基于知识库的chatbot或者FAQ
背景
最近突然想做一个基于自己的知识库(knowlegebase)的chatbot或者FAQ的项目。未来如果可以在公司用chatgpt或者gpt3.5之后的模型的话,还可以利用gpt强大的语言理解力和搜索出来的用户问题的相关业务文档来回答用户在业务中的问题。
Chatbot UI
FAQ UI
后端代码实现
1. 建立一个基于excel的简单的知识库,
2.利用knowlege_base_service.py文件来获取上面知识库中所有的问题。
import pandas as pd
knowledge_base = pd.read_excel("./data/knowledge_base.xlsx")require_to_reload = False
def get_all_questions():knowledge_base = get_knowlege_base()return knowledge_base["Question"].tolist();passdef get_knowlege_base():global require_to_reload, knowledge_base# knowledge_base_dict = knowledge_base.to_dict(orient="records")if require_to_reload == True:knowledge_base = pd.read_excel("./data/knowledge_base.xlsx")require_to_reload = Falsereturn knowledge_base
3. 创建一个句子相似度比较的模型,用来比较用户输入的问题和我们知识库中问题的相似度。
base 类
class BaseSentenceSimilarityModel():def calculate_sentence_similarity(self, source_sentence, sentences_to_compare):print("padding to be overided by subclass")results = []return resultsdef find_most_similar_question(self, source_sentence, sentences_to_compare):print("padding to be overided by subclass")return ''
模型1. TF-IDF
class TFIDFModel(BaseSentenceSimilarityModel):def calculate_sentence_similarity(self, source_sentence, sentences_to_compare):# Combine source_sentence and sentences_to_compare into one list for vectorizationsentences = [source_sentence] + sentences_to_compare# Create a TF-IDF vectorizervectorizer = TfidfVectorizer()# Compute the TF-IDF matrixtfidf_matrix = vectorizer.fit_transform(sentences)# Calculate cosine similarity between the source_sentence and sentences_to_comparesimilarity_scores = cosine_similarity(tfidf_matrix[0], tfidf_matrix[1:])scores = similarity_scores.flatten();results = []for idx, score in enumerate(scores):# print('sentence:', sentences_to_compare[idx], f", score: {score:.4f}")results.append( {'sentence': sentences_to_compare[idx], 'score': round(score, 4) })print(results)return resultsdef find_most_similar_question(self, source_sentence, sentences_to_compare):results = self.calculate_sentence_similarity(source_sentence, sentences_to_compare)most_similar_question = ''score = 0for result in results:# print('sentence:', sentences_to_compare[idx], f", score: {score:.4f}")if result['score'] > score and result['score']>0.7:score = result['score']most_similar_question = result['sentence']return most_similar_question
模型二,基于glove词向量的模型
class Word2VectorModel(BaseSentenceSimilarityModel):def calculate_sentence_similarity(self, source_sentence, sentences_to_compare):# Parse the sentences using spaCysentences = [source_sentence] + sentences_to_comparegloveHelper = GloveHelper()source_sentence_vector = gloveHelper.getVector(source_sentence)sentences_vector_mean = []for sentence in sentences:sentences_vector = gloveHelper.getVector(sentence)# sentences_vector_mean.append(sentences_vector)sentences_vector_mean.append(np.mean(sentences_vector, axis=0))# Calculate cosine similarity between the source_sentence and sentences_to_compareprint(np.array(sentences_vector_mean[0]).shape)print(np.array(sentences_vector_mean[1:]).shape)similarity_scores = cosine_similarity([sentences_vector_mean[0]], np.array(sentences_vector_mean[1:]))scores = similarity_scores.flatten();results = []for idx, score in enumerate(scores):# print('sentence:', sentences_to_compare[idx], f", score: {score:.4f}")results.append({'sentence': sentences_to_compare[idx], 'score': round(float(score), 4)})print(results)return resultsdef find_most_similar_question(self, source_sentence, sentences_to_compare):results = self.calculate_sentence_similarity(source_sentence, sentences_to_compare)most_similar_question = ''score = 0for result in results:# print('sentence:', sentences_to_compare[idx], f", score: {score:.4f}")if result['score'] > score and result['score']>0.7:score = result['score']most_similar_question = result['sentence']return most_similar_question
模型三,tensorhub 里的模型 universal-sentence-encoder_4
import tensorflow_hub as hubenable_universal_sentence_encoder_Model = True
if enable_universal_sentence_encoder_Model:print('loading universal-sentence-encoder_4 model...')embed = hub.load("C:/apps/ml_model/universal-sentence-encoder_4")class UniversalSentenceEncoderModel(BaseSentenceSimilarityModel):def calculate_sentence_similarity(self, source_sentence, sentences_to_compare):# Parse the sentences using spaCysentences = [source_sentence] + sentences_to_comparesentences_vectors = embed(sentences)sentences_vectors = sentences_vectors.numpy()print(sentences_vectors)# sentences_vector_mean = np.mean(sentences_vectors, axis=1)# for sentences_vector in sentences_vectors:# sentences_vector_mean.append(np.mean(sentences_vector, axis=0))# Calculate cosine similarity between the source_sentence and sentences_to_compareprint(np.array(sentences_vectors[0]).shape)print(np.array(sentences_vectors[1:]).shape)similarity_scores = cosine_similarity([sentences_vectors[0]], np.array(sentences_vectors[1:]))scores = similarity_scores.flatten();results = []for idx, score in enumerate(scores):# print('sentence:', sentences_to_compare[idx], f", score: {score:.4f}")results.append({'sentence': sentences_to_compare[idx], 'score': round(float(score), 4)})print(results)return resultsdef find_most_similar_question(self, source_sentence, sentences_to_compare):print("universal sentence encoder model....")results = self.calculate_sentence_similarity(source_sentence, sentences_to_compare)most_similar_question = ''score = 0for result in results:# print('sentence:', sentences_to_compare[idx], f", score: {score:.4f}")if result['score'] > score and result['score']>0.6:score = result['score']most_similar_question = result['sentence']return most_similar_question
4. 利用flask 创建一个rest api
app = Flask(__name__)
CORS(app)@app.route('/')
def index():return 'welcome to my webpage!'@app.route('/api/chat', methods=['POST','GET'])
def send_message():user_message = request.json.get('user_message')# Find the most similar question in the knowledge baseanswer = find_most_similar_question(user_message)return jsonify({'bot_response': answer})def find_most_similar_question(user_question , model = 'tf_idf_model'):knowledge_base = get_knowlege_base()print('model name :', model)if model == 'tf_idf_model':sentenceSimilarityModel = TFIDFModel()passelif model == 'word2vector_model':sentenceSimilarityModel = Word2VectorModel()elif model == 'UniversalSentenceEncoder_Model':from nlp.sentence_similarity.universal_sentence_encoder_model import UniversalSentenceEncoderModelsentenceSimilarityModel = UniversalSentenceEncoderModel()else:sentenceSimilarityModel = TFIDFModel()most_similar_question = sentenceSimilarityModel.find_most_similar_question(user_question, knowledge_base["Question"].tolist())filtered_df = knowledge_base[knowledge_base["Question"] == most_similar_question]# Check if any matching rows were foundif not filtered_df.empty:found_answer = filtered_df.iloc[0]["Answer"]print("Answer:", found_answer)return found_answerelse:print("No answer found for the question:", user_question)return 'No answer found for the question';def get_top_faq():# Count the frequency of each questiontop_question = knowledge_base.head(3).to_dict(orient="records")print(top_question)return top_questionif __name__=="__main__":app.run(port=2020,host="127.0.0.1",debug=True)
前端Angular UI
chat.component.css
.chat-container {max-width: 60%;margin: 0 auto;background-color: #f7f7f7;box-shadow: 0 4px 8px rgba(0, 0, 0, 0.2);
}.chat-area {max-height: 550px;overflow-y: auto;padding: 20px;background-color: #f7f7f7;border-radius: 10px;
}
.chat-header {color: black; /* Set text color */background-color: #ececf1;text-align: center;padding: 10px;/*box-shadow: 0px 2px 4px rgba(0, 0, 0, 0.1); !* Add a subtle shadow *!*/border-bottom: 1px solid #ccc; /* Add a border at the bottom */font-size: 35px; /* Adjust the font size as needed */
}.chat-foot{padding: 10px 15px;margin: 10px}.user-bubble {--tw-border-opacity: 1;background-color: white; /* User message background color */border-color: rgba(255,255,255,var(--tw-border-opacity));border-radius: 10px;padding: 10px 10px;margin: 10px 0;/* max-width: 85%;*/align-self: flex-end;}
.chat-message{display: flex;}.bot-bubble {--tw-border-opacity: 1;background-color: #ececf1; /* Chatbot message background color */border-collapse: rgba(255,255,255,var(--tw-border-opacity));border-radius: 10px;padding: 10px 10px;margin: 10px 0;/*max-width: 85%;*/align-self: flex-start;justify-content: right;}
.form-container {display: flex;align-items: center;
}.user-input {/* width: 86%;*/flex-grow: 1;padding: 10px;border: 1px solid #ccc;border-radius: 5px;outline: none;font-size: 16px;/*margin-top: 10px;*/margin-right: 10px;
}.indented-div {margin-right: 10px; /* Adjust this value as needed */padding: 15px 1px 10px 10px
}/* Send button */
.send-button {/* width: 10%;*/width: 100px;background-color: #3f51b5;color: #fff;border: none;border-radius: 5px;padding: 10px 20px;font-size: 16px;cursor: pointer;transition: background-color 0.3s;
}.send-button:hover {background-color: #303f9f;
}.chat_left{display: flex;padding: 0px 0px 0px 10px;margin: 1px 0;}.chat_right {/* float: right;*/ /* Align bot actions to the right *//* margin-left: 10px;*/ /* Add some spacing between the chat message and bot actions */width: 50px;/* padding: 10px 15px;*//* margin: 20px 5px;*/margin: 20px 20px 20px 2px
}.chat_right i {color: #000;transition: color 0.3s;cursor: pointer;
}.chat_right i:hover {color: darkorange;}/* text-suggestion.component.css */
.suggestion-container {position: relative;width: calc(100% - 110px);}.suggestion-container ul {list-style: none;padding: 0;margin: 0;/* width: 91.6%;*/width : 100%;position: absolute;/*top: -195px; !* Adjust this value to control the distance from the input *!*/background-color: #fff; /* Customize this background color *//*border: 1px solid #ccc;*/border-radius: 5px; /* Add border radius for styling */box-shadow: 0 2px 5px rgba(0, 0, 0, 0.2); /* Add box shadow for a card-like effect */
}.selected {background-color: #f0f0f0; /* Highlight color */
}.suggestion-container li {padding: 10px;cursor: pointer;
}.suggestion-container li:hover {background-color: #f2f2f2; /* Hover effect */
}.category-button {background-color: #fff;color: #333;border: 1px solid #ccc;padding: 5px 10px;margin: 5px;border-radius: 5px;cursor: pointer;font-size: 15px;transition: background-color 0.3s, border-color 0.3s;
}
.category{margin-bottom: 10px
}.category-button.selected {/*background-color: #007bff;*//*color: #fff;*//*border-color: #007bff;*/color: #007bff;border: 1px solid #007bff;
}.category-button:hover {/*background-color: #007bff;*/color: #007bff;border: 1px solid #007bff;/*border-color: #007bff;*/
}
chat.component.html
<div class="chat-container"><div class="chat-header">ChatBot</div><div #chatArea class="chat-area"><!-- <div *ngFor="let message of chatMessages" style = " flex-direction: column; display: flex;">--><div *ngFor="let message of chatMessages" style = "display: flex; justify-content: space-between"><div class="chat_left" style="flex-grow: 1; flex:8"><ng-container *ngIf="message.type === 'user'" ><div class = "indented-div"> <img style = "height: 25px" src = "assets/user.svg" alt="User:"/></div></ng-container><ng-container *ngIf="message.type === 'bot'" style = "word-break:break-word"><div class = "indented-div"> <img style = "height: 25px" src = "assets/bot.svg" alt="Bot:"/></div></ng-container><div [ngClass]="{'user-bubble': message.type === 'user', 'bot-bubble': message.type === 'bot'}" style="flex-grow: 1" ><div [innerHTML]="message.text" style = "word-break:break-word"> </div></div></div><div class="chat_right" style = ""><div *ngIf="message.type === 'bot'" ><i (click)="onThumbsUpClick(message)"> <img style = "height:20px" src = "assets/thumb-up.svg" alt="ThumbsUp"/></i><i (click)="onThumbsDownClick(message)"> <img style = "height:20px" src = "assets/thumb-down.svg" alt="ThumbsDown"/> </i></div></div></div></div><div class = "chat-foot" ><div class="category"><button class="category-button" [class.selected]="selectedCategory === 'general'" (click)="selectCategory('general')">General</button><button class="category-button" [class.selected]="selectedCategory === 'ecs'" (click)="selectCategory('ecs')">ecs</button><button class="category-button" [class.selected]="selectedCategory === 'jdk17'" (click)="selectCategory('jdk17')">jdk17</button><button class="category-button" [class.selected]="selectedCategory === 'kafka'" (click)="selectCategory('kafka')">kafka</button><button class="category-button" [class.selected]="selectedCategory === 'Permission'" (click)="selectCategory('Permission')">Permission</button></div><div ><form (submit)="sendMessage()"><div class="suggestion-container"><ul *ngIf="showSuggestions" [style.top.px] = "-suggestions.length*41.3"><li *ngFor="let suggestion of suggestions; let i = index" [class.selected]="i === selectedSuggestionIndex" (click)="onSuggestionClick(suggestion)">{{ suggestion }}</li></ul></div><div style = "display: flex"><input class="user-input" name="userMessage" placeholder="Type your message..." [(ngModel)]="userMessage" (input)="onQueryChange()" (keydown)="onKeyDown($event)" autocomplete="off"/><button class="send-button" >Send</button></div></form></div></div></div>
chat.component.ts
import { Component, ElementRef, ViewChild, AfterViewChecked, OnInit } from '@angular/core';
import { HttpClient } from '@angular/common/http';
import { DomSanitizer } from '@angular/platform-browser';
import {host} from "../app-config";@Component({selector: 'app-chat',templateUrl: './chat.component.html',styleUrls: ['./chat.component.css']
})
export class ChatComponent implements AfterViewChecked, OnInit {@ViewChild('chatArea') private chatArea!: ElementRef;userMessage: string = '';chatMessages: any[] = [];suggestions: string[] = [];allSuggestions: string[] = [];showSuggestions = false;selectedSuggestionIndex: number = -1;selectedCategory: string = 'general'; // Default categoryconstructor(private http: HttpClient,private sanitizer: DomSanitizer) {this.http.get<string[]>(host+'/faq/all-suggestions').subscribe(data => {this.allSuggestions = data});}ngOnInit() {this.sanitizeMessages();this.chatMessages.push({ text: 'Hello! How can I assist you?', type: 'bot' });}selectCategory(category: string) {this.selectedCategory = category;// Implement category-specific logic or fetching here}ngAfterViewChecked() {this.scrollToBottom();}onKeyDown(event: KeyboardEvent) {// console.info("....."+event.key)if (event.key === 'ArrowDown') {event.preventDefault();this.selectedSuggestionIndex =(this.selectedSuggestionIndex + 1) % this.suggestions.length;this.userMessage = this.suggestions[this.selectedSuggestionIndex];} else if (event.key === 'ArrowUp') {event.preventDefault();this.selectedSuggestionIndex =(this.selectedSuggestionIndex - 1 + this.suggestions.length) % this.suggestions.length;this.userMessage = this.suggestions[this.selectedSuggestionIndex];}}onSuggestionClick(suggestion: string) {this.userMessage = suggestion;this.showSuggestions = false;}sendMessage() {if (this.userMessage === undefined || this.userMessage.trim() === ''){return;}this.showSuggestions=falsethis.chatMessages.push({ text: this.userMessage, type: 'user' });this.http.post<any>(host+'/api/chat', { user_message: this.userMessage }).subscribe(response => {this.chatMessages.push({ text: response.bot_response, type: 'bot' });this.userMessage = '';});}scrollToBottom() {try {this.chatArea.nativeElement.scrollTop = this.chatArea.nativeElement.scrollHeight;} catch (err) {}}onThumbsUpClick(message: any) {console.log('Thumbs up clicked for the bot message: ', message.text);}onThumbsDownClick(message: any) {console.log('Thumbs down clicked for the bot message: ', message.text);}// Sanitize messages with HTML contentsanitizeMessages() {for (let message of this.chatMessages) {if (message.type === 'bot') {message.text = this.sanitizer.bypassSecurityTrustHtml(message.text);}}}onQueryChange() {this.showSuggestions = true;this.suggestions = this.getTop5SimilarSuggestions(this.allSuggestions, this.userMessage);}getTop5SimilarSuggestions(suggestions: string[], query: string): string[] {return suggestions.filter(suggestion => suggestion.toLowerCase().includes(query.toLowerCase())).sort((a, b) => this.calculateSimilarity(a, query) - this.calculateSimilarity(b, query)).slice(0, 5);}calculateSimilarity(suggestion: string, query: string): number {// You can use Levenshtein distance or any other similarity metric here// Example: Using Levenshtein distanceif (suggestion === query) return 0;const matrix = [];const len1 = suggestion.length;const len2 = query.length;for (let i = 0; i <= len2; i++) {matrix[i] = [i];}for (let i = 0; i <= len1; i++) {matrix[0][i] = i;}for (let i = 1; i <= len2; i++) {for (let j = 1; j <= len1; j++) {const cost = suggestion[j - 1] === query[i - 1] ? 0 : 1;matrix[i][j] = Math.min(matrix[i - 1][j] + 1,matrix[i][j - 1] + 1,matrix[i - 1][j - 1] + cost);}}return matrix[len2][len1];}}
相关文章:
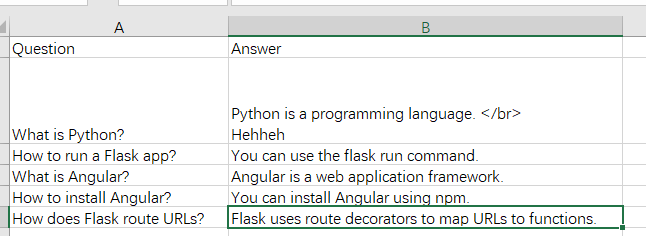
基于知识库的chatbot或者FAQ
背景 最近突然想做一个基于自己的知识库(knowlegebase)的chatbot或者FAQ的项目。未来如果可以在公司用chatgpt或者gpt3.5之后的模型的话,还可以利用gpt强大的语言理解力和搜索出来的用户问题的相关业务文档来回答用户在业务中的问题。 Chat…...
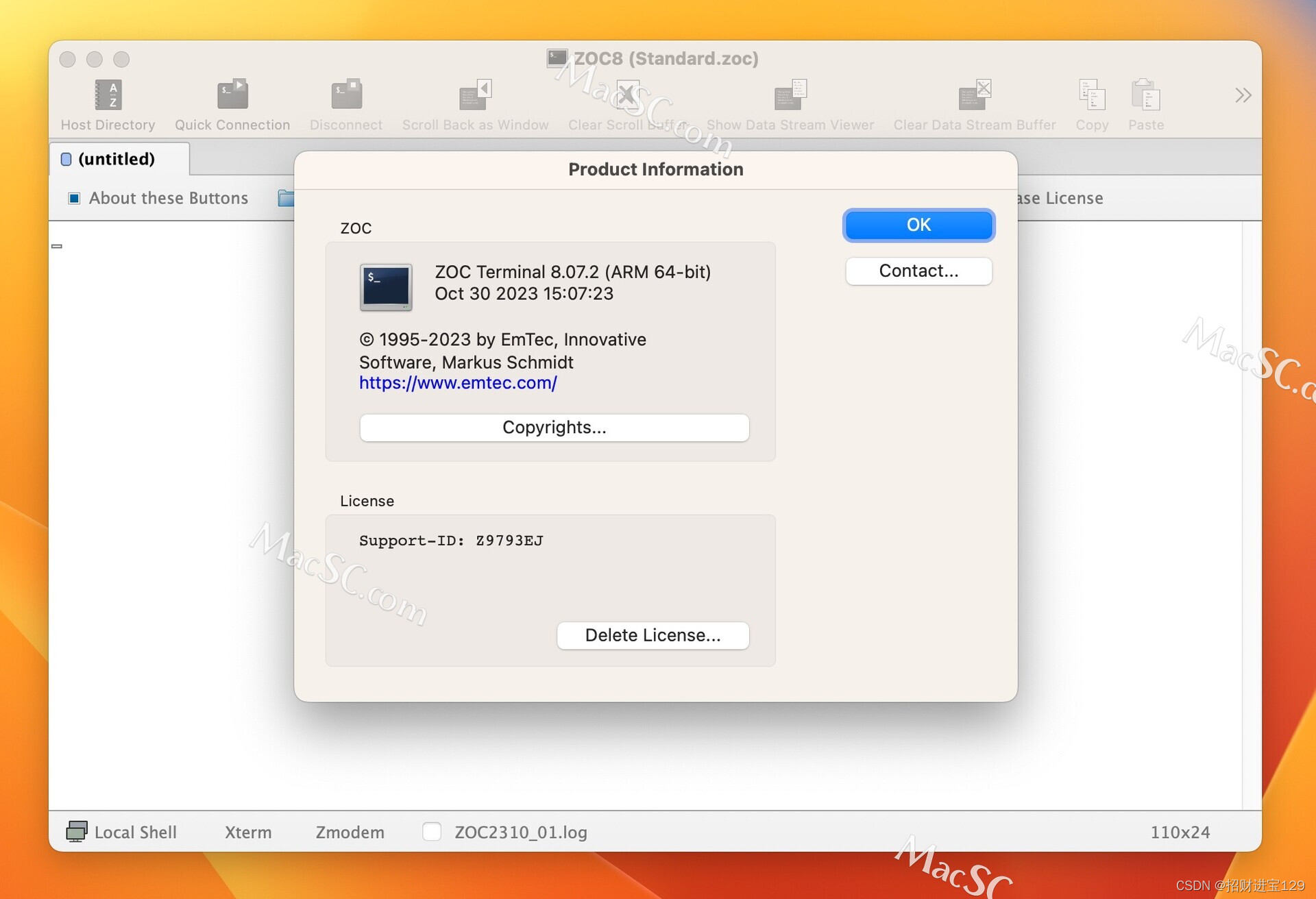
ZOC8 for Mac:超越期待的终端仿真器
在Mac上,一个优秀的终端仿真器是每位开发者和系统管理员的必备工具。ZOC8,作为一款广受好评的终端仿真器,以其强大的功能和易用性,已经在Mac用户中积累了良好的口碑。本文将为您详细介绍ZOC8的各项特性,以及为什么它会…...
织梦dedecms后台档案列表显示空白或显示不了文章的解决方法
织梦dedecms后台档案列表显示空白或显示不了文章的解决方法 dede/content_list.php空白解决方法如下 dede/content_list.php空白 在DEDE后台可以查看栏目文章,但是所有档案列表却为空白或者显示不了文章,如图所示: 后来找到dede/content_list.php,看了下…...
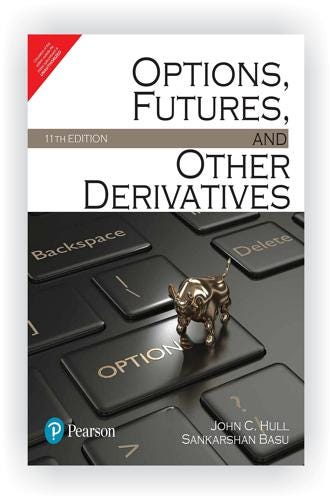
10本值得阅读的量化交易书籍
什么是量化交易? 量化交易是利用数学模型或算法来创建交易策略并进行交易。量化交易通常由大型机构交易员或对冲基金雇用,他们雇用大量的博士和工程师团队。从历史上看,量化交易领域一直非常隐秘,有效的想法往往受到公司的严密保…...
c++通过对象的地址初始化指针,需要对指针进行释放么(企业链表衍生)
在C中,如果你通过对象的地址来初始化指针,通常情况下是不需要手动释放指针的。这是因为对象的生存期与指针所指向的对象的生存期相关联。当对象超出其作用域或被销毁时,指向该对象的指针也会自动成为悬挂指针,这种情况下再访问该指…...
CentOS安装MySQL
参考官方链接:https://dev.mysql.com/doc/refman/8.0/en/linux-installation-rpm.html CentOS版本 [rootlocalhost ~]# cat /etc/redhat-release CentOS Linux release 7.9.2009 (Core) 下载MySQL安装包(版本:8.0.35) 访问地址…...
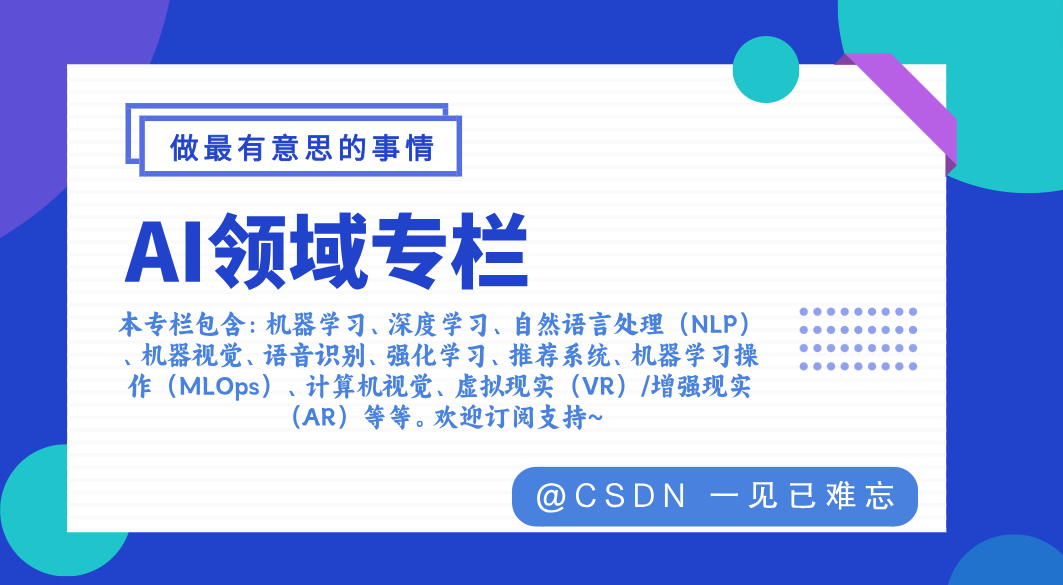
AI:45-基于深度学习的声纹识别
🚀 本文选自专栏:AI领域专栏 从基础到实践,深入了解算法、案例和最新趋势。无论你是初学者还是经验丰富的数据科学家,通过案例和项目实践,掌握核心概念和实用技能。每篇案例都包含代码实例,详细讲解供大家学习。 📌📌📌本专栏包含以下学习方向: 机器学习、深度学…...
Spring-cloud-openfeign拦截器RequestInterceptor接口
RequestInterceptor接口位于包io.github.openfeign-core下,使用Spring Cloud Feign的时候会自动依赖这个包 下面的代码会在每次调用Feign1的m1方法时,向HTTP头追加键值对武汉3:晴川历历汉阳树 FeignClient(value "feignA", url "XXX或…...

自动化测试开发 —— 如何封装自动化测试框架?
封装自动化测试框架,测试人员不用关注框架的底层实现,根据指定的规则进行测试用例的创建、执行即可,这样就降低了自动化测试门槛,能解放出更多的人力去做更深入的测试工作。本篇文章就来介绍下,如何封装自动化测试框架…...
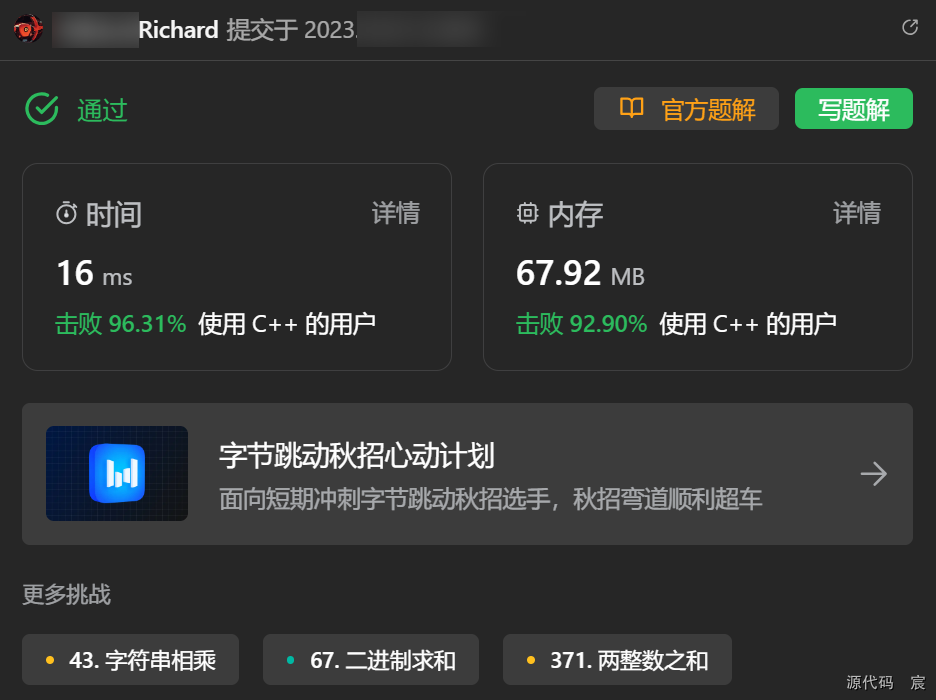
Leetcode—2.两数相加【中等】
2023每日刷题(十五) Leetcode—2.两数相加 迭代法实现代码 /*** Definition for singly-linked list.* struct ListNode {* int val;* struct ListNode *next;* };*/ struct ListNode* addTwoNumbers(struct ListNode* l1, struct ListNode* l…...
拷贝音频、视频、word等二进制文件的实现方法,不掉帧
拷贝音频、视频、word等二进制文件的实现方法: 演示使用BufferedOutputStream 和 BufferedInputStream 使用 使用他们,可以完成二进制文件 思考:字节流可以操作二进制文件,可以操作文本文件吗?True public class B…...
dmfldr-快速装载-载入(DM8:达梦数据库)
dmfldr-快速装载-DM8:达梦数据库 介绍1 准备数据文件2 根据数据文件在数据库创建表3 根据数据文件,配置快速装载的控制文件4 在数据库bin执行目录执行命令5 日志6 达梦数据库学习使用列表 介绍 DM 提供了快速装载工具:dmfldr;通过使用快速装载工具能够把…...
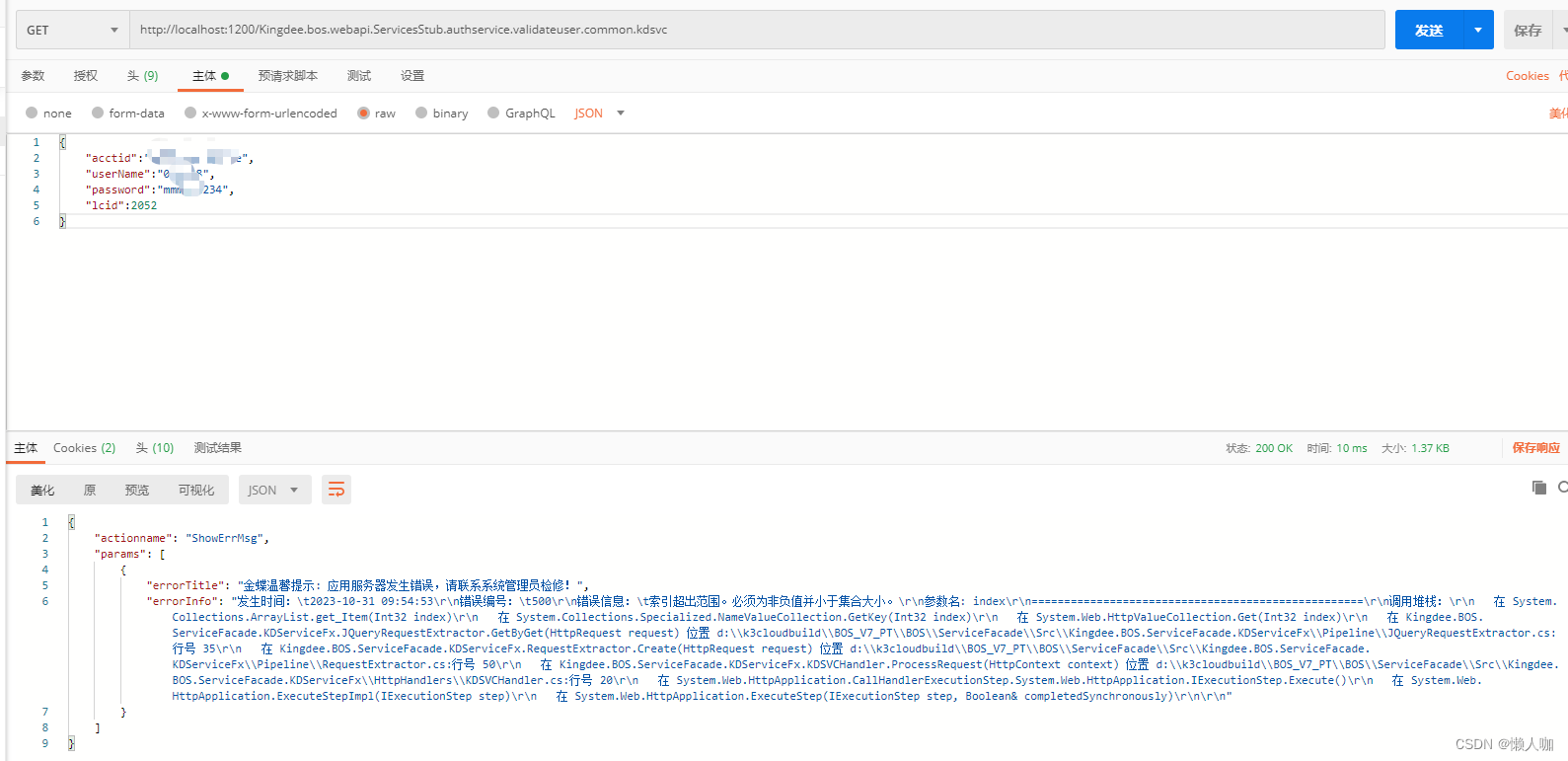
Postman测试金蝶云星空Webapi【协同开发云】
文章目录 Postman测试金蝶云星空Webapi【协同开发云】环境说明业务背景大致流程具体操作请求登录接口请求标准接口查看保存提交审核反审核撤销 请求自定义接口参数是字符串参数是实体类单个实体类实体类是集合 其他 Postman测试金蝶云星空Webapi【协同开发云】 环境说明 金蝶…...
mongo常用操作符及查询例子
比较操作符: $eq:匹配字段值等于指定值。 $ne:匹配字段值不等于指定值。 $gt:匹配字段值大于指定值。 $gte:匹配字段值大于或等于指定值。 $lt:匹配字段值小于指定值。 $lte:匹配字段值小于或等…...
41.排序练习题(王道2023数据结构第8章综合练习)
试题1(王道8.3.3节综合练习2): 编写双向冒泡排序算法,在正反两个方向交替扫描。即第一趟把关键字最大的元素放在序列的最后面,第二趟把关键字最小的元素放在序列最前面,如此反复。 首先实现冒泡排序&…...
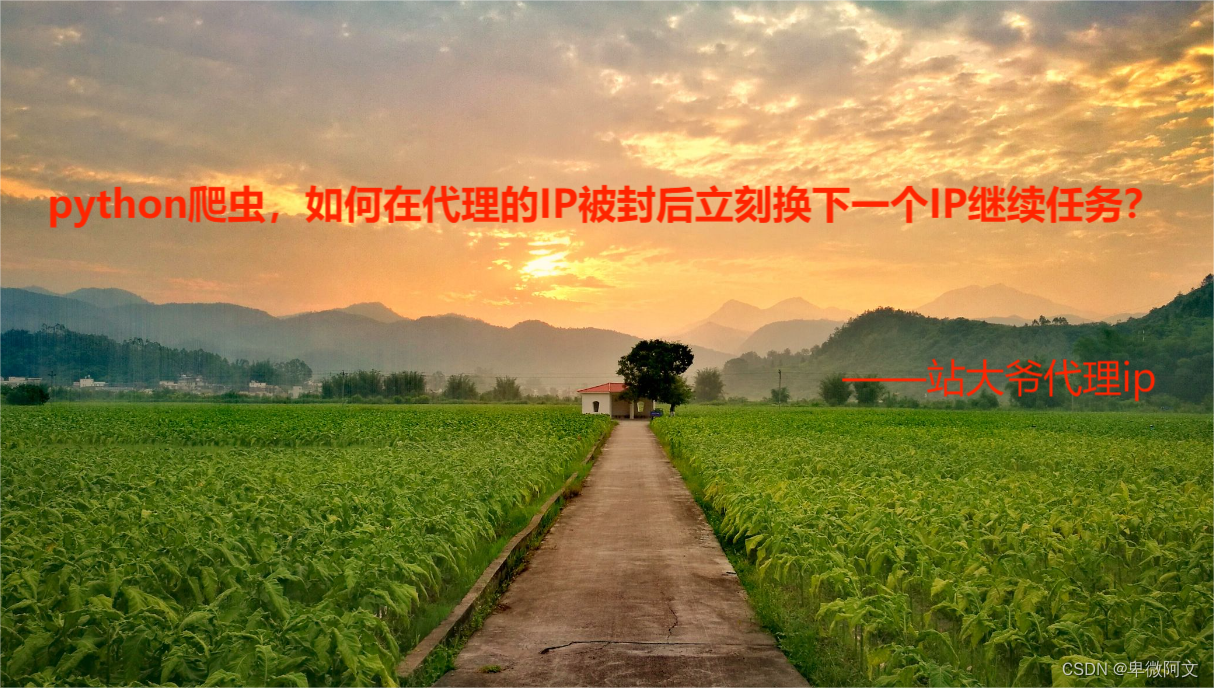
python爬虫,如何在代理的IP被封后立刻换下一个IP继续任务?
前言 在实际的爬虫应用中,爬虫程序经常会通过代理服务器来进行网络访问,以避免访问过于频繁而受到网站服务器的限制。但是,代理服务器的IP地址也可能被目标网站限制,导致无法正常访问。这时候,我们需要在代理IP被封后…...
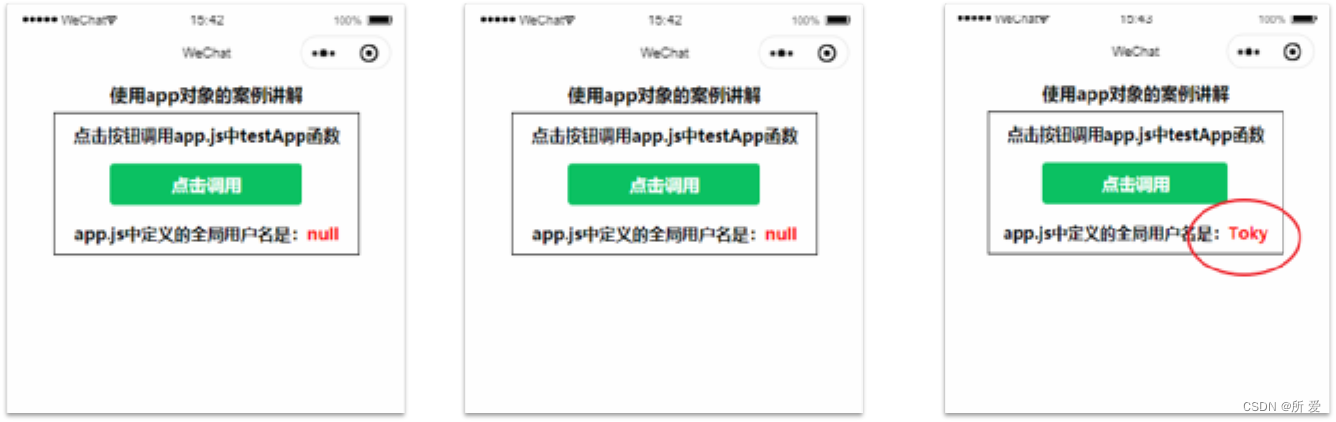
小程序开发——小程序项目的配置与生命周期
1.app.json配置属性 app.json配置属性 2.页面配置 app的页面配置指的是pages属性, pages数组的第一个页面将默认作为小程序的启动页。利用开发工具新建页面时,则pages属性对应的数组将自动添加该页面的路径,若是在硬盘中添加文件的形式则不…...
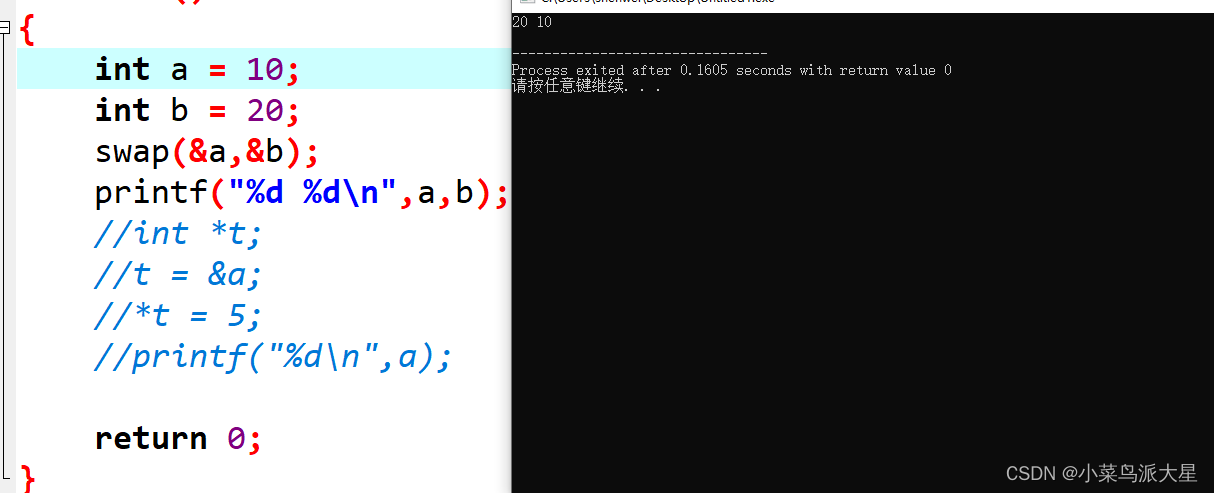
C语言之用指针交换两个数
1.指针存放是是地址,所以在用指针交换两个数的时候,需要对指针进行解引用(*p)。 用指针交换两个数,需要知道p1p2与*p1*p2。 p1p1是将p2的值赋值给p1. *p1*p2是将p2指针地址存放的值,赋值给p1指针地址存放的值,即p1地…...
Day 48 动态规划 part14
Day 48 动态规划 part14 解题理解1143103553 3道题目 1143. 最长公共子序列 1035. 不相交的线 53. 最大子数组和 解题理解 1143 设dp[i][j]为text10: i-1text20: j-1的最长公共子序列。 class Solution:def longestCommonSubsequence(self, text1: str, text2: str) -> …...
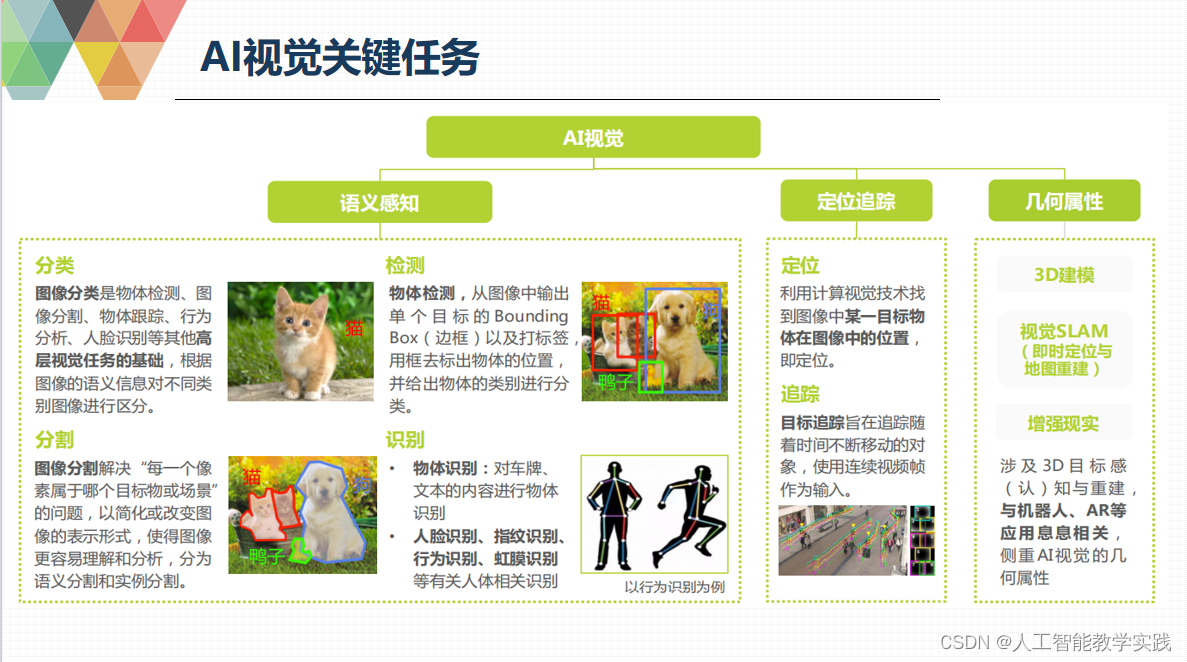
目标检测与图像识别分类的区别?
目标检测与图像识别分类的区别 目标检测和图像识别分类是计算机视觉领域中两个重要的任务,它们在处理图像数据时有一些区别。 目标检测是指在图像中定位和识别多个目标的过程。其主要目标是确定图像中每个目标的边界框位置以及对应的类别标签。目标检测任务通常涉…...
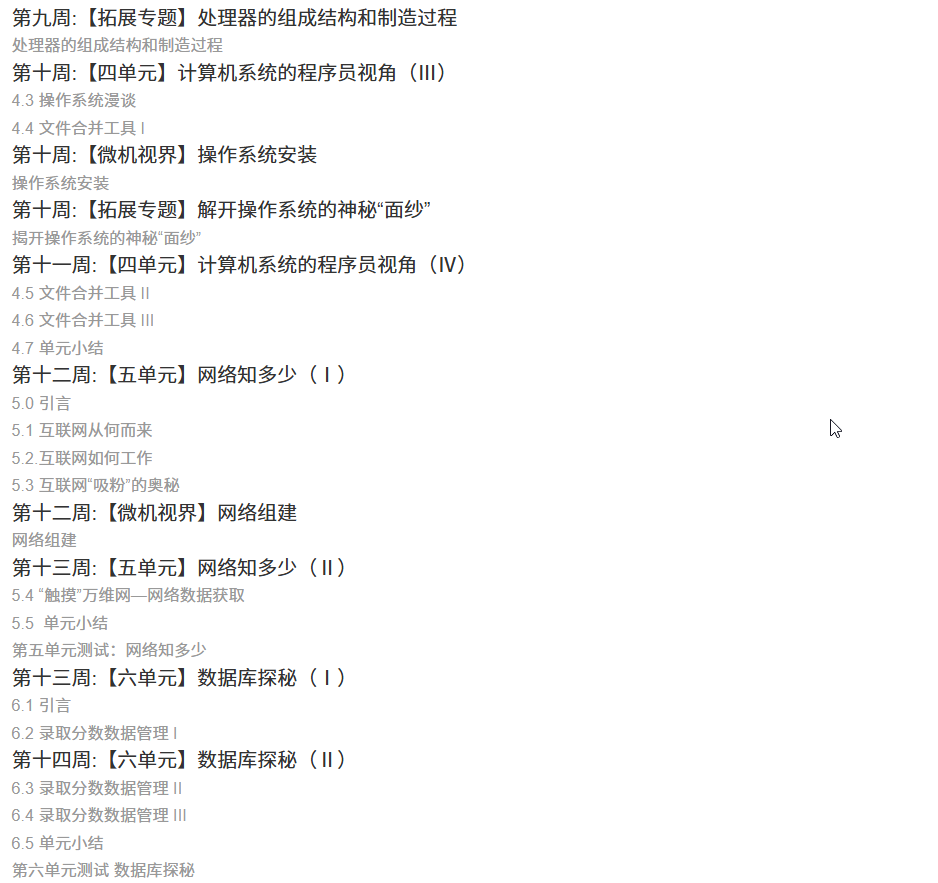
国防科技大学计算机基础课程笔记02信息编码
1.机内码和国标码 国标码就是我们非常熟悉的这个GB2312,但是因为都是16进制,因此这个了16进制的数据既可以翻译成为这个机器码,也可以翻译成为这个国标码,所以这个时候很容易会出现这个歧义的情况; 因此,我们的这个国…...
conda相比python好处
Conda 作为 Python 的环境和包管理工具,相比原生 Python 生态(如 pip 虚拟环境)有许多独特优势,尤其在多项目管理、依赖处理和跨平台兼容性等方面表现更优。以下是 Conda 的核心好处: 一、一站式环境管理:…...
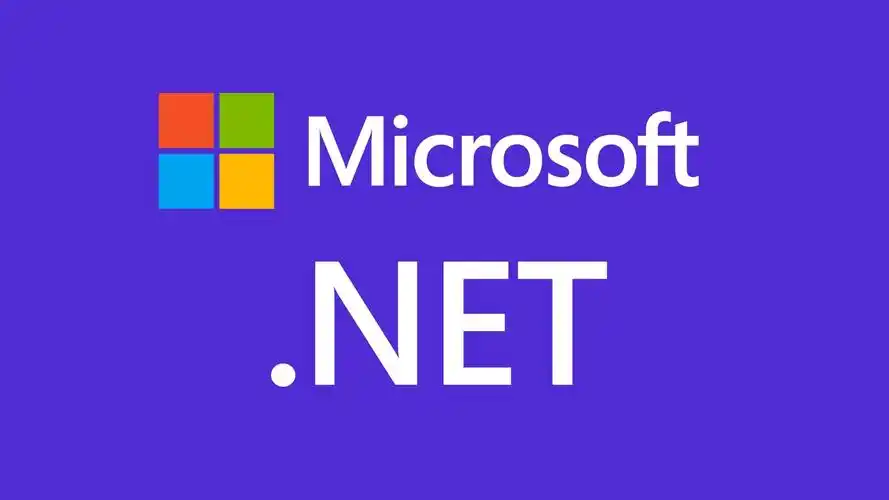
.Net框架,除了EF还有很多很多......
文章目录 1. 引言2. Dapper2.1 概述与设计原理2.2 核心功能与代码示例基本查询多映射查询存储过程调用 2.3 性能优化原理2.4 适用场景 3. NHibernate3.1 概述与架构设计3.2 映射配置示例Fluent映射XML映射 3.3 查询示例HQL查询Criteria APILINQ提供程序 3.4 高级特性3.5 适用场…...
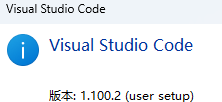
无法与IP建立连接,未能下载VSCode服务器
如题,在远程连接服务器的时候突然遇到了这个提示。 查阅了一圈,发现是VSCode版本自动更新惹的祸!!! 在VSCode的帮助->关于这里发现前几天VSCode自动更新了,我的版本号变成了1.100.3 才导致了远程连接出…...
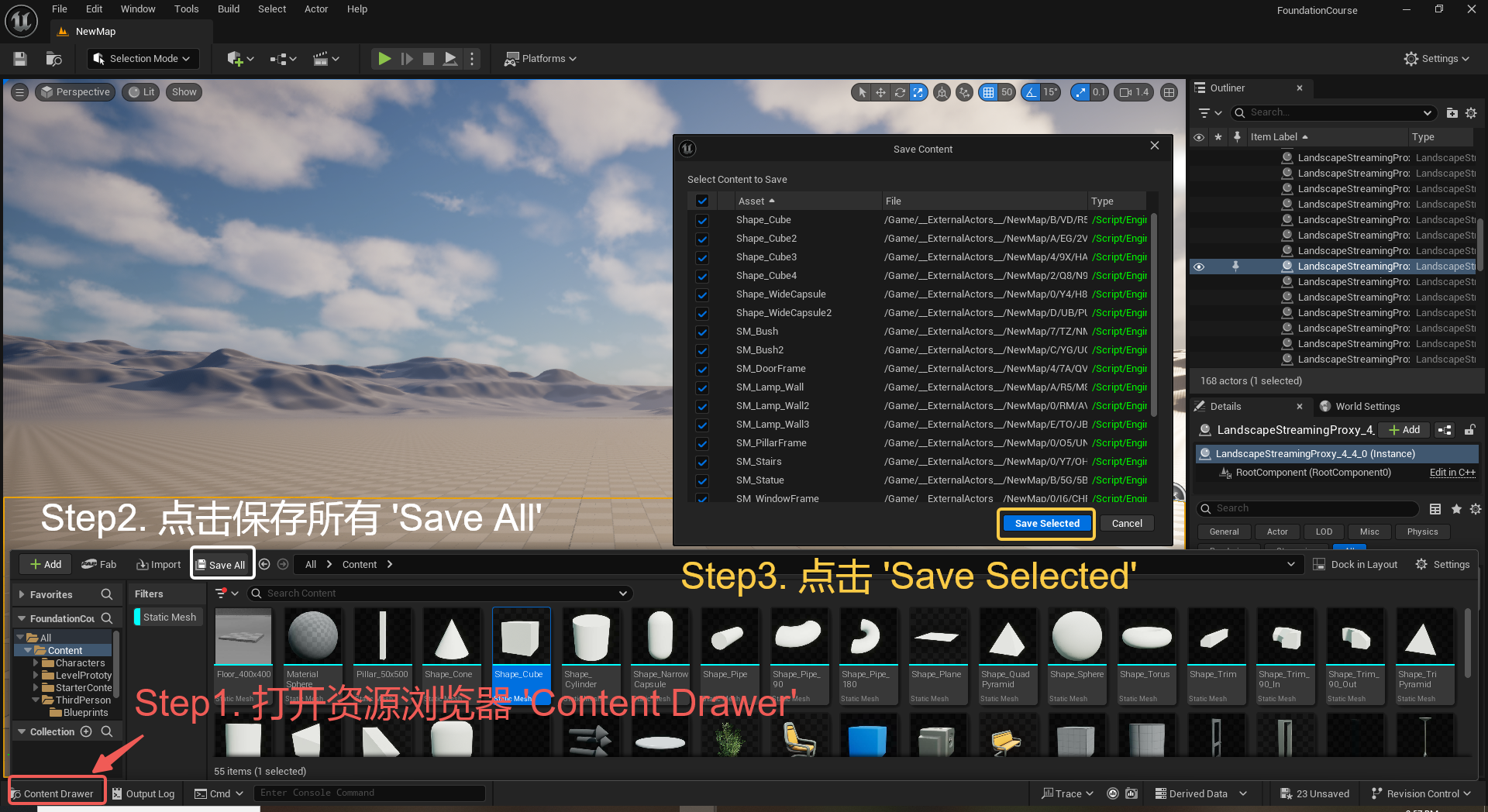
UE5 学习系列(三)创建和移动物体
这篇博客是该系列的第三篇,是在之前两篇博客的基础上展开,主要介绍如何在操作界面中创建和拖动物体,这篇博客跟随的视频链接如下: B 站视频:s03-创建和移动物体 如果你不打算开之前的博客并且对UE5 比较熟的话按照以…...
蓝桥杯 2024 15届国赛 A组 儿童节快乐
P10576 [蓝桥杯 2024 国 A] 儿童节快乐 题目描述 五彩斑斓的气球在蓝天下悠然飘荡,轻快的音乐在耳边持续回荡,小朋友们手牵着手一同畅快欢笑。在这样一片安乐祥和的氛围下,六一来了。 今天是六一儿童节,小蓝老师为了让大家在节…...
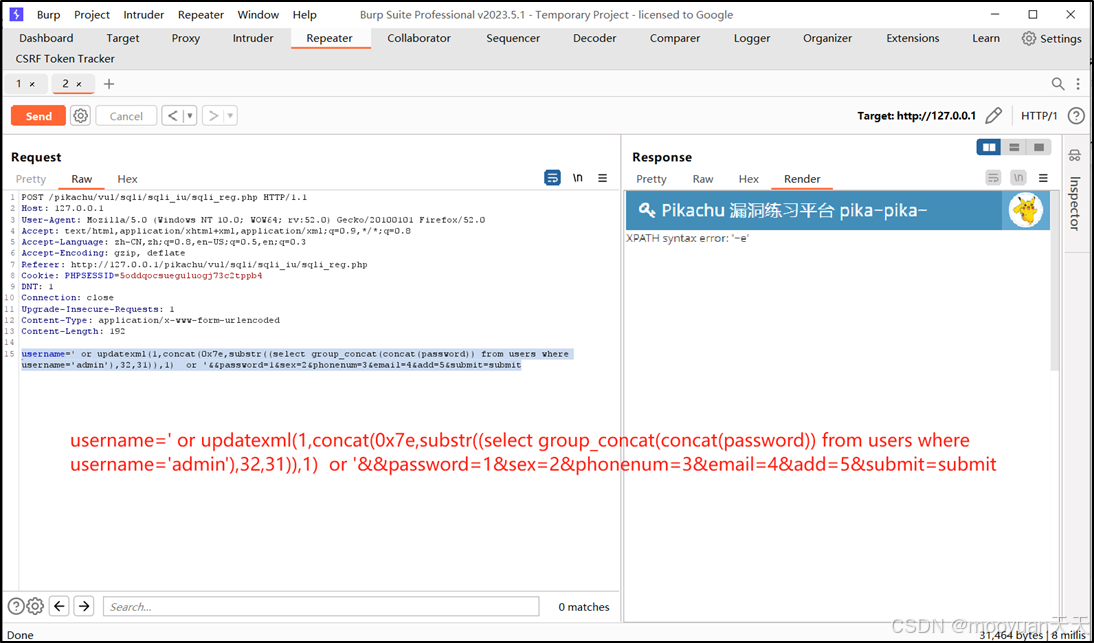
pikachu靶场通关笔记22-1 SQL注入05-1-insert注入(报错法)
目录 一、SQL注入 二、insert注入 三、报错型注入 四、updatexml函数 五、源码审计 六、insert渗透实战 1、渗透准备 2、获取数据库名database 3、获取表名table 4、获取列名column 5、获取字段 本系列为通过《pikachu靶场通关笔记》的SQL注入关卡(共10关࿰…...
React---day11
14.4 react-redux第三方库 提供connect、thunk之类的函数 以获取一个banner数据为例子 store: 我们在使用异步的时候理应是要使用中间件的,但是configureStore 已经自动集成了 redux-thunk,注意action里面要返回函数 import { configureS…...
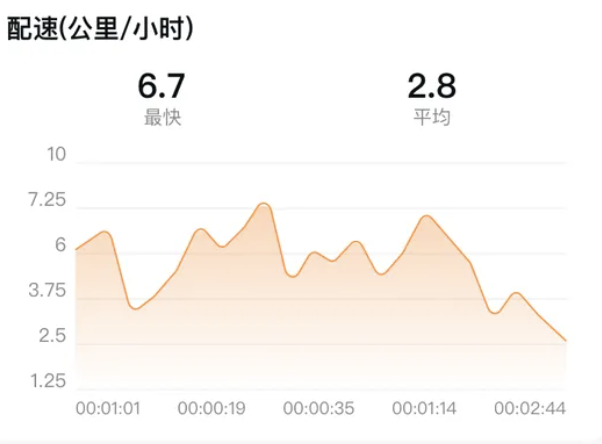
HarmonyOS运动开发:如何用mpchart绘制运动配速图表
##鸿蒙核心技术##运动开发##Sensor Service Kit(传感器服务)# 前言 在运动类应用中,运动数据的可视化是提升用户体验的重要环节。通过直观的图表展示运动过程中的关键数据,如配速、距离、卡路里消耗等,用户可以更清晰…...
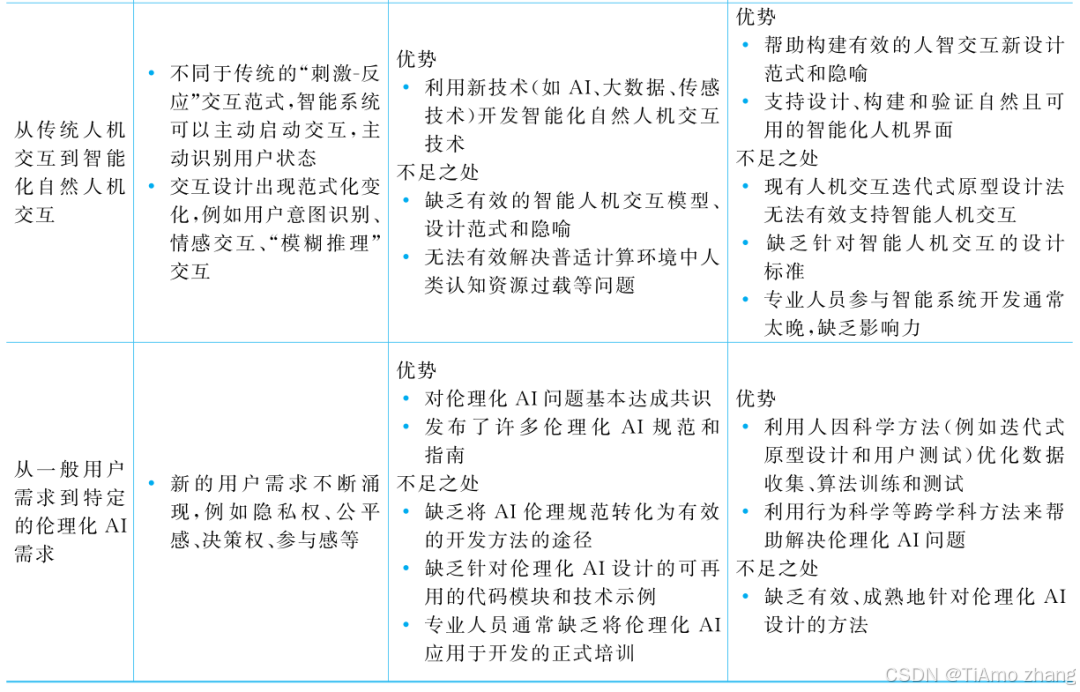
人机融合智能 | “人智交互”跨学科新领域
本文系统地提出基于“以人为中心AI(HCAI)”理念的人-人工智能交互(人智交互)这一跨学科新领域及框架,定义人智交互领域的理念、基本理论和关键问题、方法、开发流程和参与团队等,阐述提出人智交互新领域的意义。然后,提出人智交互研究的三种新范式取向以及它们的意义。最后,总结…...