YOLOv8-Pose推理详解及部署实现
目录
- 前言
- 一、YOLOv8-Pose推理(Python)
- 1. YOLOv8-Pose预测
- 2. YOLOv8-Pose预处理
- 3. YOLOv8-Pose后处理
- 4. YOLOv8-Pose推理
- 二、YOLOv8-Pose推理(C++)
- 1. ONNX导出
- 2. YOLOv8-Pose预处理
- 3. YOLOv8-Pose后处理
- 4. YOLOv8-Pose推理
- 三、YOLOv8-Pose部署
- 1. 源码下载
- 2. 环境配置
- 2.1 配置CMakeLists.txt
- 2.2 配置Makefile
- 3. ONNX导出
- 4. 源码修改
- 5. 运行
- 结语
- 下载链接
- 参考
前言
梳理下 YOLOv8-Pose 的预处理和后处理流程,顺便让 tensorRT_Pro 支持 YOLOv8-Pose
参考:https://github.com/shouxieai/tensorRT_Pro
实现:https://github.com/Melody-Zhou/tensorRT_Pro-YOLOv8
一、YOLOv8-Pose推理(Python)
1. YOLOv8-Pose预测
我们先尝试利用官方预训练权重来推理一张图片并保存,看能否成功
在 YOLOv8 主目录下新建 predict-pose.py 预测文件,其内容如下:
import cv2
import numpy as np
from ultralytics import YOLOdef hsv2bgr(h, s, v):h_i = int(h * 6)f = h * 6 - h_ip = v * (1 - s)q = v * (1 - f * s)t = v * (1 - (1 - f) * s)r, g, b = 0, 0, 0if h_i == 0:r, g, b = v, t, pelif h_i == 1:r, g, b = q, v, pelif h_i == 2:r, g, b = p, v, telif h_i == 3:r, g, b = p, q, velif h_i == 4:r, g, b = t, p, velif h_i == 5:r, g, b = v, p, qreturn int(b * 255), int(g * 255), int(r * 255)def random_color(id):h_plane = (((id << 2) ^ 0x937151) % 100) / 100.0s_plane = (((id << 3) ^ 0x315793) % 100) / 100.0return hsv2bgr(h_plane, s_plane, 1)skeleton = [[16, 14], [14, 12], [17, 15], [15, 13], [12, 13], [6, 12], [7, 13], [6, 7], [6, 8], [7, 9], [8, 10], [9, 11], [2, 3], [1, 2], [1, 3], [2, 4], [3, 5], [4, 6], [5, 7]]
pose_palette = np.array([[255, 128, 0], [255, 153, 51], [255, 178, 102], [230, 230, 0], [255, 153, 255],[153, 204, 255], [255, 102, 255], [255, 51, 255], [102, 178, 255], [51, 153, 255],[255, 153, 153], [255, 102, 102], [255, 51, 51], [153, 255, 153], [102, 255, 102],[51, 255, 51], [0, 255, 0], [0, 0, 255], [255, 0, 0], [255, 255, 255]],dtype=np.uint8)
kpt_color = pose_palette[[16, 16, 16, 16, 16, 0, 0, 0, 0, 0, 0, 9, 9, 9, 9, 9, 9]]
limb_color = pose_palette[[9, 9, 9, 9, 7, 7, 7, 0, 0, 0, 0, 0, 16, 16, 16, 16, 16, 16, 16]]if __name__ == "__main__":model = YOLO("yolov8s-pose.pt")img = cv2.imread("ultralytics/assets/bus.jpg")results = model(img)[0]names = results.namesboxes = results.boxes.data.tolist()# keypoints.data.shape -> n,17,3keypoints = results.keypoints.cpu().numpy()# keypoint -> 每个人的关键点for keypoint in keypoints.data:for i, (x, y, conf) in enumerate(keypoint):color_k = [int(x) for x in kpt_color[i]]if conf < 0.5:continueif x != 0 and y != 0:cv2.circle(img, (int(x), int(y)), 5, color_k , -1, lineType=cv2.LINE_AA)for i, sk in enumerate(skeleton):pos1 = (int(keypoint[(sk[0] - 1), 0]), int(keypoint[(sk[0] - 1), 1]))pos2 = (int(keypoint[(sk[1] - 1), 0]), int(keypoint[(sk[1] - 1), 1]))conf1 = keypoint[(sk[0] - 1), 2]conf2 = keypoint[(sk[1] - 1), 2]if conf1 < 0.5 or conf2 < 0.5:continueif pos1[0] == 0 or pos1[1] == 0 or pos2[0] == 0 or pos2[1] == 0:continuecv2.line(img, pos1, pos2, [int(x) for x in limb_color[i]], thickness=2, lineType=cv2.LINE_AA)for obj in boxes:left, top, right, bottom = int(obj[0]), int(obj[1]), int(obj[2]), int(obj[3])confidence = obj[4]label = int(obj[5])color = random_color(label)cv2.rectangle(img, (left, top), (right, bottom), color = color ,thickness=2, lineType=cv2.LINE_AA)caption = f"{names[label]} {confidence:.2f}"w, h = cv2.getTextSize(caption, 0, 1, 2)[0]cv2.rectangle(img, (left - 3, top - 33), (left + w + 10, top), color, -1)cv2.putText(img, caption, (left, top - 5), 0, 1, (0, 0, 0), 2, 16)cv2.imwrite("predict-pose.jpg", img)print("save done")
在上述代码中我们通过 opencv 读取了一张图像,并送入模型中推理得到输出 results,results 中保存着不同任务的结果,我们这里是姿态点估计任务,因此只需要拿到对应的 boxes 和 keypoints 即可。
拿到 boxes 后我们就可以将对应的框和置信度绘制在图像上,拿到 keypoints 后我们就可以将对应的人体 17 个关键点绘制在图像上并保存。
关于 boxes 可视化的代码实现参考自 tensorRT_Pro 中的实现,可以参考:app_yolo.cpp#L95
关于 keypoints 可视化的代码实现参考自 ultralytics/utils/plotting.py 中的实现,可以参考:plotting.py#L171
关于随机颜色的代码实现参考自 tensorRT_Pro 中的实现,可以参考:ilogger.cpp#L90
模型推理保存的结果图像如下所示:
2. YOLOv8-Pose预处理
模型预测成功后我们就需要自己动手来写下 YOLOv8-Pose 的预处理和后处理,方便后续在 C++ 上的实现,我们先来看看预处理的实现
经过我们的调试分析可知 YOLOv8-Pose 的预处理过程在 ultralytics/engine/predictor.py 文件中,可以参考:predictor.py#L111
代码如下:
def preprocess(self, im):"""Prepares input image before inference.Args:im (torch.Tensor | List(np.ndarray)): BCHW for tensor, [(HWC) x B] for list."""not_tensor = not isinstance(im, torch.Tensor)if not_tensor:im = np.stack(self.pre_transform(im))im = im[..., ::-1].transpose((0, 3, 1, 2)) # BGR to RGB, BHWC to BCHW, (n, 3, h, w)im = np.ascontiguousarray(im) # contiguousim = torch.from_numpy(im)im = im.to(self.device)im = im.half() if self.model.fp16 else im.float() # uint8 to fp16/32if not_tensor:im /= 255 # 0 - 255 to 0.0 - 1.0return im
它包含以下步骤:
- self.pre_transform:即 letterbox 添加灰条
- im[…,::-1]:BGR → RGB
- transpose((0, 3, 1, 2)):添加 batch 维度,HWC → CHW
- torch.from_numpy:to Tensor
- im /= 255:除以 255,归一化
大家如果对 YOLOv5 的预处理熟悉的话,会发现 YOLOv8-Pose 的预处理和 YOLOv5 的预处理一模一样,因此我们不难写出对应的预处理代码,如下所示:
def preprocess_warpAffine(image, dst_width=640, dst_height=640):scale = min((dst_width / image.shape[1], dst_height / image.shape[0]))ox = (dst_width - scale * image.shape[1]) / 2oy = (dst_height - scale * image.shape[0]) / 2M = np.array([[scale, 0, ox],[0, scale, oy]], dtype=np.float32)img_pre = cv2.warpAffine(image, M, (dst_width, dst_height), flags=cv2.INTER_LINEAR,borderMode=cv2.BORDER_CONSTANT, borderValue=(114, 114, 114))IM = cv2.invertAffineTransform(M)img_pre = (img_pre[...,::-1] / 255.0).astype(np.float32)img_pre = img_pre.transpose(2, 0, 1)[None]img_pre = torch.from_numpy(img_pre)return img_pre, IM
其中的 letterbox 添加灰条步骤我们可以通过仿射变换 warpAffine 实现,warpAffine 非常适合在 CUDA 上加速,关于 warpAffine 仿射变换的细节大家可以参考 YOLOv5推理详解及预处理高性能实现,这边不再赘述。其它步骤倒是和官方的没有区别。
值得注意得是,letterbox 的操作是先将长边缩放到 640,再将短边按比例缩放,同时确保缩放后的短边能整除 32,如果不能则向上取整多余部分填充。warpAffine 的操作则是将图像分辨率固定在 640x640,多余部分添加灰条,博主对一张 1080x810 分辨率的图像经过两种不同预处理后的结果进行了对比,如下图所示:
可以看到二者明显的差别,letterbox 中没有灰条,因为长边缩放到 640 后短边刚好缩放到 480,能整除 32。而 warpAffine 则是固定分辨率 640x640,因此短边多余部分将用灰条填充。
warpAffine 预处理方法将图像分辨率固定在 640x640,主要有以下几点考虑:(from chatGPT)
- 简化处理逻辑:所有预处理后的图像分辨率相同,可以简化 CUDA 中并行处理的逻辑,使得代码更易于编写和维护。
- 优化内存访问:在 GPU 上,连续的内存访问模式通常比非连续的访问更高效。如果所有图像具有相同的大小和布局,这可以帮助优化内存访问,提高处理速度。
- 避免动态内存分配:动态内存分配和释放是昂贵的操作,特别是在 GPU 上。固定分辨率意味着可以预先分配足够的内存,而不需要根据每个图像的大小动态调整内存大小。
这两种不同的预处理方法生成的图片输入到神经网络时的维度不同,letterbox 的输入是 torch.Size([1, 3, 640, 480]),warpAffine 的输入是 torch.Size([1, 3, 640, 640])。由于输入维度不同将导致模型输出维度的差异,leetrbox 的输出是 torch.Size([1, 56, 6300]) 只有 6300 个框,而 warpAffine 的输出是 torch.Size([1, 56, 8400]) 有 8400 个框,这点大家需要清楚。
3. YOLOv8-Pose后处理
我们再来看看后处理的实现
经过我们的调试分析可知 YOLOv8-Pose 的后处理过程在 ultralytics/models/yolo/pose/predict.py 文件中,可以参考:pose/predict.py#L31
class PosePredictor(DetectionPredictor):"""A class extending the DetectionPredictor class for prediction based on a pose model.Example:```pythonfrom ultralytics.utils import ASSETSfrom ultralytics.models.yolo.pose import PosePredictorargs = dict(model='yolov8n-pose.pt', source=ASSETS)predictor = PosePredictor(overrides=args)predictor.predict_cli()"""def __init__(self, cfg=DEFAULT_CFG, overrides=None, _callbacks=None):"""Initializes PosePredictor, sets task to 'pose' and logs a warning for using 'mps' as device."""super().__init__(cfg, overrides, _callbacks)self.args.task = 'pose'if isinstance(self.args.device, str) and self.args.device.lower() == 'mps':LOGGER.warning("WARNING ⚠️ Apple MPS known Pose bug. Recommend 'device=cpu' for Pose models. "'See https://github.com/ultralytics/ultralytics/issues/4031.')def postprocess(self, preds, img, orig_imgs):"""Return detection results for a given input image or list of images."""preds = ops.non_max_suppression(preds,self.args.conf,self.args.iou,agnostic=self.args.agnostic_nms,max_det=self.args.max_det,classes=self.args.classes,nc=len(self.model.names))if not isinstance(orig_imgs, list): # input images are a torch.Tensor, not a listorig_imgs = ops.convert_torch2numpy_batch(orig_imgs)results = []for i, pred in enumerate(preds):orig_img = orig_imgs[i]pred[:, :4] = ops.scale_boxes(img.shape[2:], pred[:, :4], orig_img.shape).round()pred_kpts = pred[:, 6:].view(len(pred), *self.model.kpt_shape) if len(pred) else pred[:, 6:]pred_kpts = ops.scale_coords(img.shape[2:], pred_kpts, orig_img.shape)img_path = self.batch[0][i]results.append(Results(orig_img, path=img_path, names=self.model.names, boxes=pred[:, :6], keypoints=pred_kpts))return results
它包含以下步骤:
- ops.non_max_suppression:非极大值抑制,即 NMS
- ops.scale_boxes:框的解码,即 decode boxes
- ops.scale_coords:关键点的解码,即 decode keypoints
大家如果对 YOLOv5 的后处理熟悉的话,会发现 YOLOv8-Pose 的后处理中检测框的处理和 YOLOv5 中的基本一样,只是需要大家额外处理下关键点,因此我们不难写出对应的后处理代码,如下所示:
def iou(box1, box2):def area_box(box):return (box[2] - box[0]) * (box[3] - box[1])left, top = max(box1[:2], box2[:2])right, bottom = min(box1[2:4], box2[2:4])union = max((right - left), 0) * max((bottom - top), 0)cross = area_box(box1) + area_box(box2) - unionif cross == 0 or union == 0:return 0return union / crossdef NMS(boxes, iou_thres):remove_flags = [False] * len(boxes)keep_boxes = []for i, ibox in enumerate(boxes):if remove_flags[i]:continuekeep_boxes.append(ibox)for j in range(i + 1, len(boxes)):if remove_flags[j]:continuejbox = boxes[j]if iou(ibox, jbox) > iou_thres:remove_flags[j] = Truereturn keep_boxesdef postprocess(pred, IM=[], conf_thres=0.25, iou_thres=0.45):# 输入是模型推理的结果,即8400个预测框# 1,8400,56 [cx,cy,w,h,conf,17*3]boxes = []for img_id, box_id in zip(*np.where(pred[...,4] > conf_thres)):item = pred[img_id, box_id]cx, cy, w, h, conf = item[:5]left = cx - w * 0.5top = cy - h * 0.5right = cx + w * 0.5bottom = cy + h * 0.5keypoints = item[5:].reshape(-1, 3)keypoints[:, 0] = keypoints[:, 0] * IM[0][0] + IM[0][2]keypoints[:, 1] = keypoints[:, 1] * IM[1][1] + IM[1][2]boxes.append([left, top, right, bottom, conf, *keypoints.reshape(-1).tolist()])boxes = np.array(boxes)lr = boxes[:,[0, 2]]tb = boxes[:,[1, 3]]boxes[:,[0,2]] = IM[0][0] * lr + IM[0][2]boxes[:,[1,3]] = IM[1][1] * tb + IM[1][2]boxes = sorted(boxes.tolist(), key=lambda x:x[4], reverse=True)return NMS(boxes, iou_thres)
其中预测框的解码我们是通过仿射变换逆矩阵 IM 实现的,关于 IM 的细节大家可以参考 YOLOv5推理详解及预处理高性能实现,这边不再赘述。关于 NMS 的代码参考自 tensorRT_Pro 中的实现:yolo.cpp#L119
关键点的解码我们同样可以通过 IM 将其映射回原图上,因此 YOLOv8-Pose 的后处理和 YOLOv5 的基本上没什么区别,只是需要大家清楚模型预测的结果中每个维度所代表的含义即可
对于一张 640x640 的图片来说,YOLOv8-Pose 预测框的总数量是 8400,每个预测框的维度是 56(针对 COCO 数据集的人体 17 个关键点而言)
8400 × 56 = 80 × 80 × 56 + 40 × 40 × 56 + 20 × 20 × 56 = 80 × 80 × ( 5 + 51 ) + 40 × 40 × ( 5 + 51 ) + 20 × 20 × ( 5 + 51 ) = 80 × 80 × ( 5 + 17 × 3 ) + 40 × 40 × ( 5 + 17 × 3 ) + 20 × 20 × ( 5 + 17 × 3 ) \begin{aligned} 8400\times56&=80\times80\times56+40\times40\times56+20\times20\times56\\ &=80\times80\times(5+51)+40\times40\times(5+51)+20\times20\times(5+51)\\ &=80\times80\times(5+17\times3)+40\times40\times(5+17\times3)+20\times20\times(5+17\times3)\\ \end{aligned} 8400×56=80×80×56+40×40×56+20×20×56=80×80×(5+51)+40×40×(5+51)+20×20×(5+51)=80×80×(5+17×3)+40×40×(5+17×3)+20×20×(5+17×3)
其中的 5 对应的是 cx, cy, w, h, conf,分别代表的含义是边界框中心点坐标、宽高以及置信度;17 对应的是 COCO 数据集中的人体 17 个关键点,3 代表每个关键点的信息,包括 x, y, visibility,分别代表的含义是关键点的 x 和 y 坐标以及可见性或者说置信度,在对关键点进行可视化时我们只会可视化那些 visibility 大于 0.5 的关键点,因为低于 0.5 的关键点我们认为它被遮挡或者不在图像上。
目前主流的姿态点估计算法分为两种,一种是 top-down 自顶向下,先检测出图像中所有的人体检测框,再根据每个检测框识别姿态;另一种是 bottom-up 自低向上,先检测出图像中所有的骨骼点,再通过拼接得到多个人的骨架。两种方法各有优缺点,其中自顶向上的方法,姿态检测的准确度非常依赖目标检测框的质量;而自低向上的方法,如果两人离得非常近,容易出现模棱两可的情况,而且由于是依赖两个骨骼点之间的关系,所以失去了对全局的信息获取。
像 AlphaPose 和 YOLOv8-Pose 模型都是采用的自顶向下的方法,即先检测出所有的人体框再对每个人体做姿态估计。
4. YOLOv8-Pose推理
通过上面对 YOLOv8-Pose 的预处理和后处理分析之后,整个推理过程就显而易见了。YOLOv8-Pose 的推理包括图像预处理、模型推理、预测结果后处理三部分,其中预处理主要包括 warpAffine 仿射变换,后处理主要包括 boxes、keypoints 的 decode 解码和 NMS 两部分。
完整的推理代码如下:
import cv2
import torch
import numpy as np
from ultralytics.data.augment import LetterBox
from ultralytics.nn.autobackend import AutoBackenddef preprocess_letterbox(image):letterbox = LetterBox(new_shape=640, stride=32, auto=True)image = letterbox(image=image)image = (image[..., ::-1] / 255.0).astype(np.float32) # BGR to RGB, 0 - 255 to 0.0 - 1.0image = image.transpose(2, 0, 1)[None] # BHWC to BCHW (n, 3, h, w)image = torch.from_numpy(image)return imagedef preprocess_warpAffine(image, dst_width=640, dst_height=640):scale = min((dst_width / image.shape[1], dst_height / image.shape[0]))ox = (dst_width - scale * image.shape[1]) / 2oy = (dst_height - scale * image.shape[0]) / 2M = np.array([[scale, 0, ox],[0, scale, oy]], dtype=np.float32)img_pre = cv2.warpAffine(image, M, (dst_width, dst_height), flags=cv2.INTER_LINEAR,borderMode=cv2.BORDER_CONSTANT, borderValue=(114, 114, 114))IM = cv2.invertAffineTransform(M)img_pre = (img_pre[...,::-1] / 255.0).astype(np.float32)img_pre = img_pre.transpose(2, 0, 1)[None]img_pre = torch.from_numpy(img_pre)return img_pre, IMdef iou(box1, box2):def area_box(box):return (box[2] - box[0]) * (box[3] - box[1])left, top = max(box1[:2], box2[:2])right, bottom = min(box1[2:4], box2[2:4])union = max((right-left), 0) * max((bottom-top), 0)cross = area_box(box1) + area_box(box2) - unionif cross == 0 or union == 0:return 0return union / crossdef NMS(boxes, iou_thres):remove_flags = [False] * len(boxes)keep_boxes = []for i, ibox in enumerate(boxes):if remove_flags[i]:continuekeep_boxes.append(ibox)for j in range(i + 1, len(boxes)):if remove_flags[j]:continuejbox = boxes[j]if iou(ibox, jbox) > iou_thres:remove_flags[j] = Truereturn keep_boxesdef postprocess(pred, IM=[], conf_thres=0.25, iou_thres=0.45):# 输入是模型推理的结果,即8400个预测框# 1,8400,56 [cx,cy,w,h,conf,17*3]boxes = []for img_id, box_id in zip(*np.where(pred[...,4] > conf_thres)):item = pred[img_id, box_id]cx, cy, w, h, conf = item[:5]left = cx - w * 0.5top = cy - h * 0.5right = cx + w * 0.5bottom = cy + h * 0.5keypoints = item[5:].reshape(-1, 3)keypoints[:, 0] = keypoints[:, 0] * IM[0][0] + IM[0][2]keypoints[:, 1] = keypoints[:, 1] * IM[1][1] + IM[1][2]boxes.append([left, top, right, bottom, conf, *keypoints.reshape(-1).tolist()])boxes = np.array(boxes)lr = boxes[:,[0, 2]]tb = boxes[:,[1, 3]]boxes[:,[0,2]] = IM[0][0] * lr + IM[0][2]boxes[:,[1,3]] = IM[1][1] * tb + IM[1][2]boxes = sorted(boxes.tolist(), key=lambda x:x[4], reverse=True)return NMS(boxes, iou_thres)def hsv2bgr(h, s, v):h_i = int(h * 6)f = h * 6 - h_ip = v * (1 - s)q = v * (1 - f * s)t = v * (1 - (1 - f) * s)r, g, b = 0, 0, 0if h_i == 0:r, g, b = v, t, pelif h_i == 1:r, g, b = q, v, pelif h_i == 2:r, g, b = p, v, telif h_i == 3:r, g, b = p, q, velif h_i == 4:r, g, b = t, p, velif h_i == 5:r, g, b = v, p, qreturn int(b * 255), int(g * 255), int(r * 255)def random_color(id):h_plane = (((id << 2) ^ 0x937151) % 100) / 100.0s_plane = (((id << 3) ^ 0x315793) % 100) / 100.0return hsv2bgr(h_plane, s_plane, 1)skeleton = [[16, 14], [14, 12], [17, 15], [15, 13], [12, 13], [6, 12], [7, 13], [6, 7], [6, 8], [7, 9], [8, 10], [9, 11], [2, 3], [1, 2], [1, 3], [2, 4], [3, 5], [4, 6], [5, 7]]
pose_palette = np.array([[255, 128, 0], [255, 153, 51], [255, 178, 102], [230, 230, 0], [255, 153, 255],[153, 204, 255], [255, 102, 255], [255, 51, 255], [102, 178, 255], [51, 153, 255],[255, 153, 153], [255, 102, 102], [255, 51, 51], [153, 255, 153], [102, 255, 102],[51, 255, 51], [0, 255, 0], [0, 0, 255], [255, 0, 0], [255, 255, 255]],dtype=np.uint8)
kpt_color = pose_palette[[16, 16, 16, 16, 16, 0, 0, 0, 0, 0, 0, 9, 9, 9, 9, 9, 9]]
limb_color = pose_palette[[9, 9, 9, 9, 7, 7, 7, 0, 0, 0, 0, 0, 16, 16, 16, 16, 16, 16, 16]]if __name__ == "__main__":img = cv2.imread("ultralytics/assets/bus.jpg")# img = preprocess_letterbox(img)img_pre, IM = preprocess_warpAffine(img)model = AutoBackend(weights="yolov8s-pose.pt")names = model.namesresult = model(img_pre)[0].transpose(-1, -2) # 1,8400,56boxes = postprocess(result, IM)for box in boxes:left, top, right, bottom = int(box[0]), int(box[1]), int(box[2]), int(box[3])confidence = box[4]label = 0color = random_color(label)cv2.rectangle(img, (left, top), (right, bottom), color, 2, cv2.LINE_AA)caption = f"{names[label]} {confidence:.2f}"w, h = cv2.getTextSize(caption, 0, 1, 2)[0]cv2.rectangle(img, (left - 3, top - 33), (left + w + 10, top), color, -1)cv2.putText(img, caption, (left, top - 5), 0, 1, (0, 0, 0), 2, 16)keypoints = box[5:]keypoints = np.array(keypoints).reshape(-1, 3)for i, keypoint in enumerate(keypoints):x, y, conf = keypointcolor_k = [int(x) for x in kpt_color[i]]if conf < 0.5:continueif x != 0 and y != 0:cv2.circle(img, (int(x), int(y)), 5, color_k, -1, lineType=cv2.LINE_AA)for i, sk in enumerate(skeleton):pos1 = (int(keypoints[(sk[0] - 1), 0]), int(keypoints[(sk[0] - 1), 1]))pos2 = (int(keypoints[(sk[1] - 1), 0]), int(keypoints[(sk[1] - 1), 1]))conf1 = keypoints[(sk[0] - 1), 2]conf2 = keypoints[(sk[1] - 1), 2]if conf1 < 0.5 or conf2 < 0.5:continueif pos1[0] == 0 or pos1[1] == 0 or pos2[0] == 0 or pos2[1] == 0:continuecv2.line(img, pos1, pos2, [int(x) for x in limb_color[i]], thickness=2, lineType=cv2.LINE_AA)cv2.imwrite("infer-pose.jpg", img)print("save done")
推理效果如下图所示:
至此,我们在 Python 上面完成了 YOLOv8-Pose 的整个推理过程,下面我们去 C++ 上实现。
二、YOLOv8-Pose推理(C++)
C++ 上的实现我们使用的 repo 依旧是 tensorRT_Pro,现在我们就基于 tensorRT_Pro 完成 YOLOv8-Pose 在 C++ 上的推理。
1. ONNX导出
首先我们需要将 YOLOv8-Pose 模型导出为 ONNX,为了适配 tensorRT_Pro 我们需要做一些修改,主要有以下几点:
- 修改输出节点名为 output,输入输出只让 batch 维度动态,宽高不动态
- 增加 transpose 节点交换输出的 2、3 维度
具体修改如下:
1. 在 ultralytics/engine/exporter.py 文件中改动一处
- 323 行:输出节点名修改为 output
- 326 行:输入只让 batch 维度动态,宽高不动态
- 327 行:输出只让 batch 维度动态,宽高不动态
# ========== exporter.py ==========# ultralytics/engine/exporter.py第323行
# output_names = ['output0', 'output1'] if isinstance(self.model, SegmentationModel) else ['output0']
# dynamic = self.args.dynamic
# if dynamic:
# dynamic = {'images': {0: 'batch', 2: 'height', 3: 'width'}} # shape(1,3,640,640)
# if isinstance(self.model, SegmentationModel):
# dynamic['output0'] = {0: 'batch', 2: 'anchors'} # shape(1, 116, 8400)
# dynamic['output1'] = {0: 'batch', 2: 'mask_height', 3: 'mask_width'} # shape(1,32,160,160)
# elif isinstance(self.model, DetectionModel):
# dynamic['output0'] = {0: 'batch', 2: 'anchors'} # shape(1, 84, 8400)
# 修改为:output_names = ['output0', 'output1'] if isinstance(self.model, SegmentationModel) else ['output']
dynamic = self.args.dynamic
if dynamic:dynamic = {'images': {0: 'batch'}} # shape(1,3,640,640)dynamic['output'] = {0: 'batch'}if isinstance(self.model, SegmentationModel):dynamic['output0'] = {0: 'batch', 2: 'anchors'} # shape(1, 116, 8400)dynamic['output1'] = {0: 'batch', 2: 'mask_height', 3: 'mask_width'} # shape(1,32,160,160)elif isinstance(self.model, DetectionModel):dynamic['output0'] = {0: 'batch', 2: 'anchors'} # shape(1, 84, 8400)
2. 在 ultralytics/nn/modules/head.py 文件中改动一处
- 130 行:添加 transpose 节点交换第 2 和第 3 维度
# ========== head.py ==========# ultralytics/nn/modules/head.py第130行,forward函数
# return torch.cat([x, pred_kpt], 1) if self.export else (torch.cat([x[0], pred_kpt], 1), (x[1], kpt))
# 修改为:return torch.cat([x, pred_kpt], 1).permute(0, 2, 1) if self.export else (torch.cat([x[0], pred_kpt], 1), (x[1], kpt))
以上就是为了适配 tensorRT_Pro 而做出的代码修改,修改好以后,将预训练权重 yolov8s-pose.pt 放在 ultralytics-main 主目录下,新建导出文件 export.py,内容如下:
from ultralytics import YOLOmodel = YOLO("yolov8s-pose.pt")success = model.export(format="onnx", dynamic=True, simplify=True)
在终端执行如下指令即可完成 onnx 导出:
python export.py
导出过程如下图所示:
可以看到导出的 pytorch 模型的输入 shape 是 1x3x640x640,输出 shape 是 1x8400x56,符合我们的预期。
导出成功后会在当前目录下生成 yolov8s-pose.onnx 模型,我们可以使用 Netron 可视化工具查看,如下图所示:
可以看到输入节点名是 images, 维度是 batchx3x640x640,保证只有 batch 维度动态,输出节点名是 output,维度是 batchxTransposeoutput_dim_1xTransposeoutput_dim_2,保证只有 batch 维度动态,符合 tensorRT_Pro 的格式。
大家不要看到 Transposeoutput_dim_1 和 Transposeoutput_dim_2 就认为这也是动态的,其实输出节点的维度是根据输入节点的维度和模型的结构生成的,而额外的维度 Transposeoutput_dim_1 和 Transposeoutput_dim_2 可能是由模型结构中某些操作决定的,如通道数变换(Transpose)操作的输出维度,而不是由动态维度决定的。因此,通常情况下,这些维度是静态的,不会在推理时改变。
2. YOLOv8-Pose预处理
之前有提到过 YOLOv8-Pose 的预处理部分和 YOLOv5 实现一模一样,因此我们在 tensorRT_Pro 中 YOLOv8-Pose 模型的预处理可以直接使用 YOLOv5 的预处理。
tensorRT_Pro 中预处理的代码如下:
__global__ void warp_affine_bilinear_and_normalize_plane_kernel(uint8_t* src, int src_line_size, int src_width, int src_height, float* dst, int dst_width, int dst_height, uint8_t const_value_st, float* warp_affine_matrix_2_3, Norm norm, int edge){int position = blockDim.x * blockIdx.x + threadIdx.x;if (position >= edge) return;float m_x1 = warp_affine_matrix_2_3[0];float m_y1 = warp_affine_matrix_2_3[1];float m_z1 = warp_affine_matrix_2_3[2];float m_x2 = warp_affine_matrix_2_3[3];float m_y2 = warp_affine_matrix_2_3[4];float m_z2 = warp_affine_matrix_2_3[5];int dx = position % dst_width;int dy = position / dst_width;float src_x = m_x1 * dx + m_y1 * dy + m_z1;float src_y = m_x2 * dx + m_y2 * dy + m_z2;float c0, c1, c2;if(src_x <= -1 || src_x >= src_width || src_y <= -1 || src_y >= src_height){// out of rangec0 = const_value_st;c1 = const_value_st;c2 = const_value_st;}else{int y_low = floorf(src_y);int x_low = floorf(src_x);int y_high = y_low + 1;int x_high = x_low + 1;uint8_t const_value[] = {const_value_st, const_value_st, const_value_st};float ly = src_y - y_low;float lx = src_x - x_low;float hy = 1 - ly;float hx = 1 - lx;float w1 = hy * hx, w2 = hy * lx, w3 = ly * hx, w4 = ly * lx;uint8_t* v1 = const_value;uint8_t* v2 = const_value;uint8_t* v3 = const_value;uint8_t* v4 = const_value;if(y_low >= 0){if (x_low >= 0)v1 = src + y_low * src_line_size + x_low * 3;if (x_high < src_width)v2 = src + y_low * src_line_size + x_high * 3;}if(y_high < src_height){if (x_low >= 0)v3 = src + y_high * src_line_size + x_low * 3;if (x_high < src_width)v4 = src + y_high * src_line_size + x_high * 3;}// same to opencvc0 = floorf(w1 * v1[0] + w2 * v2[0] + w3 * v3[0] + w4 * v4[0] + 0.5f);c1 = floorf(w1 * v1[1] + w2 * v2[1] + w3 * v3[1] + w4 * v4[1] + 0.5f);c2 = floorf(w1 * v1[2] + w2 * v2[2] + w3 * v3[2] + w4 * v4[2] + 0.5f);}if(norm.channel_type == ChannelType::Invert){float t = c2;c2 = c0; c0 = t;}if(norm.type == NormType::MeanStd){c0 = (c0 * norm.alpha - norm.mean[0]) / norm.std[0];c1 = (c1 * norm.alpha - norm.mean[1]) / norm.std[1];c2 = (c2 * norm.alpha - norm.mean[2]) / norm.std[2];}else if(norm.type == NormType::AlphaBeta){c0 = c0 * norm.alpha + norm.beta;c1 = c1 * norm.alpha + norm.beta;c2 = c2 * norm.alpha + norm.beta;}int area = dst_width * dst_height;float* pdst_c0 = dst + dy * dst_width + dx;float* pdst_c1 = pdst_c0 + area;float* pdst_c2 = pdst_c1 + area;*pdst_c0 = c0;*pdst_c1 = c1;*pdst_c2 = c2;
}
关于预处理部分其实就是调用了上述 CUDA 核函数来实现 warpAffine,由于在 CUDA 中我们是对每个像素进行操作,因此非常容易实现 BGR → RGB,/255.0 等操作。关于代码的具体分析可以参考 YOLOv5推理详解及预处理高性能实现,这边不再赘述。
3. YOLOv8-Pose后处理
之前有提到过 YOLOv8-Pose 的检测框后处理部分和 YOLOv5 相同,只是需要添加关键点的解码即可,因此我们可以借鉴 YOLOv5 中 decode 解码部分的实现,添加关键点部分的解码即可,代码可参考:yolo_decode.cu#L13
因此我们不难写出 YOLOv8-Pose 的 decode 解码部分的实现代码,如下所示:
static __global__ void decode_kernel_v8_Pose(float *predict, int num_bboxes, float confidence_threshold, float* invert_affine_matrix, float* parray, int MAX_IMAGE_BOXES){int position = blockDim.x * blockIdx.x + threadIdx.x;if(position >= num_bboxes) return;float* pitem = predict + (5 + 3 * NUM_KEYPOINTS) * position;float cx = *pitem++;float cy = *pitem++;float width = *pitem++;float height = *pitem++;float confidence = *pitem++;if(confidence < confidence_threshold)return;int index = atomicAdd(parray, 1);if(index >= MAX_IMAGE_BOXES)return;float left = cx - width * 0.5f;float top = cy - height * 0.5f;float right = cx + width * 0.5f; float bottom = cy + height * 0.5f;affine_project(invert_affine_matrix, left, top, &left, &top);affine_project(invert_affine_matrix, right, bottom, &right, &bottom);float* pout_item = parray + 1 + index * NUM_BOX_ELEMENT; *pout_item++ = left;*pout_item++ = top;*pout_item++ = right;*pout_item++ = bottom;*pout_item++ = confidence;*pout_item++ = 1; // 1 = keep, 0 = ignorefor(int i = 0; i < NUM_KEYPOINTS; ++i){float keypoint_x = *pitem++;float keypoint_y = *pitem++;float keypoint_confidence = *pitem++;affine_project(invert_affine_matrix, keypoint_x, keypoint_y, &keypoint_x, &keypoint_y);*pout_item++ = keypoint_x;*pout_item++ = keypoint_y;*pout_item++ = keypoint_confidence; }
}
关于 decode 的具体实现其实就是启动多个线程,每个线程处理一个框的解码,包括框坐标和关键点坐标的解码,我们会通过仿射变换逆矩阵 IM 将坐标映射回原图上的,关于 decode 代码的详细分析可参考 infer源码阅读之yolo.cu,这边不再赘述。
另外关于 NMS 部分,由于在 YOLOv8-Pose 模型中没有 label 类别标签维度,因此也需要适当调整,调整后的 NMS 代码如下:
static __global__ void nms_kernel_v8_Pose(float* bboxes, int max_objects, float threshold){int position = (blockDim.x * blockIdx.x + threadIdx.x);int count = min((int)*bboxes, max_objects);if (position >= count) return;// left, top, right, bottom, confidence, keepflag, (keypoint_x, keypoint_y, keypoint_confidence) * 17float* pcurrent = bboxes + 1 + position * NUM_BOX_ELEMENT;for(int i = 0; i < count; ++i){float* pitem = bboxes + 1 + i * NUM_BOX_ELEMENT;if(i == position) continue;if(pitem[4] >= pcurrent[4]){if(pitem[4] == pcurrent[4] && i < position)continue;float iou = box_iou(pcurrent[0], pcurrent[1], pcurrent[2], pcurrent[3],pitem[0], pitem[1], pitem[2], pitem[3]);if(iou > threshold){pcurrent[5] = 0; // 1=keep, 0=ignorereturn;}}}
}
关于 NMS 的具体实现也是启动多个线程,每个线程处理一个框,如果剩余框中的置信度大于当前线程中处理的框,则计算两个框的 IoU,通过 IoU 值判断是否保留该框。相比于 CPU 版的 NMS 应该是少套了一层循环,另外一层循环是通过 CUDA 上线程的并行操作处理的,代码参考自:yolo_decode.cu#L81
4. YOLOv8-Pose推理
通过上面对 YOLOv8-Pose 的预处理和后处理分析之后,整个推理过程就显而易见了。C++ 上 YOLOv8-Pose 的预处理部分可直接沿用 YOLOv5 的预处理,后处理中的 decode 解码和 NMS 部分需要简单修改。
我们在终端执行如下指令即可完成推理(注意!完整流程博主会在后续内容介绍,这边只是简单演示)
make yolo_pose
编译图解如下所示:
推理结果如下图所示:
至此,我们在 C++ 上面完成了 YOLOv8-Pose 的整个推理过程,下面我们将完整的走一遍流程。
三、YOLOv8-Pose部署
博主新建了一个仓库 tensorRT_Pro-YOLOv8,该仓库基于 shouxieai/tensorRT_Pro,并进行了调整以支持 YOLOv8 的各项任务,目前已支持分类、检测、分割、姿态点估计任务。
下面我们就来具体看看如何利用 tensorRT_Pro-YOLOv8 这个 repo 完成 YOLOv8-Pose 的推理。
1. 源码下载
tensorRT_Pro-YOLOv8 的代码可以直接从 GitHub 官网上下载,源码下载地址是 https://github.com/Melody-Zhou/tensorRT_Pro-YOLOv8,Linux 下代码克隆指令如下:
git clone https://github.com/Melody-Zhou/tensorRT_Pro-YOLOv8.git
也可手动点击下载,点击右上角的 Code
按键,将代码下载下来。至此整个项目就已经准备好了。也可以点击 here 下载博主准备好的源代码(注意代码下载于 2023/11/7 日,若有改动请参考最新)
2. 环境配置
需要使用的软件环境有 TensorRT、CUDA、cuDNN、OpenCV、Protobuf,所有软件环境的安装可以参考 Ubuntu20.04软件安装大全,这里不再赘述,需要各位看官自行配置好相关环境😄,外网访问较慢,这里提供下博主安装过程中的软件安装包下载链接 Baidu Drive【pwd:yolo】🚀🚀🚀
tensorRT_Pro-YOLOv8 提供 CMakeLists.txt 和 Makefile 两种方式编译,二者选一即可
2.1 配置CMakeLists.txt
主要修改五处
1. 修改第 13 行,修改 OpenCV 路径
set(OpenCV_DIR "/usr/local/include/opencv4")
2. 修改第 15 行,修改 CUDA 路径
set(CUDA_TOOLKIT_ROOT_DIR "/usr/local/cuda-11.6")
3. 修改第 16 行,修改 cuDNN 路径
set(CUDNN_DIR "/usr/local/cudnn8.4.0.27-cuda11.6")
4. 修改第 17 行,修改 tensorRT 路径
set(TENSORRT_DIR "/opt/TensorRT-8.4.1.5")
5. 修改第 20 行,修改 protobuf 路径
set(PROTOBUF_DIR "/home/jarvis/protobuf")
2.2 配置Makefile
主要修改五处
1. 修改第 4 行,修改 protobuf 路径
lean_protobuf := /home/jarvis/protobuf
2. 修改第 5 行,修改 tensorRT 路径
lean_tensor_rt := /opt/TensorRT-8.4.1.5
3. 修改第 6 行,修改 cuDNN 路径
lean_cudnn := /usr/local/cudnn8.4.0.27-cuda11.6
4. 修改第 7 行,修改 OpenCV 路径
lean_opencv := /usr/local
5. 修改第 8 行,修改 CUDA 路径
lean_cuda := /usr/local/cuda-11.6
3. ONNX导出
导出细节可以查看之前的内容,这边不再赘述。记得将导出的 ONNX 模型放在 tensorRT_Pro-YOLOv8/workspace 文件夹下。
4. 源码修改
如果你想推理自己训练的模型还需要修改下源代码,YOLOv8-Pose 模型的推理代码主要在 app_yolo_pose.cpp 文件中,我们就只需要修改这一个文件中的内容即可,源码修改较简单主要有以下几点:
- 1. app_yolo_pose.cpp 292行,“yolov8s-pose” 修改为你导出的 ONNX 模型名
具体修改示例如下:
test(TRT::Mode::FP32, "best") // 修改1 292行"yolov8s-pose"改成"best"
5. 运行
OK!源码修改好了,Makefile 编译文件也搞定了,ONNX 模型也准备好了,现在可以编译运行了,直接在终端执行如下指令即可:
make yolo_pose
编译过程如下所示:
编译运行成功后在 workspace 文件夹下会生成 engine 文件 yolov8s-pose.FP32.trtmodel 用于模型推理,同时它还会生成 yolov8s-pose_YoloV8-Pose_FP32_result 文件夹,该文件夹下保存了推理的图片。
模型推理效果如下图所示:
OK!以上就是使用 tensorRT_Pro-YOLOv8 推理 YOLOv8-Pose 的大致流程,若有问题,欢迎各位看官批评指正。
结语
博主在这里针对 YOLOv8-Pose 的预处理和后处理做了简单分析,同时与大家分享了 C++ 上的实现流程,目的是帮大家理清思路,更好的完成后续的部署工作😄。感谢各位看到最后,创作不易,读后有收获的看官请帮忙点个👍⭐️
最后大家如果觉得 tensorRT_Pro-YOLOv8 这个 repo 对你有帮助的话,不妨点个 ⭐️ 支持一波,这对博主来说非常重要,感谢各位🙏。
下载链接
- 软件安装包下载链接【提取码:yolo】🚀🚀🚀
- 源代码、权重下载链接【提取码:yolo】
参考
- https://github.com/shouxieai/infer
- https://github.com/ultralytics/ultralytics
- https://github.com/shouxieai/tensorRT_Pro
- https://github.com/Melody-Zhou/tensorRT_Pro-YOLOv8
- YOLOv5推理详解及预处理高性能实现
相关文章:
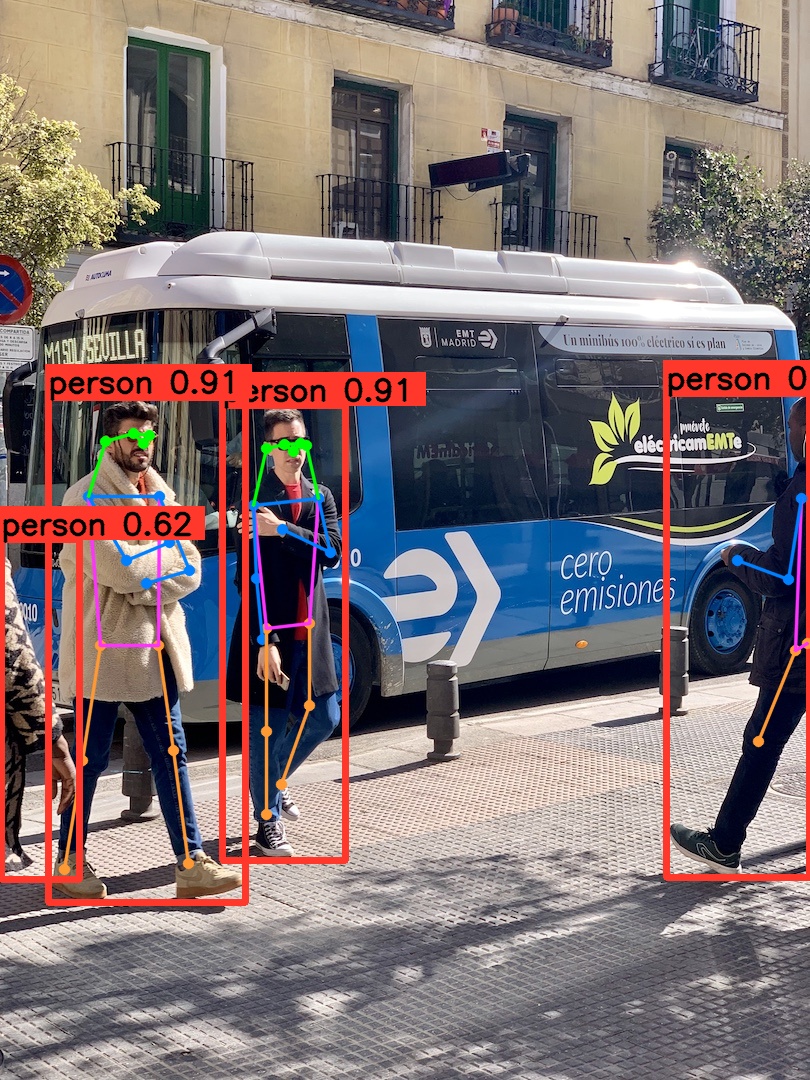
YOLOv8-Pose推理详解及部署实现
目录 前言一、YOLOv8-Pose推理(Python)1. YOLOv8-Pose预测2. YOLOv8-Pose预处理3. YOLOv8-Pose后处理4. YOLOv8-Pose推理 二、YOLOv8-Pose推理(C)1. ONNX导出2. YOLOv8-Pose预处理3. YOLOv8-Pose后处理4. YOLOv8-Pose推理 三、YOLOv8-Pose部署1. 源码下载2. 环境配置2.1 配置CM…...
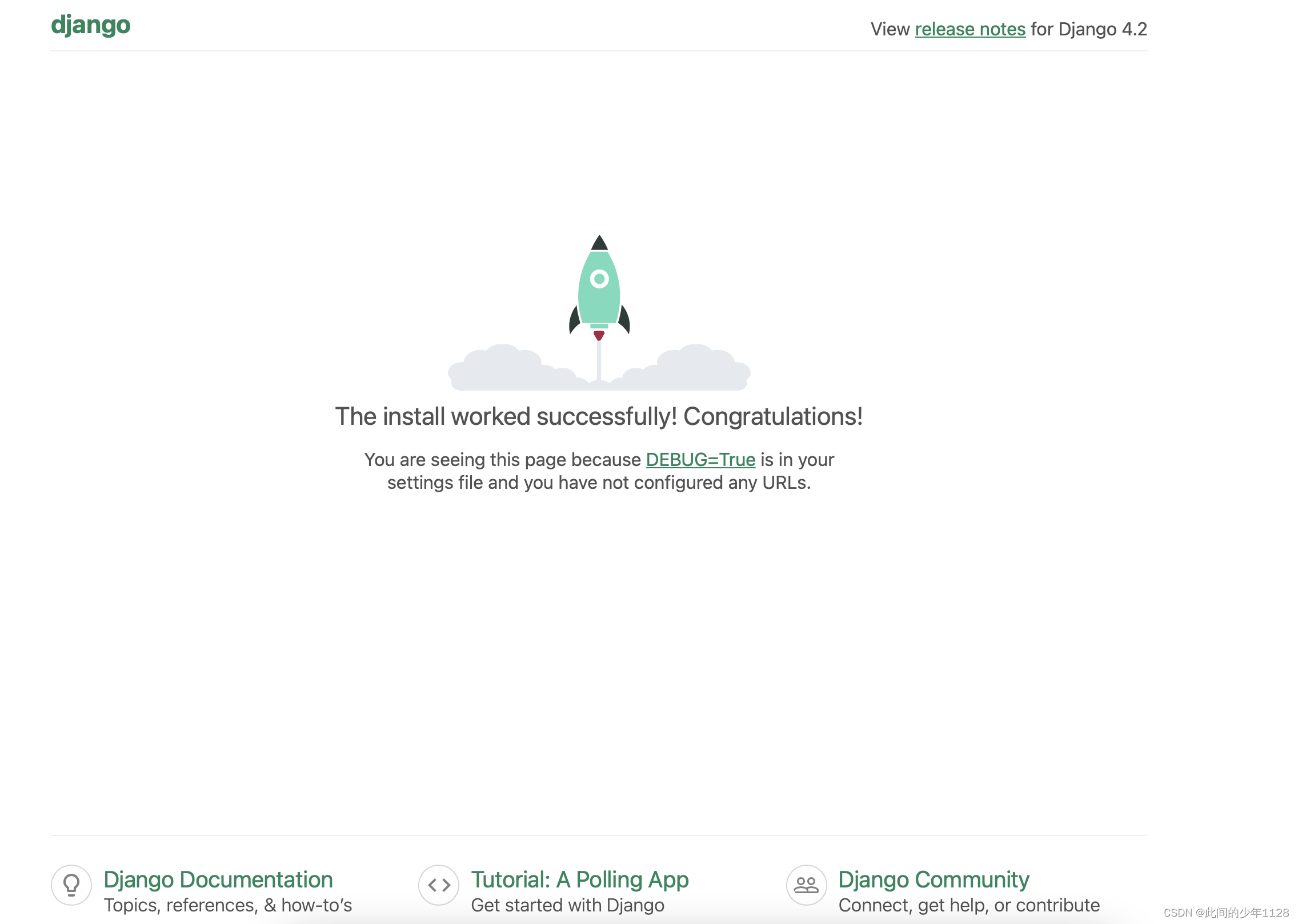
django+drf+vue 简单系统搭建 (1) - django创建项目
本系列文章为了记录自己第一个系统生成过程,主要使用django,drf,vue。本人非专业人士,此文只为记录学习,若有部分描述不够准确的地方,烦请指正。 建立这个系统的原因是因为,在生活中,很多觉得可以一两行代码…...
各种NoSQL数据库
NoSQL数据库是一类非关系型数据库,它们在数据存储和检索方面与传统的关系型数据库不同。不同类型的NoSQL数据库适用于不同的使用场景,因为它们具有各自的特点。以下是一些主要类型的NoSQL数据库及其特性和使用场景: 键值存储数据库 代表性数据…...
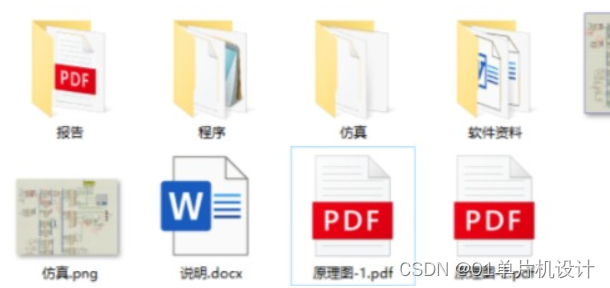
基于8086家具门安全控制系统设计
**单片机设计介绍,基于8086家具门安全控制系统设计 文章目录 一 概要二、功能设计设计思路 三、 软件设计原理图 五、 程序六、 文章目录 一 概要 # 8086家具门安全控制系统设计介绍 8086家具门安全控制系统是一种用于保护家具和保证室内安全的系统。该系统基于808…...

k8s:kubectl 详解
目录 1 kubectl 2 基本信息查看 2.1 查看 master 节点状态 2.2 查看命名空间 2.3 查看default命名空间的所有资源 2.4 创建命名空间app 2.5 删除命名空间app 2.6 在命名空间kube-public 创建副本控制器(deployment)来启动Pod(nginx-wl…...
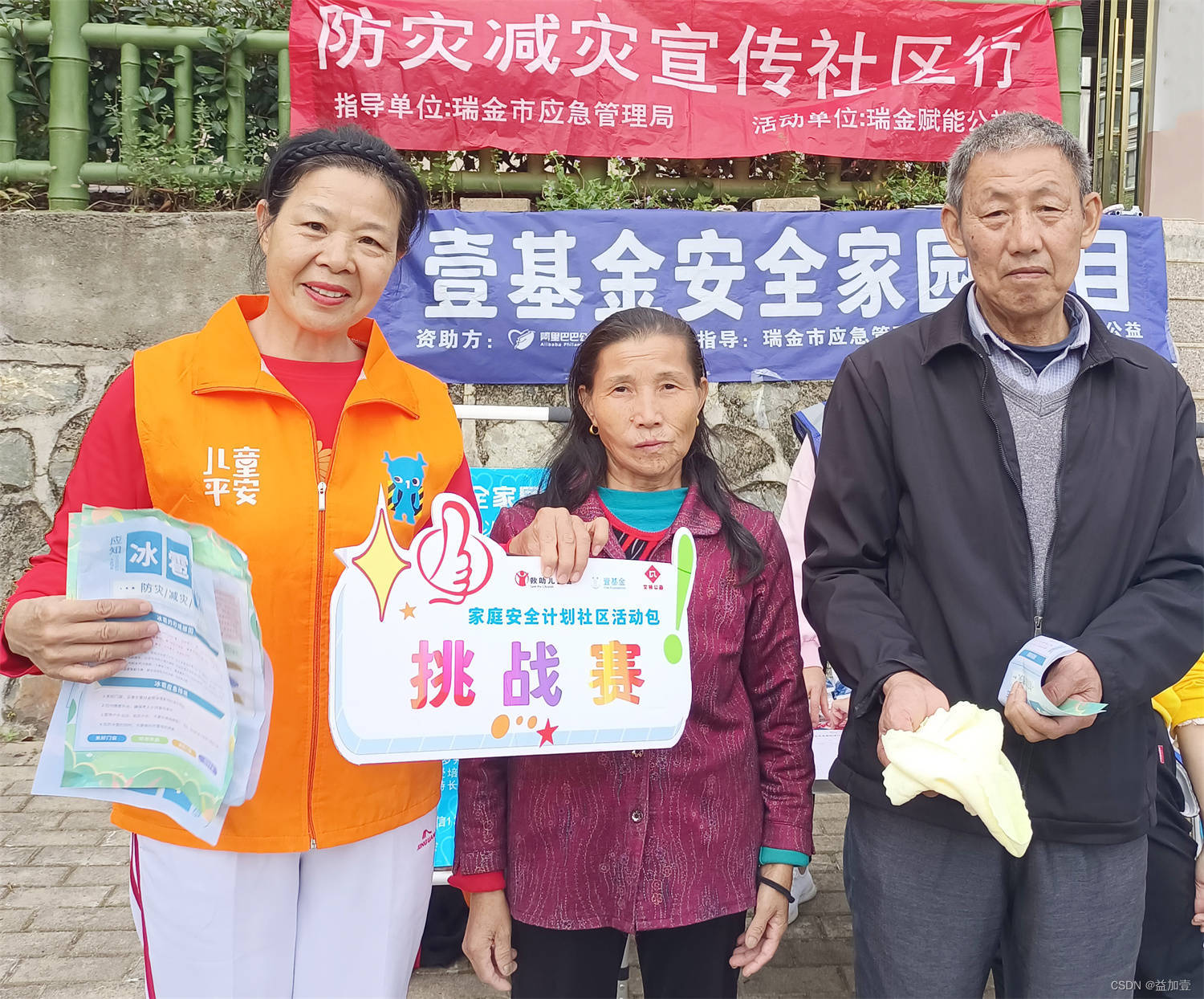
壹基金防灾减灾宣传进社区 提升家庭安全能力
11月7日,瑞金市赋能济困公益协会、蓝天救援队等联合沙洲坝镇红都新城社区一起走进梦想家园小区,开展家庭安全计划社区活动包挑战赛活动暨壹基金安全家园项目防灾减灾宣传社区行活动。 活动中,志愿者针对从洪涝灾害、风灾、火灾、雪灾、地质灾…...
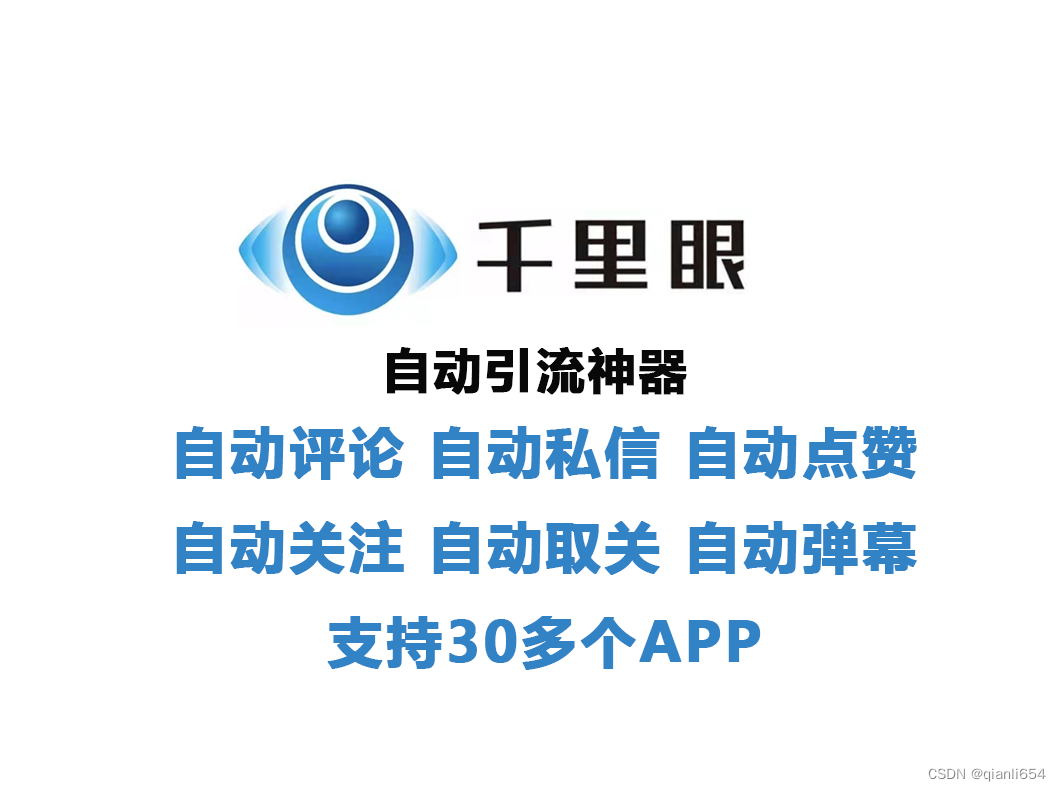
抖音自动发评论软件下载方法与其使用方法与案例分析分享
先来看成果,↑↑需要的同学可看我名字↖↖↖↖↖,或评论888无偿分享 一、引言 亲爱的知友们,你是否在抖音上遇到过这样的情况:看到一个非常棒的短视频,但想要表达的评论太多,手动逐一回复既耗时又费力。那…...
Java代码实现当前时间增加3个月
import java.time.LocalDate; import java.time.temporal.TemporalAdjusters;public class DateUtils {public static LocalDate addThreeMonths(LocalDate date) {// 使用TemporalAdjusters类将日期增加3个月return date.plusMonths(3).with(TemporalAdjusters.lastDayOfMonth…...
互联网系统安全(一)
目录 一、互联网系统安全概述 互联网系统的安全性为什么如此重要 互联网系统中的典型威胁 二、 威胁分析 2.1、跨站脚本(XSS) 2.1.1、跨站脚本 (XSS)攻击 2.1.2、SQL注入攻击: 2.2、跨站请求伪造(C…...
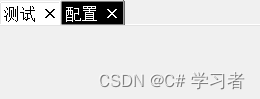
C# TabControl实现为每一个TabPage添加关闭按钮
默认情况下TabControl是无法通过界面关闭TabPage的 有些情况下我们需要手动关闭任意一个TabPage,如下图所示 TabControl控件自带属性是无法满足以上需求,下面简单介绍实现过程 1、首先需要对TabPage进行重绘,其目的是为了在TabPage上画出…...
Win10系统下torch.cuda.is_available()返回为False的问题解决
Q: Win10系统下torch.cuda.is_available()返回为False (l2) D:\opt\l2>pythonPython 3.10.12 | packaged by conda-forge | (main, Jun 23 2023, 22:34:57) [MSC v.1936 64 bit (AMD64)] on win32Type "help", "copyright", "credits" or &q…...
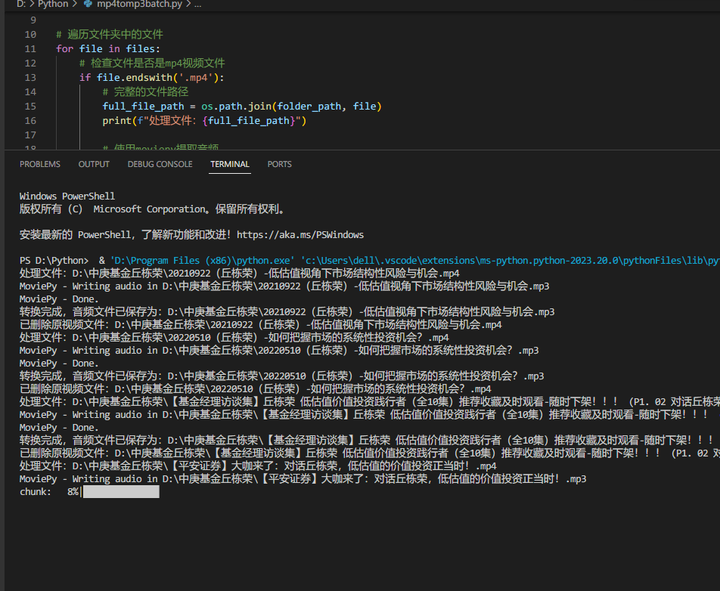
零代码编程:用ChatGPT批量将Mp4视频转为Mp3音频
文件夹中有很多mp4视频文件,如何利用ChatGPT来全部转换为mp3音频呢? 在ChatGPT中输入提示词: 你是一个Python编程专家,要完成一个批量将Mp4视频转为Mp3音频的任务,具体步骤如下: 打开文件夹:…...
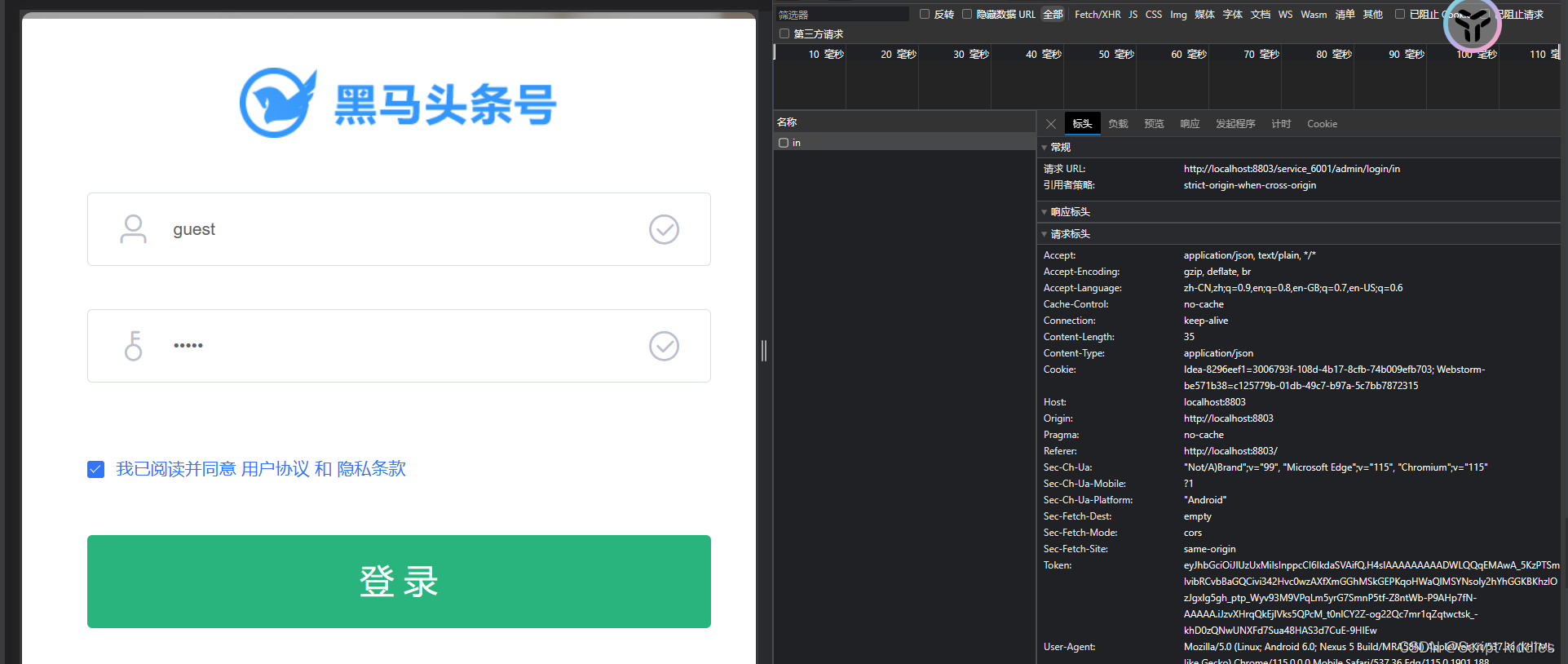
微服务项目,请求从发出到后端处理器的历程
点击登录按钮,发出 http://localhost:8803/service_6001/admin/login/in请求,这是一个由nginx配置的前端项目 查看配置文件,该条请求会被映射形成对http://localhost:51603/admin/login/in的post请求 upstream heima-admin-gateway {server localhost:51603; } server {liste…...
pandas教程:String Manipulation 字符串处理和正则表达式re
文章目录 7.3 String Manipulation(字符串处理)1 String Object Methods(字符串对象方法)2 Regular Expressions(正则表达式)3 Vectorized String Functions in pandas(pandas中的字符串向量化函…...
Apache Doris 是什么
Apache Doris 是一个开源的、基于MPP(Massively Parallel Processing)架构的SQL数据仓库。它旨在提供高性能、高可靠性的数据分析服务,特别适合处理大规模数据集。 Doris 的主要特点包括: 高性能:通过MPP架构…...

【NeurIPS 2020】基于蒙特卡罗树搜索的黑箱优化学习搜索空间划分
Learning Search Space Partition for Black-box Optimization using Monte Carlo Tree Search 目标:从采样(Dt ∩ ΩA)中学习一个边界,从而最大化两方的差异 先使用Kmeans在特征向量上( [x, f(x)] )聚类…...
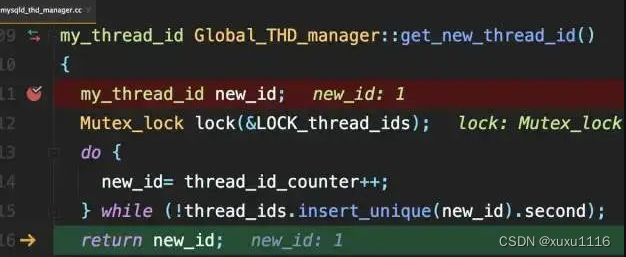
面试题:线上MySQL的自增id用尽怎么办?
文章目录 前言表定义自增值idInnoDB系统自增row_idXidInnodb trx_id InnoDB数据可见性的核心思想为什么要加248?为何只读事务不分配trx_id?thread_id 总结 前言 MySQL的自增id都定义了初始值,然后不断加步长。虽然自然数没有上限,…...
Java集合框架:Collection 与 Map 接口深度解析
Java的集合框架提供了丰富的工具和数据结构,其中 Collection 和 Map 接口是这个框架的核心。这两个接口分别用于处理一组对象和键值对的映射关系,是Java编程中不可或缺的部分。让我们深入挖掘这两个接口的特性以及它们的实际应用场景。 1. Collection 接…...
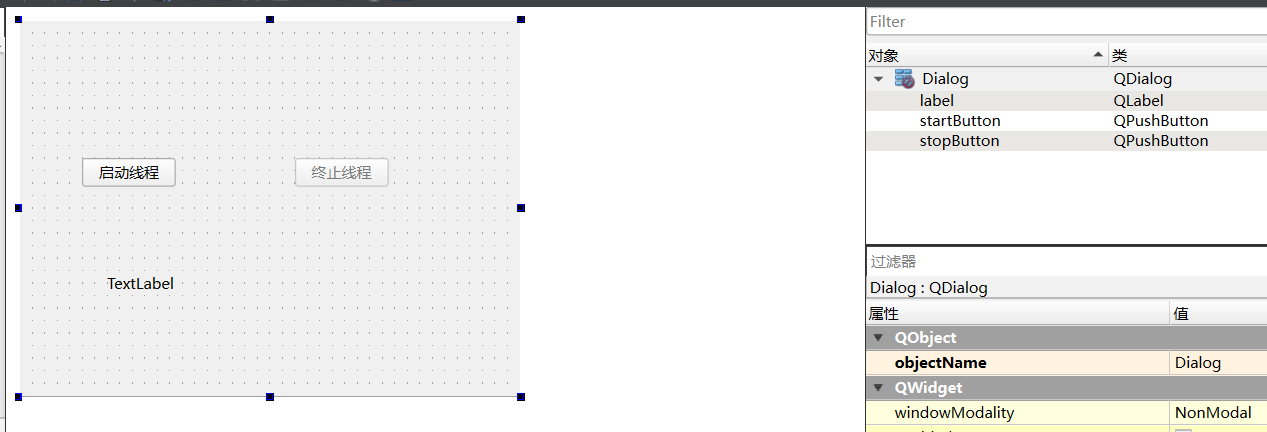
qt多线程例子,不断输出数字
dialog.h #include "dialog.h" #include "ui_dialog.h"Dialog::Dialog(QWidget *parent) :QDialog(parent),ui(new Ui::Dialog) {ui->setupUi(this); }Dialog::~Dialog() {delete ui; }// 启动线程按钮 void Dialog::on_startButton_clicked() {//conn…...
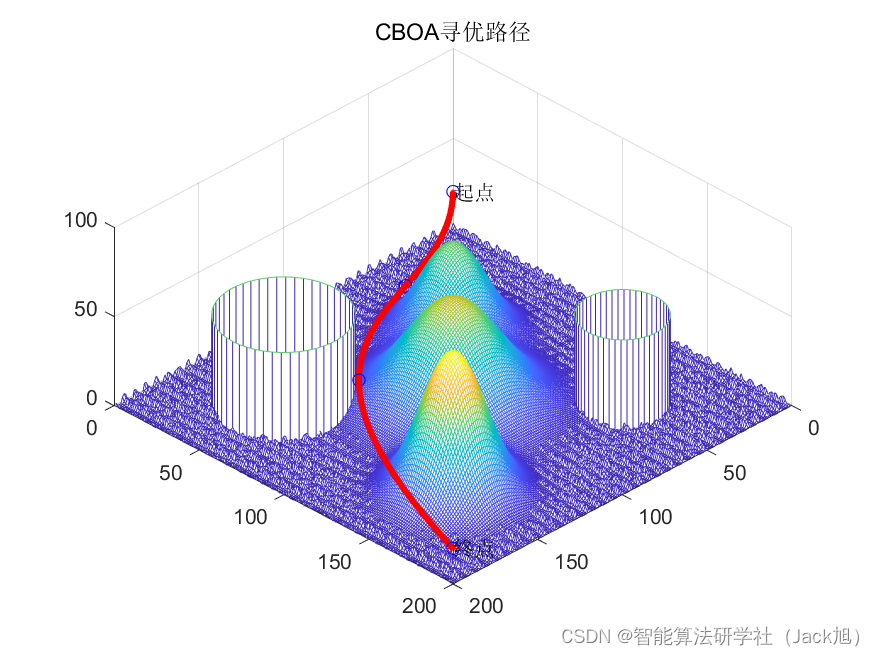
基于厨师算法的无人机航迹规划-附代码
基于厨师算法的无人机航迹规划 文章目录 基于厨师算法的无人机航迹规划1.厨师搜索算法2.无人机飞行环境建模3.无人机航迹规划建模4.实验结果4.1地图创建4.2 航迹规划 5.参考文献6.Matlab代码 摘要:本文主要介绍利用厨师算法来优化无人机航迹规划。 1.厨师搜索算法 …...
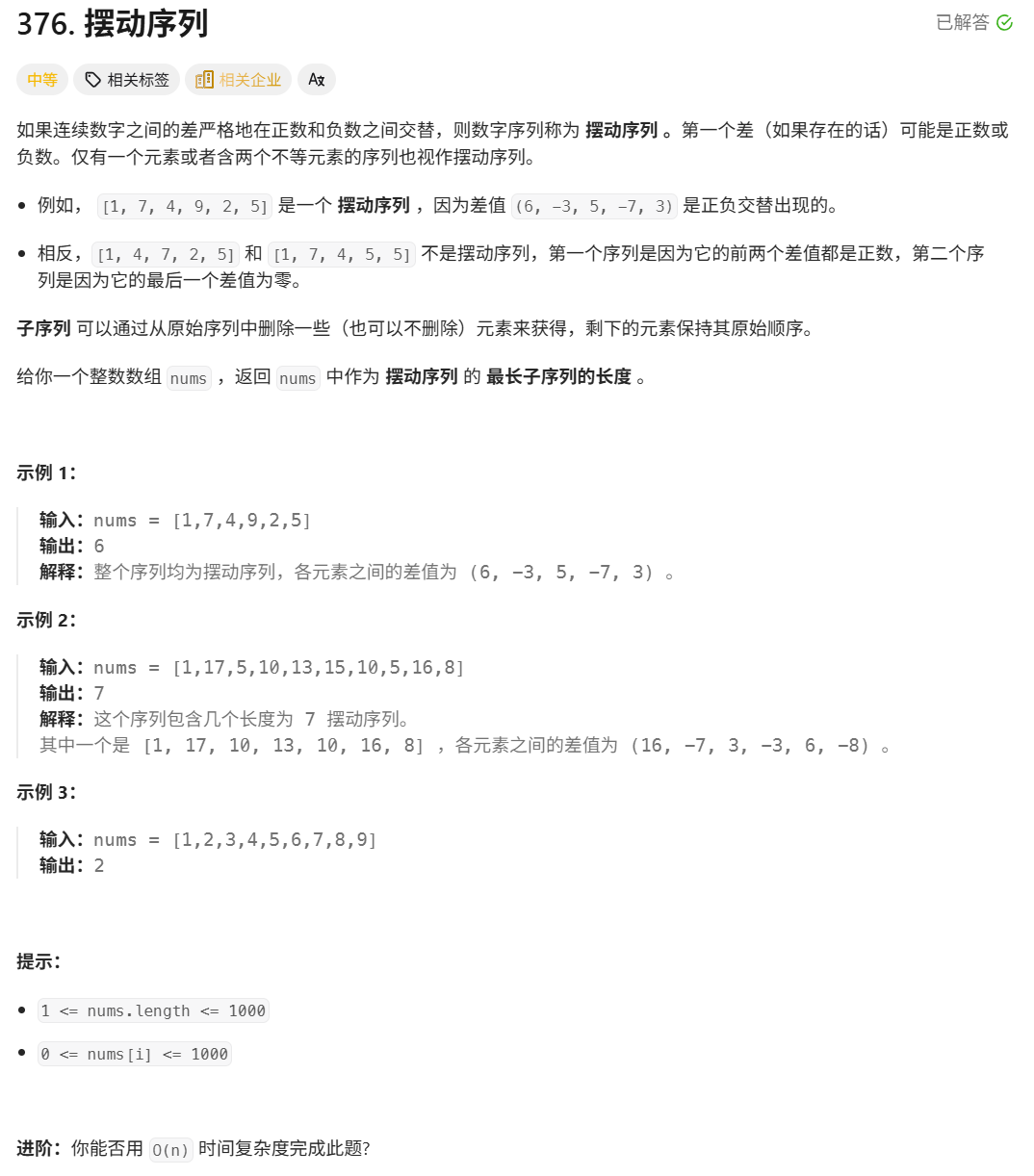
376. Wiggle Subsequence
376. Wiggle Subsequence 代码 class Solution { public:int wiggleMaxLength(vector<int>& nums) {int n nums.size();int res 1;int prediff 0;int curdiff 0;for(int i 0;i < n-1;i){curdiff nums[i1] - nums[i];if( (prediff > 0 && curdif…...
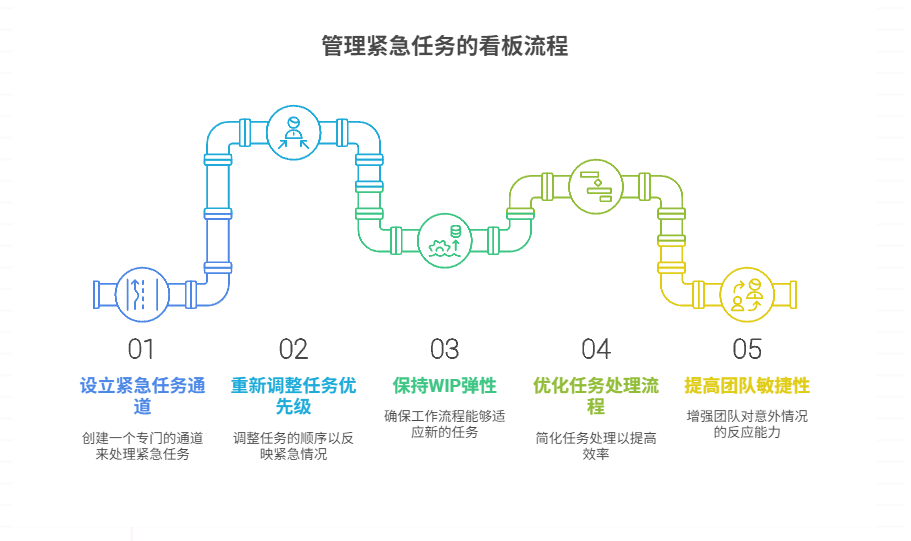
如何在看板中有效管理突发紧急任务
在看板中有效管理突发紧急任务需要:设立专门的紧急任务通道、重新调整任务优先级、保持适度的WIP(Work-in-Progress)弹性、优化任务处理流程、提高团队应对突发情况的敏捷性。其中,设立专门的紧急任务通道尤为重要,这能…...
在Ubuntu中设置开机自动运行(sudo)指令的指南
在Ubuntu系统中,有时需要在系统启动时自动执行某些命令,特别是需要 sudo权限的指令。为了实现这一功能,可以使用多种方法,包括编写Systemd服务、配置 rc.local文件或使用 cron任务计划。本文将详细介绍这些方法,并提供…...
今日科技热点速览
🔥 今日科技热点速览 🎮 任天堂Switch 2 正式发售 任天堂新一代游戏主机 Switch 2 今日正式上线发售,主打更强图形性能与沉浸式体验,支持多模态交互,受到全球玩家热捧 。 🤖 人工智能持续突破 DeepSeek-R1&…...
MySQL账号权限管理指南:安全创建账户与精细授权技巧
在MySQL数据库管理中,合理创建用户账号并分配精确权限是保障数据安全的核心环节。直接使用root账号进行所有操作不仅危险且难以审计操作行为。今天我们来全面解析MySQL账号创建与权限分配的专业方法。 一、为何需要创建独立账号? 最小权限原则…...
在QWebEngineView上实现鼠标、触摸等事件捕获的解决方案
这个问题我看其他博主也写了,要么要会员、要么写的乱七八糟。这里我整理一下,把问题说清楚并且给出代码,拿去用就行,照着葫芦画瓢。 问题 在继承QWebEngineView后,重写mousePressEvent或event函数无法捕获鼠标按下事…...

免费数学几何作图web平台
光锐软件免费数学工具,maths,数学制图,数学作图,几何作图,几何,AR开发,AR教育,增强现实,软件公司,XR,MR,VR,虚拟仿真,虚拟现实,混合现实,教育科技产品,职业模拟培训,高保真VR场景,结构互动课件,元宇宙http://xaglare.c…...
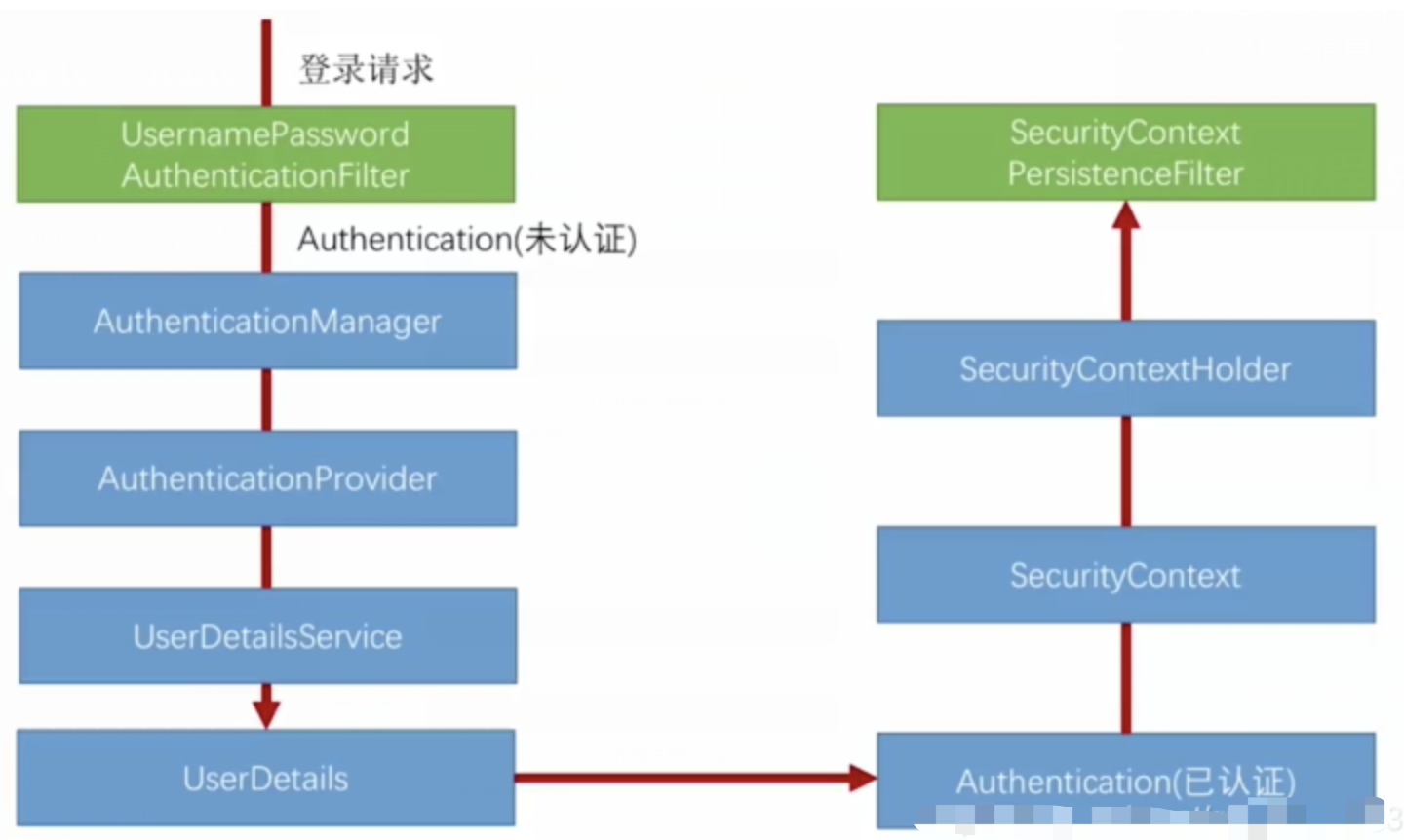
spring Security对RBAC及其ABAC的支持使用
RBAC (基于角色的访问控制) RBAC (Role-Based Access Control) 是 Spring Security 中最常用的权限模型,它将权限分配给角色,再将角色分配给用户。 RBAC 核心实现 1. 数据库设计 users roles permissions ------- ------…...
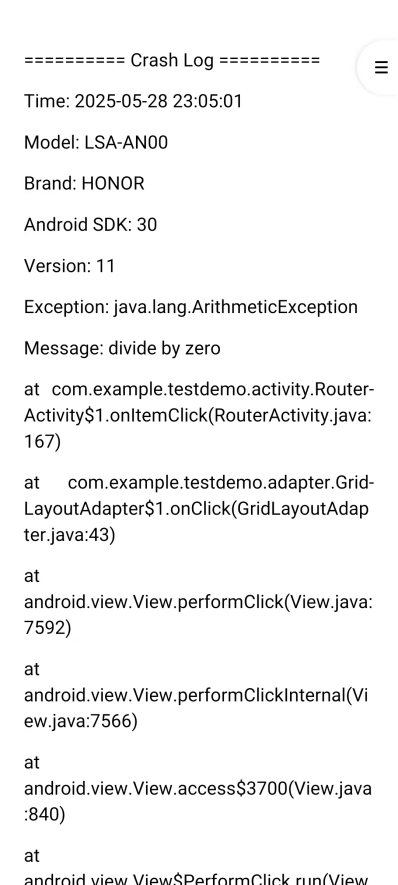
Android写一个捕获全局异常的工具类
项目开发和实际运行过程中难免会遇到异常发生,系统提供了一个可以捕获全局异常的工具Uncaughtexceptionhandler,它是Thread的子类(就是package java.lang;里线程的Thread)。本文将利用它将设备信息、报错信息以及错误的发生时间都…...
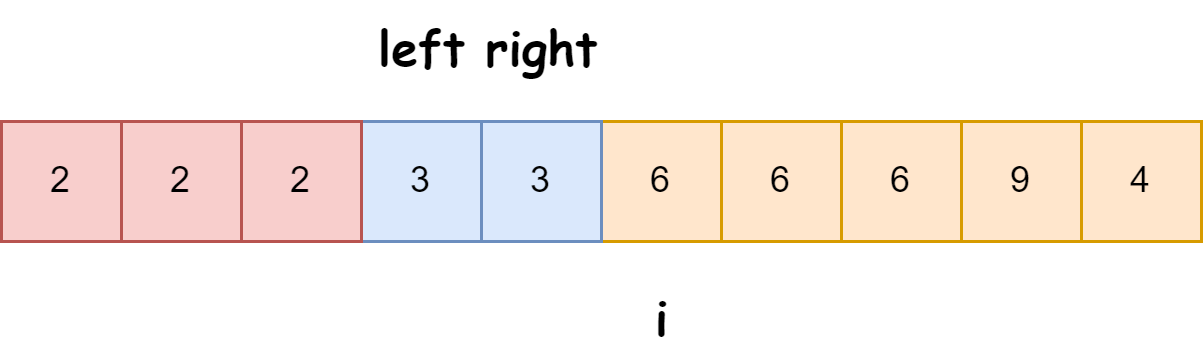
快速排序算法改进:随机快排-荷兰国旗划分详解
随机快速排序-荷兰国旗划分算法详解 一、基础知识回顾1.1 快速排序简介1.2 荷兰国旗问题 二、随机快排 - 荷兰国旗划分原理2.1 随机化枢轴选择2.2 荷兰国旗划分过程2.3 结合随机快排与荷兰国旗划分 三、代码实现3.1 Python实现3.2 Java实现3.3 C实现 四、性能分析4.1 时间复杂度…...