.txt
$ ./main -m ./ggml_weights/ -p "this is an audio"
运行
$ ./build/bin/main -h
usage: ./build/bin/main [options]options:-h, --help show this help message and exit-t N, --threads N number of threads to use during computation (default: 4)-s N, --seed N seed for random number generator (default: 0)-p PROMPT, --prompt PROMPTprompt to start generation with (default: random)-m FNAME, --model FNAMEmodel path (default: /home/pdd/le/bark.cpp/ggml_weights)-o FNAME, --outwav FNAMEoutput generated wav (default: output.wav)
$ ./build/bin/main -m ./ggml_weights/ -p "this is an audio"
bark_load_model_from_file: loading model from './ggml_weights/'
bark_load_model_from_file: reading bark text model
gpt_model_load: n_in_vocab = 129600
gpt_model_load: n_out_vocab = 10048
gpt_model_load: block_size = 1024
gpt_model_load: n_embd = 1024
gpt_model_load: n_head = 16
gpt_model_load: n_layer = 24
gpt_model_load: n_lm_heads = 1
gpt_model_load: n_wtes = 1
gpt_model_load: ftype = 0
gpt_model_load: qntvr = 0
gpt_model_load: ggml tensor size = 304 bytes
gpt_model_load: ggml ctx size = 1894.87 MB
gpt_model_load: memory size = 192.00 MB, n_mem = 24576
gpt_model_load: model size = 1701.69 MB
bark_load_model_from_file: reading bark vocabbark_load_model_from_file: reading bark coarse model
gpt_model_load: n_in_vocab = 12096
gpt_model_load: n_out_vocab = 12096
gpt_model_load: block_size = 1024
gpt_model_load: n_embd = 1024
gpt_model_load: n_head = 16
gpt_model_load: n_layer = 24
gpt_model_load: n_lm_heads = 1
gpt_model_load: n_wtes = 1
gpt_model_load: ftype = 0
gpt_model_load: qntvr = 0
gpt_model_load: ggml tensor size = 304 bytes
gpt_model_load: ggml ctx size = 1443.87 MB
gpt_model_load: memory size = 192.00 MB, n_mem = 24576
gpt_model_load: model size = 1250.69 MBbark_load_model_from_file: reading bark fine model
gpt_model_load: n_in_vocab = 1056
gpt_model_load: n_out_vocab = 1056
gpt_model_load: block_size = 1024
gpt_model_load: n_embd = 1024
gpt_model_load: n_head = 16
gpt_model_load: n_layer = 24
gpt_model_load: n_lm_heads = 7
gpt_model_load: n_wtes = 8
gpt_model_load: ftype = 0
gpt_model_load: qntvr = 0
gpt_model_load: ggml tensor size = 304 bytes
gpt_model_load: ggml ctx size = 1411.25 MB
gpt_model_load: memory size = 192.00 MB, n_mem = 24576
gpt_model_load: model size = 1218.26 MBbark_load_model_from_file: reading bark codec model
encodec_model_load: model size = 44.32 MBbark_load_model_from_file: total model size = 4170.64 MBbark_tokenize_input: prompt: 'this is an audio'
bark_tokenize_input: number of tokens in prompt = 513, first 8 tokens: 20579 20172 20199 33733 129595 129595 129595 129595
bark_forward_text_encoder: ...........................................................................................................bark_print_statistics: mem per token = 4.81 MB
bark_print_statistics: sample time = 16.03 ms / 109 tokens
bark_print_statistics: predict time = 9644.73 ms / 87.68 ms per token
bark_print_statistics: total time = 9663.29 msbark_forward_coarse_encoder: ...................................................................................................................................................................................................................................................................................................................................bark_print_statistics: mem per token = 8.53 MB
bark_print_statistics: sample time = 4.43 ms / 324 tokens
bark_print_statistics: predict time = 52071.64 ms / 160.22 ms per token
bark_print_statistics: total time = 52080.24 msggml_new_object: not enough space in the context's memory pool (needed 4115076720, available 4112941056)
段错误 (核心已转储)
- 一开始以为是内存不足,去增加了虚拟内存,但仍然报错
$ sudo dd if=/dev/zero of=swapfile bs=1024 count=10000000
记录了10000000+0 的读入
记录了10000000+0 的写出
10240000000字节(10 GB,9.5 GiB)已复制,55.3595 s,185 MB/s
$ sudo chmod 600 ./swapfile # delete the swapfile if you dont need it
$ sudo mkswap -f ./swapfile
正在设置交换空间版本 1,大小 = 9.5 GiB (10239995904 个字节)
无标签, UUID=f3e2a0be-b880-48da-b598-950b7d69f94f
$ sudo swapon ./swapfile
$ free -mtotal used free shared buff/cache available
内存: 15731 6441 307 1242 8982 7713
交换: 11813 2047 9765$ ./build/bin/main -m ./ggml_weights/ -p "this is an audio"
ggml_new_object: not enough space in the context's memory pool (needed 4115076720, available 4112941056)
- 去看了报错的函数,应该不是内存的原因
static struct ggml_object * ggml_new_object(struct ggml_context * ctx, enum ggml_object_type type, size_t size) {// always insert objects at the end of the context's memory poolstruct ggml_object * obj_cur = ctx->objects_end;const size_t cur_offs = obj_cur == NULL ? 0 : obj_cur->offs;const size_t cur_size = obj_cur == NULL ? 0 : obj_cur->size;const size_t cur_end = cur_offs + cur_size;// align to GGML_MEM_ALIGNsize_t size_needed = GGML_PAD(size, GGML_MEM_ALIGN);char * const mem_buffer = ctx->mem_buffer;struct ggml_object * const obj_new = (struct ggml_object *)(mem_buffer + cur_end);if (cur_end + size_needed + GGML_OBJECT_SIZE > ctx->mem_size) {GGML_PRINT("%s: not enough space in the context's memory pool (needed %zu, available %zu)\n",__func__, cur_end + size_needed, ctx->mem_size);assert(false);return NULL;}*obj_new = (struct ggml_object) {.offs = cur_end + GGML_OBJECT_SIZE,.size = size_needed,.next = NULL,.type = type,};ggml_assert_aligned(mem_buffer + obj_new->offs);if (obj_cur != NULL) {obj_cur->next = obj_new;} else {// this is the first object in this contextctx->objects_begin = obj_new;}ctx->objects_end = obj_new;//printf("%s: inserted new object at %zu, size = %zu\n", __func__, cur_end, obj_new->size);return obj_new;
}
- 然后找到了https://github.com/PABannier/bark.cpp/issues/122
$ cd bark.cpp/
$ git checkout -f 07e651618b3a8a27de3bfa7f733cdb0aa8f46b8a
HEAD 目前位于 07e6516 ENH Decorrelate fine GPT graph (#111)
- 运行成功
/home/pdd/le/bark.cpp/cmake-build-debug/bin/main
bark_load_model_from_file: loading model from '/home/pdd/le/bark.cpp/ggml_weights'
bark_load_model_from_file: reading bark text model
gpt_model_load: n_in_vocab = 129600
gpt_model_load: n_out_vocab = 10048
gpt_model_load: block_size = 1024
gpt_model_load: n_embd = 1024
gpt_model_load: n_head = 16
gpt_model_load: n_layer = 24
gpt_model_load: n_lm_heads = 1
gpt_model_load: n_wtes = 1
gpt_model_load: ftype = 0
gpt_model_load: qntvr = 0
gpt_model_load: ggml tensor size = 272 bytes
gpt_model_load: ggml ctx size = 1894.87 MB
gpt_model_load: memory size = 192.00 MB, n_mem = 24576
gpt_model_load: model size = 1701.69 MB
bark_load_model_from_file: reading bark vocabbark_load_model_from_file: reading bark coarse model
gpt_model_load: n_in_vocab = 12096
gpt_model_load: n_out_vocab = 12096
gpt_model_load: block_size = 1024
gpt_model_load: n_embd = 1024
gpt_model_load: n_head = 16
gpt_model_load: n_layer = 24
gpt_model_load: n_lm_heads = 1
gpt_model_load: n_wtes = 1
gpt_model_load: ftype = 0
gpt_model_load: qntvr = 0
gpt_model_load: ggml tensor size = 272 bytes
gpt_model_load: ggml ctx size = 1443.87 MB
gpt_model_load: memory size = 192.00 MB, n_mem = 24576
gpt_model_load: model size = 1250.69 MBbark_load_model_from_file: reading bark fine model
gpt_model_load: n_in_vocab = 1056
gpt_model_load: n_out_vocab = 1056
gpt_model_load: block_size = 1024
gpt_model_load: n_embd = 1024
gpt_model_load: n_head = 16
gpt_model_load: n_layer = 24
gpt_model_load: n_lm_heads = 7
gpt_model_load: n_wtes = 8
gpt_model_load: ftype = 0
gpt_model_load: qntvr = 0
gpt_model_load: ggml tensor size = 272 bytes
gpt_model_load: ggml ctx size = 1411.25 MB
gpt_model_load: memory size = 192.00 MB, n_mem = 24576
gpt_model_load: model size = 1218.26 MBbark_load_model_from_file: reading bark codec modelbark_load_model_from_file: total model size = 4170.64 MBbark_tokenize_input: prompt: 'this is an audio'
bark_tokenize_input: number of tokens in prompt = 513, first 8 tokens: 20579 20172 20199 33733 129595 129595 129595 129595
encodec_model_load: model size = 44.32 MB
bark_forward_text_encoder: ...........................................................................................................bark_print_statistics: mem per token = 4.80 MB
bark_print_statistics: sample time = 59.49 ms / 109 tokens
bark_print_statistics: predict time = 24761.95 ms / 225.11 ms per token
bark_print_statistics: total time = 24826.76 msbark_forward_coarse_encoder: ...................................................................................................................................................................................................................................................................................................................................bark_print_statistics: mem per token = 8.51 MB
bark_print_statistics: sample time = 19.74 ms / 324 tokens
bark_print_statistics: predict time = 178366.69 ms / 548.82 ms per token
bark_print_statistics: total time = 178396.22 msbark_forward_fine_encoder: .....bark_print_statistics: mem per token = 0.66 MB
bark_print_statistics: sample time = 304.20 ms / 6144 tokens
bark_print_statistics: predict time = 407086.19 ms / 58155.17 ms per token
bark_print_statistics: total time = 407399.91 msbark_forward_encodec: mem per token = 760209 bytes
bark_forward_encodec: predict time = 4349.03 ms
bark_forward_encodec: total time = 4349.07 msNumber of frames written = 51840.main: load time = 11441.58 ms
main: eval time = 614987.69 ms
main: total time = 626429.31 msProcess finished with exit code 0
CG
- 科大讯飞 语义理解 AIUI封装
- https://github.com/iboB/pytorch-ggml-plugin
相关文章:
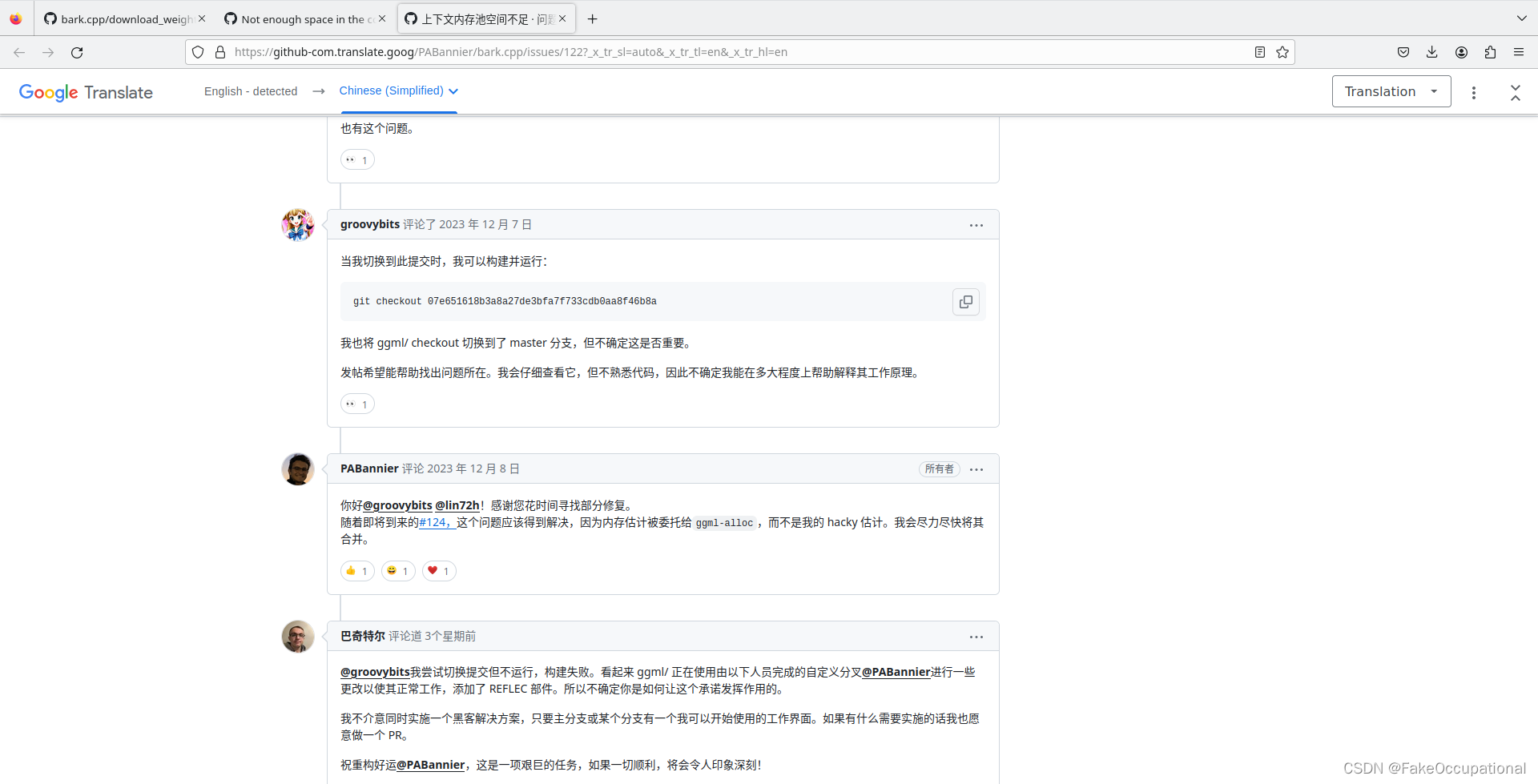
webassembly003 TTS BARK.CPP
TTS task TTS(Text-to-Speech)任务是一种自然语言处理(NLP)任务,其中模型的目标是将输入的文本转换为声音,实现自动语音合成。具体来说,模型需要理解输入的文本并生成对应的语音输出࿰…...

HiveSQL题——排序函数(row_number/rank/dense_rank)
一、窗口函数的知识点 1.1 窗户函数的定义 窗口函数可以拆分为【窗口函数】。窗口函数官网指路: LanguageManual WindowingAndAnalytics - Apache Hive - Apache Software Foundationhttps://cwiki.apache.org/confluence/display/Hive/LanguageManual%20Windowin…...
【C语言】(9)分支结构
一.if-else 语句 if-else 适用于简单和复杂的条件判断。 a. 基本 if 语句 用途:基本的条件测试。语法:if (condition) {// 代码块 }示例:if (score > 60) {printf("及格\n"); }b. if-else 语句 用途:二选一的条件…...
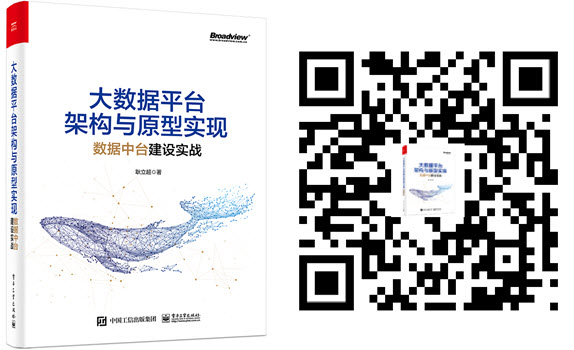
Flink 集成 Debezium Confluent Avro ( format=debezium-avro-confluent )
博主历时三年精心创作的《大数据平台架构与原型实现:数据中台建设实战》一书现已由知名IT图书品牌电子工业出版社博文视点出版发行,点击《重磅推荐:建大数据平台太难了!给我发个工程原型吧!》了解图书详情,京东购书链接:https://item.jd.com/12677623.html,扫描左侧二维…...

R语言(数据导入,清洗,可视化,特征工程,建模)
记录一下痛失的超级轻松的数据分析实习(线上),hr问我有没有相关经历,我说我会用jupyter book进行数据导入,清洗,可视化,特征工程,建模,python学和用的比较多,…...
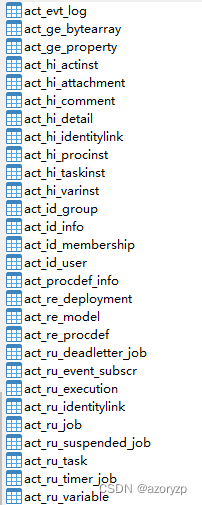
springboot 整合 Activiti6
1.添加maven依赖 <dependency><groupId>org.activiti</groupId><artifactId>activiti-spring-boot-starter-basic</artifactId><version>6.0.0</version> </dependency>2.添加配置 spring:activiti:check-process-definitio…...
微信小程序canvas画布实现直线自由缩放、移动功能
目录 实现效果 一、获取画布信息并绘制背景 二、绘制直线...
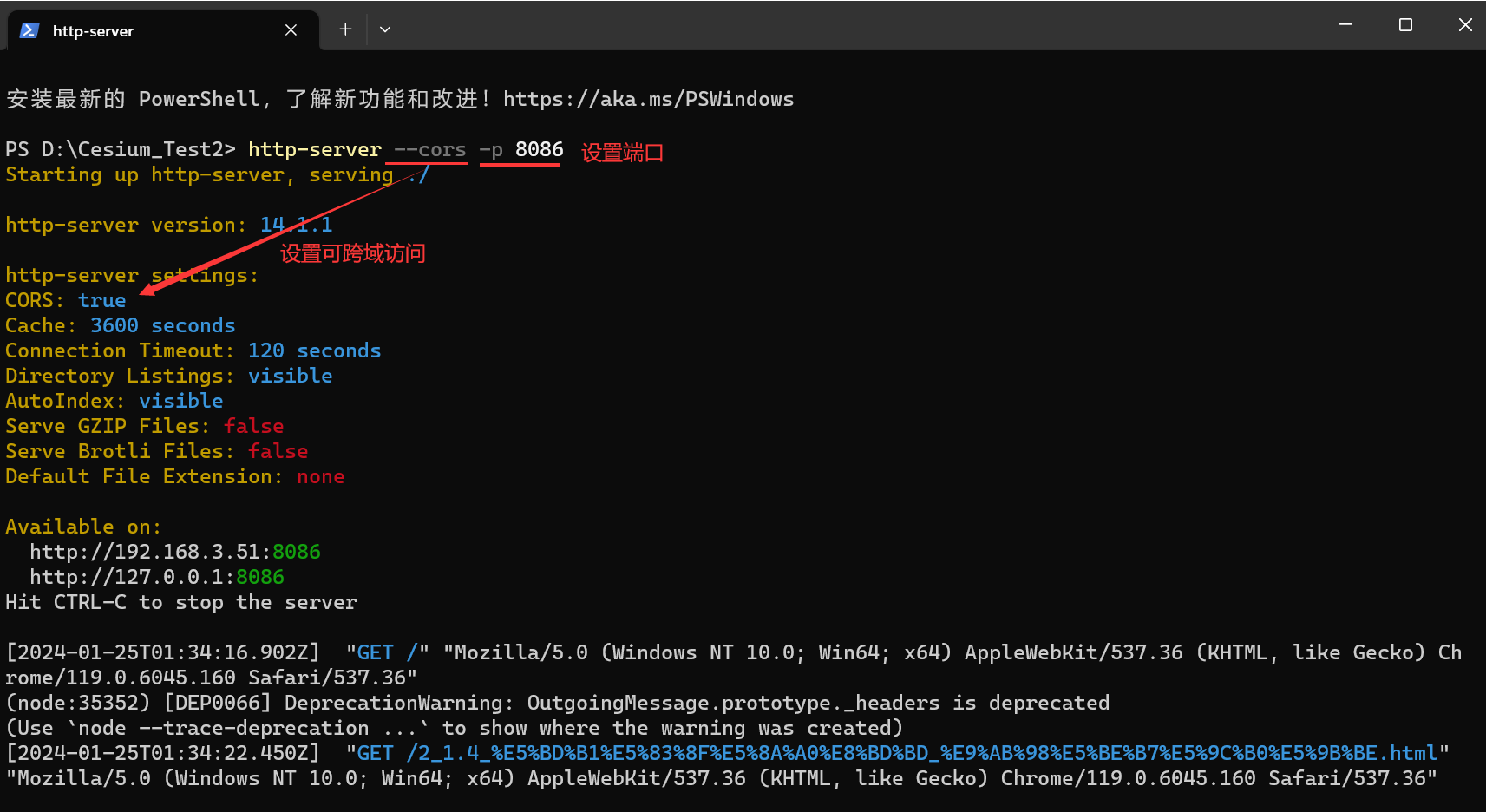
Cesium数据加载
文章目录 0.引言1.影像加载1.1Bing地图1.2天地图1.3ArcGIS在线地图1.4高德地图1.5OSM影像1.6MapBox影像 2.OGC地图服务2.1WMS2.2WMTS2.3TMS 3.GeoJSON数据加载4.KML数据加载5.TIFF数据加载6.点云数据加载7.地形数据加载7.1在线地形数据加载7.2本地地形数据加载 8.倾斜摄影模型数…...
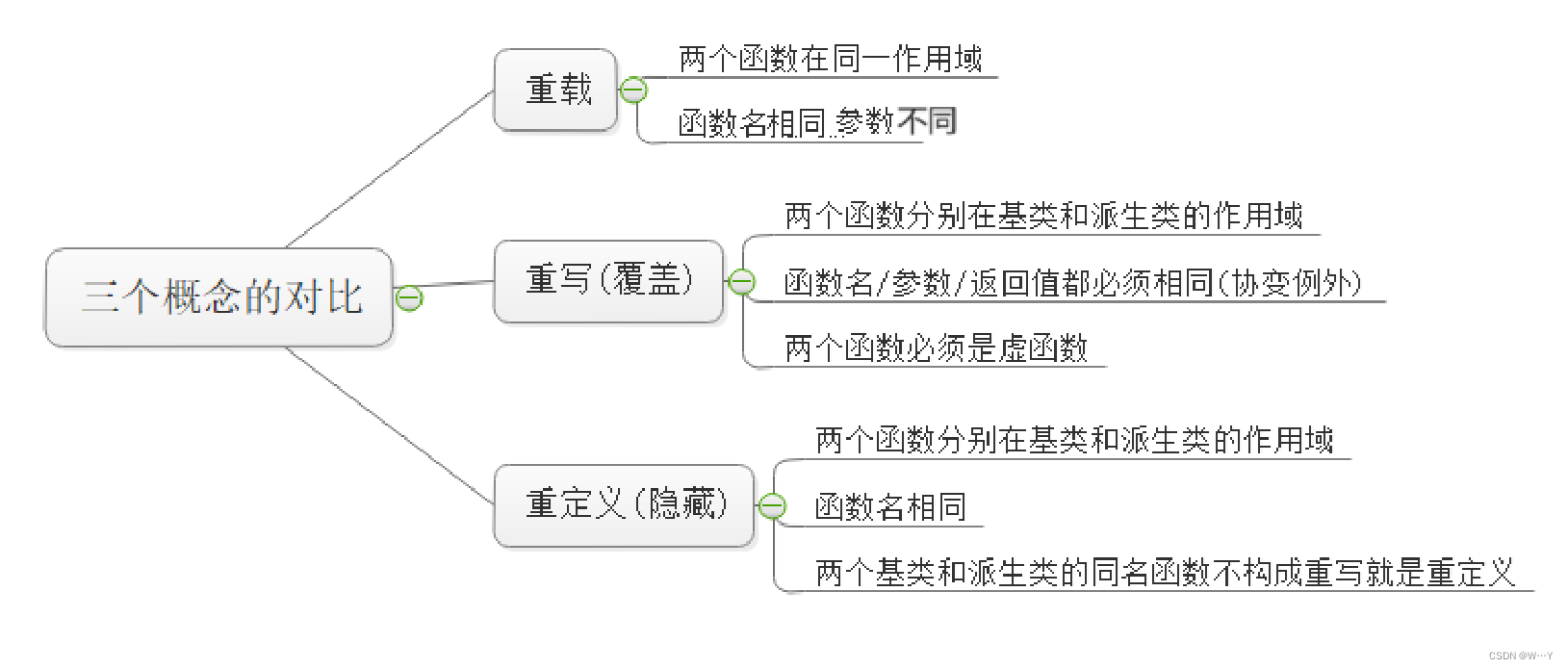
【C++历练之路】探秘C++三大利器之一——多态
W...Y的主页 😊 代码仓库分享💕 前言🍔: 在计算机科学的广袤领域中,C多态性是一门令人着迷的技术艺术,它赋予我们的代码更强大的灵活性和可维护性。想象一下,你正在构建一个程序,需要适应不断…...
业务逐字稿
1.WEB端旅游线路发布模块 旅游公司在Web端点击新增旅游线路按钮,浏览器请求发送到Nginx,Nginx反向代理到网关,网关去找微服务,微服务实现具体的旅游线路发布功能 旅游公司工作人员在Web端点击新增旅游线路按钮,浏览器…...
微服务舞台上的“三步曲“:Spring Cloud 服务注册、服务发现与服务调用
在当今软件开发的舞台上,微服务架构已然成为引领潮流的主角。而在这场微服务的大戏中,Spring Cloud 以其强大的工具集成为关键演员,为我们呈现了一个完美的"三步曲":服务注册、服务发现与服务调用。 第一步:…...
中间件
在 Java 开发中,中间件是指位于应用程序和操作系统之间的软件层,它提供了一些通用的功能和服务,帮助简化开发和部署过程,提高系统的可靠性、性能和可扩展性。 常见的 Java 中间件包括: 1.应用服务器(Appl…...
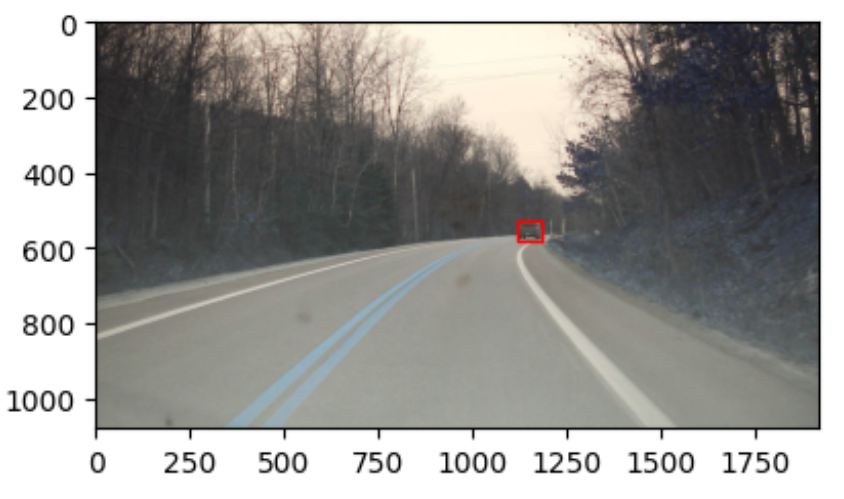
4D毫米波雷达——ADCNet 原始雷达数据 目标检测与可行驶区域分割
前言 本文介绍使用4D毫米波雷达,基于原始雷达数据,实现目标检测与可行驶区域分割,它是来自2023-12的论文。 会讲解论文整体思路、输入分析、模型框架、设计理念、损失函数等,还有结合代码进行分析。 论文地址:ADCNe…...
「优选算法刷题」:提莫攻击
一、题目 在《英雄联盟》的世界中,有一个叫 “提莫” 的英雄。他的攻击可以让敌方英雄艾希(编者注:寒冰射手)进入中毒状态。 当提莫攻击艾希,艾希的中毒状态正好持续 duration 秒。 正式地讲,提莫在 t 发…...
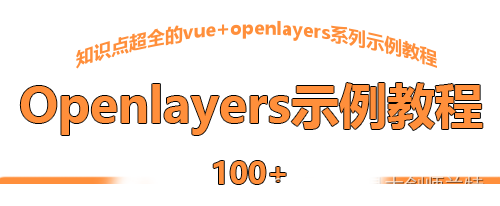
260:vue+openlayers 通过webgl方式加载矢量图层
第260个 点击查看专栏目录 本示例介绍如何在vue+openlayers中通过webgl方式加载矢量图层。在做这个示例的时候,采用vite的方式而非webpack的方式。这里的基础设置需要改变一下。 ol的版本7.5.2或者更高。 直接复制下面的 vue+openlayers源代码,操作2分钟即可运行实现效果 文…...
Android 8.1 相关修改
一些常用修改,做个记录,为了节约时间和防止踩坑。 一、修改默认中文 修改位置: build\make\target\product\full_base.mk 修改内容: # Put en_US first in the list, so make it default. PRODUCT_LOCALES : zh_…...
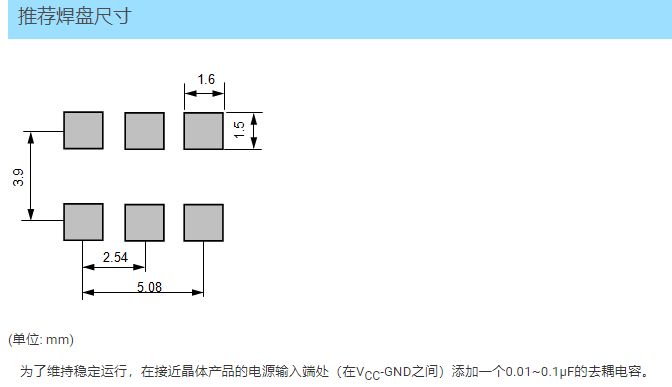
EG-2121CA (晶体振荡器 低抖动表面声波(SAW)振荡器)
在当今高度数字化的时代,稳定的信号传输显得尤为重要。若要实现信号的稳定传输,晶体振荡器必不可少。EG-2121CA,它是一款低抖动表面声波(SAW)振荡器设计的产品,凭借其出色的频率范围、稳定的电源电压和可靠…...
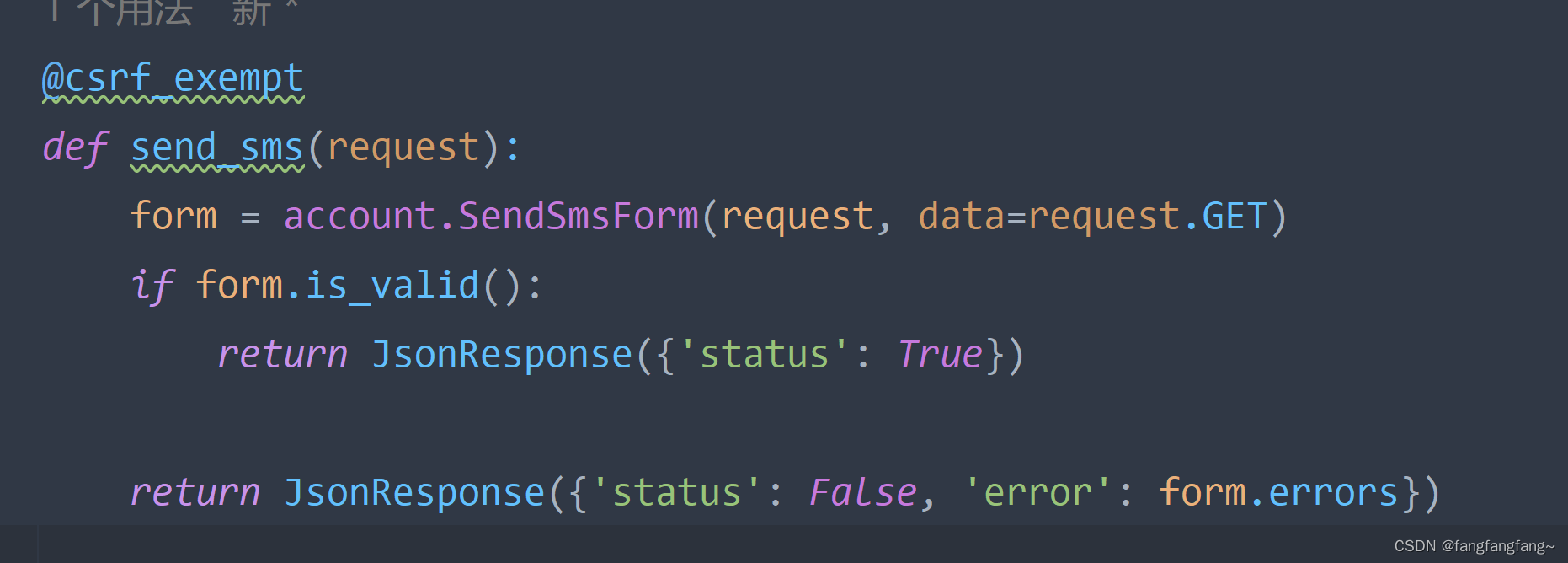
Django知识随笔
目录 1.如何再ajax中传输post数据? 2.在form表单中使用jquery序列化,input框过多。 1.如何再ajax中传输post数据? 在ajax传递的那个网址,会调用你路由的视图函数,在视图函数上面加一句 csrf_exempt 。写上之后会有提…...
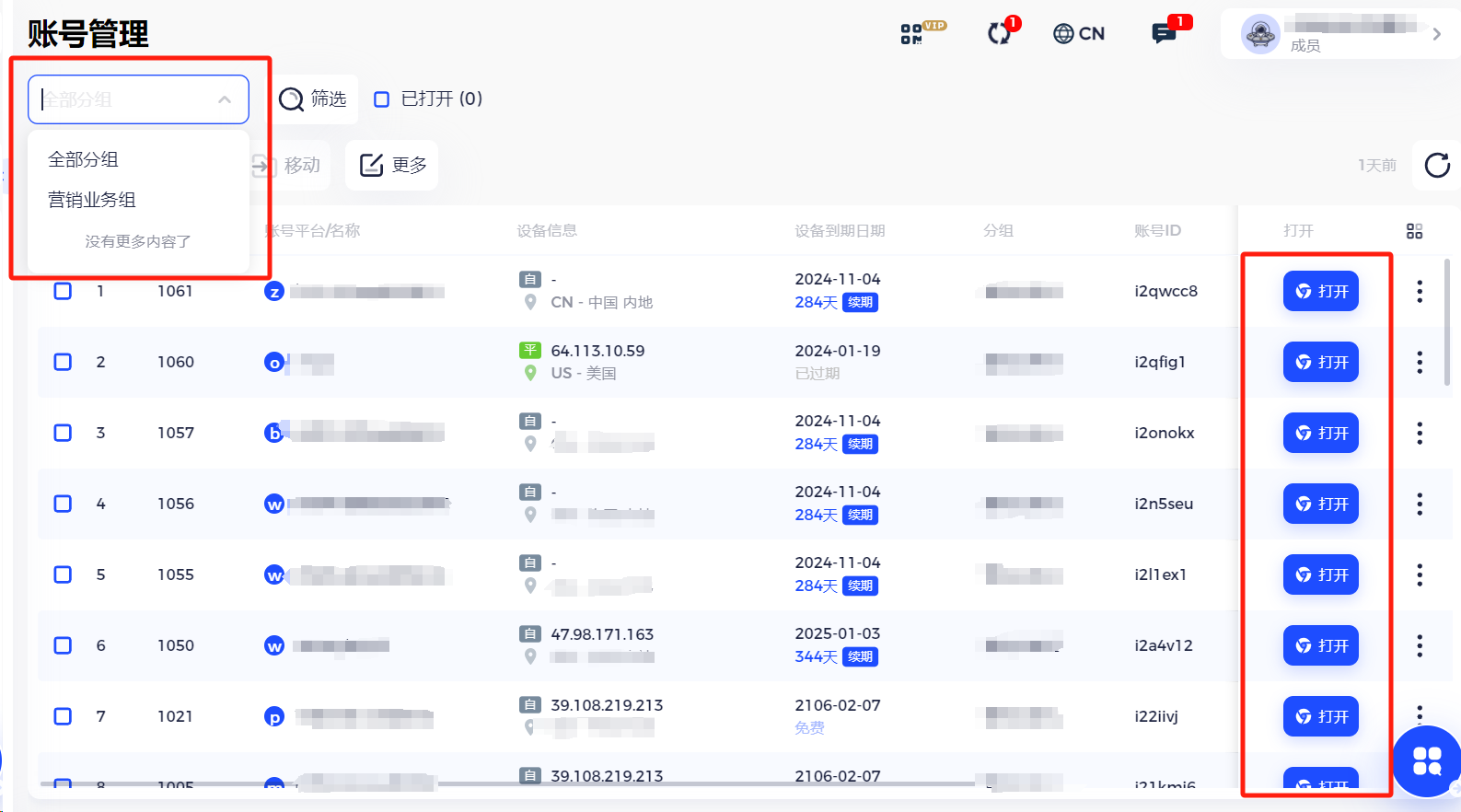
Facebook 广告帐户:多账号运营如何防止封号?
Facebook目前是全球最受欢迎的社交媒体平台之一,拥有超过27亿活跃用户。因此,它已成为个人和企业向全球受众宣传其产品和服务的重要平台。 然而,Facebook 制定了广告商必须遵守的严格政策和准则,以确保其广告的质量和相关性&…...
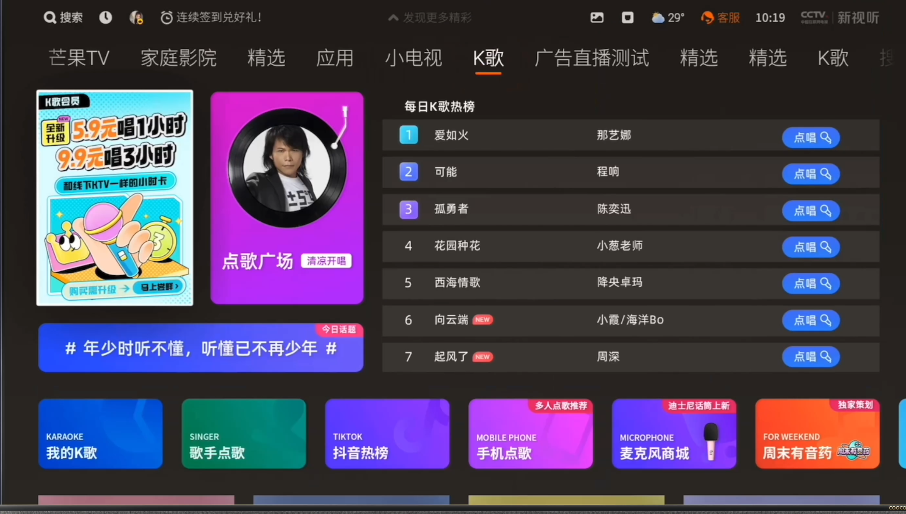
酷开会员 | 用酷开系统点亮多彩休闲时刻
现代人的休闲方式多种多样,随着科技的发展和生活水平的提高,人们有了更多的选择。而电视,作为一个休闲娱乐的载体,在生活中扮演着重要的角色。 以前,电视是家家户户休闲娱乐的重要组成部分,现在࿰…...
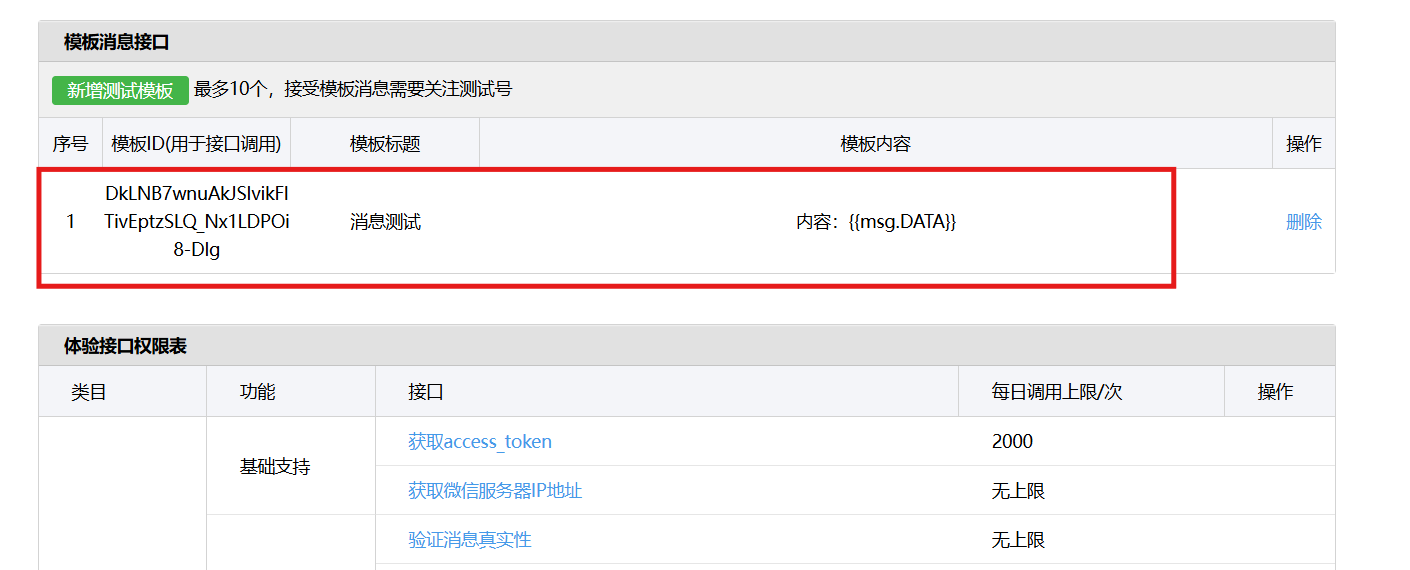
测试微信模版消息推送
进入“开发接口管理”--“公众平台测试账号”,无需申请公众账号、可在测试账号中体验并测试微信公众平台所有高级接口。 获取access_token: 自定义模版消息: 关注测试号:扫二维码关注测试号。 发送模版消息: import requests da…...
FFmpeg 低延迟同屏方案
引言 在实时互动需求激增的当下,无论是在线教育中的师生同屏演示、远程办公的屏幕共享协作,还是游戏直播的画面实时传输,低延迟同屏已成为保障用户体验的核心指标。FFmpeg 作为一款功能强大的多媒体框架,凭借其灵活的编解码、数据…...
Qwen3-Embedding-0.6B深度解析:多语言语义检索的轻量级利器
第一章 引言:语义表示的新时代挑战与Qwen3的破局之路 1.1 文本嵌入的核心价值与技术演进 在人工智能领域,文本嵌入技术如同连接自然语言与机器理解的“神经突触”——它将人类语言转化为计算机可计算的语义向量,支撑着搜索引擎、推荐系统、…...

[10-3]软件I2C读写MPU6050 江协科技学习笔记(16个知识点)
1 2 3 4 5 6 7 8 9 10 11 12 13 14 15 16...
论文解读:交大港大上海AI Lab开源论文 | 宇树机器人多姿态起立控制强化学习框架(一)
宇树机器人多姿态起立控制强化学习框架论文解析 论文解读:交大&港大&上海AI Lab开源论文 | 宇树机器人多姿态起立控制强化学习框架(一) 论文解读:交大&港大&上海AI Lab开源论文 | 宇树机器人多姿态起立控制强化…...
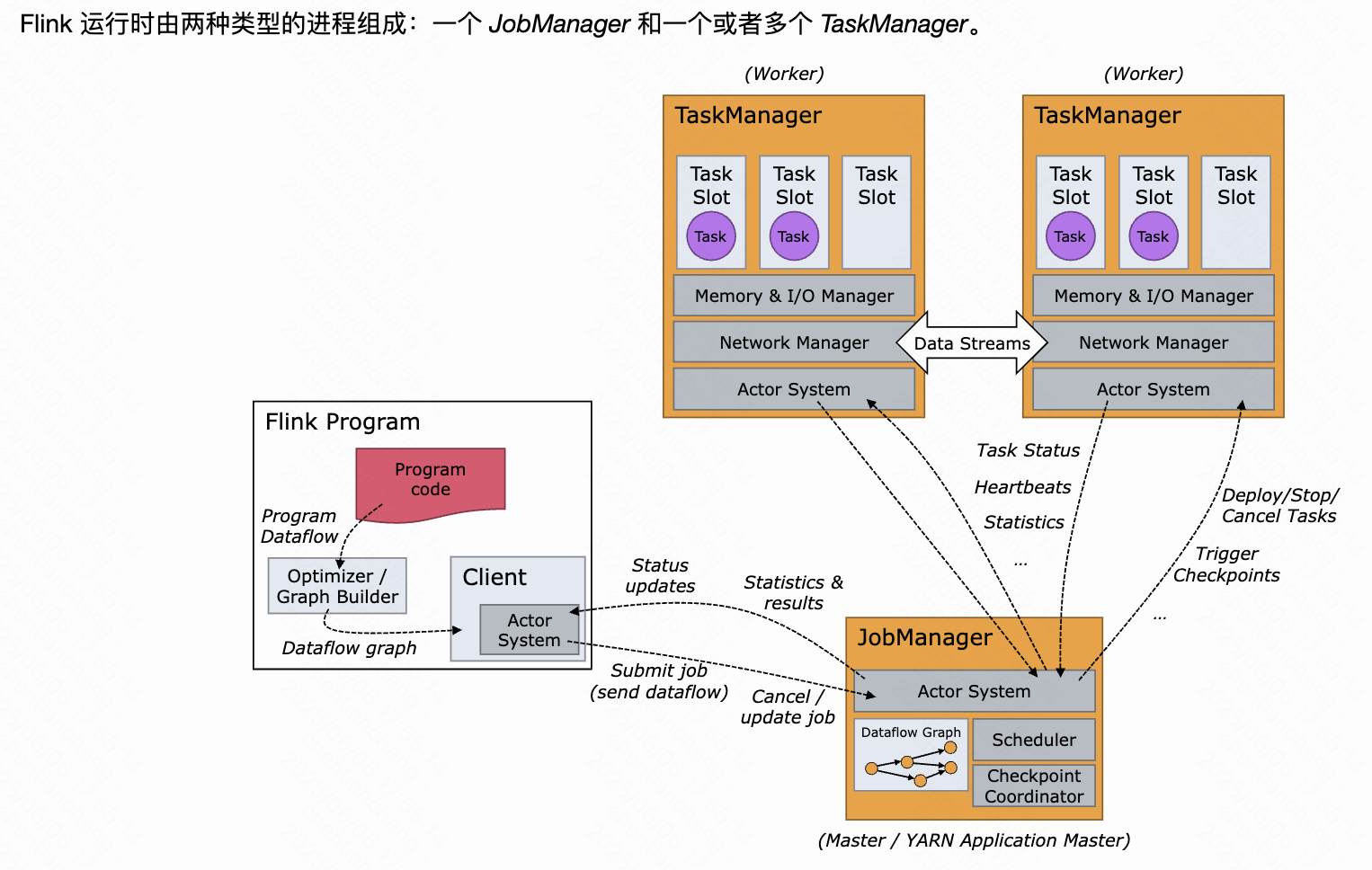
《基于Apache Flink的流处理》笔记
思维导图 1-3 章 4-7章 8-11 章 参考资料 源码: https://github.com/streaming-with-flink 博客 https://flink.apache.org/bloghttps://www.ververica.com/blog 聚会及会议 https://flink-forward.orghttps://www.meetup.com/topics/apache-flink https://n…...
HTML前端开发:JavaScript 常用事件详解
作为前端开发的核心,JavaScript 事件是用户与网页交互的基础。以下是常见事件的详细说明和用法示例: 1. onclick - 点击事件 当元素被单击时触发(左键点击) button.onclick function() {alert("按钮被点击了!&…...
爬虫基础学习day2
# 爬虫设计领域 工商:企查查、天眼查短视频:抖音、快手、西瓜 ---> 飞瓜电商:京东、淘宝、聚美优品、亚马逊 ---> 分析店铺经营决策标题、排名航空:抓取所有航空公司价格 ---> 去哪儿自媒体:采集自媒体数据进…...
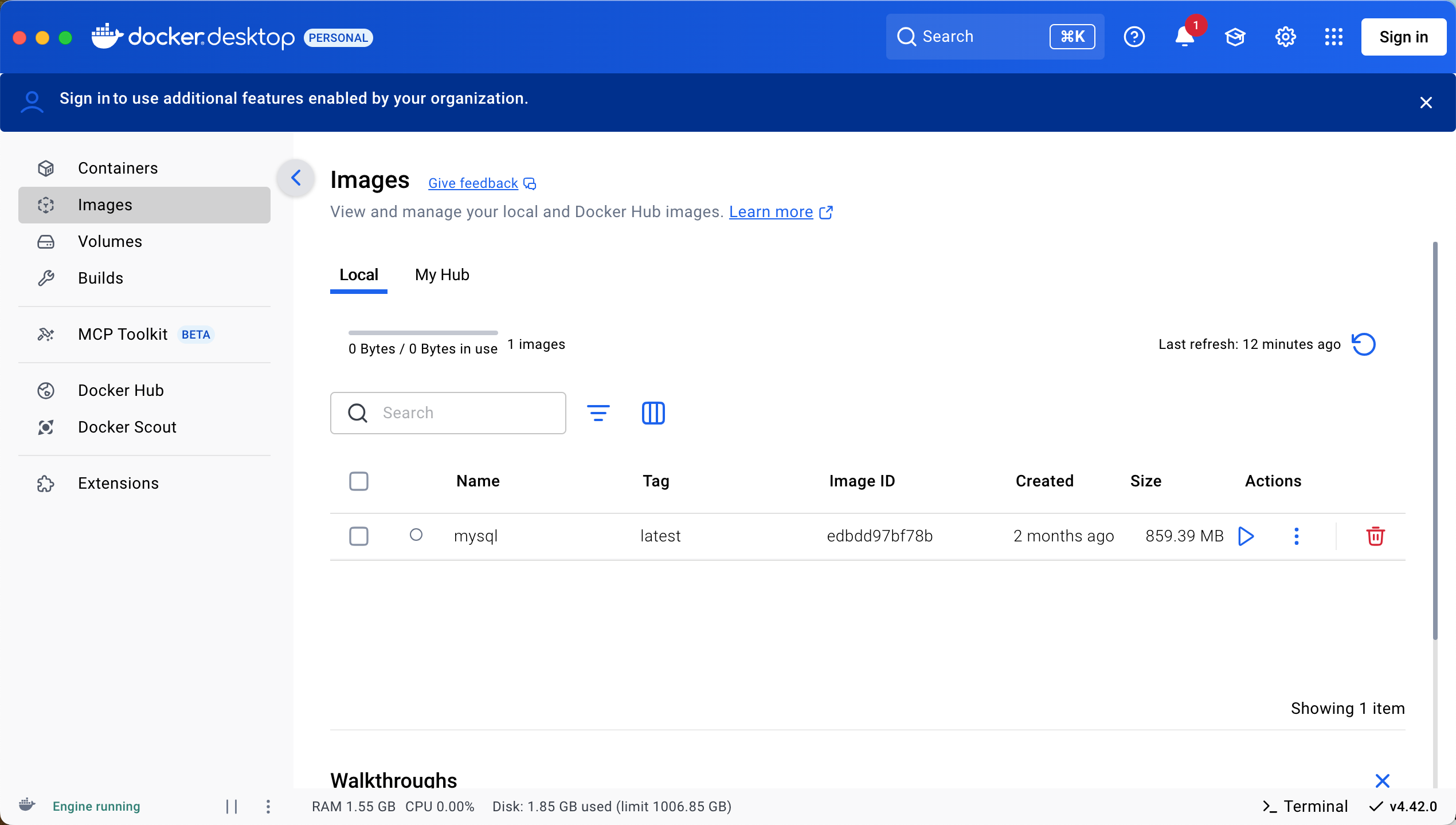
Docker 本地安装 mysql 数据库
Docker: Accelerated Container Application Development 下载对应操作系统版本的 docker ;并安装。 基础操作不再赘述。 打开 macOS 终端,开始 docker 安装mysql之旅 第一步 docker search mysql 》〉docker search mysql NAME DE…...
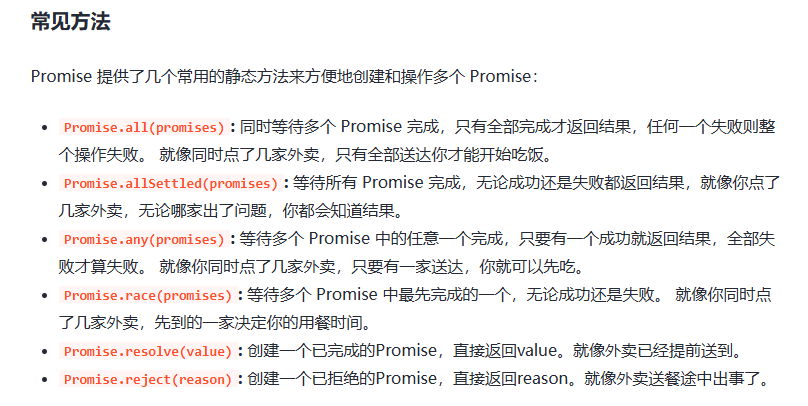
处理vxe-table 表尾数据是单独一个接口,表格tableData数据更新后,需要点击两下,表尾才是正确的
修改bug思路: 分别把 tabledata 和 表尾相关数据 console.log() 发现 更新数据先后顺序不对 settimeout延迟查询表格接口 ——测试可行 升级↑:async await 等接口返回后再开始下一个接口查询 ________________________________________________________…...