【NLP的python库(01/4) 】: NLTK
一、说明
NLTK是一个复杂的库。自 2009 年以来不断发展,它支持所有经典的 NLP 任务,从标记化、词干提取、词性标记,包括语义索引和依赖关系解析。它还具有一组丰富的附加功能,例如内置语料库,NLP任务的不同模型以及与SciKit Learn和其他Python库的集成。
本文是对NLTK的简要介绍。您将看到NLTK的实际操作,可用于各种NLP任务的简短代码片段。
这篇文章最初出现在我的博客 admantium.com。
本文的技术上下文是 和 。所有示例也应该适用于较新的版本。Python v3.11
NLTK v3.8.1
二、NLTK 库安装
NLTK可以通过Python pip安装:
python3 -m pip install nltk
一些NLTK功能需要使用其他数据,例如停用词或集成语料库。为此,使用内置下载器。下面是一个示例:
import nltknltk.download('stopwords')
nltk.download('punkt')
nltk.download('averaged_perceptron_tagger')
nltk.download('reuters')
其他部分,如专用分词器或停用词,需要安装 Java 库。请参阅此 Github Gist 以开始使用。
三、自然语言处理任务
NLTK支持多个NLP任务。下面是一个简短的概述,接下来的部分将提供更多详细信息:
文本处理
- 标记化
- 堵塞
- 词形还原
文本语法
- 词性标记
文本语义
- 命名实体识别
文档语义
- 聚类
- 分类
此外,NLTK 还支持以下附加功能:
- 数据
- 语料库管理
- 机器学习聚类和分类模型
四、文本处理
4.1 标记化
标记化是文本处理中必不可少的第一步。通常,应根据项目要求和后续NLP任务来选择标记化方法。例如,当文本包含表示实体或人的多名词,但分词器只是按空格拆分时,命名实体识别变得困难。
NLTK提供了一个简单的空格标记器,几个内置的标记器,如NIST或Stanford,以及基于正则表达式的自定义标记器选项。
下面是内置句子和单词分词器的示例:
from nltk.tokenize import sent_tokenize, word_tokenize# Source: Wikipedia, Artificial Intelligence, https://en.wikipedia.org/wiki/Artificial_intelligence
paragraph = '''Artificial intelligence was founded as an academic discipline in 1956, and in the years since it has experienced several waves of optimism, followed by disappointment and the loss of funding (known as an "AI winter"), followed by new approaches, success, and renewed funding. AI research has tried and discarded many different approaches, including simulating the brain, modeling human problem solving, formal logic, large databases of knowledge, and imitating animal behavior. In the first decades of the 21st century, highly mathematical and statistical machine learning has dominated the field, and this technique has proved highly successful, helping to solve many challenging problems throughout industry and academia.'''
sentences = []for sent in sent_tokenize(paragraph):sentences.append(word_tokenize(sent))sentences[0]
# ['Artificial', 'intelligence', 'was', 'founded', 'as', 'an', 'academic', 'discipline'
4.2 词干提取和词形还原
与标记化一样,选择合适的词干提取(将屈折词替换为词干,如用厨师烹饪)和词形还原(用词干替换词组)方法取决于后续的 NLP 任务。词形还原具有特殊作用,因为它需要一些词性标记或词义消歧来正确识别单词组。
NLTK提供了几个词干模块,如Porter,Lancaster和Isri。对于词形还原,仅提供 Wordnet。
让我们比较一下维基百科文章中关于人工智能的第一句话的词干和词形还原。
from nltk.stem import LancasterStemmersent = 'Artificial intelligence was founded as an academic discipline in 1956, and in the years since it has experienced several waves of optimism, followed by disappointment and the loss of funding (known as an "AI winter"), followed by new approaches, success, and renewed funding.'
stemmer = LancasterStemmer()
stemmed_sent = [stemmer.stem(word) for word in word_tokenize(sent)]print(stemmed_sent)
# ['art', 'intellig', 'was', 'found', 'as', 'an', 'academ', 'disciplin',
用WordNet词形还原器处理的同一个句子:
from nltk.stem import WordNetLemmatizersent = 'Artificial intelligence was founded as an academic discipline in 1956, and in the years since it has experienced several waves of optimism, followed by disappointment and the loss of funding (known as an "AI winter"), followed by new approaches, success, and renewed funding.'
lemmatizer = WordNetLemmatizer()
lemmas = [lemmatizer.lemmatize(word) for word in word_tokenize(sent)]print(lemmas)
# ['Artificial', 'intelligence', 'wa', 'founded', 'a', 'an', 'academic', 'discipline'
五、文本语法
词性标记
NLTK还提供不同的词性标记器(pos)。使用内置标记器,将生成以下注释:
标签含义 ADJ 形容词 ADP 附加置词 ADV 副词 CONJ 连词 DET 决定符,文章名词名词 NUM 数字 PRT 助词 PRON 代词 动词 动词 .标点符号 X 其他
取维基百科关于人工智能的文章的第一句话,词性标记产生以下结果。
from nltk import pos_tagsent = 'Artificial intelligence was founded as an academic discipline in 1956, and in the years since it has experienced several waves of optimism, followed by disappointment and the loss of funding (known as an "AI winter"), followed by new approaches, success, and renewed funding.'
pos_tag(sentences[0])
# [('Artificial', 'JJ'),
# ('intelligence', 'NN'),
# ('was', 'VBD'),
# ('founded', 'VBN'),
# ('as', 'IN'),
# ('an', 'DT'),
# ('academic', 'JJ'),
# ('discipline', 'NN'),
要使用其他NLTK pos标记器,例如Stanford或Brill,需要下载外部Java库。
六、文本语义
命名实体识别
NLTK包括预先训练的NER标记器,但需要先下载几个额外的包。
import nltk nltk.download('maxent_ne_chunker')nltk.download('punkt')nltk.download('averaged_perceptron_tagger')nltk.download
('words')
NER 标记器使用 POS 标记的句子,并将分类标签添加到表示形式中。在示例段落上使用它不会产生任何结果,因此以下示例从维基百科文章中引用了另一个句子,其中提到了人物。
from nltk.tokenize import sent_tokenize# Source: Wikipedia, Artificial Intelligence, https://en.wikipedia.org/wiki/Artificial_intelligence
sentence= '''
In 2011, in a Jeopardy! quiz show exhibition match, IBM's question answering system, Watson, defeated the two greatest Jeopardy! champions, Brad Rutter and Ken Jennings, by a significant margin.
'''tagged_sentence = nltk.pos_tag(word_tokenize(sentence))
tagged_sentence
# [('In', 'IN'),
# ('2011', 'CD'),
# (',', ','),
# ('in', 'IN'),
# ('a', 'DT'),
# ('Jeopardy', 'NN'),print(nltk.ne_chunk(tagged_sentence))
# (S
# In/IN
# 2011/CD
# ,/,
# in/IN
# a/DT
# Jeopardy/NN
# !/.
# quiz/NN
# show/NN
# exhibition/NN
# match/NN
# ,/,
# (ORGANIZATION IBM/NNP)
# 's/POS
# question/NN
# answering/NN
# system/NN
# ,/,
# (PERSON Watson/NNP)
如您所见,个人和组织得到了认可。Watson
IBM
七、文档语义
7.1 聚类
支持三种聚类分析算法,请参阅完整文档。
- K 均值
- 电磁集群
- 组平均集聚聚类器 (GAAC)
7.2 分类
以下分类器在 NLTK 中实现,另请参阅完整文档。
- 决策树
- 最大熵建模
- 兆米最大优化
- 朴素贝叶斯(及其变体)
支持外部包,如用于语言识别的TextCat,Java库Weka或SciKitLearn分类器。
八、附加功能
8.1 数据
NLTK提供了100多个内置语料库,请参阅完整列表。一些例子:路透社新闻文章,Treebank 2华尔街日报校园,Twitter新闻或WordNet词汇数据库。
以下是如何访问路透社语料库的示例。
from nltk.corpus import reutersprint(reuters.categories()[:10])
#['acq', 'alum', 'barley', 'bop', 'carcass', 'castor-oil', 'cocoa', 'coconut', 'coconut-oil', 'coffee']print(reuters.fileids()[:10])
# ['test/14826', 'test/14828', 'test/14829', 'test/14832', 'test/14833', 'test/14839', 'test/14840', 'test/14841', 'test/14842', 'test/14843']sample = 'test/14829'
categories = reuters.categories(sample)print(categories)
# ['acq', 'alum', 'barley', 'bop', 'carcass', 'castor-oil', 'cocoa', 'coconut', 'coconut-oil', 'coffee']content = ""
with reuters.open(sample) as stream:content = stream.read()print(f"Categories #{categories} / file #{sample}")
# Categories #['crude', 'nat-gas'] / file #test/14829print(f"Content:\#{content}")
# Content:\#JAPAN TO REVISE LONG-TERM ENERGY DEMAND DOWNWARDS
# The Ministry of International Trade and
# Industry (MITI) will revise its long-term energy supply/demand
# outlook by August to meet a forecast downtrend in Japanese
# energy demand, ministry officials said.
# MITI is expected to lower the projection for primary energy
# supplies in the year 2000 to 550 mln kilolitres (kl) from 600
# mln, they said.
# The decision follows the emergence of structural changes in
# Japanese industry following the rise in the value of the yen
# and a decline in domestic electric power demand.
# MITI is planning to work out a revised energy supply/demand
# outlook through deliberations of committee meetings of the
# Agency of Natural Resources and Energy, the officials said.
# They said MITI will also review the breakdown of energy
# supply sources, including oil, nuclear, coal and natural gas.
# Nuclear energy provided the bulk of Japan's electric power
# in the fiscal year ended March 31, supplying an estimated 27
# pct on a kilowatt/hour basis, followed by oil (23 pct) and
# liquefied natural gas (21 pct), they noted.
8.2 语料库管理
语料库阅读器
NLTK 文档集读取器对象提供读取、筛选、解码和预处理结构化文件列表或 zip 文件。
存在许多不同的语料库读取器对象,请参阅完整列表。最常见的读者是:
- PlaintextCorpusReader:阅读段落拆分为空白行的文本文档。
- Markdown:处理 Markdown 文件,其中其类别在文件名中表示
- 已标记:需要已标记语料库的特殊语料库读取器对象,例如 Conl。请注意,对于多个内置语料库对象,标记的版本已经存在。
- 推特:处理 JSON 格式的推文
- XML:处理 XML 文件
作为一个简短的例子,下面是一个将读取文件。PlaintextCorpusReader
*.txt
from nltk.corpus.reader.plaintext import PlaintextCorpusReadercorpus = PlaintextCorpusReader('wikipedia_articles', r'.*\.txt')print(corpus.fileids())
# ['AI_alignment.txt', 'AI_safety.txt', 'Artificial_intelligence.txt', 'Machine_learning.txt']print(corpus.sents())
# [['In', 'the', 'field', 'of', 'artificial', 'intelligence', '(', 'AI', '),', 'AI', 'alignment', 'research', 'aims', 'to', 'steer', 'AI', 'systems', 'towards', 'humans', ''', 'intended', 'goals', ',', 'preferences', ',', 'or', 'ethical', 'principles', '.'], ['An', 'AI', 'system', 'is', 'considered', 'aligned', 'if', 'it', 'advances', 'the', 'intended', 'objectives', '.'], ...]
8.3 文本集合
另一个从语料库访问结构化信息的实用工具是 TextCollection 类。在标记化文本上实例化,它提供以下功能:
collocations(num, window_size)
:返回到长度的元组,单词并置出现num
window_size
collocation_list(num, window_size)
:将并置单词输出为元组列表common_contexts(word, num)
:打印显示的上下文word
concordance(word, width, lines)
:打印给定的索引(单个单词或句子)word
concordance_list(word, width, lines)
:打印元组列表count(word)
:单词的绝对外观tf
, , : 单词的频率idf
tf_idf
generate
:基于三元组语言模型创建随机文本。vocab
:所有代币的频率分布plot
:绘制频率分布
下面是一个示例:
from nltk.corpus.reader.plaintext import PlaintextCorpusReader
from nltk.text import TextCollectioncorpus = PlaintextCorpusReader('wikipedia_articles', r'.*\.txt')
col = TextCollection(corpus.sents())
print(col.count('the'))
# 973
print(col.common_contexts(['intelligence']))
# artificial_( general_( artificial_. artificial_is general_,
# artificial_, artificial_in artificial_". artificial_and "_"
# artificial_was general_and general_. artificial_; artificial_" of_or
# artificial_– artificial_to artificial_: and_.
九、机器学习聚类和分类模型
NLTK 提供了几种聚类和分类算法。但在使用任何算法之前,需要手动设计和从文本中提取特征。
在有关分类的 API 文档页面上,步骤定义如下:
- 定义与 ML 任务相关的功能
- 实现从语料库中提取特征的方法(例如,文档中的词频)
- 创建一个包含单个元组的 Python 字典对象,并将它们传递给训练算法
(feature_name, labels)
让我们用 NLTK 手册中的一个例子来说明这一点,以构建文本分类器。
首先,我们建立一个包含所有单词的词汇表:
from nltk.corpus.reader.plaintext import PlaintextCorpusReader
corpus = PlaintextCorpusReader('wikipedia_articles', r'.*\.txt')vocab = nltk.FreqDist(w.lower() for w in corpus.words())
# FreqDist({'the': 65590, ',': 63310, '.': 52247, 'of': 39000, 'and': 30868, 'a': 30130, 'to': 27881, 'in': 24501, '-': 19867, '(': 18243, ...})
all_words = nltk.FreqDist(w.lower() for w in corpus.words())
word_features = list(all_words)
# ['the', ':']
其次,我们定义一种方法,该方法返回一个热编码的单词向量,该向量表示文档中是否存在单词。生成的特征向量必须包含布尔值,才能用于分类任务。
def document_features(document):document_words = set(corpus.words(document))features = {}for word in word_features:features['contains({})'.format(word)] = (word in document_words)return featuresf = document_features('Artificial_intelligence.txt')
# {'contains(the)': True,
# 'contains(,)': True,
# 'contains(.)': True,
第三,我们选择一种分类算法,并将特征化的文档传递给它。
featuresets = [(document_features(d), d) for d in corpus.fileids()]
featuresets
# featuresets = [(document_features(d), d) for d in corpus.fileids()]train_set, test_set = featuresets[100:], featuresets[:100]
classifier = nltk.NaiveBayesClassifier.train(train_set)
# <nltk.classify.naivebayes.NaiveBayesClassifier at 0x185ec5dd0>
十、总结
NLTK 是一个支持多个 NLP 任务的多功能库。对于标记化、词干提取/词形还原和词性标记的核心任务,包括内置方法以及科学论文中的方法。为了管理文档语料库,NLTK处理文本,Markdown,XML和其他格式,并提供API来获取文件,类别,句子和单词。特别有用的是能够收集单词搭配和计算术语频率的类。塞巴斯蒂安 最后,NLTK还提供了聚类和分类算法,如KMeans。决策树或朴素贝叶斯。TextCollection
相关文章:
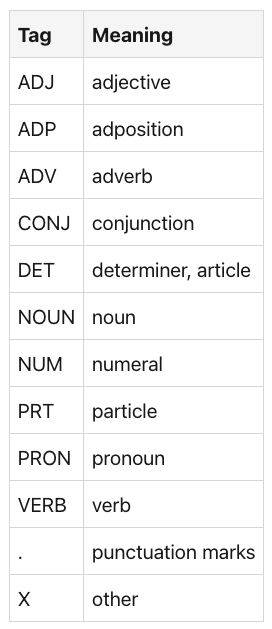
【NLP的python库(01/4) 】: NLTK
一、说明 NLTK是一个复杂的库。自 2009 年以来不断发展,它支持所有经典的 NLP 任务,从标记化、词干提取、词性标记,包括语义索引和依赖关系解析。它还具有一组丰富的附加功能,例如内置语料库,NLP任务的不同模型以及与S…...
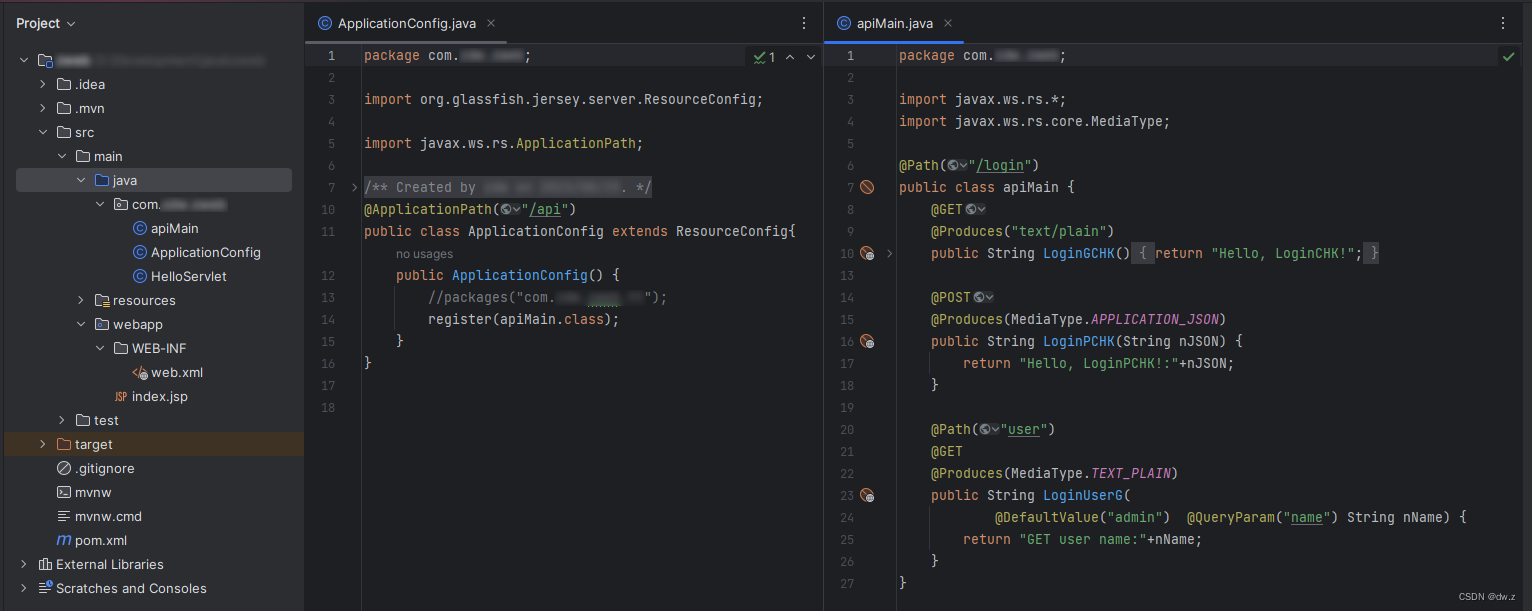
Java IDEA Web 项目 1、创建
环境: IEDA 版本:2023.2 JDK:1.8 Tomcat:apache-tomcat-9.0.58 maven:尚未研究 自行完成 IDEA、JDK、Tomcat等安装配置。 创建项目: IDEA -> New Project 选择 Jakarta EE Template:选择…...
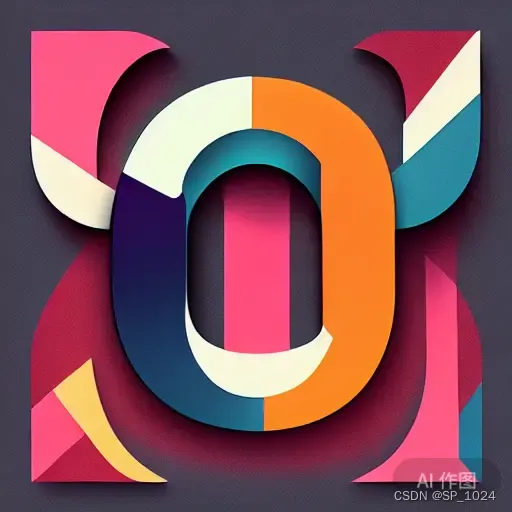
leetcode316. 去除重复字母(单调栈 - java)
去除重复字母 题目描述单调栈代码演示进阶优化 上期经典 题目描述 难度 - 中等 leetcode316. 去除重复字母 给你一个字符串 s ,请你去除字符串中重复的字母,使得每个字母只出现一次。需保证 返回结果的字典序最小(要求不能打乱其他字符的相对…...

零散笔记:《Spring实战》Thymeleaf
1、Thymeleaf模板就是增加一些额外元素属性的HTML,这些属性能够指导模板如何渲染request数据。 <p th:test "${message}">placeholder message</p> th我推测是中文的”替换“。 2、th:each,迭代元素集合。 <div th:each &qu…...
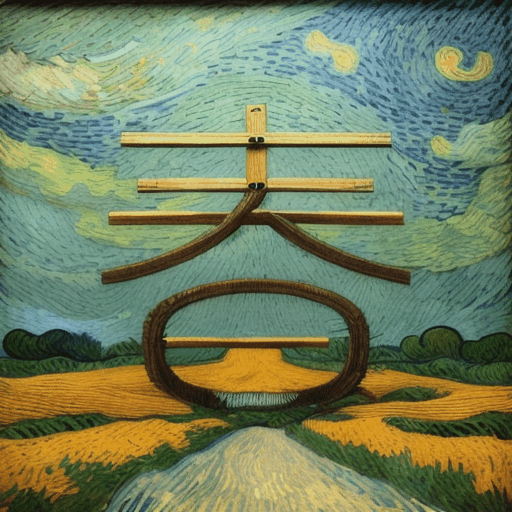
WordArt Designer:基于用户驱动与大语言模型的艺术字生成
AIGC推荐 FaceChain人物写真开源项目,支持风格与穿着自定义,登顶github趋势榜首! 前言 本文介绍了一个基于用户驱动,依赖于大型语言模型(LLMs)的艺术字生成框架,WordArt Designer。 该系统包含四个关键模块:LLM引擎、…...
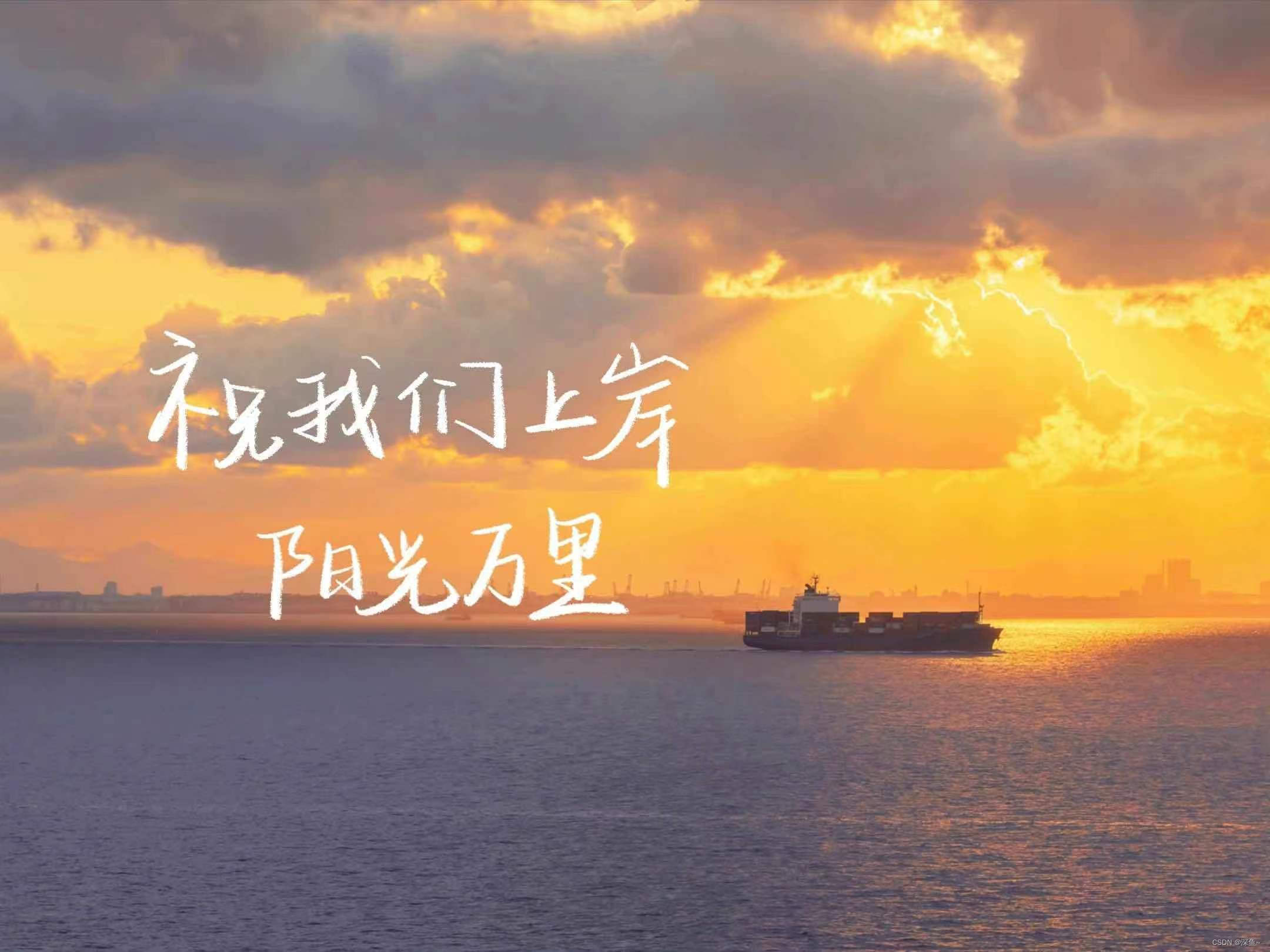
【C进阶】深度剖析数据在内存中的存储
目录 一、数据类型的介绍 1.类型的意义: 2.类型的基本分类 二、整形在内存中的存储 1.原码 反码 补码 2.大小端介绍 3.练习 三、浮点型在内存中的存储 1.一个例子 2.浮点数存储规则 一、数据类型的介绍 前面我们已经学习了基本的内置类型以及他们所占存储…...
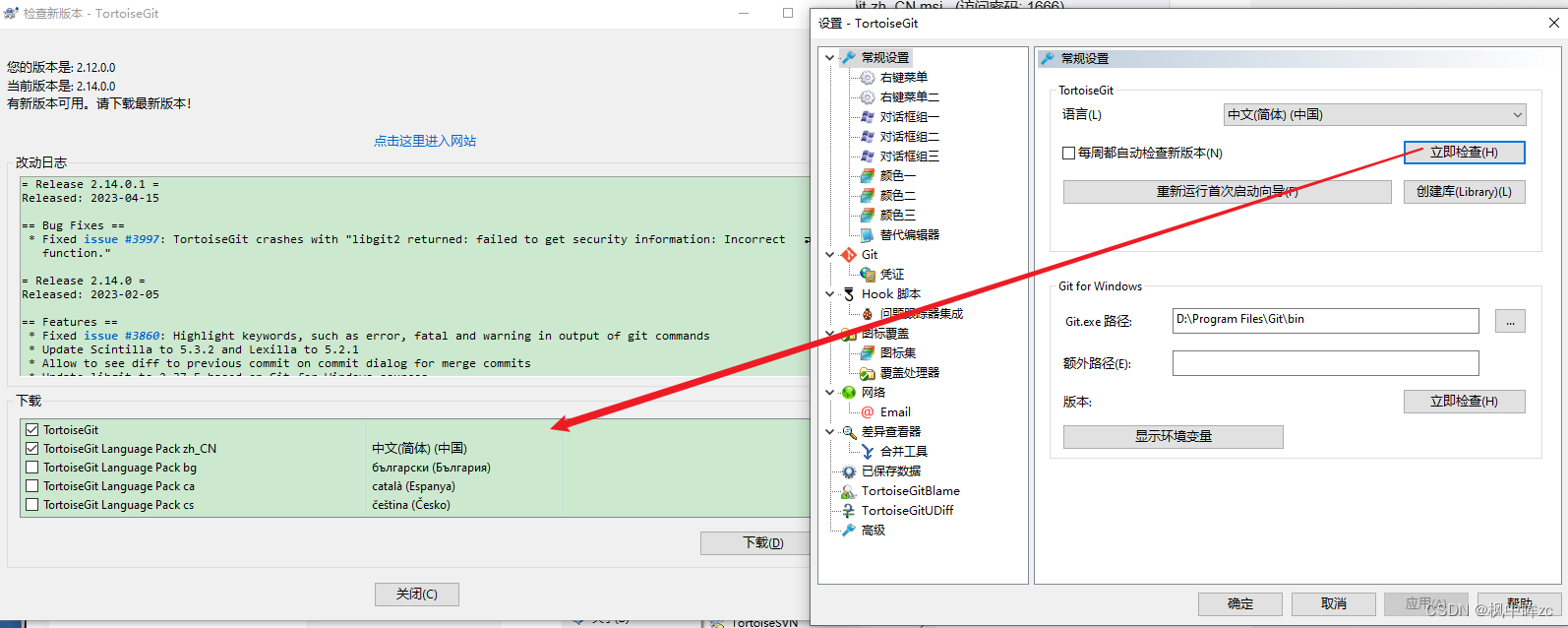
TortoiseGit安装
一、安装Git环境 Git-2.42.0-64-bit.exe (访问密码: 1666)https://url48.ctfile.com/f/33868548-924037167-76e273?p1666 二、安装TortoiseGit TortoiseGit-2.14.0.1-64bit.msi (访问密码: 1666)https://url48.ctfile.com/f/33868548-924037173-d395c7?p1666 三、安装T…...
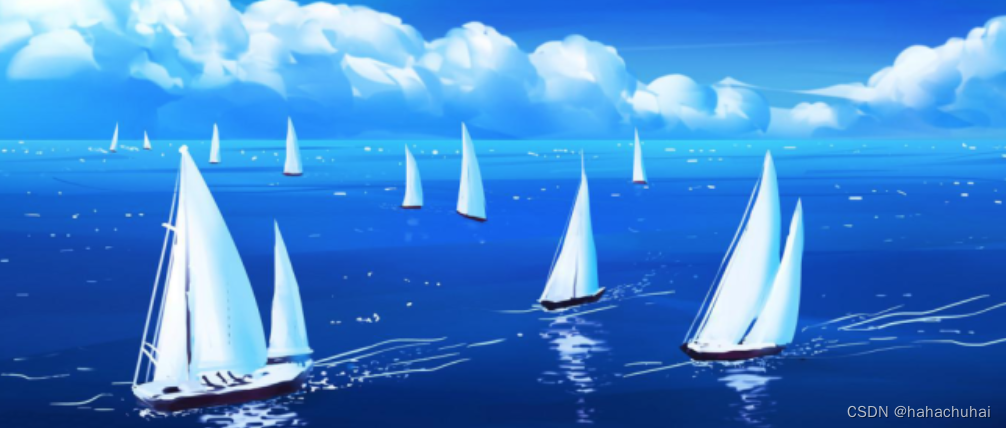
巨人互动|游戏出海游戏出海的趋势如何
随着全球游戏市场的不断扩大和消费者需求的多元化,游戏出海作为游戏行业的重要战略之一,正面临着新的发展趋势。本文小编将讲讲游戏出海的趋势,探讨一下未来游戏出海的发展方向与前景。 巨人互动|游戏出海&2023国内游戏厂商加快“出海”发…...
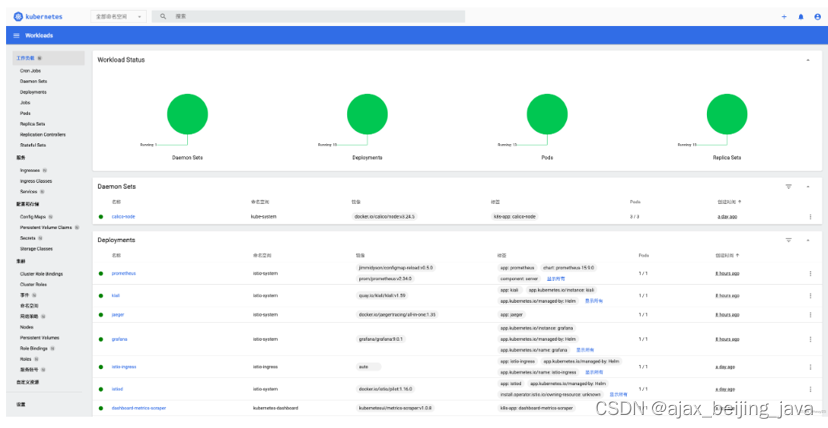
k8s 安装 istio(二)
3.3 部署服务网格调用链检测工具 Jaeger 部署 Jaeger 服务 kubectl apply -f https://raw.githubusercontent.com/istio/istio/release-1.16/samples/addons/jaeger.yaml 创建 jaeger-vs.yaml 文件 apiVersion: networking.istio.io/v1alpha3 kind: VirtualService metadata…...
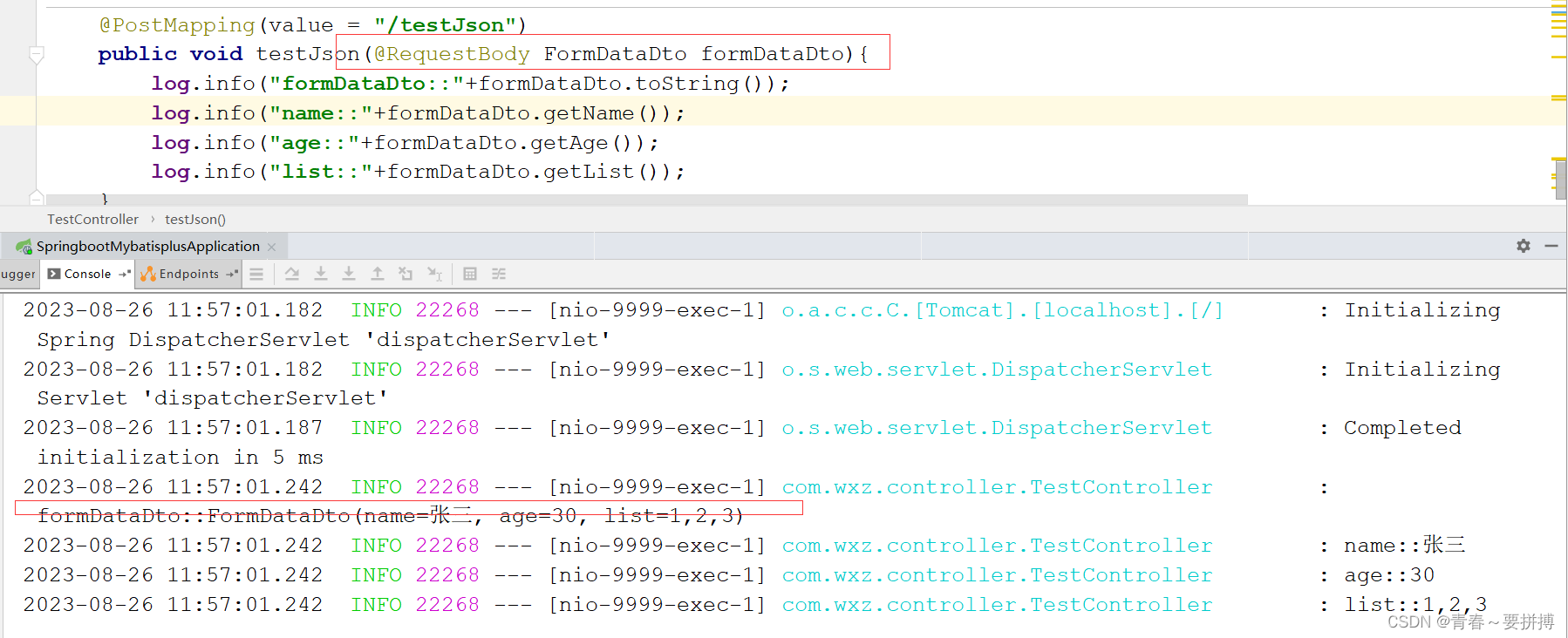
Postman中参数区别及使用说明
一、Params与Body 二者区别在于请求参数在http协议中位置不一样。Params 它会将参数放入url中以?区分以&拼接Body则是将请求参数放在请求体中 后端接受数据: 二、body中不同格式 2.1 multipart/form-data key - value 格式输入,主要特点是可以上…...
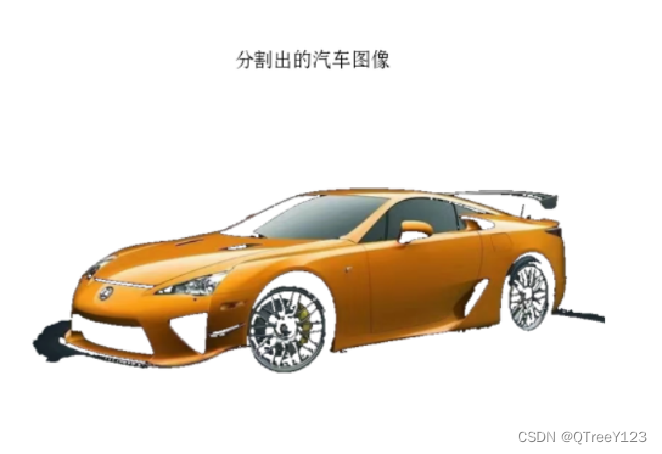
基于python+pyqt的opencv汽车分割系统
目录 一、实现和完整UI视频效果展示 主界面: 识别结果界面: 查看分割处理过程图片界面: 二、原理介绍: 加权灰度化 编辑 二值化 滤波降噪处理 锐化处理 边缘特征提取 图像分割 完整演示视频: 完整代码链…...

游戏设计的主要部分
游戏设计的主要部分 介绍 游戏设计是创建有趣、挑战性和令人满足的游戏体验的过程。它涵盖了许多方面,从概念开发到实际实施,以及最终的游戏测试和优化。游戏设计师需要考虑玩家的情感、技能挑战、故事情节、游戏世界等多个要素,以确保游戏…...

架构师成长之路Redis第二篇|Redis配置文件参数讲解
Redis.conf文件 官网Redis文档链接:Redis官网 官网Redis config配置文件参数讲解:https://redis.io/docs/management/config/ Redis.conf参考模板例子 : https://redis.io/docs/management/config-file/ Redis 可以使用内置的默认配置在没有配置文件的情况下启动,但是仅…...
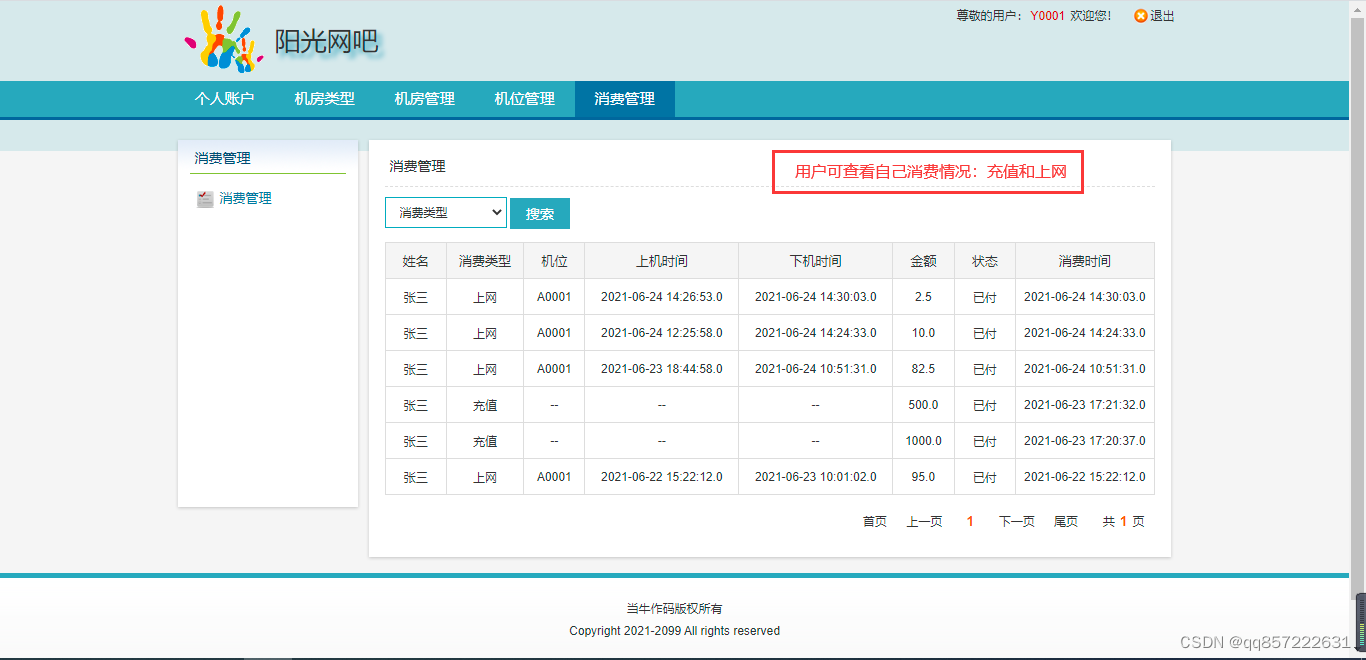
jsp+servlet+mysql阳光网吧管理系统
项目介绍: 本系统使用jspservletmysql开发的阳光网吧管理系统,纯手工敲打,系统管理员和用户角色,功能如下: 管理员:修改个人信息、修改密码;机房类型管理;机房管理;机位…...
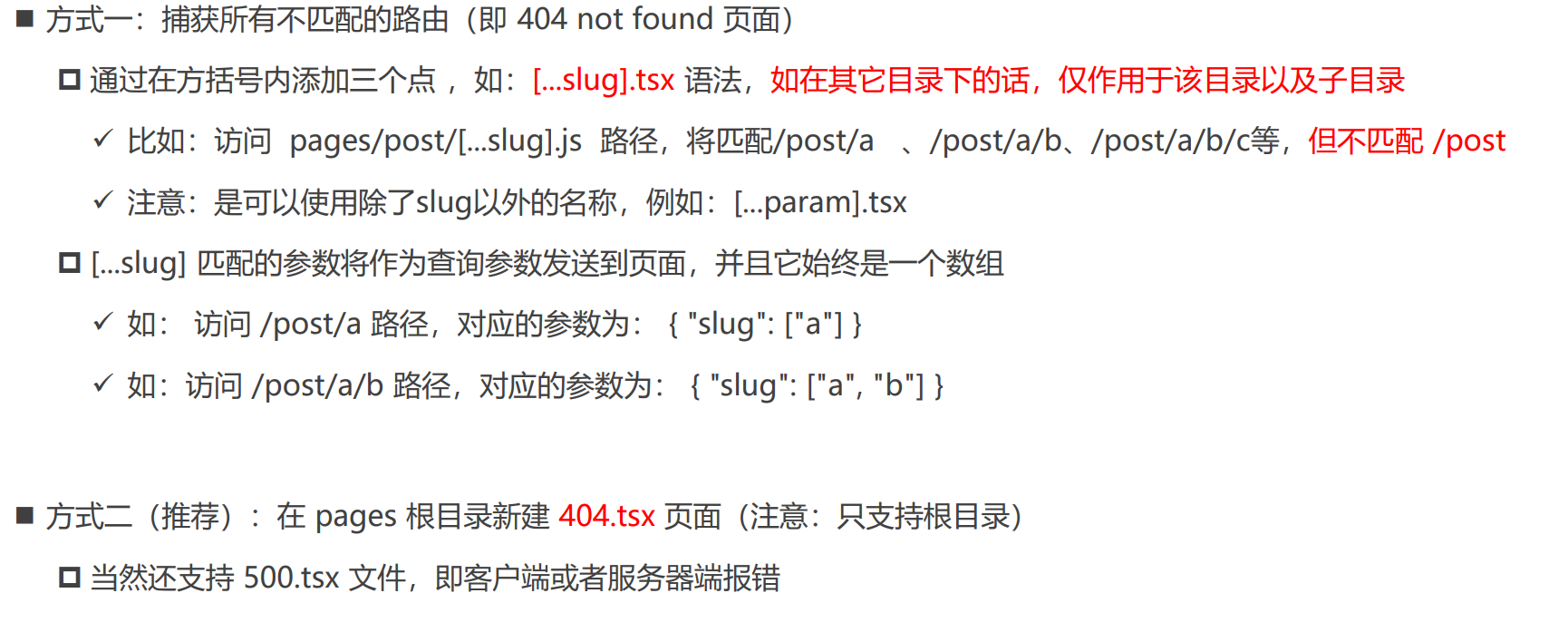
Next.js基础语法
Next.js 目录结构 入口App组件(_app.tsx) _app.tsx是项目的入口组件,主要作用: 可以扩展自定义的布局(Layout)引入全局的样式文件引入Redux状态管理引入主题组件等等全局监听客户端路由的切换 ts.config…...

selenium进阶之web自动化项目框架搭建(Python版)
web自动化项目框架搭建 1、项目结构 web自动化框架的设计,同接口自动化框架一样,采用分层设计。 文件或目录说明common常用模块,常用的一些函数封装testcases用例模块,所有的测试用例test_data用例数据logs日志目录reports报告s…...
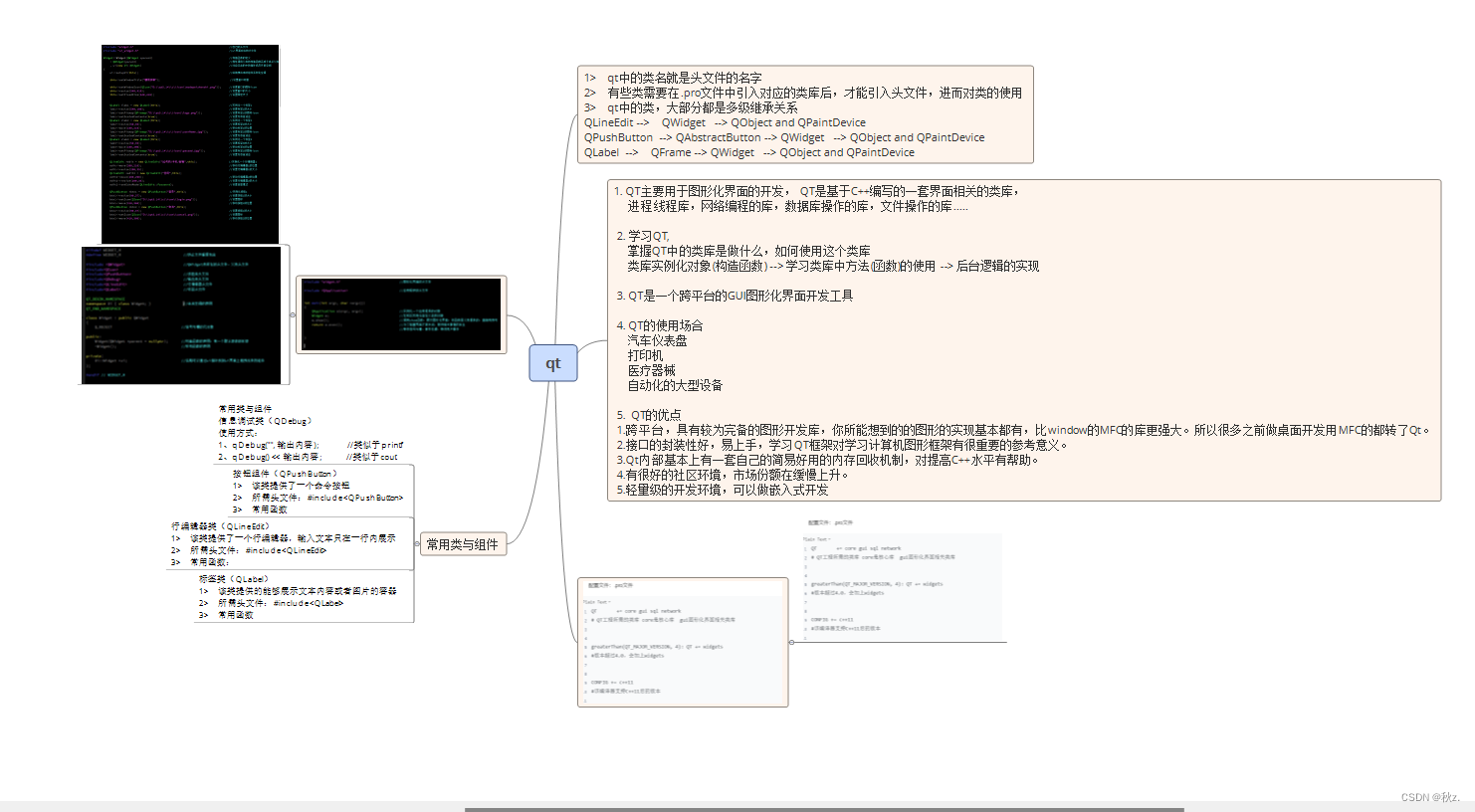
qt设计界面
widget.h #ifndef WIDGET_H #define WIDGET_H //防止文件重复包含#include <QWidget> //QWidget类所在的头文件,父类头文件 #include<QIcon> #include<QPushButton> …...
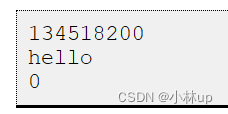
《C和指针》笔记12: 存储类型(自动变量、静态变量和寄存器变量)
文章目录 1. 自动变量(auto)1.1 自动变量的初始化 2. 静态变量(static)2.1 静态变量的初始化 3. 寄存器变量(register) 1. 自动变量(auto) 在代码块内部声明的变量的缺省存储类型是…...
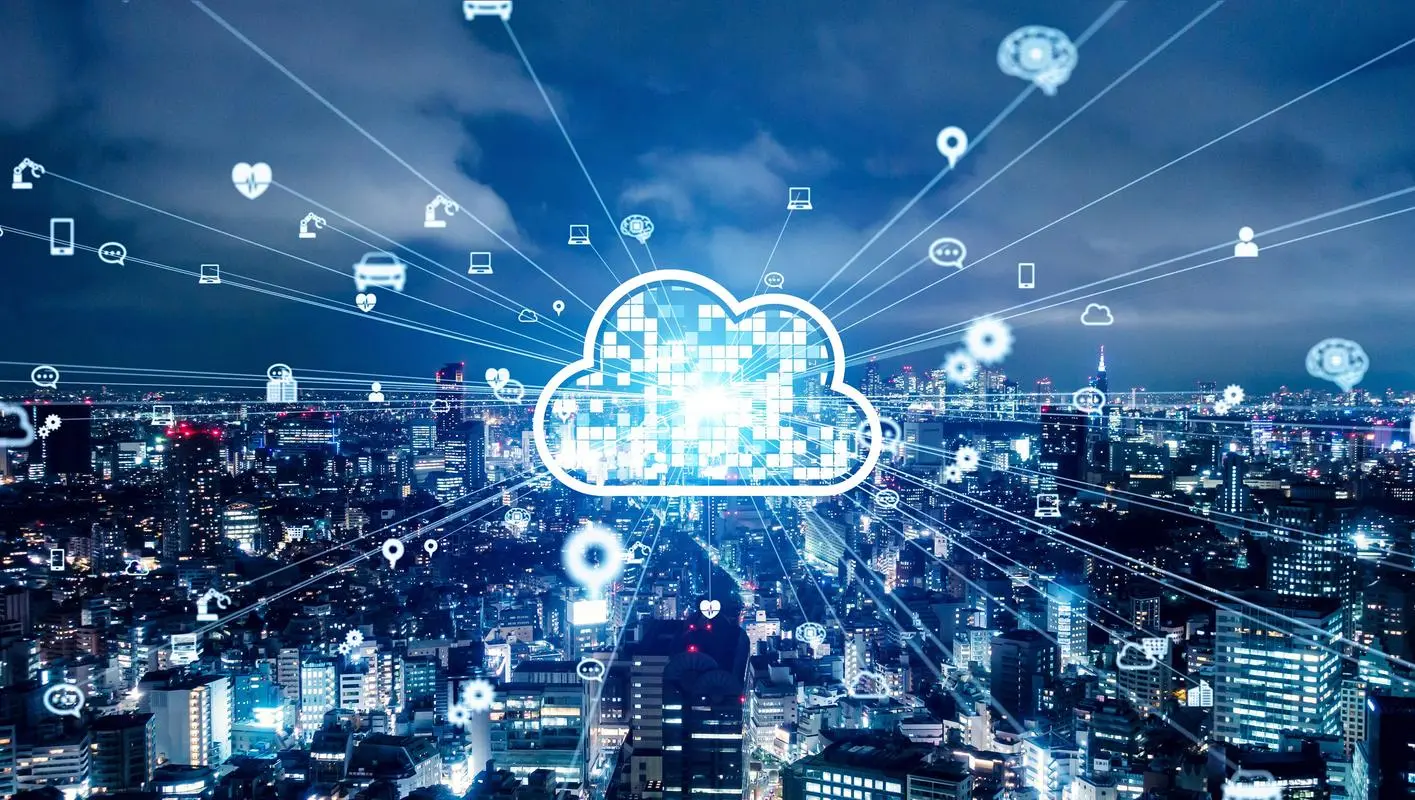
无限计算力:探索云计算的无限可能性
这里写目录标题 前言云计算介绍服务模型: 应用领域:云计算主要体现在生活中的地方云计算未来发展的方向 前言 云计算是一种基于互联网的计算模型,通过它可以实现资源的共享、存储、管理和处理。它已经成为许多个人、企业和组织的重要技术基础…...

【赋权算法】Python实现熵权法
在开始之前,我们先说一下信息熵的概念。 当一件事情发生,如果是意料之中,那么这个事情就并不能拿来当做茶余饭后的谈资,我们可以说这个事情并没有什么信息和价值。而当一件不可能发生的事情发生的时候,我们可能就会觉…...
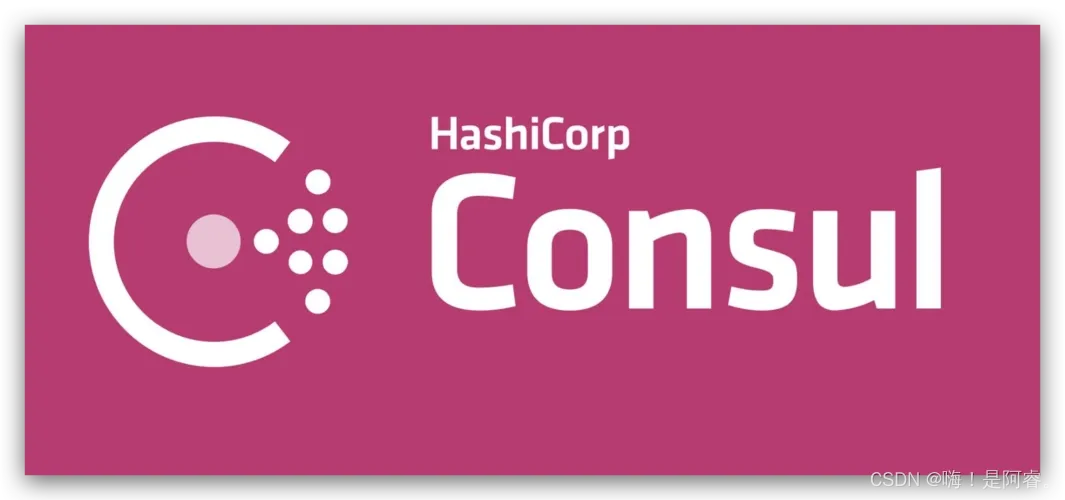
docker之 Consul(注册与发现)
目录 一、什么是服务注册与发现? 二、什么是consul 三、consul 部署 3.1建立Consul服务 3.1.1查看集群状态 3.1.2通过 http api 获取集群信息 3.2registrator服务器 3.2.1安装 Gliderlabs/Registrator 3.2.2测试服务发现功能是否正常 3.2.3验证 http 和 ng…...
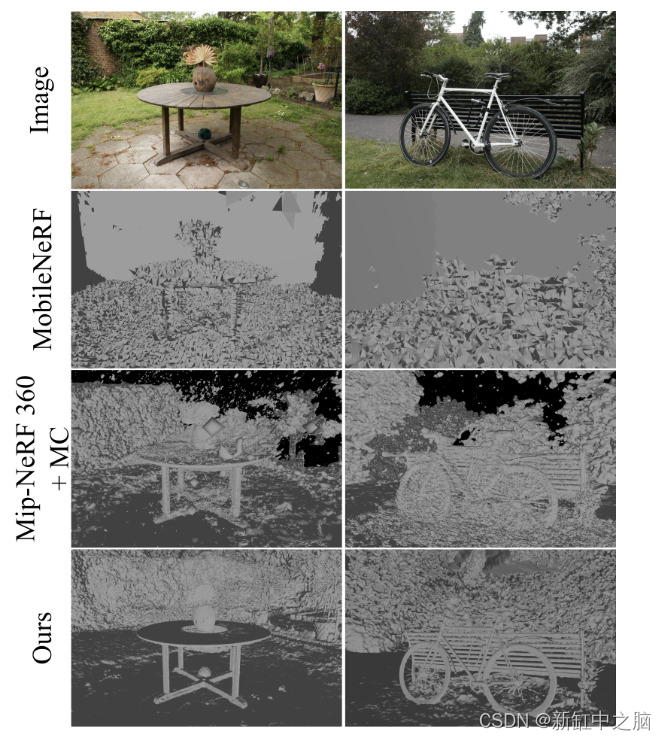
用NeRFMeshing精确提取NeRF网络中的3D网格
准确的 3D 场景和对象重建对于机器人、摄影测量和 AR/VR 等各种应用至关重要。 NeRF 在合成新颖视图方面取得了成功,但在准确表示底层几何方面存在不足。 推荐:用 NSDT编辑器 快速搭建可编程3D场景 我们已经看到了最新的进展,例如 NVIDIA 的…...
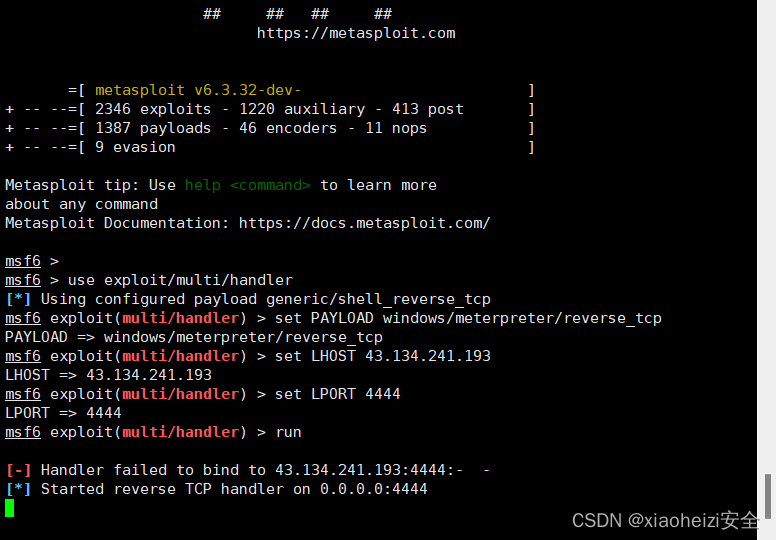
权限提升-Windows本地提权-AT+SC+PS命令-进程迁移-令牌窃取-getsystem+UAC
权限提升基础信息 1、具体有哪些权限需要我们了解掌握的? 后台权限,网站权限,数据库权限,接口权限,系统权限,域控权限等 2、以上常见权限获取方法简要归类说明? 后台权限:SQL注入,数…...
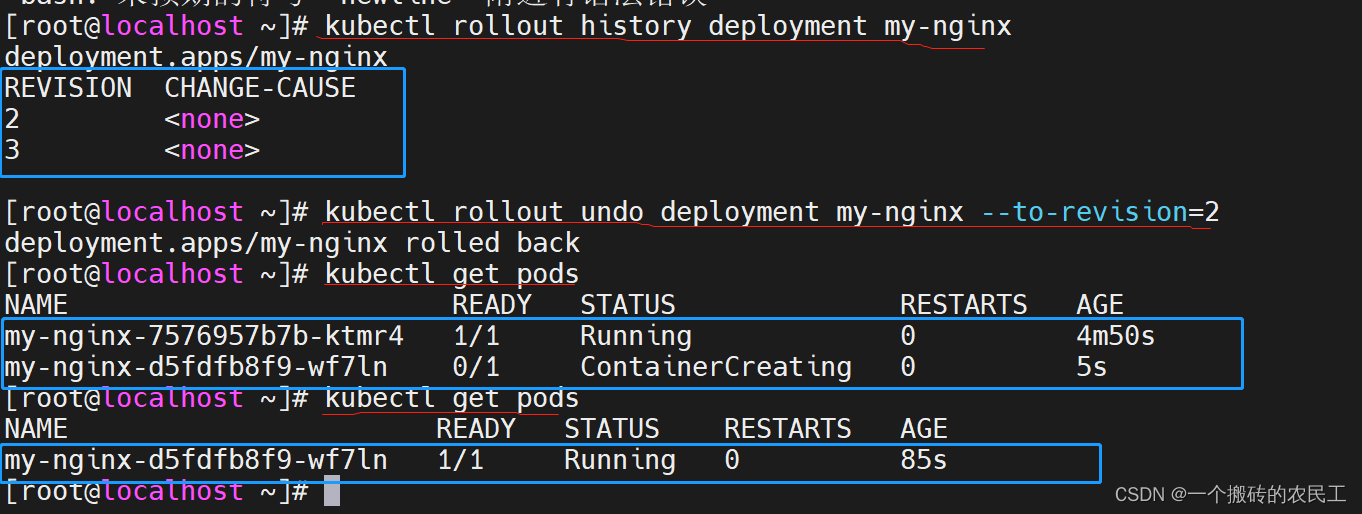
深入了解Kubernetes(k8s):安装、使用和Java部署指南(持续更新中)
目录 Docker 和 k8s 简介1、kubernetes 组件及其联系1.1 Node1.2 Pod1.3 Service 2、安装docker3、单节点 kubernetes 和 KubeSphere 安装3.1 安装KubeKey3.2 安装 kubernetes 和 KubeSphere3.3 验证安装结果 4、集群版 kubernetes 和 KubeSphere 安装5、kubectl 常用命令6、资…...

Oracle的学习心得和知识总结(二十九)|Oracle数据库数据库回放功能之论文三翻译及学习
目录结构 注:提前言明 本文借鉴了以下博主、书籍或网站的内容,其列表如下: 1、参考书籍:《Oracle Database SQL Language Reference》 2、参考书籍:《PostgreSQL中文手册》 3、EDB Postgres Advanced Server User Gui…...
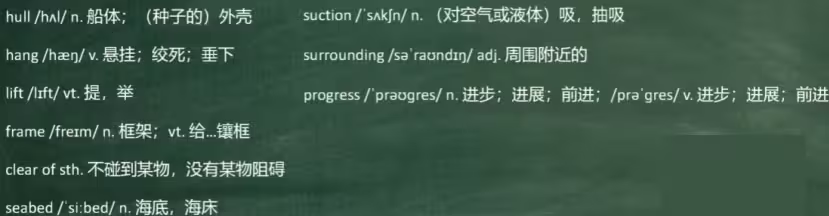
新版100句学完7000雅思单词
新版100句学完7000雅思单词 1. As the medical world continues to grapple with what’s acceptable and what’s not, it is clear that companies must continue to be heavily scrutinized for their sales and marketing strategies.(剑桥雅思6) 随着医学界持续努力解决…...
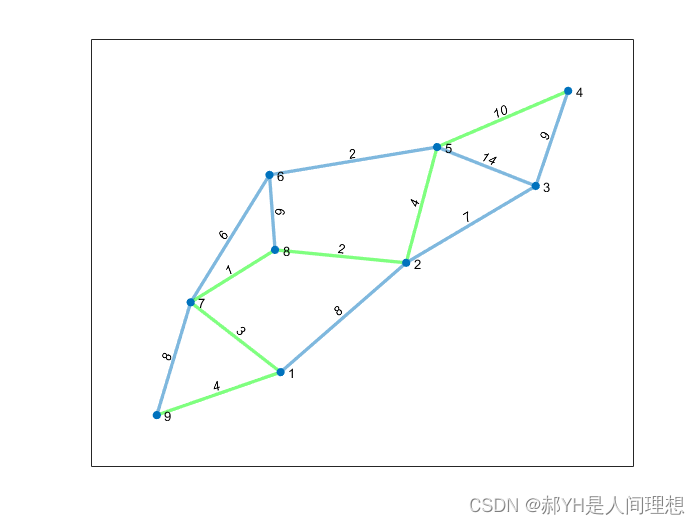
MATLAB图论合集(三)Dijkstra算法计算最短路径
本贴介绍最短路径的计算,实现方式为迪杰斯特拉算法;对于弗洛伊德算法,区别在于计算了所有结点之间的最短路径,考虑到MATLAB计算的便捷性,计算时只需要反复使用迪杰斯特拉即可,暂不介绍弗洛伊德的实现 迪杰斯…...

MySQL 8.0.xx 版本解决group by分组的问题
因为版本升级5.7版本以下是没有这个问题的,8.0版本以上会出现分组问题 1055 - Expression #1 of SELECT list is not in GROUP BY clause and contains nonaggregated column test1.sys_t.id which is not functionally dependent on columns in GROUP BY clause; t…...
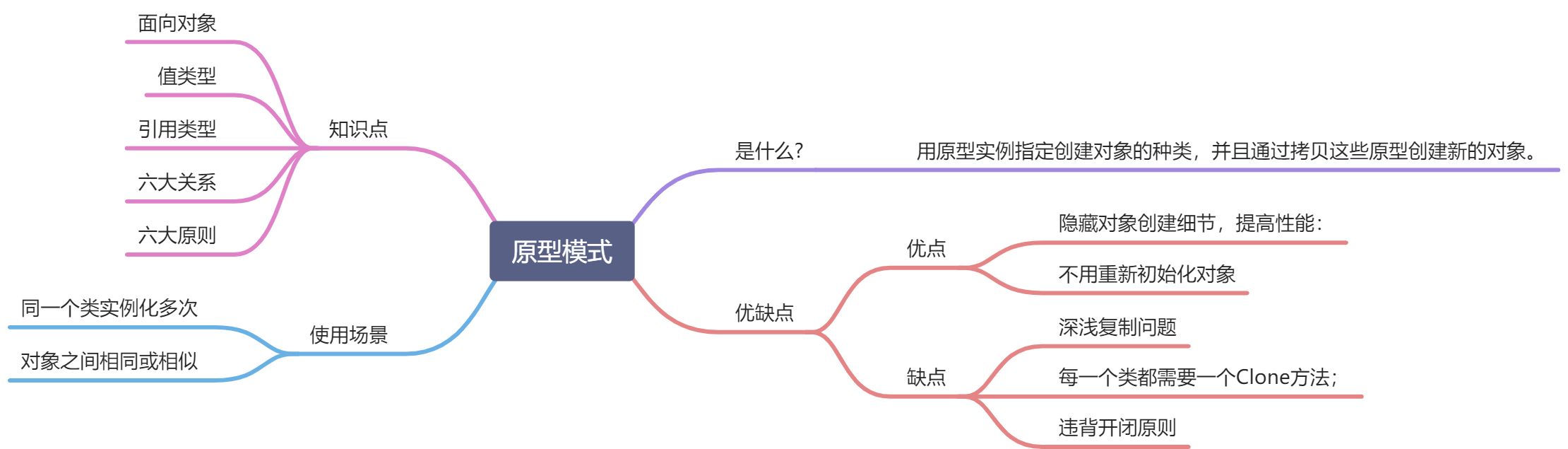
设计模式—原型模式(Prototype)
目录 一、什么是原型模式? 二、原型模式具有什么优缺点吗? 三、有什么缺点? 四、什么时候用原型模式? 五、代码展示 ①、简历代码初步实现 ②、原型模式 ③、简历的原型实现 ④、深复制 ⑤、浅复制 一、什么是原型模式&…...
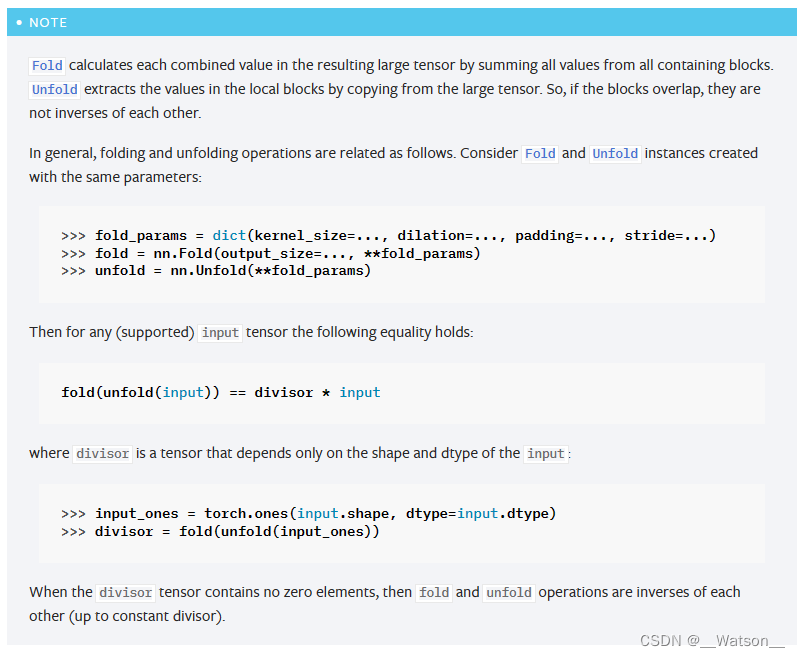
【pytorch】Unfold和Fold的互逆操作
1. 参数定义 Unfold https://pytorch.org/docs/stable/generated/torch.nn.Unfold.html#torch.nn.Unfold Fold https://pytorch.org/docs/stable/generated/torch.nn.Fold.html#torch.nn.Fold 注意:参数当中的padding是在四周边补零,而当fold后的尺寸…...