ONNX版本YOLOV5-DeepSort (rknn版本已经Ready)
目录
1. 前言
2. 储备知识
3. 准备工作
4. 代码修改的地方
5.结果展示
1. 前言
之前一直在忙着写文档,之前一直做分类,检测和分割,现在看到跟踪算法,花了几天时间找代码调试,看了看,展示效果比单纯的检测要更加的炸裂一点。
2. 储备知识
DeepSORT(Deep Learning to Track Multi-Object in SORT)是一种基于深度学习的多目标跟踪算法,它结合了深度学习的目标检测和传统的轨迹跟踪方法,旨在实现在复杂场景中准确和稳定地跟踪多个移动目标。以下是关于DeepSORT的检测思想、特点和应用方面的介绍:
检测思想: DeepSORT的核心思想是结合深度学习目标检测和轨迹跟踪方法,以实现多目标跟踪。首先,利用深度学习目标检测模型(如YOLO、Faster R-CNN等)检测出每一帧图像中的所有目标物体,并提取其特征。然后,通过应用传统的轨迹跟踪算法(如卡尔曼滤波器和轨迹关联等),将目标在连续帧之间进行关联,从而生成每个目标的运动轨迹。
特点:
- 多目标跟踪: DeepSORT专注于同时跟踪多个目标,适用于需要同时监测和追踪多个物体的场景,如交通监控、人群管理等。
- 深度特征: 通过使用深度学习模型提取目标的特征,DeepSORT可以更准确地表示目标,从而提高跟踪的精度和鲁棒性。
- 轨迹关联: DeepSORT使用传统的轨迹关联技术来连接不同帧之间的目标,确保在物体出现、消失、重叠等情况下仍能准确跟踪。
- 实时性能: DeepSORT设计用于实时应用,可以在视频流中高效地进行目标跟踪,适用于要求实时性能的应用场景。
需要了解的算法内容:详细介绍
- 目前主流的目标跟踪算法都是基于Tracking-by-Detecton策略,即基于目标检测的结果来进行目标跟踪。DeepSORT运用的就是这个策略,上面的视频是DeepSORT对人群进行跟踪的结果,每个bbox左上角的数字是用来标识某个人的唯一ID号。
-
这里就有个问题,视频中不同时刻的同一个人,位置发生了变化,那么是如何关联上的呢?答案就是匈牙利算法和卡尔曼滤波。
匈牙利算法可以告诉我们当前帧的某个目标,是否与前一帧的某个目标相同。卡尔曼滤波可以基于目标前一时刻的位置,来预测当前时刻的位置,并且可以比传感器(在目标跟踪中即目标检测器,比如Yolo等)更准确的估计目标的位置。
3. 准备工作
基础代码:黄老师的github,参考的是这位博主的,我做了相应的修改
4. 代码修改的地方
具体需要修改的有两个py文件
(1) main.py文件,里面的检测器yolo用onnx做推理,onnx模型参考我的博文yolov5转rknn(聪明的你应该会的)
import cv2
import torch
import numpy as np
import onnxruntime as rtdef sigmoid(x):return 1 / (1 + np.exp(-x))def nms_boxes(boxes, scores):"""Suppress non-maximal boxes.# Argumentsboxes: ndarray, boxes of objects.scores: ndarray, scores of objects.# Returnskeep: ndarray, index of effective boxes."""x = boxes[:, 0]y = boxes[:, 1]w = boxes[:, 2] - boxes[:, 0]h = boxes[:, 3] - boxes[:, 1]areas = w * horder = scores.argsort()[::-1]keep = []while order.size > 0:i = order[0]keep.append(i)xx1 = np.maximum(x[i], x[order[1:]])yy1 = np.maximum(y[i], y[order[1:]])xx2 = np.minimum(x[i] + w[i], x[order[1:]] + w[order[1:]])yy2 = np.minimum(y[i] + h[i], y[order[1:]] + h[order[1:]])w1 = np.maximum(0.0, xx2 - xx1 + 0.00001)h1 = np.maximum(0.0, yy2 - yy1 + 0.00001)inter = w1 * h1ovr = inter / (areas[i] + areas[order[1:]] - inter)inds = np.where(ovr <= 0.45)[0]order = order[inds + 1]keep = np.array(keep)return keepdef process(input, mask, anchors):anchors = [anchors[i] for i in mask]grid_h, grid_w = map(int, input.shape[0:2])box_confidence = sigmoid(input[..., 4])box_confidence = np.expand_dims(box_confidence, axis=-1)box_class_probs = sigmoid(input[..., 5:])box_xy = sigmoid(input[..., :2])*2 - 0.5col = np.tile(np.arange(0, grid_w), grid_w).reshape(-1, grid_w)row = np.tile(np.arange(0, grid_h).reshape(-1, 1), grid_h)col = col.reshape(grid_h, grid_w, 1, 1).repeat(3, axis=-2)row = row.reshape(grid_h, grid_w, 1, 1).repeat(3, axis=-2)grid = np.concatenate((col, row), axis=-1)box_xy += gridbox_xy *= int(img_size/grid_h)box_wh = pow(sigmoid(input[..., 2:4])*2, 2)box_wh = box_wh * anchorsbox = np.concatenate((box_xy, box_wh), axis=-1)return box, box_confidence, box_class_probsdef filter_boxes(boxes, box_confidences, box_class_probs):"""Filter boxes with box threshold. It's a bit different with origin yolov5 post process!# Argumentsboxes: ndarray, boxes of objects.box_confidences: ndarray, confidences of objects.box_class_probs: ndarray, class_probs of objects.# Returnsboxes: ndarray, filtered boxes.classes: ndarray, classes for boxes.scores: ndarray, scores for boxes."""box_classes = np.argmax(box_class_probs, axis=-1)box_class_scores = np.max(box_class_probs, axis=-1)pos = np.where(box_confidences[..., 0] >= 0.5)boxes = boxes[pos]classes = box_classes[pos]scores = box_class_scores[pos]return boxes, classes, scoresdef yolov5_post_process(input_data):masks = [[0, 1, 2], [3, 4, 5], [6, 7, 8]]anchors = [[10, 13], [16, 30], [33, 23], [30, 61], [62, 45],[59, 119], [116, 90], [156, 198], [373, 326]]boxes, classes, scores = [], [], []for input,mask in zip(input_data, masks):b, c, s = process(input, mask, anchors)b, c, s = filter_boxes(b, c, s)boxes.append(b)classes.append(c)scores.append(s)boxes = np.concatenate(boxes)boxes = xywh2xyxy(boxes)classes = np.concatenate(classes)scores = np.concatenate(scores)nboxes, nclasses, nscores = [], [], []for c in set(classes):inds = np.where(classes == c)b = boxes[inds]c = classes[inds]s = scores[inds]keep = nms_boxes(b, s)nboxes.append(b[keep])nclasses.append(c[keep])nscores.append(s[keep])if not nclasses and not nscores:return None, None, Noneboxes = np.concatenate(nboxes)classes = np.concatenate(nclasses)scores = np.concatenate(nscores)return boxes, classes, scoresdef letterbox(img, new_shape=(640, 640), color=(114, 114, 114), auto=True, scaleFill=False, scaleup=True, stride=32):# Resize and pad image while meeting stride-multiple constraintsshape = img.shape[:2] # current shape [height, width]if isinstance(new_shape, int):new_shape = (new_shape, new_shape)# Scale ratio (new / old)r = min(new_shape[0] / shape[0], new_shape[1] / shape[1])if not scaleup: # only scale down, do not scale up (for better test mAP)r = min(r, 1.0)# Compute paddingratio = r, r # width, height ratiosnew_unpad = int(round(shape[1] * r)), int(round(shape[0] * r))dw, dh = new_shape[1] - new_unpad[0], new_shape[0] - new_unpad[1] # wh paddingif auto: # minimum rectangledw, dh = np.mod(dw, stride), np.mod(dh, stride) # wh paddingelif scaleFill: # stretchdw, dh = 0.0, 0.0new_unpad = (new_shape[1], new_shape[0])ratio = new_shape[1] / shape[1], new_shape[0] / shape[0] # width, height ratiosdw /= 2 # divide padding into 2 sidesdh /= 2if shape[::-1] != new_unpad: # resizeimg = cv2.resize(img, new_unpad, interpolation=cv2.INTER_LINEAR)top, bottom = int(round(dh - 0.1)), int(round(dh + 0.1))left, right = int(round(dw - 0.1)), int(round(dw + 0.1))img = cv2.copyMakeBorder(img, top, bottom, left, right, cv2.BORDER_CONSTANT, value=color) # add borderreturn img, ratio, (dw, dh)def clip_coords(boxes, img_shape):# Clip bounding xyxy bounding boxes to image shape (height, width)boxes[:, 0].clamp_(0, img_shape[1]) # x1boxes[:, 1].clamp_(0, img_shape[0]) # y1boxes[:, 2].clamp_(0, img_shape[1]) # x2boxes[:, 3].clamp_(0, img_shape[0]) # y2def xywh2xyxy(x):# Convert nx4 boxes from [x, y, w, h] to [x1, y1, x2, y2] where xy1=top-left, xy2=bottom-righty = x.clone() if isinstance(x, torch.Tensor) else np.copy(x)y[:, 0] = x[:, 0] - x[:, 2] / 2 # top left xy[:, 1] = x[:, 1] - x[:, 3] / 2 # top left yy[:, 2] = x[:, 0] + x[:, 2] / 2 # bottom right xy[:, 3] = x[:, 1] + x[:, 3] / 2 # bottom right yreturn yCLASSES = ['person', 'bicycle', 'car', 'motorcycle', 'airplane', 'bus', 'train', 'truck', 'boat', 'traffic light','fire hydrant', 'stop sign', 'parking meter', 'bench', 'bird', 'cat', 'dog', 'horse', 'sheep', 'cow','elephant', 'bear', 'zebra', 'giraffe', 'backpack', 'umbrella', 'handbag', 'tie', 'suitcase', 'frisbee','skis', 'snowboard', 'sports ball', 'kite', 'baseball bat', 'baseball glove', 'skateboard', 'surfboard','tennis racket', 'bottle', 'wine glass', 'cup', 'fork', 'knife', 'spoon', 'bowl', 'banana', 'apple', 'sandwich','orange', 'broccoli', 'carrot', 'hot dog', 'pizza', 'donut', 'cake', 'chair', 'couch', 'potted plant', 'bed','dining table', 'toilet', 'tv', 'laptop', 'mouse', 'remote', 'keyboard', 'cell phone', 'microwave', 'oven', 'toaster', 'sink','refrigerator', 'book', 'clock', 'vase', 'scissors', 'teddy bear', 'hair drier', 'toothbrush']def preprocess(img, img_size):img0 = img.copy()img = letterbox(img, new_shape=img_size)[0]img = img[:, :, ::-1].transpose(2, 0, 1)img = np.ascontiguousarray(img).astype(np.float32)img = torch.from_numpy(img)img /= 255.0if img.ndimension() == 3:img = img.unsqueeze(0)return img0, imgdef draw(image, boxes, scores, classes):"""Draw the boxes on the image.# Argument:image: original image.boxes: ndarray, boxes of objects.classes: ndarray, classes of objects.scores: ndarray, scores of objects.all_classes: all classes name."""for box, score, cl in zip(boxes, scores, classes):top, left, right, bottom = box# print('class: {}, score: {}'.format(CLASSES[cl], score))# print('box coordinate left,top,right,down: [{}, {}, {}, {}]'.format(top, left, right, bottom))top = int(top)left = int(left)right = int(right)bottom = int(bottom)cv2.rectangle(image, (top, left), (right, bottom), (255, 0, 0), 2)cv2.putText(image, '{0} {1:.2f}'.format(CLASSES[cl], score),(top, left - 6),cv2.FONT_HERSHEY_SIMPLEX,0.6, (0, 0, 255), 2)def detect(im, img_size, sess, input_name, outputs_name):im0, img = preprocess(im, img_size)input_data = onnx_inference(img.numpy(), sess, input_name, outputs_name)boxes, classes, scores = yolov5_post_process(input_data)if boxes is not None:draw(im, boxes, scores, classes)cv2.imshow('demo', im)cv2.waitKey(1)def onnx_inference(img, sess, input_name, outputs_name):# 模型推理:模型输出节点名,模型输入节点名,输入数据(注意节点名的格式!!!!!)outputs = sess.run(outputs_name, {input_name: img})input0_data = outputs[0]input1_data = outputs[1]input2_data = outputs[2]input0_data = input0_data.reshape([3, 80, 80, 85])input1_data = input1_data.reshape([3, 40, 40, 85])input2_data = input2_data.reshape([3, 20, 20, 85])input_data = list()input_data.append(np.transpose(input0_data, (1, 2, 0, 3)))input_data.append(np.transpose(input1_data, (1, 2, 0, 3)))input_data.append(np.transpose(input2_data, (1, 2, 0, 3)))return input_datadef load_onnx_model():# onnx模型前向推理sess = rt.InferenceSession('./weights/modified_yolov5s.onnx')# 模型的输入和输出节点名,可以通过netron查看input_name = 'images'outputs_name = ['396', '440', '484']return sess, input_name, outputs_nameif __name__ == '__main__':# create onnx_modelsess, input_name, outputs_name = load_onnx_model()# input_model_sizeimg_size = 640# read videovideo = cv2.VideoCapture('./video/cut3.avi')print("Loaded video ...")frame_interval = 2 # 间隔帧数,例如每隔10帧获取一次frame_count = 0while True:# 读取每帧图片_, im = video.read()if frame_count % frame_interval == 0:if im is None:break# 缩小尺寸,1920x1080->960x540im = cv2.resize(im, (640, 640))list_bboxs = []# det_objectdetect(im, img_size, sess, input_name, outputs_name)frame_count += 1video.release()cv2.destroyAllWindows()
(2) feature_extractor.py的修改:
这里有4种推理情况:ckpt.t7是ReID( Re-identification利用算法),在图像库中找到要搜索的目标的技术,所以它是属于图像检索的一个子问题。
(1) 动态的batch_size推理:由于检测到的目标是多个object,在本项目的代码REID推理中,会将目标通过torch.cat连接起来,变成(n, 64, 128)的形状,所以需要用动态的onnx模型
(2)那我就想要静态的怎么办,安排!!!,思路就是将cat的拆分开就行了,shape变成(1, 64 , 128),单个推理后将结果cat起来就行了,easy的。
重要!!!!ckpt文件转onnx的代码
import os
import cv2
import time
import argparse
import torch
import numpy as np
from deep_sort import build_tracker
from utils.draw import draw_boxes
from utils.parser import get_config
from tqdm import tqdmif __name__ == '__main__':parser = argparse.ArgumentParser()parser.add_argument("--config_deepsort", type=str, default="./configs/deep_sort.yaml", help='Configure tracker')parser.add_argument("--cpu", dest="use_cuda", action="store_false", default=True, help='Run in CPU')args = parser.parse_args()cfg = get_config()cfg.merge_from_file(args.config_deepsort)use_cuda = args.use_cuda and torch.cuda.is_available()torch.set_grad_enabled(False)model = build_tracker(cfg, use_cuda=False)model.reid = Truemodel.extractor.net.eval()device = 'cpu'output_onnx = 'deepsort.onnx'# ------------------------ export -----------------------------print("==> Exporting model to ONNX format at '{}'".format(output_onnx))input_names = ['input']output_names = ['output']input_tensor = torch.randn(1, 3, 128, 64, device=device)torch.onnx.export(model.extractor.net, input_tensor, output_onnx, export_params=True, verbose=False,input_names=input_names, output_names=output_names, opset_version=13,do_constant_folding=True)
(3)但是要转rknn怎么办,ckpt.t7转onnx后,有一个ReduceL2,不支持量化,我就转的fp16(在RK3588上是可以的,rk1808不知道行不行),不过我尝试了将最后两个节点删除,对结果好像没有什么影响(用的是cut后的onnx推理),有懂的朋友可以解释一下!!!
(4) 就是rknn的推理,这里就不展示了,需要的私聊我吧
import torch
import torchvision.transforms as transforms
import numpy as np
import cv2
# import onnxruntime as rt
# from rknnlite.api import RKNNLiteclass Extractor(object):def __init__(self, model_path):self.model_path = model_pathself.device = "cpu"self.size = (64, 128)self.norm = transforms.Compose([transforms.ToTensor(),transforms.Normalize([0.485, 0.456, 0.406], [0.229, 0.224, 0.225]),])def _preprocess(self, im_crops):"""TODO:1. to float with scale from 0 to 12. resize to (64, 128) as Market1501 dataset did3. concatenate to a numpy array3. to torch Tensor4. normalize"""def _resize(im, size):return cv2.resize(im.astype(np.float32) / 255., size)im_batch = torch.cat([self.norm(_resize(im, self.size)).unsqueeze(0) for im in im_crops], dim=0).float()return im_batchdef __call__(self, im_crops):im_batch = self._preprocess(im_crops)# sess = rt.InferenceSession(self.model_path)# 模型的输入和输出节点名,可以通过netron查看# input_name = 'input'# outputs_name = ['output']# (1)动态输出# features = sess.run(outputs_name, {input_name: im_batch.numpy()})# print('features:', np.array(features)[0, :, :].shape)# return np.array(features)[0, :, :]# (2)静态态输出# sort_results = []# n = im_batch.numpy().shape[0]# for i in range(n):# img = im_batch.numpy()[i, :, :].reshape(1, 3, 128, 64)# feature = sess.run(outputs_name, {input_name: img})# feature = np.array(feature)# sort_results.append(feature)# features = np.concatenate(sort_results, axis=1)[0, :, :]# print(features.shape)# return np.array(features)# (3)去掉onnx的最后两个节点的静态模型输出# input_name = 'input'# outputs_name = ['204']# sort_results = []# n = im_batch.numpy().shape[0]# for i in range(n):# img = im_batch.numpy()[i, :, :].reshape(1, 3, 128, 64)# feature = sess.run(outputs_name, {input_name: img})# feature = np.array(feature)# sort_results.append(feature)# features = np.concatenate(sort_results, axis=1)[0, :, :]# print(features.shape)# return np.array(features)# (4 )rk模型修改# rknn_lite = RKNNLite()# rknn_lite.load_rknn('./weights/ckpt_fp16.rknn')# ret = rknn_lite.init_runtime(core_mask=RKNNLite.NPU_CORE_0_1_2)# if ret != 0:# print('Init runtime environment failed')# exit(ret)# print('done')# sort_results = []# n = im_batch.numpy().shape[0]# for i in range(n):# img = im_batch.numpy()[i, :, :].reshape(1, 3, 128, 64)# feature = self.model_path.inference(inputs=[img])# feature = np.array(feature)# sort_results.append(feature)# features = np.concatenate(sort_results, axis=1)[0, :, :]# print(features.shape)# return np.array(features)
5.结果展示
onnx的转换结果(测试视频地址)
检测结果
相关文章:
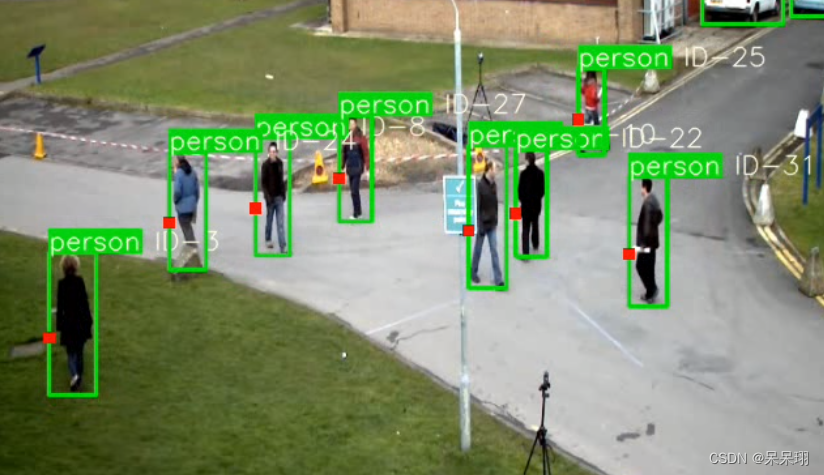
ONNX版本YOLOV5-DeepSort (rknn版本已经Ready)
目录 1. 前言 2. 储备知识 3. 准备工作 4. 代码修改的地方 5.结果展示 1. 前言 之前一直在忙着写文档,之前一直做分类,检测和分割,现在看到跟踪算法,花了几天时间找代码调试,看了看,展示效果比单纯的检…...

MySQL的约束
文章目录 1、约束的概念2、约束的分类2.1 主键约束2.1.1 概念2.1.2 主键操作 2.2 自增约束2.2.1 概念2.2.2 自增操作 2.3 唯一约束2.3.1 概念2.3.2 唯一操作 2.4 非空约束2.4.1 概念2.4.2 非空操作 2.5 默认约束2.5.1 概念2.5.2 默认操作 2.6 外键约束2.6.1 概念2.6.2 外键操作…...
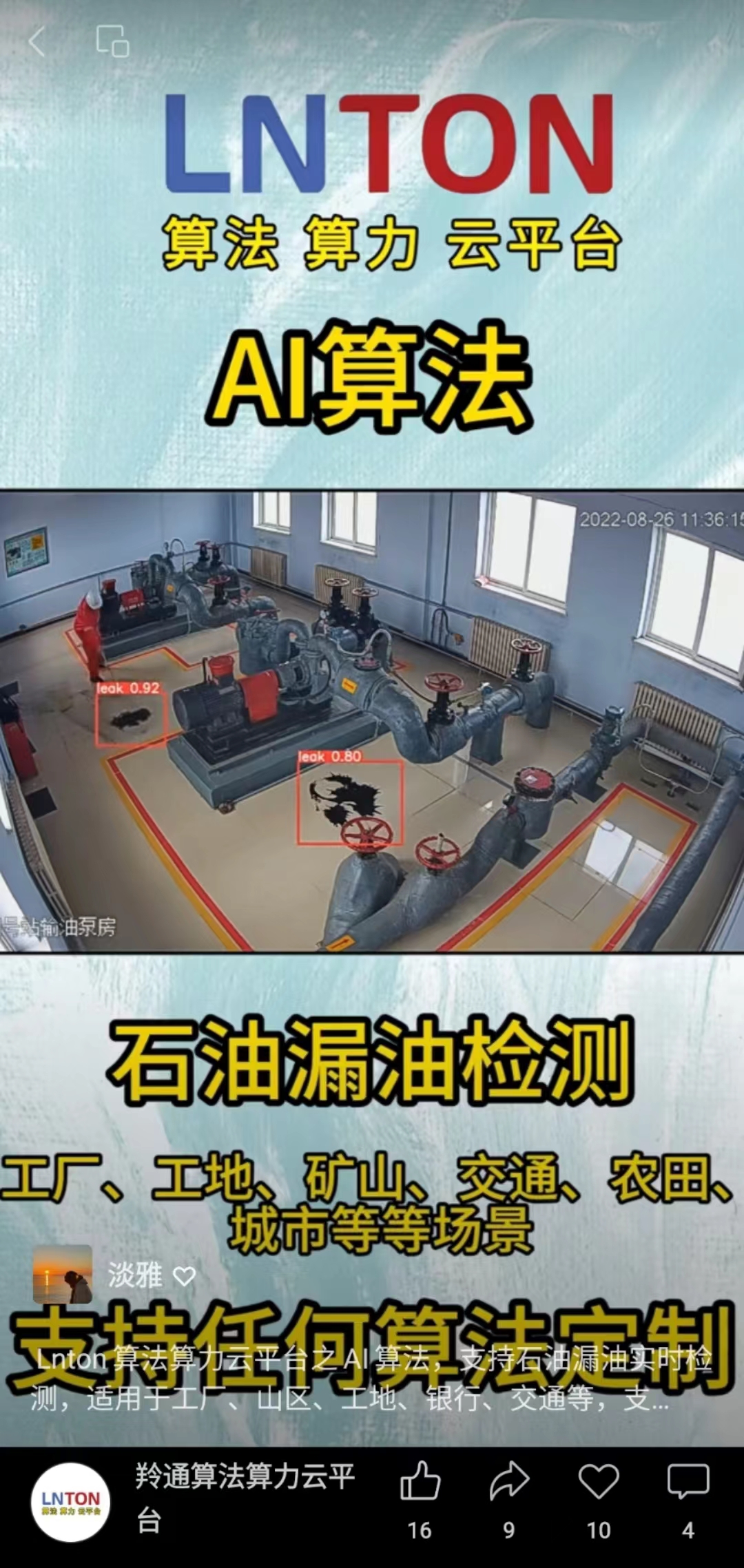
Lnton羚通关于【PyTorch】教程:torchvision 目标检测微调
torchvision 目标检测微调 本教程将使用Penn-Fudan Database for Pedestrian Detection and Segmentation 微调 预训练的Mask R-CNN 模型。 它包含 170 张图片,345 个行人实例。 定义数据集 用于训练目标检测、实例分割和人物关键点检测的参考脚本允许轻松支持添加…...

AMD fTPM RNG的BUG使得Linus Torvalds不满
导读因为在 Ryzen 系统上对内核造成了困扰,Linus Torvalds 最近在邮件列表中表达了对 AMD fTPM 硬件随机数生成器的不满,并提出了禁用该功能的建议。 因为在 Ryzen 系统上对内核造成了困扰,Linus Torvalds 最近在邮件列表中表达了对 AMD fTPM…...
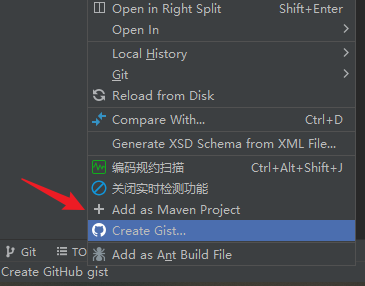
idea 转换为 Maven Project 的方法
选项: Add as Maven Project...

es1.7.2 按照_type先聚合,再按照时间二次聚合
// 设置查询条件if (this.query ! null) {this.searchbuilder.setQuery(this.query);}TermsBuilder typeAggregation AggregationBuilders.terms("agg_type").field("_type");DateHistogramBuilder dateTermsBuilder AggregationBuilders.dateHistogram(…...

pyqt5 如何修改QplainTextEdit 背景色和主窗口的一样颜色
如果您希望将 QPlainTextEdit 的背景颜色设置为与窗口背景相似的灰色,您可以使用窗口的背景颜色作为基准来设置 QPlainTextEdit 的背景颜色。以下是一个示例代码,展示如何实现这一点: from PyQt5.QtWidgets import QApplication, QMainWindo…...

解决使用element ui时el-input的属性type=number,仍然可以输入e的问题。
使用element ui时el-input的属性typenumber,仍然可以输入e, 其他的中文特殊字符都不可以输入,但是只有e是可以输入的,原因是e也输入作为科学计数法的时候,e是可以被判定为数字的, 但是有些场景是需要把e这种…...

ShardingSphere 可观测 SQL 指标监控
ShardingSphere并不负责如何采集、存储以及展示应用性能监控的相关数据,而是将SQL解析与SQL执行这两块数据分片的最核心的相关信息发送至应用性能监控系统,并交由其处理。 换句话说,ShardingSphere仅负责产生具有价值的数据,并通过…...

Redisson实现分布式锁示例
一、引入依赖 <dependency><groupId>org.redisson</groupId><artifactId>redisson</artifactId><version>3.16.0</version></dependency>二、配置类 import org.redisson.Redisson; import org.redisson.api.RedissonClient;…...

使用Nginx作为一个普通代理服务器
使用Nginx作为一个普通代理服务器, 请不要用于违法用途哦 nginx作为一个反向代理工具,除了可以进行反向代理之外,还可以用来作为代理工具来使用,作为代理工具使用的步骤如下,这个配置目前支持80端口 Windows系统代理设置对应IP, …...
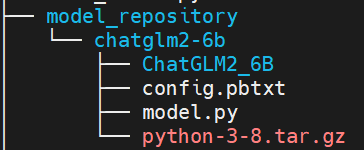
chatglm2-6b模型在9n-triton中部署并集成至langchain实践 | 京东云技术团队
一.前言 近期, ChatGLM-6B 的第二代版本ChatGLM2-6B已经正式发布,引入了如下新特性: ①. 基座模型升级,性能更强大,在中文C-Eval榜单中,以51.7分位列第6; ②. 支持8K-32k的上下文;…...
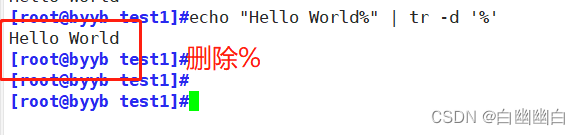
Shell编程之正则表达式(非常详细)
正则表达式 1.通配符和正则表达式的区别2.基本正则表达式2.1 元字符 (字符匹配)2.2 表示匹配次数2.4 位置锚定2.5 分组 和 或者 3.扩展正则表达式4.部分文本处理工具4.1 tr 命令4.2 cut命令4.3 sort命令4.4 uniq命令 1.通配符和正则表达式的区别 通配符一般用于文件…...
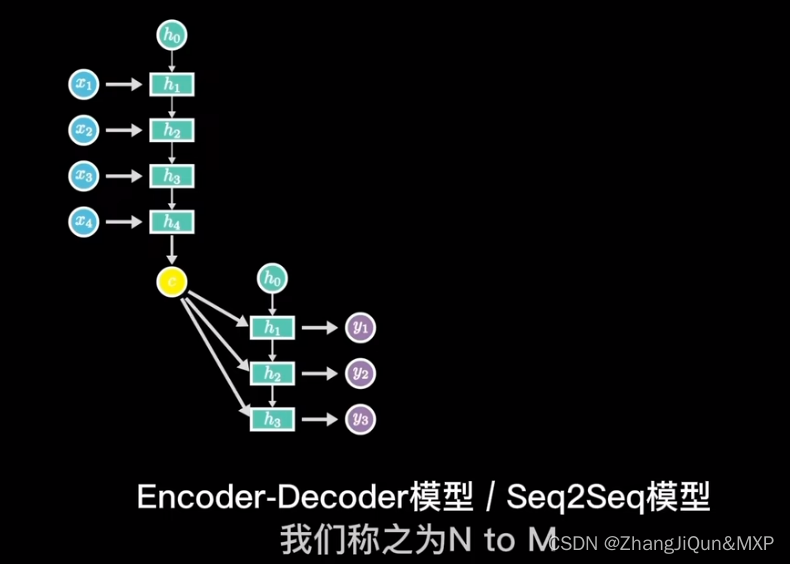
RNN模型简单理解和CNN区别
目录 神经网络:水平方向延伸,数据不具有关联性 RNN:在神经网络的基础上加上了时间顺序,语义理解 RNN: 训练中采用梯度下降,反向传播 长短期记忆模型 输出关系:1 toN,N to N 单入…...
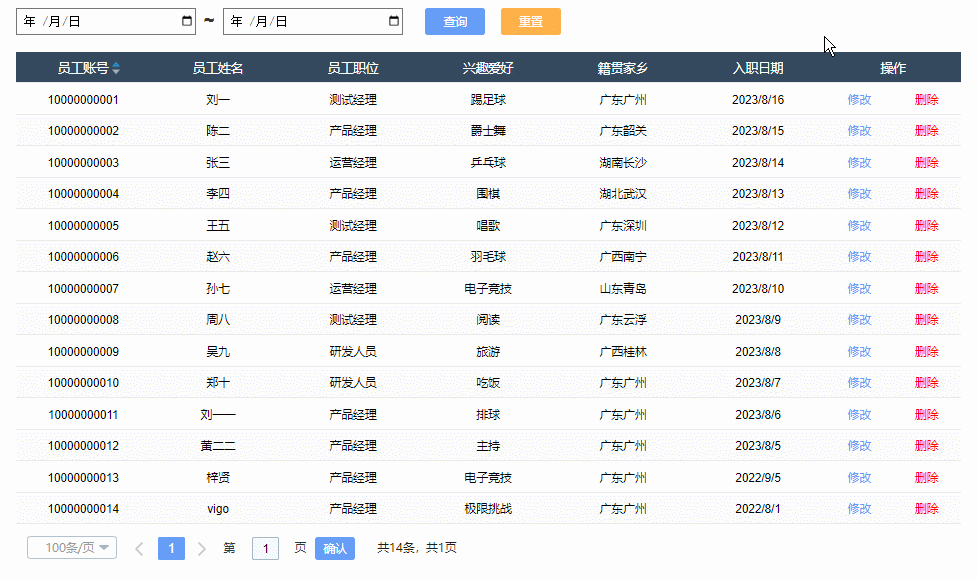
【Axure高保真原型】JS日期选择器筛选中继器表格
今天和大家分享JS日期选择器筛选中继器表格的原型模板,通过调用浏览器的日期选择器,所以可以获取真实的日历效果,具体包括哪一年二月份有29天,几号对应星期几,都是真实的,获取日期值后,通过交互…...

android bp脚本
一。android大约从7.0开始引入 .bp文件代替以前的.mk文件,用于帮助android项目的编译配置文件。 二。mk文件转化为bp文件,可以使用下面命令转化,注意命令中>,这是写入文件。androidmk是android源码自带的工具,他可…...
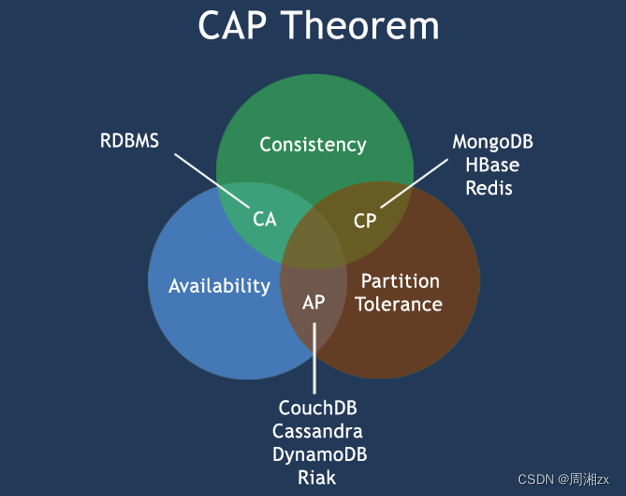
Redis 数据库 NoSQL
目录 一、NoSQL 二、为什么会出现NoSQL技术 三、NoSQL的类别 键值(Key-Value)存储数据库 列存储数据库 文档型数据库 图形(Graph)数据库 四、NoSQL适应场景 五、在分布式数据库中CAP原理 1、CAP 2、BASE 一、NoSQL NoS…...

RN 项目异常问题整理
常见问题 无法找到 CardStackStyleInterpolator StackViewStyleInterpolator 这个方法集来代替 CardStackStyleInterpolator的,这个方法集的路径也需要注意一下,在2.12.1版本之前, 该文件在react-navigation/src/views/StackView/中…...

STM8编程[TIM1多路PWM输出选项字节(Option Byte)操作和IO复用]
TIM1多路PWM输出选项字节(Option Byte)操作和IO复用 本文摘录于:https://blog.csdn.net/freeape/article/details/47008033只是做学习备份之用,绝无抄袭之意,有疑惑请联系本人! 代码上要使用TIME1输出3路PWM,代码如下: void tim…...
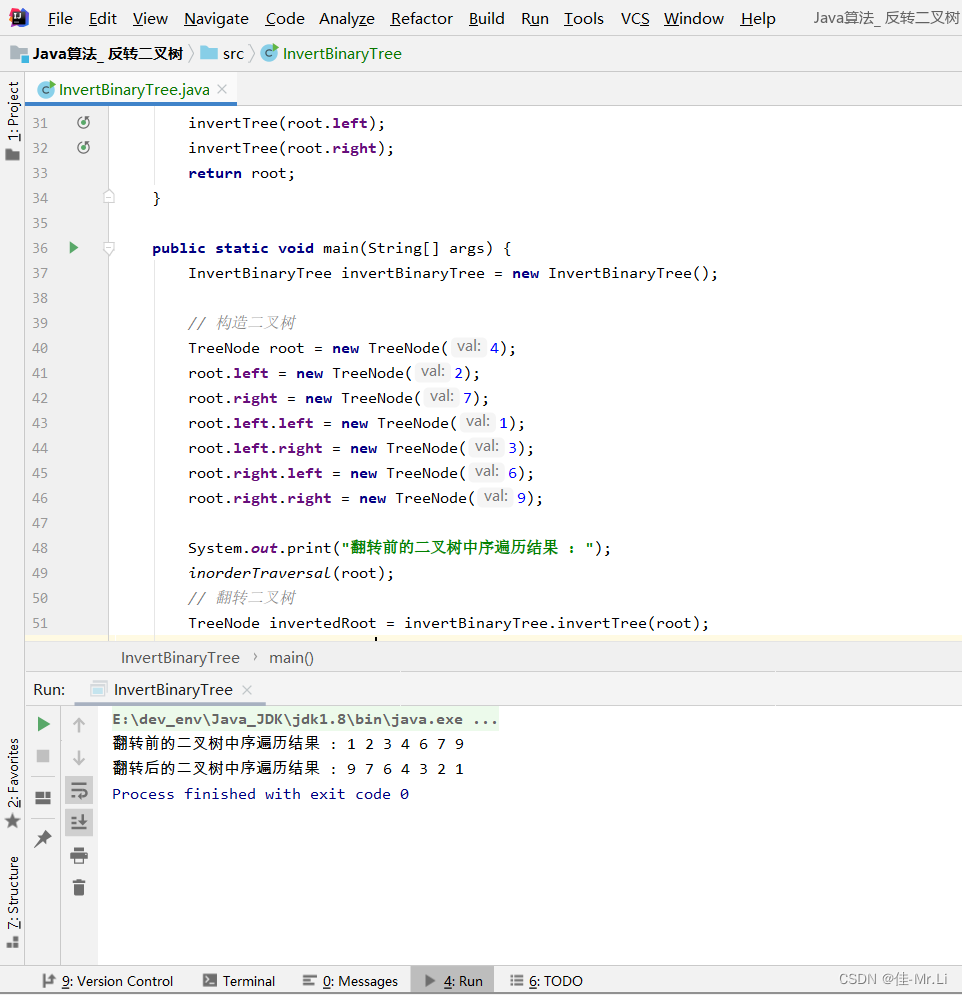
Java算法_ 反转二叉树(LeetCode_Hot100)
题目描述:给你一棵二叉树的根节点 ,翻转这棵二叉树,并返回其根节点。root。 获得更多?算法思路:代码文档,算法解析的私得。 运行效果 完整代码 /*** 2 * Author: LJJ* 3 * Date: 2023/8/16 13:18* 4*/public class In…...

C/C++ 标准模版库STL(持续更新版)
标准模版库STL 目录 算法库 栈 队列 向量 映射 列表 双向链表 集合 Iterator 送代器 <algorithm> 算法库 max, min 用于找出一组值中的最大值和最小值 swap 用于交换两个变量的值 sort 用于对一个范围内的元素进行排序 lower_bound, upper_bound 用于在已排序的容器…...
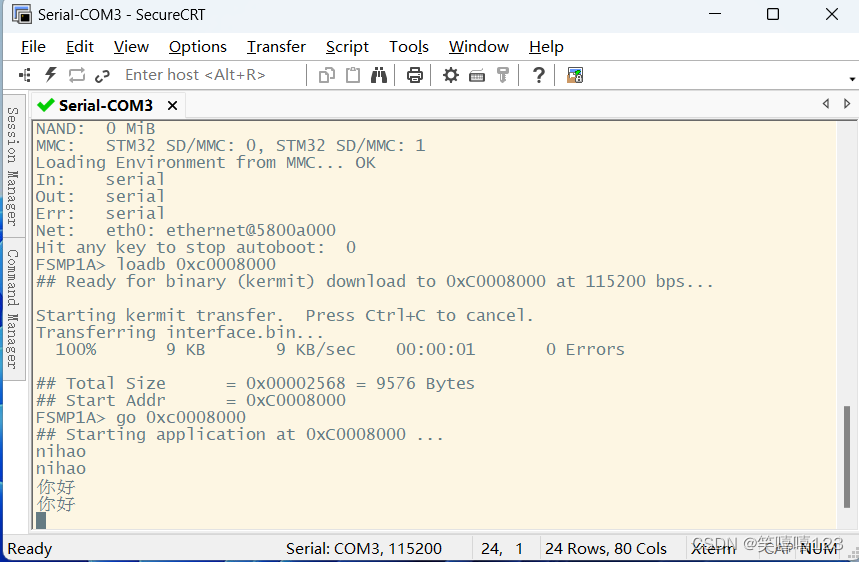
ARM(实验二)
uart4.h #ifndef __H__ #define __H__#include "stm32mp1xx_rcc.h" #include "stm32mp1xx_gpio.h" #include "stm32mp1xx_uart.h"//RCC/GPIO/UART4章节初始化 void hal_uart4_init();//发送一个字符函数 void hal_put_char(const char str);//发…...
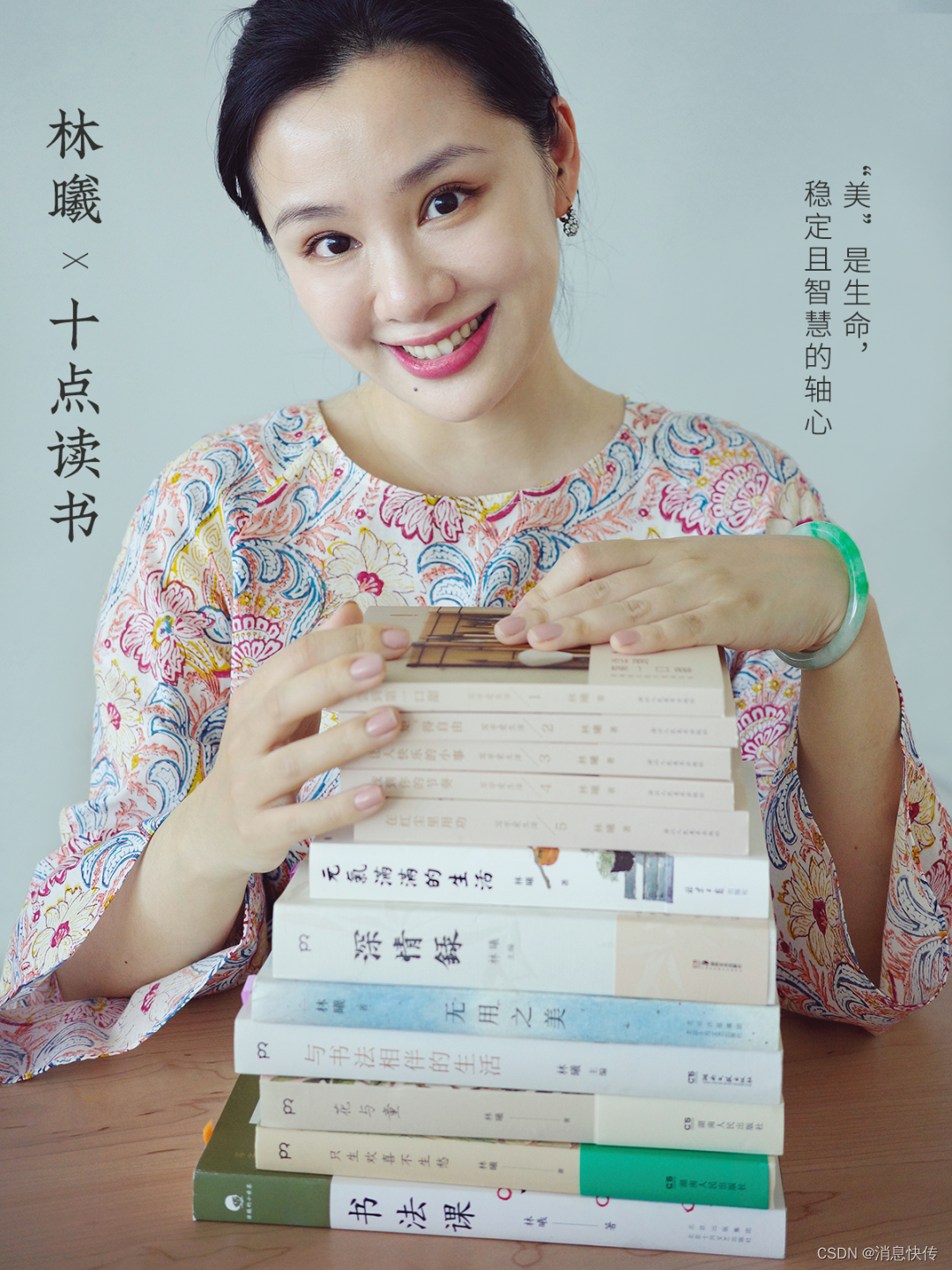
由“美”出发 听艺术家林曦关于美育与智慧的探讨
不久前,林曦老师与我们的老朋友「十点读书」进行了一次线上直播,有关林曦老师十余年的书法教学,和传统美育的心得,以及因此诞生的新书《无用之美》。 这一次的直播,由“美”的主题出发,延伸出美育…...

Serial与Parallel GC之间的不同之处是什么?
Serial GC(串行垃圾回收器)和Parallel GC(并行垃圾回收器)都是Java虚拟机(JVM)中用于进行垃圾回收的两种基本算法。它们在性能、资源利用和回收效率等方面存在一些不同之处。下面是它们之间的详细比较: 1.工作方式 Serial GC:它是一种单线程的垃圾回收器…...
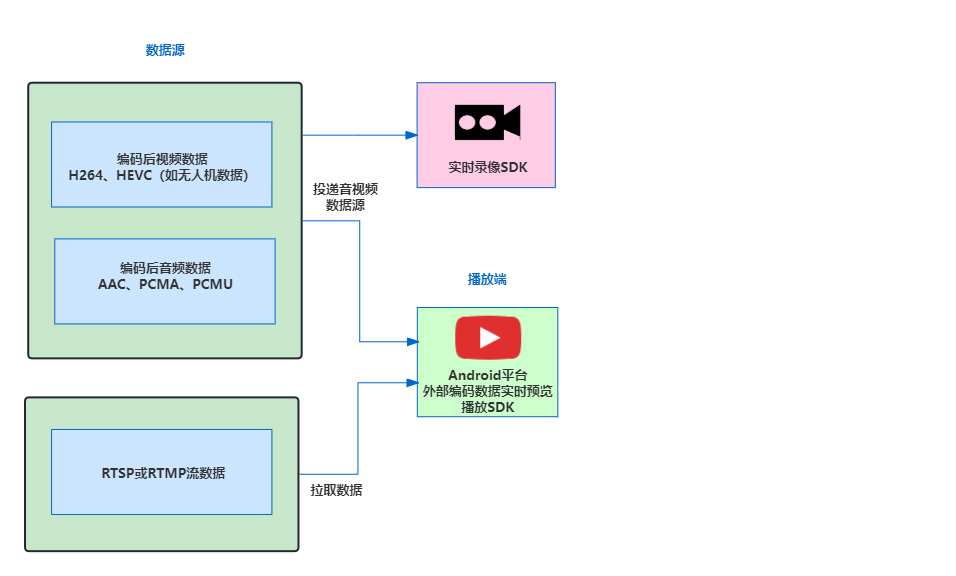
GB28181设备接入侧如何对接外部编码后音视频数据并实现预览播放
技术背景 我们在对接GB28181设备接入模块的时候,遇到这样的技术诉求,好多开发者期望能提供编码后(H.264/H.265、AAC/PCMA)数据对接,确保外部采集设备,比如无人机类似回调过来的数据,直接通过模…...
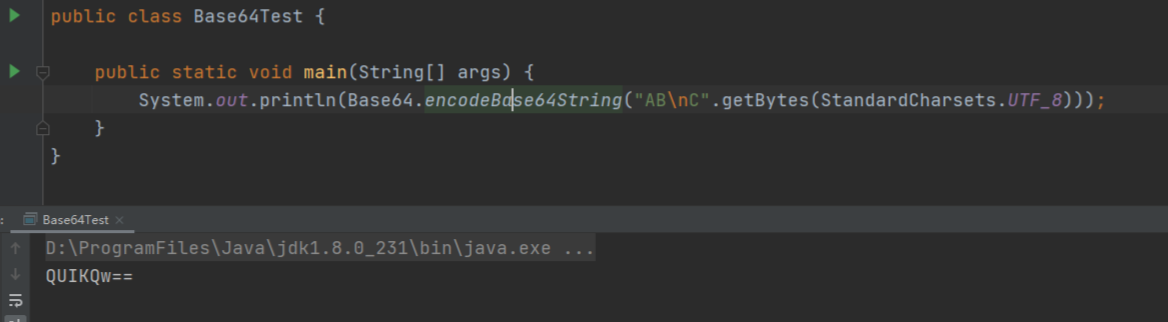
【java】为什么文件上传要转成Base64?
文章目录 1 前言2 multipart/form-data上传3 Base64上传3.1 Base64编码原理3.2 Base64编码的作用 4 总结 1 前言 最近在开发中遇到文件上传采用Base64的方式上传,记得以前刚开始学http上传文件的时候,都是通过content-type为multipart/form-data方式直接…...
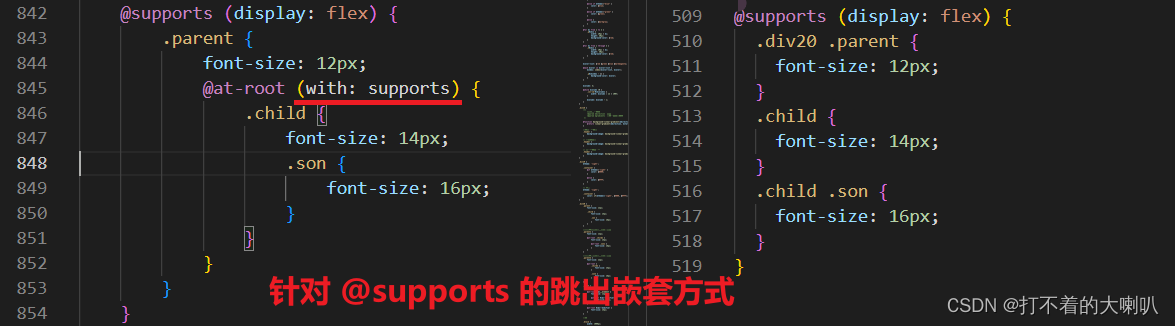
SCSS 学习笔记 和 vscode下载live sass compiler插件配置
1、下载livelive sass compiler插件并配置 // 在 已有代码 下面 添加下面 代码,一般刚刚下载打开最后一行是:// "liveSassCompile.settings.autoprefix": [],// 所以直接 把下面复制进去保存就行"liveSassCompile.settings.autoprefix&qu…...

CSS中的字体属性有哪些值,并分别描述它们的作用。
聚沙成塔每天进步一点点 ⭐ 专栏简介⭐ font-style⭐ font-weight⭐ font-size⭐ font-family⭐ font-variant⭐ line-height⭐ letter-spacing⭐ word-spacing⭐ font⭐ 写在最后 ⭐ 专栏简介 前端入门之旅:探索Web开发的奇妙世界 记得点击上方或者右侧链接订阅本专…...
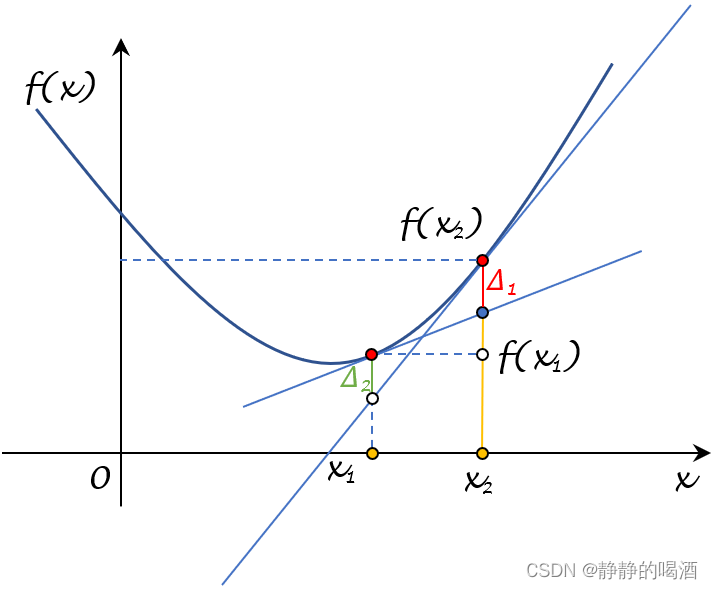
机器学习笔记之优化算法(十五)Baillon Haddad Theorem简单认识
机器学习笔记之优化算法——Baillon Haddad Theorem简单认识 引言 Baillon Haddad Theorem \text{Baillon Haddad Theorem} Baillon Haddad Theorem简单认识证明过程证明:条件 1 ⇒ 1 \Rightarrow 1⇒ 条件 2 2 2证明:条件 3 ⇒ 3 \Rightarrow 3⇒条件 1…...

HighTec工程用命令行编译
当工程中含有太多模型生成的代码的时候,如果修改了一部分代码,HighTec自带的编译器编译时间会非常的慢,有的需要半个小时甚至一个小时,这是因为每次修改之后HighTec都会从头重新检索更新,太浪费时间了,于是…...
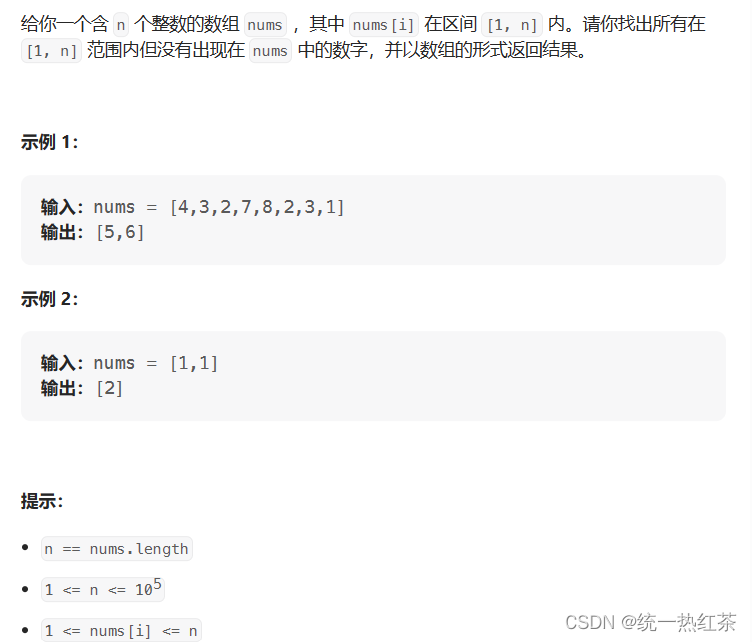
【C语言】每日一题(找到所有数组中消失的数字)
找到所有数组中消失的数字,链接奉上。 这里简单说一下,因为还没有接触到动态内存,数据结构,所以知识有限,也是尽力而为,结合题库的评论区找到了适合我的解法,以后有机会,会补上各种…...
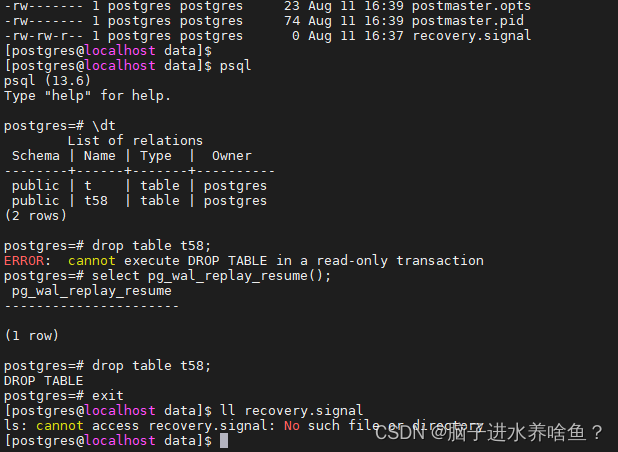
PostgreSql 备份恢复
一、概述 数据库备份一般可分为物理备份和逻辑备份,其中物理备份又可分为物理冷备和物理热备,下面就各种备份方式进行详细说明(一般情况下,生产环境采取的定时物理热备逻辑备份的方式,均是以下述方式为基础进一步研发编…...

鲲鹏916/920处理器性能比较
CPUKunpeng916Kunpeng920指令集Cotex-A75TaiShan-V110主频2.4GHz2.6GHz/3.0GHz核数3224/32/48/64CacheL1: 48 KB instruction cache and 32 KB data cache L2: 256 KB private per core L3: 32 MB L1: 64 KB instruction cache and 64 KB data cache L2: 512 KB private per co…...
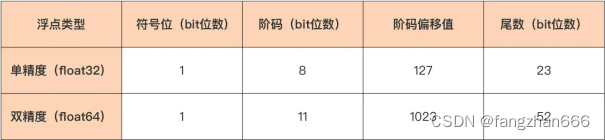
《Go 语言第一课》课程学习笔记(八)
基本数据类型 Go 原生支持的数值类型有哪些? Go 语言的类型大体可分为基本数据类型、复合数据类型和接口类型这三种。 其中,我们日常 Go 编码中使用最多的就是基本数据类型,而基本数据类型中使用占比最大的又是数值类型。 整型 Go 语言的…...

管理类联考——逻辑——真题篇——按知识分类——汇总篇——一、形式逻辑——联选言
文章目录 第五节 联言+选言-摩根定理-非(A或B)=非A且非B,非(A且B)=非A或非B真题(2013-49)-联言+选言-摩根定理-非(A或B)=非A且非B,非(A且B)=非A或非B真题(2012-33)-联言+选言-摩根定理-非(A或B)=非A且非B,非(A且B)=非A或非B真题(2014-42)-联言+选言-摩根定理-非(A或B…...
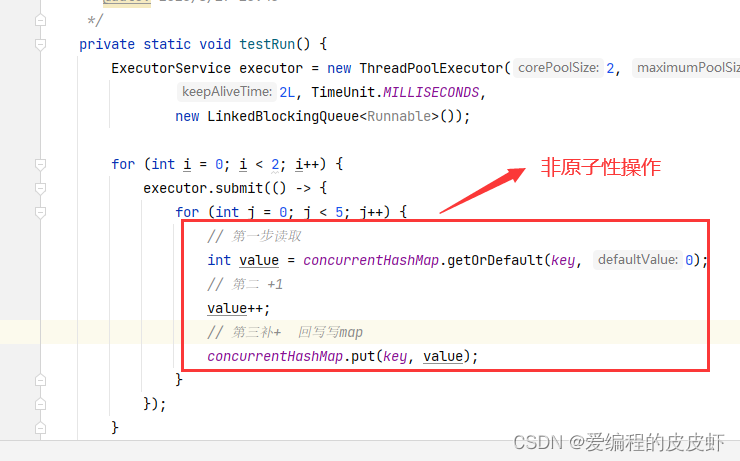
CAS 一些隐藏的知识,您了解吗
目录 ConcurrentHashMap 一定是线程安全的吗 CAS 机制的注意事项 使用java 并行流 ,您要留意了 ConcurrentHashMap 在JDK1.8中ConcurrentHashMap 内部使用的是数组加链表加红黑树的结构,通过CASvolatile或synchronized的方式来保证线程安全的,这些原理…...
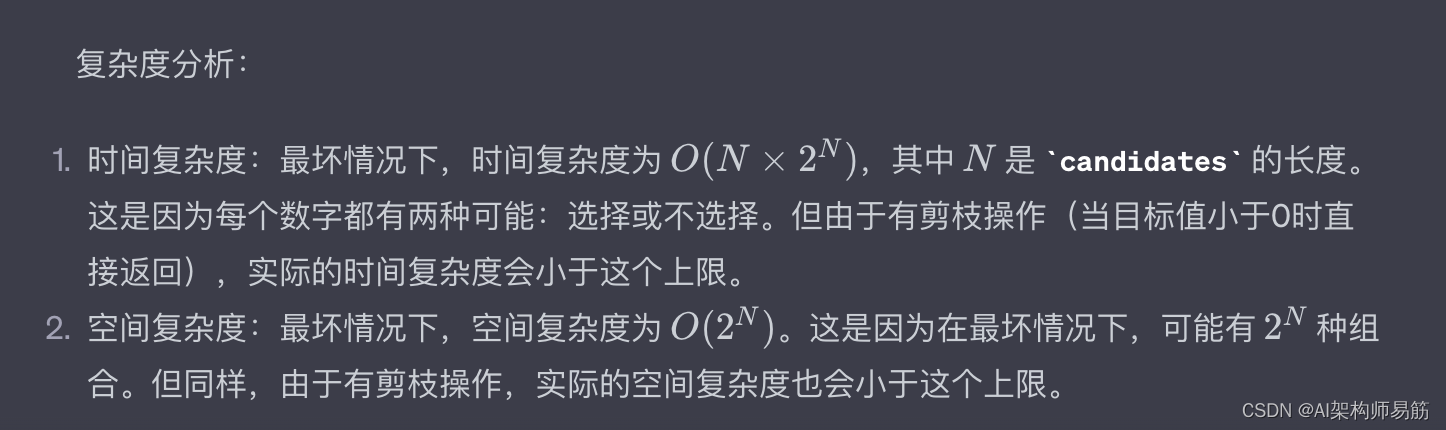
ChatGPT逐句逐句地解释代码并分析复杂度的提示词prompt
前提安装chrome 插件 AI Prompt Genius, 请参考 3 个 ChatGPT 插件您需要立即下载 你是首席软件工程师。请解释这段代码:{{code}} 添加注释并重写代码,用注释解释每一行代码的作用。最后分析复杂度。快捷键 / 选择 Explain Code 输入代码提…...

【Lua语法】算术、条件、逻辑、位、三目运算符
1.算术运算符 加减乘除取余: - * / % Lua中独有的:幂运算 ^ 注意: 1.Lua中没有自增自减(、–),也没有复合运算符(、-) 2.Lua中字符串可以进行算术运算符操作,会自动转成number 如:“10.3” 1 结果为11.3…...
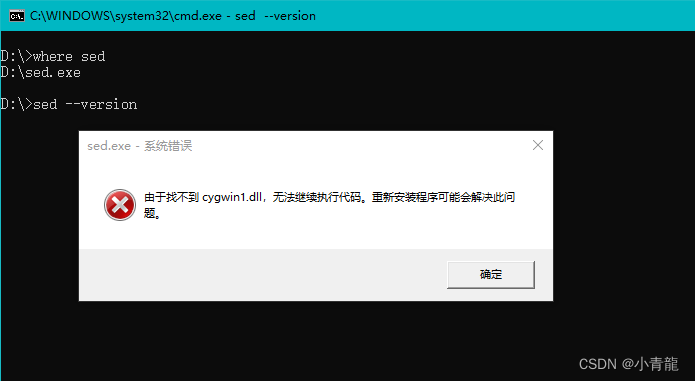
Cygwin 配置C/C++编译环境以及如何编译项目
文章目录 一、安装C、C编译环境需要的包1. 选择gcc-core、gcc-g2. 选择gdb3. 选择mingw64下的gcc-core、gcc-g4. 选择make5. 选择cmake6. 确认更改7. 查看包安装状态 二、C、C 项目编译示例step1:解压缩sed-4.9.tar.gzstep2:执行./configure生成Makefile…...
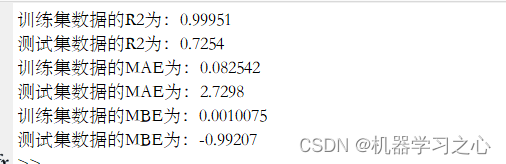
回归预测 | MATLAB实现FA-BP萤火虫算法优化BP神经网络多输入单输出回归预测(多指标,多图)
回归预测 | MATLAB实现FA-BP萤火虫算法优化BP神经网络多输入单输出回归预测(多指标,多图) 目录 回归预测 | MATLAB实现FA-BP萤火虫算法优化BP神经网络多输入单输出回归预测(多指标,多图)效果一览基本介绍程…...
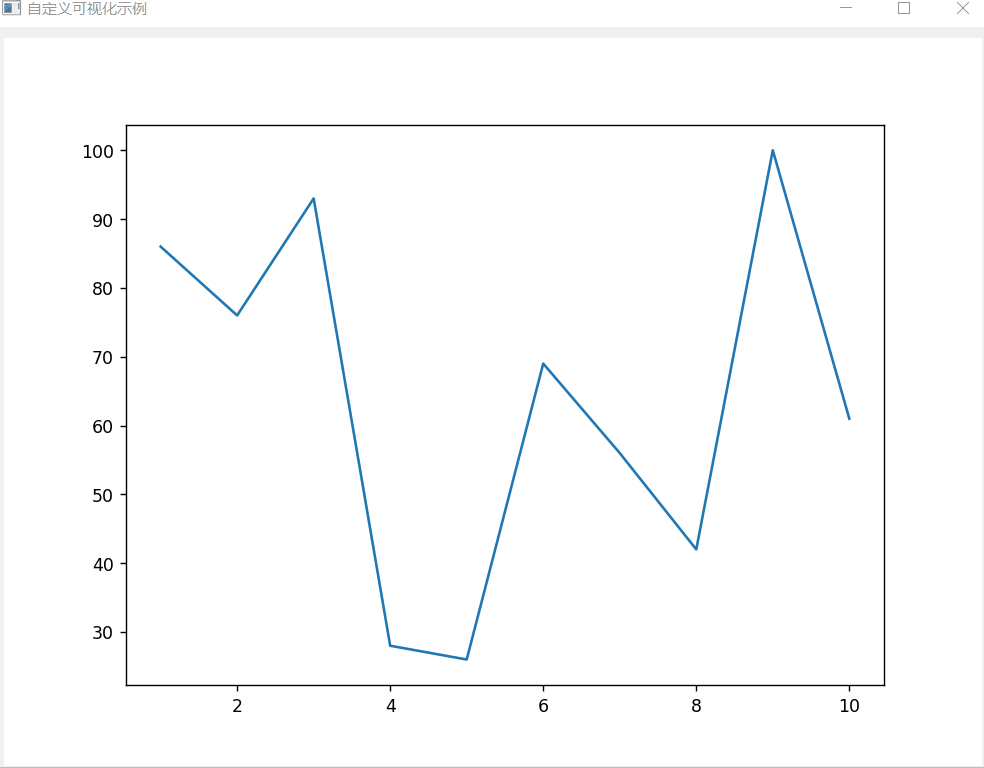
【100天精通python】Day39:GUI界面编程_PyQt 从入门到实战(下)_图形绘制和动画效果,数据可视化,刷新交互
目录 专栏导读 6 图形绘制与动画效果 6.1 绘制基本图形、文本和图片 6.2 实现动画效果和过渡效果 7 数据可视化 7.1 使用 Matplotlib绘制图表 7.2 使用PyQtGraph绘制图表 7.3 数据的实时刷新和交互操作 7.3.1 数据的实时刷新 7.3.2 交互操作 7.4 自定义数据可视化…...
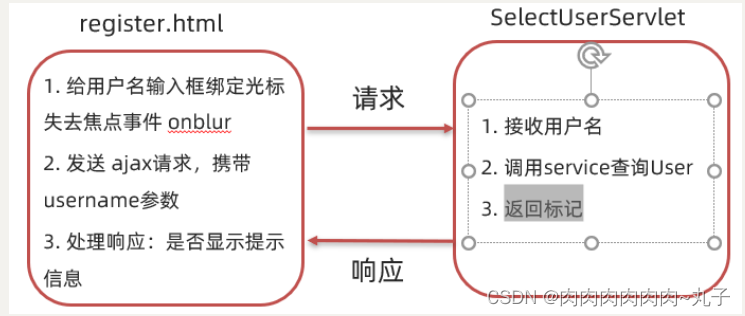
Java课题笔记~ Ajax
1.1 概述 AJAX (Asynchronous JavaScript And XML):异步的 JavaScript 和 XML。 我们先来说概念中的 JavaScript 和 XML,JavaScript 表明该技术和前端相关;XML 是指以此进行数据交换。 1.1.1 作用 AJAX 作用有以下两方面: 与服…...
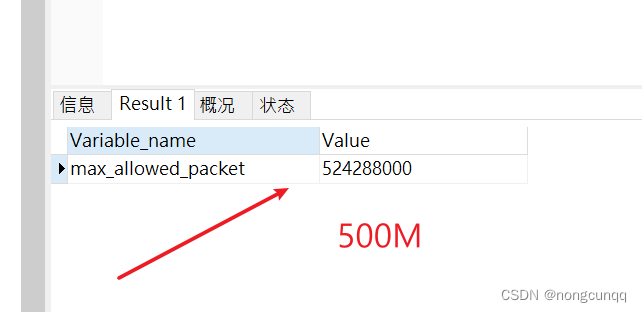
调整mysql 最大传输数据 max_allowed_packet=500M
查看 -- show VARIABLES like %max_allowed_packet%; -- set global max_allowed_packet 1024*1024*64;-- show variables like %timeout%; -- show global status like com_kill; show global variables like max_allowed_packet; -- set global max_allowed_packet1024*102…...
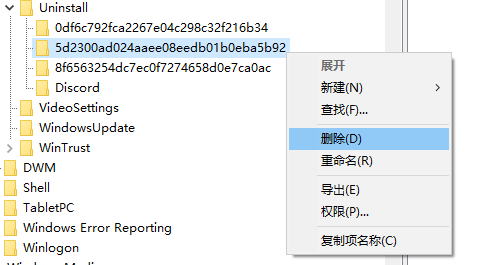
【工具】 删除Chrome安装的“创建快捷方式”
创建Chrome的快捷方式,可以放在桌面,想用时双击就可以打开网页,比书签(brookmark)结构化管理更方便。 但是,安装一时爽,卸载有问题。 如果用 windows 控制面板\所有控制面板项\程序和功能 卸载…...
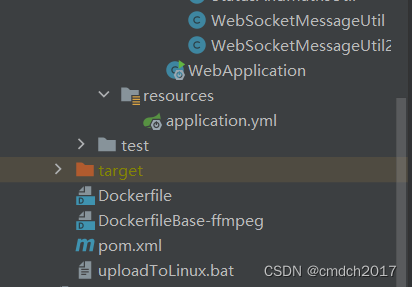
windows上的docker自动化部署到服务器脚本
1、mvn install后,双击这个bat,实现docker build后上传到124服务器,并且重启124服务器 **echo offsetlocal:: 定义镜像名称和版本变量 set IMAGE_NAMEweb set IMAGE_VERSION1.3.1:: 清理本地文件 echo Cleaning up... del service-%IMAGE_N…...
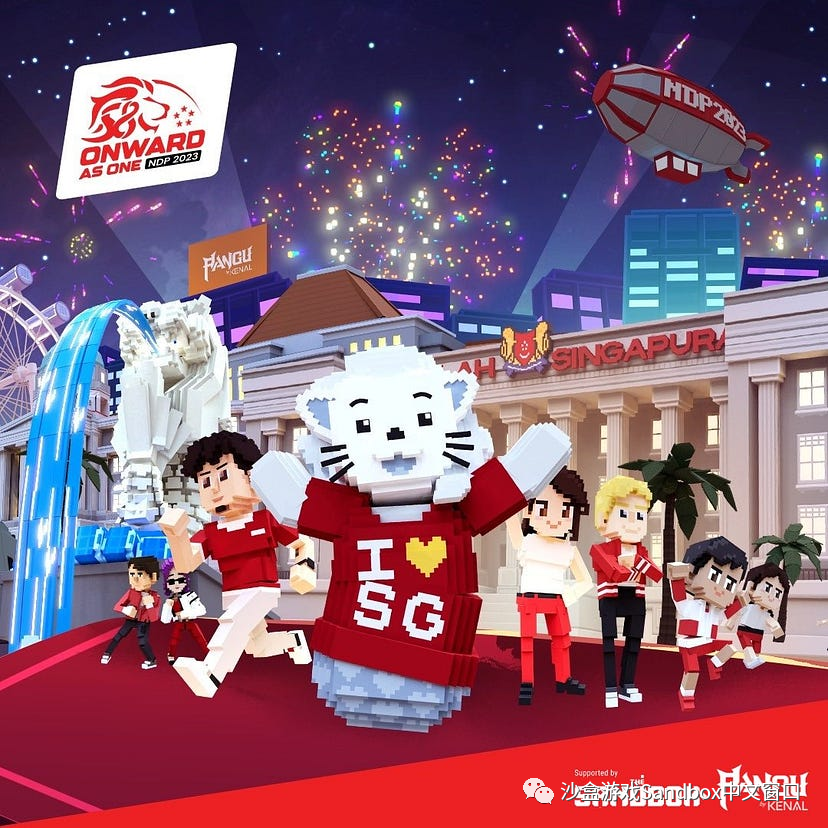
VoxWeekly|The Sandbox 生态周报|20230814
欢迎来到由 The Sandbox 发布的《VoxWeekly》。我们会在每周发布,对上一周 The Sandbox 生态系统所发生的事情进行总结。 如果你喜欢我们内容,欢迎与朋友和家人分享。请订阅我们的 Medium 、关注我们的 Twitter,并加入 Discord 社区…...
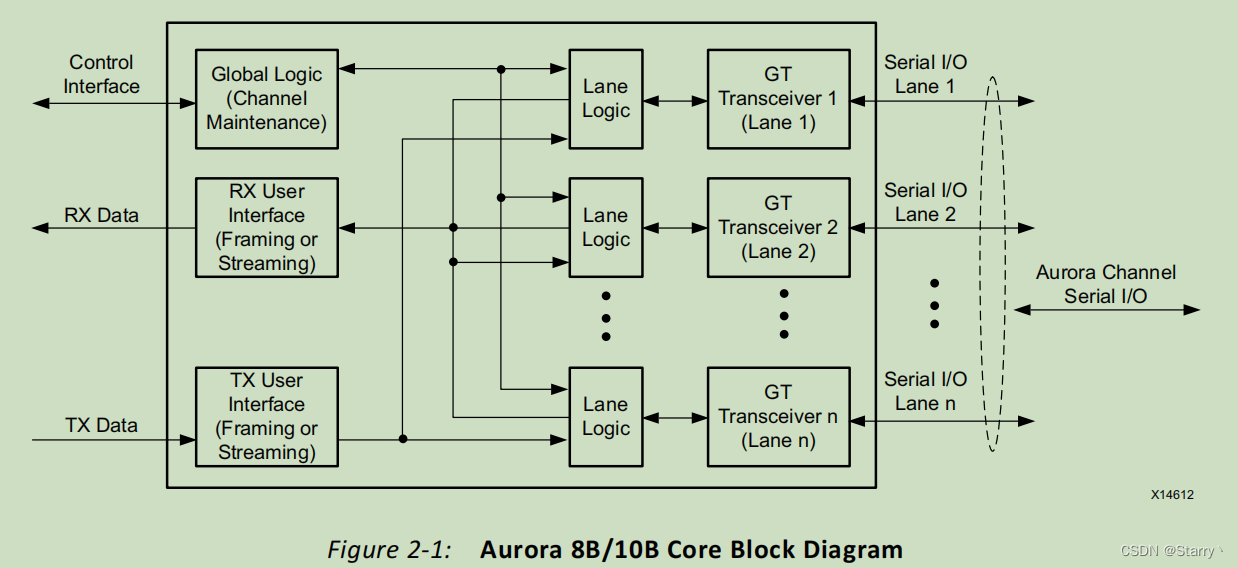
Aurora 8B/10B
目录 1. Overview2. Feature List2. Block Diagram3. Ports Description3.1. User InterfaceFraming InterfaceStreaming InterfaceUser Flow Control(UFC)Native Flow Control(NFC) 3.2. Status and Control Ports3.3. Transceiv…...
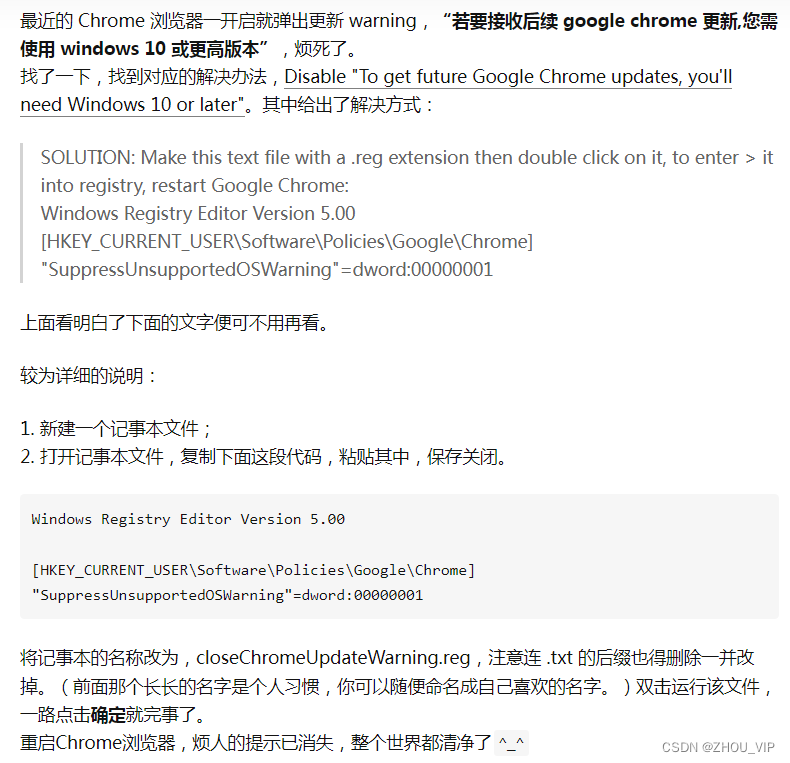
如何关闭“若要接收后续google chrome更新,您需使用windows10或更高版本”
Windows Registry Editor Version 5.00[HKEY_CURRENT_USER\Software\Policies\Google\Chrome] "SuppressUnsupportedOSWarning"dword:00000001 如何关闭“若要接收后续 google chrome 更新,您需使用 windows 10 或更高版本” - 知乎...
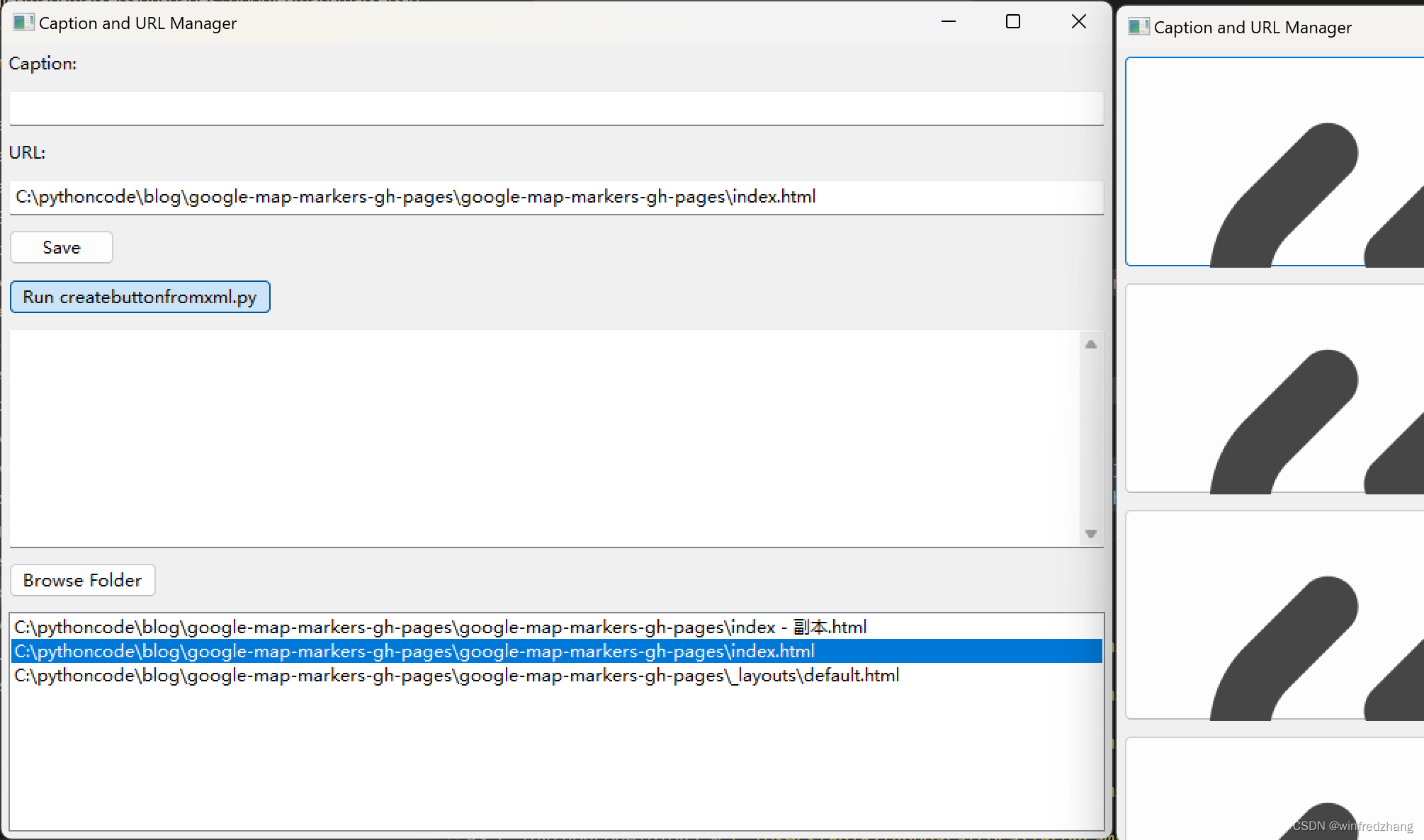
python中使用xml快速创建Caption和URL书签管理器应用程序
导语: 本文介绍如何使用wxPython库创建一个Caption和URL管理器应用程序。该应用程序具有图形用户界面,允许用户输入Caption和URL,并将其保存到XML文件中。此外,还提供了浏览文件夹并选择HTML文件的功能,并可以运行另一…...
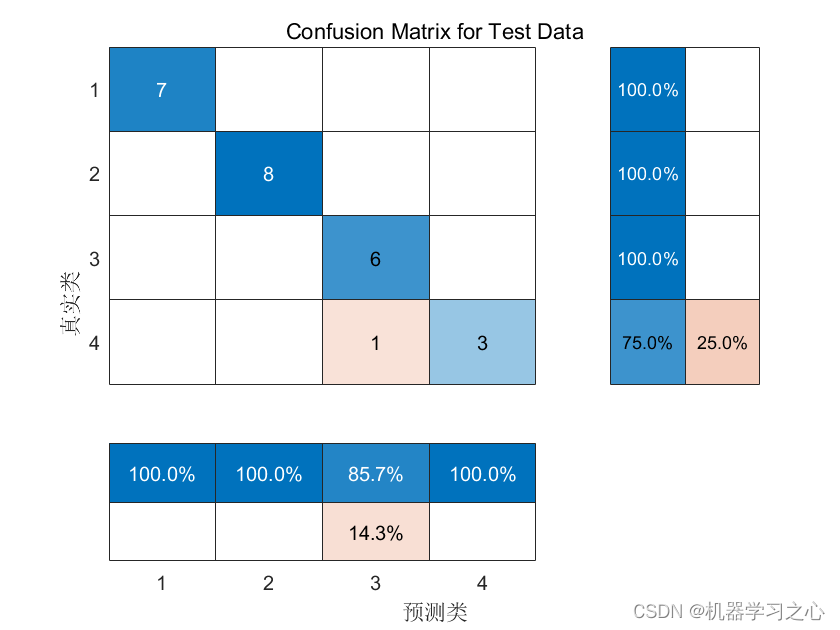
分类预测 | MATLAB实现DBN-SVM深度置信网络结合支持向量机多输入分类预测
分类预测 | MATLAB实现DBN-SVM深度置信网络结合支持向量机多输入分类预测 目录 分类预测 | MATLAB实现DBN-SVM深度置信网络结合支持向量机多输入分类预测预测效果基本介绍程序设计参考资料 预测效果 基本介绍 1.分类预测 | MATLAB实现DBN-SVM深度置信网络结合支持向量机多输入分…...