YOLOv10改进 | 损失函数篇 | SlideLoss、FocalLoss、VFLoss分类损失函数助力细节涨点(全网最全)
一、本文介绍
本文给大家带来的是分类损失 SlideLoss、VFLoss、FocalLoss损失函数,我们之前看那的那些IoU都是边界框回归损失,和本文的修改内容并不冲突,所以大家可以知道损失函数分为两种一种是分类损失另一种是边界框回归损失,上一篇文章里面我们总结了过去百分之九十的边界框回归损失的使用方法,本文我们就来介绍几种市面上流行的和最新的分类损失函数,同时在开始讲解之前推荐一下我的专栏,本专栏的内容支持(分类、检测、分割、追踪、关键点检测),专栏目前为限时折扣,欢迎大家订阅本专栏,本专栏每周更新3-5篇最新机制,更有包含我所有改进的文件和交流群提供给大家,本文支持的损失函数共有如下图片所示
欢迎大家订阅我的专栏一起学习YOLO!
专栏回顾:YOLOv10改进系列专栏——本专栏持续复习各种顶会内容——科研必备
目录
一、本文介绍
二、原理介绍
三、核心代码
三、使用方式
3.1 修改一
3.2 修改二
3.3 使用方法
四 、本文总
二、原理介绍
其中绝大多数损失在前面我们都讲过了本文主要讲一下SlidLoss的原理,SlideLoss的损失首先是由YOLO-FaceV2提出来的。
官方论文地址: 官方论文地址点击即可跳转
官方代码地址: 官方代码地址点击即可跳转
从摘要上我们可以看出SLideLoss的出现是通过权重函数来解决简单和困难样本之间的不平衡问题题,什么是简单样本和困难样本?
样本不平衡问题是一个常见的问题,尤其是在分类和目标检测任务中。它通常指的是训练数据集中不同类别的样本数量差异很大。对于人脸检测这样的任务来说,简单样本和困难样本之间的不平衡问题可以具体描述如下:
简单样本:
- 容易被模型正确识别的样本。
- 通常出现在数据集中的数量较多。
- 特征明显,分类或检测边界清晰。
- 在训练中,这些样本会给出较低的损失值,因为模型可以轻易地正确预测它们。
困难样本:
- 模型难以正确识别的样本。
- 在数据集中相对较少,但对模型性能的提升至关重要。
- 可能由于多种原因变得难以识别,如遮挡、变形、模糊、光照变化、小尺寸或者与背景的低对比度。
- 在训练中,这些样本会产生较高的损失值,因为模型很难对它们给出准确的预测。
解决样本不平衡的问题是提高模型泛化能力的关键。如果模型大部分只见过简单样本,它可能在实际应用中遇到困难样本时性能下降。因此采用各种策略来解决这个问题,例如重采样(对困难样本进行过采样或对简单样本进行欠采样)、修改损失函数(给困难样本更高的权重),或者是设计新的模型结构来专门关注困难样本。在YOLO-FaceV2中,作者通过Slide Loss这样的权重函数来让模型在训练过程中更关注那些困难样本(这也是本文的修改内容)。
三、核心代码
使用方式看章节四
import mathclass SlideLoss(nn.Module):def __init__(self, loss_fcn):super(SlideLoss, self).__init__()self.loss_fcn = loss_fcnself.reduction = loss_fcn.reductionself.loss_fcn.reduction = 'none' # required to apply SL to each elementdef forward(self, pred, true, auto_iou=0.5):loss = self.loss_fcn(pred, true)if auto_iou < 0.2:auto_iou = 0.2b1 = true <= auto_iou - 0.1a1 = 1.0b2 = (true > (auto_iou - 0.1)) & (true < auto_iou)a2 = math.exp(1.0 - auto_iou)b3 = true >= auto_ioua3 = torch.exp(-(true - 1.0))modulating_weight = a1 * b1 + a2 * b2 + a3 * b3loss *= modulating_weightif self.reduction == 'mean':return loss.mean()elif self.reduction == 'sum':return loss.sum()else: # 'none'return lossclass Focal_Loss(nn.Module):# Wraps focal loss around existing loss_fcn(), i.e. criteria = FocalLoss(nn.BCEWithLogitsLoss(), gamma=1.5)def __init__(self, loss_fcn, gamma=1.5, alpha=0.25):super().__init__()self.loss_fcn = loss_fcn # must be nn.BCEWithLogitsLoss()self.gamma = gammaself.alpha = alphaself.reduction = loss_fcn.reductionself.loss_fcn.reduction = 'none' # required to apply FL to each elementdef forward(self, pred, true):loss = self.loss_fcn(pred, true)# p_t = torch.exp(-loss)# loss *= self.alpha * (1.000001 - p_t) ** self.gamma # non-zero power for gradient stability# TF implementation https://github.com/tensorflow/addons/blob/v0.7.1/tensorflow_addons/losses/focal_loss.pypred_prob = torch.sigmoid(pred) # prob from logitsp_t = true * pred_prob + (1 - true) * (1 - pred_prob)alpha_factor = true * self.alpha + (1 - true) * (1 - self.alpha)modulating_factor = (1.0 - p_t) ** self.gammaloss *= alpha_factor * modulating_factorif self.reduction == 'mean':return loss.mean()elif self.reduction == 'sum':return loss.sum()else: # 'none'return lossdef reduce_loss(loss, reduction):"""Reduce loss as specified.Args:loss (Tensor): Elementwise loss tensor.reduction (str): Options are "none", "mean" and "sum".Return:Tensor: Reduced loss tensor."""reduction_enum = F._Reduction.get_enum(reduction)# none: 0, elementwise_mean:1, sum: 2if reduction_enum == 0:return losselif reduction_enum == 1:return loss.mean()elif reduction_enum == 2:return loss.sum()def weight_reduce_loss(loss, weight=None, reduction='mean', avg_factor=None):"""Apply element-wise weight and reduce loss.Args:loss (Tensor): Element-wise loss.weight (Tensor): Element-wise weights.reduction (str): Same as built-in losses of PyTorch.avg_factor (float): Avarage factor when computing the mean of losses.Returns:Tensor: Processed loss values."""# if weight is specified, apply element-wise weightif weight is not None:loss = loss * weight# if avg_factor is not specified, just reduce the lossif avg_factor is None:loss = reduce_loss(loss, reduction)else:# if reduction is mean, then average the loss by avg_factorif reduction == 'mean':loss = loss.sum() / avg_factor# if reduction is 'none', then do nothing, otherwise raise an errorelif reduction != 'none':raise ValueError('avg_factor can not be used with reduction="sum"')return lossdef varifocal_loss(pred,target,weight=None,alpha=0.75,gamma=2.0,iou_weighted=True,reduction='mean',avg_factor=None):"""`Varifocal Loss <https://arxiv.org/abs/2008.13367>`_Args:pred (torch.Tensor): The prediction with shape (N, C), C is thenumber of classestarget (torch.Tensor): The learning target of the iou-awareclassification score with shape (N, C), C is the number of classes.weight (torch.Tensor, optional): The weight of loss for eachprediction. Defaults to None.alpha (float, optional): A balance factor for the negative part ofVarifocal Loss, which is different from the alpha of Focal Loss.Defaults to 0.75.gamma (float, optional): The gamma for calculating the modulatingfactor. Defaults to 2.0.iou_weighted (bool, optional): Whether to weight the loss of thepositive example with the iou target. Defaults to True.reduction (str, optional): The method used to reduce the loss intoa scalar. Defaults to 'mean'. Options are "none", "mean" and"sum".avg_factor (int, optional): Average factor that is used to averagethe loss. Defaults to None."""# pred and target should be of the same sizeassert pred.size() == target.size()pred_sigmoid = pred.sigmoid()target = target.type_as(pred)if iou_weighted:focal_weight = target * (target > 0.0).float() + \alpha * (pred_sigmoid - target).abs().pow(gamma) * \(target <= 0.0).float()else:focal_weight = (target > 0.0).float() + \alpha * (pred_sigmoid - target).abs().pow(gamma) * \(target <= 0.0).float()loss = F.binary_cross_entropy_with_logits(pred, target, reduction='none') * focal_weightloss = weight_reduce_loss(loss, weight, reduction, avg_factor)return lossclass Vari_focalLoss(nn.Module):def __init__(self,use_sigmoid=True,alpha=0.75,gamma=2.0,iou_weighted=True,reduction='sum',loss_weight=1.0):"""`Varifocal Loss <https://arxiv.org/abs/2008.13367>`_Args:use_sigmoid (bool, optional): Whether the prediction isused for sigmoid or softmax. Defaults to True.alpha (float, optional): A balance factor for the negative part ofVarifocal Loss, which is different from the alpha of FocalLoss. Defaults to 0.75.gamma (float, optional): The gamma for calculating the modulatingfactor. Defaults to 2.0.iou_weighted (bool, optional): Whether to weight the loss of thepositive examples with the iou target. Defaults to True.reduction (str, optional): The method used to reduce the loss intoa scalar. Defaults to 'mean'. Options are "none", "mean" and"sum".loss_weight (float, optional): Weight of loss. Defaults to 1.0."""super(Vari_focalLoss, self).__init__()assert use_sigmoid is True, \'Only sigmoid varifocal loss supported now.'assert alpha >= 0.0self.use_sigmoid = use_sigmoidself.alpha = alphaself.gamma = gammaself.iou_weighted = iou_weightedself.reduction = reductionself.loss_weight = loss_weightdef forward(self,pred,target,weight=None,avg_factor=None,reduction_override=None):"""Forward function.Args:pred (torch.Tensor): The prediction.target (torch.Tensor): The learning target of the prediction.weight (torch.Tensor, optional): The weight of loss for eachprediction. Defaults to None.avg_factor (int, optional): Average factor that is used to averagethe loss. Defaults to None.reduction_override (str, optional): The reduction method used tooverride the original reduction method of the loss.Options are "none", "mean" and "sum".Returns:torch.Tensor: The calculated loss"""assert reduction_override in (None, 'none', 'mean', 'sum')reduction = (reduction_override if reduction_override else self.reduction)if self.use_sigmoid:loss_cls = self.loss_weight * varifocal_loss(pred,target,weight,alpha=self.alpha,gamma=self.gamma,iou_weighted=self.iou_weighted,reduction=reduction,avg_factor=avg_factor)else:raise NotImplementedErrorreturn loss_cls
三、使用方式
3.1 修改一
我们找到如下的文件'ultralytics/utils/loss.py'然后将上面的核心代码粘贴到文件的开头位置(注意是其他模块的导入之后!)粘贴后的样子如下图所示!
3.2 修改二
第二步我门中到函数class v8DetectionLoss:(没看错V10继承的v8损失函数我们修改v8就相当于修改了v10)!我们下下面的代码全部替换class v8DetectionLoss:的内容!
class v8DetectionLoss:"""Criterion class for computing training losses."""def __init__(self, model): # model must be de-paralleled"""Initializes v8DetectionLoss with the model, defining model-related properties and BCE loss function."""device = next(model.parameters()).device # get model deviceh = model.args # hyperparametersm = model.model[-1] # Detect() moduleself.bce = nn.BCEWithLogitsLoss(reduction="none")"下面的代码注释掉就是正常的损失函数,如果不注释使用的就是使用对应的损失失函数"# self.bce = Focal_Loss(nn.BCEWithLogitsLoss(reduction='none')) # Focal# self.bce = Vari_focalLoss() # VFLoss# self.bce = SlideLoss(nn.BCEWithLogitsLoss(reduction='none')) # SlideLoss# self.bce = QualityfocalLoss() # 目前仅支持者目标检测需要注意 分割 Pose 等用不了!self.hyp = hself.stride = m.stride # model stridesself.nc = m.nc # number of classesself.no = m.nc + m.reg_max * 4self.reg_max = m.reg_maxself.device = deviceself.use_dfl = m.reg_max > 1self.assigner = TaskAlignedAssigner(topk=10, num_classes=self.nc, alpha=0.5, beta=6.0)self.bbox_loss = BboxLoss(m.reg_max - 1, use_dfl=self.use_dfl).to(device)self.proj = torch.arange(m.reg_max, dtype=torch.float, device=device)def preprocess(self, targets, batch_size, scale_tensor):"""Preprocesses the target counts and matches with the input batch size to output a tensor."""if targets.shape[0] == 0:out = torch.zeros(batch_size, 0, 5, device=self.device)else:i = targets[:, 0] # image index_, counts = i.unique(return_counts=True)counts = counts.to(dtype=torch.int32)out = torch.zeros(batch_size, counts.max(), 5, device=self.device)for j in range(batch_size):matches = i == jn = matches.sum()if n:out[j, :n] = targets[matches, 1:]out[..., 1:5] = xywh2xyxy(out[..., 1:5].mul_(scale_tensor))return outdef bbox_decode(self, anchor_points, pred_dist):"""Decode predicted object bounding box coordinates from anchor points and distribution."""if self.use_dfl:b, a, c = pred_dist.shape # batch, anchors, channelspred_dist = pred_dist.view(b, a, 4, c // 4).softmax(3).matmul(self.proj.type(pred_dist.dtype))# pred_dist = pred_dist.view(b, a, c // 4, 4).transpose(2,3).softmax(3).matmul(self.proj.type(pred_dist.dtype))# pred_dist = (pred_dist.view(b, a, c // 4, 4).softmax(2) * self.proj.type(pred_dist.dtype).view(1, 1, -1, 1)).sum(2)return dist2bbox(pred_dist, anchor_points, xywh=False)def __call__(self, preds, batch):"""Calculate the sum of the loss for box, cls and dfl multiplied by batch size."""loss = torch.zeros(3, device=self.device) # box, cls, dflfeats = preds[1] if isinstance(preds, tuple) else predspred_distri, pred_scores = torch.cat([xi.view(feats[0].shape[0], self.no, -1) for xi in feats], 2).split((self.reg_max * 4, self.nc), 1)pred_scores = pred_scores.permute(0, 2, 1).contiguous()pred_distri = pred_distri.permute(0, 2, 1).contiguous()dtype = pred_scores.dtypebatch_size = pred_scores.shape[0]imgsz = torch.tensor(feats[0].shape[2:], device=self.device, dtype=dtype) * self.stride[0] # image size (h,w)anchor_points, stride_tensor = make_anchors(feats, self.stride, 0.5)# Targetstargets = torch.cat((batch["batch_idx"].view(-1, 1), batch["cls"].view(-1, 1), batch["bboxes"]), 1)targets = self.preprocess(targets.to(self.device), batch_size, scale_tensor=imgsz[[1, 0, 1, 0]])gt_labels, gt_bboxes = targets.split((1, 4), 2) # cls, xyxymask_gt = gt_bboxes.sum(2, keepdim=True).gt_(0)# pboxespred_bboxes = self.bbox_decode(anchor_points, pred_distri) # xyxy, (b, h*w, 4)target_labels, target_bboxes, target_scores, fg_mask, _ = self.assigner(pred_scores.detach().sigmoid(), (pred_bboxes.detach() * stride_tensor).type(gt_bboxes.dtype),anchor_points * stride_tensor, gt_labels, gt_bboxes, mask_gt)target_scores_sum = max(target_scores.sum(), 1)# Cls loss# loss[1] = self.varifocal_loss(pred_scores, target_scores, target_labels) / target_scores_sum # VFL wayif isinstance(self.bce, (nn.BCEWithLogitsLoss, Vari_focalLoss, Focal_Loss)):loss[1] = self.bce(pred_scores, target_scores.to(dtype)).sum() / target_scores_sum # BCE VFLoss Focalelif isinstance(self.bce, SlideLoss):if fg_mask.sum():auto_iou = bbox_iou(pred_bboxes[fg_mask], target_bboxes[fg_mask], xywh=False, CIoU=True).mean()else:auto_iou = 0.1loss[1] = self.bce(pred_scores, target_scores.to(dtype), auto_iou).sum() / target_scores_sum # SlideLosselif isinstance(self.bce, QualityfocalLoss):if fg_mask.sum():pos_ious = bbox_iou(pred_bboxes, target_bboxes / stride_tensor, xywh=False).clamp(min=1e-6).detach()# 10.0x Faster than torch.one_hottargets_onehot = torch.zeros((target_labels.shape[0], target_labels.shape[1], self.nc),dtype=torch.int64,device=target_labels.device) # (b, h*w, 80)targets_onehot.scatter_(2, target_labels.unsqueeze(-1), 1)cls_iou_targets = pos_ious * targets_onehotfg_scores_mask = fg_mask[:, :, None].repeat(1, 1, self.nc) # (b, h*w, 80)targets_onehot_pos = torch.where(fg_scores_mask > 0, targets_onehot, 0)cls_iou_targets = torch.where(fg_scores_mask > 0, cls_iou_targets, 0)else:cls_iou_targets = torch.zeros((target_labels.shape[0], target_labels.shape[1], self.nc),dtype=torch.int64,device=target_labels.device) # (b, h*w, 80)targets_onehot_pos = torch.zeros((target_labels.shape[0], target_labels.shape[1], self.nc),dtype=torch.int64,device=target_labels.device) # (b, h*w, 80)loss[1] = self.bce(pred_scores, cls_iou_targets.to(dtype), targets_onehot_pos.to(torch.bool)).sum() / max(fg_mask.sum(), 1)else:loss[1] = self.bce(pred_scores, target_scores.to(dtype)).sum() / target_scores_sum # 确保有损失可用# Bbox lossif fg_mask.sum():target_bboxes /= stride_tensorloss[0], loss[2] = self.bbox_loss(pred_distri, pred_bboxes, anchor_points, target_bboxes, target_scores,target_scores_sum, fg_mask,((imgsz[0] ** 2 + imgsz[1] ** 2) / torch.square(stride_tensor)).repeat(1,batch_size).transpose(1, 0))loss[0] *= self.hyp.box # box gainloss[1] *= self.hyp.cls # cls gainloss[2] *= self.hyp.dfl # dfl gainreturn loss.sum() * batch_size, loss.detach() # loss(box, cls, dfl)
3.3 使用方法
将上面的代码复制粘贴之后,我门找到下图所在的位置,使用方法就是那个取消注释就是使用的就是那个!
四 、本文总结
到此本文的正式分享内容就结束了,在这里给大家推荐我的YOLOv10改进有效涨点专栏,本专栏目前为新开的平均质量分98分,后期我会根据各种最新的前沿顶会进行论文复现,也会对一些老的改进机制进行补充,如果大家觉得本文帮助到你了,订阅本专栏,关注后续更多的更新~
专栏回顾:YOLOv10改进系列专栏——本专栏持续复习各种顶会内容——科研必备
相关文章:
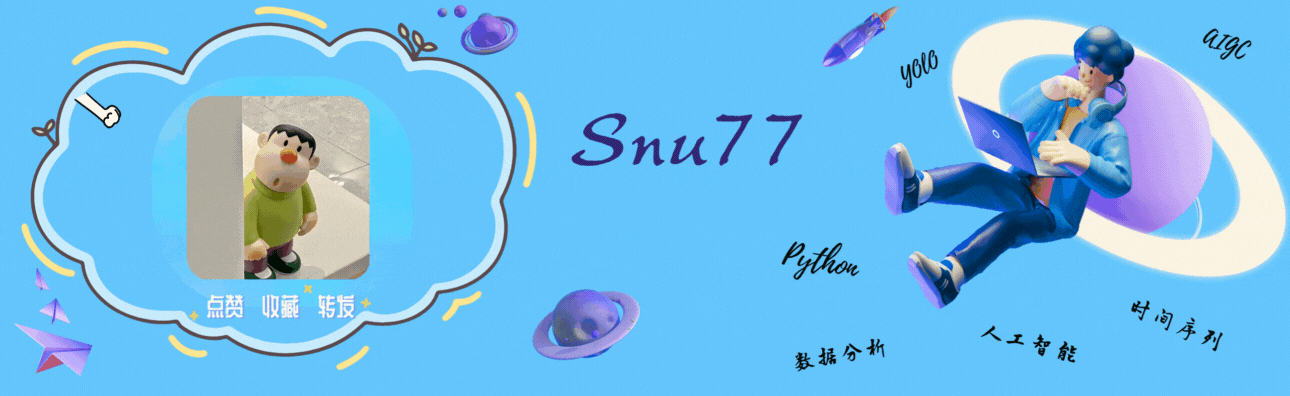
YOLOv10改进 | 损失函数篇 | SlideLoss、FocalLoss、VFLoss分类损失函数助力细节涨点(全网最全)
一、本文介绍 本文给大家带来的是分类损失 SlideLoss、VFLoss、FocalLoss损失函数,我们之前看那的那些IoU都是边界框回归损失,和本文的修改内容并不冲突,所以大家可以知道损失函数分为两种一种是分类损失另一种是边界框回归损失,…...
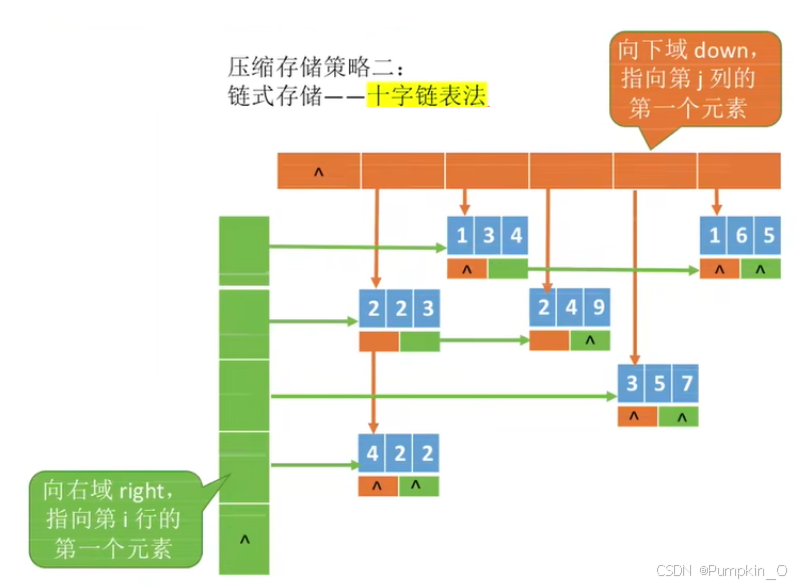
【数组、特殊矩阵的压缩存储】
目录 一、数组1.1、一维数组1.1.1 、一维数组的定义方式1.1.2、一维数组的数组名 1.2、二维数组1.2.1、二维数组的定义方式1.2.2、二维数组的数组名 二、对称矩阵的压缩存储三、三角矩阵的压缩存储四、三对角矩阵的压缩存储五、稀疏矩阵的压缩存储 一、数组 概述:数…...
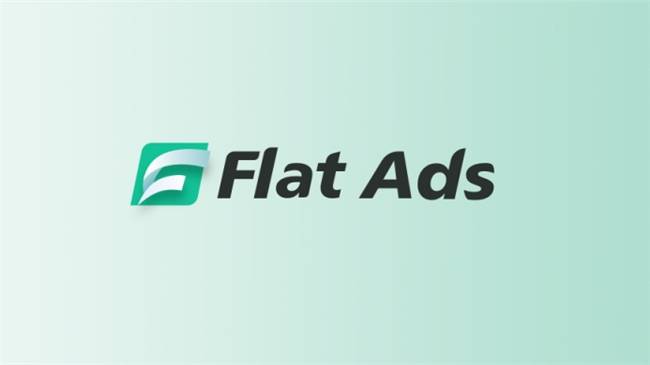
Flat Ads:金融APP海外广告投放素材的优化指南
在当今全球化的数字营销环境中,金融APP的海外营销推广已成为众多金融机构与开发者最为关注的环节之一。面对不同地域、文化及用户习惯的挑战,如何优化广告素材,以吸引目标受众的注意并促成有效转化,成为了广告主们亟待解决的问题。 作为领先的全球化营销推广平台,Flat Ads凭借…...
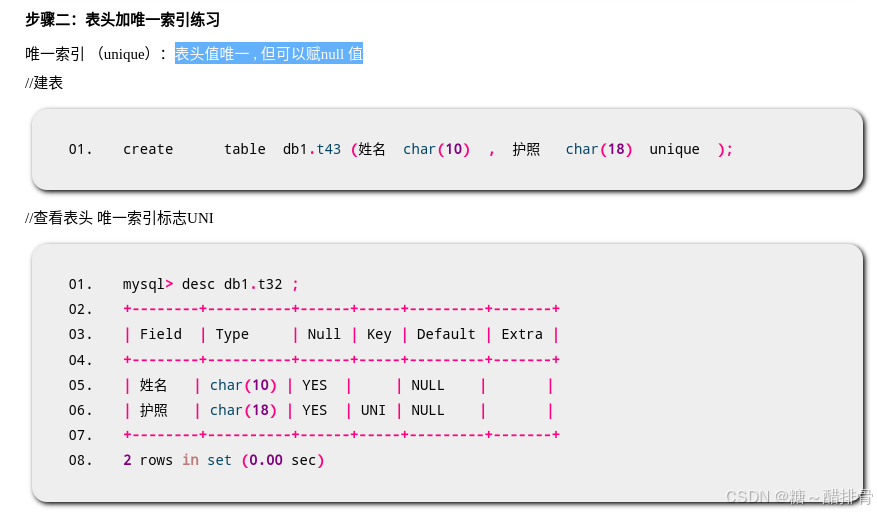
DBA 数据库管理 表管理 数据批量处理。表头约束
表管理 建库 库名命名规则:仅可以使用数字、字母、下划线、不能纯数字 不可使用MySQL命令或特殊字符 库名区分字母大小写 加if not exists 命令避免重名报错 create database if not exists gamedb; 建表 drop database if exists gamedb ; 删表…...
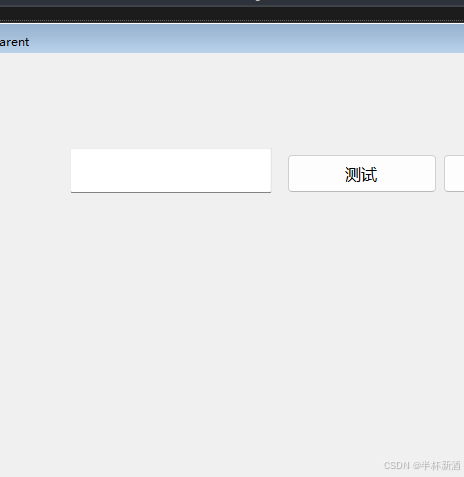
C# 上位机开发之旅-委托事件的那些事[2]
上位机项目开发过程,应该不少遇见界面同步实时刷新的情况,设备的运行情况以及设备数据的实时更新,应用场景非常之多。 那么这个时候,我们就可以用到C#语言中的一些关键功能来实现,比如事件,委托,…...
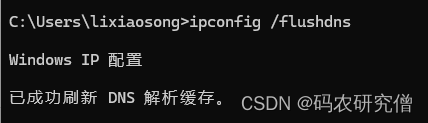
浏览器出现 502 Bad Gateway的原理分析以及解决方法
目录 前言1. 问题所示2. 原理分析3. 解决方法 前言 此类问题主要作为疑难杂症 1. 问题所示 2. 原理分析 502 Bad Gateway 错误表示服务器作为网关或代理时,从上游服务器收到了无效的响应 通常出现在充当代理或网关的网络服务器上,例如 Nginx、Apache…...
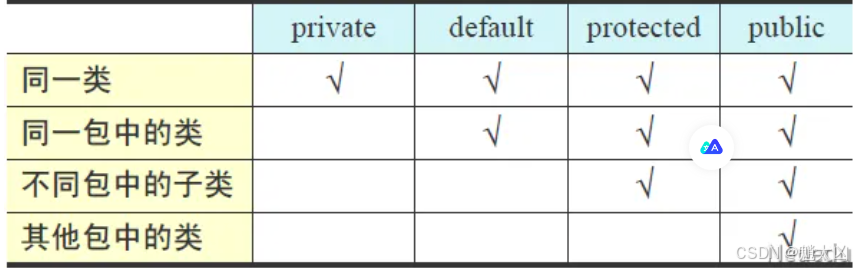
Java的高级特性
类的继承 继承是从已有的类中派生出新的类,新的类能拥有已有类的属性和行为,并且可以拓展新的属性和行为 public class 子类 extends 父类{子类类体 } 优点 代码的复用 提高编码效率 易于维护 使类与类产生关联,是多态的前提 缺点 类缺乏独…...

pip install selenium异常
error: externally-managed-environment This environment is externally managed ╰─> To install Python packages system-wide, try brew install xyz, where xyz is the package you are trying to install. If you wish to install a Python library that isnt in Ho…...

应急响应总结
应急响应 日志 windows IIS 6.0 及更早版本: C:\WINDOWS\system32\LogFiles\W3SVC[SiteID]\ IIS 7.0 及更高版本: C:\inetpub\logs\LogFiles\W3SVC[SiteID]\ Apache HTTP Server C:\Program Files (x86)\Apache Group\Apache2\logs\ 或者 C:\Prog…...

一些资源(●ˇ∀ˇ●)
GPT Kimi.ai - 帮你看更大的世界 (moonshot.cn) 文心一言 (baidu.com) 搜索AI伙伴 (baidu.com) 讯飞星火大模型-AI大语言模型-星火大模型-科大讯飞 (xfyun.cn) 秘塔AI搜索 (metaso.cn) GitHub打不开 下载Watt Toolkit...
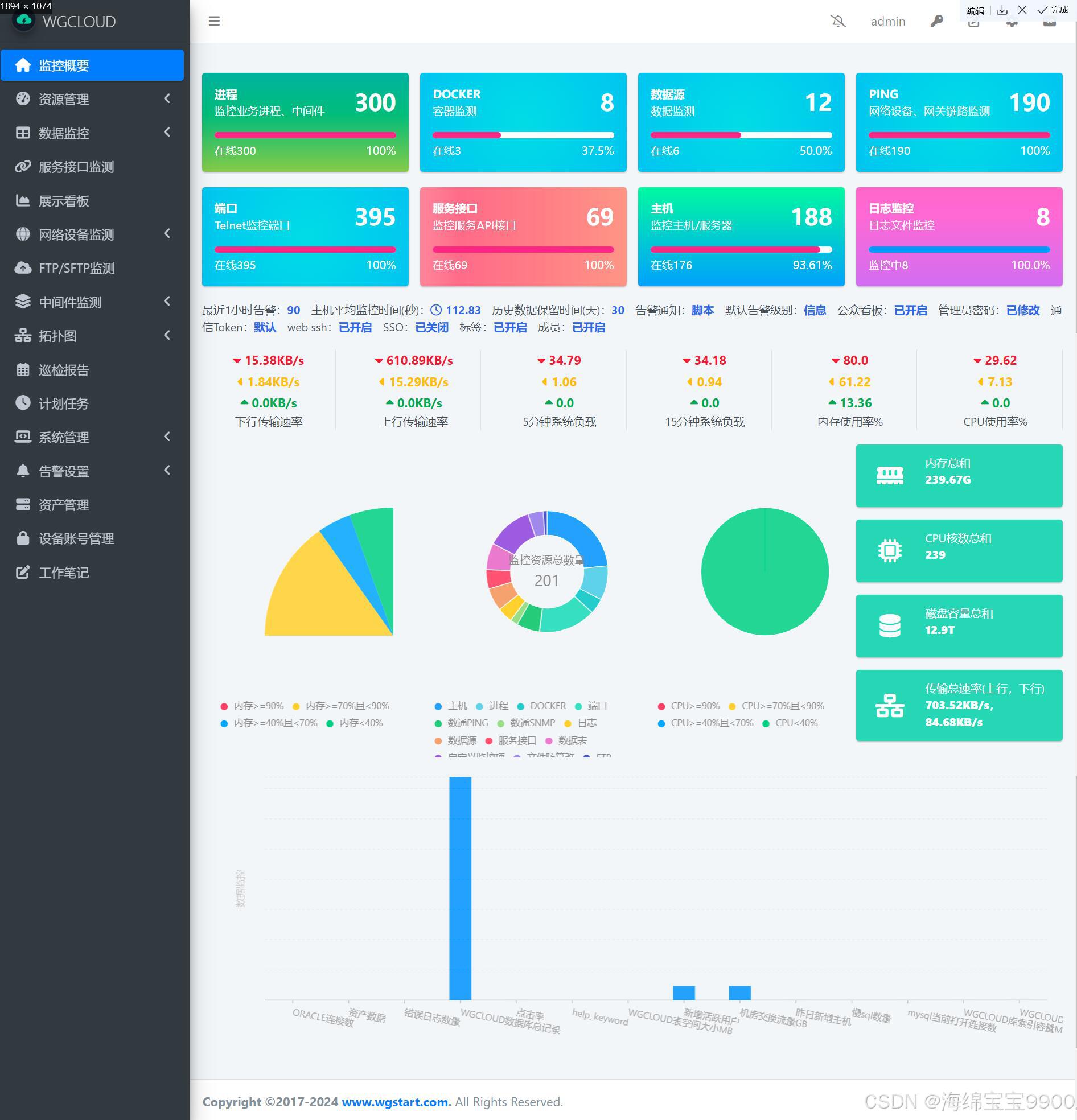
WGCLOUD的ping设备监测可以导入excel数据吗
可以的 WGCLOUD的v3.5.3版本,已经支持导入excel数据,如下说明 数通设备PING监测使用说明 - WGCLOUD...
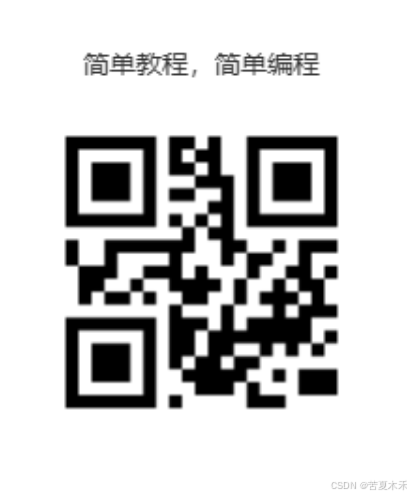
vue 画二维码及长按保存
需求 想要做如下图的二维码带文字,且能够长按保存 前期准备 一个canvas安装qrcode(命令:npm i qrcode) 画二维码及文字 初始化画布 <template><div><canvas ref"canvas" width"300" he…...
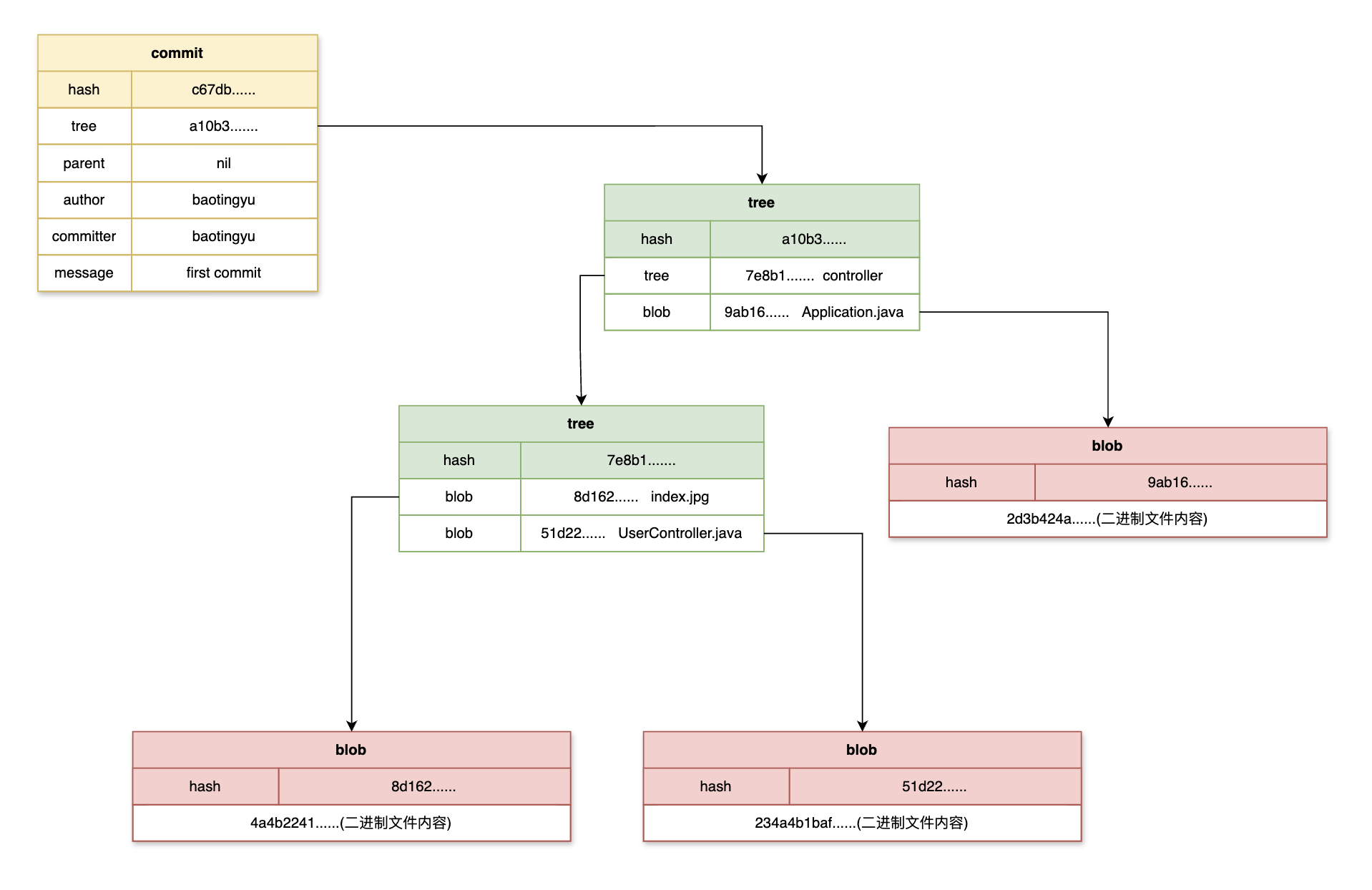
IDEA中Git常用操作及Git存储原理
Git简介与使用 Intro Git is a free and open source distributed version control system designed to handle everything from small to very large projects with speed and efficiency. Git是一款分布式版本控制系统(VSC),是团队合作开发…...
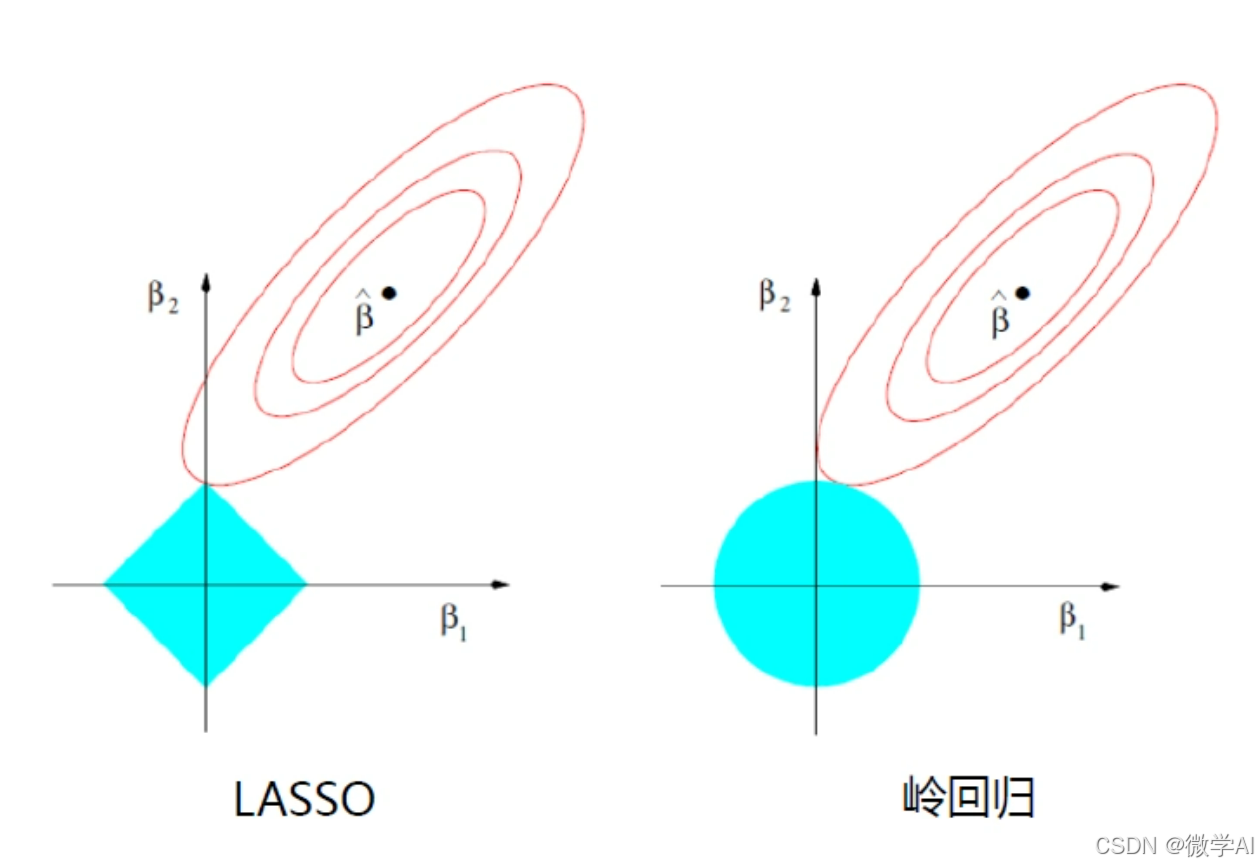
人工智能算法工程师(中级)课程4-sklearn机器学习之回归问题与代码详解
大家好,我是微学AI,今天给大家介绍一下人工智能算法工程师(中级)课程4-sklearn机器学习之回归问题与代码详解。回归分析是统计学和机器学习中的一种重要方法,用于研究因变量和自变量之间的关系。在机器学习中,回归算法被广泛应用于…...

智能制造热点词汇科普篇——工业微服务
随着互联网技术的不断发展,近十年来,微服务也逐渐走进人们的视线中来。何为微服务?让我们先来看看百度百科上的定义:微服务(或称微服务架构)是一种云原生架构方法,在单个应用中包含众多松散耦合…...
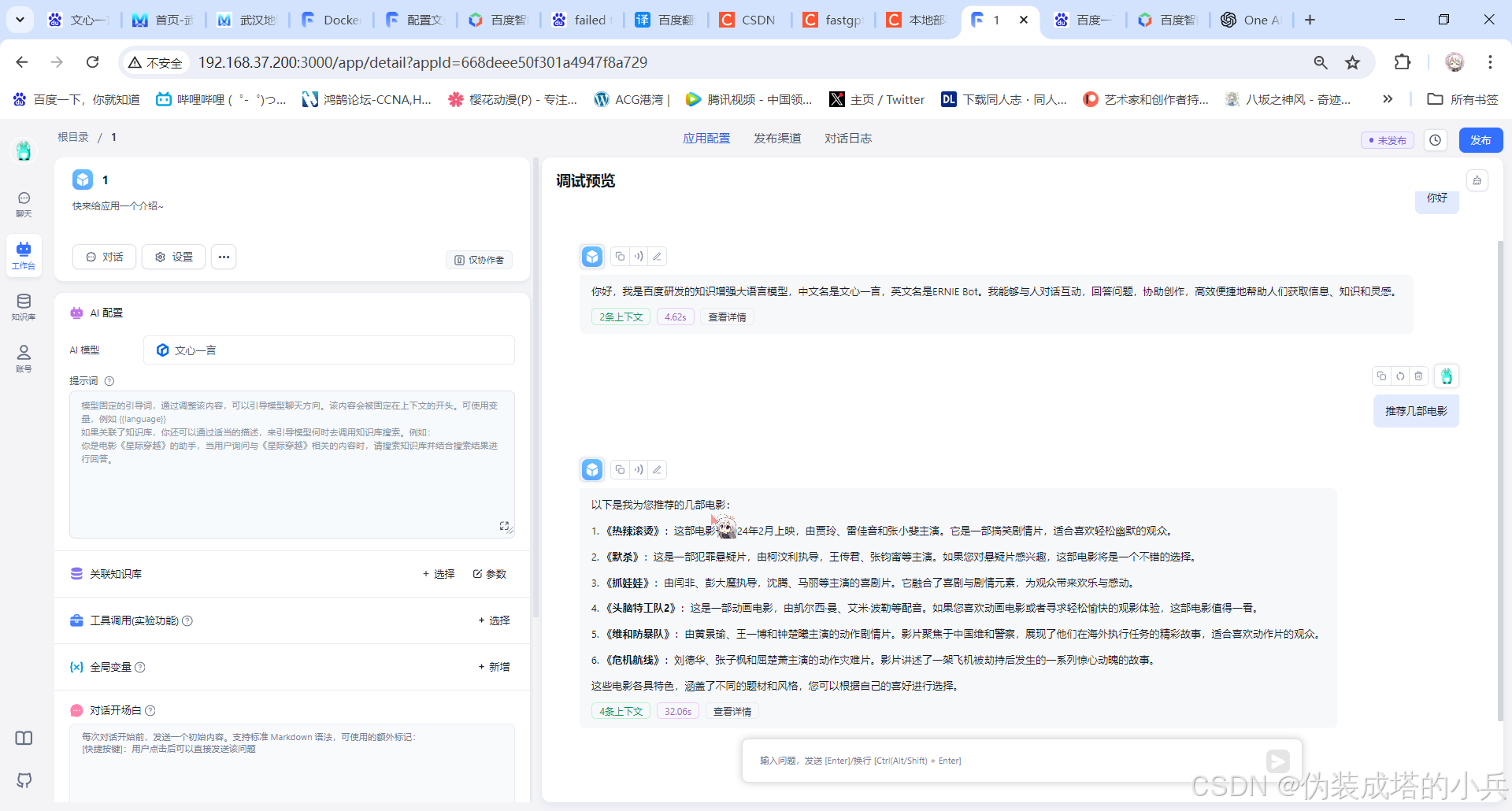
FastGPT+OneAI接入网络模型
文章目录 FastGPT连接OneAI接入网络模型1.准备工作2.开始部署2.1下载 docker-compose.yml2.2修改docker-compose.yml里的参数 3.打开FastGPT添加模型3.1打开OneAPI3.2接入网络模型3.3重启服务 FastGPT连接OneAI接入网络模型 1.准备工作 本文档参考FastGPT的官方文档 主机ip接…...
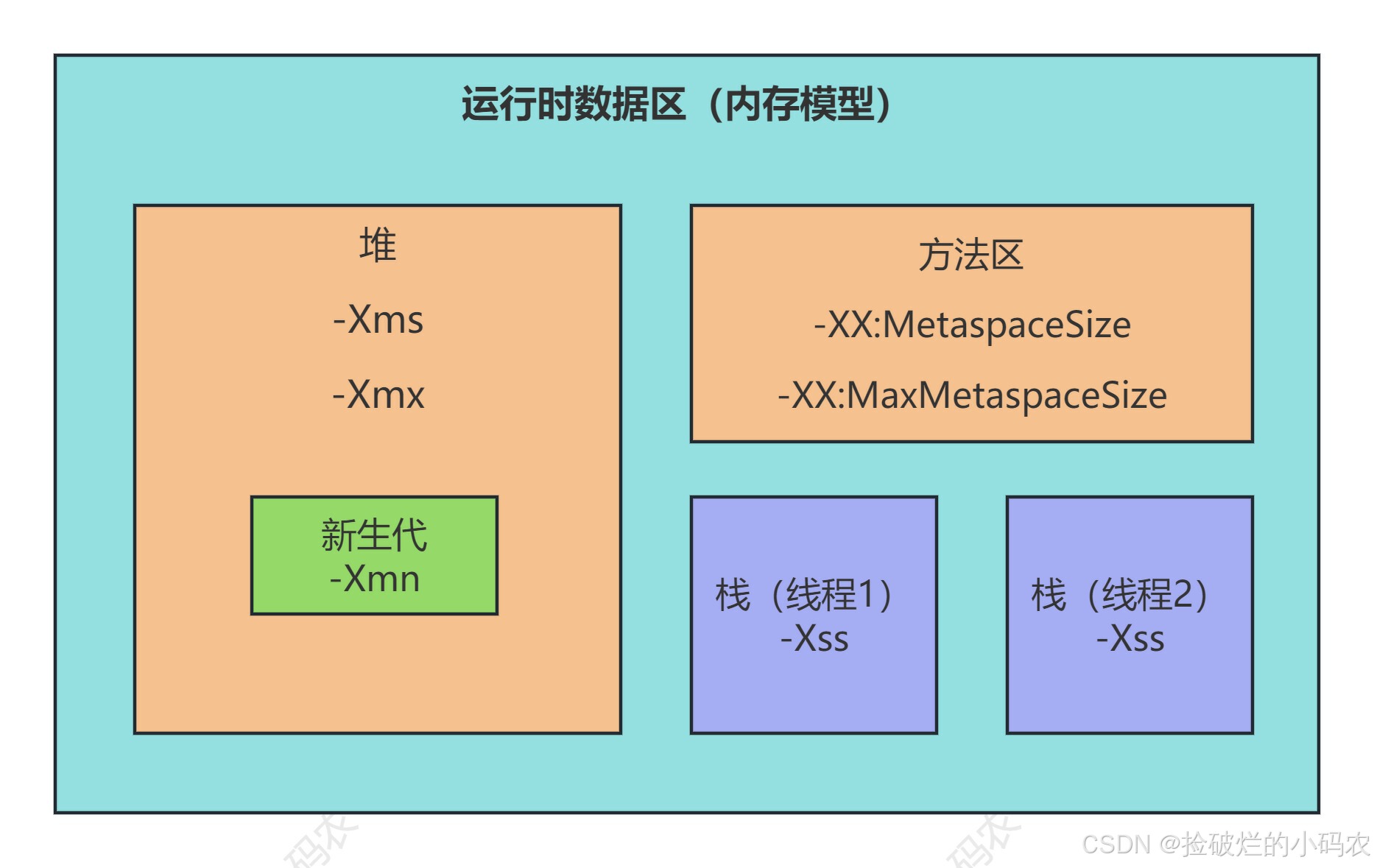
Java核心篇之JVM探秘:内存模型与管理初探
系列文章目录 第一章 Java核心篇之JVM探秘:内存模型与管理初探 第二章 Java核心篇之JVM探秘:对象创建与内存分配机制 第三章 Java核心篇之JVM探秘:垃圾回收算法与垃圾收集器 第四章 Java核心篇之JVM调优实战:Arthas工具使用及…...
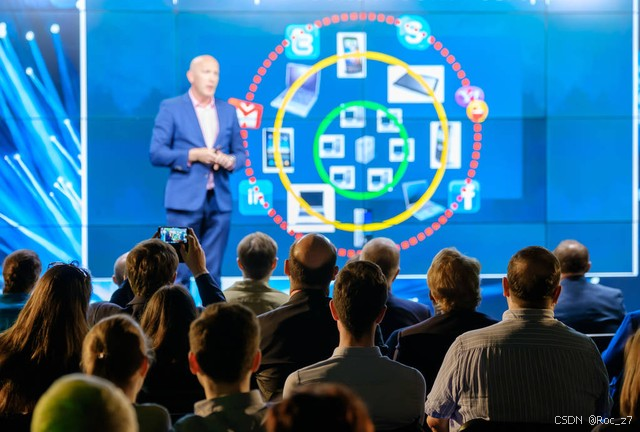
未来互联网的新篇章:深度解析Facebook的技术与战略
随着科技的飞速发展和社会的不断变迁,互联网作为全球信息交流的重要平台,正经历着前所未有的变革和演进。作为全球最大的社交媒体平台之一,Facebook不仅是人们沟通、分享和互动的重要场所,更是科技创新和数字化进程的推动者。本文…...
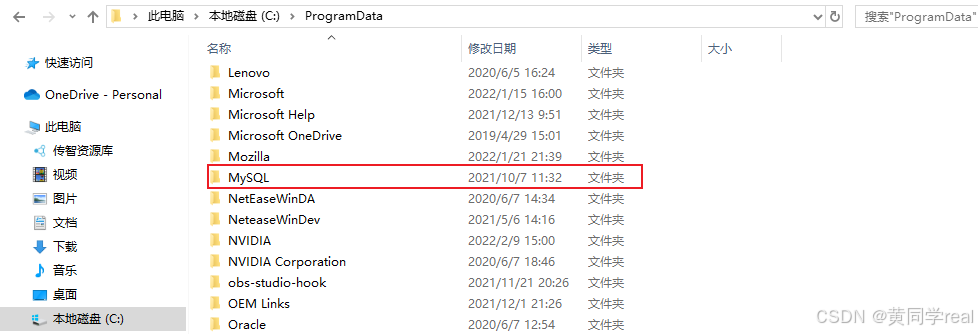
MySQL卸载 - Windows版
MySQL卸载 - Windows版 1. 停止MySQL服务 winR 打开运行,输入 services.msc 点击 “确定” 调出系统服务。 2. 卸载MySQL相关组件 打开控制面板 —> 卸载程序 —> 卸载MySQL相关所有组件 3. 删除MySQL安装目录 4. 删除MySQL数据目录 数据存放目录是在 …...
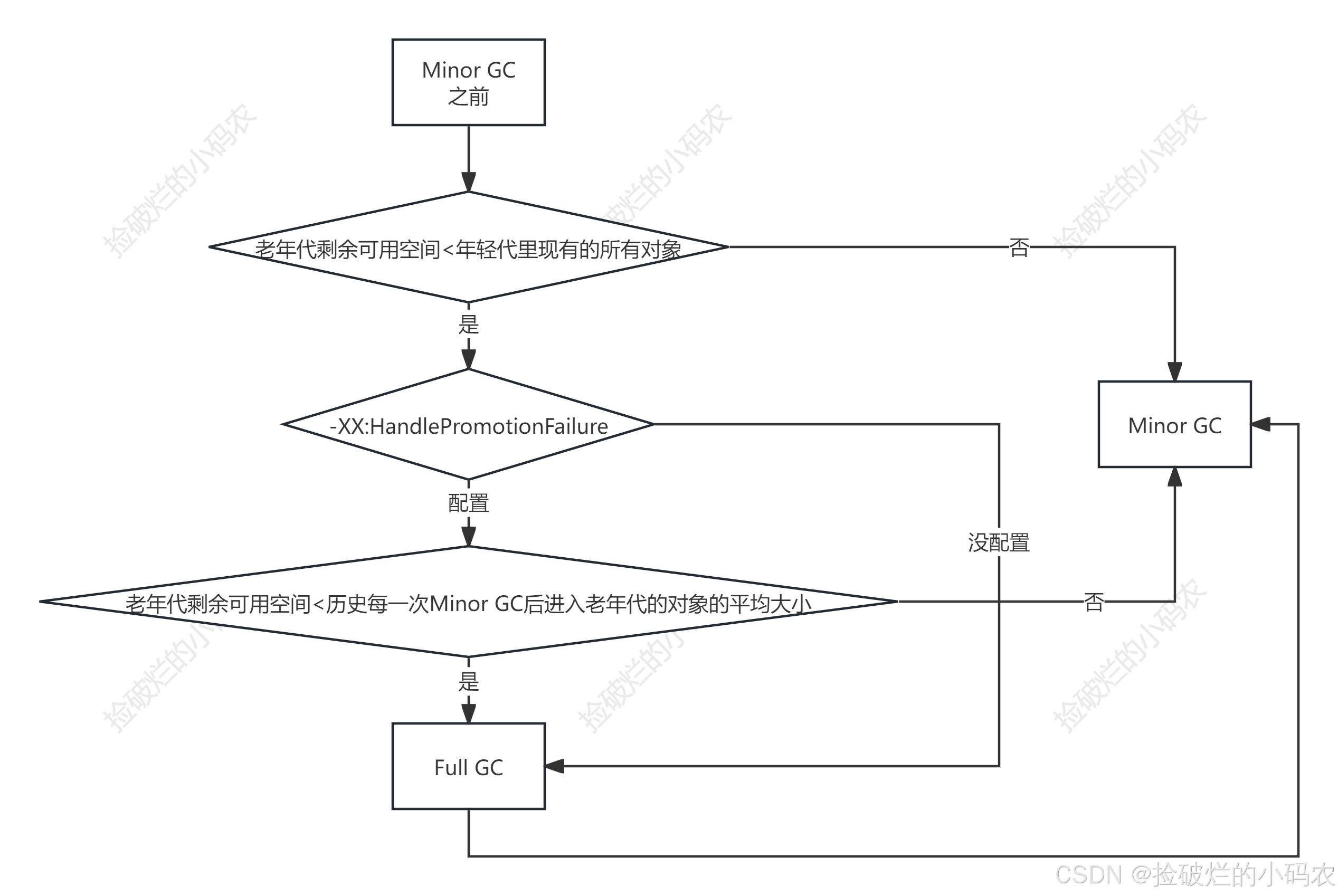
Java核心篇之JVM探秘:对象创建与内存分配机制
系列文章目录 第一章 Java核心篇之JVM探秘:内存模型与管理初探 第二章 Java核心篇之JVM探秘:对象创建与内存分配机制 第三章 Java核心篇之JVM探秘:垃圾回收算法与垃圾收集器 第四章 Java核心篇之JVM调优实战:Arthas工具使用及…...
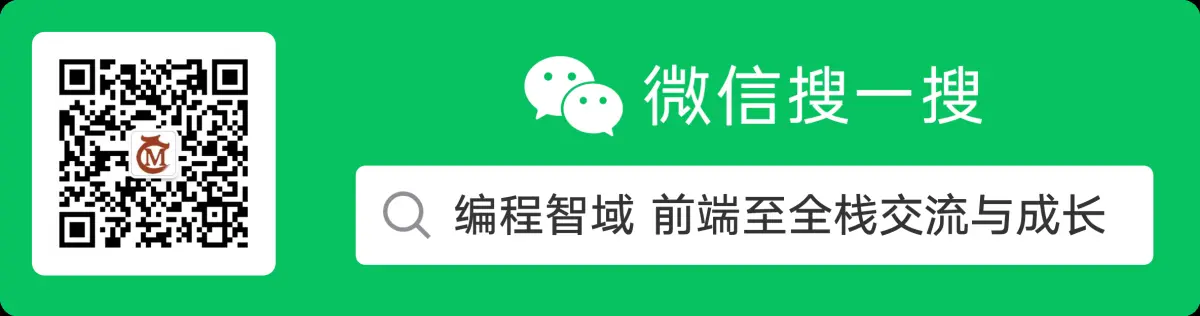
Nuxt框架中内置组件详解及使用指南(五)
title: Nuxt框架中内置组件详解及使用指南(五) date: 2024/7/10 updated: 2024/7/10 author: cmdragon excerpt: 摘要:本文详细介绍了Nuxt框架中和组件的使用方法与配置,包括安装、基本用法、属性详解、示例代码以及高级功能如…...
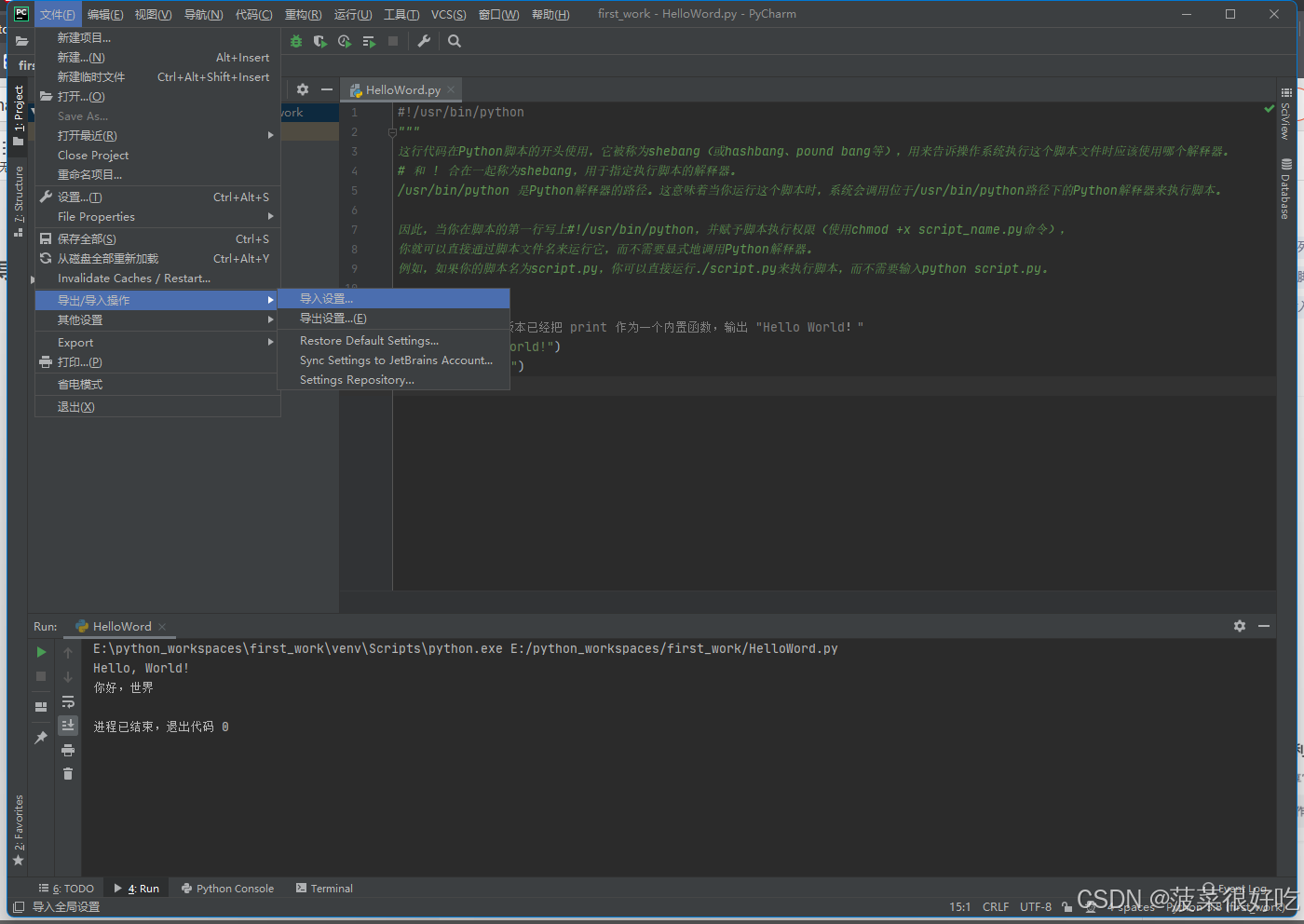
python开发遇到的坑汇总
文章目录 1.点击导入操作,所有配置全没了 1.点击导入操作,所有配置全没了 在 PyCharm 中,如果你遇到了点击导入(import)操作后,项目似乎进行了重新安装或重新部署的情况,这通常不是由简单的导入…...
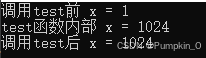
【线性表,线性表中的顺序表和链表】
目录 1、线性表的定义和基本操作1.1、线性表的定义1.2、线性表的基本操作 2、顺序表和链表的比较2.1、顺序表2.1.1、顺序表的定义和特点2.1.2、顺序表的实现(1)顺序表的静态分配:(2)顺序表的动态分配 2.1.3、顺序表的基…...

46 mysql 客户端拿不到具体的错误信息
前言 这是最近碰到的一个问题 同样的一个 环境的问题, 在正常的 mysql 环境会返回 具体的错误信息, 然后 在我的另外一个环境里面 只能返回一些 unknown error 之类的 十分抽象的环境 然后 我们这里 来看一下 具体的情况 我们这里从 错误的环境 往前推导 来查看 并解决这个…...
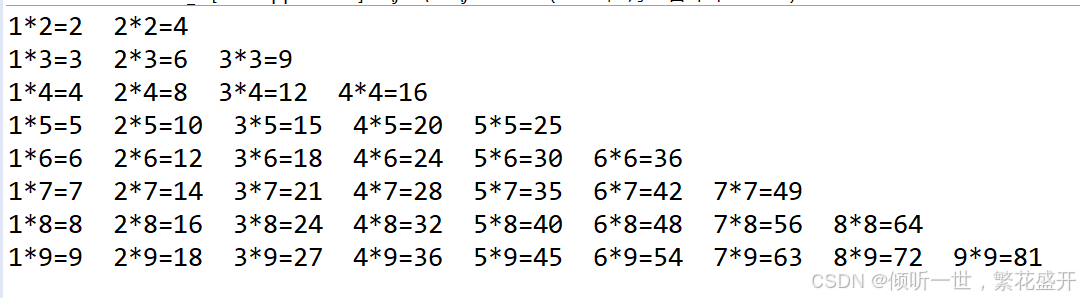
Java语言程序设计——篇三(2)
循环结构 概述1️⃣while循环例题讲解 2️⃣do-while循环例题讲解 🚩while循环与do-while循环区别3️⃣for循环例题讲解 4️⃣循环的嵌套🏮例题讲解 概述 ⭐️Java语言提供了4种循环结构: (1) while循环 (2) do-while循环 (3) for循环 (4)增…...

如何实现一个分布式锁
如何实现一个分布式锁 本篇内容主要介绍如何使用 Java 语言实现一个注解式的分布式锁,主要是通过注解AOP 环绕通知来实现。 1. 锁注解 我们首先写一个锁的注解 /*** 分布式锁注解*/ Retention(RetentionPolicy.RUNTIME) Target({ElementType.METHOD}) Documente…...
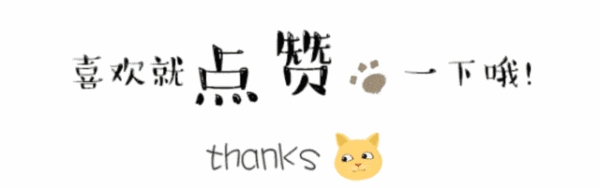
Ajax从零到实战
💝💝💝欢迎来到我的博客,很高兴能够在这里和您见面!希望您在这里可以感受到一份轻松愉快的氛围,不仅可以获得有趣的内容和知识,也可以畅所欲言、分享您的想法和见解。 非常期待和您一起在这个小…...

编程参考 - 在C++移动构造函数声明中使用noexcept
在 C 中,noexcept 是用于表示函数不抛出异常的指定符。它既可用于常规函数,也可用于特殊成员函数,包括构造函数和析构函数。使用 noexcept 可以帮助编译器进行优化,提高代码的安全性和正确性。 In C, noexcept is a specifier use…...
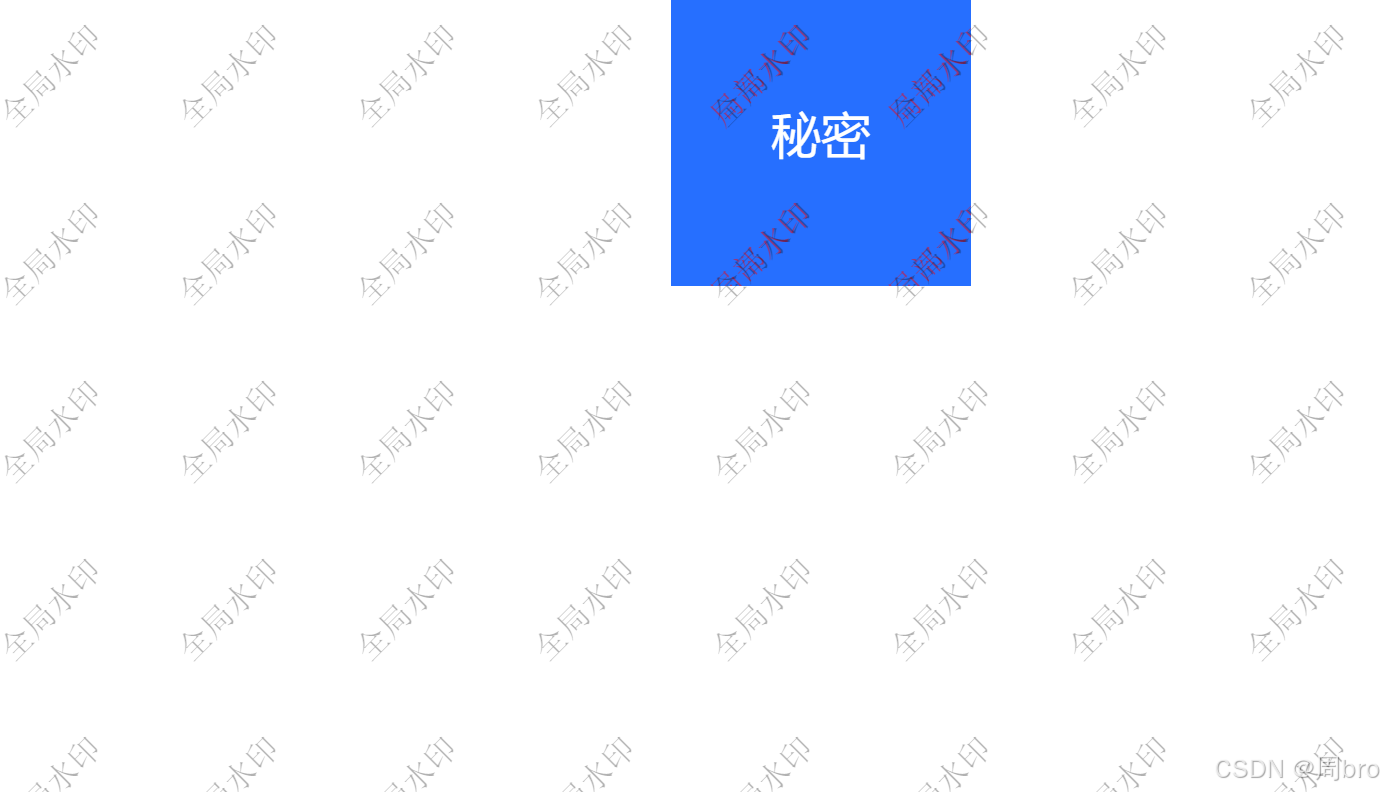
Vue2/Vue3实现全局/局部添加防篡改水印的效果。删除元素无效!更改元素属性无效!支持图片、元素、视频等等。
水印目的 版权保护:水印可以在图片、文档或视频中嵌入作者、品牌或版权所有者的信息,以防止未经授权的复制、传播或使用。当其他人使用带有水印的内容时,可以追溯到原始作者或版权所有者,从而加强版权保护。 身份识别:水印可以用作作者或品牌的标识符,使观众能够轻松识…...
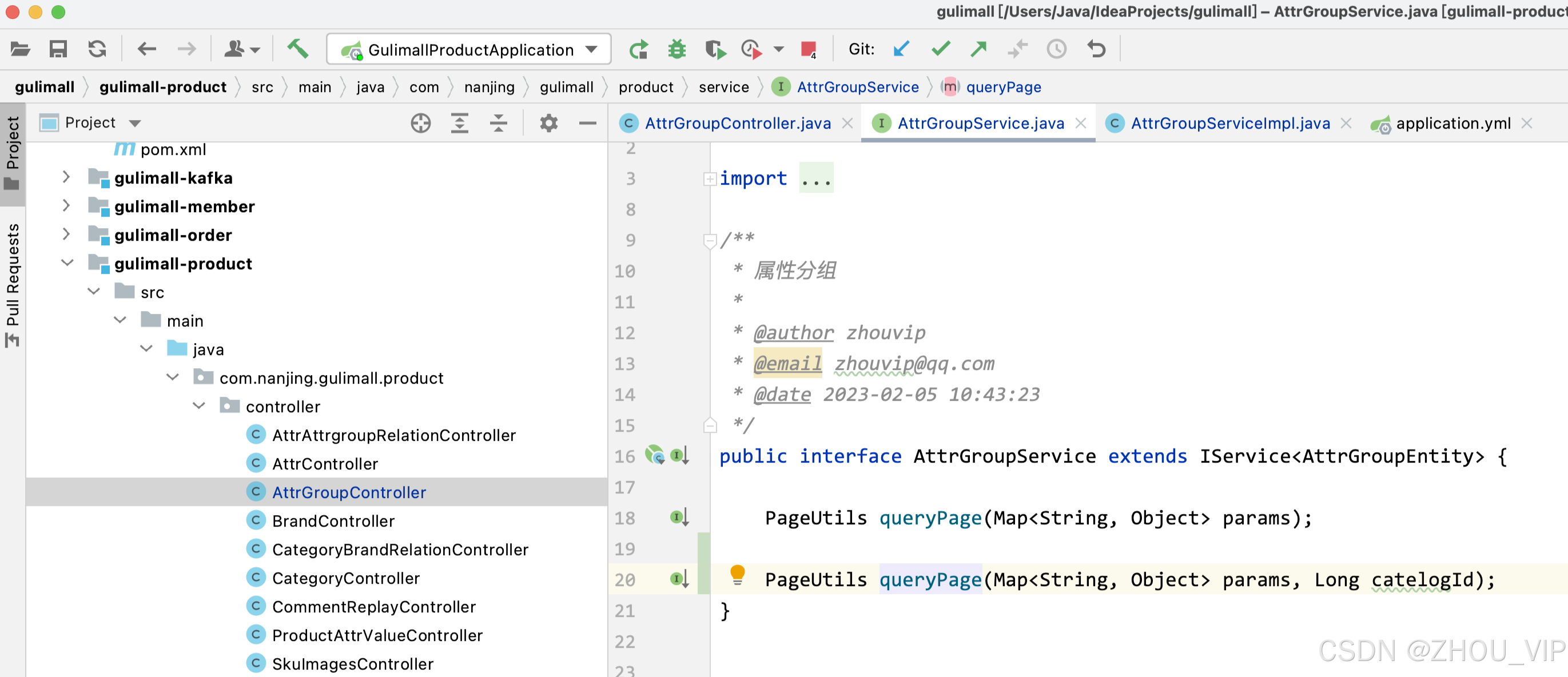
GuLi商城-商品服务-API-属性分组-获取分类属性分组
获取分类属性分组接口开发 操作的是这张表 造数据: 后台代码: @Override public PageUtils queryPage(Map<String, Object> params, Long catelogId) {//select * from pms_attr_group where catelog_id=? and (attr_group_id=key or attr_group_name like %key%)Stri…...