Spark 中 BroadCast 导致的内存溢出(SparkFatalException)
背景
本文基于
Spark 3.1.1
open-jdk-1.8.0.352
目前在排查 Spark 任务的时候,遇到了一个很奇怪的问题,在此记录一下。
现象描述
一个 Spark Application, Driver端的内存为 5GB,一直以来都是能正常调度运行,突然有一天,报错了:
Caused by: org.apache.spark.sql.catalyst.errors.package$TreeNodeException: execute, tree:
Exchange hashpartitioning(user_lable_id#530L, 500), ENSURE_REQUIREMENTS, [id=#1564]
+- *(16) Project [xxx]+- *(16) BroadcastHashJoin ...+- *(14) ColumnarToRow+- FileScan parquet xxxat org.apache.spark.sql.catalyst.errors.package$.attachTree(package.scala:56)at org.apache.spark.sql.execution.exchange.ShuffleExchangeExec.doExecute(ShuffleExchangeExec.scala:169)at org.apache.spark.sql.execution.SparkPlan.$anonfun$execute$1(SparkPlan.scala:180)at org.apache.spark.sql.execution.SparkPlan.$anonfun$executeQuery$1(SparkPlan.scala:218)at org.apache.spark.rdd.RDDOperationScope$.withScope(RDDOperationScope.scala:151)at org.apache.spark.sql.execution.SparkPlan.executeQuery(SparkPlan.scala:215)at org.apache.spark.sql.execution.SparkPlan.execute(SparkPlan.scala:176)at org.apache.spark.sql.execution.InputAdapter.inputRDD(WholeStageCodegenExec.scala:525)at org.apache.spark.sql.execution.InputRDDCodegen.inputRDDs(WholeStageCodegenExec.scala:453)at org.apache.spark.sql.execution.InputRDDCodegen.inputRDDs$(WholeStageCodegenExec.scala:452)at org.apache.spark.sql.execution.InputAdapter.inputRDDs(WholeStageCodegenExec.scala:496)at org.apache.spark.sql.execution.SortExec.inputRDDs(SortExec.scala:132)at org.apache.spark.sql.execution.WholeStageCodegenExec.doExecute(WholeStageCodegenExec.scala:746)at org.apache.spark.sql.execution.SparkPlan.$anonfun$execute$1(SparkPlan.scala:180)at org.apache.spark.sql.execution.SparkPlan.$anonfun$executeQuery$1(SparkPlan.scala:218)at org.apache.spark.rdd.RDDOperationScope$.withScope(RDDOperationScope.scala:151)at org.apache.spark.sql.execution.SparkPlan.executeQuery(SparkPlan.scala:215)at org.apache.spark.sql.execution.SparkPlan.execute(SparkPlan.scala:176)at org.apache.spark.sql.execution.InputAdapter.doExecute(WholeStageCodegenExec.scala:511)at org.apache.spark.sql.execution.SparkPlan.$anonfun$execute$1(SparkPlan.scala:180)at org.apache.spark.sql.execution.SparkPlan.$anonfun$executeQuery$1(SparkPlan.scala:218)at org.apache.spark.rdd.RDDOperationScope$.withScope(RDDOperationScope.scala:151)at org.apache.spark.sql.execution.SparkPlan.executeQuery(SparkPlan.scala:215)at org.apache.spark.sql.execution.SparkPlan.execute(SparkPlan.scala:176)at org.apache.spark.sql.execution.joins.SortMergeJoinExec.inputRDDs(SortMergeJoinExec.scala:378)at org.apache.spark.sql.execution.ProjectExec.inputRDDs(basicPhysicalOperators.scala:50)at org.apache.spark.sql.execution.WholeStageCodegenExec.doExecute(WholeStageCodegenExec.scala:746)at org.apache.spark.sql.execution.SparkPlan.$anonfun$execute$1(SparkPlan.scala:180)at org.apache.spark.sql.execution.SparkPlan.$anonfun$executeQuery$1(SparkPlan.scala:218)at org.apache.spark.rdd.RDDOperationScope$.withScope(RDDOperationScope.scala:151)at org.apache.spark.sql.execution.SparkPlan.executeQuery(SparkPlan.scala:215)at org.apache.spark.sql.execution.SparkPlan.execute(SparkPlan.scala:176)at org.apache.spark.sql.execution.exchange.ShuffleExchangeExec.inputRDD$lzycompute(ShuffleExchangeExec.scala:123)at org.apache.spark.sql.execution.exchange.ShuffleExchangeExec.inputRDD(ShuffleExchangeExec.scala:123)at org.apache.spark.sql.execution.exchange.ShuffleExchangeExec.shuffleDependency$lzycompute(ShuffleExchangeExec.scala:157)at org.apache.spark.sql.execution.exchange.ShuffleExchangeExec.shuffleDependency(ShuffleExchangeExec.scala:155)at org.apache.spark.sql.execution.exchange.ShuffleExchangeExec.$anonfun$doExecute$1(ShuffleExchangeExec.scala:172)at org.apache.spark.sql.catalyst.errors.package$.attachTree(package.scala:52)... 291 more
Caused by: java.util.concurrent.ExecutionException: org.apache.spark.util.SparkFatalExceptionat java.util.concurrent.FutureTask.report(FutureTask.java:122)at java.util.concurrent.FutureTask.get(FutureTask.java:206)at org.apache.spark.sql.execution.exchange.BroadcastExchangeExec.doExecuteBroadcast(BroadcastExchangeExec.scala:199)at org.apache.spark.sql.execution.InputAdapter.doExecuteBroadcast(WholeStageCodegenExec.scala:515)at org.apache.spark.sql.execution.SparkPlan.$anonfun$executeBroadcast$1(SparkPlan.scala:193)at org.apache.spark.sql.execution.SparkPlan.$anonfun$executeQuery$1(SparkPlan.scala:218)at org.apache.spark.rdd.RDDOperationScope$.withScope(RDDOperationScope.scala:151)at org.apache.spark.sql.execution.SparkPlan.executeQuery(SparkPlan.scala:215)at org.apache.spark.sql.execution.SparkPlan.executeBroadcast(SparkPlan.scala:189)at org.apache.spark.sql.execution.joins.BroadcastHashJoinExec.prepareBroadcast(BroadcastHashJoinExec.scala:203)at org.apache.spark.sql.execution.joins.BroadcastHashJoinExec.prepareRelation(BroadcastHashJoinExec.scala:217)at org.apache.spark.sql.execution.joins.HashJoin.codegenOuter(HashJoin.scala:497)at org.apache.spark.sql.execution.joins.HashJoin.codegenOuter$(HashJoin.scala:496)at org.apache.spark.sql.execution.joins.BroadcastHashJoinExec.codegenOuter(BroadcastHashJoinExec.scala:40)at org.apache.spark.sql.execution.joins.HashJoin.doConsume(HashJoin.scala:352)at org.apache.spark.sql.execution.joins.HashJoin.doConsume$(HashJoin.scala:349)at org.apache.spark.sql.execution.joins.BroadcastHashJoinExec.doConsume(BroadcastHashJoinExec.scala:40)at org.apache.spark.sql.execution.CodegenSupport.consume(WholeStageCodegenExec.scala:194)at org.apache.spark.sql.execution.CodegenSupport.consume$(WholeStageCodegenExec.scala:149)at org.apache.spark.sql.execution.ProjectExec.consume(basicPhysicalOperators.scala:41)at org.apache.spark.sql.execution.ProjectExec.doConsume(basicPhysicalOperators.scala:87)at org.apache.spark.sql.execution.CodegenSupport.consume(WholeStageCodegenExec.scala:194)at org.apache.spark.sql.execution.CodegenSupport.consume$(WholeStageCodegenExec.scala:149)at org.apache.spark.sql.execution.joins.BroadcastHashJoinExec.consume(BroadcastHashJoinExec.scala:40)at org.apache.spark.sql.execution.joins.HashJoin.codegenOuter(HashJoin.scala:542)at org.apache.spark.sql.execution.joins.HashJoin.codegenOuter$(HashJoin.scala:496)at org.apache.spark.sql.execution.joins.BroadcastHashJoinExec.codegenOuter(BroadcastHashJoinExec.scala:40)at org.apache.spark.sql.execution.joins.HashJoin.doConsume(HashJoin.scala:352)at org.apache.spark.sql.execution.joins.HashJoin.doConsume$(HashJoin.scala:349)at org.apache.spark.sql.execution.joins.BroadcastHashJoinExec.doConsume(BroadcastHashJoinExec.scala:40)at org.apache.spark.sql.execution.CodegenSupport.consume(WholeStageCodegenExec.scala:194)at org.apache.spark.sql.execution.CodegenSupport.consume$(WholeStageCodegenExec.scala:149)at org.apache.spark.sql.execution.ProjectExec.consume(basicPhysicalOperators.scala:41)at org.apache.spark.sql.execution.ProjectExec.doConsume(basicPhysicalOperators.scala:87)at org.apache.spark.sql.execution.CodegenSupport.consume(WholeStageCodegenExec.scala:194)at org.apache.spark.sql.execution.CodegenSupport.consume$(WholeStageCodegenExec.scala:149)at org.apache.spark.sql.execution.InputAdapter.consume(WholeStageCodegenExec.scala:496)at org.apache.spark.sql.execution.InputRDDCodegen.doProduce(WholeStageCodegenExec.scala:483)at org.apache.spark.sql.execution.InputRDDCodegen.doProduce$(WholeStageCodegenExec.scala:456)at org.apache.spark.sql.execution.InputAdapter.doProduce(WholeStageCodegenExec.scala:496)at org.apache.spark.sql.execution.CodegenSupport.$anonfun$produce$1(WholeStageCodegenExec.scala:95)at org.apache.spark.sql.execution.SparkPlan.$anonfun$executeQuery$1(SparkPlan.scala:218)at org.apache.spark.rdd.RDDOperationScope$.withScope(RDDOperationScope.scala:151)at org.apache.spark.sql.execution.SparkPlan.executeQuery(SparkPlan.scala:215)at org.apache.spark.sql.execution.CodegenSupport.produce(WholeStageCodegenExec.scala:90)at org.apache.spark.sql.execution.CodegenSupport.produce$(WholeStageCodegenExec.scala:90)at org.apache.spark.sql.execution.InputAdapter.produce(WholeStageCodegenExec.scala:496)at org.apache.spark.sql.execution.ProjectExec.doProduce(basicPhysicalOperators.scala:54)at org.apache.spark.sql.execution.CodegenSupport.$anonfun$produce$1(WholeStageCodegenExec.scala:95)at org.apache.spark.sql.execution.SparkPlan.$anonfun$executeQuery$1(SparkPlan.scala:218)at org.apache.spark.rdd.RDDOperationScope$.withScope(RDDOperationScope.scala:151)at org.apache.spark.sql.execution.SparkPlan.executeQuery(SparkPlan.scala:215)at org.apache.spark.sql.execution.CodegenSupport.produce(WholeStageCodegenExec.scala:90)at org.apache.spark.sql.execution.CodegenSupport.produce$(WholeStageCodegenExec.scala:90)at org.apache.spark.sql.execution.ProjectExec.produce(basicPhysicalOperators.scala:41)at org.apache.spark.sql.execution.joins.HashJoin.doProduce(HashJoin.scala:346)at org.apache.spark.sql.execution.joins.HashJoin.doProduce$(HashJoin.scala:345)at org.apache.spark.sql.execution.joins.BroadcastHashJoinExec.doProduce(BroadcastHashJoinExec.scala:40)at org.apache.spark.sql.execution.CodegenSupport.$anonfun$produce$1(WholeStageCodegenExec.scala:95)at org.apache.spark.sql.execution.SparkPlan.$anonfun$executeQuery$1(SparkPlan.scala:218)at org.apache.spark.rdd.RDDOperationScope$.withScope(RDDOperationScope.scala:151)at org.apache.spark.sql.execution.SparkPlan.executeQuery(SparkPlan.scala:215)at org.apache.spark.sql.execution.CodegenSupport.produce(WholeStageCodegenExec.scala:90)at org.apache.spark.sql.execution.CodegenSupport.produce$(WholeStageCodegenExec.scala:90)at org.apache.spark.sql.execution.joins.BroadcastHashJoinExec.produce(BroadcastHashJoinExec.scala:40)at org.apache.spark.sql.execution.ProjectExec.doProduce(basicPhysicalOperators.scala:54)at org.apache.spark.sql.execution.CodegenSupport.$anonfun$produce$1(WholeStageCodegenExec.scala:95)at org.apache.spark.sql.execution.SparkPlan.$anonfun$executeQuery$1(SparkPlan.scala:218)at org.apache.spark.rdd.RDDOperationScope$.withScope(RDDOperationScope.scala:151)at org.apache.spark.sql.execution.SparkPlan.executeQuery(SparkPlan.scala:215)at org.apache.spark.sql.execution.CodegenSupport.produce(WholeStageCodegenExec.scala:90)at org.apache.spark.sql.execution.CodegenSupport.produce$(WholeStageCodegenExec.scala:90)at org.apache.spark.sql.execution.ProjectExec.produce(basicPhysicalOperators.scala:41)at org.apache.spark.sql.execution.joins.HashJoin.doProduce(HashJoin.scala:346)at org.apache.spark.sql.execution.joins.HashJoin.doProduce$(HashJoin.scala:345)at org.apache.spark.sql.execution.joins.BroadcastHashJoinExec.doProduce(BroadcastHashJoinExec.scala:40)at org.apache.spark.sql.execution.CodegenSupport.$anonfun$produce$1(WholeStageCodegenExec.scala:95)at org.apache.spark.sql.execution.SparkPlan.$anonfun$executeQuery$1(SparkPlan.scala:218)at org.apache.spark.rdd.RDDOperationScope$.withScope(RDDOperationScope.scala:151)at org.apache.spark.sql.execution.SparkPlan.executeQuery(SparkPlan.scala:215)at org.apache.spark.sql.execution.CodegenSupport.produce(WholeStageCodegenExec.scala:90)at org.apache.spark.sql.execution.CodegenSupport.produce$(WholeStageCodegenExec.scala:90)at org.apache.spark.sql.execution.joins.BroadcastHashJoinExec.produce(BroadcastHashJoinExec.scala:40)at org.apache.spark.sql.execution.ProjectExec.doProduce(basicPhysicalOperators.scala:54)at org.apache.spark.sql.execution.CodegenSupport.$anonfun$produce$1(WholeStageCodegenExec.scala:95)at org.apache.spark.sql.execution.SparkPlan.$anonfun$executeQuery$1(SparkPlan.scala:218)at org.apache.spark.rdd.RDDOperationScope$.withScope(RDDOperationScope.scala:151)at org.apache.spark.sql.execution.SparkPlan.executeQuery(SparkPlan.scala:215)at org.apache.spark.sql.execution.CodegenSupport.produce(WholeStageCodegenExec.scala:90)at org.apache.spark.sql.execution.CodegenSupport.produce$(WholeStageCodegenExec.scala:90)at org.apache.spark.sql.execution.ProjectExec.produce(basicPhysicalOperators.scala:41)at org.apache.spark.sql.execution.WholeStageCodegenExec.doCodeGen(WholeStageCodegenExec.scala:655)at org.apache.spark.sql.execution.WholeStageCodegenExec.doExecute(WholeStageCodegenExec.scala:718)at org.apache.spark.sql.execution.SparkPlan.$anonfun$execute$1(SparkPlan.scala:180)at org.apache.spark.sql.execution.SparkPlan.$anonfun$executeQuery$1(SparkPlan.scala:218)at org.apache.spark.rdd.RDDOperationScope$.withScope(RDDOperationScope.scala:151)at org.apache.spark.sql.execution.SparkPlan.executeQuery(SparkPlan.scala:215)at org.apache.spark.sql.execution.SparkPlan.execute(SparkPlan.scala:176)at org.apache.spark.sql.execution.exchange.ShuffleExchangeExec.inputRDD$lzycompute(ShuffleExchangeExec.scala:123)at org.apache.spark.sql.execution.exchange.ShuffleExchangeExec.inputRDD(ShuffleExchangeExec.scala:123)at org.apache.spark.sql.execution.exchange.ShuffleExchangeExec.shuffleDependency$lzycompute(ShuffleExchangeExec.scala:157)at org.apache.spark.sql.execution.exchange.ShuffleExchangeExec.shuffleDependency(ShuffleExchangeExec.scala:155)at org.apache.spark.sql.execution.exchange.ShuffleExchangeExec.$anonfun$doExecute$1(ShuffleExchangeExec.scala:172)at org.apache.spark.sql.catalyst.errors.package$.attachTree(package.scala:52)... 328 more
Caused by: org.apache.spark.util.SparkFatalExceptionat org.apache.spark.sql.execution.exchange.BroadcastExchangeExec.$anonfun$relationFuture$1(BroadcastExchangeExec.scala:173)at org.apache.spark.sql.execution.SQLExecution$.$anonfun$withThreadLocalCaptured$1(SQLExecution.scala:190)at java.util.concurrent.FutureTask.run(FutureTask.java:266)at java.util.concurrent.ThreadPoolExecutor.runWorker(ThreadPoolExecutor.java:1149)at java.util.concurrent.ThreadPoolExecutor$Worker.run(ThreadPoolExecutor.java:624)at java.lang.Thread.run(Thread.java:750)
注意:处于安全考虑,本文隐藏了具体的物理执行计划
对于一个在大数据行业摸爬滚打了多年的老手来说,第一眼肯定是跟着堆栈信息进行排查,
理所当然的就是会找到BroadcastExchangeExec
这个类,但是就算把代码全看一遍也不会有所发现。
蓦然回首
这个问题折腾了我大约2个小时,错误发生的上下文都看了不止十遍了,还是没找到一丝头绪,可能是上帝的旨意,在离错误不到50行的地方,刚好是一个页面的距离,发现了以下错误:
53.024: [Full GC (Ergonomics) [PSYoungGen: 802227K->698101K(1191424K)] [ParOldGen: 3085945K->3085781K(3495424K)] 3888173K->3783883K(4686848K), [Metaspace: 135862K->135862K(1185792K)], 0.9651630 secs] [Times: user=25.51 sys=0.39, real=0.96 secs]
53.990: [Full GC (Allocation Failure) [PSYoungGen: 698101K->698047K(1191424K)] [ParOldGen: 3085781K->3079721K(3495424K)] 3783883K->3777769K(4686848K), [Metaspace: 135862K->134900K(1185792K)], 0.6236139 secs] [Times: user=14.05 sys=0.28, real=0.63 secs]
java.lang.OutOfMemoryError: Java heap space
Dumping heap to panda_dump ...
Heap dump file created [3938522340 bytes in 5.708 secs]
真是 众人寻他千百度,蓦然回首, 没想到是 OOM 问题。
结论
在查找错误的时候,还是得在错误的上下文中多翻几页。
相关文章:

Spark 中 BroadCast 导致的内存溢出(SparkFatalException)
背景 本文基于 Spark 3.1.1 open-jdk-1.8.0.352目前在排查 Spark 任务的时候,遇到了一个很奇怪的问题,在此记录一下。 现象描述 一个 Spark Application, Driver端的内存为 5GB,一直以来都是能正常调度运行,突然有一天,报…...
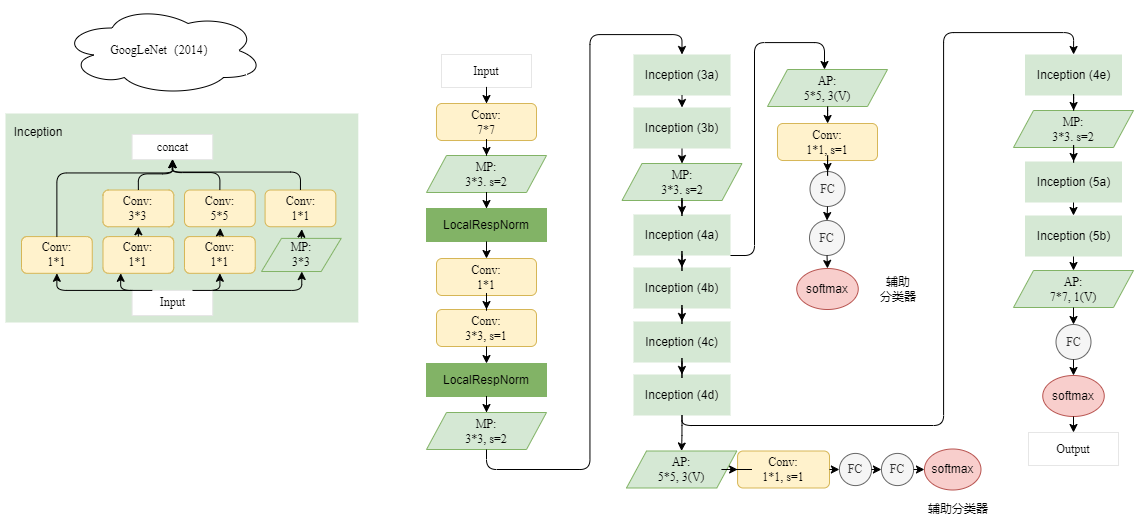
深度学习经典算法详细模型图
很早绘制的一些模型图,当时放在CSDN的草稿里,今天发现了,把它分享出来吧,还能更清晰的帮助理解! 1.AlexNet(2012) 2. VGGNet(2014) 3. SqueezeNet(2016) 4. GoogleNet(2014)...
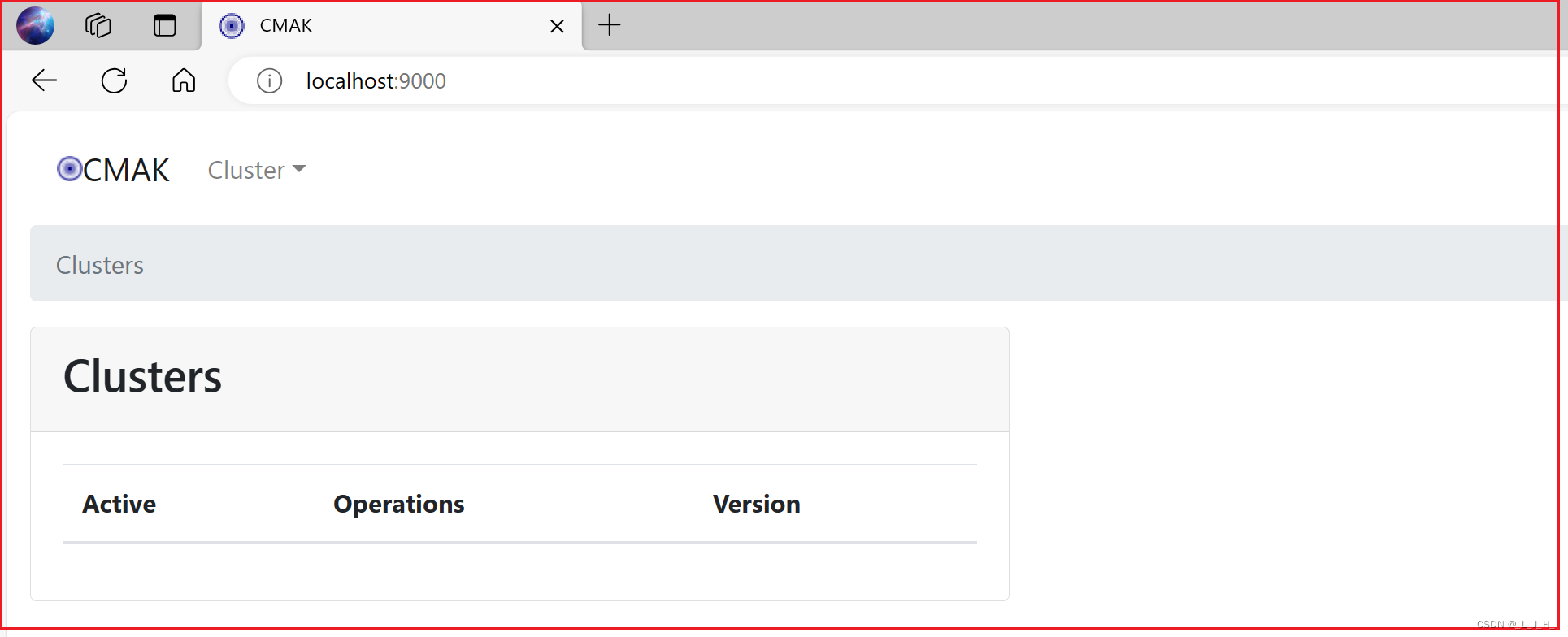
03、Kafka ------ CMAK(Kafka 图形界面管理工具) 下载、安装、启动
目录 CMAK(Kafka 图形界面管理工具)下载安装启动打开 cmak 图形界面 CMAK(Kafka 图形界面管理工具) Kafka本身并没有提供Web管理工具,而是推荐使用bin目录下各种工具命令来管理Kafka, 这些工具命令其实用起…...

复习python从入门到实践——函数function
复习python从入门到实践——函数function 函数是特别难的,大家一定要好好学、好好复习、反复巩固。函数没学好,会为后面造成很大困扰。 教科书中函数举例会稍微有点复杂。在此章复习中,我将整理出容易疏漏和混淆的知识点,并用最简…...
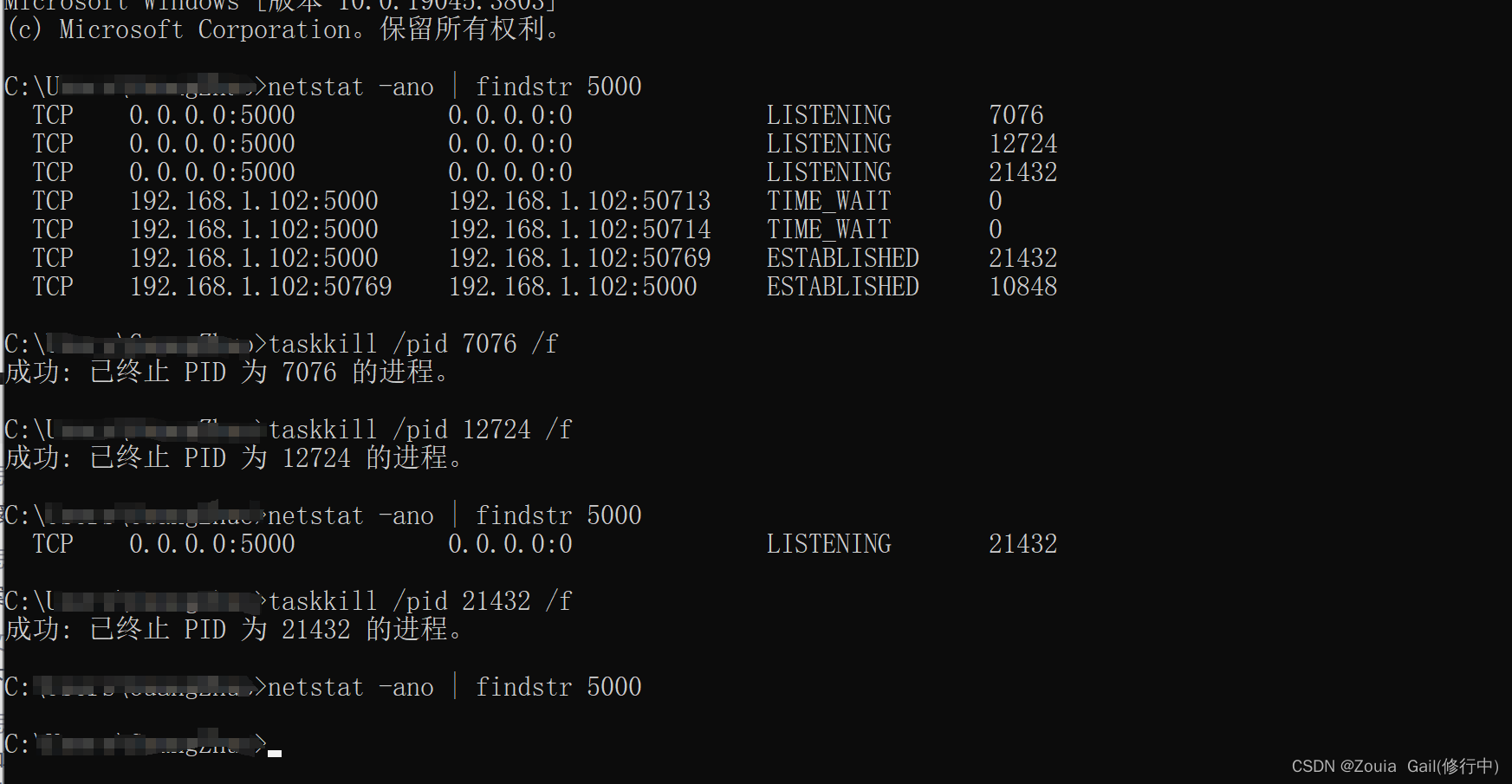
【Internal Server Error】pycharm解决关闭flask端口依然占用问题
Internal Server Error The server encountered an internal error and was unable to complete your request. Either the server is overloaded or there is an error in the application. 起因: 我们在运行flask后,断开服务依然保持运行࿰…...

torch.nn.functional.interpolate与torchvision.transforms.Resize方法对张量图像Resize应用
提示:文章写完后,目录可以自动生成,如何生成可参考右边的帮助文档 文章目录 前言一、非张量数据使用torch方法resize(transforms.Resize)二、张量数据使用torch方法resize(torch.nn.functional.interpolate) 前言 要使用 PyTorch 对张量进行…...
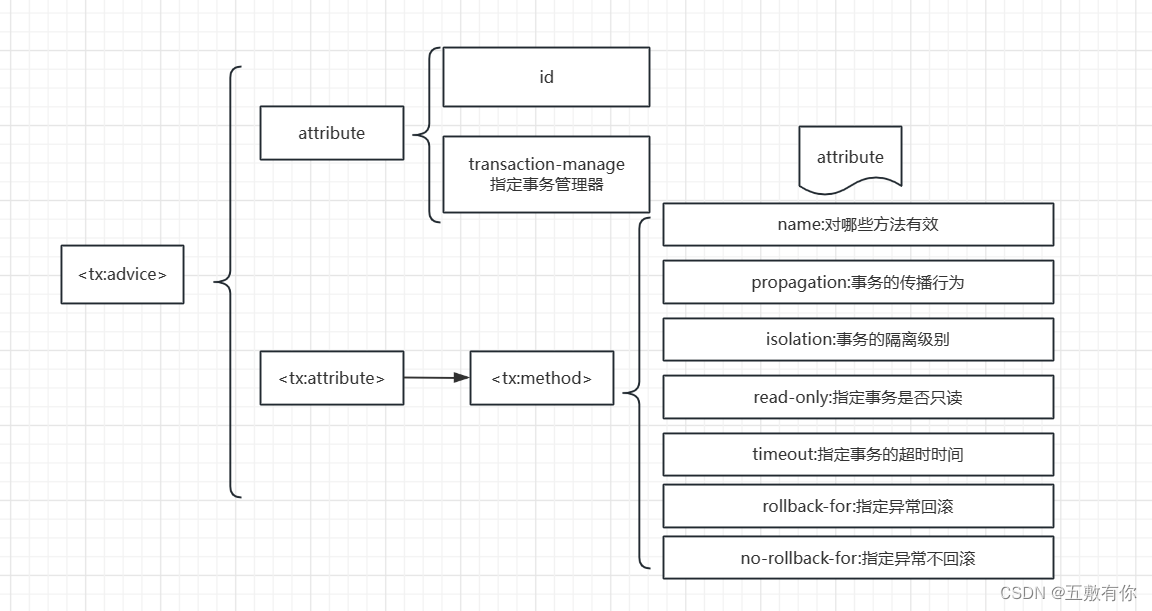
【Spring】Spring的事务管理
前言: package com.aqiuo.service.impl;import com.aqiuo.dao.AccountMapper; import com.aqiuo.pojo.Account; import com.aqiuo.service.AccountService; import org.springframework.jdbc.core.JdbcTemplate;import java.sql.Connection; import java.sql.SQLEx…...
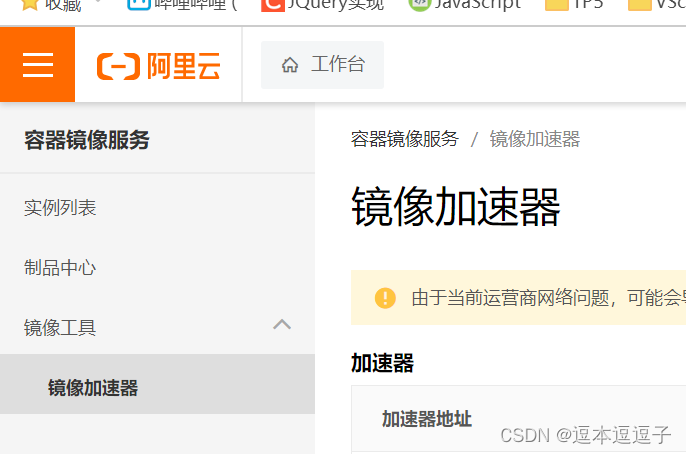
配置cendos 安装docker 配置阿里云国内加速
由于我安装的cendos是镜像版。已经被配置好了。所以只需要更新相关配置信息即可。 输入 yum update自动更新所有配置 更新完成后输入 yum list docker-ce --showduplicates | sort -r 自动查询所有可用的docker版本 输入 yum install docker-ce docker-ce-cli container…...
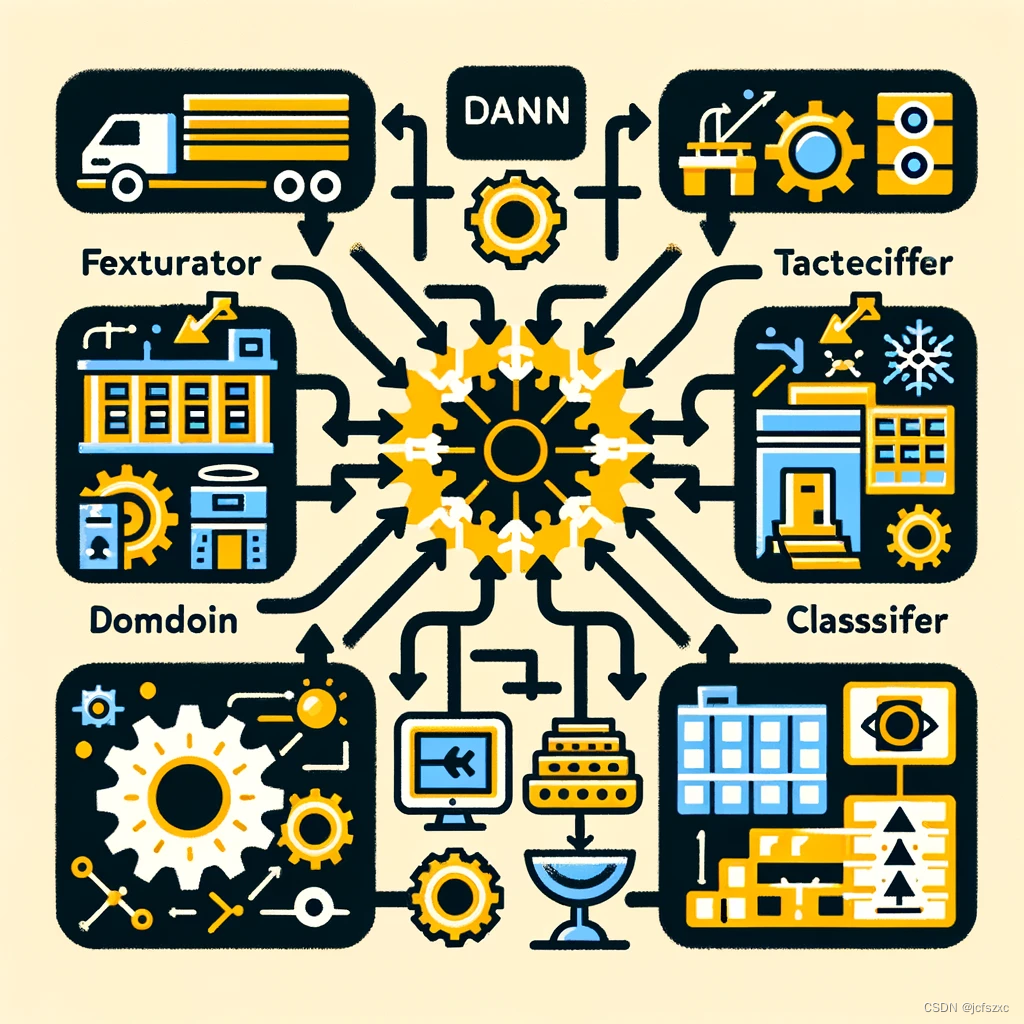
【深度学习:Domain Adversarial Neural Networks (DANN) 】领域对抗神经网络简介
【深度学习:Domain Adversarial Neural Networks】领域对抗神经网络简介 前言领域对抗神经网络DANN 模型架构DANN 训练流程DANN示例 GPT示例 前言 领域适应(DA)指的是当不同数据集的输入分布发生变化(这种变化通常被称为共变量变…...
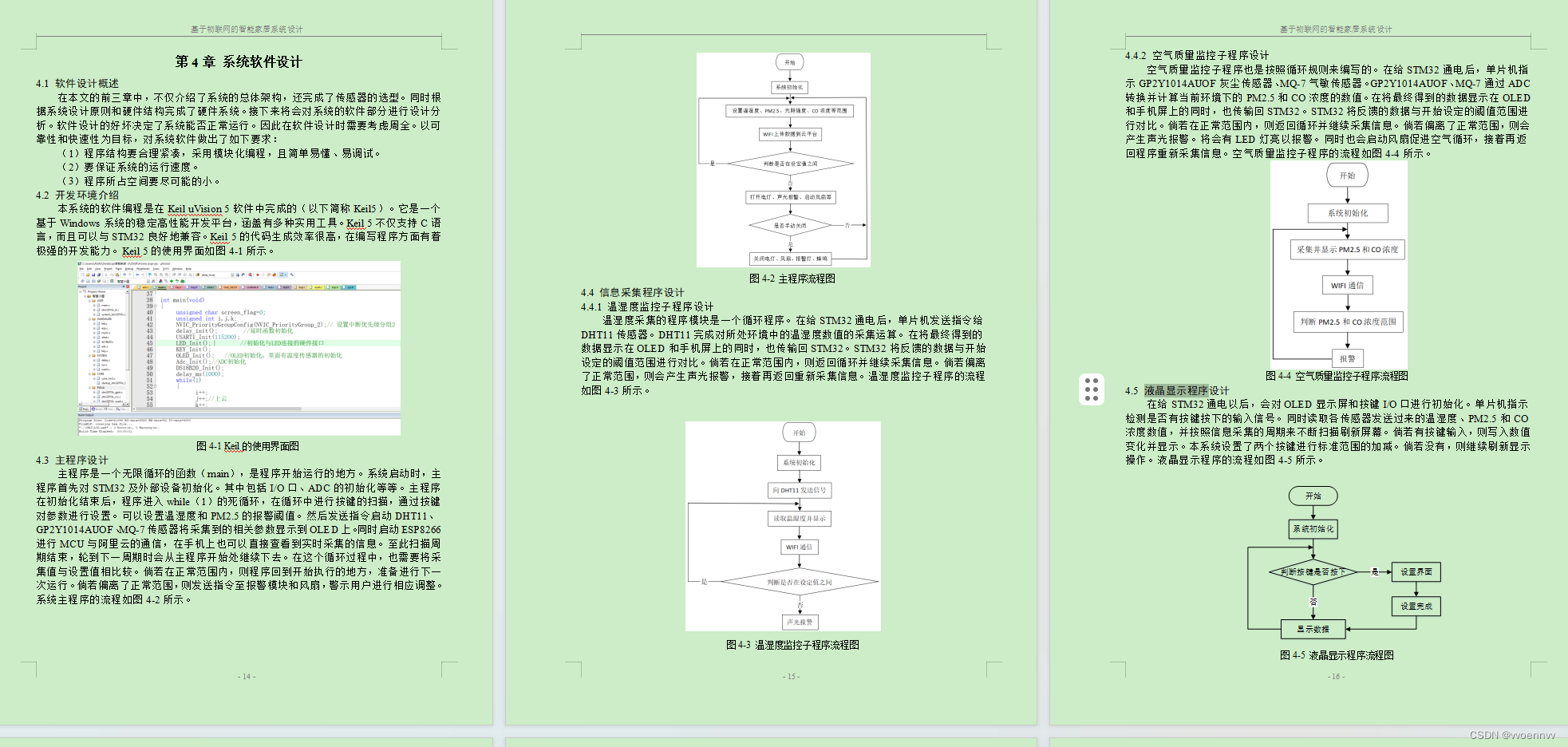
STM32 ESP8266 物联网智能温室大棚 (附源码 PCB 原理图 设计文档)
资料下载: https://download.csdn.net/download/vvoennvv/88680924 一、概述 本系统以STM32F103C8T6单片机为主控芯片,采用相关传感器构建系统硬件电路。其中使用DHT11温湿度传感器对温度和湿度的采集,MQ-7一氧化碳传感器检测CO浓度,GP2Y101…...

【DevOps-08-1】Harbor镜像仓库介绍和安装
一、简要描述 Harbor介绍Harbor安装 下载离线安装包把下载的离线安装包上传到服务器,并且解压修改Harbor配置文件启动Harbor登录Harbor管理后台Harbor管理后台首页二、Harbor介绍 前面在部署项目时,我们主要采用Jenkins推送jar包到指定服务器,再通过脚本命令让目标服务器对当…...
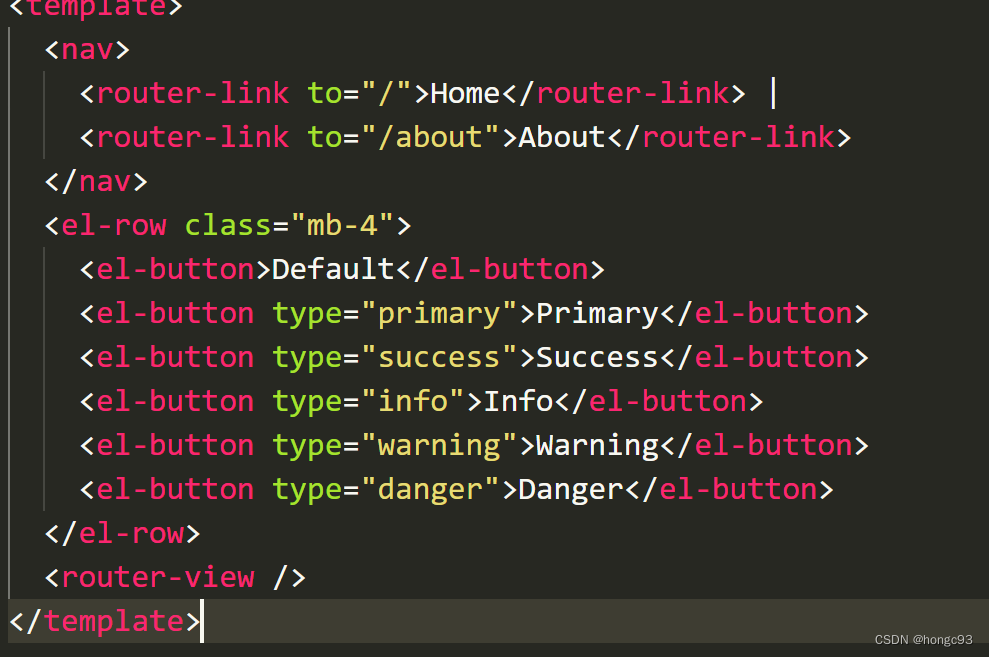
第八节 vue3新特性
系列文章目录 目录 系列文章目录 前言 操作方法 总结 前言 vue3与vue2的区别及特性。 具体信息 页面不用跟标签包裹cs...
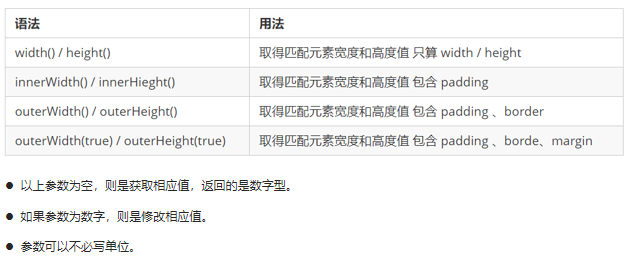
Web前端-jQuery
文章目录 jQuery1.1 jQuery 介绍1.1.1 JavaScript 库1.1.2 jQuery的概念1.1.3 jQuery的优点 1.2 jQuery 的基本使用1.2.1 jQuery 的下载1.2.2 jQuery快速入门1.2.3 jQuery入口函数1.2.4 jQuery中的顶级对象$1.2.5 jQuery 对象和 DOM 对象1.2.6. jQuery 对象和 DOM 对象转换 1.3…...

Leetcod面试经典150题刷题记录 —— 二叉搜索树篇
Leetcod面试经典150题刷题记录-系列Leetcod面试经典150题刷题记录——数组 / 字符串篇Leetcod面试经典150题刷题记录 —— 双指针篇Leetcod面试经典150题刷题记录 —— 矩阵篇Leetcod面试经典150题刷题记录 —— 滑动窗口篇Leetcod面试经典150题刷题记录 —— 哈希表篇Leetcod面…...
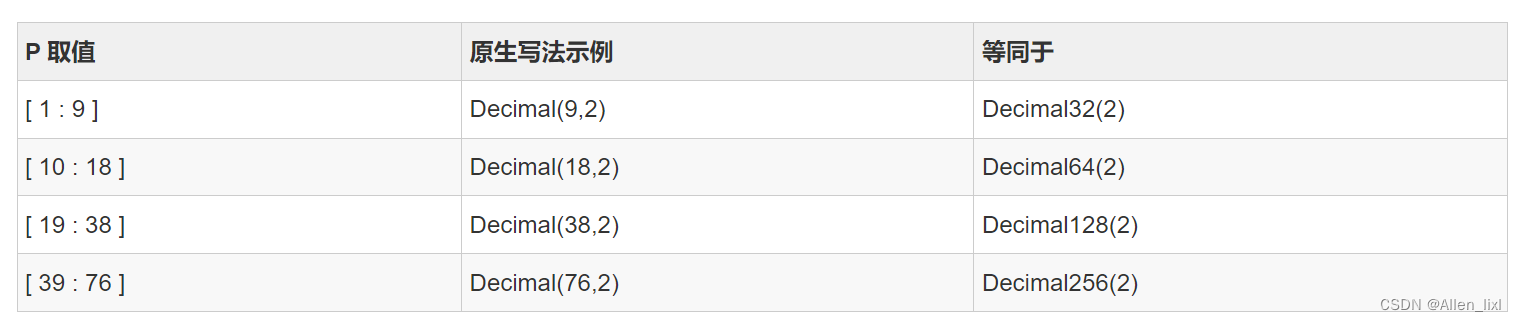
【大数据进阶第三阶段之ClickHouse学习笔记】ClickHouse的简介和使用
1、ClickHouse简介 ClickHouse是一种列式数据库管理系统(DBMS),专门用于高性能数据分析和数据仓库应用。它是一个开源的数据库系统,最初由俄罗斯搜索引擎公司Yandex开发,用于满足大规模数据分析和报告的需求。 开源地址…...
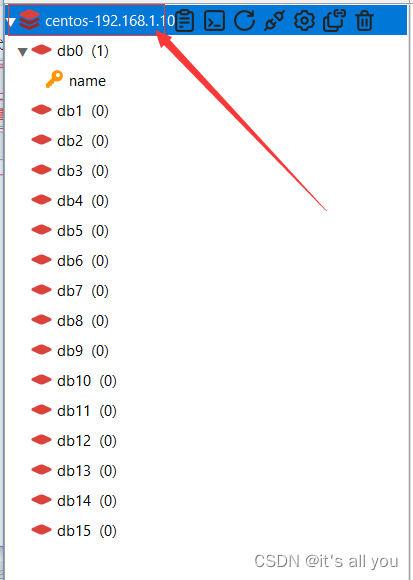
Linux下Redis6下载、安装和配置教程-2024年1月5日
Linux下Redis6下载、安装和配置教程-2024年1月5日 一、下载二、安装三、启动四、设置开机自启五、Redis的客户端1.Redis命令行客户端2.windows上的图形化桌面客户端 一、下载 1.Redis的官方下载:https://redis.io/download/ 2.网盘下载: 链接ÿ…...
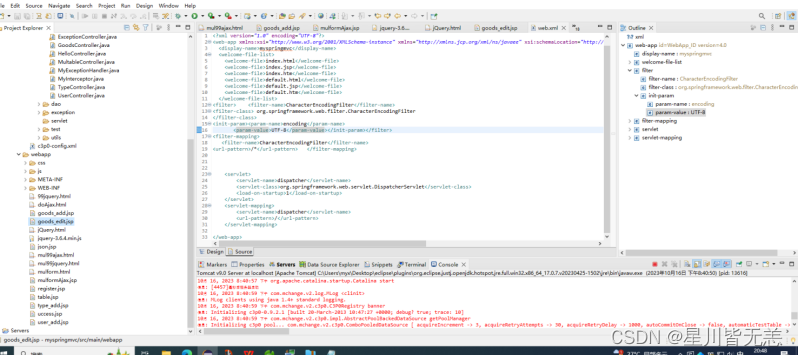
Java后端开发——Ajax、jQuery和JSON
Java后端开发——Ajax、jQuery和JSON 概述 Ajax全称是Asynchronous Javascript and XML,即异步的JavaScript和 XML。Ajax是一种Web应用技术,该技术是在JavaScript、DOM、服务器配合下,实现浏览器向服务器发送异步请求。 Ajax异步请求方式不…...
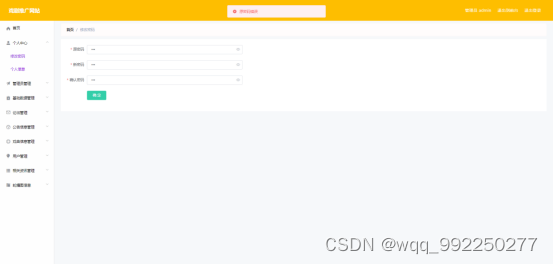
ssm基于Vue的戏剧推广网站论文
摘 要 如今社会上各行各业,都喜欢用自己行业的专属软件工作,互联网发展到这个时候,人们已经发现离不开了互联网。新技术的产生,往往能解决一些老技术的弊端问题。因为传统戏剧推广信息管理难度大,容错率低,…...

安卓adb
目录 如何开启 ADB 注意事项 如何使用 ADB ADB 能干什么 ADB(Android Debug Bridge)是一个多功能命令工具,它可以允许你与 Android 设备进行通信。它提供了多种设备权限,包括安装和调试应用,以及访问设备上未通过…...

【数位dp】【动态规划】C++算法:233.数字 1 的个数
作者推荐 【动态规划】C算法312 戳气球 本文涉及的基础知识点 动态规划 数位dp LeetCode:233数字 1 的个数 给定一个整数 n,计算所有小于等于 n 的非负整数中数字 1 出现的个数。 示例 1: 输入:n 13 输出:6 示例 2ÿ…...
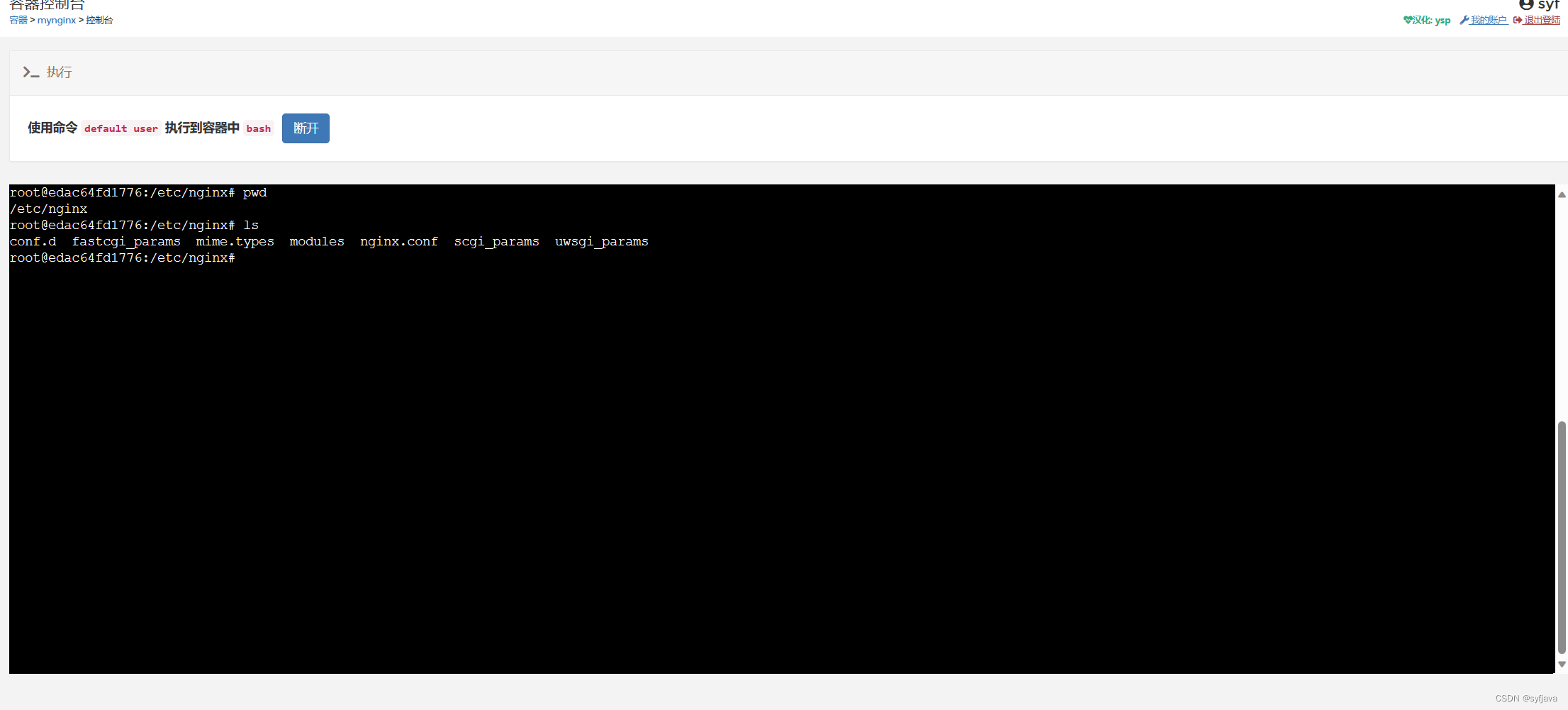
docker (portainer 安装nginx)
汉化版步骤可以参考:写文章-CSDN创作中心https://mp.csdn.net/mp_blog/creation/editor/135258056 一、创建容器 二、配置端口,以及容器卷挂载 挂载目录配置:(下方截图的目录如下,docker 改为 mydocker,用docker作为根…...

10个linux文件管理命令
1. ls – 列出目录内容 ls可能是每个Linux用户在其终端中键入的第一个命令。它允许您列出您想要的目录的内容(默认情况下是当前目录),包括文件和其他嵌套目录。 它有很多选择,所以最好使用 --help 来获得一些帮助。此标志返回所…...
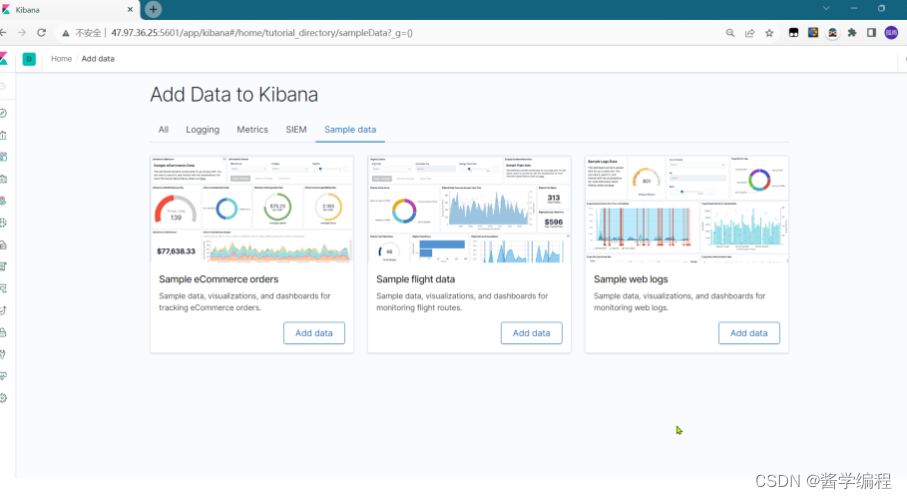
实战:使用docker容器化服务与文件挂载-2
接着上文,演示Elasticsearch 和 Kibana 的安装,并讲解文件挂载 Elasticsearch of Docker (Kibana) 1、Elasticsearch 安装 ElasticSearch 使用 Docker 安装:https://www.yuque.com/zhangshuaiyin/guli-mall/dwrp5b 1.…...

联合union
//————联合:union 1.联合的定义 联合也是一种特殊的自定义类型 #include<stdio.h> union Un//Un为联合标签 { int a; char c; }; struct St { int a; int b; }; int main() { union Un u; printf("%d\n",sizeof(u));//…...

如何在 Umi /Umi 4.0 中配置自动删除 console.log 语句?
背景,开发时需要console.log 日志,再生产、uat 、sit不想看到日志打印信息 方案1、代码规范eslint校验"no-console": true, //console.log 方案2、bable 插件 babel-plugin-transform-remove-console 配置在.umirx.ts/js中 export default…...
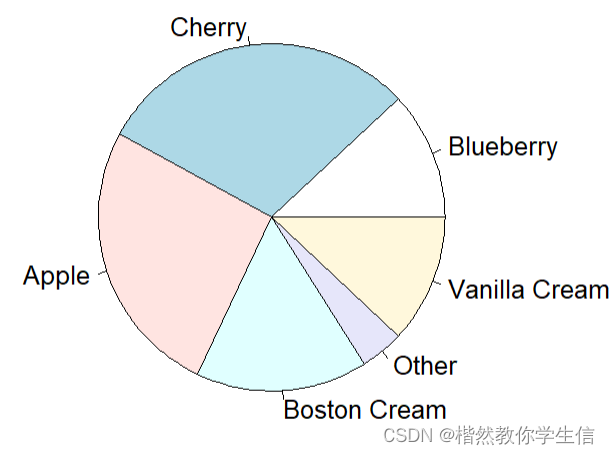
(生物信息学)R语言绘图初-中-高级——3-10分文章必备——饼图(初级)
生物信息学文章的发表要求除了思路和热点以外,图片绘制是否精美也是十分重要的,本专栏为(生物信息学)R语言绘图初-中-高级——3-10分文章必备,主要通过大量文献,总结3-10分文章中高频出现的各种图片,并给大家提供图片复现的R语言代码,及图片识读。 本专栏将向大家介绍…...
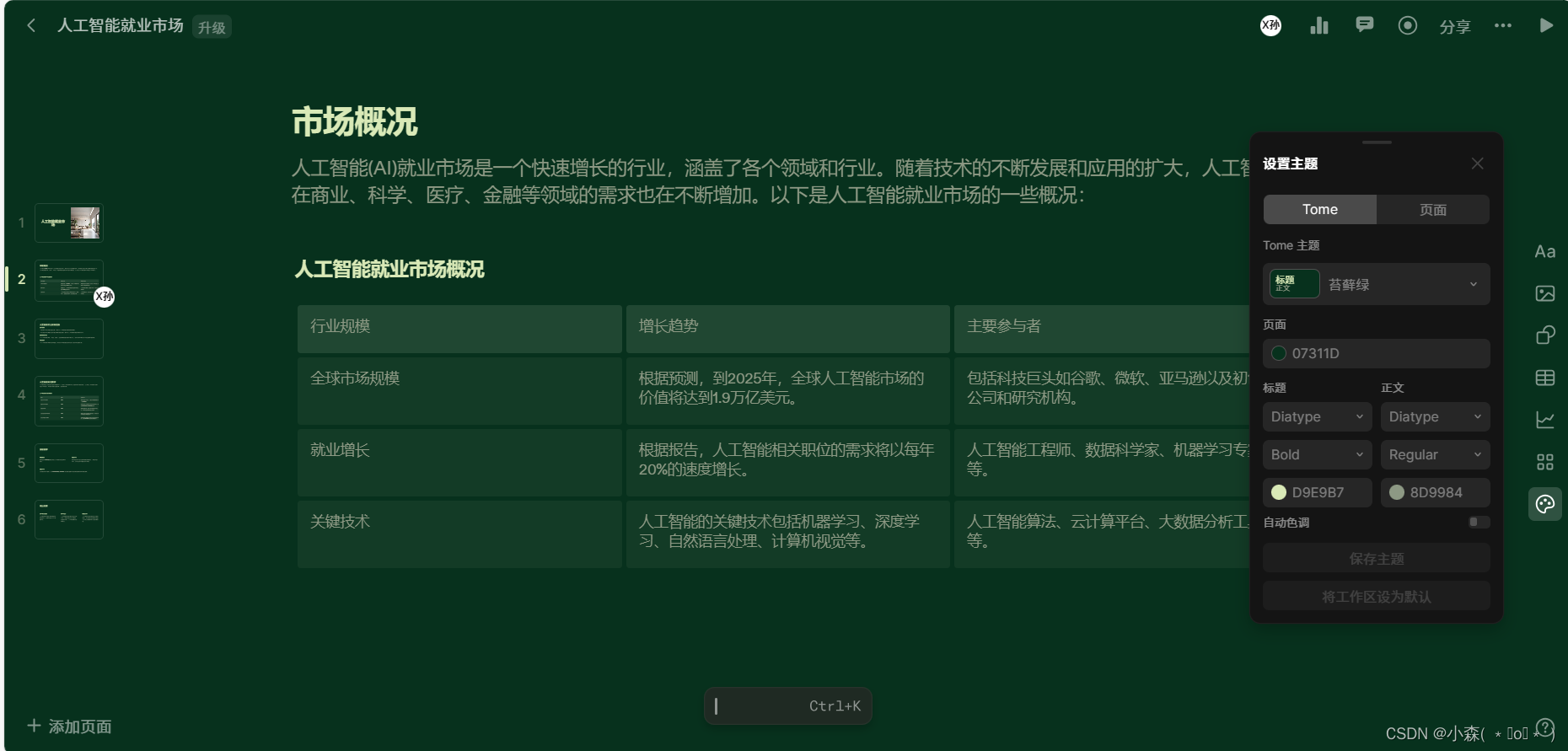
AI ppt生成器 Tome
介绍 一款 AI 驱动的 PPT/幻灯片内容辅助生成工具。只需要输入一个标题或者一段特定的描述,AI 便会自动生成一套包括标题、大纲、内容、配图的完整 PPT。 Tome平台只需要用户输入一句话,就可以自动生成完整的PPT,包括文字和图片。功能非常强…...

Linux与Windows下追踪网络路由:traceroute、tracepath与tracert命令详解
简介 在进行网络诊断或排查问题时,了解数据包从源主机到目标主机之间的具体传输路径至关重要。Linux系统提供了traceroute和tracepath工具来实时显示链路路径信息,而Windows则使用了tracert命令实现相同的功能。本文将详细介绍这三个命令的用法及其在不…...
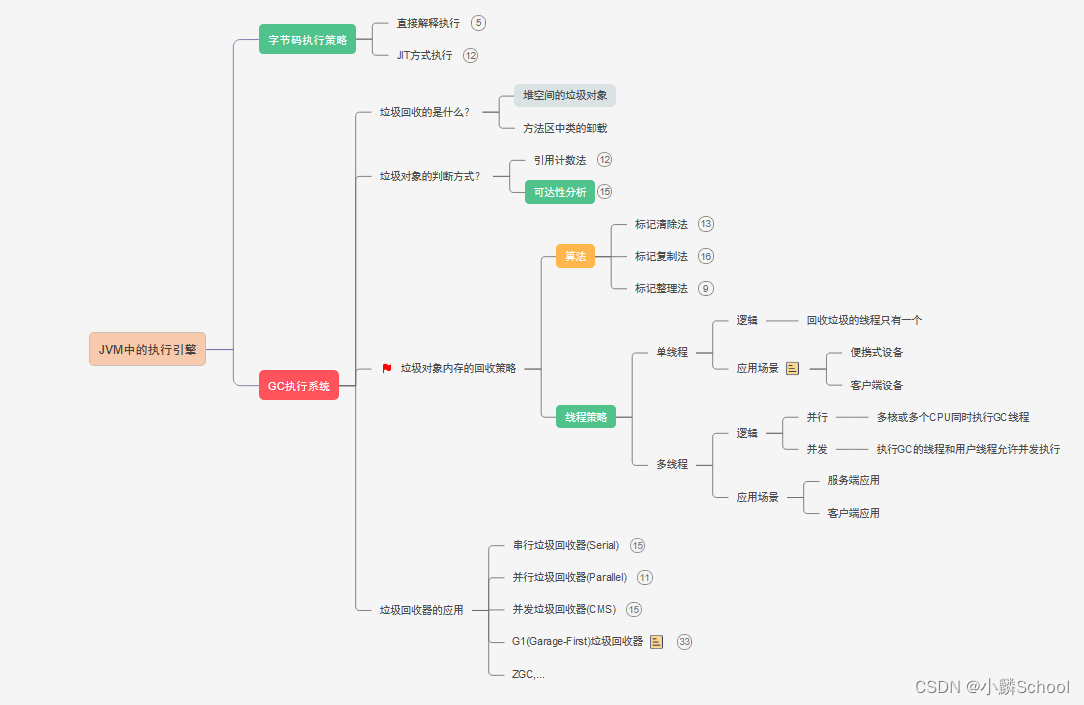
图解JVM (及一些垃圾回收\GC相关面试题 持续更新)
垃圾回收,顾名思义就是释放垃圾占用的空间,从而提升程序性能,防止内存泄露。当一个对象不再被需要时,该对象就需要被回收并释放空间。 Java 内存运行时数据区域包括程序计数器、虚拟机栈、本地方法栈、堆等区域。其中,…...
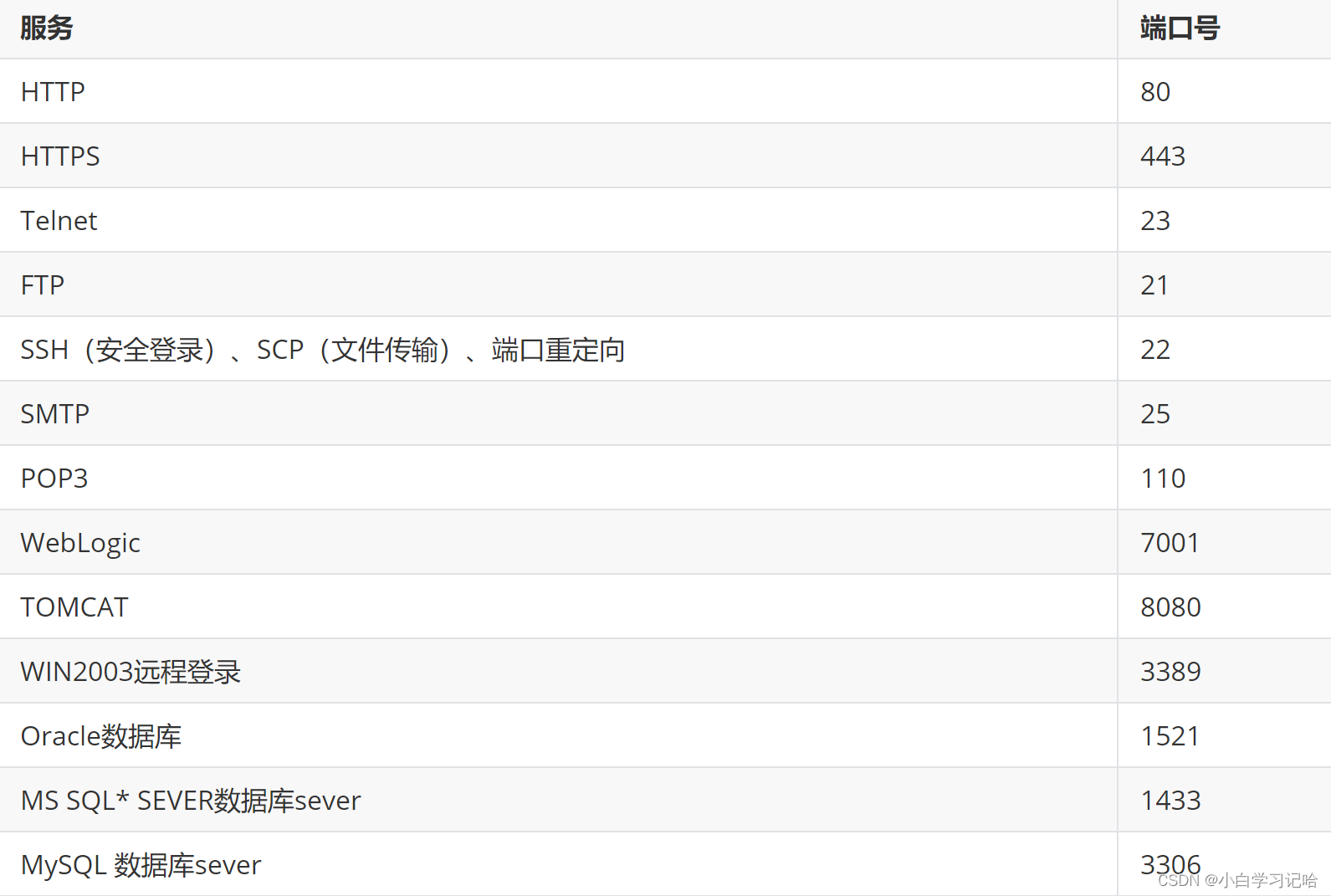
linux 系统安全及应用
一、账号安全基本措施 1.系统账号清理 1.将用户设置为无法登录 /sbin/nologin shell——/sbin/nologin却比较特殊,所谓“无法登陆”指的仅是这个用户无法使用bash或其他shell来登陆系统而已,并不是说这个账号就无法使用系统资源。举例来说,…...