qwen2 VL 多模态图文模型;图像、视频使用案例
参考:
https://huggingface.co/Qwen/Qwen2-VL-2B-Instruct
模型:
export HF_ENDPOINT=https://hf-mirror.comhuggingface-cli download --resume-download --local-dir-use-symlinks False Qwen/Qwen2-VL-2B-Instruct --local-dir qwen2-vl
安装:
transformers-4.45.0.dev0
accelerate-0.34.2 safetensors-0.4.5
pip install git+https://github.com/huggingface/transformers
pip install 'accelerate>=0.26.0'
代码:
单张图片
from PIL import Image
import requests
import torch
from torchvision import io
from typing import Dict
from transformers import Qwen2VLForConditionalGeneration, AutoTokenizer, AutoProcessor# Load the model in half-precision on the available device(s)
model = Qwen2VLForConditionalGeneration.from_pretrained("/ai/qwen2-vl", torch_dtype="auto", device_map="auto"
)
processor = AutoProcessor.from_pretrained("/ai/qwen2-vl")# Image
url = "https://qianwen-res.oss-cn-beijing.aliyuncs.com/Qwen-VL/assets/demo.jpeg"
image = Image.open(requests.get(url, stream=True).raw)conversation = [{"role": "user","content": [{"type": "image",},{"type": "text", "text": "Describe this image."},],}
]# Preprocess the inputs
text_prompt = processor.apply_chat_template(conversation, add_generation_prompt=True)
# Excepted output: '<|im_start|>system\nYou are a helpful assistant.<|im_end|>\n<|im_start|>user\n<|vision_start|><|image_pad|><|vision_end|>Describe this image.<|im_end|>\n<|im_start|>assistant\n'inputs = processor(text=[text_prompt], images=[image], padding=True, return_tensors="pt"
)
inputs = inputs.to("cuda")# Inference: Generation of the output
output_ids = model.generate(**inputs, max_new_tokens=128)
generated_ids = [output_ids[len(input_ids) :]for input_ids, output_ids in zip(inputs.input_ids, output_ids)
]
output_text = processor.batch_decode(generated_ids, skip_special_tokens=True, clean_up_tokenization_spaces=True
)
print(output_text)
这是图片:
中文问
# Image
url = "https://qianwen-res.oss-cn-beijing.aliyuncs.com/Qwen-VL/assets/demo.jpeg"
image = Image.open(requests.get(url, stream=True).raw)conversation = [{"role": "user","content": [{"type": "image",},{"type": "text", "text": "描述下这张图片."},],}
]# Preprocess the inputs
text_prompt = processor.apply_chat_template(conversation, add_generation_prompt=True)
# Excepted output: '<|im_start|>system\nYou are a helpful assistant.<|im_end|>\n<|im_start|>user\n<|vision_start|><|image_pad|><|vision_end|>Describe this image.<|im_end|>\n<|im_start|>assistant\n'inputs = processor(text=[text_prompt], images=[image], padding=True, return_tensors="pt"
)
inputs = inputs.to("cuda")
# Inference: Generation of the output
output_ids = model.generate(**inputs, max_new_tokens=128)
generated_ids = [output_ids[len(input_ids) :]for input_ids, output_ids in zip(inputs.input_ids, output_ids)
]
output_text = processor.batch_decode(generated_ids, skip_special_tokens=True, clean_up_tokenization_spaces=True
)
print(output_text)
多张图片
def load_images(image_info):images = []for info in image_info:if "image" in info:if info["image"].startswith("http"):image = Image.open(requests.get(info["image"], stream=True).raw)else:image = Image.open(info["image"])images.append(image)return images# Messages containing multiple images and a text query
messages = [{"role": "user","content": [{"type": "image", "image": "/ai/fight.png"},{"type": "image", "image": "/ai/long.png"},{"type": "text", "text": "描述下这两张图片"},],}
]# Load images
image_info = messages[0]["content"][:2] # Extract image info from the message
images = load_images(image_info)# Preprocess the inputs
text_prompt = processor.apply_chat_template(messages, add_generation_prompt=True)inputs = processor(text=[text_prompt], images=images, padding=True, return_tensors="pt"
)
inputs = inputs.to("cuda")# Inference: Generation of the output
output_ids = model.generate(**inputs, max_new_tokens=128)
generated_ids = [output_ids[len(input_ids) :]for input_ids, output_ids in zip(inputs.input_ids, output_ids)
]
output_text = processor.batch_decode(generated_ids, skip_special_tokens=True, clean_up_tokenization_spaces=True
)
print(output_text)
视频
安装
pip install qwen-vl-utils
from qwen_vl_utils import process_vision_info# Messages containing a images list as a video and a text query
messages = [{"role": "user","content": [{"type": "video","video": ["file:///path/to/frame1.jpg","file:///path/to/frame2.jpg","file:///path/to/frame3.jpg","file:///path/to/frame4.jpg",],"fps": 1.0,},{"type": "text", "text": "Describe this video."},],}
]
# Messages containing a video and a text query
messages = [{"role": "user","content": [{"type": "video","video": "/ai/血液从上肢流入上腔静脉.mp4","max_pixels": 360 * 420,"fps": 1.0,},{"type": "text", "text": "描述下这个视频"},],}
]# Preparation for inference
text = processor.apply_chat_template(messages, tokenize=False, add_generation_prompt=True
)
image_inputs, video_inputs = process_vision_info(messages)
inputs = processor(text=[text],images=image_inputs,videos=video_inputs,padding=True,return_tensors="pt",
)
inputs = inputs.to("cuda")# Inference
generated_ids = model.generate(**inputs, max_new_tokens=128)
generated_ids_trimmed = [out_ids[len(in_ids) :] for in_ids, out_ids in zip(inputs.input_ids, generated_ids)
]
output_text = processor.batch_decode(generated_ids_trimmed, skip_special_tokens=True, clean_up_tokenization_spaces=False
)
print(output_text)
相关文章:
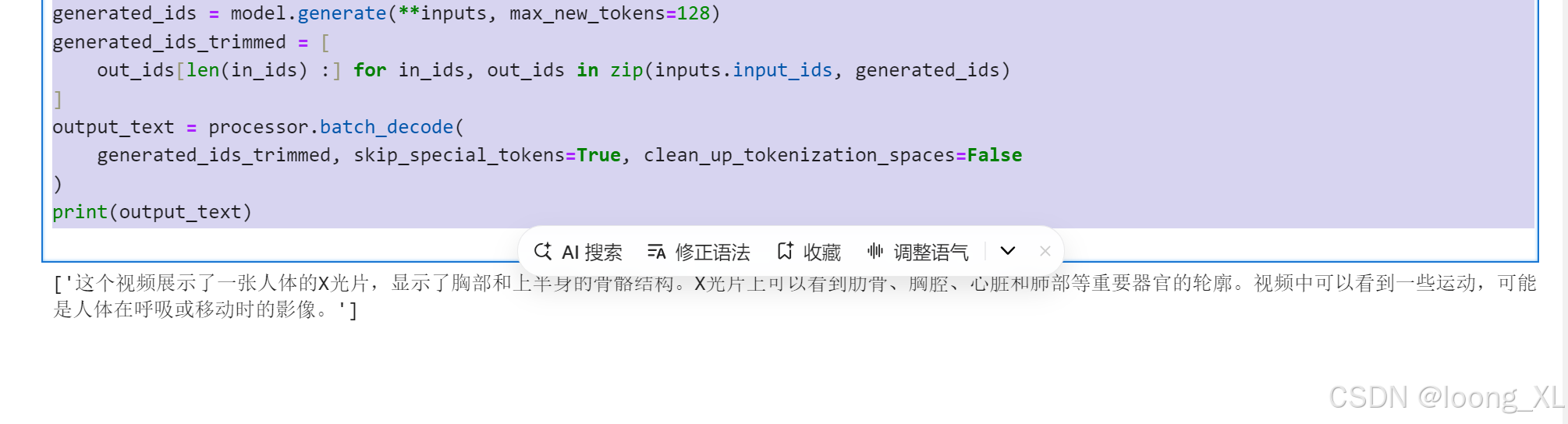
qwen2 VL 多模态图文模型;图像、视频使用案例
参考: https://huggingface.co/Qwen/Qwen2-VL-2B-Instruct 模型: export HF_ENDPOINThttps://hf-mirror.comhuggingface-cli download --resume-download --local-dir-use-symlinks False Qwen/Qwen2-VL-2B-Instruct --local-dir qwen2-vl安装&#x…...

ASPICE评估:汽车软件质量的守护神
随着汽车行业的快速发展,车载软件系统的复杂性和重要性日益凸显。为了确保汽车软件的质量和安全性, 汽车行业引入了ASPICE(Automotive SPICE)评估作为评价软件开发团队研发能力的重要工具。 本文将详细介绍ASPICE评估的概念、过…...
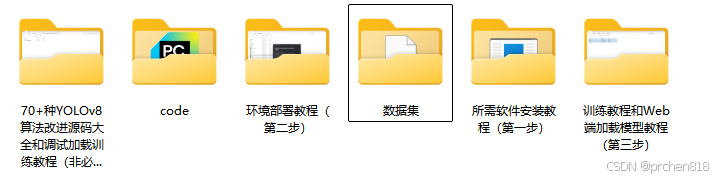
野生动物检测系统源码分享
野生动物检测检测系统源码分享 [一条龙教学YOLOV8标注好的数据集一键训练_70全套改进创新点发刊_Web前端展示] 1.研究背景与意义 项目参考AAAI Association for the Advancement of Artificial Intelligence 项目来源AACV Association for the Advancement of Computer Vis…...
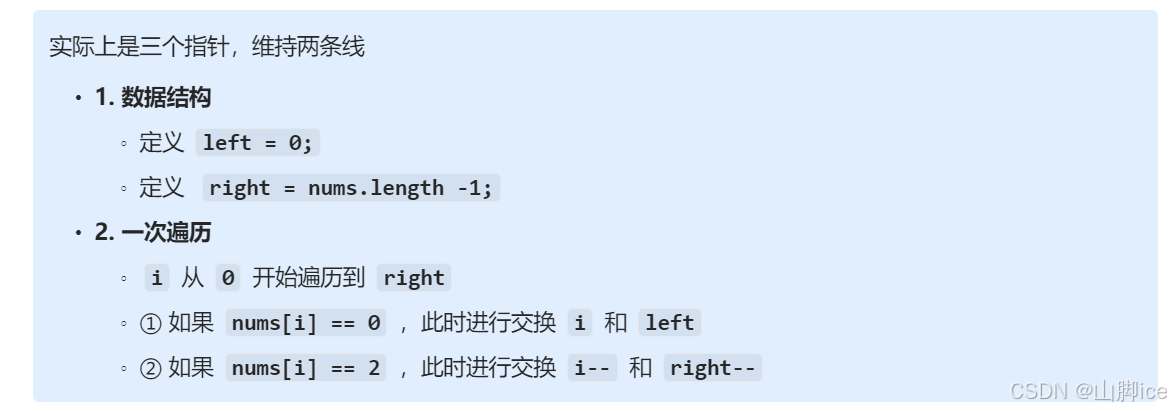
【Hot100】LeetCode—75. 颜色分类
目录 1- 思路题目识别技巧 2- 实现⭐75. 颜色分类——题解思路 3- ACM 实现 原题链接:75. 颜色分类 1- 思路 题目识别 识别1 :给定三种类型数据,使得三种数据用一次遍历实现三种数据排序。 技巧 用两条线将数组分为三部分A 线左侧&#x…...
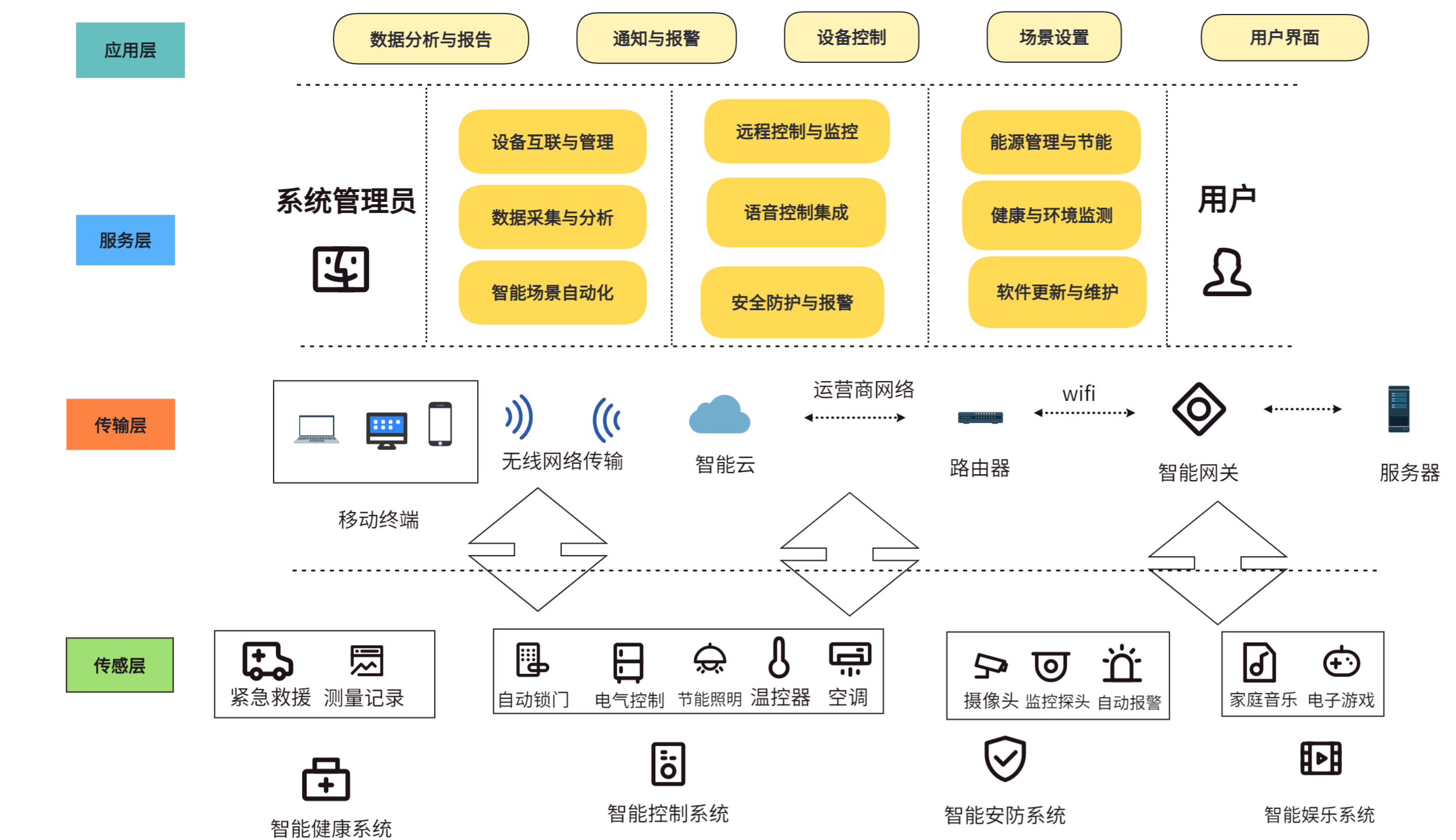
【物联网技术大作业】设计一个智能家居的应用场景
前言: 本人的物联网技术的期末大作业,希望对你有帮助。 目录 大作业设计题 (1)智能家居的概述。 (2)介绍智能家居应用。要求至少5个方面的应用,包括每个应用所采用的设备,性能&am…...
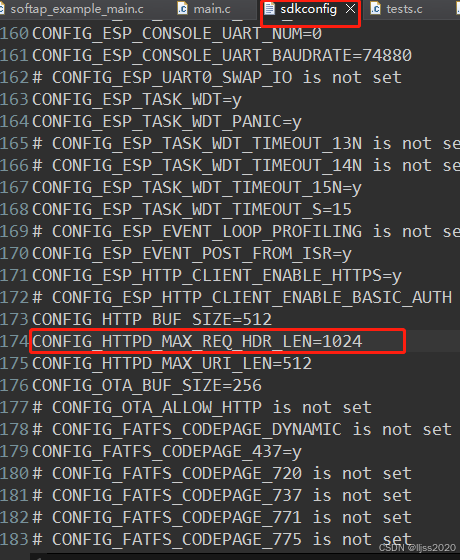
ESP8266做httpServer提示Header fields are too long for server to interpret
CONFIG_HTTP_BUF_SIZE512 CONFIG_HTTPD_MAX_REQ_HDR_LEN1024 CONFIG_HTTPD_MAX_URI_LEN512CONFIG_HTTPD_MAX_REQ_HDR_LEN由512改为1024...
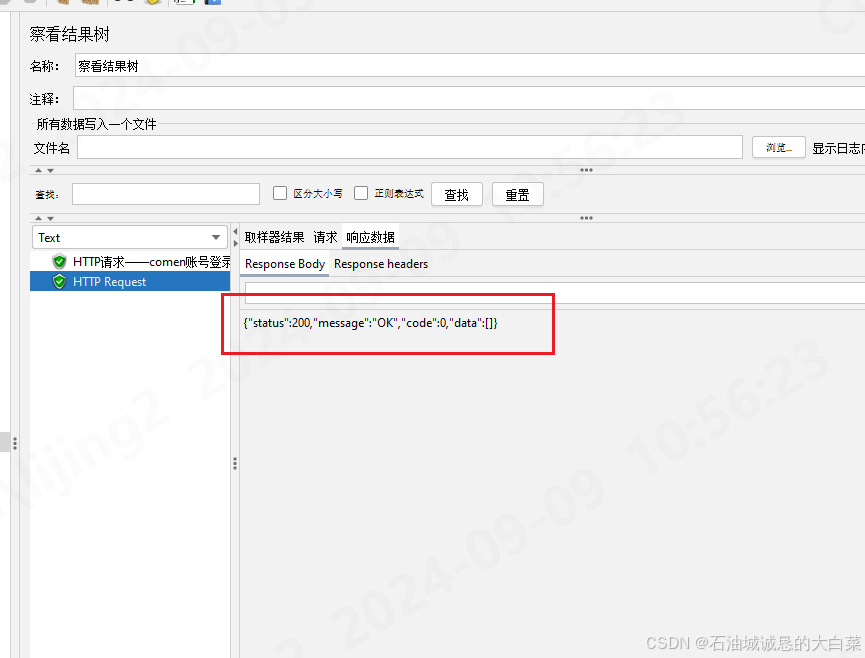
jmeter设置全局token
1、创建setup线程,获取token的接口在所有线程中优先执行,确保后续线程可以拿到token 2、添加配置原件-Http信息头管理器,添加取样器-http请求 配置好接口路径,端口,前端传参数据,调试一下,保证获…...
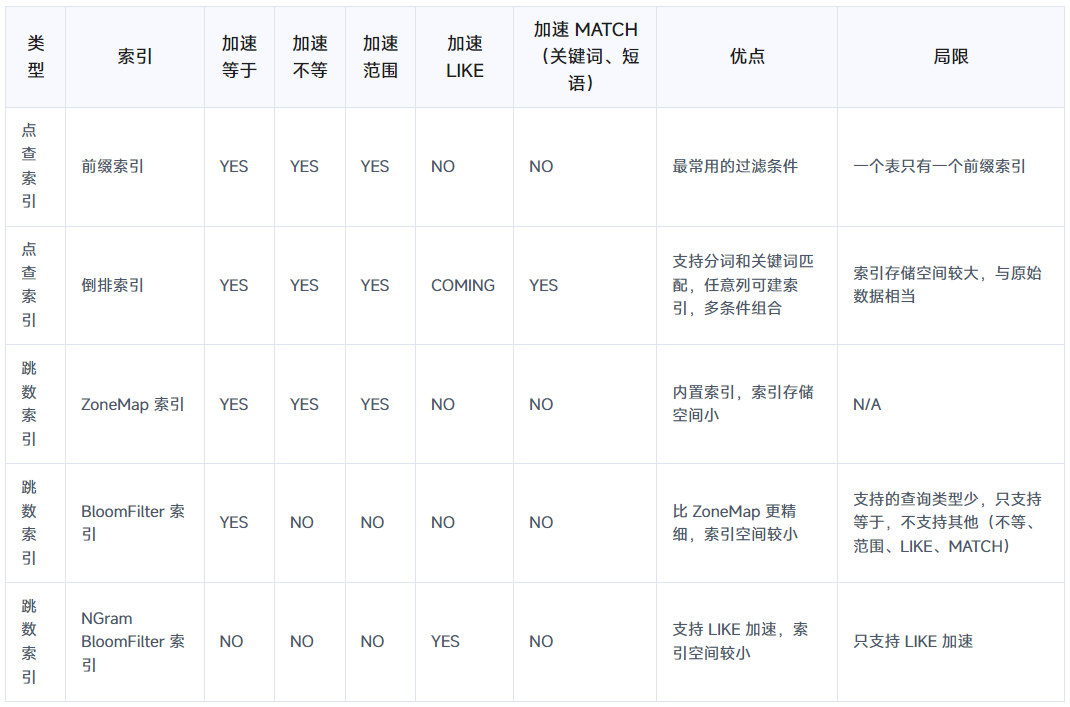
DORIS - DORIS之索引简介
索引概述 索引对比 索引建议 (1)最频繁使用的过滤条件指定为 Key字段,自动建前缀索引,它的过滤效果最好,但是一个表只能有一个前缀索引,因此要用在最频繁的过滤条件上,前缀索引比较小ÿ…...
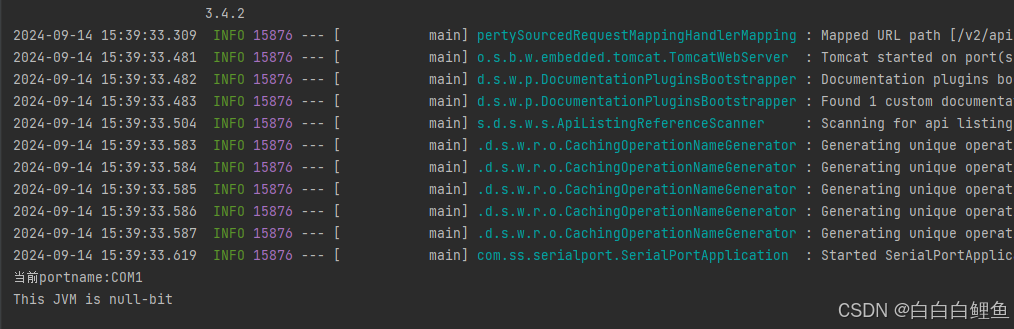
Java 串口通信—收发,监听数据(代码实现)
一、串口通信与串行通信的原理 串行通信是指仅用一根接收线和一根发送线,将数据以位进行依次传输的一种通讯方式,每一位数据占据一个固定的时间长度。 串口通信(Serial Communications)的概念非常简单,串口按位&#x…...

fileinput pdf编辑初始化预览
var $fileLinkInput $(#file_link_full); $fileLinkInput.fileinput({language: zh,uploadUrl: <?php echo Yii::$app->urlManager->createUrl([file/image, type > work_file]);?>,initialPreview: [defaultFile],initialPreviewAsData: true,initialPrevie…...
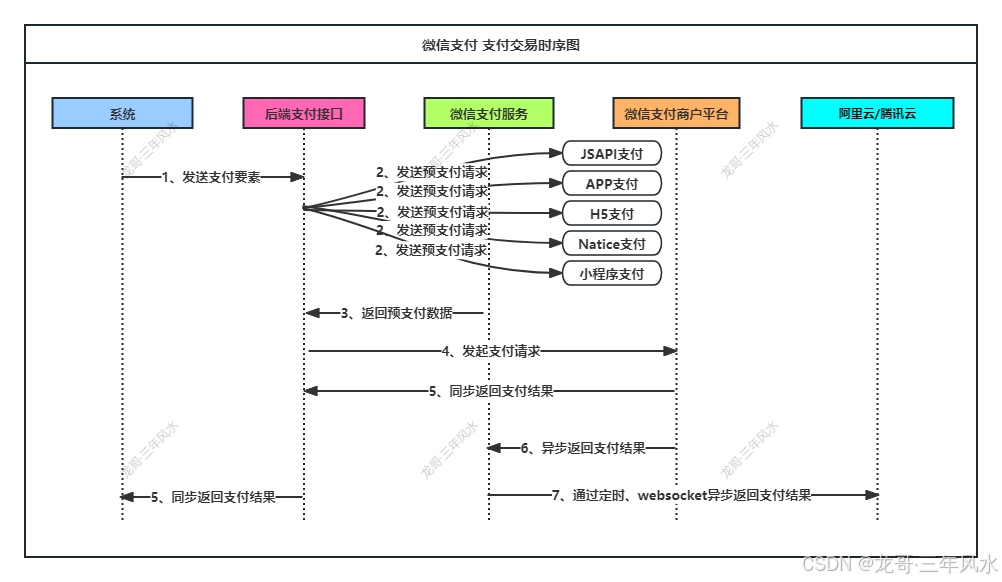
微信支付开发-需求整理及需求设计
一、客户要求 1、通过唤醒机器人参与答题项,机器人自动获取题目,用户进行答题; 2、用户答对题数与后台设置的一样或者更多,则提醒用户可以领取奖品,但是需要用户支付邮费; 3、用户在几天之内不能重复领取奖…...

vs code: pnpm : 无法加载文件 C:\Program Files\nodejs\pnpm.ps1,因为在此系统上禁止运行脚本
在visual studio code运行pnpm出错: pnpm : 无法加载文件 C:\Program Files\nodejs\pnpm.ps1,因为在此系统上禁止运行脚本 解决方案: 到C:\Program Files\nodejs文件夹下删除pnpm.ps1即可。 C:\Program Files\nodejs改成你自己的路径...

web测试必备技能:浏览器兼容性测试
如今,市面上的浏览器种类越来越多(尤其是在平板和移动设备上),这就意味着你所测试的站点需要在这些你声称支持浏览器上都能很好的工作。 同时,主流浏览器(IE,Firefox,Chrome&#x…...
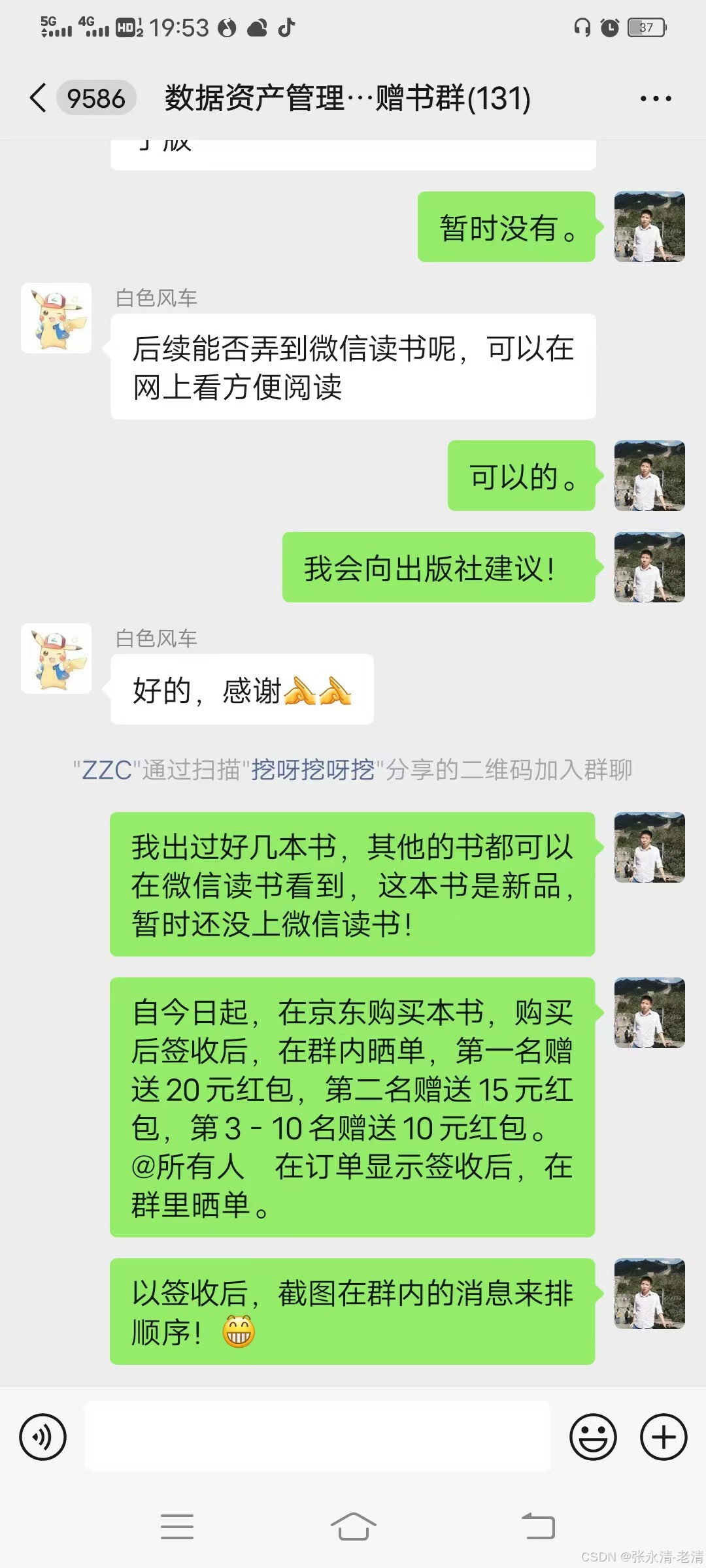
《数据资产管理核心技术与应用》首次大型赠书活动圆满结束
《数据资产管理核心技术与应用》是清华大学出版社出版的一本图书,作者为张永清等著,在2024.9.11号晚上20:00,本书作者张永清联合锋哥聊数仓公众号和清华大学出版社一起,向各大大数据技术爱好者通过三轮互动活动赠送了3本正版图书。…...

vue在一个组件引用其他组件
在vue一个组件中引用另一个组件的步骤 必须在script中导入要引用的组件需要在export default的components引用导入的组件(这一步经常忘记)在template使用导入的组件<script><!-- 第一步,导入--> import Vue01 from "@/components/Vue01.vue";...
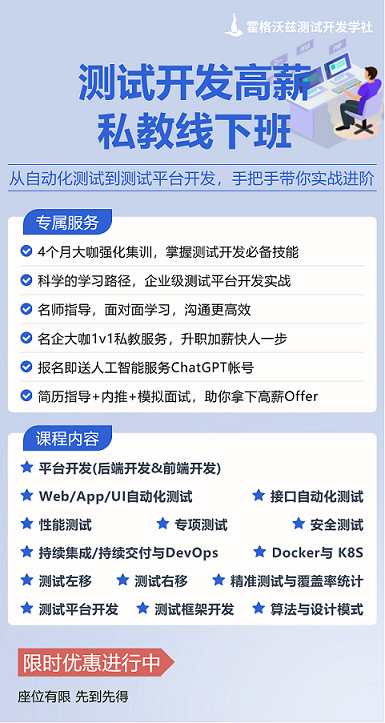
软件测试学习笔记丨Postman实战练习
本文转自测试人社区,原文链接:https://ceshiren.com/t/topic/32096#h-22 二、实战练习 2.1 宠物商店接口文档分析 接口文档:http://petstore.swagger.io ,这是宠物商店接口的 swagger 文档。 2.1.1 什么是 swagger Swagger 是…...

kubernetes微服务基础及类型
目录 1 什么是微服务 2 微服务的类型 3 ipvs模式 ipvs模式配置方式 4 微服务类型详解 4.1 ClusterIP 4.2 ClusterIP中的特殊模式headless 4.3 nodeport 4.4 metalLB配合loadbalance实现发布IP 1 什么是微服务 用控制器来完成集群的工作负载,那么应用如何暴漏出去&…...
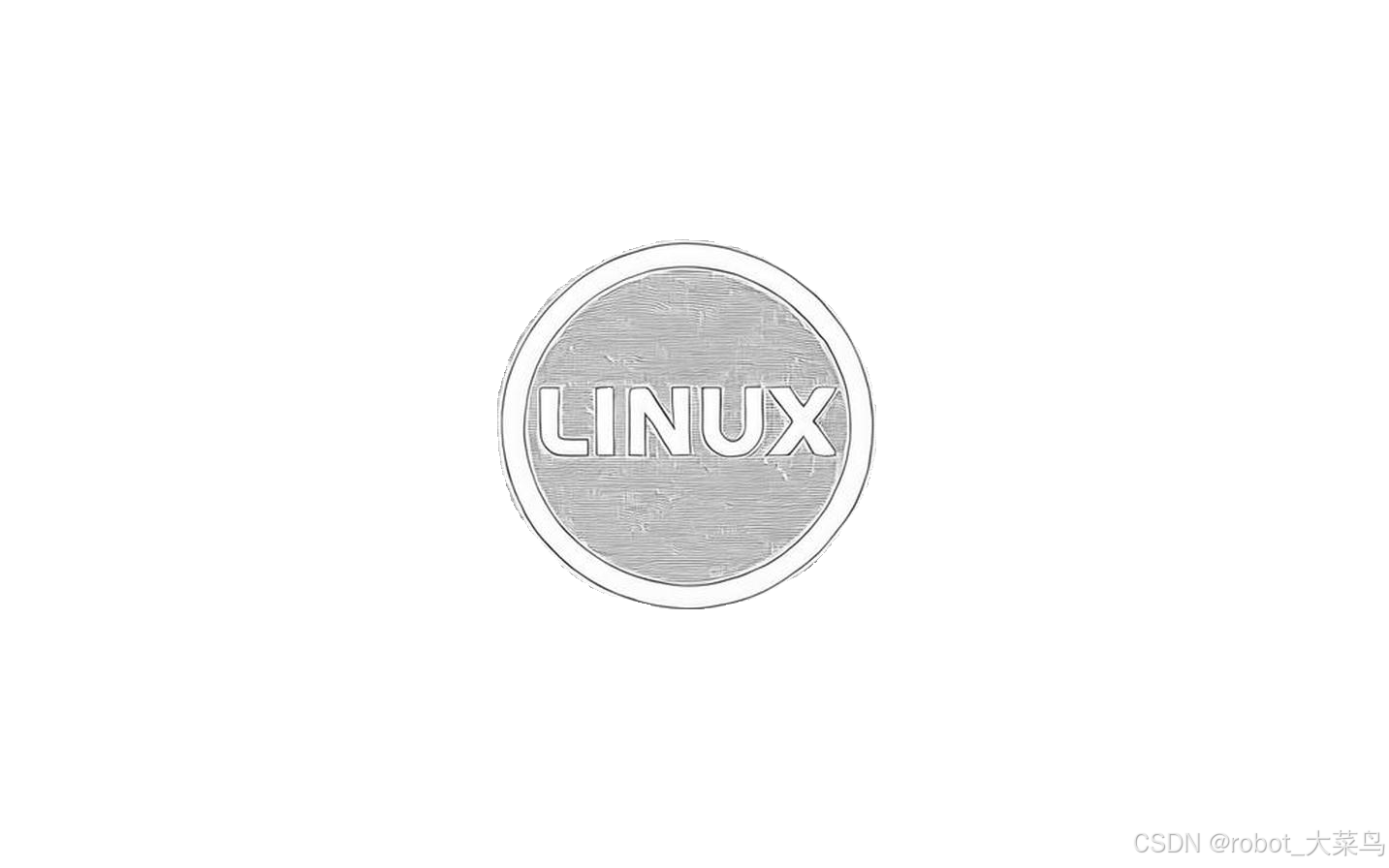
linux-L3_linux 查看进程(node-red)
linux 查看进程 以查看进程node-red为例 ps aux | grep node-red...
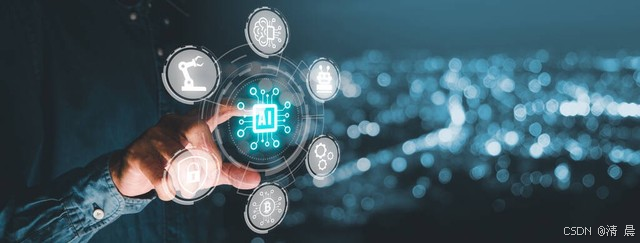
区块链之变:揭秘Web3对互联网的改变
传统游戏中,玩家的虚拟资产(如角色、装备)通常由游戏公司控制,玩家无法真正拥有这些资产或进行交易。而在区块链游戏中,虚拟资产通过去中心化技术记录在区块链上,玩家对其拥有完全的所有权,并能…...
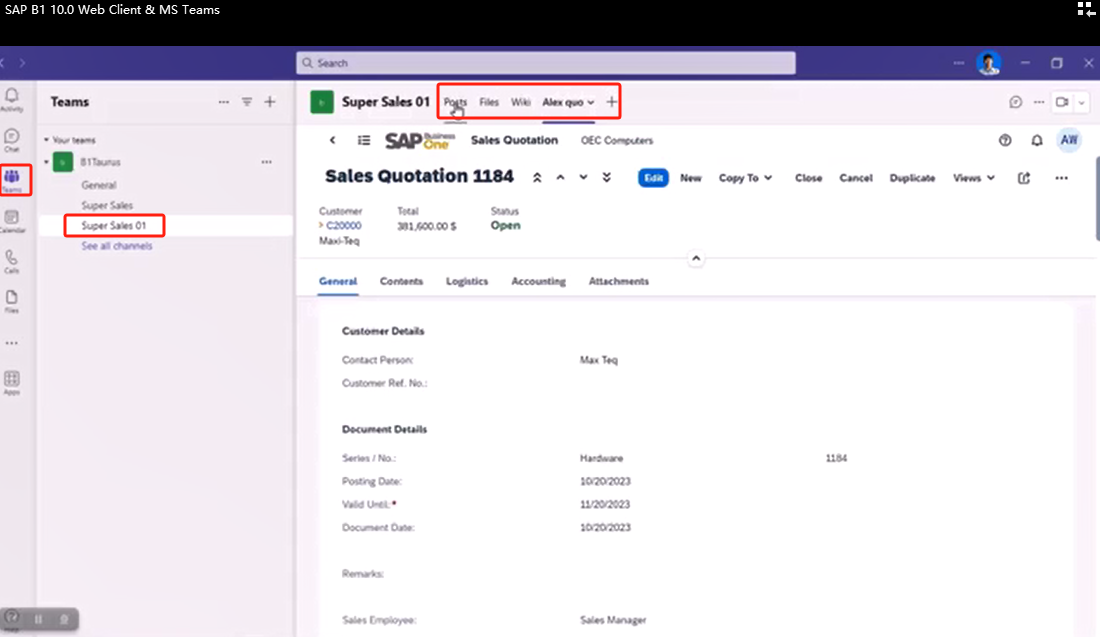
SAP B1 Web Client MS Teams App集成连载一:先决条件/Prerequisites
一、先决条件/Prerequisites 在设置 SAP Business One 应用之前,确保您已具备以下各项:Before you set up the SAP Business One app, make sure you have acquired the following: 1.Microsoft Teams 管理员账户/A Microsoft Teams admin account 您需…...
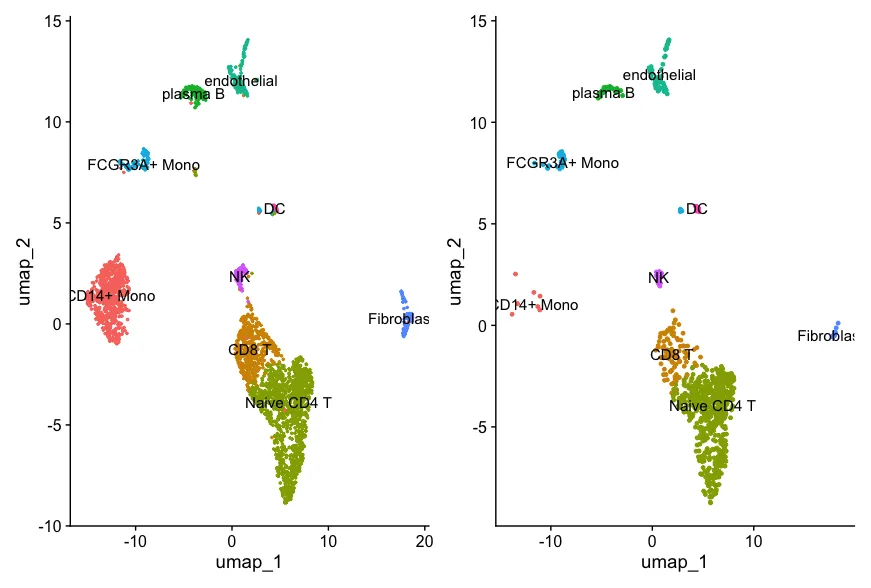
去除单细胞数据中环境游离的RNA污染-decontX工具学习
DecontX 是一种用于单细胞 RNA 测序数据的去除环境污染物(decontamination)的工具,主要用于减少由细胞外RNA造成的污染效应。 开发者在20年的文章中已经把这个工具适用的情况说的非常清楚了:简单来说就是基于微流控的单细胞技术会…...
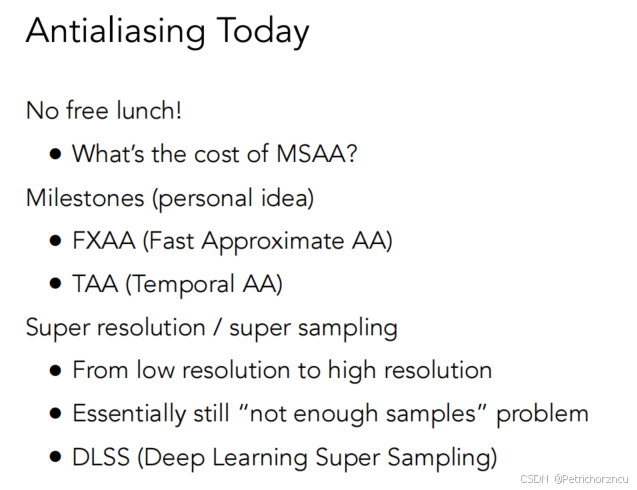
Games101图形学笔记——光栅化
这里写目录标题 Rasterization光栅化屏幕空间隔行扫描三角形采样采样产生的问题反走样处理方法:采样前模糊 频率,时域傅里叶级数展开傅里叶变换 滤波高通滤波低通滤波 卷积卷积的一些定理 反走样MSAA(Multisample Anti-Aliasing)多…...
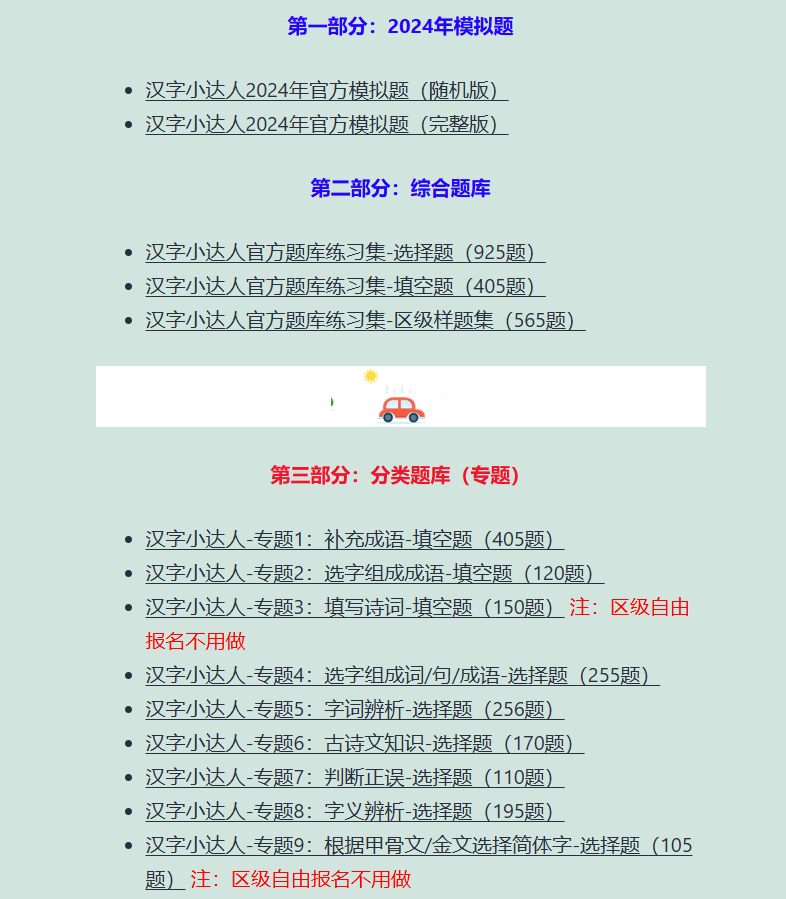
2024年汉字小达人区级自由报名的几个最新问题和真题练一练
2024年第十一届汉字小达人的区级活动的时间9月25-30日正式开赛,还有不到两周。 今天继续回答家长和孩子们的几个问题,并给大家看看一些真题,让孩子对汉字小达人的题型和比赛有直观的了解,从而更好地备考。 本专题在比赛前持续更…...
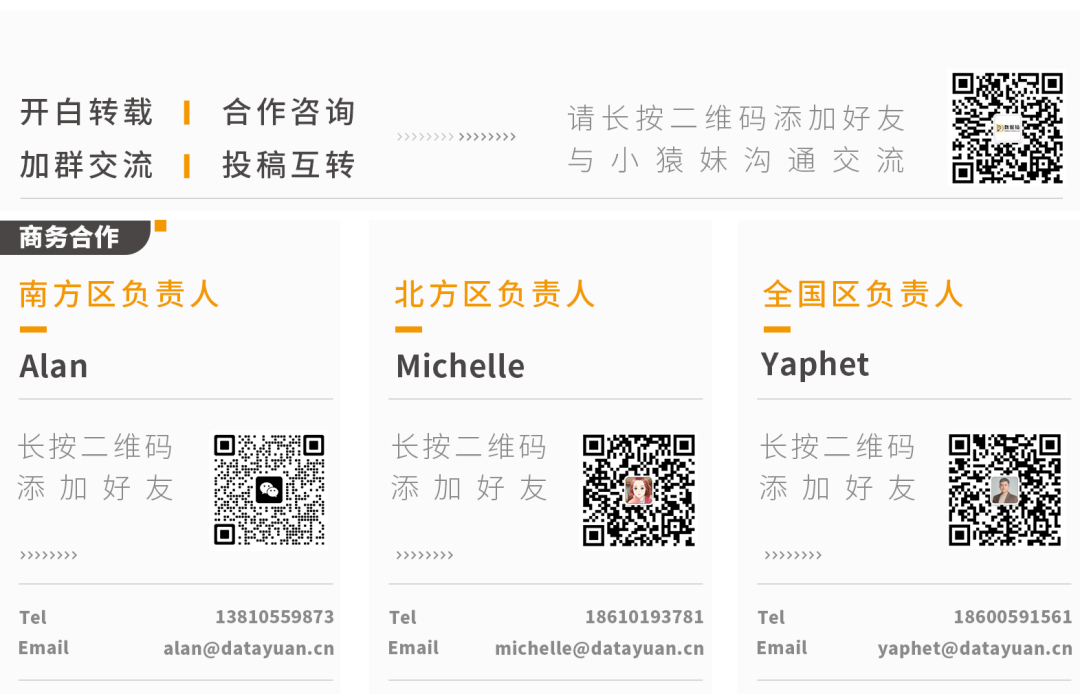
从简单分析到智能问数,Smartbi AIChat让数据回归业务
大数据产业创新服务媒体 ——聚焦数据 改变商业 在某科技公司,资深数据分析师李晨(化名)正忙于分析新产品的市场表现。面对传统自助式BI工具,李晨在功能界面中手动设置各种查询条件,进行了一番复杂的拖拉拽操作&#…...
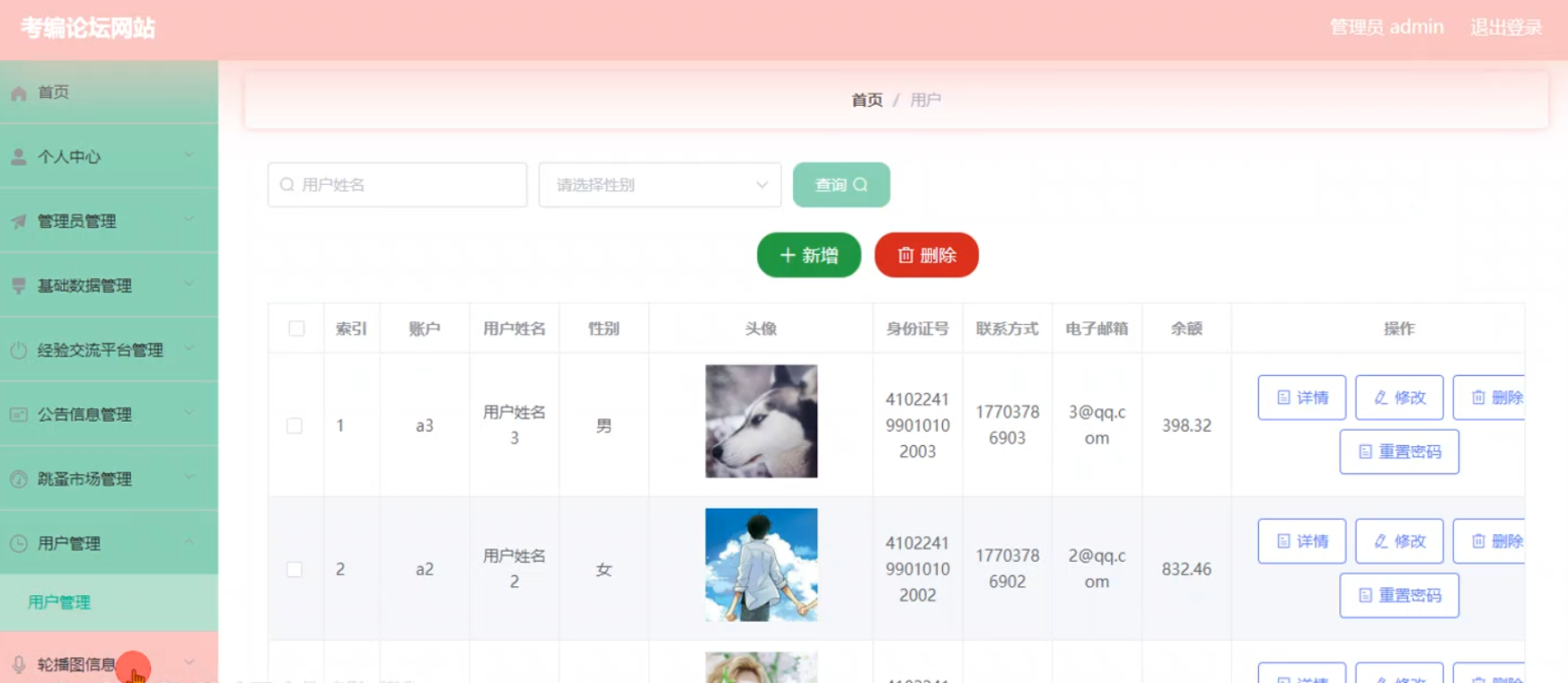
基于SpringBoot+Vue+MySQL的考编论坛网站
系统展示 用户前台界面 管理员后台界面 系统背景 在当前信息化高速发展的时代,考编已成为众多求职者的重要选择。然而,备考过程中信息获取、经验交流及资源分享的需求日益凸显。基于SpringBoot、Vue.js与MySQL构建的考编论坛网站应运而生,旨在…...
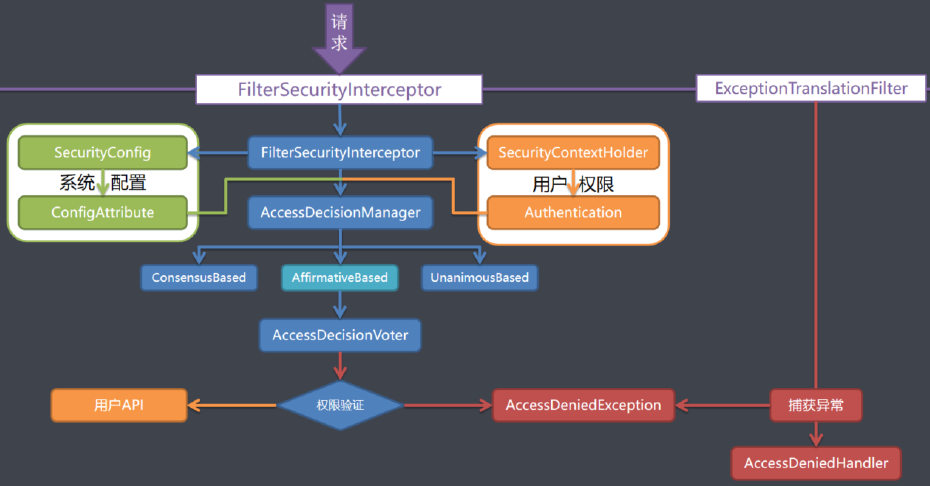
SpringSecurity剖析
1、SpringSecurity 入门 1.1、简介 Spring Security是一个功能强大且高度可定制的身份验证和访问控制框架。它是用于保护基于Spring的应用程序的实际标准。Spring Security是一个框架,致力于为Java应用程序提供身份验证和授权。与所有Spring项目一样,Sp…...

一文搞懂 Flink Graph 构建过程源码
一文搞懂 Flink Graph 构建过程 1. StreamGraph构建过程1.1 transform(): 构建的核心1.2 transformOneInputTransform1.3 构造顶点1.4 构造边1.5 transformSource1.6 transformPartition1.7 transformSink 1. StreamGraph构建过程 链接: 一文搞懂 Flink 其他重要源码点击我 e…...
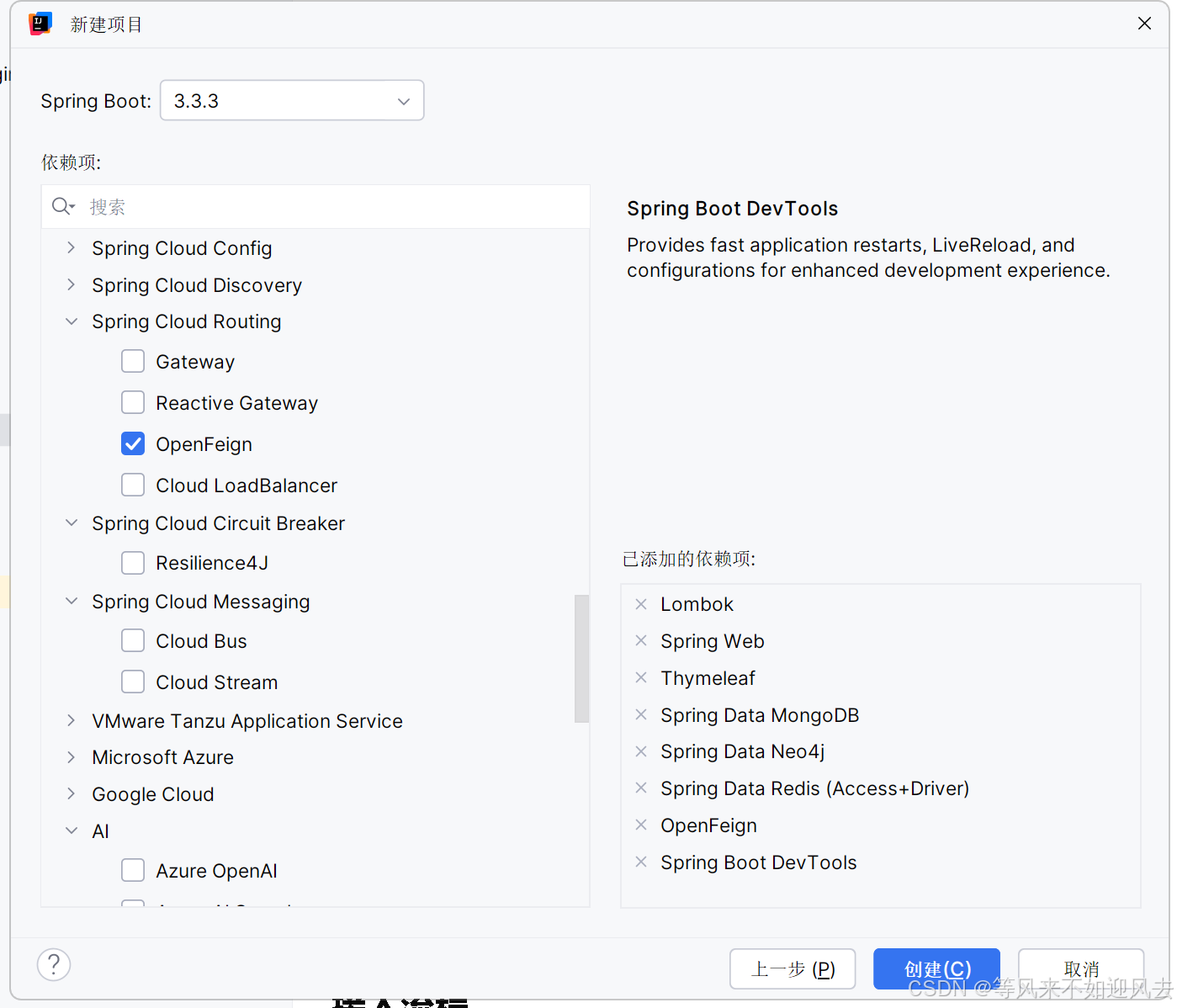
【spring】IDEA 新建一个spring boot 项目
参考新建项目-sprintboot 选择版本、依赖,我选了一堆 maven会重新下载一次么?...
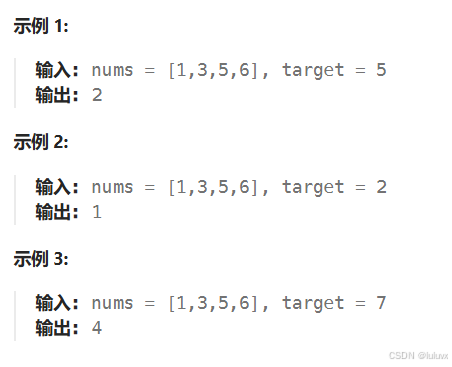
LeetCode[简单] 搜索插入位置
给定一个排序数组和一个目标值,在数组中找到目标值,并返回其索引。如果目标值不存在于数组中,返回它将会被按顺序插入的位置。 请必须使用时间复杂度为 O(log n) 的算法。 思路:类似与二分查找 唯一需要注意的是,搜索…...

(代码可运行)Bootstrap框架的HTML示例
Bootstrap:一套流行的前端开发框架,基于HTML、CSS和JavaScript,适用于快速构建响应式Web应用。 以下是一个使用Bootstrap构建的简单响应式Web应用的HTML示例: <!DOCTYPE html> <html lang"en"> <head&…...