人脸老化预测(Python)
本次项目的文件
main.py主程序如下
-
导入必要的库和模块:
- 导入 TensorFlow 库以及自定义的
FaceAging
模块。 - 导入操作系统库和参数解析库。
- 导入 TensorFlow 库以及自定义的
-
定义
str2bool
函数:- 自定义函数用于将字符串转换为布尔值。
-
创建命令行参数解析器:
- 使用
argparse.ArgumentParser
创建解析器,设置命令行参数的相关信息,如是否训练、轮数、数据集名称等。
- 使用
-
主函数
main(_)
入口:- 打印设置的参数。
- 配置 TensorFlow 会话,设置 GPU 使用等。
-
在
with tf.Session(config=config) as session
中:- 创建
FaceAging
模型实例,传入会话、训练模式标志、保存路径和数据集名称。
- 创建
-
判断是否训练模式:
- 如果是训练模式,根据参数决定是否使用预训练模型进行训练。
- 如果不使用预训练模型,执行预训练步骤,并在预训练完成后开始正式训练。
- 执行模型的训练方法,传入训练轮数等参数。
-
如果不是训练模式:
- 进入测试模式,执行模型的自定义测试方法,传入测试图像目录。
-
在
__name__ == '__main__'
中执行程序:- 执行命令行参数解析和主函数。
import tensorflow as tf from FaceAging import FaceAging # 导入自定义的 FaceAging 模块 from os import environ import argparse# 设置环境变量,控制 TensorFlow 输出日志等级 environ['TF_CPP_MIN_LOG_LEVEL'] = '3'# 自定义一个函数用于将字符串转换为布尔值 def str2bool(v):if v.lower() in ('yes', 'true', 't', 'y', '1'):return Trueelif v.lower() in ('no', 'false', 'f', 'n', '0'):return Falseelse:raise argparse.ArgumentTypeError('Boolean value expected.')# 创建命令行参数解析器 parser = argparse.ArgumentParser(description='CAAE') parser.add_argument('--is_train', type=str2bool, default=True, help='是否进行训练') parser.add_argument('--epoch', type=int, default=50, help='训练的轮数') parser.add_argument('--dataset', type=str, default='UTKFace', help='存储在./data目录中的训练数据集名称') parser.add_argument('--savedir', type=str, default='save', help='保存检查点、中间训练结果和摘要的目录') parser.add_argument('--testdir', type=str, default='None', help='测试图像所在的目录') parser.add_argument('--use_trained_model', type=str2bool, default=True, help='是否使用已有的模型进行训练') parser.add_argument('--use_init_model', type=str2bool, default=True, help='如果找不到已有模型,是否从初始模型开始训练') FLAGS = parser.parse_args()# 主函数入口 def main(_):# 打印设置参数import pprintpprint.pprint(FLAGS)# 配置 TensorFlow 会话config = tf.ConfigProto()config.gpu_options.allow_growth = Truewith tf.Session(config=config) as session:# 创建 FaceAging 模型实例model = FaceAging(session, # TensorFlow 会话is_training=FLAGS.is_train, # 是否为训练模式的标志save_dir=FLAGS.savedir, # 保存检查点、样本和摘要的路径dataset_name=FLAGS.dataset # 存储在 ./data 目录中的数据集名称)if FLAGS.is_train:print ('\n\t训练模式')if not FLAGS.use_trained_model:print ('\n\t预训练网络')model.train(num_epochs=10, # 训练轮数use_trained_model=FLAGS.use_trained_model,use_init_model=FLAGS.use_init_model,weights=(0, 0, 0))print ('\n\t预训练完成!训练将开始。')model.train(num_epochs=FLAGS.epoch, # 训练轮数use_trained_model=FLAGS.use_trained_model,use_init_model=FLAGS.use_init_model)else:print ('\n\t测试模式')model.custom_test(testing_samples_dir=FLAGS.testdir + '/*jpg')if __name__ == '__main__':# 在主程序中执行命令行解析和执行主函数tf.app.run()
2.FaceAging.py
主要流程
-
导入必要的库和模块:
- 导入所需的Python库,如NumPy、TensorFlow等。
- 导入自定义的操作(ops.py)。
-
定义
FaceAging
类:- 在初始化方法中,设置了模型的各种参数,例如输入图像大小、网络层参数、训练参数等,并创建了 TensorFlow 图的输入节点。
- 定义了图的结构,包括编码器、生成器、判别器等。
- 定义了损失函数,包括生成器、判别器、总变差(TV)等。
- 收集了需要用于TensorBoard可视化的摘要信息。
-
train
方法:- 从文件中加载训练数据集的文件名列表。
- 定义了优化器和损失函数,然后进行模型的训练。
- 在每个epoch中,随机选择一部分训练图像样本,计算并更新生成器和判别器的参数,输出训练进度等信息。
- 保存模型的中间检查点,生成样本图像用于可视化,训练结束后保存最终模型。
-
encoder
方法:- 实现了编码器结构,将输入图像转化为对应的噪声或特征。
-
generator
方法:- 实现了生成器结构,将噪声特征、年龄标签和性别标签拼接,生成相应年龄段的人脸图像。
-
discriminator_z
和discriminator_img
方法:- 实现了判别器结构,对输入的噪声特征或图像进行判别。
-
save_checkpoint
和load_checkpoint
方法:- 用于保存和加载训练过程中的模型检查点。
-
sample
和test
方法:- 生成一些样本图像以及将训练过程中的中间结果保存为图片。
-
custom_test
方法:- 运行模型进行自定义测试,加载模型并生成特定人脸的年龄化效果。
from __future__ import division
import os
import time
from glob import glob
import tensorflow as tf
import numpy as np
from scipy.io import savemat
from ops import *class FaceAging(object):def __init__(self,session, # TensorFlow sessionsize_image=128, # size the input imagessize_kernel=5, # size of the kernels in convolution and deconvolutionsize_batch=100, # mini-batch size for training and testing, must be square of an integernum_input_channels=3, # number of channels of input imagesnum_encoder_channels=64, # number of channels of the first conv layer of encodernum_z_channels=50, # number of channels of the layer z (noise or code)num_categories=10, # number of categories (age segments) in the training datasetnum_gen_channels=1024, # number of channels of the first deconv layer of generatorenable_tile_label=True, # enable to tile the labeltile_ratio=1.0, # ratio of the length between tiled label and zis_training=True, # flag for training or testing modesave_dir='./save', # path to save checkpoints, samples, and summarydataset_name='UTKFace' # name of the dataset in the folder ./data):self.session = sessionself.image_value_range = (-1, 1)self.size_image = size_imageself.size_kernel = size_kernelself.size_batch = size_batchself.num_input_channels = num_input_channelsself.num_encoder_channels = num_encoder_channelsself.num_z_channels = num_z_channelsself.num_categories = num_categoriesself.num_gen_channels = num_gen_channelsself.enable_tile_label = enable_tile_labelself.tile_ratio = tile_ratioself.is_training = is_trainingself.save_dir = save_dirself.dataset_name = dataset_name# ************************************* input to graph ********************************************************self.input_image = tf.placeholder(tf.float32,[self.size_batch, self.size_image, self.size_image, self.num_input_channels],name='input_images')self.age = tf.placeholder(tf.float32,[self.size_batch, self.num_categories],name='age_labels')self.gender = tf.placeholder(tf.float32,[self.size_batch, 2],name='gender_labels')self.z_prior = tf.placeholder(tf.float32,[self.size_batch, self.num_z_channels],name='z_prior')# ************************************* build the graph *******************************************************print ('\n\tBuilding graph ...')# encoder: input image --> zself.z = self.encoder(image=self.input_image)# generator: z + label --> generated imageself.G = self.generator(z=self.z,y=self.age,gender=self.gender,enable_tile_label=self.enable_tile_label,tile_ratio=self.tile_ratio)# discriminator on zself.D_z, self.D_z_logits = self.discriminator_z(z=self.z,is_training=self.is_training)# discriminator on Gself.D_G, self.D_G_logits = self.discriminator_img(image=self.G,y=self.age,gender=self.gender,is_training=self.is_training)# discriminator on z_priorself.D_z_prior, self.D_z_prior_logits = self.discriminator_z(z=self.z_prior,is_training=self.is_training,reuse_variables=True)# discriminator on input imageself.D_input, self.D_input_logits = self.discriminator_img(image=self.input_image,y=self.age,gender=self.gender,is_training=self.is_training,reuse_variables=True)# ************************************* loss functions *******************************************************# loss function of encoder + generator#self.EG_loss = tf.nn.l2_loss(self.input_image - self.G) / self.size_batch # L2 lossself.EG_loss = tf.reduce_mean(tf.abs(self.input_image - self.G)) # L1 loss# loss function of discriminator on zself.D_z_loss_prior = tf.reduce_mean(tf.nn.sigmoid_cross_entropy_with_logits(logits=self.D_z_prior_logits, labels=tf.ones_like(self.D_z_prior_logits)))self.D_z_loss_z = tf.reduce_mean(tf.nn.sigmoid_cross_entropy_with_logits(logits=self.D_z_logits, labels=tf.zeros_like(self.D_z_logits)))self.E_z_loss = tf.reduce_mean(tf.nn.sigmoid_cross_entropy_with_logits(logits=self.D_z_logits, labels=tf.ones_like(self.D_z_logits)))# loss function of discriminator on imageself.D_img_loss_input = tf.reduce_mean(tf.nn.sigmoid_cross_entropy_with_logits(logits=self.D_input_logits, labels=tf.ones_like(self.D_input_logits)))self.D_img_loss_G = tf.reduce_mean(tf.nn.sigmoid_cross_entropy_with_logits(logits=self.D_G_logits, labels=tf.zeros_like(self.D_G_logits)))self.G_img_loss = tf.reduce_mean(tf.nn.sigmoid_cross_entropy_with_logits(logits=self.D_G_logits, labels=tf.ones_like(self.D_G_logits)))# total variation to smooth the generated imagetv_y_size = self.size_imagetv_x_size = self.size_imageself.tv_loss = ((tf.nn.l2_loss(self.G[:, 1:, :, :] - self.G[:, :self.size_image - 1, :, :]) / tv_y_size) +(tf.nn.l2_loss(self.G[:, :, 1:, :] - self.G[:, :, :self.size_image - 1, :]) / tv_x_size)) / self.size_batch# *********************************** trainable variables ****************************************************trainable_variables = tf.trainable_variables()# variables of encoderself.E_variables = [var for var in trainable_variables if 'E_' in var.name]# variables of generatorself.G_variables = [var for var in trainable_variables if 'G_' in var.name]# variables of discriminator on zself.D_z_variables = [var for var in trainable_variables if 'D_z_' in var.name]# variables of discriminator on imageself.D_img_variables = [var for var in trainable_variables if 'D_img_' in var.name]# ************************************* collect the summary ***************************************self.z_summary = tf.summary.histogram('z', self.z)self.z_prior_summary = tf.summary.histogram('z_prior', self.z_prior)self.EG_loss_summary = tf.summary.scalar('EG_loss', self.EG_loss)self.D_z_loss_z_summary = tf.summary.scalar('D_z_loss_z', self.D_z_loss_z)self.D_z_loss_prior_summary = tf.summary.scalar('D_z_loss_prior', self.D_z_loss_prior)self.E_z_loss_summary = tf.summary.scalar('E_z_loss', self.E_z_loss)self.D_z_logits_summary = tf.summary.histogram('D_z_logits', self.D_z_logits)self.D_z_prior_logits_summary = tf.summary.histogram('D_z_prior_logits', self.D_z_prior_logits)self.D_img_loss_input_summary = tf.summary.scalar('D_img_loss_input', self.D_img_loss_input)self.D_img_loss_G_summary = tf.summary.scalar('D_img_loss_G', self.D_img_loss_G)self.G_img_loss_summary = tf.summary.scalar('G_img_loss', self.G_img_loss)self.D_G_logits_summary = tf.summary.histogram('D_G_logits', self.D_G_logits)self.D_input_logits_summary = tf.summary.histogram('D_input_logits', self.D_input_logits)# for saving the graph and variablesself.saver = tf.train.Saver(max_to_keep=2)def train(self,num_epochs=200, # number of epochslearning_rate=0.0002, # learning rate of optimizerbeta1=0.5, # parameter for Adam optimizerdecay_rate=1.0, # learning rate decay (0, 1], 1 means no decayenable_shuffle=True, # enable shuffle of the datasetuse_trained_model=True, # use the saved checkpoint to initialize the networkuse_init_model=True, # use the init model to initialize the networkweigts=(0.0001, 0, 0) # the weights of adversarial loss and TV loss):# *************************** load file names of images ******************************************************file_names = glob(os.path.join('./data', self.dataset_name, '*.jpg'))size_data = len(file_names)np.random.seed(seed=2017)if enable_shuffle:np.random.shuffle(file_names)# *********************************** optimizer **************************************************************# over all, there are three loss functions, weights may differ from the paper because of different datasetsself.loss_EG = self.EG_loss + weigts[0] * self.G_img_loss + weigts[1] * self.E_z_loss + weigts[2] * self.tv_loss # slightly increase the paramsself.loss_Dz = self.D_z_loss_prior + self.D_z_loss_zself.loss_Di = self.D_img_loss_input + self.D_img_loss_G# set learning rate decayself.EG_global_step = tf.Variable(0, trainable=False, name='global_step')EG_learning_rate = tf.train.exponential_decay(learning_rate=learning_rate,global_step=self.EG_global_step,decay_steps=size_data / self.size_batch * 2,decay_rate=decay_rate,staircase=True)# optimizer for encoder + generatorwith tf.variable_scope('opt', reuse=tf.AUTO_REUSE):self.EG_optimizer = tf.train.AdamOptimizer(learning_rate=EG_learning_rate,beta1=beta1).minimize(loss=self.loss_EG,global_step=self.EG_global_step,var_list=self.E_variables + self.G_variables)# optimizer for discriminator on zself.D_z_optimizer = tf.train.AdamOptimizer(learning_rate=EG_learning_rate,beta1=beta1).minimize(loss=self.loss_Dz,var_list=self.D_z_variables)# optimizer for discriminator on imageself.D_img_optimizer = tf.train.AdamOptimizer(learning_rate=EG_learning_rate,beta1=beta1).minimize(loss=self.loss_Di,var_list=self.D_img_variables)# *********************************** tensorboard *************************************************************# for visualization (TensorBoard): $ tensorboard --logdir path/to/log-directoryself.EG_learning_rate_summary = tf.summary.scalar('EG_learning_rate', EG_learning_rate)self.summary = tf.summary.merge([self.z_summary, self.z_prior_summary,self.D_z_loss_z_summary, self.D_z_loss_prior_summary,self.D_z_logits_summary, self.D_z_prior_logits_summary,self.EG_loss_summary, self.E_z_loss_summary,self.D_img_loss_input_summary, self.D_img_loss_G_summary,self.G_img_loss_summary, self.EG_learning_rate_summary,self.D_G_logits_summary, self.D_input_logits_summary])self.writer = tf.summary.FileWriter(os.path.join(self.save_dir, 'summary'), self.session.graph)# ************* get some random samples as testing data to visualize the learning process *********************sample_files = file_names[0:self.size_batch]file_names[0:self.size_batch] = []sample = [load_image(image_path=sample_file,image_size=self.size_image,image_value_range=self.image_value_range,is_gray=(self.num_input_channels == 1),) for sample_file in sample_files]if self.num_input_channels == 1:sample_images = np.array(sample).astype(np.float32)[:, :, :, None]else:sample_images = np.array(sample).astype(np.float32)sample_label_age = np.ones(shape=(len(sample_files), self.num_categories),dtype=np.float32) * self.image_value_range[0]sample_label_gender = np.ones(shape=(len(sample_files), 2),dtype=np.float32) * self.image_value_range[0]for i, label in enumerate(sample_files):label = int(str(sample_files[i]).split('/')[-1].split('_')[0])if 0 <= label <= 5:label = 0elif 6 <= label <= 10:label = 1elif 11 <= label <= 15:label = 2elif 16 <= label <= 20:label = 3elif 21 <= label <= 30:label = 4elif 31 <= label <= 40:label = 5elif 41 <= label <= 50:label = 6elif 51 <= label <= 60:label = 7elif 61 <= label <= 70:label = 8else:label = 9sample_label_age[i, label] = self.image_value_range[-1]gender = int(str(sample_files[i]).split('/')[-1].split('_')[1])sample_label_gender[i, gender] = self.image_value_range[-1]# ******************************************* training *******************************************************# initialize the graphtf.global_variables_initializer().run()# load check pointif use_trained_model:if self.load_checkpoint():print("\tSUCCESS ^_^")else:print("\tFAILED >_<!")# load init modelif use_init_model:if not os.path.exists('init_model/model-init.data-00000-of-00001'):from init_model.zip_opt import jointry:join('init_model/model_parts', 'init_model/model-init.data-00000-of-00001')except:raise Exception('Error joining files')self.load_checkpoint(model_path='init_model')# epoch iterationnum_batches = len(file_names) // self.size_batchfor epoch in range(num_epochs):if enable_shuffle:np.random.shuffle(file_names)for ind_batch in range(num_batches):start_time = time.time()# read batch images and labelsbatch_files = file_names[ind_batch*self.size_batch:(ind_batch+1)*self.size_batch]batch = [load_image(image_path=batch_file,image_size=self.size_image,image_value_range=self.image_value_range,is_gray=(self.num_input_channels == 1),) for batch_file in batch_files]if self.num_input_channels == 1:batch_images = np.array(batch).astype(np.float32)[:, :, :, None]else:batch_images = np.array(batch).astype(np.float32)batch_label_age = np.ones(shape=(len(batch_files), self.num_categories),dtype=np.float) * self.image_value_range[0]batch_label_gender = np.ones(shape=(len(batch_files), 2),dtype=np.float) * self.image_value_range[0]for i, label in enumerate(batch_files):label = int(str(batch_files[i]).split('/')[-1].split('_')[0])if 0 <= label <= 5:label = 0elif 6 <= label <= 10:label = 1elif 11 <= label <= 15:label = 2elif 16 <= label <= 20:label = 3elif 21 <= label <= 30:label = 4elif 31 <= label <= 40:label = 5elif 41 <= label <= 50:label = 6elif 51 <= label <= 60:label = 7elif 61 <= label <= 70:label = 8else:label = 9batch_label_age[i, label] = self.image_value_range[-1]gender = int(str(batch_files[i]).split('/')[-1].split('_')[1])batch_label_gender[i, gender] = self.image_value_range[-1]# prior distribution on the prior of zbatch_z_prior = np.random.uniform(self.image_value_range[0],self.image_value_range[-1],[self.size_batch, self.num_z_channels]).astype(np.float32)# update_, _, _, EG_err, Ez_err, Dz_err, Dzp_err, Gi_err, DiG_err, Di_err, TV = self.session.run(fetches = [self.EG_optimizer,self.D_z_optimizer,self.D_img_optimizer,self.EG_loss,self.E_z_loss,self.D_z_loss_z,self.D_z_loss_prior,self.G_img_loss,self.D_img_loss_G,self.D_img_loss_input,self.tv_loss],feed_dict={self.input_image: batch_images,self.age: batch_label_age,self.gender: batch_label_gender,self.z_prior: batch_z_prior})print("\nEpoch: [%3d/%3d] Batch: [%3d/%3d]\n\tEG_err=%.4f\tTV=%.4f" %(epoch+1, num_epochs, ind_batch+1, num_batches, EG_err, TV))print("\tEz=%.4f\tDz=%.4f\tDzp=%.4f" % (Ez_err, Dz_err, Dzp_err))print("\tGi=%.4f\tDi=%.4f\tDiG=%.4f" % (Gi_err, Di_err, DiG_err))# estimate left run timeelapse = time.time() - start_timetime_left = ((num_epochs - epoch - 1) * num_batches + (num_batches - ind_batch - 1)) * elapseprint("\tTime left: %02d:%02d:%02d" %(int(time_left / 3600), int(time_left % 3600 / 60), time_left % 60))# add to summarysummary = self.summary.eval(feed_dict={self.input_image: batch_images,self.age: batch_label_age,self.gender: batch_label_gender,self.z_prior: batch_z_prior})self.writer.add_summary(summary, self.EG_global_step.eval())# save sample images for each epochname = '{:02d}.png'.format(epoch+1)self.sample(sample_images, sample_label_age, sample_label_gender, name)self.test(sample_images, sample_label_gender, name)# save checkpoint for each 5 epochif np.mod(epoch, 5) == 4:self.save_checkpoint()# save the trained modelself.save_checkpoint()# close the summary writerself.writer.close()def encoder(self, image, reuse_variables=False):if reuse_variables:tf.get_variable_scope().reuse_variables()num_layers = int(np.log2(self.size_image)) - int(self.size_kernel / 2)current = image# conv layers with stride 2for i in range(num_layers):name = 'E_conv' + str(i)current = conv2d(input_map=current,num_output_channels=self.num_encoder_channels * (2 ** i),size_kernel=self.size_kernel,name=name)current = tf.nn.relu(current)# fully connection layername = 'E_fc'current = fc(input_vector=tf.reshape(current, [self.size_batch, -1]),num_output_length=self.num_z_channels,name=name)# outputreturn tf.nn.tanh(current)def generator(self, z, y, gender, reuse_variables=False, enable_tile_label=True, tile_ratio=1.0):if reuse_variables:tf.get_variable_scope().reuse_variables()num_layers = int(np.log2(self.size_image)) - int(self.size_kernel / 2)if enable_tile_label:duplicate = int(self.num_z_channels * tile_ratio / self.num_categories)else:duplicate = 1z = concat_label(z, y, duplicate=duplicate)if enable_tile_label:duplicate = int(self.num_z_channels * tile_ratio / 2)else:duplicate = 1z = concat_label(z, gender, duplicate=duplicate)size_mini_map = int(self.size_image / 2 ** num_layers)# fc layername = 'G_fc'current = fc(input_vector=z,num_output_length=self.num_gen_channels * size_mini_map * size_mini_map,name=name)# reshape to cube for deconvcurrent = tf.reshape(current, [-1, size_mini_map, size_mini_map, self.num_gen_channels])current = tf.nn.relu(current)# deconv layers with stride 2for i in range(num_layers):name = 'G_deconv' + str(i)current = deconv2d(input_map=current,output_shape=[self.size_batch,size_mini_map * 2 ** (i + 1),size_mini_map * 2 ** (i + 1),int(self.num_gen_channels / 2 ** (i + 1))],size_kernel=self.size_kernel,name=name)current = tf.nn.relu(current)name = 'G_deconv' + str(i+1)current = deconv2d(input_map=current,output_shape=[self.size_batch,self.size_image,self.size_image,int(self.num_gen_channels / 2 ** (i + 2))],size_kernel=self.size_kernel,stride=1,name=name)current = tf.nn.relu(current)name = 'G_deconv' + str(i + 2)current = deconv2d(input_map=current,output_shape=[self.size_batch,self.size_image,self.size_image,self.num_input_channels],size_kernel=self.size_kernel,stride=1,name=name)# outputreturn tf.nn.tanh(current)def discriminator_z(self, z, is_training=True, reuse_variables=False, num_hidden_layer_channels=(64, 32, 16), enable_bn=True):if reuse_variables:tf.get_variable_scope().reuse_variables()current = z# fully connection layerfor i in range(len(num_hidden_layer_channels)):name = 'D_z_fc' + str(i)current = fc(input_vector=current,num_output_length=num_hidden_layer_channels[i],name=name)if enable_bn:name = 'D_z_bn' + str(i)current = tf.contrib.layers.batch_norm(current,scale=False,is_training=is_training,scope=name,reuse=reuse_variables)current = tf.nn.relu(current)# output layername = 'D_z_fc' + str(i+1)current = fc(input_vector=current,num_output_length=1,name=name)return tf.nn.sigmoid(current), currentdef discriminator_img(self, image, y, gender, is_training=True, reuse_variables=False, num_hidden_layer_channels=(16, 32, 64, 128), enable_bn=True):if reuse_variables:tf.get_variable_scope().reuse_variables()num_layers = len(num_hidden_layer_channels)current = image# conv layers with stride 2for i in range(num_layers):name = 'D_img_conv' + str(i)current = conv2d(input_map=current,num_output_channels=num_hidden_layer_channels[i],size_kernel=self.size_kernel,name=name)if enable_bn:name = 'D_img_bn' + str(i)current = tf.contrib.layers.batch_norm(current,scale=False,is_training=is_training,scope=name,reuse=reuse_variables)current = tf.nn.relu(current)if i == 0:current = concat_label(current, y)current = concat_label(current, gender, int(self.num_categories / 2))# fully connection layername = 'D_img_fc1'current = fc(input_vector=tf.reshape(current, [self.size_batch, -1]),num_output_length=1024,name=name)current = lrelu(current)name = 'D_img_fc2'current = fc(input_vector=current,num_output_length=1,name=name)# outputreturn tf.nn.sigmoid(current), currentdef save_checkpoint(self):checkpoint_dir = os.path.join(self.save_dir, 'checkpoint')if not os.path.exists(checkpoint_dir):os.makedirs(checkpoint_dir)self.saver.save(sess=self.session,save_path=os.path.join(checkpoint_dir, 'model'),global_step=self.EG_global_step.eval())def load_checkpoint(self, model_path=None):if model_path is None:print("\n\tLoading pre-trained model ...")checkpoint_dir = os.path.join(self.save_dir, 'checkpoint')else:print("\n\tLoading init model ...")checkpoint_dir = model_pathcheckpoints = tf.train.get_checkpoint_state(checkpoint_dir)if checkpoints and checkpoints.model_checkpoint_path:checkpoints_name = os.path.basename(checkpoints.model_checkpoint_path)try:self.saver.restore(self.session, os.path.join(checkpoint_dir, checkpoints_name))return Trueexcept:return Falseelse:return Falsedef sample(self, images, labels, gender, name):sample_dir = os.path.join(self.save_dir, 'samples')if not os.path.exists(sample_dir):os.makedirs(sample_dir)z, G = self.session.run([self.z, self.G],feed_dict={self.input_image: images,self.age: labels,self.gender: gender})size_frame = int(np.sqrt(self.size_batch))save_batch_images(batch_images=G,save_path=os.path.join(sample_dir, name),image_value_range=self.image_value_range,size_frame=[size_frame, size_frame])def test(self, images, gender, name):test_dir = os.path.join(self.save_dir, 'test')if not os.path.exists(test_dir):os.makedirs(test_dir)images = images[:int(np.sqrt(self.size_batch)), :, :, :]gender = gender[:int(np.sqrt(self.size_batch)), :]size_sample = images.shape[0]labels = np.arange(size_sample)labels = np.repeat(labels, size_sample)query_labels = np.ones(shape=(size_sample ** 2, size_sample),dtype=np.float32) * self.image_value_range[0]for i in range(query_labels.shape[0]):query_labels[i, labels[i]] = self.image_value_range[-1]query_images = np.tile(images, [self.num_categories, 1, 1, 1])query_gender = np.tile(gender, [self.num_categories, 1])z, G = self.session.run([self.z, self.G],feed_dict={self.input_image: query_images,self.age: query_labels,self.gender: query_gender})save_batch_images(batch_images=query_images,save_path=os.path.join(test_dir, 'input.png'),image_value_range=self.image_value_range,size_frame=[size_sample, size_sample])save_batch_images(batch_images=G,save_path=os.path.join(test_dir, name),image_value_range=self.image_value_range,size_frame=[size_sample, size_sample])def custom_test(self, testing_samples_dir):if not self.load_checkpoint():print("\tFAILED >_<!")exit(0)else:print("\tSUCCESS ^_^")num_samples = int(np.sqrt(self.size_batch))file_names = glob(testing_samples_dir)if len(file_names) < num_samples:print ('The number of testing images is must larger than %d' % num_samples)exit(0)sample_files = file_names[0:num_samples]sample = [load_image(image_path=sample_file,image_size=self.size_image,image_value_range=self.image_value_range,is_gray=(self.num_input_channels == 1),) for sample_file in sample_files]if self.num_input_channels == 1:images = np.array(sample).astype(np.float32)[:, :, :, None]else:images = np.array(sample).astype(np.float32)gender_male = np.ones(shape=(num_samples, 2),dtype=np.float32) * self.image_value_range[0]gender_female = np.ones(shape=(num_samples, 2),dtype=np.float32) * self.image_value_range[0]for i in range(gender_male.shape[0]):gender_male[i, 0] = self.image_value_range[-1]gender_female[i, 1] = self.image_value_range[-1]self.test(images, gender_male, 'test_as_male.png')self.test(images, gender_female, 'test_as_female.png')print ('\n\tDone! Results are saved as %s\n' % os.path.join(self.save_dir, 'test', 'test_as_xxx.png'))
3.data,一共23708张照片
4.对数据集感兴趣的可以关注
from __future__ import division
import os
import time
from glob import glob
import tensorflow as tf
import numpy as np
from scipy.io import savemat
from ops import *#https://mbd.pub/o/bread/ZJ2UmJpp
相关文章:
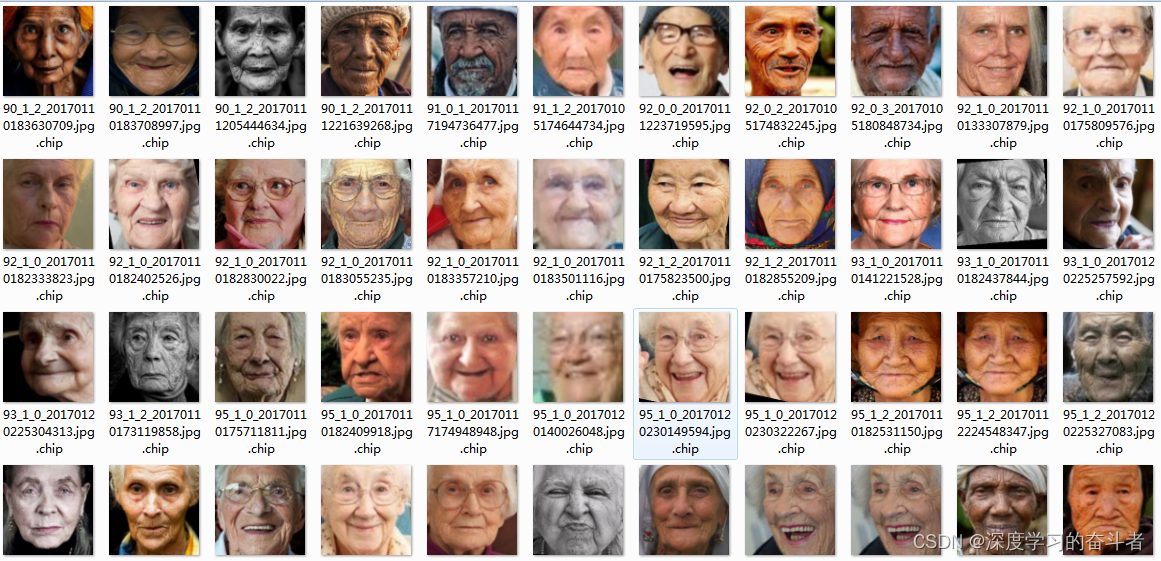
人脸老化预测(Python)
本次项目的文件 main.py主程序如下 导入必要的库和模块: 导入 TensorFlow 库以及自定义的 FaceAging 模块。导入操作系统库和参数解析库。 定义 str2bool 函数: 自定义函数用于将字符串转换为布尔值。 创建命令行参数解析器: 使用 argparse.A…...
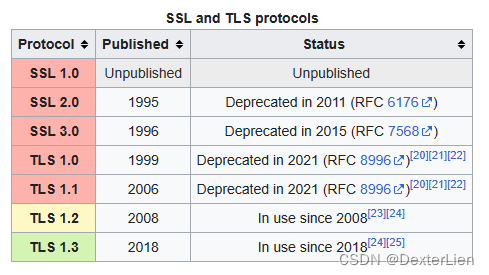
AWS SDK 3.x for .NET Framework 4.0 可行性测试
前言 为了应对日益增长的网络安全挑战, 越来越多的互联网厂商已经陆续开始或者已经彻底停止了对 SSL 3 / TLS 1.0 / TLS1.1 等上古加密算法的支持. 而对于一些同样拥有悠久历史的和 AWS 服务相关联的应用程序, 是否可以通过仅更新 SDK 版本的方式来适应新的环境. 本文将以 Win…...

两个list。如何使用流的写法将一个list中的对象中的某些属性根据另外一个list中的属性值赋值进去?
两个list。如何使用流的写法将一个list中的对象中的某些属性根据另外一个list中的属性值赋值进去? 你可以使用Java 8以上版本中的流(Stream)和Lambda表达式来实现这个需求。假设有两个List,一个是sourceList,包含要赋值属性的对象;另一个是…...
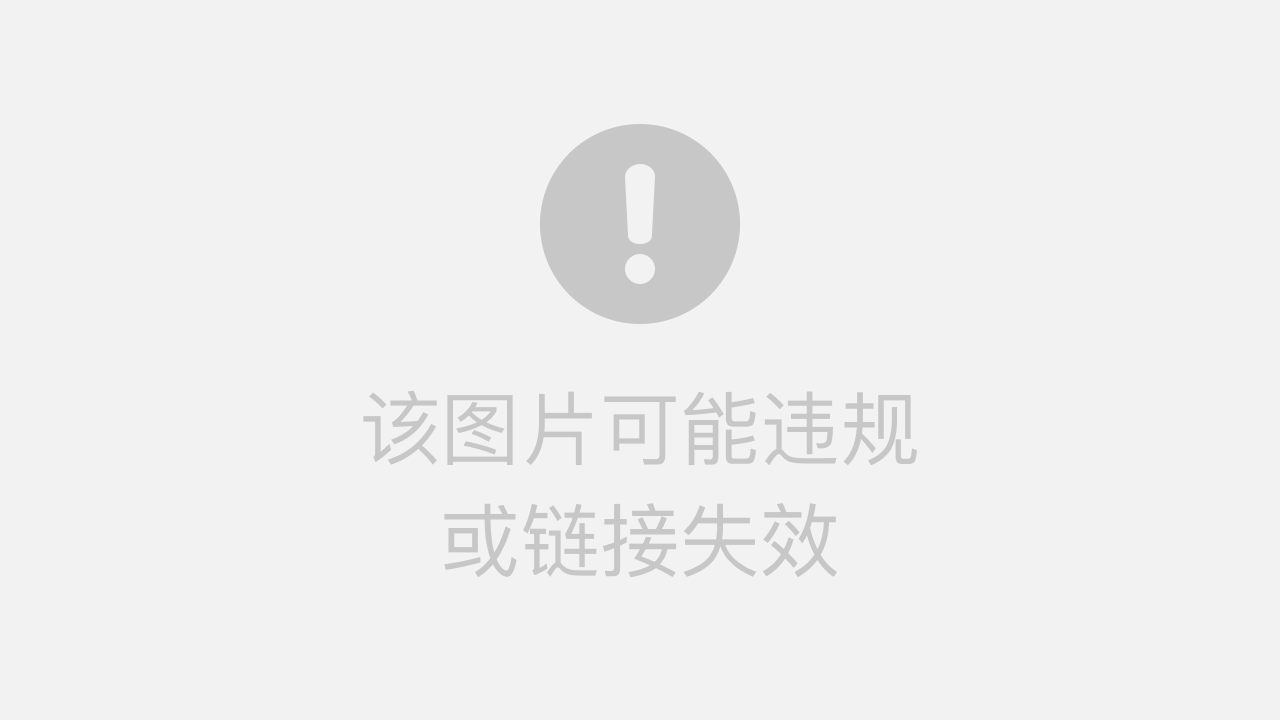
美国陆军希望大数据技术能够帮助保护其云安全
随着陆军采用更大型的云服务,一位高级官员警告说,一些在私营部门有效的快速软件开发技巧和简单解决方案(例如开放代码库)如果没有额外的安全性,将无法为军队工作。 我们知道现代软件开发确实依赖于第三方库ÿ…...

vue 文字跑马灯
<template><div class"marquee-container"><div class"marquee-content"><div>{{ marqueeText }}</div><div>{{ marqueeText }}</div> <!-- 复制一份文本,用于无缝衔接 --></div></d…...
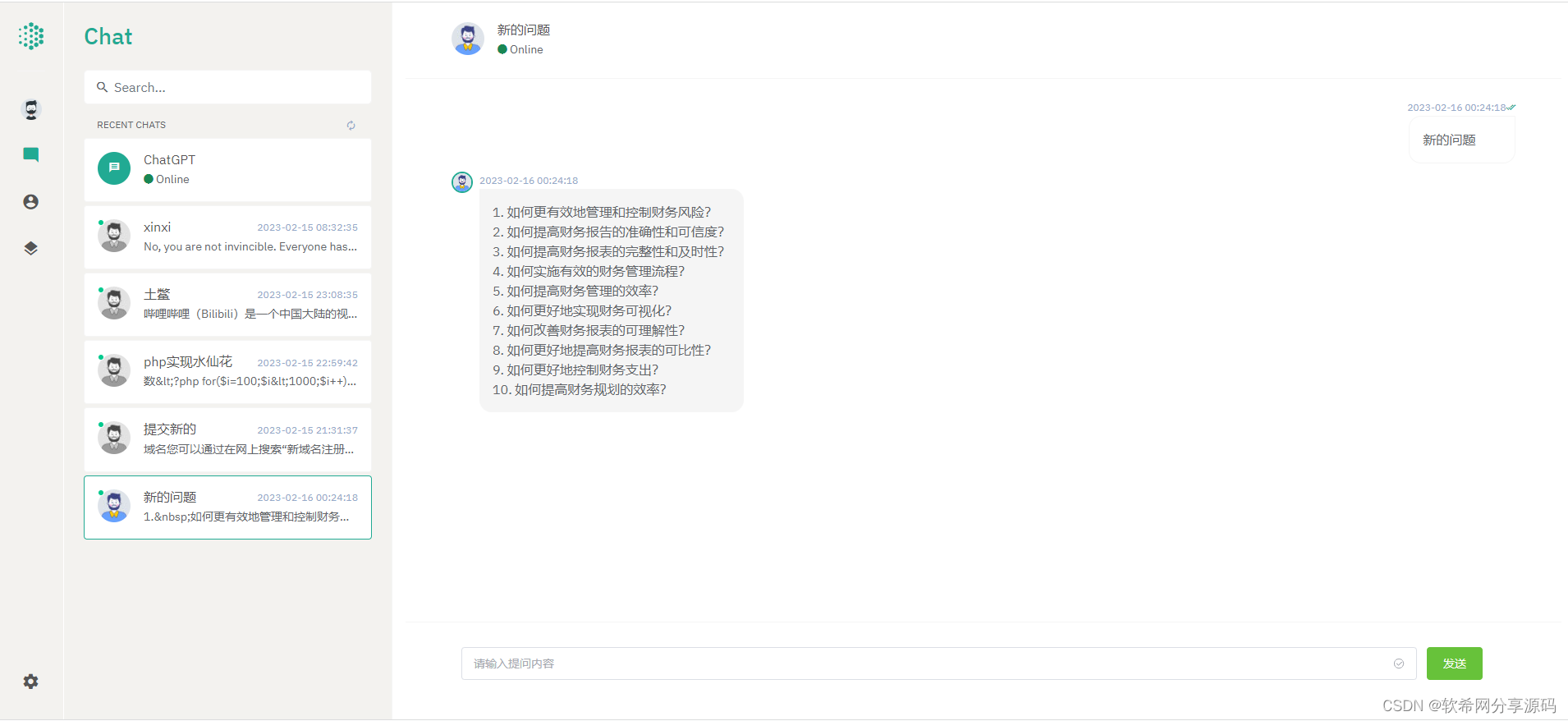
开源ChatGPT系统源码 采用NUXT3+Laravel9后端开发 前后端分离版本
开源ChatGPT系统源码 采用NUXT3Laravel9后端开发 前后端分离版本 ChatGPT是一种基于AI的聊天机器人技术,它可以帮助用户与聊天机器人进行自然语言交流,以解决用户的问题或满足用户的需求。ChatGPT的核心技术是使用自然语言处理(NLPÿ…...

【LeetCode|数据结构】剑指 Offer 33. 二叉搜索树的后序遍历序列
题目链接 剑指 Offer 33. 二叉搜索树的后序遍历序列 标签 二叉搜索树、后序遍历 步骤 二叉搜索树的左子树的节点值 ≤ \le ≤根节点值 ≤ \le ≤右子树的节点值;对于后序遍历序列最后一个元素的值为根节点的值; 由上面的两个性质可以得出ÿ…...

自定义协程
难点 自己写了一遍协程,困难的地方在于unity中的执行顺序突然发现unity里面可以 yield return 的其实有很多 WaitForSeconds WaitForSecondsRealtime WaitForEndOfFrame WaitForFixedUpdate WaitUntil WaitWhile IEnumerator(可以用于协程嵌套…...

【Atcoder】 [ABC240Ex] Sequence of Substrings
题目链接 Atcoder方向 Luogu方向 题目解法 先考虑一个性质,选出的子串长度不会超过 2 n \sqrt {2n} 2n 考虑最劣的选法是选出长度为 1 , 2 , 3 , . . . 1,2,3,... 1,2,3,... 的子串(如果后一个选出的串比前一个子串长度大超过1,那么后…...
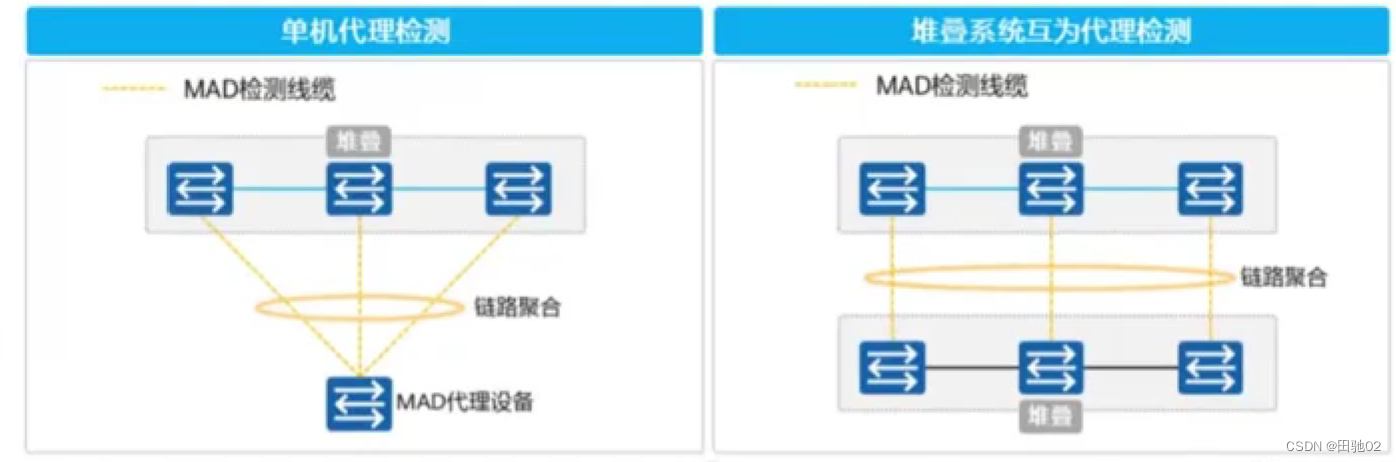
真机二阶段之堆叠技术
堆叠技术 --- 可以将多台真实的物理设备逻辑上抽象成一台 思科 -- VPC 华为 -- iStack和CSS 华三 -- IRF 锐捷 -- VSU iStack和CSS的区别: CSS --- 集群 --- 它仅支持将两台支持集群的交换机逻辑上整合成一台设备。 iStack --- 堆叠 --- 可以将多台支持堆叠的交换…...
简单、快速、无需注册的在线 MockJs 工具
简单、快速、无需注册的 MockJs 工具。通过参数来返回数据,传入什么参数就返回什么数据。 使用 接口只支持返回文本类数据,不支持图片、流数据等。 json 调用接口 https://mock.starxg.com/?responseBody{“say”:“hello”}&contentTypeapplic…...
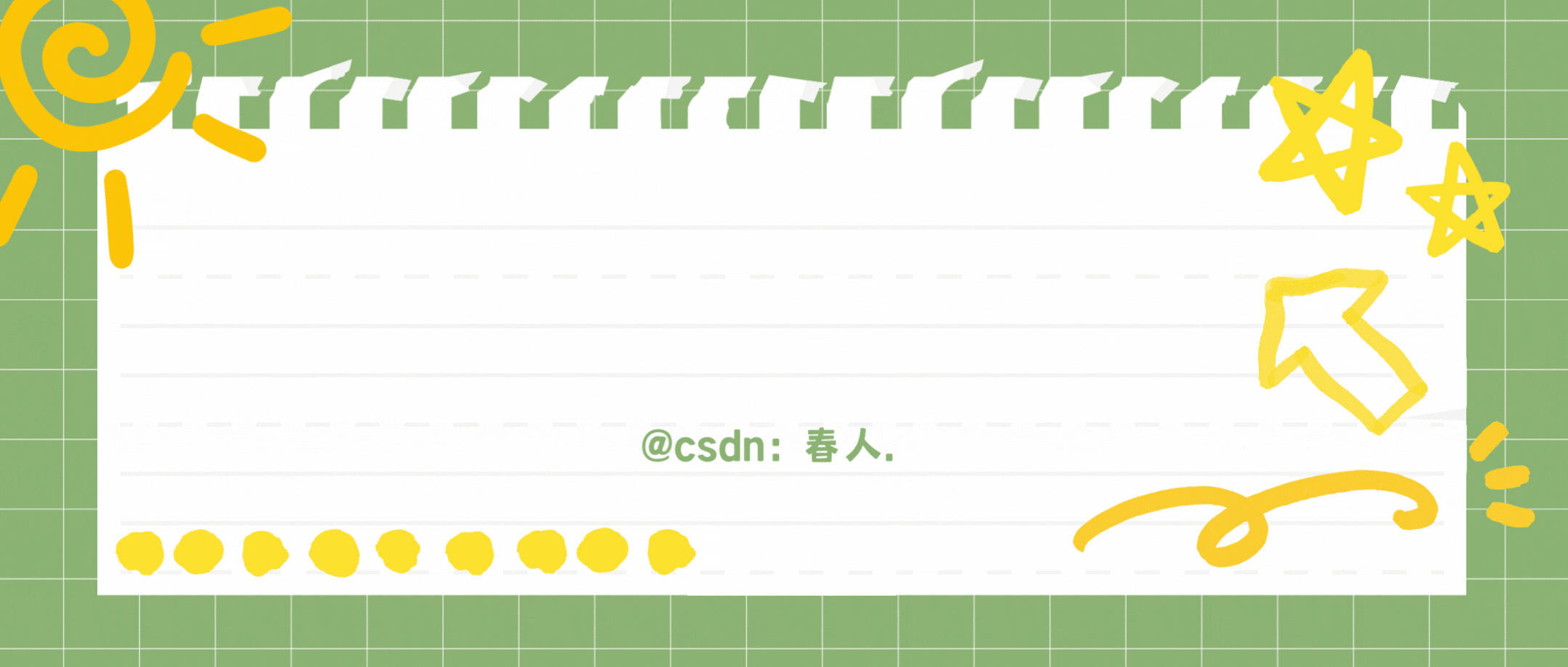
【Linux取经路】探索进程状态之僵尸进程 | 孤儿进程
文章目录 一、进程状态概述1.1 运行状态详解1.2 阻塞状态详解1.3 挂起状态详解 二、具体的Linux操作系统中的进程状态2.1 Linux内核源代码2.2 查看进程状态2.3 D磁盘休眠状态(Disk sleep)2.4 T停止状态(stopped) 三、僵尸进程3.1 僵尸进程危害总结 四、孤儿进程五、结语 一、进…...

第十二章MyBatis动态SQL
if标签与where标签 if标签 test如果为true就会拼接查询条件,否则不会 当没有使用Param,test出现arg0/param1当使用Param,test为Param指定的值当使用Pojo,test为对象的属性名 select * from car where <if test"name!n…...

redis--发布订阅
redis的发布和订阅 在Redis中,发布-订阅(Publish-Subscribe,简称Pub/Sub)是一种消息传递模式,用于在不同的客户端之间传递消息,允许一个消息发布者将消息发送给多个订阅者。这种模式适用于解耦消息发送者和…...

链表2-两两交换链表中的节点删除链表的倒数第N个节点链表相交环形链表II
今天记录的题目: ● 24. 两两交换链表中的节点 ● 19.删除链表的倒数第N个节点 ● 面试题 02.07. 链表相交 ● 142.环形链表II 两两交换链表中的节点 题目链接:24. 两两交换链表中的节点 这题比较简单,记录好两个节点,交换其nex…...
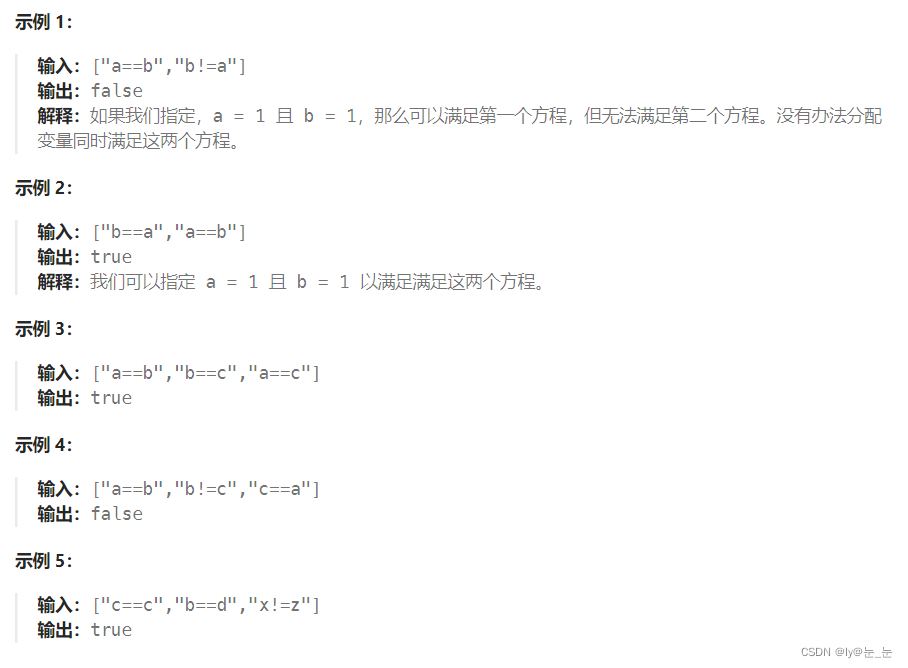
数据结构之并查集
并查集 1. 并查集原理2. 并查集实现3. 并查集应用3.1 省份数量3.2 等式方程的可满足性 4. 并查集的优缺点及时间复杂度 1. 并查集原理 并查表原理是一种树型的数据结构,用于处理一些不相交集合的合并及查询问题。并查集的思想是用一个数组表示了整片森林࿰…...

[element-ui] el-date-picker a-range-picker type=“daterange“ rules 校验
项目场景: 在项目中表单提交有时间区间校验 问题描述 想当然的就和其他单个输入框字符串校验,导致提交保存的时候 ,初次日期未选择,规则提示。后续在同一表单上继续提交时,校验失效。走进了死胡同,一直以…...
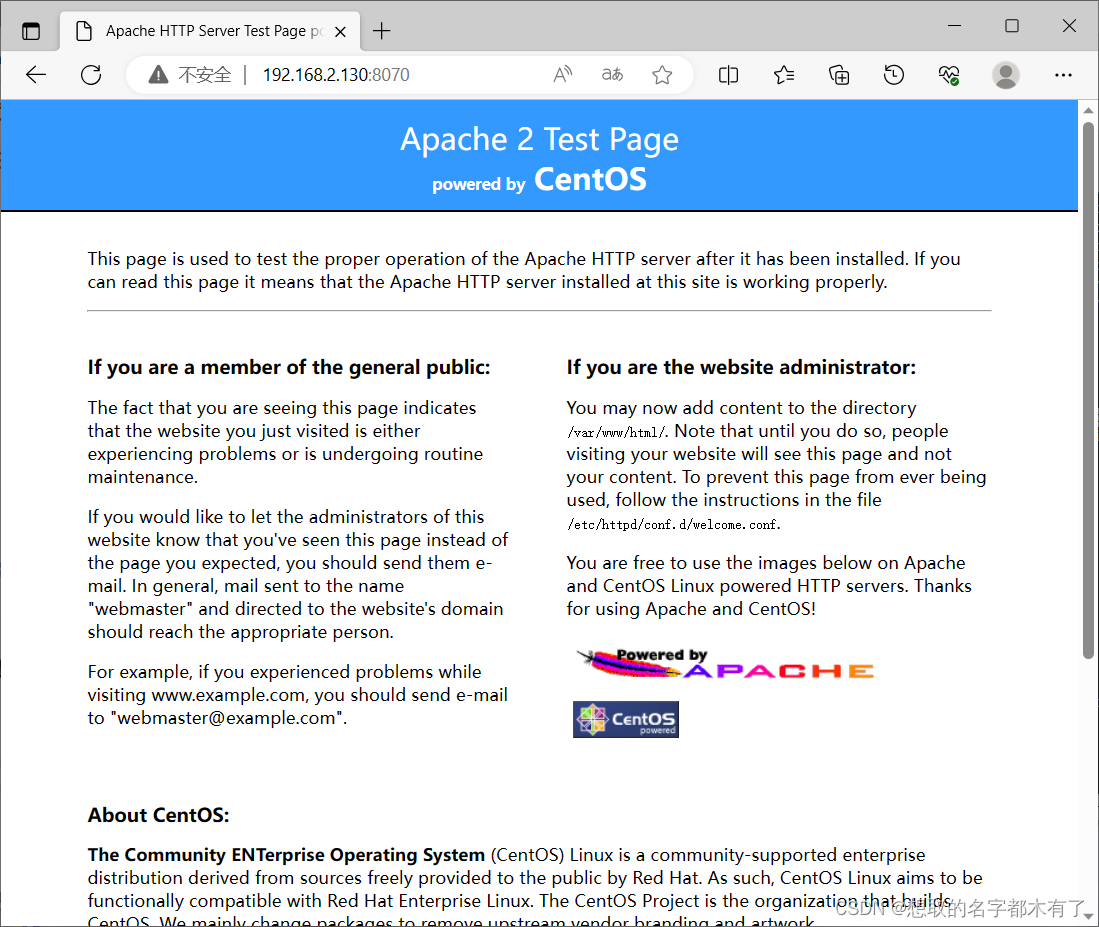
Dockers搭建个人网盘、私有仓库,Dockerfile制作Nginx、Lamp镜像
目录 1、使用mysql:5.6和 owncloud 镜像,构建一个个人网盘。 (1)下载mysql:5.6和owncloud镜像 (2)创建启动mysql:5.6和owncloud容器 (3)在浏览器中输入网盘服务器的IP地址,进行账…...

2023 CCPC 华为云计算挑战赛 hdu7401 流量监控(树形dp)
题目 流量监控 - HDU 7401 - Virtual Judge 简单来说,T(T<20)组样例,sumn不超过2e4 每次给定一棵n(n<2000)个点的树,两问: ①将n个点恰拆成n/2个pair(u,v),要求一个点是另一个点的祖先,求方案数 …...
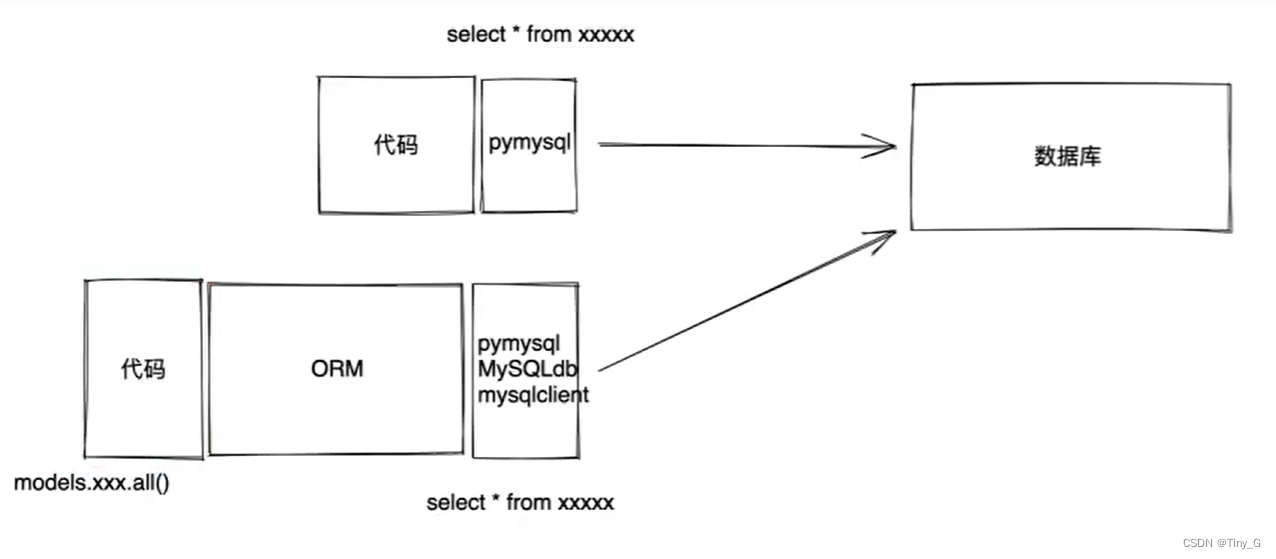
01.Django入门
1.创建项目 1.1基于终端创建Django项目 打开终端进入文件路径(打算将项目放在哪个目录,就进入哪个目录) E:\learning\python\Django 执行命令创建项目 F:\Anaconda3\envs\pythonWeb\Scripts\django-admin.exe(Django-admin.exe所…...
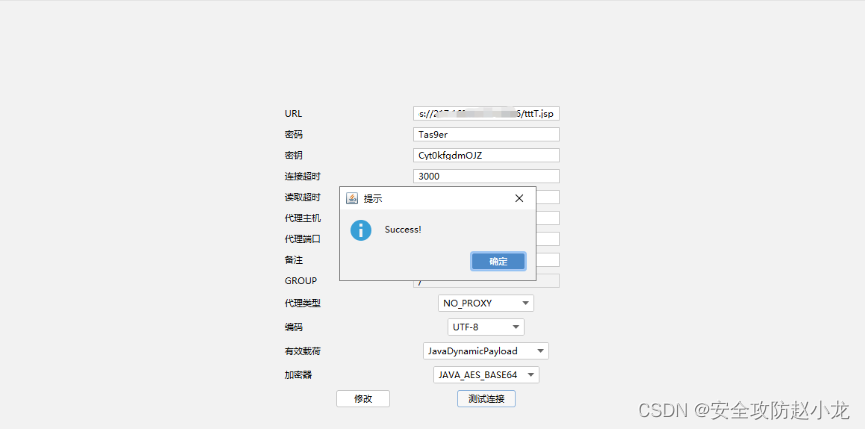
亿赛通电子文档安全管理系统任意文件上传漏洞(2023-HW)
亿赛通电子文档安全管理系统任意文件上传漏洞 一、 产品简介二、 漏洞概述三、 影响范围四、 复现环境五、 漏洞复现小龙POC检测 免责声明:请勿利用文章内的相关技术从事非法测试,由于传播、利用此文所提供的信息或者工具而造成的任何直接或者间接的后果…...
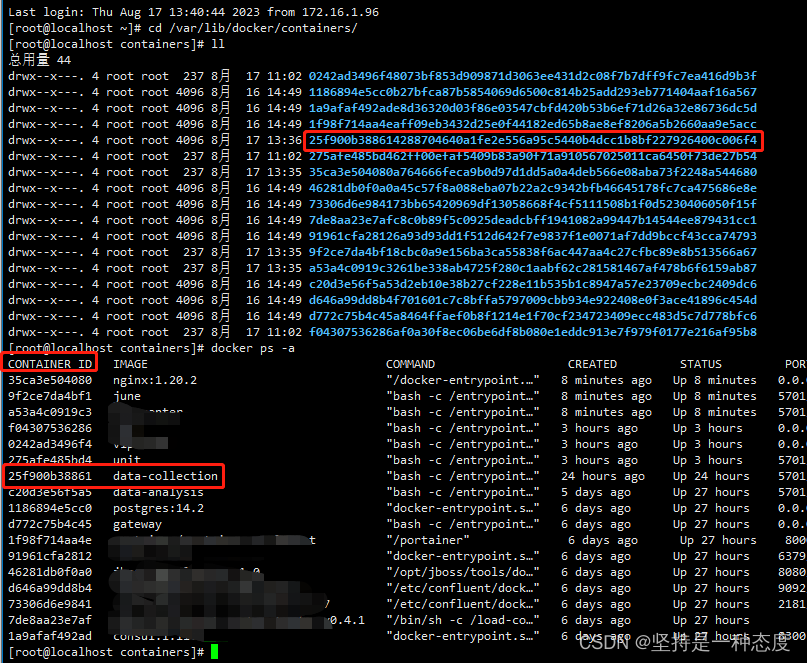
docker限制容器日志大小
文章目录 业务场景问题排查彻底解决 业务场景 我们公司做交通相关业务,我们部门主要负责信控服务,卖信号机的硬件产品和配套的信控平台 由于有部分小项目,可能只有几十个路口,客户预算有限,只给我们老旧的Windows ser…...

底层驱动实现数码管显示温湿度数值功能
开发板:STM32MP157A 温湿度传感器:si7006 显示器(数码管):m74hc595 遇到的问题:循环采集温湿度传感器数值,并将数值发送给数码管的时候两者存在竞态关系,导致数码管显示亮度很暗 …...

03架构管理之测试管理
专栏说明:针对于企业的架构管理岗位,分享架构管理岗位的职责,工作内容,指导架构师如何完成架构管理工作,完成架构师到架构管理者的转变。计划以10篇博客阐述清楚架构管理工作,专栏名称:架构管理…...

30、devtools 依赖关于自动重启(自动加载页面)的知识
devtools 依赖关于自动重启的知识 ★ 自动重启 devtools会监控类加载路径中的文件(尤其是*.class文件),只要这些文件发生了改变, devtools就会自动重启Spring Boot应用。▲ 不同工具触发自动重启的方式:Eclipse&…...

ES6 Promise/Async/Await使用
Promise应用 在工作中, 我们经常会遇到用异步请求数据, 查询一个结果, 然后把返回的参数放入到下一个执行的异步函数像这样: $.ajax({..., success(resp)>{$.ajax({..., resp.id, success(resp)>{$.ajax({..., resp.name success(resp)>{//多层嵌套的情况, 看着是不…...
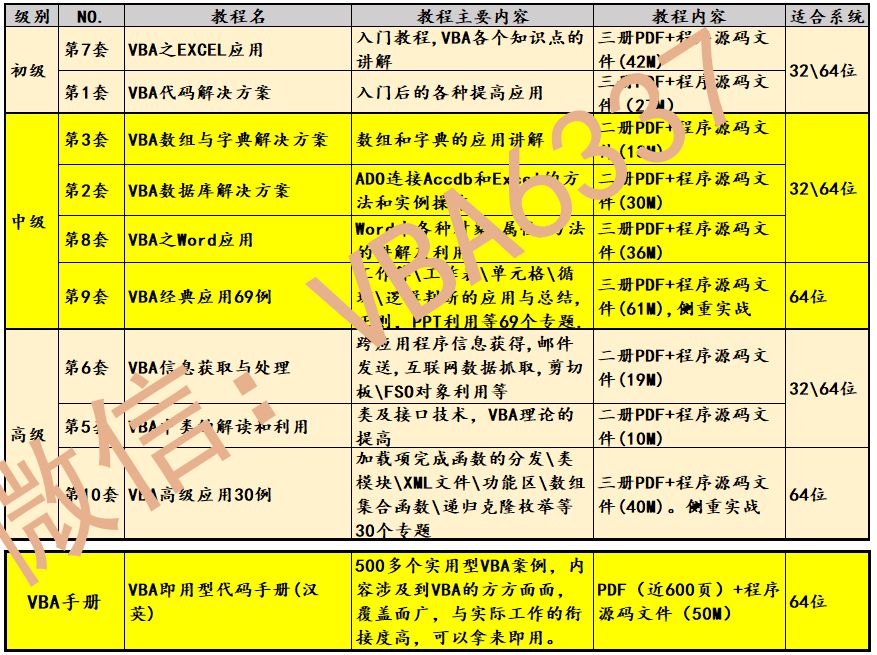
Word中对象方法(Methods)的理解及示例(上)
【分享成果,随喜正能量】奋斗没有终点,任何时候都是一个起点,沉潜是为了蓄势待发,沉潜是为了等待因缘。鲸豚沉潜于大海,幽兰深藏于山谷,能够经得起沉潜的人,才会有更高的成就。正如一年的树木只能当柴烧&am…...
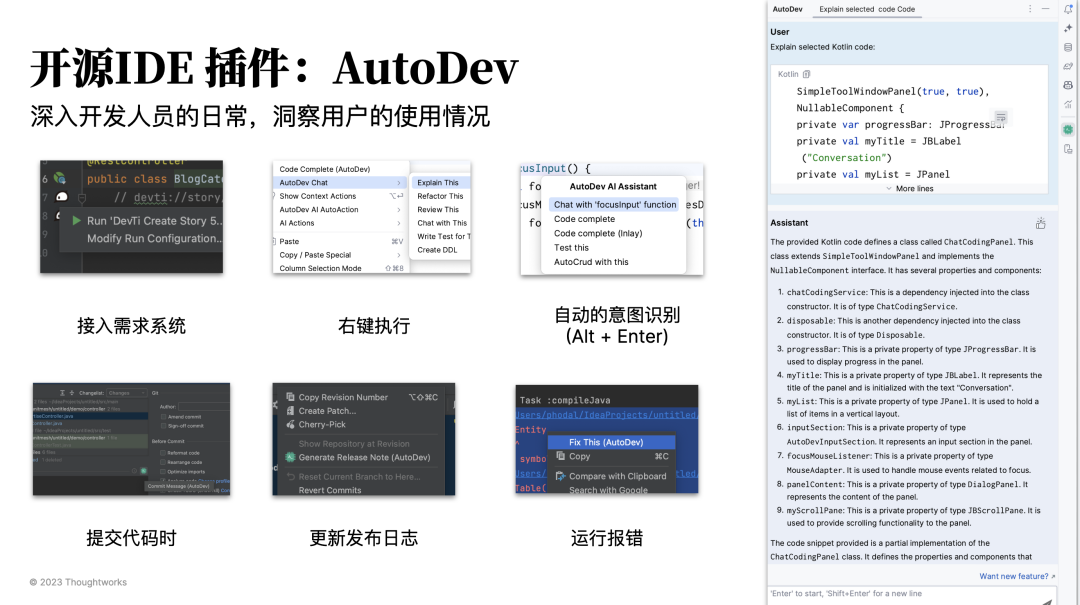
AutoDev 1.1.3 登场,个性化 AI 辅助:私有化大模型、自主设计 prompt、定义独特规则...
在过去的半个月里,我们为开源辅助编程工具 AutoDev 添加了更强大的自定义能力,现在你可以: 使用自己部署的开源大模型自己配置 Intellij IDEA 中的行为自定义开发过程中的规范 当然了,如果您自身拥有开发能力的话,建议…...

win11 python 调用edge调试过程
1、下载对应版本的驱动程序: https://developer.microsoft.com/zh-cn/microsoft-edge/tools/webdriver/ 2、和系统版本对应的exe文件(x86、x64要对应)放置的固定的目录,我放到了system32下了; 3、PATH路径添加windows/system32目录&#x…...

DS-排序回顾
快速排序相比于堆排序的优点有: 效率更高:快速排序的平均时间复杂度为 O(nlogn),而堆排序的时间复杂度为 O(nlogn)。虽然它们的时间复杂度相同,但是在实际情况下,快速排序往往比堆排序更快,因为快速排序具有…...