目标检测算法改进系列之Backbone替换为EMO
EMO:结合 Attention 重新思考移动端小模型中的基本模块
近年来,由于存储和计算资源的限制,移动应用的需求不断增加,因此,本文的研究对象是端侧轻量级小模型 (参数量一般在 10M 以下)。在众多小模型的设计中,值得注意的是 MobileNetv2[1] 提出了一种基于 Depth-wise Convolution 的高效的倒残差模块 (Inverted Residual Block, IRB),已成标准的高效轻量级模型的基本模块。此后,很少有设计基于CNN 的端侧轻量级小模型的新思想被引入,也很少有新的轻量级模块跳出 IRB 范式并部分取代它。
近年来,视觉 Transformer 由于其动态建模和对于超大数据集的泛化能力,已经成功地应用在了各种计算机视觉任务中。但是,Transformer 中的 Multi-Head Self-Attention (MHSA) 模块,受限于参数和计算量的对于输入分辨率的平方复杂度,往往会消耗大量的资源,不适合移动端侧的应用。所以说研究人员最近开始结合 Transformer 与 CNN 模型设计高效的混合模型,并在精度,参数量和 FLOPs 方面获得了比基于 CNN 的模型更好的性能,代表性的工作有:MobileViT[2],MobileViT V2[3],和 MobileViT V3[4]。但是,这些方案往往引入复杂的结构或者混合模块,这对具体应用的优化非常不利。
所以本文作者简单地结合了 IRB 和 Transformer 的设计思路,希望结合 Attention 重新思考移动端小模型中的基本模块。如下图1所示是本文模型 Efficient MOdel (EMO) 与其他端侧轻量级小模型的精度,FLOPs 和 Params 对比。EMO 实现了新的 SoTA,超越了 MViT,EdgeViT 等模型。
原文地址:Rethinking Mobile Block for Efficient Attention-based Models
EMO代码实现
import math
import numpy as np
import torch.nn as nn
from einops import rearrange, reduce
from timm.models.layers.activations import *
from timm.models.layers import DropPath, trunc_normal_, create_attn
from timm.models.efficientnet_blocks import num_groups, SqueezeExcite as SE
from functools import partial__all__ = ['EMO_1M', 'EMO_2M', 'EMO_5M', 'EMO_6M']inplace = Truedef get_act(act_layer='relu'):act_dict = {'none': nn.Identity,'sigmoid': Sigmoid,'swish': Swish,'mish': Mish,'hsigmoid': HardSigmoid,'hswish': HardSwish,'hmish': HardMish,'tanh': Tanh,'relu': nn.ReLU,'relu6': nn.ReLU6,'prelu': PReLU,'gelu': GELU,'silu': nn.SiLU}return act_dict[act_layer]class LayerNorm2d(nn.Module):def __init__(self, normalized_shape, eps=1e-6, elementwise_affine=True):super().__init__()self.norm = nn.LayerNorm(normalized_shape, eps, elementwise_affine)def forward(self, x):x = rearrange(x, 'b c h w -> b h w c').contiguous()x = self.norm(x)x = rearrange(x, 'b h w c -> b c h w').contiguous()return xdef get_norm(norm_layer='in_1d'):eps = 1e-6norm_dict = {'none': nn.Identity,'in_1d': partial(nn.InstanceNorm1d, eps=eps),'in_2d': partial(nn.InstanceNorm2d, eps=eps),'in_3d': partial(nn.InstanceNorm3d, eps=eps),'bn_1d': partial(nn.BatchNorm1d, eps=eps),'bn_2d': partial(nn.BatchNorm2d, eps=eps),'bn_3d': partial(nn.BatchNorm3d, eps=eps),'gn': partial(nn.GroupNorm, eps=eps),'ln_1d': partial(nn.LayerNorm, eps=eps),'ln_2d': partial(LayerNorm2d, eps=eps),}return norm_dict[norm_layer]class ConvNormAct(nn.Module):def __init__(self, dim_in, dim_out, kernel_size, stride=1, dilation=1, groups=1, bias=False,skip=False, norm_layer='bn_2d', act_layer='relu', inplace=True, drop_path_rate=0.):super(ConvNormAct, self).__init__()self.has_skip = skip and dim_in == dim_outpadding = math.ceil((kernel_size - stride) / 2)self.conv = nn.Conv2d(dim_in, dim_out, kernel_size, stride, padding, dilation, groups, bias)self.norm = get_norm(norm_layer)(dim_out)self.act = get_act(act_layer)(inplace=inplace)self.drop_path = DropPath(drop_path_rate) if drop_path_rate else nn.Identity()def forward(self, x):shortcut = xx = self.conv(x)x = self.norm(x)x = self.act(x)if self.has_skip:x = self.drop_path(x) + shortcutreturn xinplace = True# ========== Multi-Scale Populations, for down-sampling and inductive bias ==========
class MSPatchEmb(nn.Module):def __init__(self, dim_in, emb_dim, kernel_size=2, c_group=-1, stride=1, dilations=[1, 2, 3],norm_layer='bn_2d', act_layer='silu'):super().__init__()self.dilation_num = len(dilations)assert dim_in % c_group == 0c_group = math.gcd(dim_in, emb_dim) if c_group == -1 else c_groupself.convs = nn.ModuleList()for i in range(len(dilations)):padding = math.ceil(((kernel_size - 1) * dilations[i] + 1 - stride) / 2)self.convs.append(nn.Sequential(nn.Conv2d(dim_in, emb_dim, kernel_size, stride, padding, dilations[i], groups=c_group),get_norm(norm_layer)(emb_dim),get_act(act_layer)(emb_dim)))def forward(self, x):if self.dilation_num == 1:x = self.convs[0](x)else:x = torch.cat([self.convs[i](x).unsqueeze(dim=-1) for i in range(self.dilation_num)], dim=-1)x = reduce(x, 'b c h w n -> b c h w', 'mean').contiguous()return xclass iRMB(nn.Module):def __init__(self, dim_in, dim_out, norm_in=True, has_skip=True, exp_ratio=1.0, norm_layer='bn_2d',act_layer='relu', v_proj=True, dw_ks=3, stride=1, dilation=1, se_ratio=0.0, dim_head=64, window_size=7,attn_s=True, qkv_bias=False, attn_drop=0., drop=0., drop_path=0., v_group=False, attn_pre=False):super().__init__()self.norm = get_norm(norm_layer)(dim_in) if norm_in else nn.Identity()dim_mid = int(dim_in * exp_ratio)self.has_skip = (dim_in == dim_out and stride == 1) and has_skipself.attn_s = attn_sif self.attn_s:assert dim_in % dim_head == 0, 'dim should be divisible by num_heads'self.dim_head = dim_headself.window_size = window_sizeself.num_head = dim_in // dim_headself.scale = self.dim_head ** -0.5self.attn_pre = attn_preself.qk = ConvNormAct(dim_in, int(dim_in * 2), kernel_size=1, bias=qkv_bias, norm_layer='none', act_layer='none')self.v = ConvNormAct(dim_in, dim_mid, kernel_size=1, groups=self.num_head if v_group else 1, bias=qkv_bias, norm_layer='none', act_layer=act_layer, inplace=inplace)self.attn_drop = nn.Dropout(attn_drop)else:if v_proj:self.v = ConvNormAct(dim_in, dim_mid, kernel_size=1, bias=qkv_bias, norm_layer='none', act_layer=act_layer, inplace=inplace)else:self.v = nn.Identity()self.conv_local = ConvNormAct(dim_mid, dim_mid, kernel_size=dw_ks, stride=stride, dilation=dilation, groups=dim_mid, norm_layer='bn_2d', act_layer='silu', inplace=inplace)self.se = SE(dim_mid, rd_ratio=se_ratio, act_layer=get_act(act_layer)) if se_ratio > 0.0 else nn.Identity()self.proj_drop = nn.Dropout(drop)self.proj = ConvNormAct(dim_mid, dim_out, kernel_size=1, norm_layer='none', act_layer='none', inplace=inplace)self.drop_path = DropPath(drop_path) if drop_path else nn.Identity()def forward(self, x):shortcut = xx = self.norm(x)B, C, H, W = x.shapeif self.attn_s:# paddingif self.window_size <= 0:window_size_W, window_size_H = W, Helse:window_size_W, window_size_H = self.window_size, self.window_sizepad_l, pad_t = 0, 0pad_r = (window_size_W - W % window_size_W) % window_size_Wpad_b = (window_size_H - H % window_size_H) % window_size_Hx = F.pad(x, (pad_l, pad_r, pad_t, pad_b, 0, 0,))n1, n2 = (H + pad_b) // window_size_H, (W + pad_r) // window_size_Wx = rearrange(x, 'b c (h1 n1) (w1 n2) -> (b n1 n2) c h1 w1', n1=n1, n2=n2).contiguous()# attentionb, c, h, w = x.shapeqk = self.qk(x)qk = rearrange(qk, 'b (qk heads dim_head) h w -> qk b heads (h w) dim_head', qk=2, heads=self.num_head, dim_head=self.dim_head).contiguous()q, k = qk[0], qk[1]attn_spa = (q @ k.transpose(-2, -1)) * self.scaleattn_spa = attn_spa.softmax(dim=-1)attn_spa = self.attn_drop(attn_spa)if self.attn_pre:x = rearrange(x, 'b (heads dim_head) h w -> b heads (h w) dim_head', heads=self.num_head).contiguous()x_spa = attn_spa @ xx_spa = rearrange(x_spa, 'b heads (h w) dim_head -> b (heads dim_head) h w', heads=self.num_head, h=h, w=w).contiguous()x_spa = self.v(x_spa)else:v = self.v(x)v = rearrange(v, 'b (heads dim_head) h w -> b heads (h w) dim_head', heads=self.num_head).contiguous()x_spa = attn_spa @ vx_spa = rearrange(x_spa, 'b heads (h w) dim_head -> b (heads dim_head) h w', heads=self.num_head, h=h, w=w).contiguous()# unpaddingx = rearrange(x_spa, '(b n1 n2) c h1 w1 -> b c (h1 n1) (w1 n2)', n1=n1, n2=n2).contiguous()if pad_r > 0 or pad_b > 0:x = x[:, :, :H, :W].contiguous()else:x = self.v(x)x = x + self.se(self.conv_local(x)) if self.has_skip else self.se(self.conv_local(x))x = self.proj_drop(x)x = self.proj(x)x = (shortcut + self.drop_path(x)) if self.has_skip else xreturn xclass EMO(nn.Module):def __init__(self, dim_in=3, num_classes=1000, img_size=224,depths=[1, 2, 4, 2], stem_dim=16, embed_dims=[64, 128, 256, 512], exp_ratios=[4., 4., 4., 4.],norm_layers=['bn_2d', 'bn_2d', 'bn_2d', 'bn_2d'], act_layers=['relu', 'relu', 'relu', 'relu'],dw_kss=[3, 3, 5, 5], se_ratios=[0.0, 0.0, 0.0, 0.0], dim_heads=[32, 32, 32, 32],window_sizes=[7, 7, 7, 7], attn_ss=[False, False, True, True], qkv_bias=True,attn_drop=0., drop=0., drop_path=0., v_group=False, attn_pre=False, pre_dim=0):super().__init__()self.num_classes = num_classesassert num_classes > 0dprs = [x.item() for x in torch.linspace(0, drop_path, sum(depths))]self.stage0 = nn.ModuleList([MSPatchEmb( # down to 112dim_in, stem_dim, kernel_size=dw_kss[0], c_group=1, stride=2, dilations=[1],norm_layer=norm_layers[0], act_layer='none'),iRMB( # dsstem_dim, stem_dim, norm_in=False, has_skip=False, exp_ratio=1,norm_layer=norm_layers[0], act_layer=act_layers[0], v_proj=False, dw_ks=dw_kss[0],stride=1, dilation=1, se_ratio=1,dim_head=dim_heads[0], window_size=window_sizes[0], attn_s=False,qkv_bias=qkv_bias, attn_drop=attn_drop, drop=drop, drop_path=0.,attn_pre=attn_pre)])emb_dim_pre = stem_dimfor i in range(len(depths)):layers = []dpr = dprs[sum(depths[:i]):sum(depths[:i + 1])]for j in range(depths[i]):if j == 0:stride, has_skip, attn_s, exp_ratio = 2, False, False, exp_ratios[i] * 2else:stride, has_skip, attn_s, exp_ratio = 1, True, attn_ss[i], exp_ratios[i]layers.append(iRMB(emb_dim_pre, embed_dims[i], norm_in=True, has_skip=has_skip, exp_ratio=exp_ratio,norm_layer=norm_layers[i], act_layer=act_layers[i], v_proj=True, dw_ks=dw_kss[i],stride=stride, dilation=1, se_ratio=se_ratios[i],dim_head=dim_heads[i], window_size=window_sizes[i], attn_s=attn_s,qkv_bias=qkv_bias, attn_drop=attn_drop, drop=drop, drop_path=dpr[j], v_group=v_group,attn_pre=attn_pre))emb_dim_pre = embed_dims[i]self.__setattr__(f'stage{i + 1}', nn.ModuleList(layers))self.norm = get_norm(norm_layers[-1])(embed_dims[-1])self.apply(self._init_weights)self.channel = [i.size(1) for i in self.forward(torch.randn(1, 3, 640, 640))]def _init_weights(self, m):if isinstance(m, nn.Linear):trunc_normal_(m.weight, std=.02)if m.bias is not None:nn.init.zeros_(m.bias)elif isinstance(m, (nn.LayerNorm, nn.GroupNorm,nn.BatchNorm1d, nn.BatchNorm2d, nn.BatchNorm3d,nn.InstanceNorm1d, nn.InstanceNorm2d, nn.InstanceNorm3d)):nn.init.zeros_(m.bias)nn.init.ones_(m.weight)@torch.jit.ignoredef no_weight_decay(self):return {'token'}@torch.jit.ignoredef no_weight_decay_keywords(self):return {'alpha', 'gamma', 'beta'}@torch.jit.ignoredef no_ft_keywords(self):# return {'head.weight', 'head.bias'}return {}@torch.jit.ignoredef ft_head_keywords(self):return {'head.weight', 'head.bias'}, self.num_classesdef get_classifier(self):return self.headdef reset_classifier(self, num_classes):self.num_classes = num_classesself.head = nn.Linear(self.pre_dim, num_classes) if num_classes > 0 else nn.Identity()def check_bn(self):for name, m in self.named_modules():if isinstance(m, nn.modules.batchnorm._NormBase):m.running_mean = torch.nan_to_num(m.running_mean, nan=0, posinf=1, neginf=-1)m.running_var = torch.nan_to_num(m.running_var, nan=0, posinf=1, neginf=-1)def forward_features(self, x):for blk in self.stage0:x = blk(x)x1 = xfor blk in self.stage1:x = blk(x)x2 = xfor blk in self.stage2:x = blk(x)x3 = xfor blk in self.stage3:x = blk(x)x4 = xfor blk in self.stage4:x = blk(x)x5 = xreturn [x1, x2, x3, x4, x5]def forward(self, x):x = self.forward_features(x)x[-1] = self.norm(x[-1])return xdef update_weight(model_dict, weight_dict):idx, temp_dict = 0, {}for k, v in weight_dict.items():if k in model_dict.keys() and np.shape(model_dict[k]) == np.shape(v):temp_dict[k] = vidx += 1model_dict.update(temp_dict)print(f'loading weights... {idx}/{len(model_dict)} items')return model_dictdef EMO_1M(weights='', **kwargs):model = EMO(# dim_in=3, num_classes=1000, img_size=224,depths=[2, 2, 8, 3], stem_dim=24, embed_dims=[32, 48, 80, 168], exp_ratios=[2., 2.5, 3.0, 3.5],norm_layers=['bn_2d', 'bn_2d', 'ln_2d', 'ln_2d'], act_layers=['silu', 'silu', 'gelu', 'gelu'],dw_kss=[3, 3, 5, 5], dim_heads=[16, 16, 20, 21], window_sizes=[7, 7, 7, 7], attn_ss=[False, False, True, True],qkv_bias=True, attn_drop=0., drop=0., drop_path=0.04036, v_group=False, attn_pre=True, pre_dim=0,**kwargs)if weights:pretrained_weight = torch.load(weights)model.load_state_dict(update_weight(model.state_dict(), pretrained_weight))return modeldef EMO_2M(weights='', **kwargs):model = EMO(# dim_in=3, num_classes=1000, img_size=224,depths=[3, 3, 9, 3], stem_dim=24, embed_dims=[32, 48, 120, 200], exp_ratios=[2., 2.5, 3.0, 3.5],norm_layers=['bn_2d', 'bn_2d', 'ln_2d', 'ln_2d'], act_layers=['silu', 'silu', 'gelu', 'gelu'],dw_kss=[3, 3, 5, 5], dim_heads=[16, 16, 20, 20], window_sizes=[7, 7, 7, 7], attn_ss=[False, False, True, True],qkv_bias=True, attn_drop=0., drop=0., drop_path=0.05, v_group=False, attn_pre=True, pre_dim=0,**kwargs)if weights:pretrained_weight = torch.load(weights)model.load_state_dict(update_weight(model.state_dict(), pretrained_weight))return modeldef EMO_5M(weights='', **kwargs):model = EMO(# dim_in=3, num_classes=1000, img_size=224,depths=[3, 3, 9, 3], stem_dim=24, embed_dims=[48, 72, 160, 288], exp_ratios=[2., 3., 4., 4.],norm_layers=['bn_2d', 'bn_2d', 'ln_2d', 'ln_2d'], act_layers=['silu', 'silu', 'gelu', 'gelu'],dw_kss=[3, 3, 5, 5], dim_heads=[24, 24, 32, 32], window_sizes=[7, 7, 7, 7], attn_ss=[False, False, True, True],qkv_bias=True, attn_drop=0., drop=0., drop_path=0.05, v_group=False, attn_pre=True, pre_dim=0,**kwargs)if weights:pretrained_weight = torch.load(weights)model.load_state_dict(update_weight(model.state_dict(), pretrained_weight))return modeldef EMO_6M(weights='', **kwargs):model = EMO(# dim_in=3, num_classes=1000, img_size=224,depths=[3, 3, 9, 3], stem_dim=24, embed_dims=[48, 72, 160, 320], exp_ratios=[2., 3., 4., 5.],norm_layers=['bn_2d', 'bn_2d', 'ln_2d', 'ln_2d'], act_layers=['silu', 'silu', 'gelu', 'gelu'],dw_kss=[3, 3, 5, 5], dim_heads=[16, 24, 20, 32], window_sizes=[7, 7, 7, 7], attn_ss=[False, False, True, True],qkv_bias=True, attn_drop=0., drop=0., drop_path=0.05, v_group=False, attn_pre=True, pre_dim=0,**kwargs)if weights:pretrained_weight = torch.load(weights)model.load_state_dict(update_weight(model.state_dict(), pretrained_weight))return modelif __name__ == '__main__':model = EMO_1M('EMO_1M/net.pth')model = EMO_2M('EMO_2M/net.pth')model = EMO_5M('EMO_5M/net.pth')model = EMO_6M('EMO_6M/net.pth')
Backbone替换
yolo.py修改
def parse_model函数
def parse_model(d, ch): # model_dict, input_channels(3)# Parse a YOLOv5 model.yaml dictionaryLOGGER.info(f"\n{'':>3}{'from':>18}{'n':>3}{'params':>10} {'module':<40}{'arguments':<30}")anchors, nc, gd, gw, act = d['anchors'], d['nc'], d['depth_multiple'], d['width_multiple'], d.get('activation')if act:Conv.default_act = eval(act) # redefine default activation, i.e. Conv.default_act = nn.SiLU()LOGGER.info(f"{colorstr('activation:')} {act}") # printna = (len(anchors[0]) // 2) if isinstance(anchors, list) else anchors # number of anchorsno = na * (nc + 5) # number of outputs = anchors * (classes + 5)is_backbone = Falselayers, save, c2 = [], [], ch[-1] # layers, savelist, ch outfor i, (f, n, m, args) in enumerate(d['backbone'] + d['head']): # from, number, module, argstry:t = mm = eval(m) if isinstance(m, str) else m # eval stringsexcept:passfor j, a in enumerate(args):with contextlib.suppress(NameError):try:args[j] = eval(a) if isinstance(a, str) else a # eval stringsexcept:args[j] = an = n_ = max(round(n * gd), 1) if n > 1 else n # depth gainif m in {Conv, GhostConv, Bottleneck, GhostBottleneck, SPP, SPPF, DWConv, MixConv2d, Focus, CrossConv,BottleneckCSP, C3, C3TR, C3SPP, C3Ghost, nn.ConvTranspose2d, DWConvTranspose2d, C3x}:c1, c2 = ch[f], args[0]if c2 != no: # if not outputc2 = make_divisible(c2 * gw, 8)args = [c1, c2, *args[1:]]if m in {BottleneckCSP, C3, C3TR, C3Ghost, C3x}:args.insert(2, n) # number of repeatsn = 1elif m is nn.BatchNorm2d:args = [ch[f]]elif m is Concat:c2 = sum(ch[x] for x in f)# TODO: channel, gw, gdelif m in {Detect, Segment}:args.append([ch[x] for x in f])if isinstance(args[1], int): # number of anchorsargs[1] = [list(range(args[1] * 2))] * len(f)if m is Segment:args[3] = make_divisible(args[3] * gw, 8)elif m is Contract:c2 = ch[f] * args[0] ** 2elif m is Expand:c2 = ch[f] // args[0] ** 2elif isinstance(m, str):t = mm = timm.create_model(m, pretrained=args[0], features_only=True)c2 = m.feature_info.channels()elif m in {EMO_1M'}: #可添加更多Backbonem = m(*args)c2 = m.channelelse:c2 = ch[f]if isinstance(c2, list):is_backbone = Truem_ = mm_.backbone = Trueelse:m_ = nn.Sequential(*(m(*args) for _ in range(n))) if n > 1 else m(*args) # modulet = str(m)[8:-2].replace('__main__.', '') # module typenp = sum(x.numel() for x in m_.parameters()) # number paramsm_.i, m_.f, m_.type, m_.np = i + 4 if is_backbone else i, f, t, np # attach index, 'from' index, type, number paramsLOGGER.info(f'{i:>3}{str(f):>18}{n_:>3}{np:10.0f} {t:<40}{str(args):<30}') # printsave.extend(x % (i + 4 if is_backbone else i) for x in ([f] if isinstance(f, int) else f) if x != -1) # append to savelistlayers.append(m_)if i == 0:ch = []if isinstance(c2, list):ch.extend(c2)for _ in range(5 - len(ch)):ch.insert(0, 0)else:ch.append(c2)return nn.Sequential(*layers), sorted(save)
def _forward_once函数
def _forward_once(self, x, profile=False, visualize=False):y, dt = [], [] # outputsfor m in self.model:if m.f != -1: # if not from previous layerx = y[m.f] if isinstance(m.f, int) else [x if j == -1 else y[j] for j in m.f] # from earlier layersif profile:self._profile_one_layer(m, x, dt)if hasattr(m, 'backbone'):x = m(x)for _ in range(5 - len(x)):x.insert(0, None)for i_idx, i in enumerate(x):if i_idx in self.save:y.append(i)else:y.append(None)x = x[-1]else:x = m(x) # runy.append(x if m.i in self.save else None) # save outputif visualize:feature_visualization(x, m.type, m.i, save_dir=visualize)return x
yaml配置文件
# YOLOv5 🚀 by Ultralytics, GPL-3.0 license# Parameters
nc: 80 # number of classes
depth_multiple: 0.33 # model depth multiple
width_multiple: 0.25 # layer channel multiple
anchors:- [10,13, 16,30, 33,23] # P3/8- [30,61, 62,45, 59,119] # P4/16- [116,90, 156,198, 373,326] # P5/32# 0-P1/2
# 1-P2/4
# 2-P3/8
# 3-P4/16
# 4-P5/32# YOLOv5 v6.0 backbone
backbone:# [from, number, module, args][[-1, 1, EMO_1M, [False]], # 4[-1, 1, SPPF, [1024, 5]], # 5]# YOLOv5 v6.0 head
head:[[-1, 1, Conv, [512, 1, 1]], # 6[-1, 1, nn.Upsample, [None, 2, 'nearest']], # 7[[-1, 3], 1, Concat, [1]], # cat backbone P4 8[-1, 3, C3, [512, False]], # 9[-1, 1, Conv, [256, 1, 1]], # 10[-1, 1, nn.Upsample, [None, 2, 'nearest']], # 11[[-1, 2], 1, Concat, [1]], # cat backbone P3 12[-1, 3, C3, [256, False]], # 13 (P3/8-small)[-1, 1, Conv, [256, 3, 2]], # 14[[-1, 10], 1, Concat, [1]], # cat head P4 15[-1, 3, C3, [512, False]], # 16 (P4/16-medium)[-1, 1, Conv, [512, 3, 2]], # 17[[-1, 5], 1, Concat, [1]], # cat head P5 18[-1, 3, C3, [1024, False]], # 19 (P5/32-large)[[13, 16, 19], 1, Detect, [nc, anchors]], # Detect(P3, P4, P5)]
相关文章:
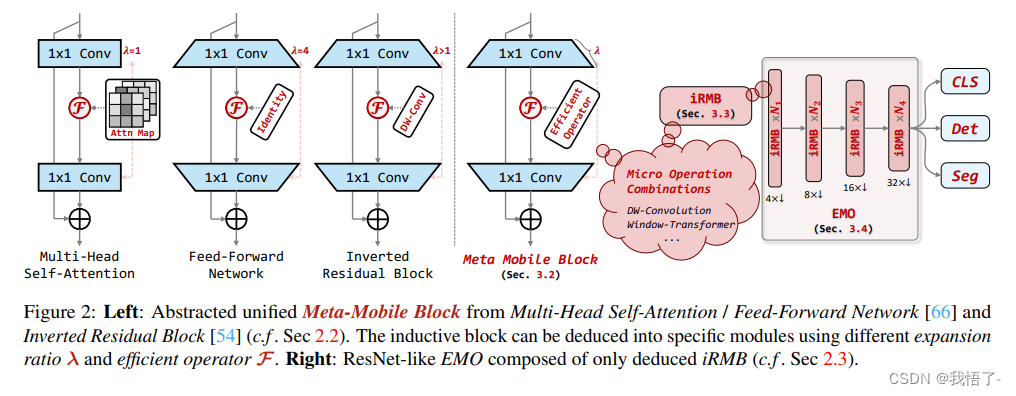
目标检测算法改进系列之Backbone替换为EMO
EMO:结合 Attention 重新思考移动端小模型中的基本模块 近年来,由于存储和计算资源的限制,移动应用的需求不断增加,因此,本文的研究对象是端侧轻量级小模型 (参数量一般在 10M 以下)。在众多小模型的设计中࿰…...

Laravel一些优雅的写法
1. 新增操作 // 原则,所有服务类只有一个public入口,或者多个public入口,但是他们做都是同一件事情 Class CreateService {// 创建类的入口, 根据dto去新建public function create(Dto $dto){// 先构建model对象, 不要在事务期间构建,减少事务…...
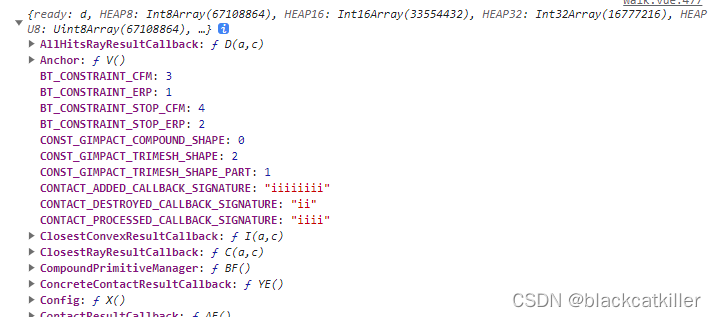
vue+three.js中使用Ammo.js
直接通过npm i ammo.js安装进webpack的项目里调用时,会出现如下报错: ERROR in ./node_modules/ammo.js/ammo.js 1:1683-1696 Mo…...
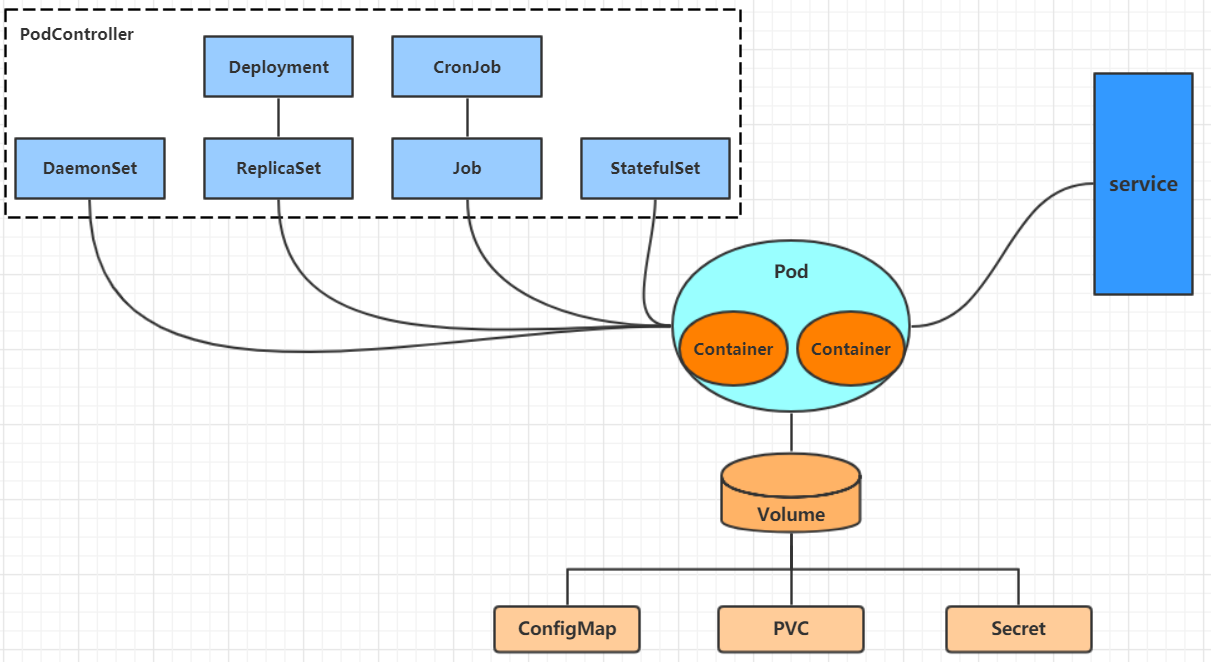
【k8s】kubectl命令详解
文章目录 命令行工具 kubectl在slave下配置kubectl资源操作创建对象 API概述类型访问控制认证授权 废弃API说明 资源管理资源管理介绍资源管理方式命令式对象管理命令式对象配置创建yaml文件创建资源查看资源删除资源 声明式对象配置 kebectl在node节点上运行查看每种资源的可配…...
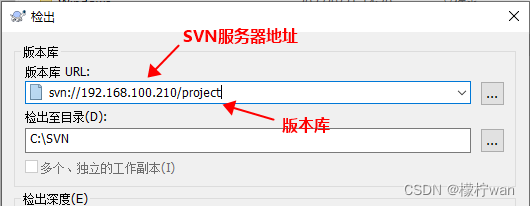
Centos 7 部署SVN服务器
一、安装SVN 1、安装Subversion sudo yum -y install subversion2、验证是否安装成功(查看svn版本号) svnserve --version二、创建版本库 1、先建立目录,目录位置可修改 mkdir -p /var/svn cd /var/svn2、创建版本库,添加权限…...
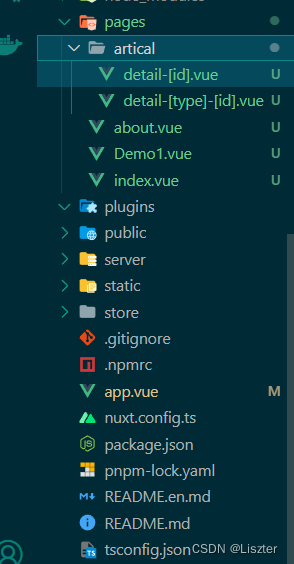
SEO方案尝试--Nuxtjs项目基础配置
Nuxtjs 最新版 Nuxt3 项目配置 安装nuxtjs 最新版 Nuxt3 参考官网安装安装插件安装ElementPlus页面怎么跳转,路由怎么实现404页面该怎么配置配置 网页的title 安装nuxtjs 最新版 Nuxt3 参考官网安装 安装插件 安装ElementPlus 安装 Element Plus 和图标库 # 首先&…...
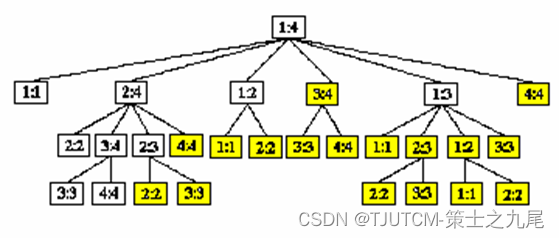
【算法分析与设计】动态规划(上)
目录 一、学习要点二、算法总体思想三、动态规划基本步骤四、矩阵连乘问题4.1 完全加括号的矩阵连乘积4.2 穷举法4.3 动态规划4.3.1 分析最优解的结构4.3.2 建立递归关系4.3.3 计算最优值4.3.4 用动态规划法求最优解 五、动态规划算法的基本要素5.1 最优子结构5.2 重叠子问题5.…...
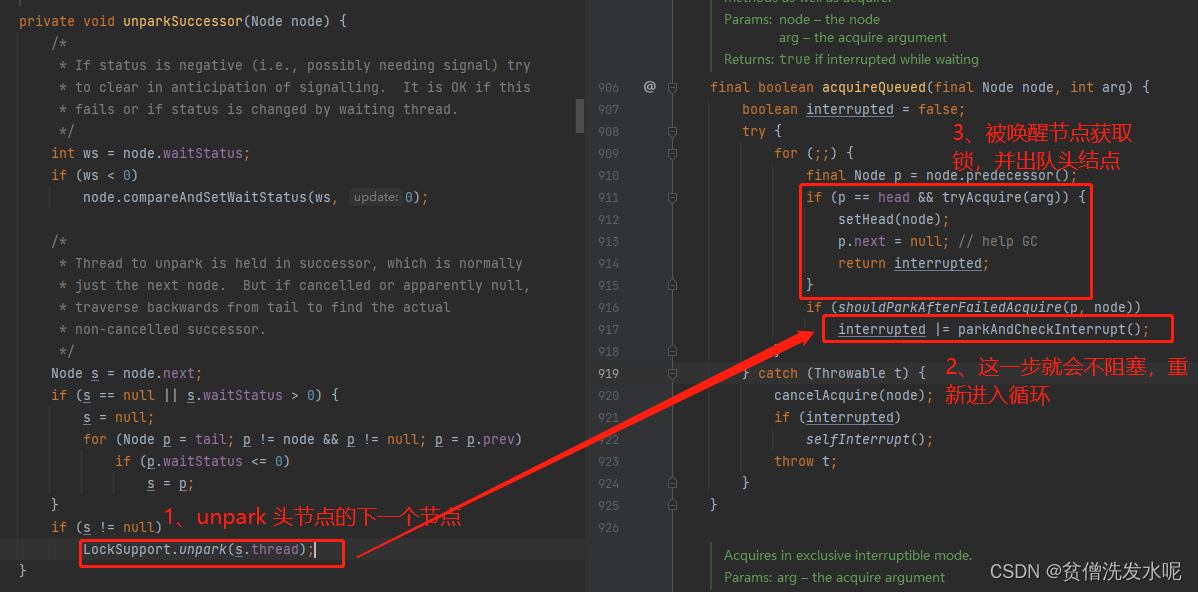
Java多线程篇(6)——AQS之ReentrantLock
文章目录 1、管程2、AQS3、ReentrantLock3.1、lock/unlock3.1.1、lock3.1.2、unlock 3.2、一些思考 1、管程 什么是管程? 管理协调多个线程对共享资源的访问,是一种高级的同步机制。 有哪些管程模型? hansen:唤醒其他线程的代码…...
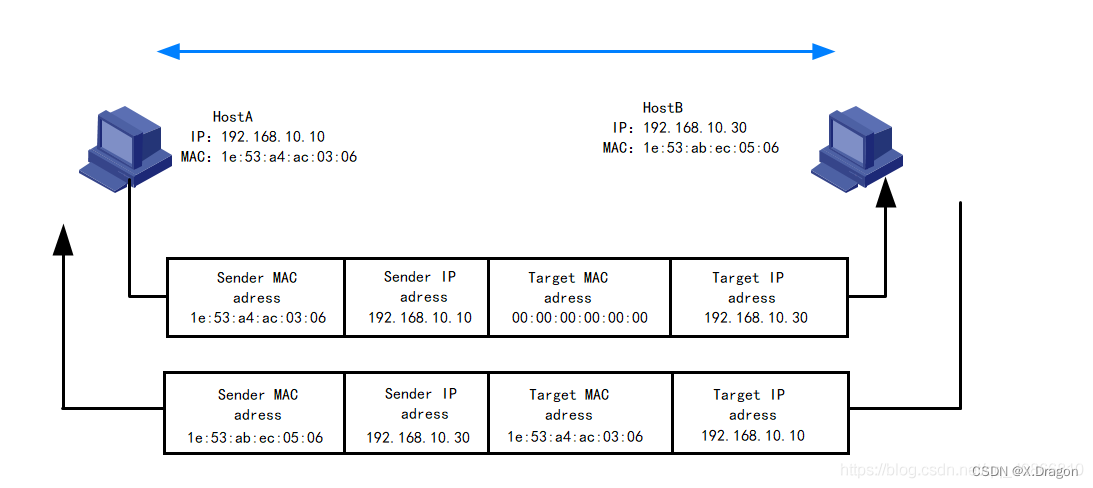
【计算机网络】IP协议第二讲(Mac帧、IP地址、碰撞检测、ARP协议介绍)
IP协议第二讲 1.IP和Mac帧2.碰撞检测2.1介绍2.2如何减少碰撞发生2.3MTU2.4一些补充 3.ARP协议3.1协议介绍3.2报文格式分析 1.IP和Mac帧 IP(Internet Protocol)和MAC(Media Access Control)帧是计算机网络中两个不同层次的概念&am…...
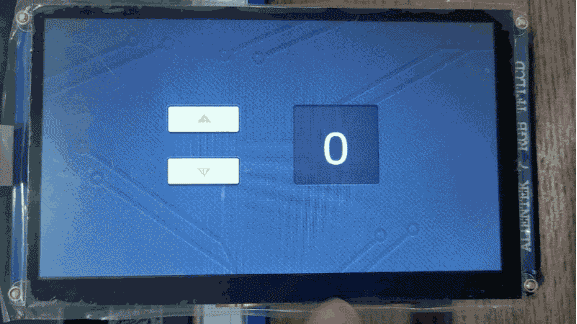
TouchGFX界面开发 | 按钮控件应用示例
按钮控件应用示例 按钮是最常见的部件之一,有了按钮就可以点击,从而响应事件,达到人机交互的目的。TouchGFX Designer内置了七种按钮部件: 下压按钮:能够在被释放时发送回调,按下和释放状态都关联了图像标…...
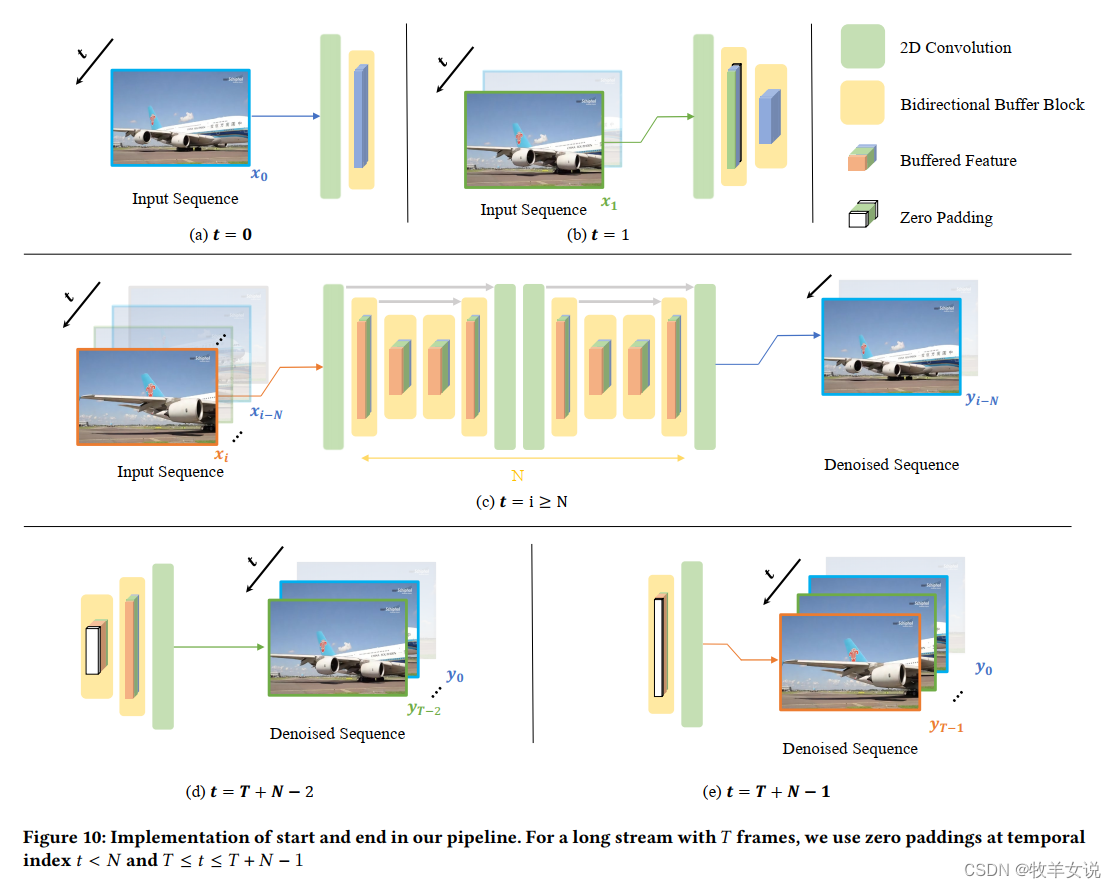
BSVD论文理解:Real-time Streaming Video Denoising with Bidirectional Buffers
BSVD是来自香港科技大学的一篇比较新的视频去噪论文,经实践,去噪效果不错,在这里分享一下对这篇论文的理解。 论文地址:https://arxiv.org/abs/2207.06937 代码地址:GitHub - ChenyangQiQi/BSVD: [ACM MM 2022] Real…...
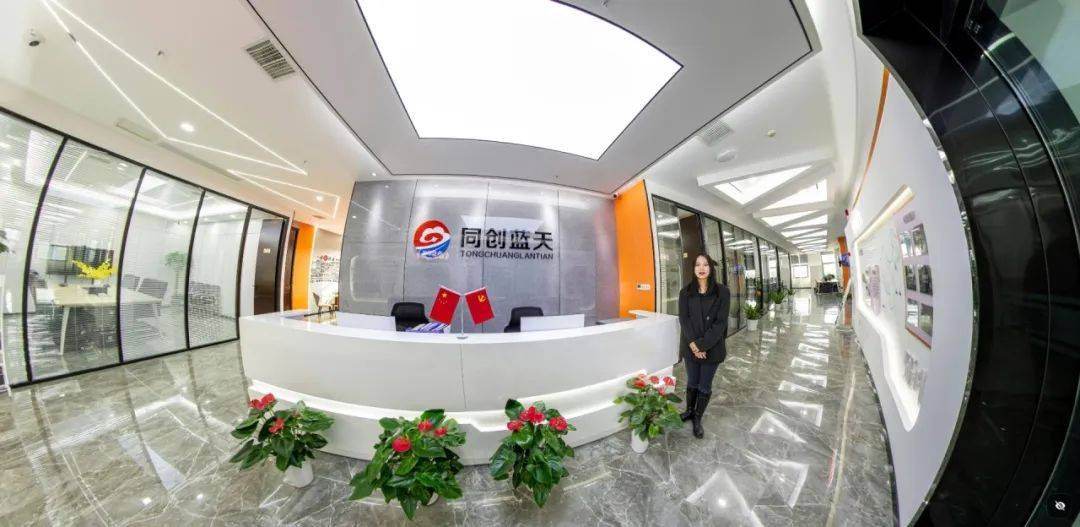
共同见证丨酷雷曼武汉运营中心成立2周年
酷雷曼武汉运营中心2周年 全国合作商齐贺武汉公司2周年庆 2021年 作为酷雷曼辐射全国版图的又一重要据点 酷雷曼武汉运营中心 在“中国光谷”正式成立 沉浸式参观酷雷曼武汉公司 2年时间 尽管历经诸多客观因素的挑战 但后浪扬帆,依然交出了不斐的成绩 解决…...
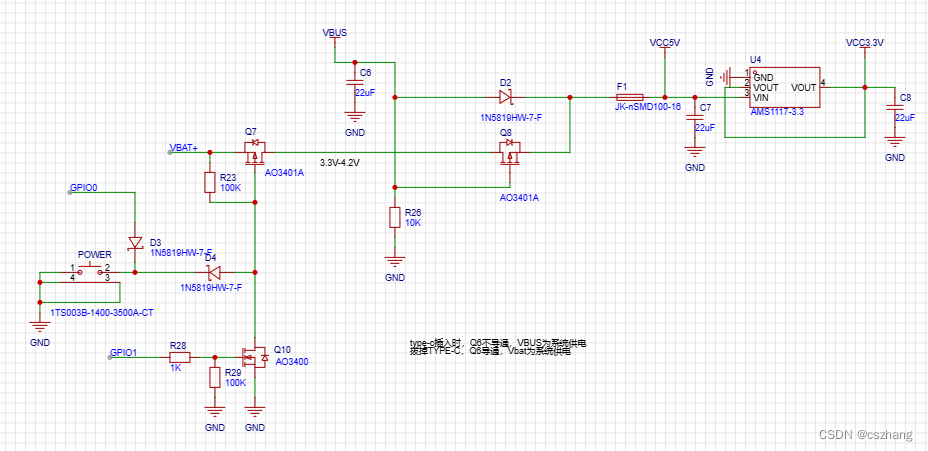
一种单键开关机电路图
我们设计产品时,通常需要设计单键开关机功能。 单键开关机,通常需要单片机的两个IO完成,一个IO用于保持开机状态。另外,一个IO用于判定关机状态。 下面就是一种单键开关机电路原理图: 此单键开关电路已经在S2W-M02、S2…...
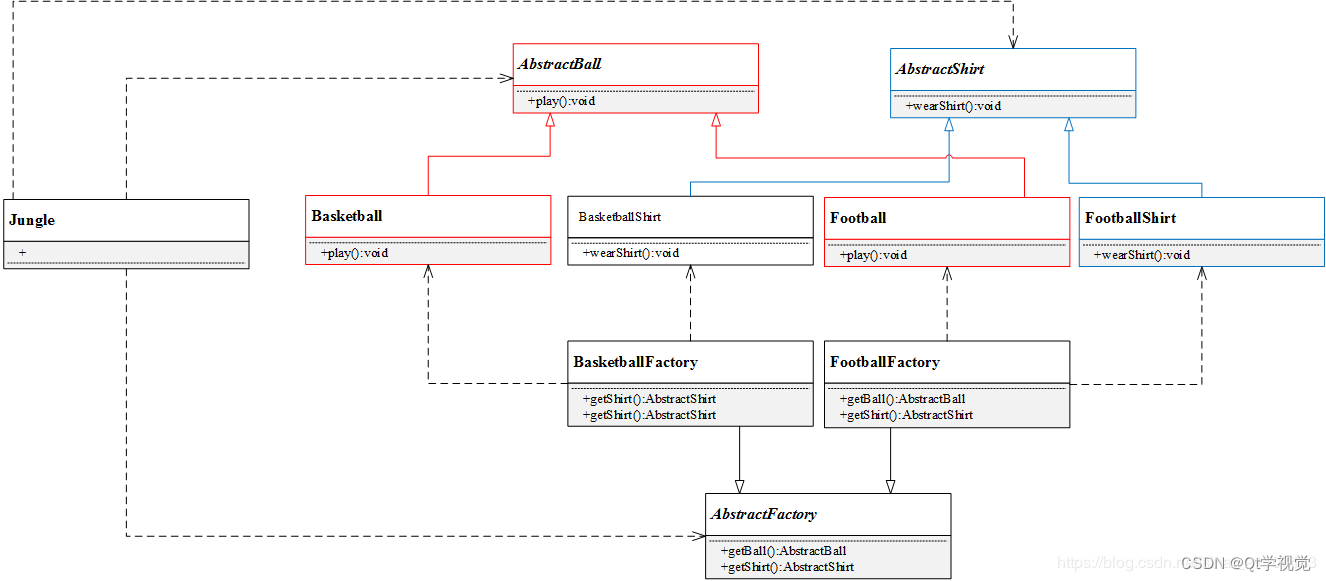
设计模式2、抽象工厂模式 Abstract Factory
解释说明:提供一个创建一系列相关或相互依赖对象的接口,而无需指定他们具体的类。 简言之,一个工厂可以提供创建多种相关产品的接口,而无需像工厂方法一样,为每一个产品都提供一个具体工厂 抽象工厂(Abstra…...

C++ 32盏灯,利用进制和 与 或 进行设计
一共32盏灯,设计一个灯光控制系统,其中 台球部8盏灯 桌游区8盏灯 酒吧区8盏灯 休息区8盏灯 满足以下功能 1、能够独立控制每一盏灯 2、能够一次性打开或关闭一个区域的全部灯光 3、能够获取各个区域的灯光打开关闭情况 4、能够一次性关闭打开的灯&#x…...

Ffmpeg-(1)-安装:ubuntu系统安装Ffmpeg应用
1、下载源码压缩包 https://ffmpeg.org/download.html 点击Download Source Code下载即可 解压: tar -xvjf ffmpeg-snapshot.tar.bz2 得到:ffmpeg目录 cd ffmpeg 或者:直接下 wget http://www.ffmpeg.org/releases/ffmpeg-5.1.tar.gztar -zx…...
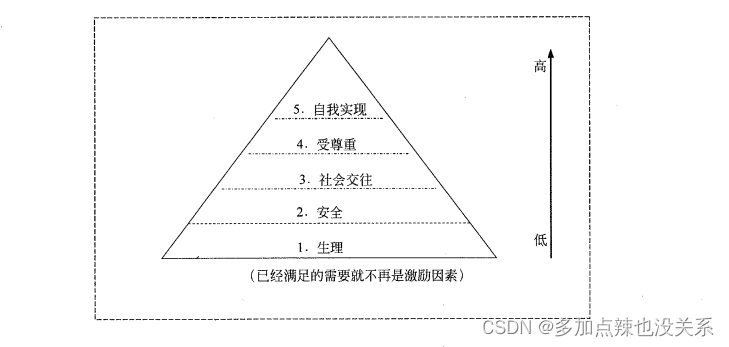
系统集成|第十一章(笔记)
目录 第十一章 项目人力资源管理11.1 项目人力资源管理的定义及有关概念11.2 主要过程11.2.1 编制项目人力资源管理计划11.2.2 组建项目团队11.2.3 建设项目团队11.2.4 管理项目团队 11.3 现代激励理论11.4 项目经理所需具备的影响力11.5 常见问题 上篇:第十章、质量…...
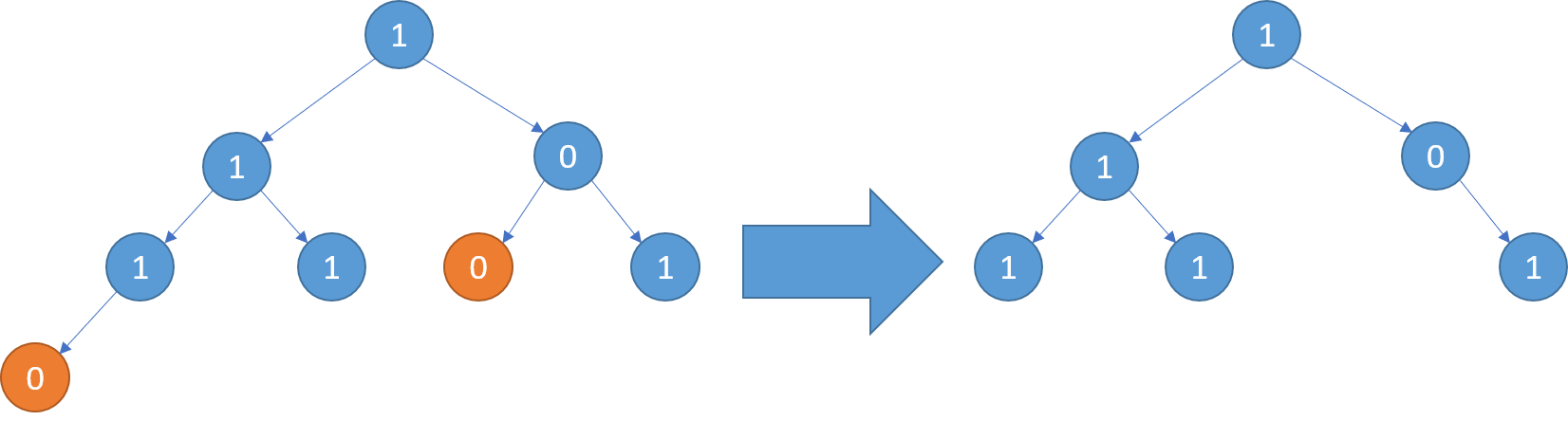
二叉树题目:二叉树剪枝
文章目录 题目标题和出处难度题目描述要求示例数据范围 解法思路和算法代码复杂度分析 题目 标题和出处 标题:二叉树剪枝 出处:814. 二叉树剪枝 难度 4 级 题目描述 要求 给定二叉树的根结点 root \texttt{root} root,返回移除了所有…...
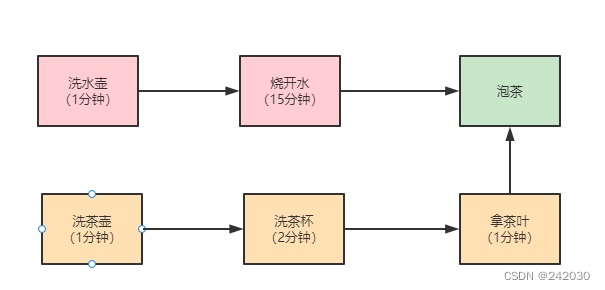
JAVA中使用CompletableFuture进行异步编程
JAVA中使用CompletableFuture进行异步编程 1、什么是CompletableFuture CompletableFuture 是 JDK8 提供的 Future 增强类,CompletableFuture 异步任务执行线程池,默认是把异步任 务都放在 ForkJoinPool 中执行。 在这种方式中,主线程不会…...

uniapp:配置动态接口域名,根据图片访问速度,选择最快的接口
common.js // 动态测速选择的域名 // h5直接返回默认第一个域名 // vue文件用到域名的话用this.$baseURL let domains [{uri:192.168.31.215:9523, speed:0},{uri:api.ceshi.org, speed:0}, ]export const protocol {api: http://,//本地// api: https://api.,//正式h5Url: h…...

Lambda表达式常见用法(提高效率神器)
Java8中一个非常重要的特性就是Lambda表达式,我们可以把它看成是一种闭包,它允许把函数当做参数来使用,是面向函数式编程的思想,一定程度上可以使代码看起来更加简洁。 其实以上都不重要,重要的是能够提高我的开发效率…...

2023旷视自驾感知算法暑期实习一面
来源:投稿 作者:LSC 编辑:学姐 1. 问下项目,问下我的情况 2. 是否了解最新的BEV算法,讲一下 3. 是否了解三维重建 4. 考察相机坐标系的转换 5. 手撕代码,翻车了,不考leetcode,考…...
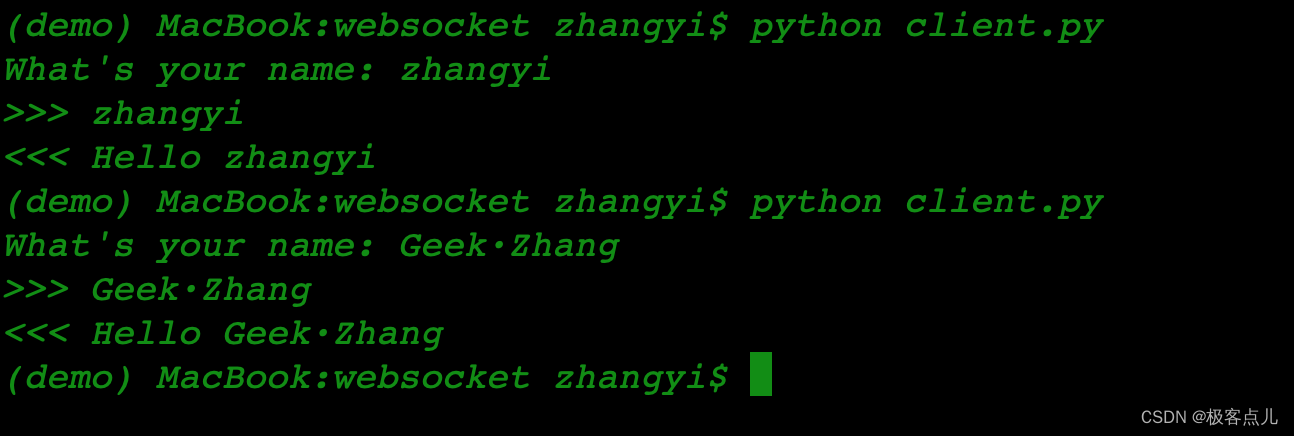
Python3 如何实现 websocket 服务?
Python 实现 websocket 服务很简单,有很多的三方包可以用,我从网上大概找到三种常用的包:websocket、websockets、Flask-Sockets。 但这些包很多都“年久失修”, 比如 websocket 在 2010 年就不维护了。 而 Flask-Sockets 也在 2…...
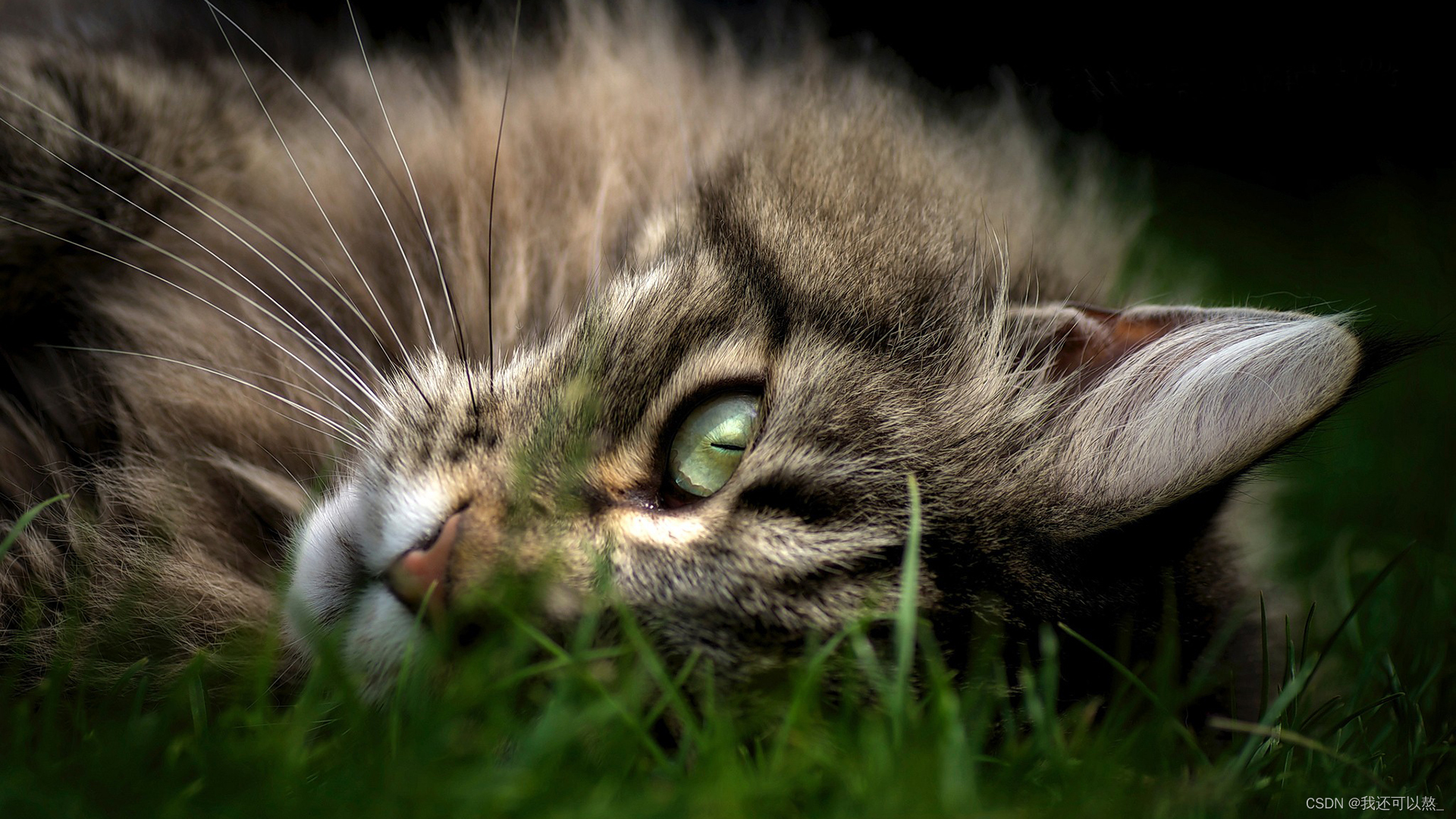
SQLAlchemy常用数据类型
目录 SQLAlchemy常用数据类型 代码演示 代码分析 SQLAlchemy常用数据类型 SQLAlchemy 是一个Python的SQL工具库和对象关系映射(ORM)工具,它提供了一种在Python中操作数据库的高效方式。下面是SQLAlchemy中常用的一些数据类型: Integer:整形&…...
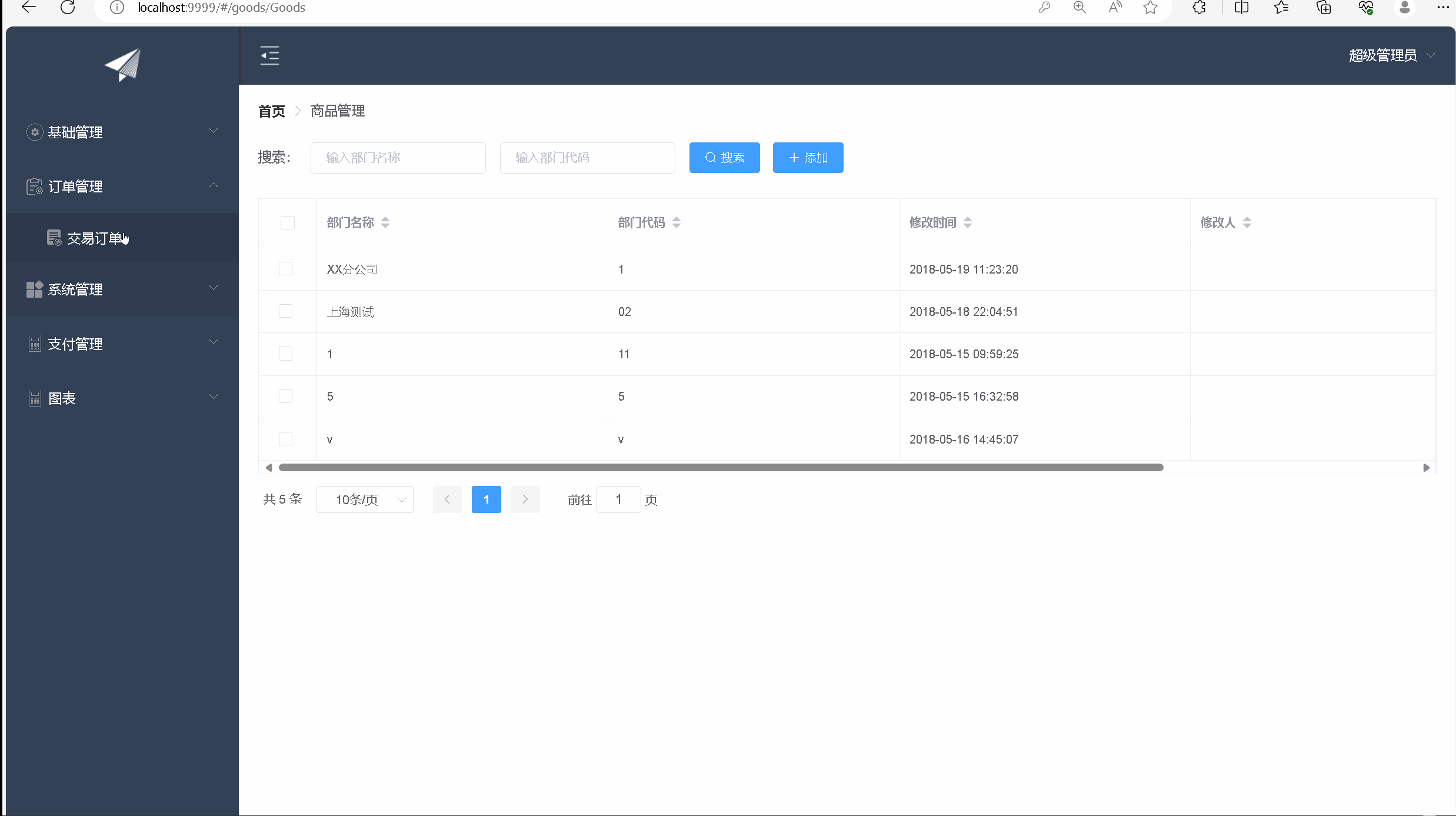
Vue路由与nodejs下载安装及环境变量的配置
目录 前言 一、Vue路由 1.路由简介 是什么 作用 应用场景 2.SPA简介 SPA是什么 SPA的优点 注意事项 3.路由实现思路 1.引入路由的js依赖 2.定义组件 3.定义组件与路径的对应关系 4.通过路由关系获取路由对象router 5.将路由对象挂载到实例中 6.触发路由事…...
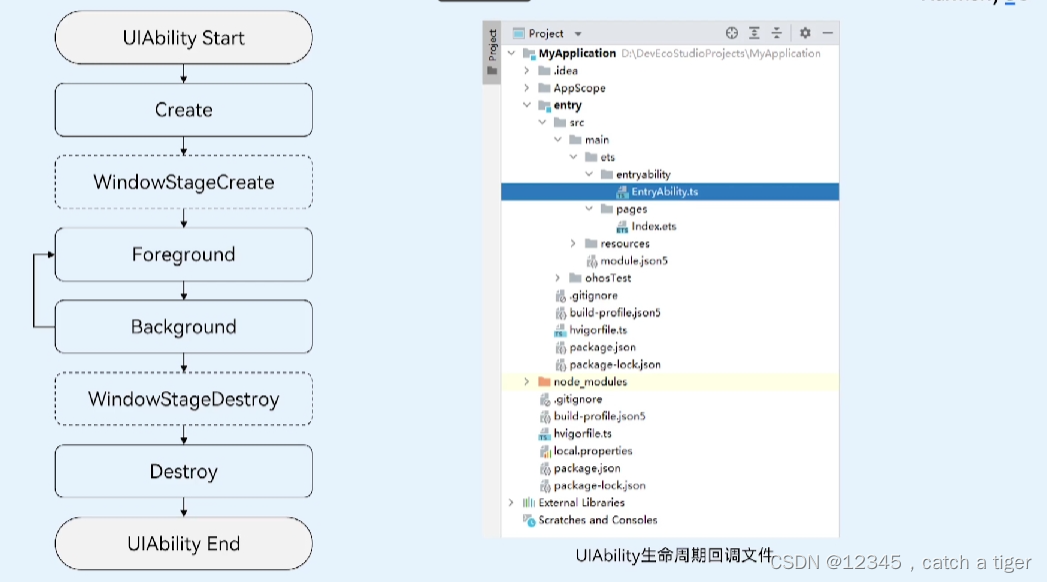
HarmonyOS之 应用程序页面UIAbility
一 UIAbility介绍: 1.1 UIAbility是一种包含用户界面的应用组件,主要用于和用户进行交互 1.2 UIAbility也是系统调度的单元,为应用提供窗口在其中绘制界面 二 UIAbility跳转和传参 2.1 页面间的导航可以通过页面路由router模块来实现。页…...
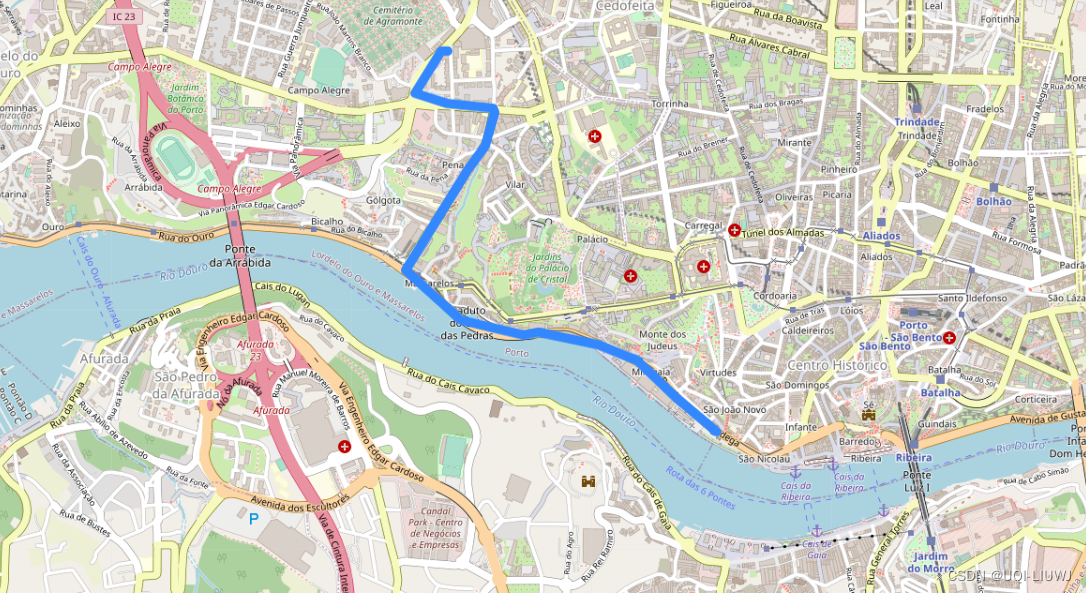
数据集笔记: Porto
数据来源:Taxi Trajectory Data_数据集-阿里云天池 (aliyun.com) 1 数据介绍 葡萄牙波尔图市运行的所有442辆出租车的全年轨迹(从2013年7月1日至2014年6月30日) 2 读取数据 import pandas as pdtrapd.read_csv(C:/Users/16000/Download…...
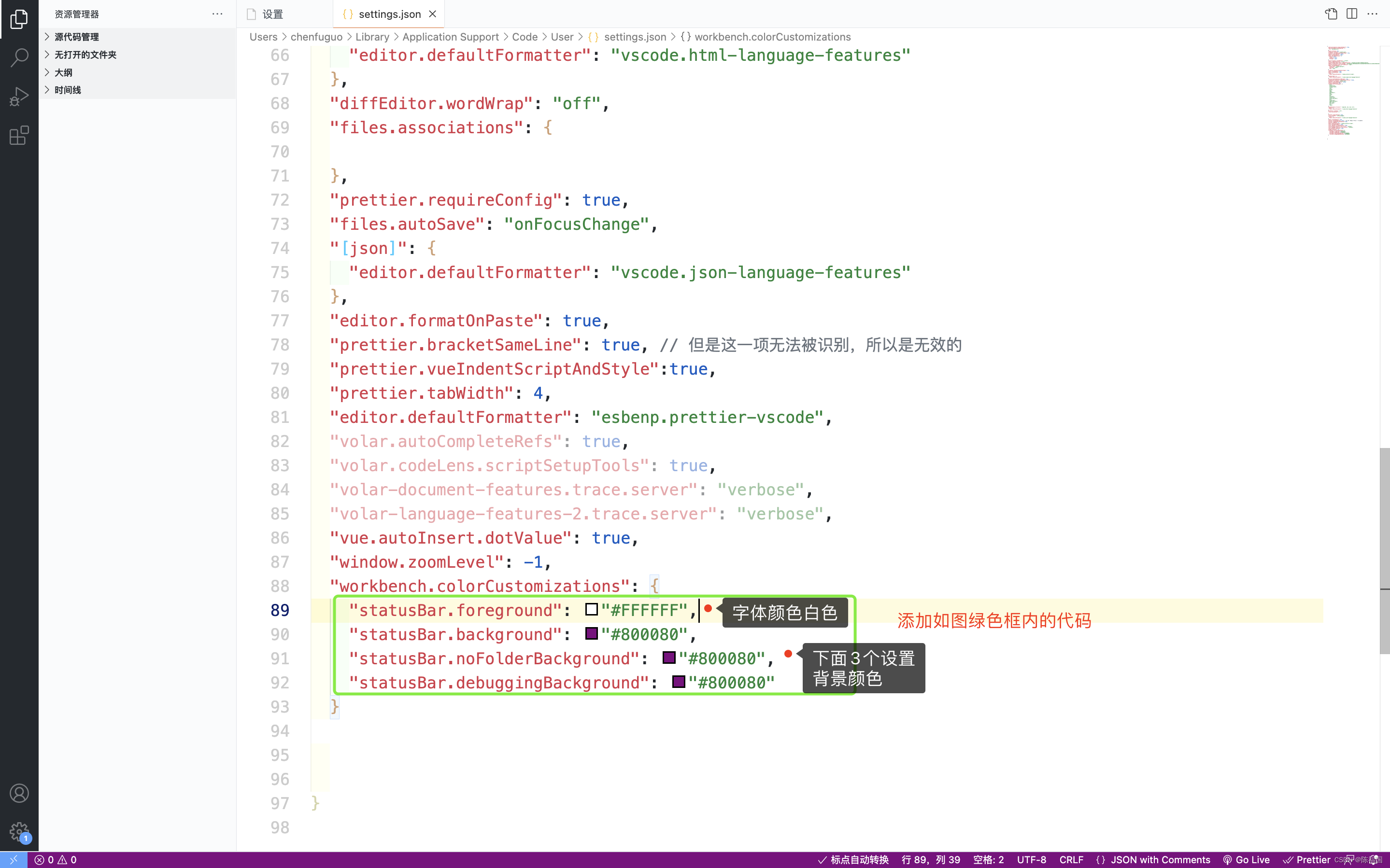
修改vscode底部栏背景和字体颜色
修改vscode底部栏背景和字体颜色 如图: 首先打开齿轮,打开设置搜索workbench.colorCustomizations,然后点击编辑setting.json修改setting.json内内容 "workbench.colorCustomizations": {"statusBar.foreground": "#FFFFFF…...

加速企业AI实施:成功策略和效率方法
文章目录 写在前面面临的挑战MlOps简介好书推荐 写作末尾 写在前面 作为计算机科学领域的一个关键分支,机器学习在当今人工智能领域中占据着至关重要的地位,广受瞩目。机器学习通过深入分析大规模数据并总结其中的规律,为我们提供了解决许多…...
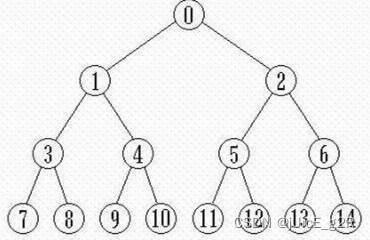
【图论C++】树的重心——教父POJ 3107(链式前向星的使用)
》》》算法竞赛 /*** file * author jUicE_g2R(qq:3406291309)————彬(bin-必应)* 一个某双流一大学通信与信息专业大二在读 * * brief 一直在竞赛算法学习的路上* * copyright 2023.9* COPYRIGHT 原创技术笔记:转载…...

视频网站如何做盗链/威海网站制作
文章目录1 报错2 解决办法1 报错 尝试在linux上通过Pycharm IDE使用matplotlib包。当我运行此代码时: from matplotlib import pyplot结果报错: ImportError: No module named tkinter2 解决办法 ubuntu的系统: sudo apt-get install py…...

wordpress的搭建环境/百度广告运营
常规的这两个校验工具的用法,可以阅读之前的博文,链接如下: @Valid 和@Validated的区别 那么如果大家看完了基础用法,就可以继续深入学习了,本篇博文主要对这个两个注解的用法进行自定义,实现扩展 废话不多说,上正菜…… 案例一 /*** 大版本信息表** @author fangh*…...

网站运营部的职责/核心关键词
上篇文章中我们谈到了,Service的远程沟通,既当Activity和Service不在一个进程中,它们之间是怎么相互通信的,不过只是停留在原理层面,今天傻蛋写了一个测试程序来进一步说明远程沟通机制。 Android框架的IPC沟通其实是依…...

个人网站如何赚钱/百度推广代理查询
在linux下面,为了保证服务器安全,通常会记录所敲命令的历史记录,但是记录为1000条,并且退出重新登录后,之前的变会没有了,通过编辑/etc/bashrc文件记录历史命令到日志文件下面,并已登录来源IP&a…...

wordpress广告管理插件/seo和sem的区别
Android 学习已有一年半有余,先后做过两款游戏、三款应用和搭建一台服务端,也了解过一些Android相关的源码(JDK、SDK和NDK) 后来想学深入点,搞过两款开源项目(LGame和AChartEngine),…...

做标签网站/理发培训专业学校
docker images|grep none|awk {print $3}|xargs docker rmi 转载于:https://www.cnblogs.com/jiuchongxiao/p/9597069.html...