pytorch中的torch.nn.Linear
torch.nn.Linear是pytorch中的线性层,应该是最常见的网络层了,官方文档:torch.nn.Linear。
torch.nn.Linear(in_features, out_features, bias=True, device=None, dtype=None)
其中,in_features表示输入的维度;out_features表示输出的维度;bias表示是否包含偏置,默认为True。
nn.linear的作用其实就是对输入进行了一个线性变换,中学时我们学习的线性变换是y=kx+b,但是对于神经网络来说,我们的输入、输出和权重都是一个矩阵,即: o u t p u t = i n p u t ∗ W + b output=input*W+b output=input∗W+b 其中, i n p u t ∈ R n × i input\in R^{n×i} input∈Rn×i, W ∈ R i × o W\in R^{i×o} W∈Ri×o, o u t p u t ∈ R n × o output\in R^{n×o} output∈Rn×o,n为输入向量的行数(通常为batch数),i为输入神经元的个数,o为输出神经元的个数。使用举例:
FC = nn.Linear(20, 40)
input = torch.randn(128, 20) # (128,20)
output = FC(input)
print(output.size()) # (128,40)
官方源码:
import mathimport torch
from torch import Tensor
from torch.nn.parameter import Parameter, UninitializedParameter
from .. import functional as F
from .. import init
from .module import Module
from .lazy import LazyModuleMixinclass Identity(Module):r"""A placeholder identity operator that is argument-insensitive.Args:args: any argument (unused)kwargs: any keyword argument (unused)Shape:- Input: :math:`(*)`, where :math:`*` means any number of dimensions.- Output: :math:`(*)`, same shape as the input.Examples::>>> m = nn.Identity(54, unused_argument1=0.1, unused_argument2=False)>>> input = torch.randn(128, 20)>>> output = m(input)>>> print(output.size())torch.Size([128, 20])"""def __init__(self, *args, **kwargs):super(Identity, self).__init__()def forward(self, input: Tensor) -> Tensor:return inputclass Linear(Module):r"""Applies a linear transformation to the incoming data: :math:`y = xA^T + b`This module supports :ref:`TensorFloat32<tf32_on_ampere>`.Args:in_features: size of each input sampleout_features: size of each output samplebias: If set to ``False``, the layer will not learn an additive bias.Default: ``True``Shape:- Input: :math:`(*, H_{in})` where :math:`*` means any number ofdimensions including none and :math:`H_{in} = \text{in\_features}`.- Output: :math:`(*, H_{out})` where all but the last dimensionare the same shape as the input and :math:`H_{out} = \text{out\_features}`.Attributes:weight: the learnable weights of the module of shape:math:`(\text{out\_features}, \text{in\_features})`. The values areinitialized from :math:`\mathcal{U}(-\sqrt{k}, \sqrt{k})`, where:math:`k = \frac{1}{\text{in\_features}}`bias: the learnable bias of the module of shape :math:`(\text{out\_features})`.If :attr:`bias` is ``True``, the values are initialized from:math:`\mathcal{U}(-\sqrt{k}, \sqrt{k})` where:math:`k = \frac{1}{\text{in\_features}}`Examples::>>> m = nn.Linear(20, 30)>>> input = torch.randn(128, 20)>>> output = m(input)>>> print(output.size())torch.Size([128, 30])"""__constants__ = ['in_features', 'out_features']in_features: intout_features: intweight: Tensordef __init__(self, in_features: int, out_features: int, bias: bool = True,device=None, dtype=None) -> None:factory_kwargs = {'device': device, 'dtype': dtype}super(Linear, self).__init__()self.in_features = in_featuresself.out_features = out_featuresself.weight = Parameter(torch.empty((out_features, in_features), **factory_kwargs))if bias:self.bias = Parameter(torch.empty(out_features, **factory_kwargs))else:self.register_parameter('bias', None)self.reset_parameters()def reset_parameters(self) -> None:# Setting a=sqrt(5) in kaiming_uniform is the same as initializing with# uniform(-1/sqrt(in_features), 1/sqrt(in_features)). For details, see# https://github.com/pytorch/pytorch/issues/57109init.kaiming_uniform_(self.weight, a=math.sqrt(5))if self.bias is not None:fan_in, _ = init._calculate_fan_in_and_fan_out(self.weight)bound = 1 / math.sqrt(fan_in) if fan_in > 0 else 0init.uniform_(self.bias, -bound, bound)def forward(self, input: Tensor) -> Tensor:return F.linear(input, self.weight, self.bias)def extra_repr(self) -> str:return 'in_features={}, out_features={}, bias={}'.format(self.in_features, self.out_features, self.bias is not None)# This class exists solely to avoid triggering an obscure error when scripting
# an improperly quantized attention layer. See this issue for details:
# https://github.com/pytorch/pytorch/issues/58969
# TODO: fail fast on quantization API usage error, then remove this class
# and replace uses of it with plain Linear
class NonDynamicallyQuantizableLinear(Linear):def __init__(self, in_features: int, out_features: int, bias: bool = True,device=None, dtype=None) -> None:super().__init__(in_features, out_features, bias=bias,device=device, dtype=dtype)[docs]class Bilinear(Module):r"""Applies a bilinear transformation to the incoming data::math:`y = x_1^T A x_2 + b`Args:in1_features: size of each first input samplein2_features: size of each second input sampleout_features: size of each output samplebias: If set to False, the layer will not learn an additive bias.Default: ``True``Shape:- Input1: :math:`(*, H_{in1})` where :math:`H_{in1}=\text{in1\_features}` and:math:`*` means any number of additional dimensions including none. All but the last dimensionof the inputs should be the same.- Input2: :math:`(*, H_{in2})` where :math:`H_{in2}=\text{in2\_features}`.- Output: :math:`(*, H_{out})` where :math:`H_{out}=\text{out\_features}`and all but the last dimension are the same shape as the input.Attributes:weight: the learnable weights of the module of shape:math:`(\text{out\_features}, \text{in1\_features}, \text{in2\_features})`.The values are initialized from :math:`\mathcal{U}(-\sqrt{k}, \sqrt{k})`, where:math:`k = \frac{1}{\text{in1\_features}}`bias: the learnable bias of the module of shape :math:`(\text{out\_features})`.If :attr:`bias` is ``True``, the values are initialized from:math:`\mathcal{U}(-\sqrt{k}, \sqrt{k})`, where:math:`k = \frac{1}{\text{in1\_features}}`Examples::>>> m = nn.Bilinear(20, 30, 40)>>> input1 = torch.randn(128, 20)>>> input2 = torch.randn(128, 30)>>> output = m(input1, input2)>>> print(output.size())torch.Size([128, 40])"""__constants__ = ['in1_features', 'in2_features', 'out_features']in1_features: intin2_features: intout_features: intweight: Tensordef __init__(self, in1_features: int, in2_features: int, out_features: int, bias: bool = True,device=None, dtype=None) -> None:factory_kwargs = {'device': device, 'dtype': dtype}super(Bilinear, self).__init__()self.in1_features = in1_featuresself.in2_features = in2_featuresself.out_features = out_featuresself.weight = Parameter(torch.empty((out_features, in1_features, in2_features), **factory_kwargs))if bias:self.bias = Parameter(torch.empty(out_features, **factory_kwargs))else:self.register_parameter('bias', None)self.reset_parameters()def reset_parameters(self) -> None:bound = 1 / math.sqrt(self.weight.size(1))init.uniform_(self.weight, -bound, bound)if self.bias is not None:init.uniform_(self.bias, -bound, bound)def forward(self, input1: Tensor, input2: Tensor) -> Tensor:return F.bilinear(input1, input2, self.weight, self.bias)def extra_repr(self) -> str:return 'in1_features={}, in2_features={}, out_features={}, bias={}'.format(self.in1_features, self.in2_features, self.out_features, self.bias is not None)class LazyLinear(LazyModuleMixin, Linear):r"""A :class:`torch.nn.Linear` module where `in_features` is inferred.In this module, the `weight` and `bias` are of :class:`torch.nn.UninitializedParameter`class. They will be initialized after the first call to ``forward`` is done and themodule will become a regular :class:`torch.nn.Linear` module. The ``in_features`` argumentof the :class:`Linear` is inferred from the ``input.shape[-1]``.Check the :class:`torch.nn.modules.lazy.LazyModuleMixin` for further documentationon lazy modules and their limitations.Args:out_features: size of each output samplebias: If set to ``False``, the layer will not learn an additive bias.Default: ``True``Attributes:weight: the learnable weights of the module of shape:math:`(\text{out\_features}, \text{in\_features})`. The values areinitialized from :math:`\mathcal{U}(-\sqrt{k}, \sqrt{k})`, where:math:`k = \frac{1}{\text{in\_features}}`bias: the learnable bias of the module of shape :math:`(\text{out\_features})`.If :attr:`bias` is ``True``, the values are initialized from:math:`\mathcal{U}(-\sqrt{k}, \sqrt{k})` where:math:`k = \frac{1}{\text{in\_features}}`"""cls_to_become = Linear # type: ignore[assignment]weight: UninitializedParameterbias: UninitializedParameter # type: ignore[assignment]def __init__(self, out_features: int, bias: bool = True,device=None, dtype=None) -> None:factory_kwargs = {'device': device, 'dtype': dtype}# bias is hardcoded to False to avoid creating tensor# that will soon be overwritten.super().__init__(0, 0, False)self.weight = UninitializedParameter(**factory_kwargs)self.out_features = out_featuresif bias:self.bias = UninitializedParameter(**factory_kwargs)def reset_parameters(self) -> None:if not self.has_uninitialized_params() and self.in_features != 0:super().reset_parameters()def initialize_parameters(self, input) -> None: # type: ignore[override]if self.has_uninitialized_params():with torch.no_grad():self.in_features = input.shape[-1]self.weight.materialize((self.out_features, self.in_features))if self.bias is not None:self.bias.materialize((self.out_features,))self.reset_parameters()
# TODO: PartialLinear - maybe in sparse?
相关文章:

pytorch中的torch.nn.Linear
torch.nn.Linear是pytorch中的线性层,应该是最常见的网络层了,官方文档:torch.nn.Linear。 torch.nn.Linear(in_features, out_features, biasTrue, deviceNone, dtypeNone)其中,in_features表示输入的维度;out_featu…...

03-MySQl数据库的-用户管理
一、创建新用户 mysql> create user xjzw10.0.0.% identified by 1; Query OK, 0 rows affected (0.01 sec) 二、查看当前数据库正在登录的用户 mysql> select user(); ---------------- | user() | ---------------- | rootlocalhost | ---------------- 1 row …...
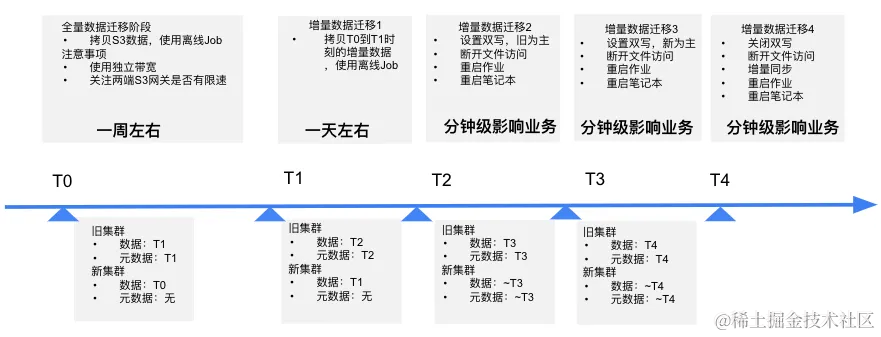
知乎:多云架构下大模型训练,如何保障存储稳定性?
知乎,中文互联网领域领先的问答社区和原创内容平台,2011 年 1 月正式上线,月活跃用户超过 1 亿。平台的搜索和推荐服务得益于先进的 AI 算法,数百名算法工程师基于数据平台和机器学习平台进行海量数据处理和算法训练任务。 为了提…...
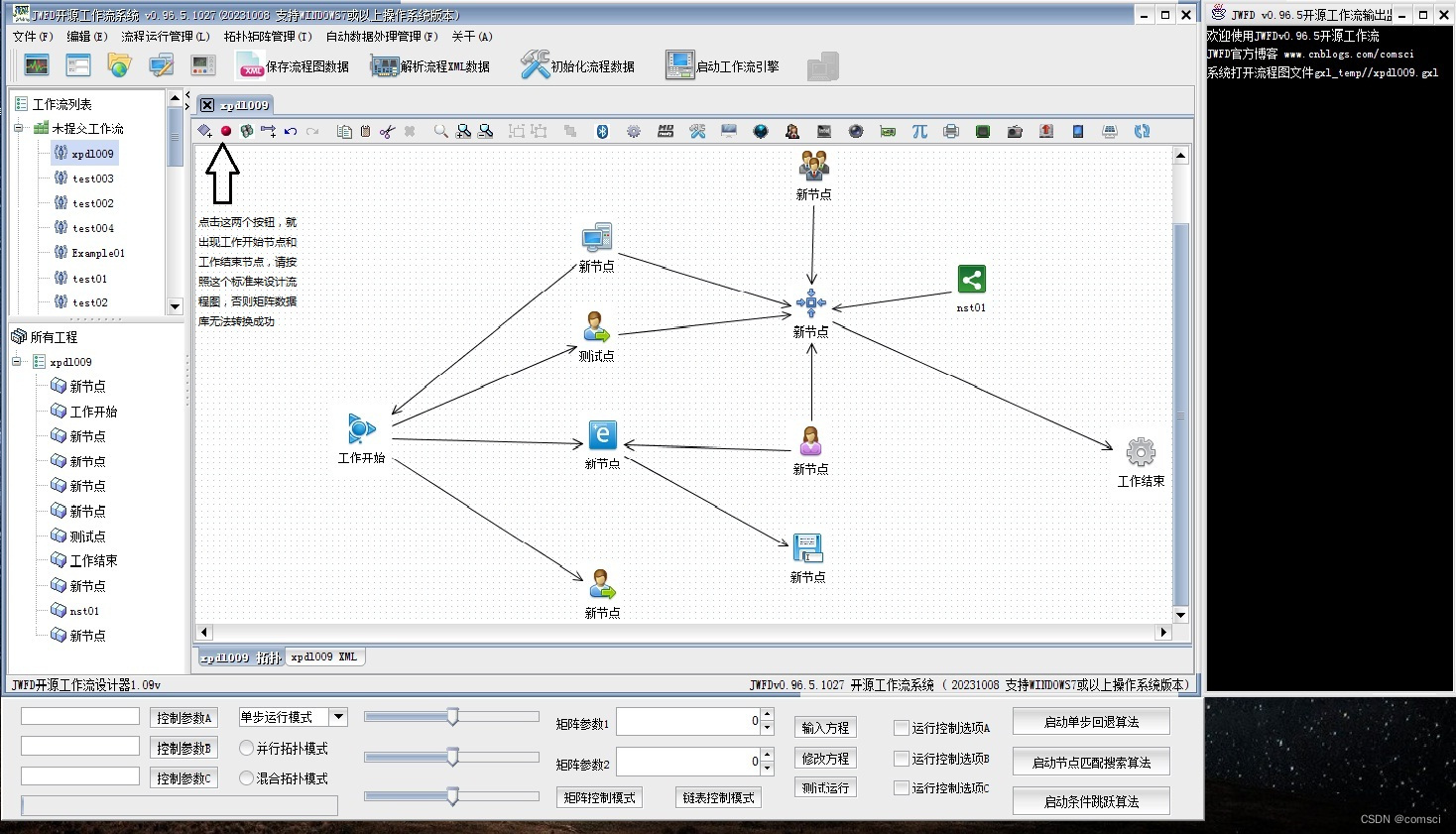
JWFD流程图转换为矩阵数据库的过程说明
在最开始设计流程图的时候,请务必先把开始节点和结束节点画到流程图上面,就是设计器面板的最开始两个按钮,先画开始点和结束点,再画中间的流程,然后保存,这样提交到矩阵数据库就不会出任何问题,…...
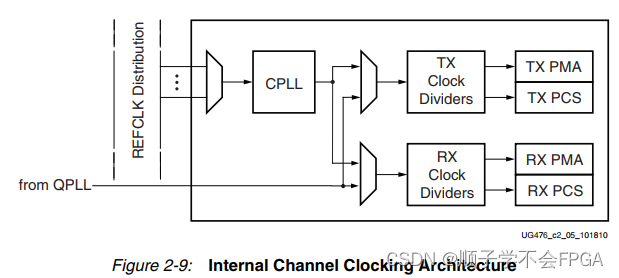
GT收发器第一篇_总体结构介绍
文章目录 前言GT收发器介绍 前言 之前写过一篇简单介绍GT的文章https://blog.csdn.net/m0_56222647/article/details/136730026,可以先通过这篇文章对整体进行简单了解一下。 GT收发器介绍 参考xilinx手册ug476 对于7系列的FPGA,共有3个系列…...
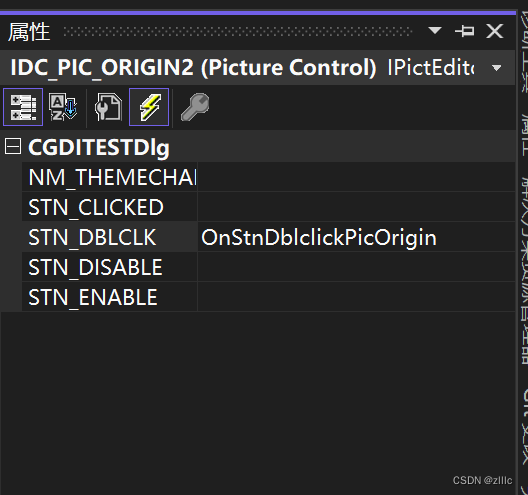
[图像处理] MFC载入图片并进行二值化处理和灰度处理及其效果显示
文章目录 工程效果重要代码完整代码参考 工程效果 载入图片,并在左侧显示原始图片、二值化图片和灰度图片。 双击左侧的图片控件,可以在右侧的大控件中,显示双击的图片。 初始画面: 载入图片: 双击左侧的第二个控件…...

centos7.5 安装gitlab-ce (Omnibus)
一、安装前置依赖 # 安装基础依赖 $ sudo yum -y install policycoreutils openssh-server openssh-clients postfix# 启动 ssh 服务 & 设置为开机启动 $ sudo systemctl enable sshd & sudo systemctl start sshd二、安装gitlab rpm包 # 下载并执行社区版脚本 curl …...
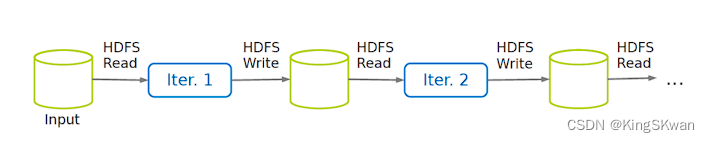
深入理解MapReduce:从Map到Reduce的工作原理解析
当谈到分布式计算和大数据处理时,MapReduce是一个经典的范例。它是一种编程模型和处理框架,用于在大规模数据集上并行运行计算任务。MapReduce包含三个主要阶段:Map、Shuffle 和 Reduce。 ** Map 阶段 ** Map 阶段是 MapReduce 的第一步&am…...
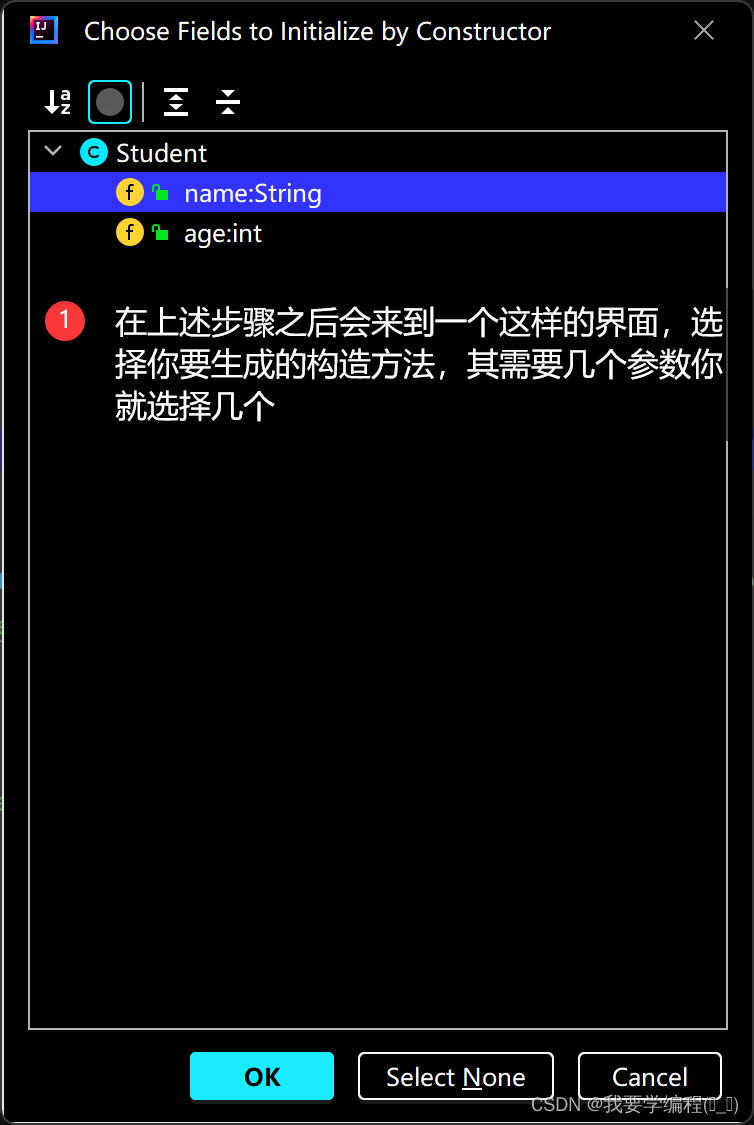
初始Java篇(JavaSE基础语法)(5)(类和对象(上))
个人主页(找往期文章包括但不限于本期文章中不懂的知识点):我要学编程(ಥ_ಥ)-CSDN博客 目录 面向对象的初步认知 面向对象与面向过程的区别 类的定义和使用 类的定义格式 类的实例化 this引用 什么是this引用? this引用…...
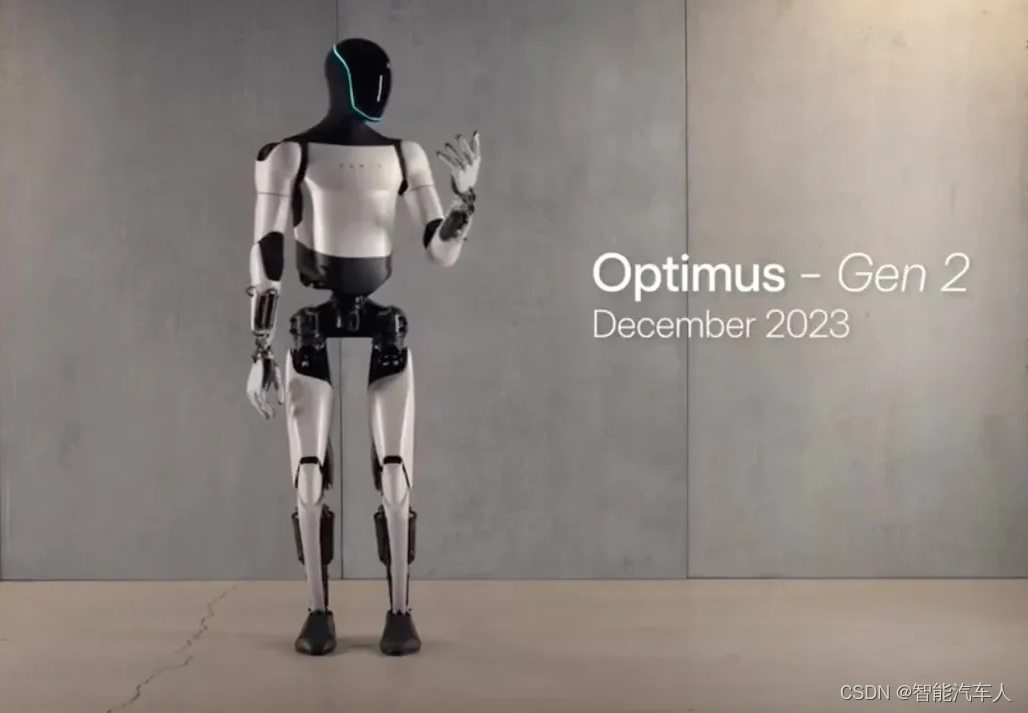
机器人---人形机器人之技术方向
1 背景介绍 在前面的文章《行业杂谈---人形机器人的未来》中,笔者初步介绍了人形机器人的未来发展趋势。同智能汽车一样,它也会是未来机器人领域的一个重要分支。目前地球上最高智慧的结晶体就是人类,那么人形机器人的未来会有非常大的发展空…...
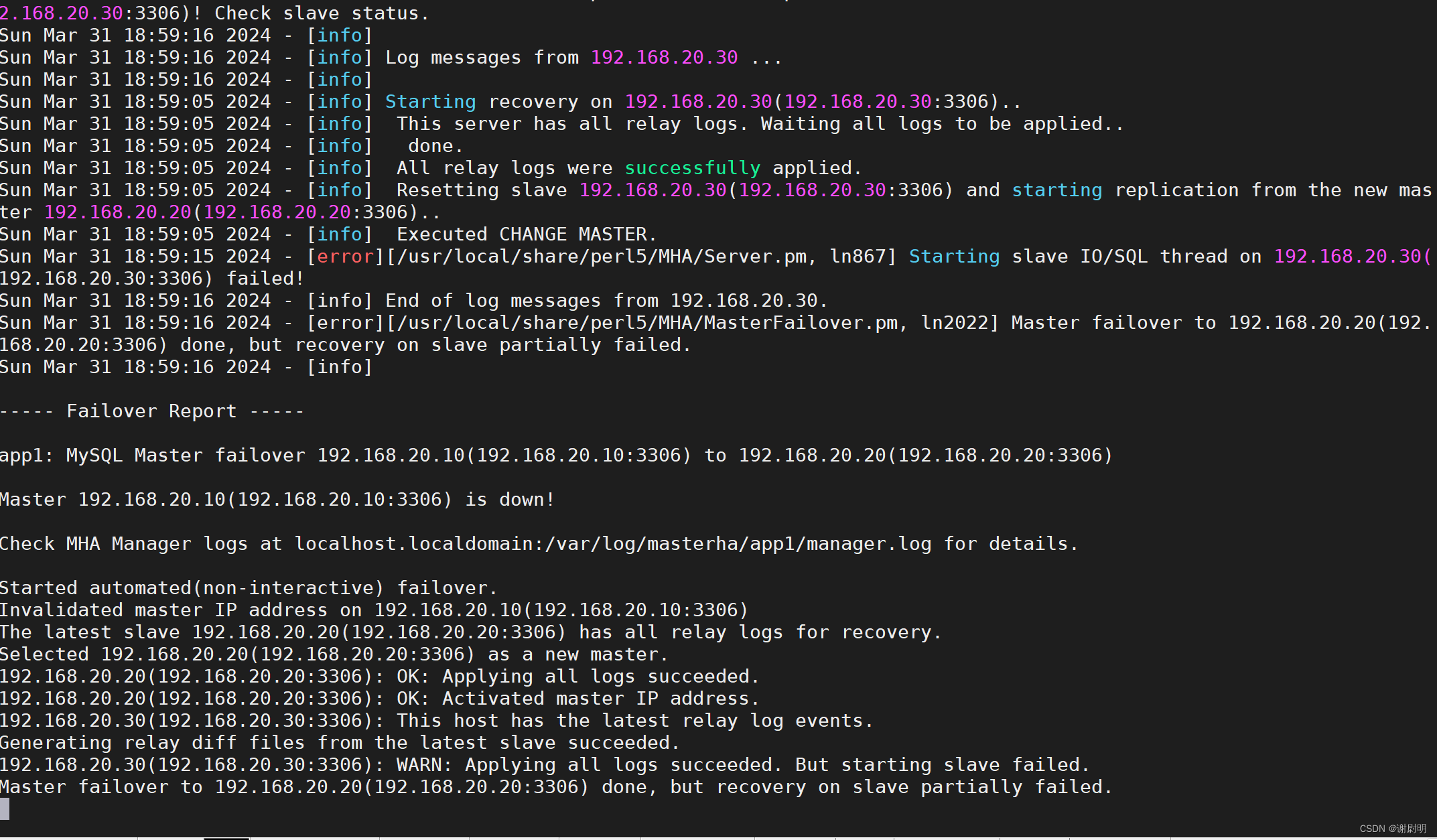
MySQL MHA高可用数据库
文章目录 MySQL MHA高可用数据库搭建MySQL MHA模拟故障故障修复: MySQL MHA高可用数据库 MHA(MySQL High Availability)是一个开源的高可用解决方案,用于实现MySQL主从复制集群的故障自动切换。MHA的主要目的是确保MySQL数据库集…...

LVS(Layout versus schematic)比的是什么?
概述 LVS不是一个简单地将版图与电路原理图进行比较的过程,它需要分两步完成。第一步“抽取”,第二步“比较”。首先根据LVS提取规则,EDA 工具从版图中抽取出版图所确定的网表文件;然后将抽取出的网表文件与电路网表文件进行比较…...
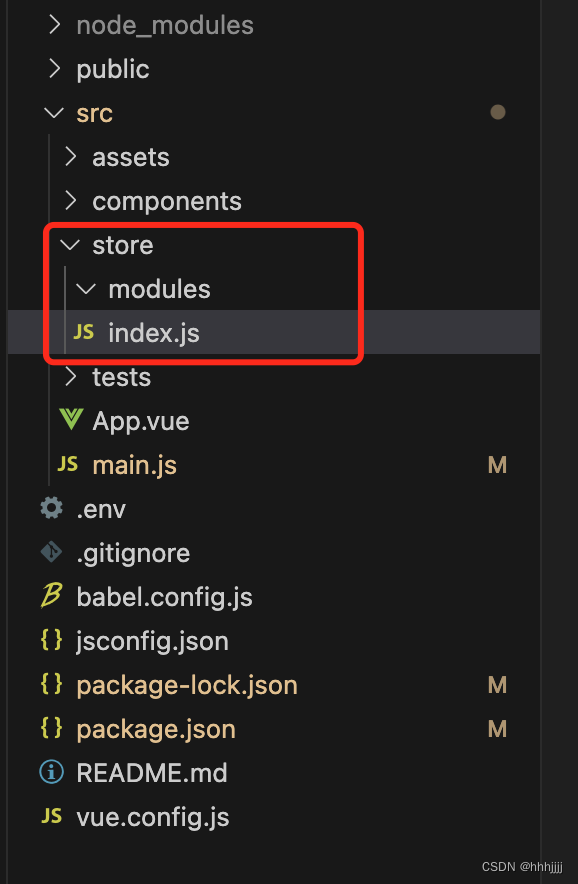
从0开始搭建基于VUE的前端项目(三) Vuex的使用与配置
准备与版本 vuex 3.6.2(https://v3.vuex.vuejs.org/zh/)概念 vuex是什么? 是用作 【状态管理】的 流程图如下 state 数据状态,成员是个对象 mapState 组件使用this.$store.state.xxx获取state里面的数据 getters 成员是个函数,方便获取state里面的数据,也可以加工数据 ma…...
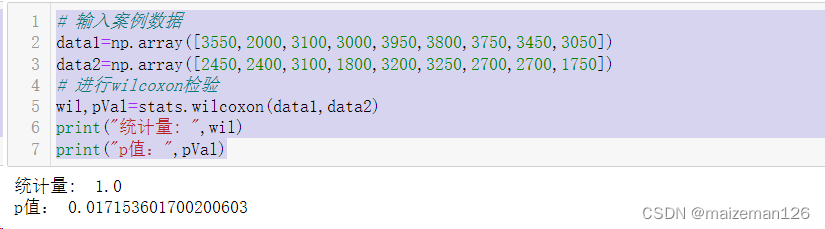
python统计分析——双样本均值比较
参考资料:python统计分析【托马斯】 1、配对样本t检验 在进行两组数据之间的比较时,有两种情况必须区分开。在第一种情况中,同一对象在不同时候的两个记录值进行相互比较。例如,用学生们进入初中时的身高和他们一年后的身高&…...
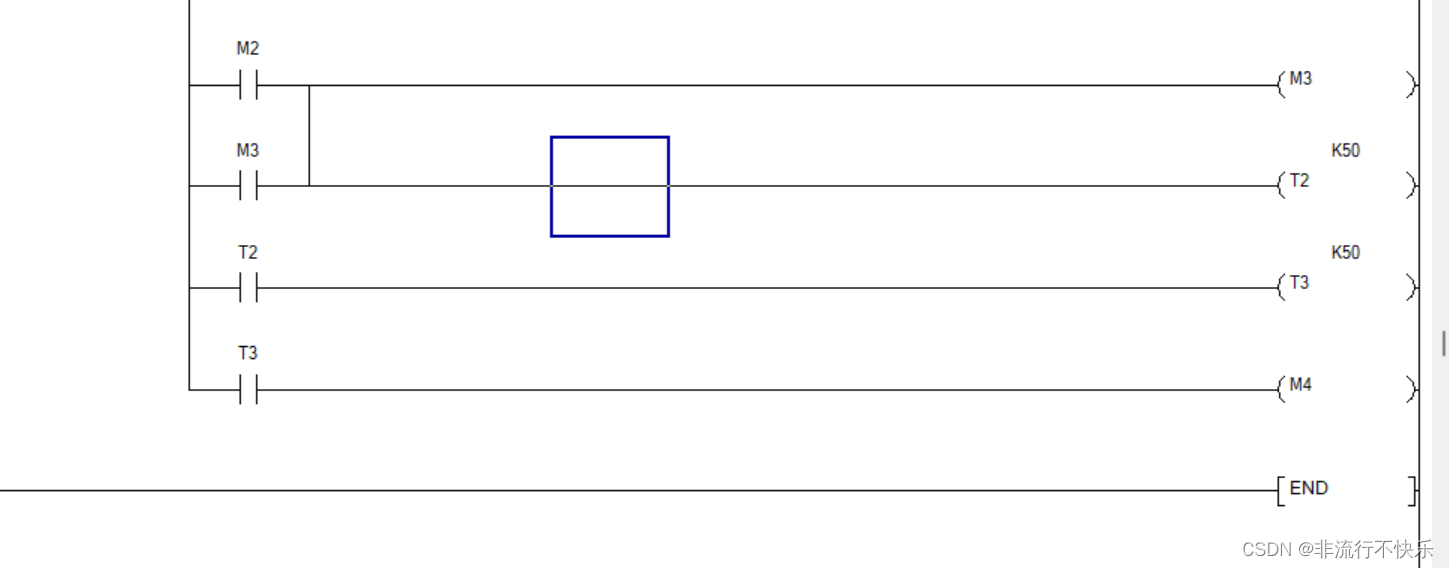
三台电机的顺启逆停
1,开启按钮输入信号是 电机一开始启动,5秒回电机2启动 ,在5秒电机三启动 关闭按钮输入时电机3关闭 ,5秒后电机2关闭 最后电机一关闭 2,思路开启按钮按下接通电机1 并且接通定时器T0 定时器T0 到时候接通电机2 并且开…...
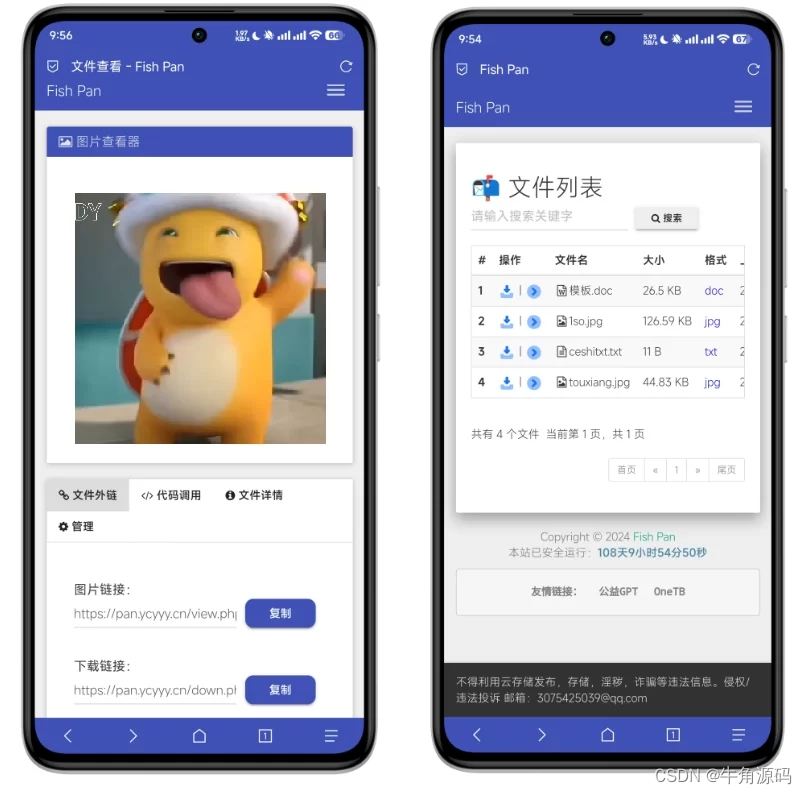
彩虹外链网盘界面UI美化版超级简洁好看
彩虹外链网盘,是一款PHP网盘与外链分享程序,支持所有格式文件的上传,可以生成文件外链、图片外链、音乐视频外链,生成外链同时自动生成相应的UBB代码和HTML代码,还可支持文本、图片、音乐、视频在线预览,这…...
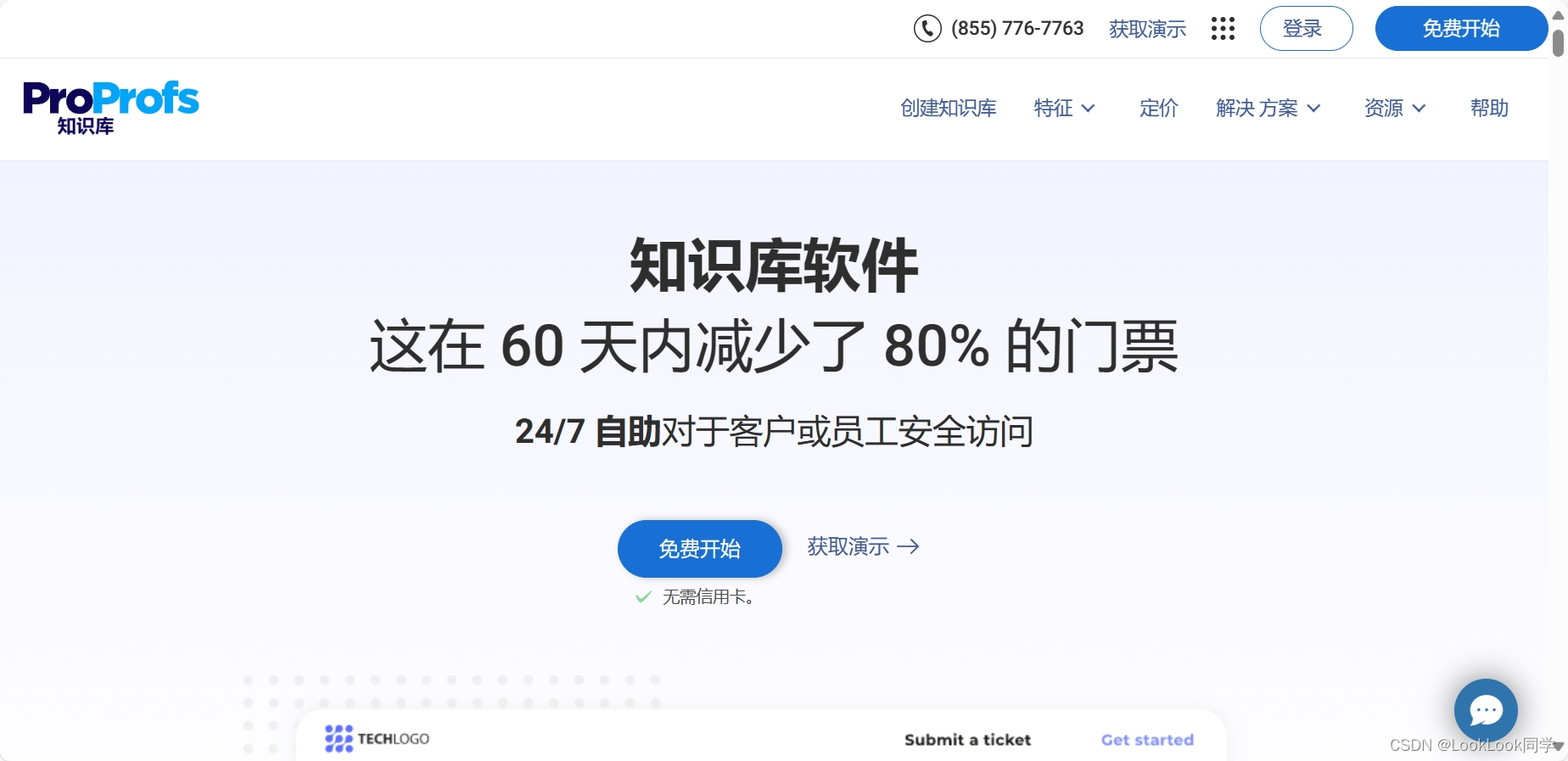
企业微信知识库:从了解到搭建的全流程
你是否也有这样的疑惑:为什么现在的企业都爱创建企业微信知识库?企业微信知识库到底有什么用?如果想要使用企业微信知识库企业应该如何创建?这就是我今天要探讨的问题,感兴趣的话一起往下看吧! | 为什么企业…...

【华为OD机试C++】合并表记录
《最新华为OD机试题目带答案解析》:最新华为OD机试题目带答案解析,语言包括C、C++、Python、Java、JavaScript等。订阅专栏,获取专栏内所有文章阅读权限,持续同步更新! 文章目录 描述输入描述输出描述示例1示例2代码描述 数据表记录包含表索引index和数值value(int范围的…...
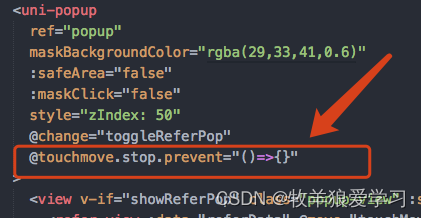
uniapp中使用u-popup组件导致的弹框下面的页面可滑动现象
添加代码: touchmove.stop.prevent"()>{}"...
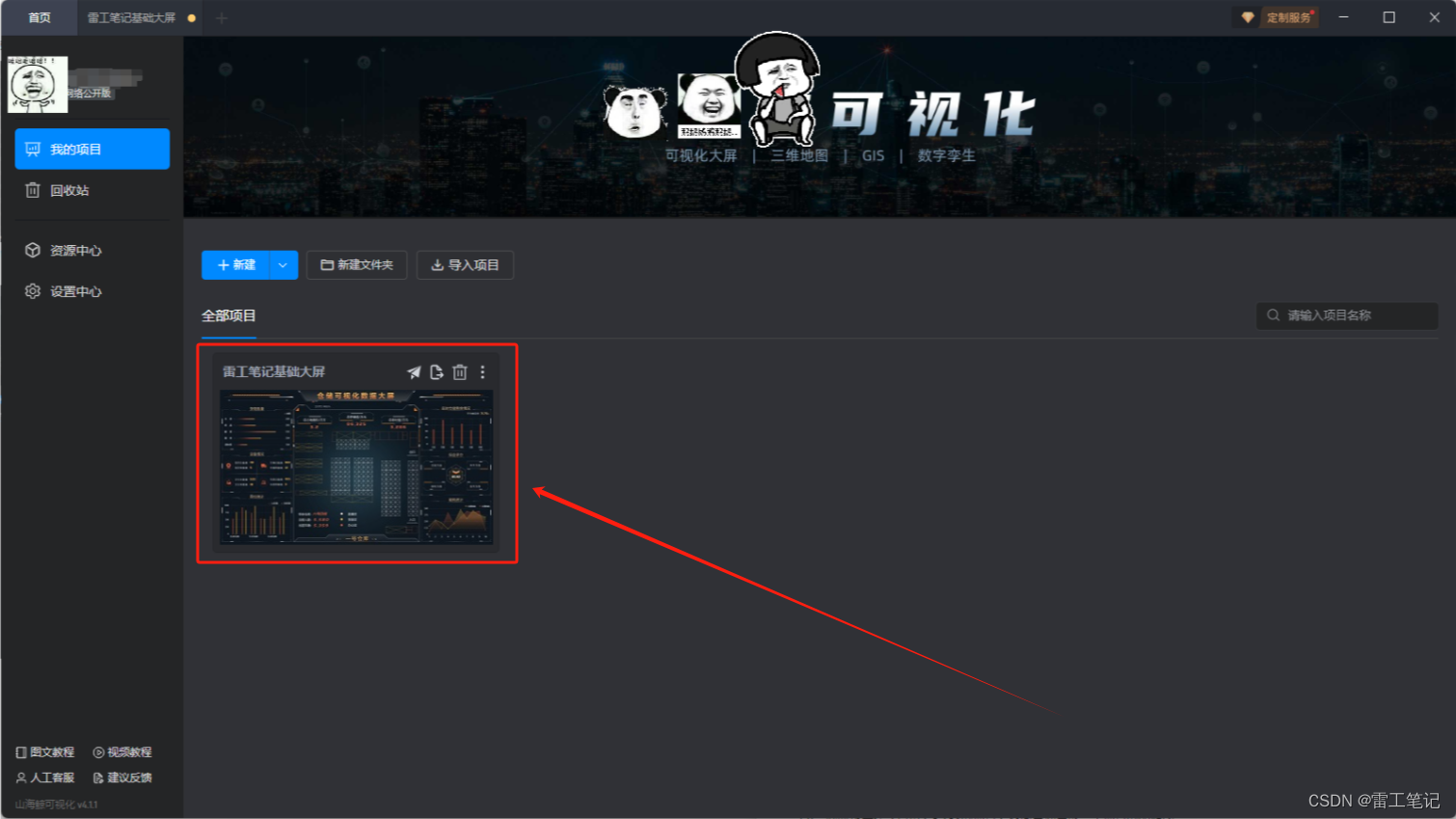
数字孪生|山海鲸可视化快速入门
哈喽,你好啊,我是雷工! 今天继续学习山海鲸可视化软件,以下为学习记录。 (一)新建项目 1.1、打开软件后,默认打开我的项目界面,初次打开需要注册,可以通过手机号快速注册。 点击“新建”按钮,新建一个项目。 1.2、根据项目需要选择一个快捷的项目模板,填写项目名称…...
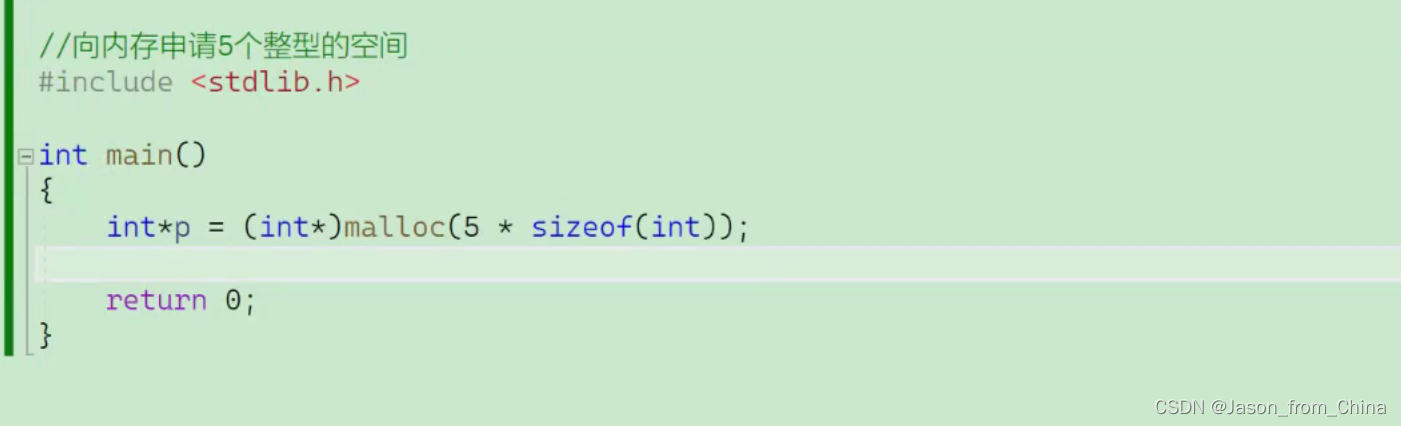
C语言-malloc(申请函数)free(释放函数)
malloc和free的语法格式 malloc 函数是 C 语言标准库中的一个重要函数,用于动态分配内存。其语法如下: void *malloc(size_t size);这里的 void * 表示返回的是一个 void 类型的指针,实际上这个指针指向的是一个 char 类型的内存块。size_t …...

2024年150道高频Java面试题(十一)
21. 什么是 Java 中的内部类?它有哪些类型? Java 中的内部类是定义在另一个类内部的类。内部类能够访问其外部类的成员,包括那些声明为私有的成员。内部类是面向对象编程中的一个特色,可以用来逻辑上组织相关的类,并且…...
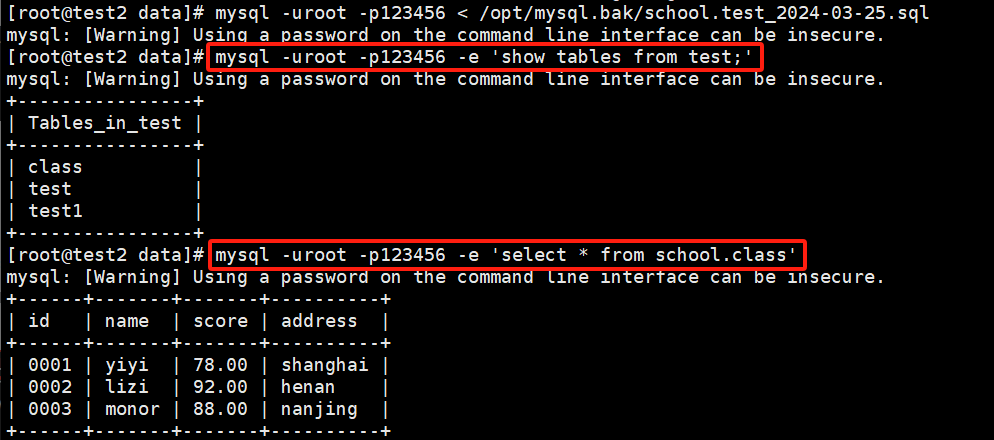
【MySQL】4.MySQL日志管理与数据库的备份和恢复
备份的目的只要是为了灾难恢复,备份还可以测试应用,回滚数据,修改和查询历史数据,审计等 日志在备份、恢复中起着重要作用 一、数据库备份的重要性 在生产环境中,数据的安全性至关重要 任何数据丢失都可能产生严重的…...

os模块篇(三)
专栏目录 文章目录 专栏目录os.putenv(key, value, /)os.setegid(egid, /)os.seteuid(euid, /)os.setgid(gid, /)os.setgroups(groups, /)os.setns(fd, nstype0)os.setpgrp()os.setpgid(pid, pgrp, /)os.setpriority(which, who, priority) os.putenv(key, value, /) os.puten…...

kvm虚拟机迁移--来自gpt
离线迁移 离线迁移KVM虚拟机主要涉及将虚拟机完全关闭,然后移动虚拟机的磁盘文件和配置文件到新的宿主机上,并在新宿主机上启动虚拟机。下面是具体的步骤和命令: 步骤 1: 关闭虚拟机 首先,在源宿主机上关闭目标虚拟机。确保虚拟…...
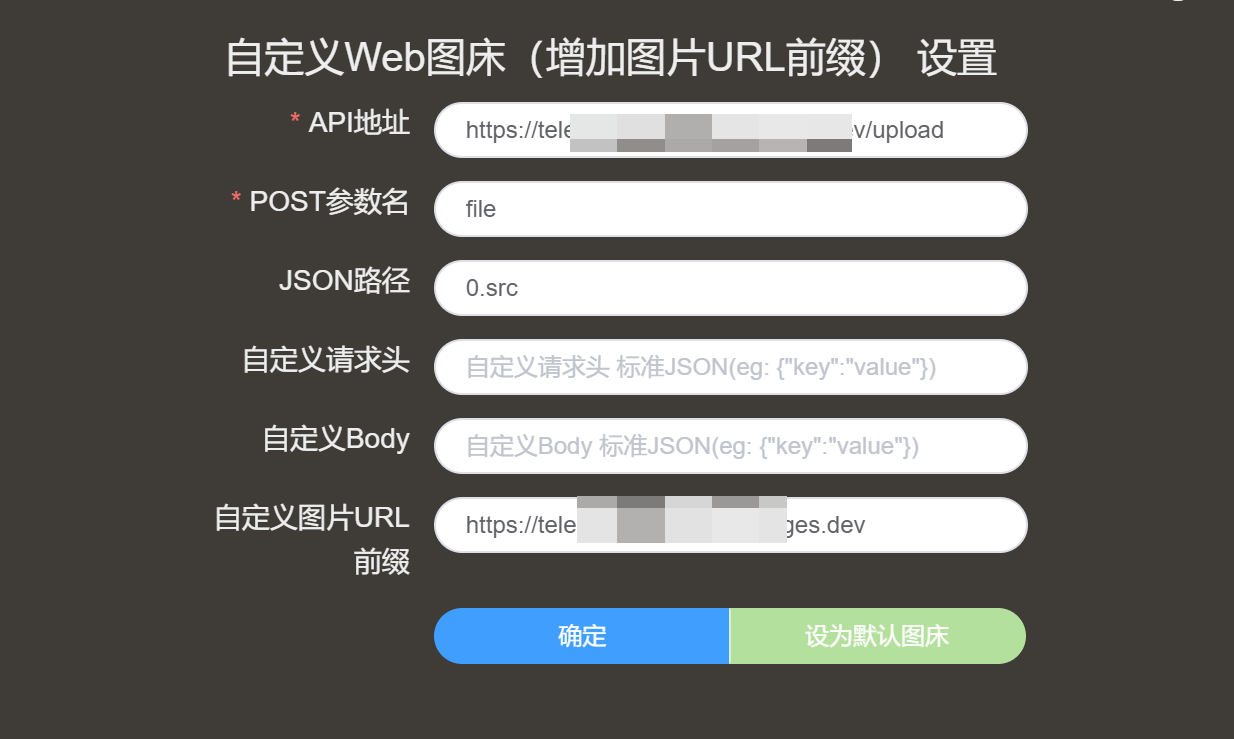
用Typora+picgo+cloudflare+Telegraph-image的免费,无需服务器,无限空间的图床搭建(避坑指南)
用TyporapicgocloudflareTelegraph-image的免费,无需服务器,无限空间的图床搭建(避坑指南) 前提:有github何cloudflare (没有的话注册也很快) 首先,是一个别人写的详细的配置流程,傻瓜式教程&am…...
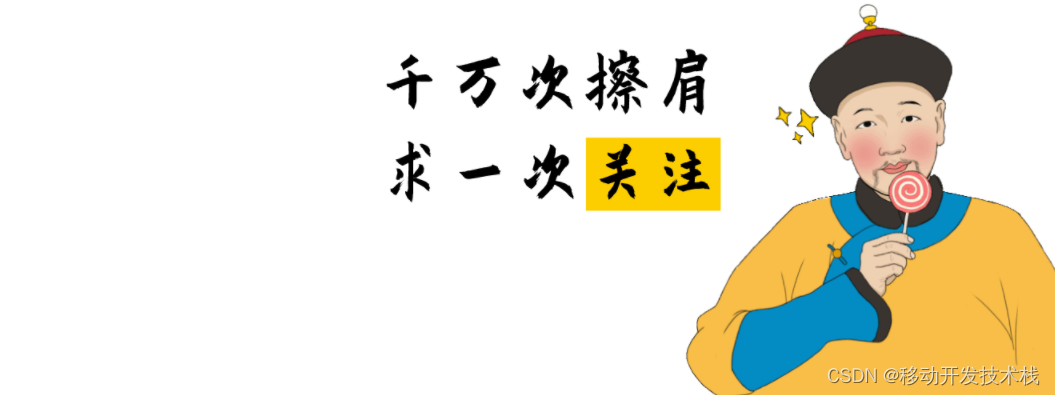
鸿蒙TypeScript开发入门学习第3天:【TS基础类型】
1、TypeScript 基础类型 TypeScript 包含的数据类型如下表: 注意: TypeScript 和 JavaScript 没有整数类型。 2、Any 类型 任意值是 TypeScript 针对编程时类型不明确的变量使用的一种数据类型,它常用于以下三种情况。 1、变量的值会动态改变时&…...
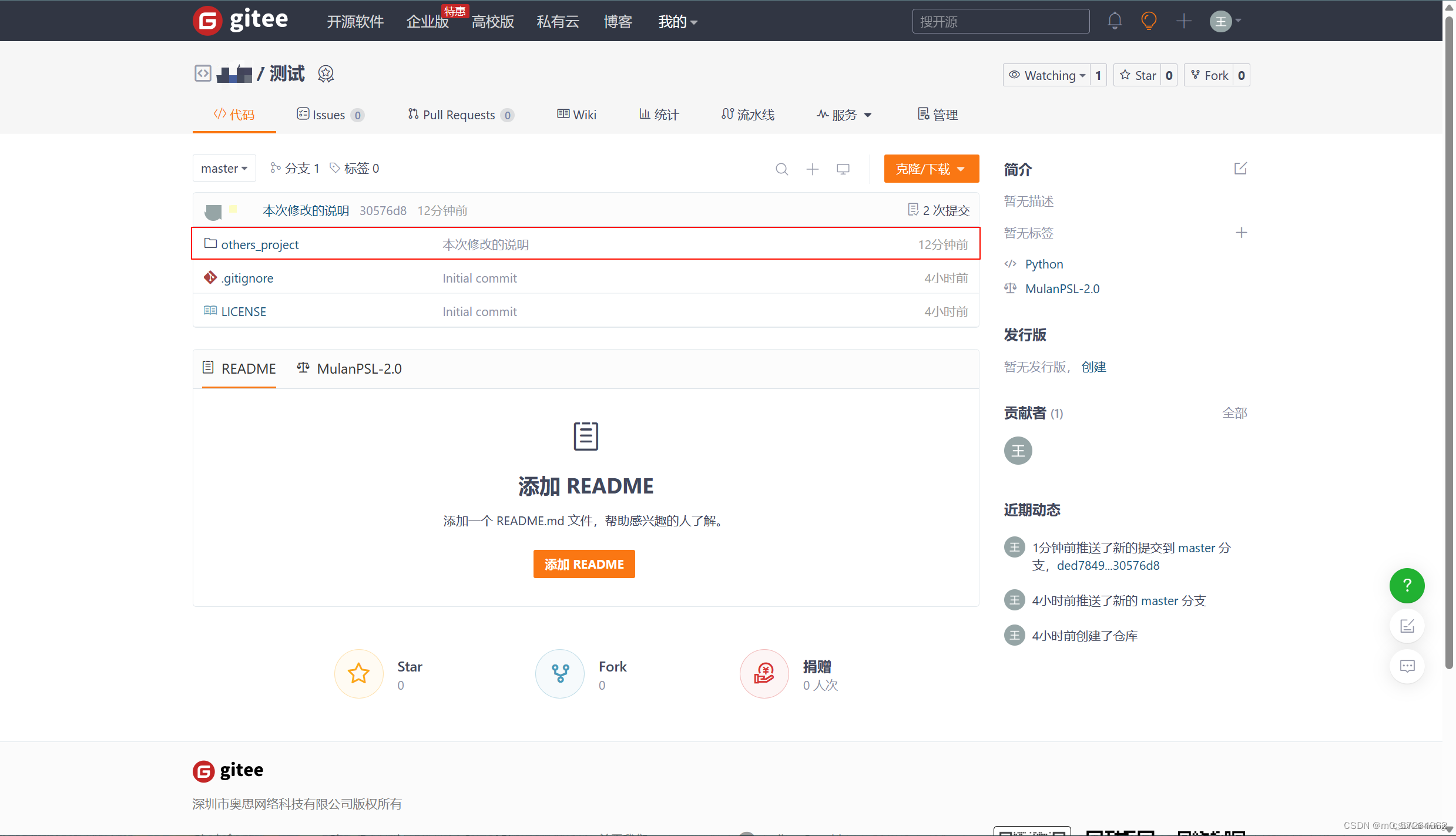
gitee 本地文件提交到仓库
一、准备工作 1.下载Git Bash Git Bash官网下载地址 http://www.git-scm.com/download/ 点此跳转 2.注册或登录gitee gitee官网地址 https://gitee.com/ 点此跳转 没有账号选择注册有账号的话直接登陆 3.在gitee中新建一个空的仓库 登陆成功后点进个人主页,点击…...

TemperatureTop-kTop-p
一、温度 在语言模型中使用温度(temperature)这个参数是为了控制文本生成过程中的随机性和可预测性。这个概念来自于统计力学中的温度概念,在那里它用来描述系统的熵(或随机性)水平。在语言模型中,输出概率…...

HTTP 协议中常用的两种请求方法:get请求与post请求
一、get请求 (1)什么是 GET 请求? GET 请求是一种向服务器请求获取资源的方式。它通过 URL 将请求参数附加到请求中,并将请求发送给服务器。GET 请求通常用于获取数据,例如读取文章、获取用户信息等操作。 在Node.j…...

作业3 主题资源网站建设/产品推广的目的和意义
NGS建库试剂 一、基本信息: 1、产品名称:SynplSeq DNA Library Prep Kit for Illumina 2、货号及规格 3、保存条件:-20℃ 二、产品描述: 1、产品介绍 文库构建是NGS测序的关键环节。SynplSeq DNA Library Prep Kit for illu…...

安徽省建设干部学校网站/整站seo
在做provider_admin的过程中,大家可以发现有比较多框架相关的配置文件和框架运行过程中的文件操作,这篇文章集中讨论下这些文件的操作,总的文件分下面的几类,其中和应用相关的按框架提供的example应用举例。 1、框架的全局配置文…...

汕头公司做网站/seo分析师
我试图在约束布局的运行时将TextViews添加到另一个之下。但我总是只有一个文本视图,其余的隐藏在它后面。我尝试了几件事情,包括链接视图,但似乎没有任何工作。Android ConstraintLayout:如何将动态视图添加到另一个下方private v…...

武汉市官方网站/推广引流渠道有哪些
http://www.cnblogs.com/mecity/archive/2011/06/11/2078527.html http://docs.mongodb.org/manual/tutorial/install-mongodb-on-windows/转载于:https://www.cnblogs.com/faxian/p/4352816.html...

山西省建设厅网站首页6/seo内部优化具体做什么
如图:有2种自定义方法,一种是改源码,一种是初始化 初始化,如下代码: var ue UE.getEditor(XXXid,{//contextMenu:[{label:, cmdName:selectall},{label:,cmdName:cleardoc,exec:function () {this.execCommand( clear…...
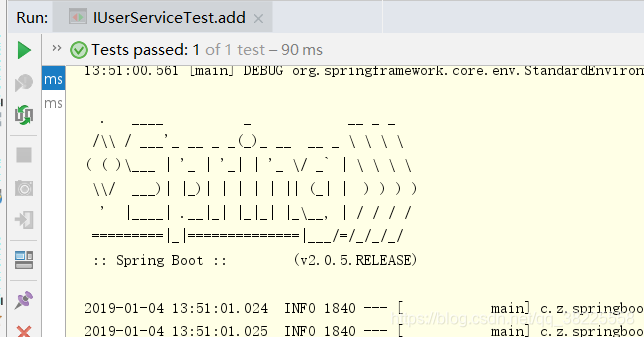
网站建设业务文案/推广普通话手抄报图片大全
如下图 :报 Caused by: java.lang.IllegalStateException: SpringJUnit4ClassRunner requires JUnit 4.12 or higher. 有道翻译一下:告诉我们junit测试jar依赖包版本太低了 然后我去查看一下自己的项目pom文件的依赖 发现是4.11版本,于是修改…...