LORA项目源码解读
大模型fineturn技术中类似于核武器的LORA,简单而又高效。其理论基础为:在将通用大模型迁移到具体专业领域时,仅需要对其高维参数的低秩子空间进行更新。基于该朴素的逻辑,LORA降低大模型的fineturn门槛,模型训练时不需要保存原始参数的梯度,仅需对低秩子空间参数进行优化即可。且其低秩子空间在训练完成后,可以叠加到原始参数中,从而实现模型能力的专业领域迁移。为了解这种高维参数空间=》低秩子空间投影实现研究其项目源码。
项目地址:https://github.com/microsoft/LoRA LORA提出至今已经2年了,但现在任然在更新项目代码
论文地址:https://arxiv.org/pdf/2106.09685.pdf
简读地址:https://blog.csdn.net/a486259/article/details/132767182?spm=1001.2014.3001.5501
1、基本介绍
1.1 实施效果
LORA技术使用RoBERTa(Liu et al.,2019)base和large以及DeBERTa(He et al.,2020)XXL 1.5B在GLUE基准上获得了与完全微调相当或优于完全微调的结果,而只训练和存储了一小部分参数。 LORA技术展现了与全参数迁移学习相同甚至更优的效果
在GPT-2上,LoRA与完全微调和其他大模型微调的方法(如Adapter(Houlsby et al.,2019)和Prefix(Li和Liang,2021))相比都要好。
以上两图不仅展示了LORA在大模型上的微调效果,同时也透露了大模型性能提升的困难。DeBERTa
XXL的参数量是RoBERTa base的一百倍以上,而平均精度仅高4.6%;GPT2 L的参数量是GPT M的两倍以上,而平均精度仅高0.5%左右。这种参数增长与精度增长的差异在图像领域是少见的,尤其是目标检测|语义分割|图像分类中。
1.2 安装使用
这里仅限于官网给出的使用案例。LORA的实际使用应该是基于其他框架展开的
安装命令
pip install loralib
# Alternatively
# pip install git+https://github.com/microsoft/LoRA
构建可低秩训练层
LORA目前除了Linear层外,还支持其他layer。基于lora创建的layer是lora的子类,同时也是torch.nn.module的子类。
# ===== Before =====
# layer = nn.Linear(in_features, out_features)# ===== After ======
import loralib as lora
# Add a pair of low-rank adaptation matrices with rank r=16
layer = lora.Linear(in_features, out_features, r=16)
设置仅LORA层可训练
这里要求model对象中的一些层是lora的子类,mark_only_lora_as_trainable函数会将参数name中不包含lora_的部分都设置为不可训练
import loralib as lora
model = BigModel()
# This sets requires_grad to False for all parameters without the string "lora_" in their names
lora.mark_only_lora_as_trainable(model)
# Training loop
for batch in dataloader:...
保存模型参数
包含LORA层的模型,参数保存分两步完成,第一步保存原始模型的参数(通常可以忽略),第二步才是保存lora层的参数,对应代码为:torch.save(lora.lora_state_dict(model), checkpoint_path)
# ===== Before =====
torch.save(model.state_dict(), checkpoint_path)
# ===== After =====
torch.save(lora.lora_state_dict(model), checkpoint_path)
加载模型参数
包含lora层的模型参数加载也是分两步完成,第一步加载原始参数,第二步为加载lora层参数。
# Load the pretrained checkpoint first
model.load_state_dict(torch.load('ckpt_pretrained.pt'), strict=False)
# Then load the LoRA checkpoint
model.load_state_dict(torch.load('ckpt_lora.pt'), strict=False)
额外说明
某些Transformer实现使用单个nn.Linear
。查询、键和值的投影矩阵为nn.Linear。如果希望将更新的秩约束到单个矩阵,则必须将其分解为三个单独的矩阵或使用lora.MergedLinear
。如果选择分解层,请确保相应地修改checkpoint 。
# ===== Before =====
# qkv_proj = nn.Linear(d_model, 3*d_model)
# ===== After =====
# Break it up (remember to modify the pretrained checkpoint accordingly)
q_proj = lora.Linear(d_model, d_model, r=8)
k_proj = nn.Linear(d_model, d_model)
v_proj = lora.Linear(d_model, d_model, r=8)
# Alternatively, use lora.MergedLinear (recommended)
qkv_proj = lora.MergedLinear(d_model, 3*d_model, r=8, enable_lora=[True, False, True])
2、代码解读
lora项目的源码如下所示,其核心代码仅有layers.py和utils.py两个文件。
examples是两个使用案例,为第三方代码,这里不深入探讨。
2.1 Layer.py
在lora源码中,共有Embedding、Linear、MergedLinear、ConvLoRA 四种layer对象,均为nn.Module与 LoRALayer的子类。
样板layer解析
lora源码中layer对象比较多,这里只对Linear和·ConvLoRA 进行详细描述
Linear
在lora中,对于Linear的低秩分解由矩阵A、B的乘法所实现,其在forward时,lora分支BA
lora_dropout操作,并对BA的输出结果进行scale操作。当调用layer.train(True)时,会根据self.merged参数将weight中的BA参数累加进行移除,当调用layer.train(False)时,则会将将BA参数累加到weight中。
这里需要注意,LoRA.Linear是nn.Linear的子类,在使用时直接参考nn.Linear的用法即可。
class Linear(nn.Linear, LoRALayer):# LoRA implemented in a dense layerdef __init__(self, in_features: int, out_features: int, r: int = 0, lora_alpha: int = 1, lora_dropout: float = 0.,fan_in_fan_out: bool = False, # Set this to True if the layer to replace stores weight like (fan_in, fan_out)merge_weights: bool = True,**kwargs):nn.Linear.__init__(self, in_features, out_features, **kwargs)LoRALayer.__init__(self, r=r, lora_alpha=lora_alpha, lora_dropout=lora_dropout,merge_weights=merge_weights)self.fan_in_fan_out = fan_in_fan_out# Actual trainable parametersif r > 0:self.lora_A = nn.Parameter(self.weight.new_zeros((r, in_features)))self.lora_B = nn.Parameter(self.weight.new_zeros((out_features, r)))self.scaling = self.lora_alpha / self.r# Freezing the pre-trained weight matrixself.weight.requires_grad = Falseself.reset_parameters()if fan_in_fan_out:self.weight.data = self.weight.data.transpose(0, 1)def reset_parameters(self):nn.Linear.reset_parameters(self)if hasattr(self, 'lora_A'):# initialize A the same way as the default for nn.Linear and B to zeronn.init.kaiming_uniform_(self.lora_A, a=math.sqrt(5))nn.init.zeros_(self.lora_B)def train(self, mode: bool = True):def T(w):return w.transpose(0, 1) if self.fan_in_fan_out else wnn.Linear.train(self, mode)if mode:if self.merge_weights and self.merged:# Make sure that the weights are not mergedif self.r > 0:self.weight.data -= T(self.lora_B @ self.lora_A) * self.scalingself.merged = Falseelse:if self.merge_weights and not self.merged:# Merge the weights and mark itif self.r > 0:self.weight.data += T(self.lora_B @ self.lora_A) * self.scalingself.merged = True def forward(self, x: torch.Tensor):def T(w):return w.transpose(0, 1) if self.fan_in_fan_out else wif self.r > 0 and not self.merged:result = F.linear(x, T(self.weight), bias=self.bias) result += (self.lora_dropout(x) @ self.lora_A.transpose(0, 1) @ self.lora_B.transpose(0, 1)) * self.scalingreturn resultelse:return F.linear(x, T(self.weight), bias=self.bias)
ConvLoRA
LORA能对conv进行低秩分解,是博主意料之外的。该操作完整的将LoRALinear的思想应用到conv kernel中,有self.lora_B 和 self.lora_A两个可训练参数表述conv的kernel参数,将self.lora_B @ self.lora_A的结果直接作用到conv.weight中,然后调用self.conv._conv_forward完成卷积操作。
这里需要注意的是,使用ConvLoRA跟使用torch.nn.Conv是没有任何区别。这里只有一个问题,我们不能直接将conv对象转换为ConvLoRA对象。需要在构建网络时就使用ConvLoRA layer
class Conv2d(ConvLoRA):def __init__(self, *args, **kwargs):super(Conv2d, self).__init__(nn.Conv2d, *args, **kwargs)class Conv1d(ConvLoRA):def __init__(self, *args, **kwargs):super(Conv1d, self).__init__(nn.Conv1d, *args, **kwargs)class Conv3d(ConvLoRA):def __init__(self, *args, **kwargs):super(Conv3d, self).__init__(nn.Conv3d, *args, **kwargs)class ConvLoRA(nn.Module, LoRALayer):def __init__(self, conv_module, in_channels, out_channels, kernel_size, r=0, lora_alpha=1, lora_dropout=0., merge_weights=True, **kwargs):super(ConvLoRA, self).__init__()self.conv = conv_module(in_channels, out_channels, kernel_size, **kwargs)LoRALayer.__init__(self, r=r, lora_alpha=lora_alpha, lora_dropout=lora_dropout, merge_weights=merge_weights)assert isinstance(kernel_size, int)# Actual trainable parametersif r > 0:self.lora_A = nn.Parameter(self.conv.weight.new_zeros((r * kernel_size, in_channels * kernel_size)))self.lora_B = nn.Parameter(self.conv.weight.new_zeros((out_channels//self.conv.groups*kernel_size, r*kernel_size)))self.scaling = self.lora_alpha / self.r# Freezing the pre-trained weight matrixself.conv.weight.requires_grad = Falseself.reset_parameters()self.merged = Falsedef reset_parameters(self):self.conv.reset_parameters()if hasattr(self, 'lora_A'):# initialize A the same way as the default for nn.Linear and B to zeronn.init.kaiming_uniform_(self.lora_A, a=math.sqrt(5))nn.init.zeros_(self.lora_B)def train(self, mode=True):super(ConvLoRA, self).train(mode)if mode:if self.merge_weights and self.merged:if self.r > 0:# Make sure that the weights are not mergedself.conv.weight.data -= (self.lora_B @ self.lora_A).view(self.conv.weight.shape) * self.scalingself.merged = Falseelse:if self.merge_weights and not self.merged:if self.r > 0:# Merge the weights and mark itself.conv.weight.data += (self.lora_B @ self.lora_A).view(self.conv.weight.shape) * self.scalingself.merged = Truedef forward(self, x):if self.r > 0 and not self.merged:return self.conv._conv_forward(x, self.conv.weight + (self.lora_B @ self.lora_A).view(self.conv.weight.shape) * self.scaling,self.conv.bias)return self.conv(x)
完整代码
# ------------------------------------------------------------------------------------------
# Copyright (c) Microsoft Corporation. All rights reserved.
# Licensed under the MIT License (MIT). See LICENSE in the repo root for license information.
# ------------------------------------------------------------------------------------------
import torch
import torch.nn as nn
import torch.nn.functional as Fimport math
from typing import Optional, Listclass LoRALayer():def __init__(self, r: int, lora_alpha: int, lora_dropout: float,merge_weights: bool,):self.r = rself.lora_alpha = lora_alpha# Optional dropoutif lora_dropout > 0.:self.lora_dropout = nn.Dropout(p=lora_dropout)else:self.lora_dropout = lambda x: x# Mark the weight as unmergedself.merged = Falseself.merge_weights = merge_weightsclass Embedding(nn.Embedding, LoRALayer):# LoRA implemented in a dense layerdef __init__(self,num_embeddings: int,embedding_dim: int,r: int = 0,lora_alpha: int = 1,merge_weights: bool = True,**kwargs):nn.Embedding.__init__(self, num_embeddings, embedding_dim, **kwargs)LoRALayer.__init__(self, r=r, lora_alpha=lora_alpha, lora_dropout=0,merge_weights=merge_weights)# Actual trainable parametersif r > 0:self.lora_A = nn.Parameter(self.weight.new_zeros((r, num_embeddings)))self.lora_B = nn.Parameter(self.weight.new_zeros((embedding_dim, r)))self.scaling = self.lora_alpha / self.r# Freezing the pre-trained weight matrixself.weight.requires_grad = Falseself.reset_parameters()def reset_parameters(self):nn.Embedding.reset_parameters(self)if hasattr(self, 'lora_A'):# initialize A the same way as the default for nn.Linear and B to zeronn.init.zeros_(self.lora_A)nn.init.normal_(self.lora_B)def train(self, mode: bool = True):nn.Embedding.train(self, mode)if mode:if self.merge_weights and self.merged:# Make sure that the weights are not mergedif self.r > 0:self.weight.data -= (self.lora_B @ self.lora_A).transpose(0, 1) * self.scalingself.merged = Falseelse:if self.merge_weights and not self.merged:# Merge the weights and mark itif self.r > 0:self.weight.data += (self.lora_B @ self.lora_A).transpose(0, 1) * self.scalingself.merged = Truedef forward(self, x: torch.Tensor):if self.r > 0 and not self.merged:result = nn.Embedding.forward(self, x)after_A = F.embedding(x, self.lora_A.transpose(0, 1), self.padding_idx, self.max_norm,self.norm_type, self.scale_grad_by_freq, self.sparse)result += (after_A @ self.lora_B.transpose(0, 1)) * self.scalingreturn resultelse:return nn.Embedding.forward(self, x)class Linear(nn.Linear, LoRALayer):# LoRA implemented in a dense layerdef __init__(self, in_features: int, out_features: int, r: int = 0, lora_alpha: int = 1, lora_dropout: float = 0.,fan_in_fan_out: bool = False, # Set this to True if the layer to replace stores weight like (fan_in, fan_out)merge_weights: bool = True,**kwargs):nn.Linear.__init__(self, in_features, out_features, **kwargs)LoRALayer.__init__(self, r=r, lora_alpha=lora_alpha, lora_dropout=lora_dropout,merge_weights=merge_weights)self.fan_in_fan_out = fan_in_fan_out# Actual trainable parametersif r > 0:self.lora_A = nn.Parameter(self.weight.new_zeros((r, in_features)))self.lora_B = nn.Parameter(self.weight.new_zeros((out_features, r)))self.scaling = self.lora_alpha / self.r# Freezing the pre-trained weight matrixself.weight.requires_grad = Falseself.reset_parameters()if fan_in_fan_out:self.weight.data = self.weight.data.transpose(0, 1)def reset_parameters(self):nn.Linear.reset_parameters(self)if hasattr(self, 'lora_A'):# initialize A the same way as the default for nn.Linear and B to zeronn.init.kaiming_uniform_(self.lora_A, a=math.sqrt(5))nn.init.zeros_(self.lora_B)def train(self, mode: bool = True):def T(w):return w.transpose(0, 1) if self.fan_in_fan_out else wnn.Linear.train(self, mode)if mode:if self.merge_weights and self.merged:# Make sure that the weights are not mergedif self.r > 0:self.weight.data -= T(self.lora_B @ self.lora_A) * self.scalingself.merged = Falseelse:if self.merge_weights and not self.merged:# Merge the weights and mark itif self.r > 0:self.weight.data += T(self.lora_B @ self.lora_A) * self.scalingself.merged = True def forward(self, x: torch.Tensor):def T(w):return w.transpose(0, 1) if self.fan_in_fan_out else wif self.r > 0 and not self.merged:result = F.linear(x, T(self.weight), bias=self.bias) result += (self.lora_dropout(x) @ self.lora_A.transpose(0, 1) @ self.lora_B.transpose(0, 1)) * self.scalingreturn resultelse:return F.linear(x, T(self.weight), bias=self.bias)class MergedLinear(nn.Linear, LoRALayer):# LoRA implemented in a dense layerdef __init__(self, in_features: int, out_features: int, r: int = 0, lora_alpha: int = 1, lora_dropout: float = 0.,enable_lora: List[bool] = [False],fan_in_fan_out: bool = False,merge_weights: bool = True,**kwargs):nn.Linear.__init__(self, in_features, out_features, **kwargs)LoRALayer.__init__(self, r=r, lora_alpha=lora_alpha, lora_dropout=lora_dropout,merge_weights=merge_weights)assert out_features % len(enable_lora) == 0, \'The length of enable_lora must divide out_features'self.enable_lora = enable_loraself.fan_in_fan_out = fan_in_fan_out# Actual trainable parametersif r > 0 and any(enable_lora):self.lora_A = nn.Parameter(self.weight.new_zeros((r * sum(enable_lora), in_features)))self.lora_B = nn.Parameter(self.weight.new_zeros((out_features // len(enable_lora) * sum(enable_lora), r))) # weights for Conv1D with groups=sum(enable_lora)self.scaling = self.lora_alpha / self.r# Freezing the pre-trained weight matrixself.weight.requires_grad = False# Compute the indicesself.lora_ind = self.weight.new_zeros((out_features, ), dtype=torch.bool).view(len(enable_lora), -1)self.lora_ind[enable_lora, :] = Trueself.lora_ind = self.lora_ind.view(-1)self.reset_parameters()if fan_in_fan_out:self.weight.data = self.weight.data.transpose(0, 1)def reset_parameters(self):nn.Linear.reset_parameters(self)if hasattr(self, 'lora_A'):# initialize A the same way as the default for nn.Linear and B to zeronn.init.kaiming_uniform_(self.lora_A, a=math.sqrt(5))nn.init.zeros_(self.lora_B)def zero_pad(self, x):result = x.new_zeros((len(self.lora_ind), *x.shape[1:]))result[self.lora_ind] = xreturn resultdef merge_AB(self):def T(w):return w.transpose(0, 1) if self.fan_in_fan_out else wdelta_w = F.conv1d(self.lora_A.unsqueeze(0), self.lora_B.unsqueeze(-1), groups=sum(self.enable_lora)).squeeze(0)return T(self.zero_pad(delta_w))def train(self, mode: bool = True):def T(w):return w.transpose(0, 1) if self.fan_in_fan_out else wnn.Linear.train(self, mode)if mode:if self.merge_weights and self.merged:# Make sure that the weights are not mergedif self.r > 0 and any(self.enable_lora):self.weight.data -= self.merge_AB() * self.scalingself.merged = Falseelse:if self.merge_weights and not self.merged:# Merge the weights and mark itif self.r > 0 and any(self.enable_lora):self.weight.data += self.merge_AB() * self.scalingself.merged = True def forward(self, x: torch.Tensor):def T(w):return w.transpose(0, 1) if self.fan_in_fan_out else wif self.merged:return F.linear(x, T(self.weight), bias=self.bias)else:result = F.linear(x, T(self.weight), bias=self.bias)if self.r > 0:result += self.lora_dropout(x) @ T(self.merge_AB().T) * self.scalingreturn resultclass ConvLoRA(nn.Module, LoRALayer):def __init__(self, conv_module, in_channels, out_channels, kernel_size, r=0, lora_alpha=1, lora_dropout=0., merge_weights=True, **kwargs):super(ConvLoRA, self).__init__()self.conv = conv_module(in_channels, out_channels, kernel_size, **kwargs)LoRALayer.__init__(self, r=r, lora_alpha=lora_alpha, lora_dropout=lora_dropout, merge_weights=merge_weights)assert isinstance(kernel_size, int)# Actual trainable parametersif r > 0:self.lora_A = nn.Parameter(self.conv.weight.new_zeros((r * kernel_size, in_channels * kernel_size)))self.lora_B = nn.Parameter(self.conv.weight.new_zeros((out_channels//self.conv.groups*kernel_size, r*kernel_size)))self.scaling = self.lora_alpha / self.r# Freezing the pre-trained weight matrixself.conv.weight.requires_grad = Falseself.reset_parameters()self.merged = Falsedef reset_parameters(self):self.conv.reset_parameters()if hasattr(self, 'lora_A'):# initialize A the same way as the default for nn.Linear and B to zeronn.init.kaiming_uniform_(self.lora_A, a=math.sqrt(5))nn.init.zeros_(self.lora_B)def train(self, mode=True):super(ConvLoRA, self).train(mode)if mode:if self.merge_weights and self.merged:if self.r > 0:# Make sure that the weights are not mergedself.conv.weight.data -= (self.lora_B @ self.lora_A).view(self.conv.weight.shape) * self.scalingself.merged = Falseelse:if self.merge_weights and not self.merged:if self.r > 0:# Merge the weights and mark itself.conv.weight.data += (self.lora_B @ self.lora_A).view(self.conv.weight.shape) * self.scalingself.merged = Truedef forward(self, x):if self.r > 0 and not self.merged:return self.conv._conv_forward(x, self.conv.weight + (self.lora_B @ self.lora_A).view(self.conv.weight.shape) * self.scaling,self.conv.bias)return self.conv(x)class Conv2d(ConvLoRA):def __init__(self, *args, **kwargs):super(Conv2d, self).__init__(nn.Conv2d, *args, **kwargs)class Conv1d(ConvLoRA):def __init__(self, *args, **kwargs):super(Conv1d, self).__init__(nn.Conv1d, *args, **kwargs)# Can Extend to other ones like thisclass Conv3d(ConvLoRA):def __init__(self, *args, **kwargs):super(Conv3d, self).__init__(nn.Conv3d, *args, **kwargs)
2.2 utils.py
期内有mark_only_lora_as_trainable、lora_state_dict两个函数。mark_only_lora_as_trainable函数用于冻结模型的非lora layer参数,该函数基于name区分lora layer 层name中包含lora_
。其参数bias设置用于设model中的bias是否可训练,bias == 'none'表示忽略bias
,bias == 'all'表示所有偏置都可以训练
,bias == 'lora_only'表示仅有lora layer的bias可以训练
lora_state_dict函数用于加载lora保存的参数,参数bias == 'none'表明只加载lora参数
,参数bias == 'all'表明加载lora参数和所有bias参数
,
import torch
import torch.nn as nn
from typing import Dict
from .layers import LoRALayerdef mark_only_lora_as_trainable(model: nn.Module, bias: str = 'none') -> None:for n, p in model.named_parameters():if 'lora_' not in n:p.requires_grad = Falseif bias == 'none':returnelif bias == 'all':for n, p in model.named_parameters():if 'bias' in n:p.requires_grad = Trueelif bias == 'lora_only':for m in model.modules():if isinstance(m, LoRALayer) and \hasattr(m, 'bias') and \m.bias is not None:m.bias.requires_grad = Trueelse:raise NotImplementedErrordef lora_state_dict(model: nn.Module, bias: str = 'none') -> Dict[str, torch.Tensor]:my_state_dict = model.state_dict()if bias == 'none':return {k: my_state_dict[k] for k in my_state_dict if 'lora_' in k}elif bias == 'all':return {k: my_state_dict[k] for k in my_state_dict if 'lora_' in k or 'bias' in k}elif bias == 'lora_only':to_return = {}for k in my_state_dict:if 'lora_' in k:to_return[k] = my_state_dict[k]bias_name = k.split('lora_')[0]+'bias'if bias_name in my_state_dict:to_return[bias_name] = my_state_dict[bias_name]return to_returnelse:raise NotImplementedError
相关文章:
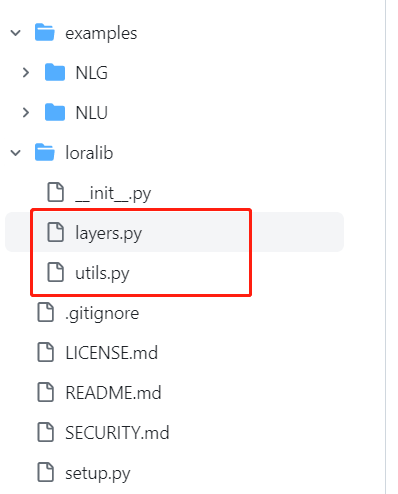
LORA项目源码解读
大模型fineturn技术中类似于核武器的LORA,简单而又高效。其理论基础为:在将通用大模型迁移到具体专业领域时,仅需要对其高维参数的低秩子空间进行更新。基于该朴素的逻辑,LORA降低大模型的fineturn门槛,模型训练时不需…...

Azure + React + ASP.NET Core 项目笔记一:项目环境搭建(一)
不重要的目录标题 前提条件第一步:新建文件夹第二步:使用VS/ VS code/cmd 打开该文件夹第三步:安装依赖第四步:试运行react第五步:整理项目结构 前提条件 安装dotnet core sdk 安装Node.js npm 第一步:新…...
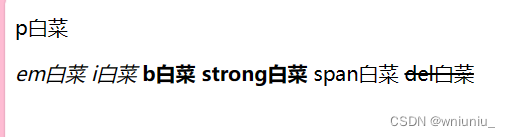
html 学习 之 文本标签
下面是一些常见的HTML文本标签(,,,,和)以及它们的作用: 标签 (Emphasis - 强调): 作用:用于在文本中表示强调或重要性。 示例: <p>这是一段文本,&l…...
联发科3纳米芯片预计2024年量产,此前称仍未获批给华为供货
9月7日,联发科与台积电共同宣布,联发科首款采用台积电3纳米制程生产的天玑旗舰芯片开发进度顺利,已成功流片,预计将在2024年量产,并将于下半年正式上市。这款旗舰芯片并非今年上市的天玑9300。 据联发科总经理陈冠州介…...
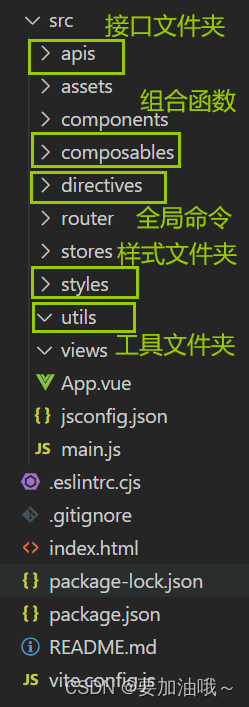
搭建vue3项目并git管理
搭建vue3项目 采用vue3的create-vue脚手架搭建项目,底层是vite,要求环境 node 16.0及以上(node -v检查node版本) 在文件夹右键->终端-> npm init vuelatest,输入项目名称,根据需要选择是否装包 src…...
【Azure OpenAI】OpenAI Function Calling 101
概述 本文是结合 github:OpenAI Function Calling 101在 Azure OpenAI 上的实现: Github Function Calling 101 如何将函数调用与 Azure OpenAI 服务配合使用 - Azure OpenAI Service 使用像ChatGPT这样的llm的困难之一是它们不产生结构化的数据输出…...
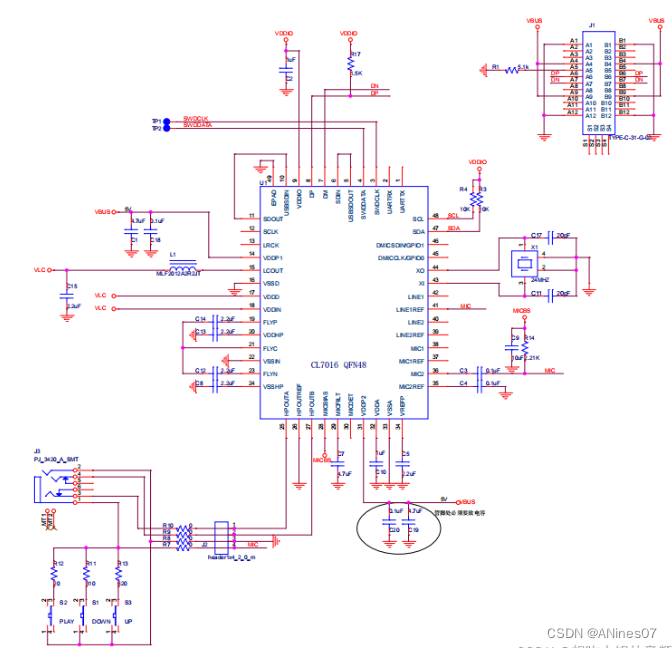
立晶半导体Cubic Lattice Inc 专攻音频ADC,音频DAC,音频CODEC,音频CLASS D等CL7016
概述: CL7016是一款高保真USB Type-C兼容音频编解码芯片。可以录制和回放有24比特音乐和声音。内置回放通路信号动态压缩, 最大42db录音通路增益,PDM数字麦克风,和立体声无需电容耳机驱动放大器。 5V单电源供电。兼容USB 2.0全速工…...
【Flutter】支持多平台 多端保存图片到本地相册 (兼容 Web端 移动端 android 保存到本地)
免责声明: 我只测试了Web端 和 Android端 可行哈 import dart:io; import package:flutter/services.dart; import package:http/http.dart as http; import package:universal_html/html.dart as html; import package:oktoast/oktoast.dart; import package:image_gallery_sa…...
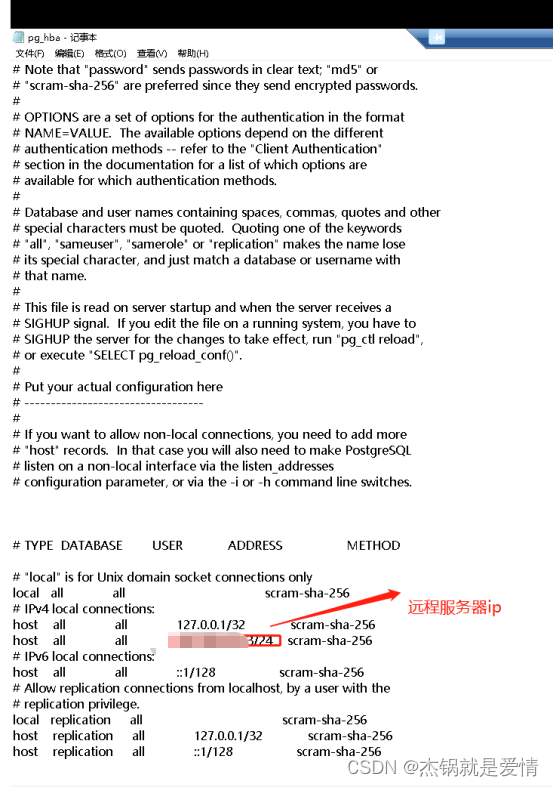
postgresql 安装教程
postgresql 安装教程 本文以window 15版本为教程 文章目录 postgresql 安装教程1.下载地址2.以管理员身份运行3.选择安装路径,点击Next4.选择组件(默认都勾选),点击Next5.选择数据存储路径,点击Next6.设置超级用户的…...
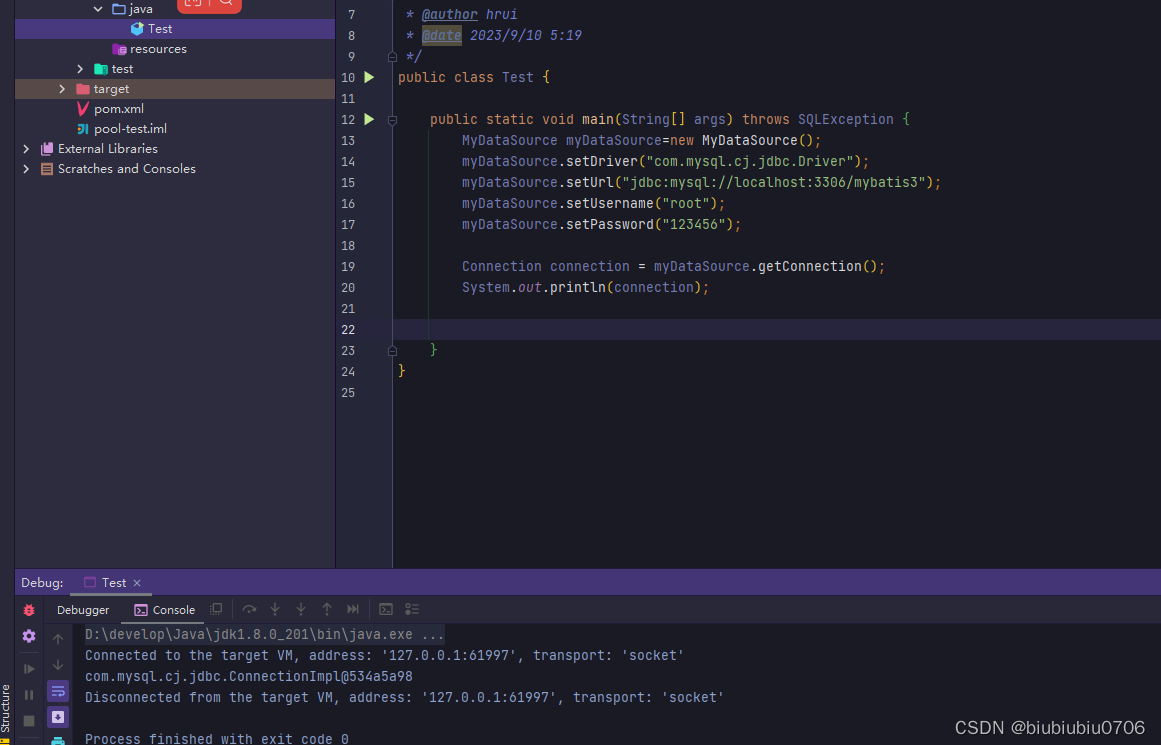
手写数据库连接池
数据库连接是个耗时操作.对数据库连接的高效管理影响应用程序的性能指标. 数据库连接池正是针对这个问题提出来的. 数据库连接池负责分配,管理和释放数据库连接.它允许应用程序重复使用一个现有的数据路连接,而不需要每次重新建立一个新的连接,利用数据库连接池将明显提升对数…...
在CentOS7上增加swap空间
在CentOS7上增加swap空间 在CentOS7上增加swap空间,可以按照以下步骤进行操作: 使用以下命令检查当前swap使用情况: swapon --show创建一个新的swap文件。你可以根据需要指定大小。例如,要创建一个2GB的swap文件,使用…...
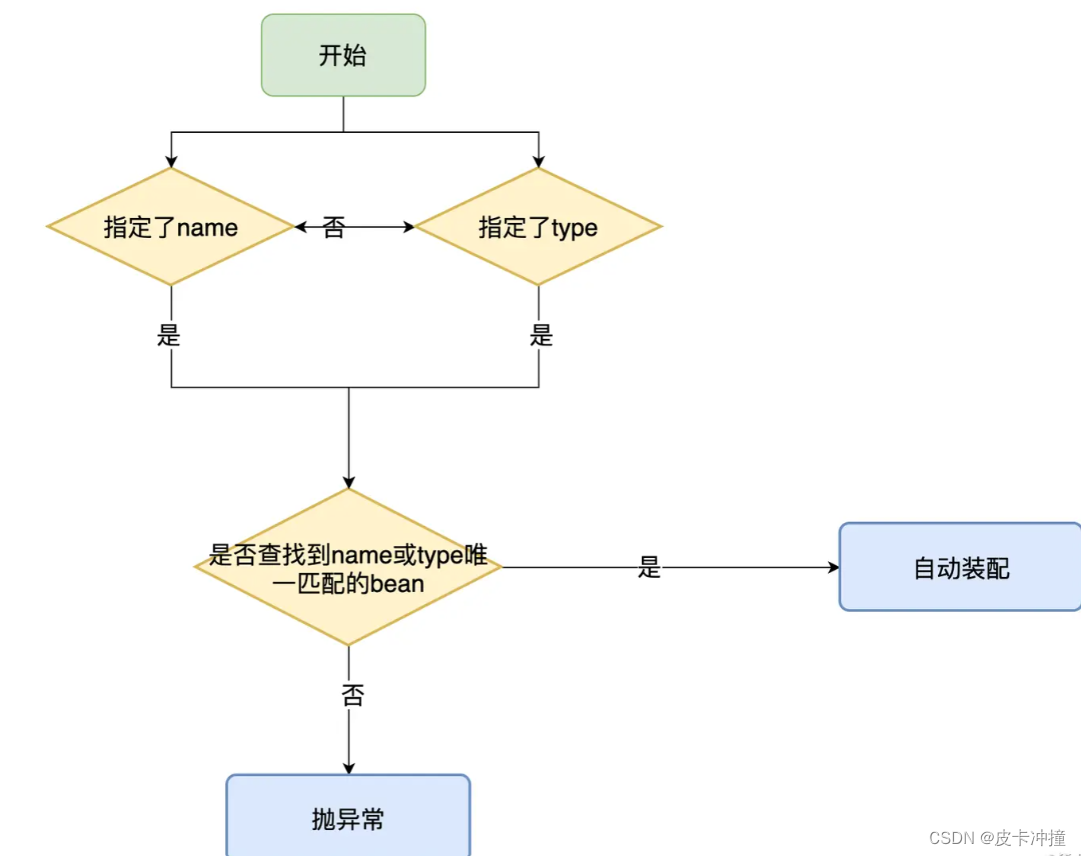
@Autowired和@Resource
文章目录 简介Autowired注解什么是Autowired注解Autowired注解的使用方式Autowired注解的优势和不足 Qualifier总结: Resource注解什么是Resource注解Resource注解的使用方式Resource注解的优势和不足 Autowired vs ResourceAutowired和Resource的区别为什么推荐使用…...
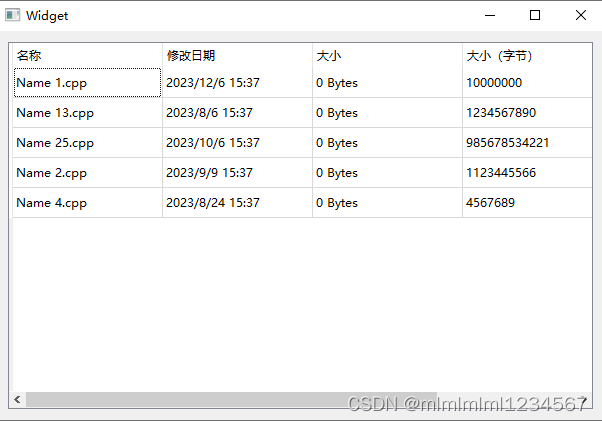
QTableView通过setColumnWidth设置了列宽无效的问题
在用到QT的QTableView时,为了显示效果,向手动的设置每一列的宽度,但是如下的代码是无效的。 ui->tableView->setColumnWidth(0,150);ui->tableView->setColumnWidth(1,150);ui->tableView->setColumnWidth(2,150);ui->t…...
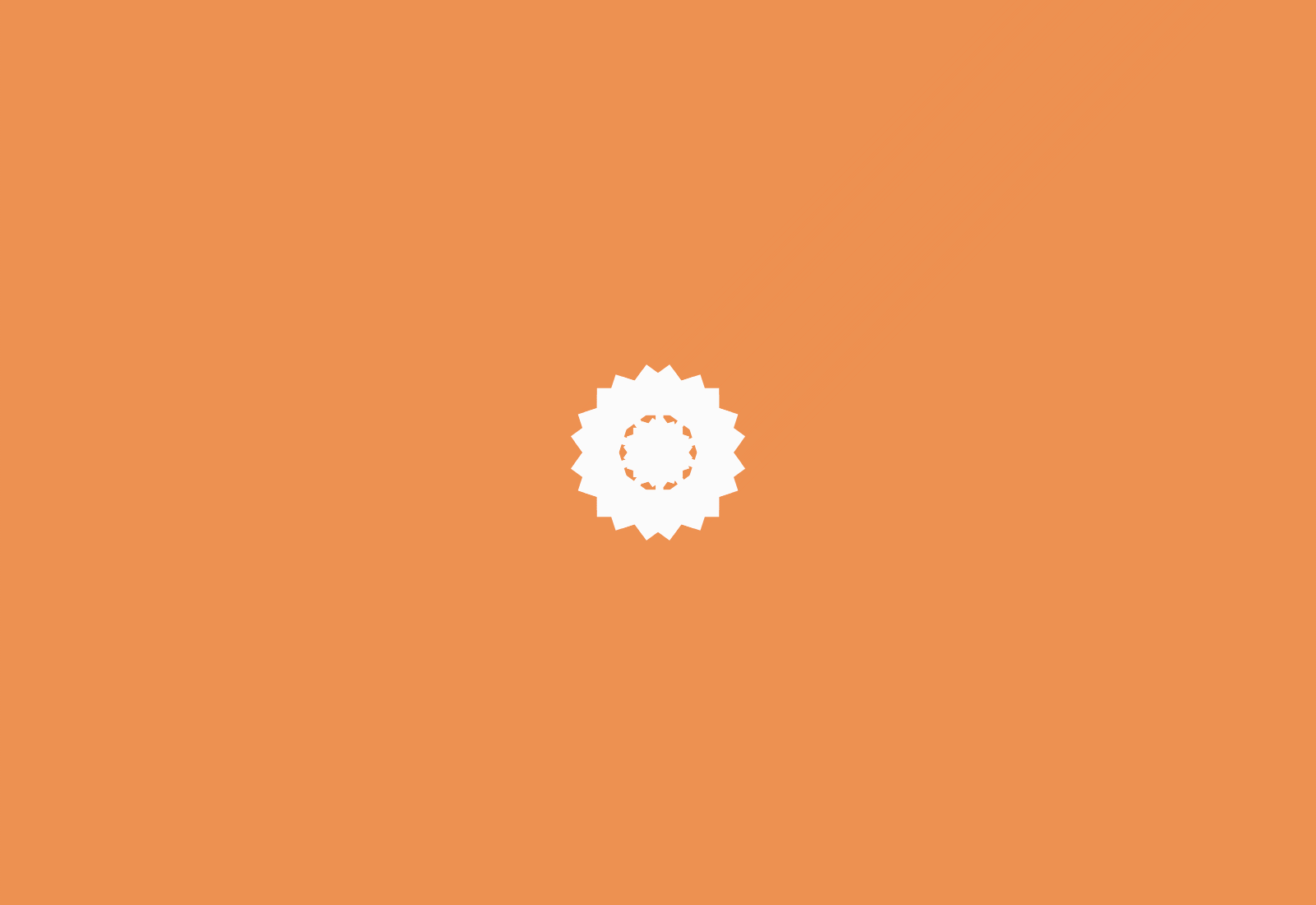
【用unity实现100个游戏之10】复刻经典俄罗斯方块游戏
文章目录 前言开始项目网格生成Block方块脚本俄罗斯方块基类,绘制方块形状移动逻辑限制移动自由下落下落后设置对应风格为不可移动类型检查当前方块是否可以向指定方向移动旋转逻辑消除逻辑游戏结束逻辑怪物生成源码参考完结 前言 当今游戏产业中,经典游…...
Docker容器内数据备份到系统本地
Docker运行容器时没将目录映射出来,或者因docker容器内外数据不一致,导致docker运行错误的,可以使用以下步骤处理: 1.进入要备份的容器: docker exec -it <容器名称或ID> /bin/bash2.在容器内创建一个临时目录…...
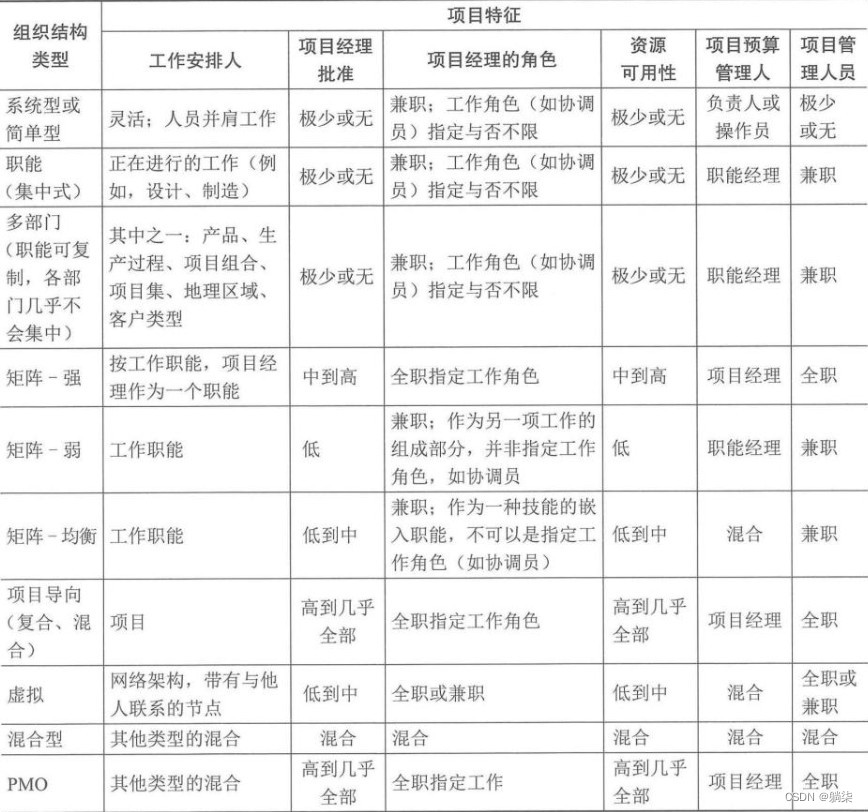
学信息系统项目管理师第4版系列06_项目管理概论
1. 项目基础 1.1. 项目是为创造独特的产品、服务或成果而进行的临时性工作 1.1.1. 独特的产品、服务或成果 1.1.2. 临时性工作 1.1.2.1. 项目有明确的起点和终点 1.1.2.2. 不一定意味着项目的持续时间短 1.1.2.3. 临时性是项目的特点,不是项目目标的特点 1.1…...
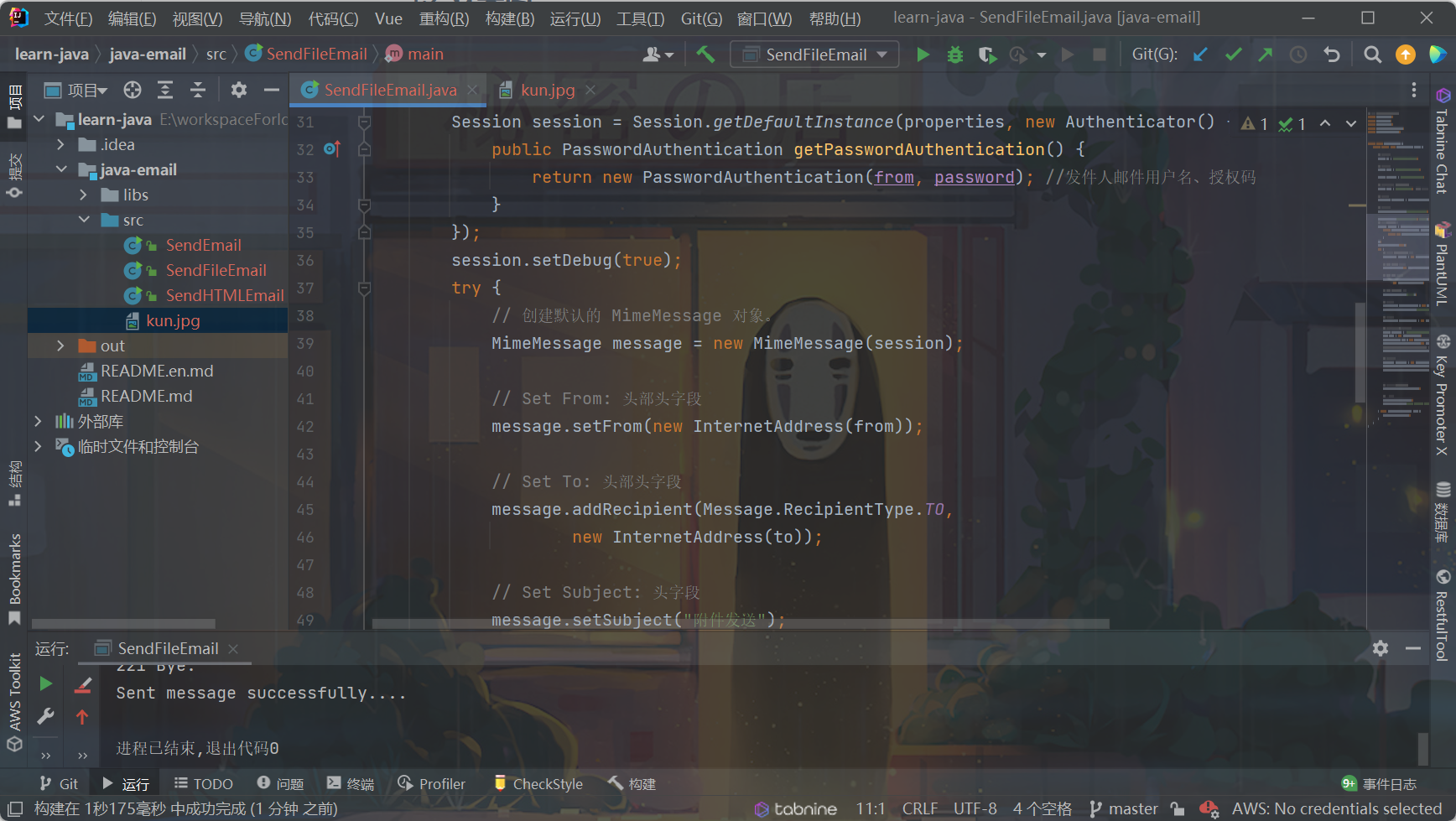
Java发送(QQ)邮箱、验证码发送
前言 使用Java应用程序发送 E-mail 十分简单,但是首先需要在项目中导入 JavaMail API 和Java Activation Framework (JAF) 的jar包。 菜鸟教程提供的下载链接: JavaMail mail.jar 1.4.5JAF(版本 1.1.1) activation.jar 1、准备…...
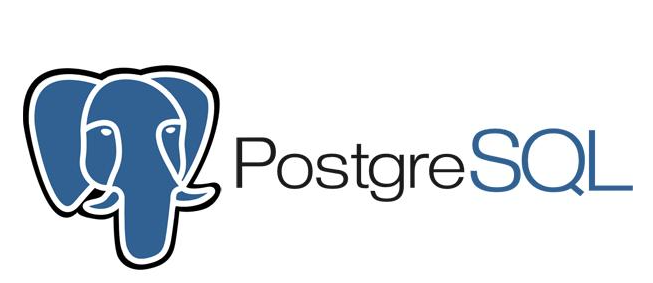
PostgresSQL----基于Kubernetes部署PostgresSQL
【PostgresSQL----基于Kubernetes部署PostgresSQL】 文章目录 一、创建SC、PV和PVC存储对象1.1 准备一个nfs服务器1.2 编写SC、PV、PVC等存储资源文件1.3 编写部署PostgresSQL数据库的资源声明文件 二、部署PostgresSQL2.1 部署 PV、PVC等存储对象2.2 部署PostgresSQL数据库2.3…...
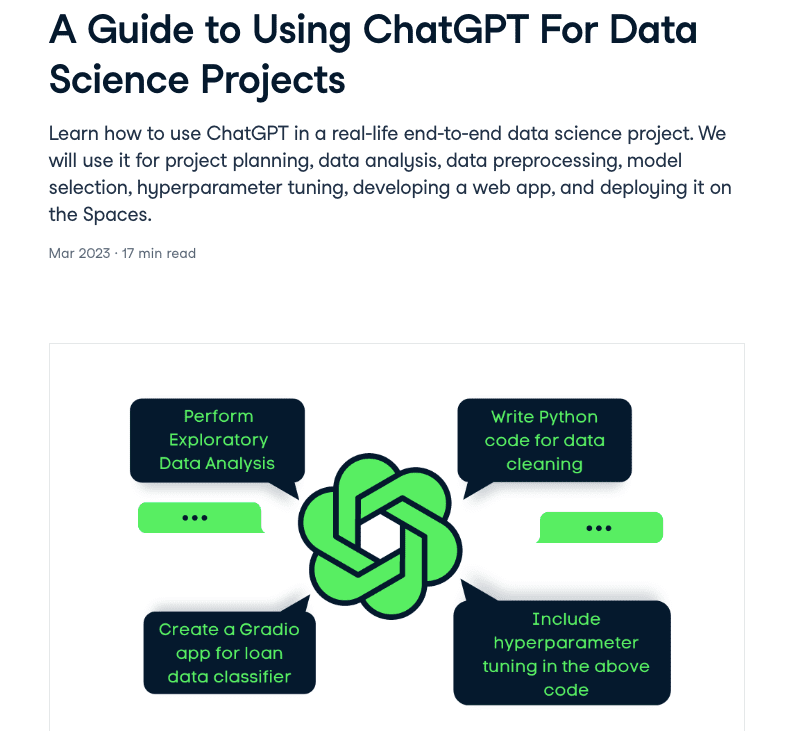
7 个适合初学者的项目,可帮助您开始使用 ChatGPT
推荐:使用 NSDT场景编辑器快速搭建3D应用场景 从自动化日常任务到预测复杂模式,人工智能正在重塑行业并重新定义可能性。 当我们站在这场人工智能革命中时,我们必须了解它的潜力并将其整合到我们的日常工作流程中。 然而。。。我知道开始使…...
JDBC操作SQLite的工具类
直接调用无需拼装sql 注入依赖 <dependency><groupId>org.xerial</groupId><artifactId>sqlite-jdbc</artifactId><version>3.43.0.0</version></dependency>工具类 import org.sqlite.SQLiteConnection;/*** Author cpf* Dat…...
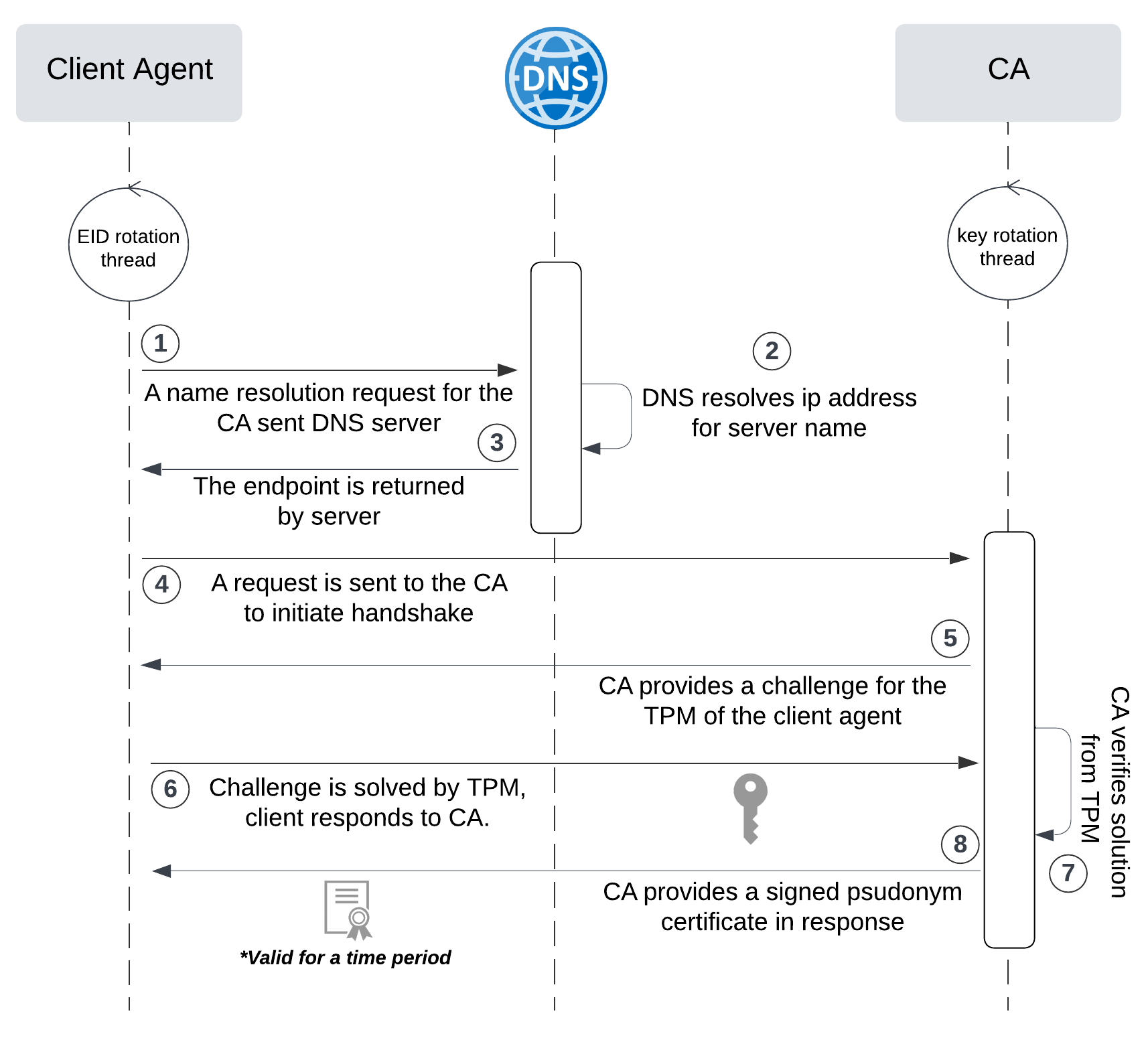
网络六边形受到攻击
大家读完觉得有帮助记得关注和点赞!!! 抽象 现代智能交通系统 (ITS) 的一个关键要求是能够以安全、可靠和匿名的方式从互联车辆和移动设备收集地理参考数据。Nexagon 协议建立在 IETF 定位器/ID 分离协议 (…...

19c补丁后oracle属主变化,导致不能识别磁盘组
补丁后服务器重启,数据库再次无法启动 ORA01017: invalid username/password; logon denied Oracle 19c 在打上 19.23 或以上补丁版本后,存在与用户组权限相关的问题。具体表现为,Oracle 实例的运行用户(oracle)和集…...
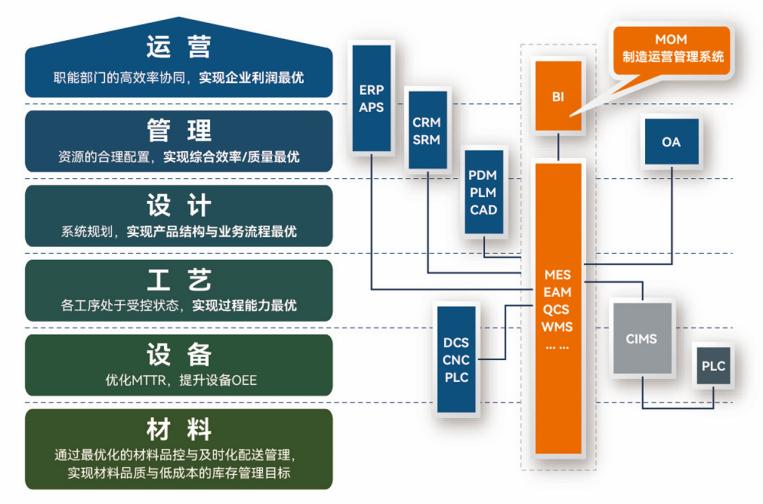
盘古信息PCB行业解决方案:以全域场景重构,激活智造新未来
一、破局:PCB行业的时代之问 在数字经济蓬勃发展的浪潮中,PCB(印制电路板)作为 “电子产品之母”,其重要性愈发凸显。随着 5G、人工智能等新兴技术的加速渗透,PCB行业面临着前所未有的挑战与机遇。产品迭代…...
ssc377d修改flash分区大小
1、flash的分区默认分配16M、 / # df -h Filesystem Size Used Available Use% Mounted on /dev/root 1.9M 1.9M 0 100% / /dev/mtdblock4 3.0M...
MVC 数据库
MVC 数据库 引言 在软件开发领域,Model-View-Controller(MVC)是一种流行的软件架构模式,它将应用程序分为三个核心组件:模型(Model)、视图(View)和控制器(Controller)。这种模式有助于提高代码的可维护性和可扩展性。本文将深入探讨MVC架构与数据库之间的关系,以…...
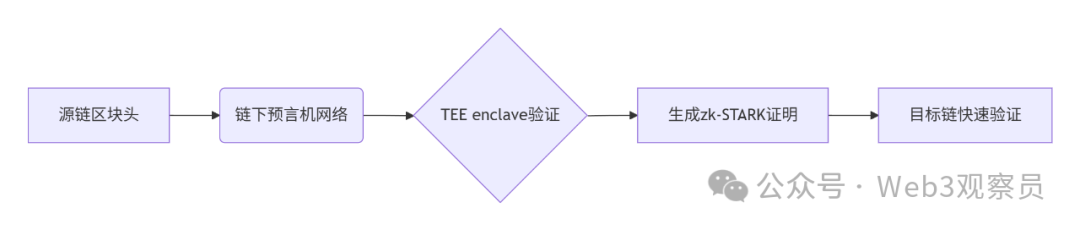
跨链模式:多链互操作架构与性能扩展方案
跨链模式:多链互操作架构与性能扩展方案 ——构建下一代区块链互联网的技术基石 一、跨链架构的核心范式演进 1. 分层协议栈:模块化解耦设计 现代跨链系统采用分层协议栈实现灵活扩展(H2Cross架构): 适配层…...
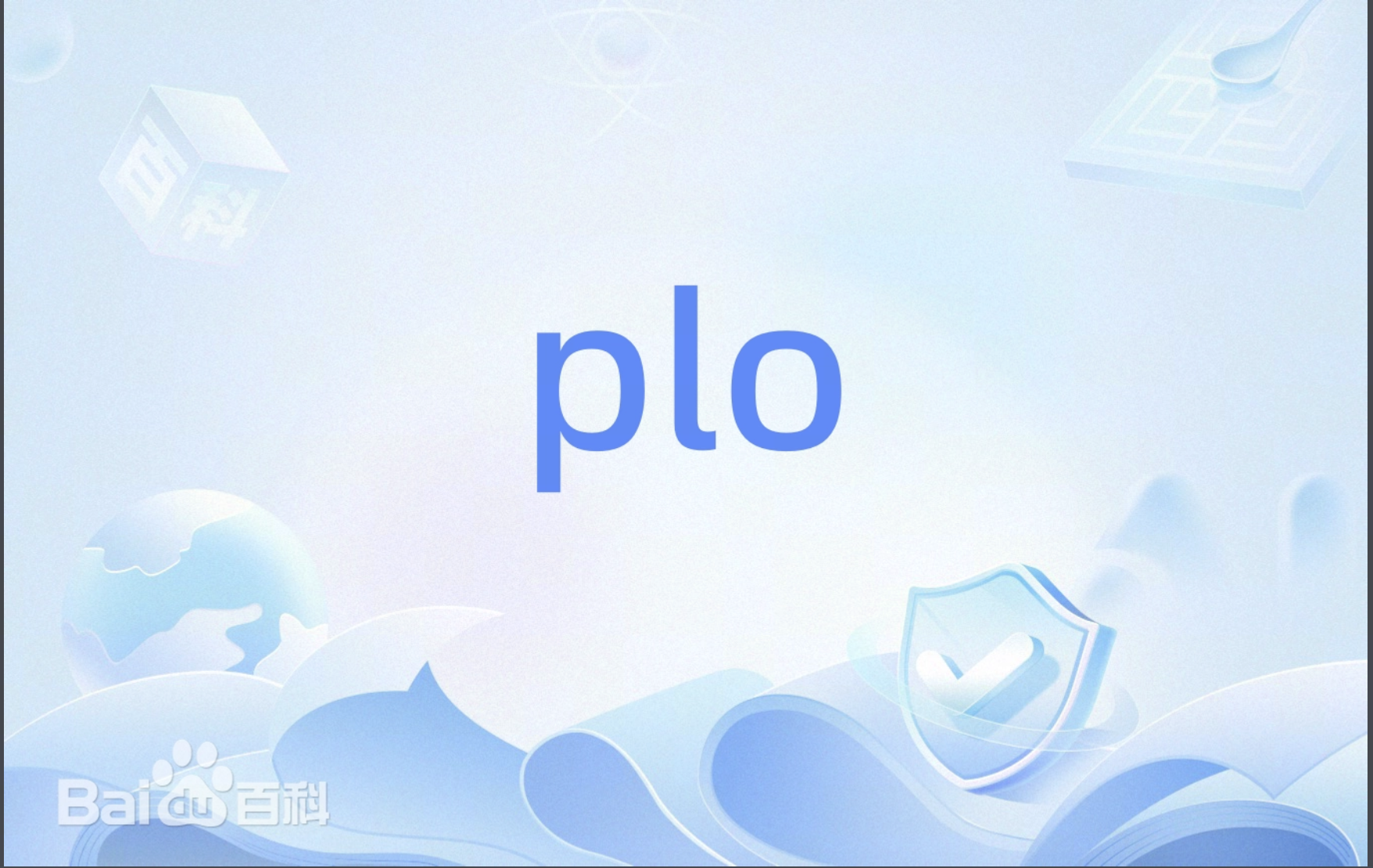
PL0语法,分析器实现!
简介 PL/0 是一种简单的编程语言,通常用于教学编译原理。它的语法结构清晰,功能包括常量定义、变量声明、过程(子程序)定义以及基本的控制结构(如条件语句和循环语句)。 PL/0 语法规范 PL/0 是一种教学用的小型编程语言,由 Niklaus Wirth 设计,用于展示编译原理的核…...
【python异步多线程】异步多线程爬虫代码示例
claude生成的python多线程、异步代码示例,模拟20个网页的爬取,每个网页假设要0.5-2秒完成。 代码 Python多线程爬虫教程 核心概念 多线程:允许程序同时执行多个任务,提高IO密集型任务(如网络请求)的效率…...
【HTTP三个基础问题】
面试官您好!HTTP是超文本传输协议,是互联网上客户端和服务器之间传输超文本数据(比如文字、图片、音频、视频等)的核心协议,当前互联网应用最广泛的版本是HTTP1.1,它基于经典的C/S模型,也就是客…...
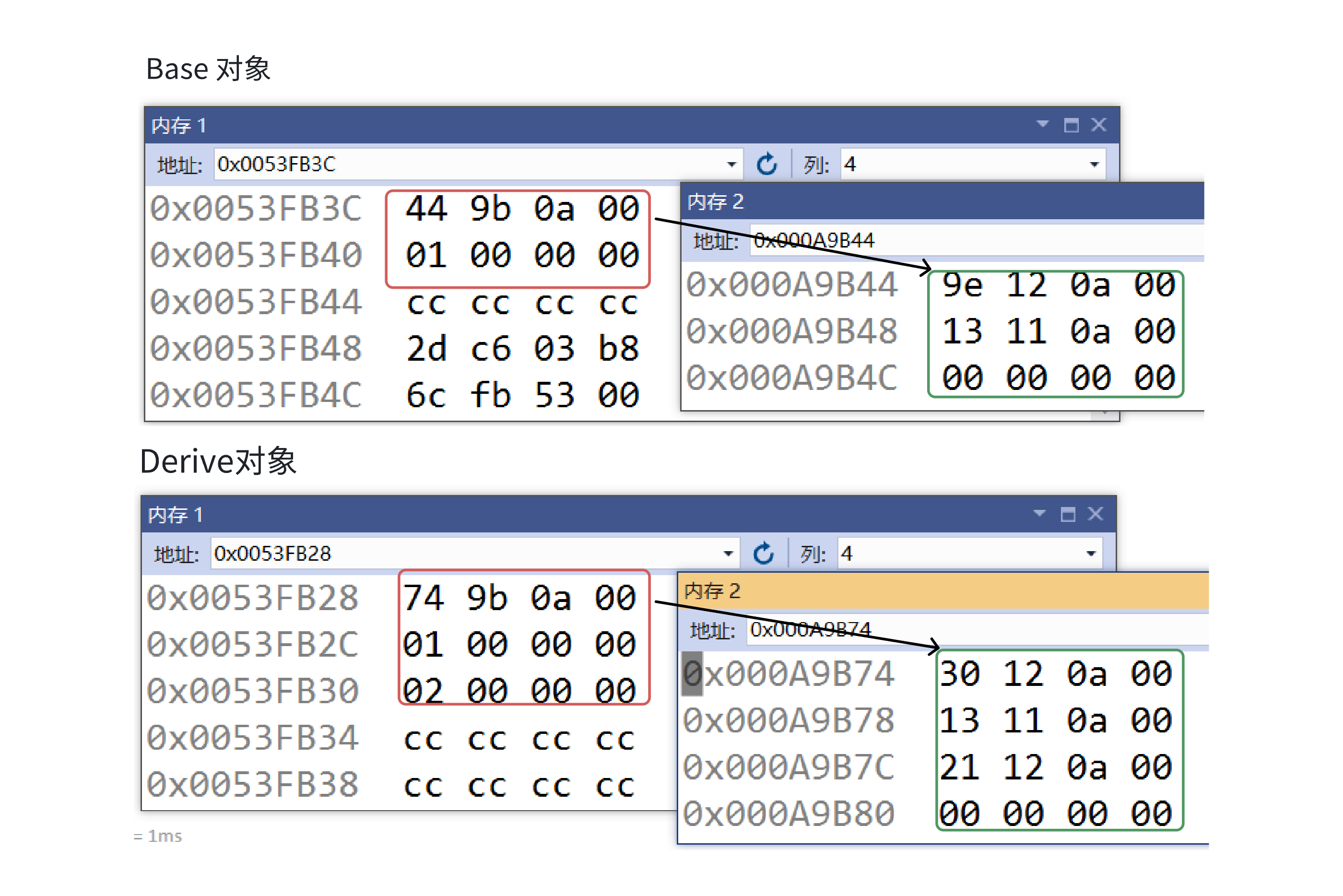
C++:多态机制详解
目录 一. 多态的概念 1.静态多态(编译时多态) 二.动态多态的定义及实现 1.多态的构成条件 2.虚函数 3.虚函数的重写/覆盖 4.虚函数重写的一些其他问题 1).协变 2).析构函数的重写 5.override 和 final关键字 1&#…...