高明网站建设公司/seo教程下载
前言
学习资料:
TensorRT 源码示例
B站视频:TensorRT 教程 | 基于 8.6.1 版本
视频配套代码 cookbook
参考源码:cookbook → 07-Tool → trtexec
官方文档:trtexec
在 TensorRT 的安装目录 xxx/TensorRT-8.6.1.6/bin
下有命令行工具 trtexec,主要功能:
(1)由 ONNX 文件生成 TensorRT 引擎并序列化为 plan 文件
(2)查看 ONNX 或 plan 文件的网络逐层信息
(3)模型性能测试,即测试 TensorRT 引擎在随机输入或给定输入下的性能
示例一:解析 ONNX 生成引擎并推理测速
目录结构
├── resnet18.onnx
└── test_trtexec.sh
在终端中临时添加环境变量后运行脚本
export PATH=xxx/TensorRT-8.6.1.6/bin${PATH:+:${PATH}}
bash test_trtexec.sh
脚本内容如下,使用之前 Python 篇生成的 ResNet18 ONNX 文件生成 TensorRT 引擎,并且会做推理测试,输出日志信息存储在 result-fp32.txt
中。
trtexec \--onnx=resnet18.onnx \--saveEngine=resnet18-fp32.plan \--minShapes=x:1x3x224x224 \--optShapes=x:4x3x224x224 \--maxShapes=x:16x3x224x224 \--memPoolSize=workspace:1024MiB \--verbose \> result-fp32.txt 2>&1
>
为输出重定向操作符,将输出重定向到指定位置。
2>&1
中 2
为标准错误流 stderr
,1
为标准输出流 stdout
。表示把报错信息和正常输出一起保存到文件中
命令行常用选项
(1)构建阶段
--onnx=resnet18.onnx 指定模型文件
--saveEngine=resnet18-fp32.plan 输出引擎文件名--minShapes=x:1x3x224x224
--optShapes=x:4x3x224x224
--maxShapes=x:16x3x224x224 输入形状的最小值、常见值、最大值
--memPoolSize=workspace:1024MiB 优化过程可使用的显存最大值
--verbose 打印详细日志, 但无法设置日志等级
--skipInference 只创建引擎不进行推理--fp16
--int8
--noTF32
--best 指定引擎精度
--sparsity=[disable|enable|force] 指定稀疏性
--builderOptimizationLevel=5 设置优化等级(默认2)
--timingCacheFile=timing.cache 指定输出优化计时缓存文件
--profilingVerbosity=detailed 构建期保留更多的逐层信息
(2)运行阶段
--loadEngine=resnet18-fp32.plan 读取引擎文件
--shapes=x:4x3x224x224 指定输入形状
--warmUp=1000 预热阶段最短运行时间(单位:ms)
--duration=10 测试阶段最短运行时间(单位:s)
--iterations=100 测试阶段运行最小迭代次数
--useCudaGraph 使用 CudaGraph 捕获和执行推理过程
--noDataTransfers 关闭 Host 和 Device 之间数据传输
--streams=2 使用多个 stream 运行推理
--dumpProfile 显示逐层性能数据
--exportProfile=layerProfile.txt 保存逐层性能数据
解析输出日志信息
输出的日志信息非常多,分块进行解读
(1)各种参数配置
=== Model Options ===
Format: ONNX
Model: resnet18.onnx
Output:=== Build Options ===
Max batch: explicit batch
Memory Pools: workspace: 1024 MiB, dlaSRAM: default, dlaLocalDRAM: default, dlaGlobalDRAM: default
minTiming: 1
avgTiming: 8
Precision: FP32
LayerPrecisions:
Layer Device Types:
Calibration:
Refit: Disabled
Version Compatible: Disabled
TensorRT runtime: full
Lean DLL Path:
Tempfile Controls: { in_memory: allow, temporary: allow }
Exclude Lean Runtime: Disabled
Sparsity: Disabled
Safe mode: Disabled
Build DLA standalone loadable: Disabled
Allow GPU fallback for DLA: Disabled
DirectIO mode: Disabled
Restricted mode: Disabled
Skip inference: Disabled
Save engine: resnet18-fp32.plan
Load engine:
Profiling verbosity: 0
Tactic sources: Using default tactic sources
timingCacheMode: local
timingCacheFile:
Heuristic: Disabled
Preview Features: Use default preview flags.
MaxAuxStreams: -1
BuilderOptimizationLevel: -1
Input(s)s format: fp32:CHW
Output(s)s format: fp32:CHW
Input build shape: x=1x3x224x224+4x3x224x224+16x3x224x224
Input calibration shapes: model=== System Options ===
Device: 0
DLACore:
Plugins:
setPluginsToSerialize:
dynamicPlugins:
ignoreParsedPluginLibs: 0=== Inference Options ===
Batch: Explicit
Input inference shape: x=4x3x224x224
Iterations: 10
Duration: 3s (+ 200ms warm up)
Sleep time: 0ms
Idle time: 0ms
Inference Streams: 1
ExposeDMA: Disabled
Data transfers: Enabled
Spin-wait: Disabled
Multithreading: Disabled
CUDA Graph: Disabled
Separate profiling: Disabled
Time Deserialize: Disabled
Time Refit: Disabled
NVTX verbosity: 0
Persistent Cache Ratio: 0
Inputs:=== Reporting Options ===
Verbose: Enabled
Averages: 10 inferences
Percentiles: 90,95,99
Dump refittable layers:Disabled
Dump output: Disabled
Profile: Disabled
Export timing to JSON file:
Export output to JSON file:
Export profile to JSON file: === Device Information ===
Selected Device: NVIDIA GeForce RTX 3090
Compute Capability: 8.6
SMs: 82
Device Global Memory: 24258 MiB
Shared Memory per SM: 100 KiB
Memory Bus Width: 384 bits (ECC disabled)
Application Compute Clock Rate: 1.695 GHz
Application Memory Clock Rate: 9.751 GHzNote: The application clock rates do not reflect the actual clock rates that the GPU is currently running at.TensorRT version: 8.6.1
"较长的一段内容在加载标准插件"
Loading standard plugins
Registered plugin creator - ::BatchedNMSDynamic_TRT version 1
...
Registered plugin creator - ::VoxelGeneratorPlugin version 1
[MemUsageChange] Init CUDA: CPU +13, GPU +0, now: CPU 19, GPU 774 (MiB)
Trying to load shared library libnvinfer_builder_resource.so.8.6.1
Loaded shared library libnvinfer_builder_resource.so.8.6.1
[MemUsageChange] Init builder kernel library: CPU +1450, GPU +266, now: CPU 1545, GPU 1034 (MiB)
CUDA lazy loading is enabled.
(2)Parse 过程
Start parsing network model.
----------------------------------------------------------------
"onnx 模型相关信息"
Input filename: resnet18.onnx
ONNX IR version: 0.0.7
Opset version: 12
Producer name: pytorch
Producer version: 2.0.1
Domain:
Model version: 0
Doc string:
----------------------------------------------------------------
"已加载插件"
Plugin creator already registered - ::xxx"添加输入、构建网络"
Adding network input: x with dtype: float32, dimensions: (-1, 3, 224, 224)
Registering tensor: x for ONNX tensor: x
Importing initializer: fc.weight
Importing initializer: fc.bias
Importing initializer: onnx::Conv_193
...
Importing initializer: onnx::Conv_251"每个 Parsing node 作为一段进行查看, 主要关于输入输出和层"
Parsing node: /conv1/Conv [Conv]
Searching for input: x
Searching for input: onnx::Conv_193
Searching for input: onnx::Conv_194
/conv1/Conv [Conv] inputs: [x -> (-1, 3, 224, 224)[FLOAT]], [onnx::Conv_193 -> (64, 3, 7, 7)[FLOAT]], [onnx::Conv_194 -> (64)[FLOAT]],
Convolution input dimensions: (-1, 3, 224, 224)
Registering layer: /conv1/Conv for ONNX node: /conv1/Conv
Using kernel: (7, 7), strides: (2, 2), prepadding: (3, 3), postpadding: (3, 3), dilations: (1, 1), numOutputs: 64
Convolution output dimensions: (-1, 64, 112, 112)
Registering tensor: /conv1/Conv_output_0 for ONNX tensor: /conv1/Conv_output_0
/conv1/Conv [Conv] outputs: [/conv1/Conv_output_0 -> (-1, 64, 112, 112)[FLOAT]], Parsing node: /relu/Relu [Relu]
Searching for input: /conv1/Conv_output_0
/relu/Relu [Relu] inputs: [/conv1/Conv_output_0 -> (-1, 64, 112, 112)[FLOAT]],
Registering layer: /relu/Relu for ONNX node: /relu/Relu
Registering tensor: /relu/Relu_output_0 for ONNX tensor: /relu/Relu_output_0
/relu/Relu [Relu] outputs: [/relu/Relu_output_0 -> (-1, 64, 112, 112)[FLOAT]],
...
Finished parsing network model. Parse time: 0.144613"对模型进行的一系列优化和融合操作, 优化后的层数, 耗时等信息"
Original: 53 layers
After dead-layer removal: 53 layers
Graph construction completed in 0.00150762 seconds.
Running: ConstShuffleFusion on fc.bias
ConstShuffleFusion: Fusing fc.bias with (Unnamed Layer* 56) [Shuffle]
After Myelin optimization: 52 layers
...
After scale fusion: 49 layers
...
After dupe layer removal: 24 layers
After final dead-layer removal: 24 layers
After tensor merging: 24 layers
After vertical fusions: 24 layers
After dupe layer removal: 24 layers
After final dead-layer removal: 24 layers
After tensor merging: 24 layers
After slice removal: 24 layers
After concat removal: 24 layers
Trying to split Reshape and strided tensor
Graph optimization time: 0.0239815 seconds.
Building graph using backend strategy 2
Local timing cache in use. Profiling results in this builder pass will not be stored.
Constructing optimization profile number 0 [1/1].
Applying generic optimizations to the graph for inference.
Reserving memory for host IO tensors. Host: 0 bytes
TODO:
(1)哪些层可以优化?如何优化?
(2)优化前后结构有何区别?
(3)自动优化
=============== Computing costs for /conv1/Conv + /relu/Relu
*************** Autotuning format combination: Float(150528,50176,224,1) -> Float(802816,12544,112,1) ***************
--------------- Timing Runner: /conv1/Conv + /relu/Relu (CaskConvolution[0x80000009])
"Tactic Name: 策略名称, Tactic: 策略编号, Time: 耗时"
Tactic Name: ampere_scudnn_128x128_relu_medium_nn_v1 Tactic: 0xf067e6205da31c2e Time: 0.11264
...
Tactic Name: ampere_scudnn_128x64_relu_medium_nn_v1 Tactic: 0xf64396b97c889179 Time: 0.0694857
"分析耗时, 最快策略编号及其耗时"
/conv1/Conv + /relu/Relu (CaskConvolution[0x80000009]) profiling completed in 0.124037 seconds. Fastest Tactic: 0xf64396b97c889179 Time: 0.0694857
--------------- Timing Runner: /conv1/Conv + /relu/Relu (CudnnConvolution[0x80000000])
"无有效策略, 跳过"
CudnnConvolution has no valid tactics for this config, skipping
--------------- Timing Runner: /conv1/Conv + /relu/Relu (CaskFlattenConvolution[0x80000036])
CaskFlattenConvolution has no valid tactics for this config, skipping
>>>>>>>>>>>>>>> Chose Runner Type: CaskConvolution Tactic: 0xf64396b97c889179*************** Autotuning format combination: Float(150528,1,672,3) -> Float(802816,1,7168,64) ***************
--------------- Timing Runner: /conv1/Conv + /relu/Relu (CaskConvolution[0x80000009])
Tactic Name: sm80_xmma_fprop_implicit_gemm_indexed_f32f32_f32f32_f32_nhwckrsc_nhwc_tilesize64x64x8_stage3_warpsize1x4x1_g1_ffma_aligna4_alignc4 Tactic: 0x19b688348f983aa0 Time: 0.155648
...
Tactic Name: sm80_xmma_fprop_implicit_gemm_indexed_f32f32_f32f32_f32_nhwckrsc_nhwc_tilesize128x32x8_stage3_warpsize2x2x1_g1_ffma_aligna4_alignc4 Tactic: 0xa6448a1e79f1ca6f Time: 0.174373
/conv1/Conv + /relu/Relu (CaskConvolution[0x80000009]) profiling completed in 0.0186587 seconds. Fastest Tactic: 0xf231cca3335919a4 Time: 0.063488
--------------- Timing Runner: /conv1/Conv + /relu/Relu (CaskFlattenConvolution[0x80000036])
CaskFlattenConvolution has no valid tactics for this config, skipping
>>>>>>>>>>>>>>> Chose Runner Type: CaskConvolution Tactic: 0xf231cca3335919a4*************** Autotuning format combination: Float(50176,1:4,224,1) -> Float(802816,12544,112,1) ***************
--------------- Timing Runner: /conv1/Conv + /relu/Relu (CaskConvolution[0x80000009])
Tactic Name: sm80_xmma_fprop_implicit_gemm_indexed_f32f32_tf32f32_f32_nhwckrsc_nchw_tilesize128x128x16_stage4_warpsize2x2x1_g1_tensor16x8x8_alignc4 Tactic: 0xe8f7b6a5bab325f8 Time: 0.35957
Tactic Name: sm80_xmma_fprop_implicit_gemm_indexed_f32f32_tf32f32_f32_nhwckrsc_nchw_tilesize128x128x16_stage4_warpsize2x2x1_g1_tensor16x8x8 Tactic: 0xe0a307ffe0ffb6a5 Time: 0.344503
/conv1/Conv + /relu/Relu (CaskConvolution[0x80000009]) profiling completed in 0.0105646 seconds. Fastest Tactic: 0xe0a307ffe0ffb6a5 Time: 0.344503
--------------- Timing Runner: /conv1/Conv + /relu/Relu (CaskFlattenConvolution[0x80000036])
CaskFlattenConvolution has no valid tactics for this config, skipping
>>>>>>>>>>>>>>> Chose Runner Type: CaskConvolution Tactic: 0xe0a307ffe0ffb6a5*************** Autotuning format combination: Float(50176,1:4,224,1) -> Float(200704,1:4,1792,16) ***************
--------------- Timing Runner: /conv1/Conv + /relu/Relu (CaskConvolution[0x80000009])
Tactic Name: ampere_scudnn_128x64_sliced1x2_ldg4_relu_exp_large_nhwc_tn_v1 Tactic: 0xbdfdef6b84f7ccc9 Time: 0.279845
...
Tactic Name: sm80_xmma_fprop_implicit_gemm_indexed_wo_smem_f32f32_tf32f32_f32_nhwckrsc_nhwc_tilesize128x16x32_stage1_warpsize4x1x1_g1_tensor16x8x8 Tactic: 0xae48d3ccfe1edfcd Time: 0.0678278
/conv1/Conv + /relu/Relu (CaskConvolution[0x80000009]) profiling completed in 0.0911707 seconds. Fastest Tactic: 0x9cb304e2edbc1221 Time: 0.059392
--------------- Timing Runner: /conv1/Conv + /relu/Relu (CaskFlattenConvolution[0x80000036])
CaskFlattenConvolution has no valid tactics for this config, skipping
>>>>>>>>>>>>>>> Chose Runner Type: CaskConvolution Tactic: 0x9cb304e2edbc1221
..."计算重新格式化成本"
=============== Computing reformatting costs
=============== Computing reformatting costs:
*************** Autotuning Reformat: Float(150528,50176,224,1) -> Float(150528,1,672,3) ***************
--------------- Timing Runner: Optimizer Reformat(x -> <out>) (Reformat[0x80000006])
Tactic: 0x00000000000003e8 Time: 0.00663667
Tactic: 0x00000000000003ea Time: 0.016319
Tactic: 0x0000000000000000 Time: 0.0147323
Optimizer Reformat(x -> <out>) (Reformat[0x80000006]) profiling completed in 0.00680021 seconds. Fastest Tactic: 0x00000000000003e8 Time: 0.00663667
*************** Autotuning Reformat: Float(150528,50176,224,1) -> Float(50176,1:4,224,1) ***************
--------------- Timing Runner: Optimizer Reformat(x -> <out>) (Reformat[0x80000006])
Tactic: 0x00000000000003e8 Time: 0.0116016
Tactic: 0x00000000000003ea Time: 0.0174405
Tactic: 0x0000000000000000 Time: 0.0147739
Optimizer Reformat(x -> <out>) (Reformat[0x80000006]) profiling completed in 0.00589612 seconds. Fastest Tactic: 0x00000000000003e8 Time: 0.0116016
...
=============== Computing reformatting costs"添加重新格式化层"
Adding reformat layer: Reformatted Input Tensor 0 to /fc/Gemm (/avgpool/GlobalAveragePool_output_0) from Float(512,1,1,1) to Float(128,1:4,128,128)
Adding reformat layer: Reformatted Input Tensor 0 to reshape_after_/fc/Gemm (/fc/Gemm_out_tensor) from Float(250,1:4,250,250) to Float(1000,1,1,1)
Formats and tactics selection completed in 6.81485 seconds.
After reformat layers: 26 layers
Total number of blocks in pre-optimized block assignment: 26
Detected 1 inputs and 1 output network tensors.
"内存、显存、临时内存"
Layer: /conv1/Conv + /relu/Relu Host Persistent: 4016 Device Persistent: 75776 Scratch Memory: 0
...
Layer: /fc/Gemm Host Persistent: 7200 Device Persistent: 0 Scratch Memory: 0
Skipped printing memory information for 3 layers with 0 memory size i.e. Host Persistent + Device Persistent + Scratch Memory == 0.
Total Host Persistent Memory: 86272
Total Device Persistent Memory: 75776
Total Scratch Memory: 4608
"峰值"
[MemUsageStats] Peak memory usage of TRT CPU/GPU memory allocators: CPU 25 MiB, GPU 98 MiB"块偏移, 用于管理和分配不同层的内存块, 以便在GPU上高效执行计算"
[BlockAssignment] Started assigning block shifts. This will take 27 steps to complete.
STILL ALIVE: Started step 26 of 27
[BlockAssignment] Algorithm ShiftNTopDown took 0.430364ms to assign 4 blocks to 27 nodes requiring 77074944 bytes.
Total number of blocks in optimized block assignment: 4
Total Activation Memory: 77074944
Finalize: /conv1/Conv + /relu/Relu Set kernel index: 0
...
Finalize: /fc/Gemm Set kernel index: 10
Total number of generated kernels selected for the engine: 11
Kernel: 0 CASK_STATIC
...
Kernel: 10 CASK_STATIC
"禁用未使用的策略源, 提高引擎生成的效率"
Disabling unused tactic source: JIT_CONVOLUTIONS
"引擎生成总耗时"
Engine generation completed in 6.96604 seconds.
"删除计时缓存"
Deleting timing cache: 144 entries, served 172 hits since creation.
Engine Layer Information:
Layer(CaskConvolution): /conv1/Conv + /relu/Relu, Tactic: 0xf64396b97c889179, x (Float[-1,3,224,224]) -> /relu/Relu_output_0 (Float[-1,64,112,112])
...
Layer(NoOp): reshape_after_/fc/Gemm, Tactic: 0x0000000000000000, Reformatted Input Tensor 0 to reshape_after_/fc/Gemm (Float[-1,1000,1,1]) -> y (Float[-1,1000])
[MemUsageChange] TensorRT-managed a
Adding 1 engine(s) to plan file.
Engine built in 18.0591 sec.
Loaded engine size: 73 MiB
"反序列化需20415微秒"
Deserialization required 20415 microseconds.
"反序列化中内存变化"
[MemUsageChange] TensorRT-managed allocation in engine deserialization: CPU +0, GPU +72, now: CPU 0, GPU 72 (MiB)
Engine deserialized in 0.0260023 sec.
Total per-runner device persistent memory is 75776
Total per-runner host persistent memory is 86272
Allocated activation device memory of size 77074944
"执行上下文创建过程中内存变化"
[MemUsageChange] TensorRT-managed allocation in IExecutionContext creation: CPU +0, GPU +74, now: CPU 0, GPU 146 (MiB)
TODO:
(1)策略名称包含了关于硬件架构、操作类型、数据类型、优化级别等信息,具体每一项代表了什么含义?
(2)Autotuning format combination 自动优化时使用不同的数据格式,这些输入输出形状是如何确定的?
(4)推理测速
CUDA lazy loading is enabled.
Setting persistentCacheLimit to 0 bytes.
Using enqueueV3.
Using random values for input x
Input binding for x with dimensions 4x3x224x224 is created.
Output binding for y with dimensions 4x1000 is created.
Starting inference
Warmup completed 185 queries over 200 ms
Timing trace has 2523 queries over 3.00267 s=== Trace details ===
Trace averages of 10 runs:
Average on 10 runs - GPU latency: 1.11339 ms - Host latency: 1.52892 ms (enqueue 0.235403 ms)
...
Average on 10 runs - GPU latency: 1.11299 ms - Host latency: 1.55708 ms (enqueue 0.262207 ms)=== Performance summary ===
Throughput: 840.251 qps
Latency: min = 1.4353 ms, max = 5.20972 ms, mean = 1.62134 ms, median = 1.55011 ms, percentile(90%) = 1.91345 ms, percentile(95%) = 2.07056 ms, percentile(99%) = 2.51172 ms
Enqueue Time: min = 0.174561 ms, max = 4.94312 ms, mean = 0.261935 ms, median = 0.261475 ms, percentile(90%) = 0.29071 ms, percentile(95%) = 0.315125 ms, percentile(99%) = 0.348938 ms
H2D Latency: min = 0.362305 ms, max = 0.622559 ms, mean = 0.430081 ms, median = 0.433594 ms, percentile(90%) = 0.449829 ms, percentile(95%) = 0.45874 ms, percentile(99%) = 0.493317 ms
GPU Compute Time: min = 1.05573 ms, max = 4.69727 ms, mean = 1.18524 ms, median = 1.11011 ms, percentile(90%) = 1.48682 ms, percentile(95%) = 1.6311 ms, percentile(99%) = 2.10425 ms
D2H Latency: min = 0.00427246 ms, max = 0.0371094 ms, mean = 0.00601927 ms, median = 0.00561523 ms, percentile(90%) = 0.00732422 ms, percentile(95%) = 0.00775146 ms, percentile(99%) = 0.0085144 ms
Total Host Walltime: 3.00267 s
Total GPU Compute Time: 2.99037 s
"GPU计算时间不稳定, 方差系数=18.1606%, 锁定GPU时钟频率或添加--useSpinWait可能提高稳定性"
* GPU compute time is unstable, with coefficient of variance = 18.1606%.If not already in use, locking GPU clock frequency or adding --useSpinWait may improve the stability.
Explanations of the performance metrics are printed in the verbose logs.
"性能指标说明, 此处注释根据官方文档中的解释进行补充"
=== Explanations of the performance metrics ===
"预热过后第一个 query 加入队列到最后一个 query 完成的时间"
Total Host Walltime: the host walltime from when the first query (after warmups) is enqueued to when the last query is completed."GPU执行一个 query 的延迟"
GPU Compute Time: the GPU latency to execute the kernels for a query."GPU执行所有 query 的延迟"
"如果明显比 Total Host Walltime 短, 可能由于 host 端开销或数据传输导致 GPU 利用低效"
Total GPU Compute Time: the summation of the GPU Compute Time of all the queries. If this is significantly shorter than Total Host Walltime, the GPU may be under-utilized because of host-side overheads or data transfers."吞吐量: 每秒完成 query 数量"
"如果明显小于 GPU Compute Time 的倒数, 可能由于 host 端开销或数据传输导致 GPU 利用低效"
"throughput = the number of inferences / Total Host Walltime"
"使用 CUDA graphs(--useCudaGraph) 或禁用 H2D/D2H 传输(--noDataTransfer) 可能会提高 GPU 利用率"
"检测到 GPU 未充分利用时, 输出日志会提供相关指导"
Throughput: the observed throughput computed by dividing the number of queries by the Total Host Walltime. If this is significantly lower than the reciprocal of GPU Compute Time, the GPU may be under-utilized because of host-side overheads or data transfers."query 排队的 host 延迟"
"如果比 GPU Compute Time 长, GPU 利用低效, 吞吐量可能由 host 端开销主导"
"包括调用 H2D/D2H CUDA APIs、运行 host-side heuristics(host端启发式算法)、启动 CUDA 内核"
"使用 CUDA graphs(--useCudaGraph) 可以减少 Enqueue Time"
Enqueue Time: the host latency to enqueue a query. If this is longer than GPU Compute Time, the GPU may be under-utilized.H2D Latency: the latency for host-to-device data transfers for input tensors of a single query.D2H Latency: the latency for device-to-host data transfers for output tensors of a single query."官方文档中为 Host Latency, 单个推理的延迟"
"Host Latency = H2D Latency + GPU Compute Time + D2H Latency"
Latency: the summation of H2D Latency, GPU Compute Time, and D2H Latency. This is the latency to infer a single query.
query 在官方文档中是 inference,理解为一次推理过程;host-side heuristics 翻译为主机端启发式算法,暂不理解具体是什么。
下图源于官方文档,辅助理解推理过程。
示例二:7项功能
# 当命令返回值不等于0时, 立刻退出脚本, 不会执行后续命令
set -e
# 执行每个命令前, 打印命令及其参数
set -xclear
rm -rf ./*log ./*.plan ./*.cache ./*.lock ./*.json ./*.raw# 01 运行onnx
trtexec \--onnx=resnet18.onnx \> 01-run_onnx.log 2>&1# 02 parse onnx生成engine
trtexec \--onnx=resnet18.onnx \--saveEngine=resnet18.plan \--timingCacheFile=resnet18.cache \--minShapes=x:1x3x224x224 \--optShapes=x:4x3x224x224 \--maxShapes=x:16x3x224x224 \--fp16 \--noTF32 \--memPoolSize=workspace:1024MiB \--builderOptimizationLevel=5 \--maxAuxStreams=4 \--skipInference \--verbose \> 02-generate_engine.log 2>&1# 03 运行engine
trtexec \--loadEngine=resnet18.plan \--shapes=x:4x3x224x224 \--noDataTransfers \--useSpinWait \--useCudaGraph \--verbose \> 03-run_engine.log 2>&1# 04 导出engine信息
trtexec \--onnx=resnet18.onnx \--skipInference \--profilingVerbosity=detailed \--dumpLayerInfo \--exportLayerInfo="./04-exportLayerInfo.log" \> 04-export_layer_info.log 2>&1# 05 导出profiling信息
trtexec \--loadEngine=resnet18.plan \--dumpProfile \--exportTimes="./05-exportTimes.json" \--exportProfile="./05-exportProfile.json" \> 05-export_profile.log 2>&1# 06 保存输入输出数据
trtexec \--loadEngine=resnet18.plan \--dumpOutput \--dumpRawBindingsToFile \> 06-save_data.log 2>&1# 07 读取数据进行推理
trtexec \--loadEngine=resnet18.plan \--loadInputs=x:x.input.1.3.224.224.Float.raw \--dumpOutput \> 07-load_data.log 2>&1
在 cookbook 中,第三个命令无法正常运行就做了下修改;第八个命令需要 plugin 便去除了,后续到 plugin 部分再专门研究。
# 03-Load TensorRT engine built above and do inference
trtexec model-02.plan \--trt \--shapes=tensorX:4x1x28x28 \--noDataTransfers \--useSpinWait \--useCudaGraph \--verbose \> result-03.log 2>&1
相关文章:
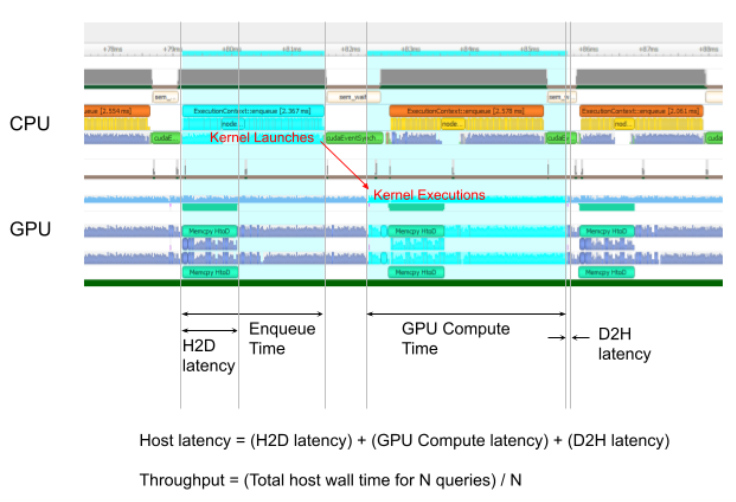
从零开始 TensorRT(4)命令行工具篇:trtexec 基本功能
前言 学习资料: TensorRT 源码示例 B站视频:TensorRT 教程 | 基于 8.6.1 版本 视频配套代码 cookbook 参考源码:cookbook → 07-Tool → trtexec 官方文档:trtexec 在 TensorRT 的安装目录 xxx/TensorRT-8.6.1.6/bin 下有命令行…...
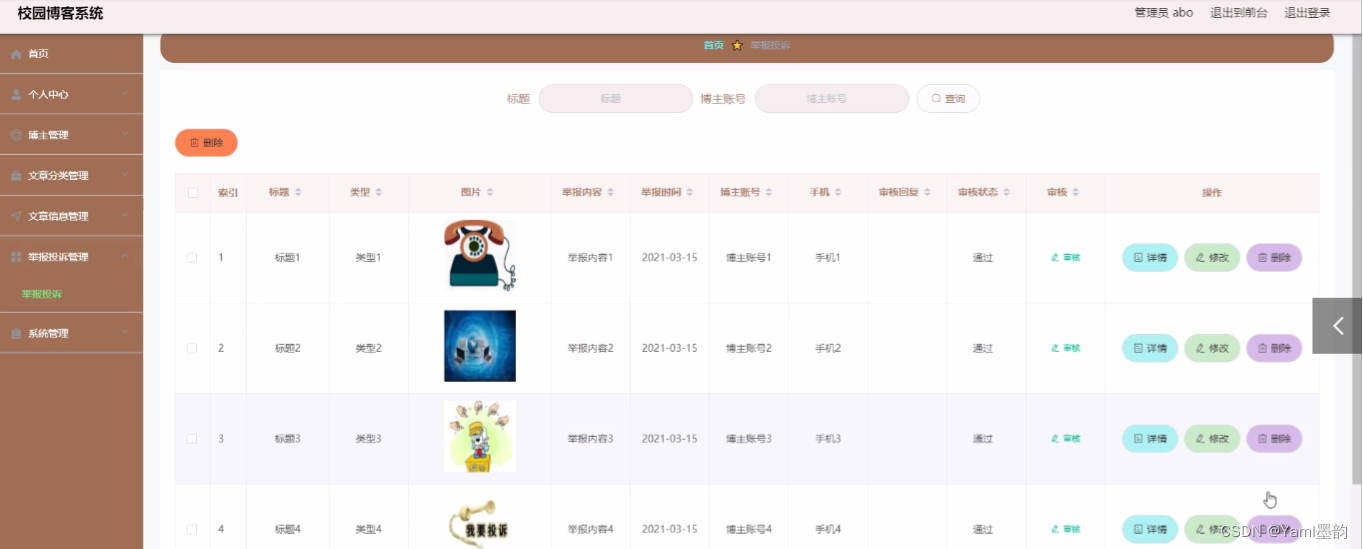
基于SpringBoot+Vue的校园博客管理系统
末尾获取源码作者介绍:大家好,我是墨韵,本人4年开发经验,专注定制项目开发 更多项目:CSDN主页YAML墨韵 学如逆水行舟,不进则退。学习如赶路,不能慢一步。 目录 一、项目简介 二、开发技术与环…...
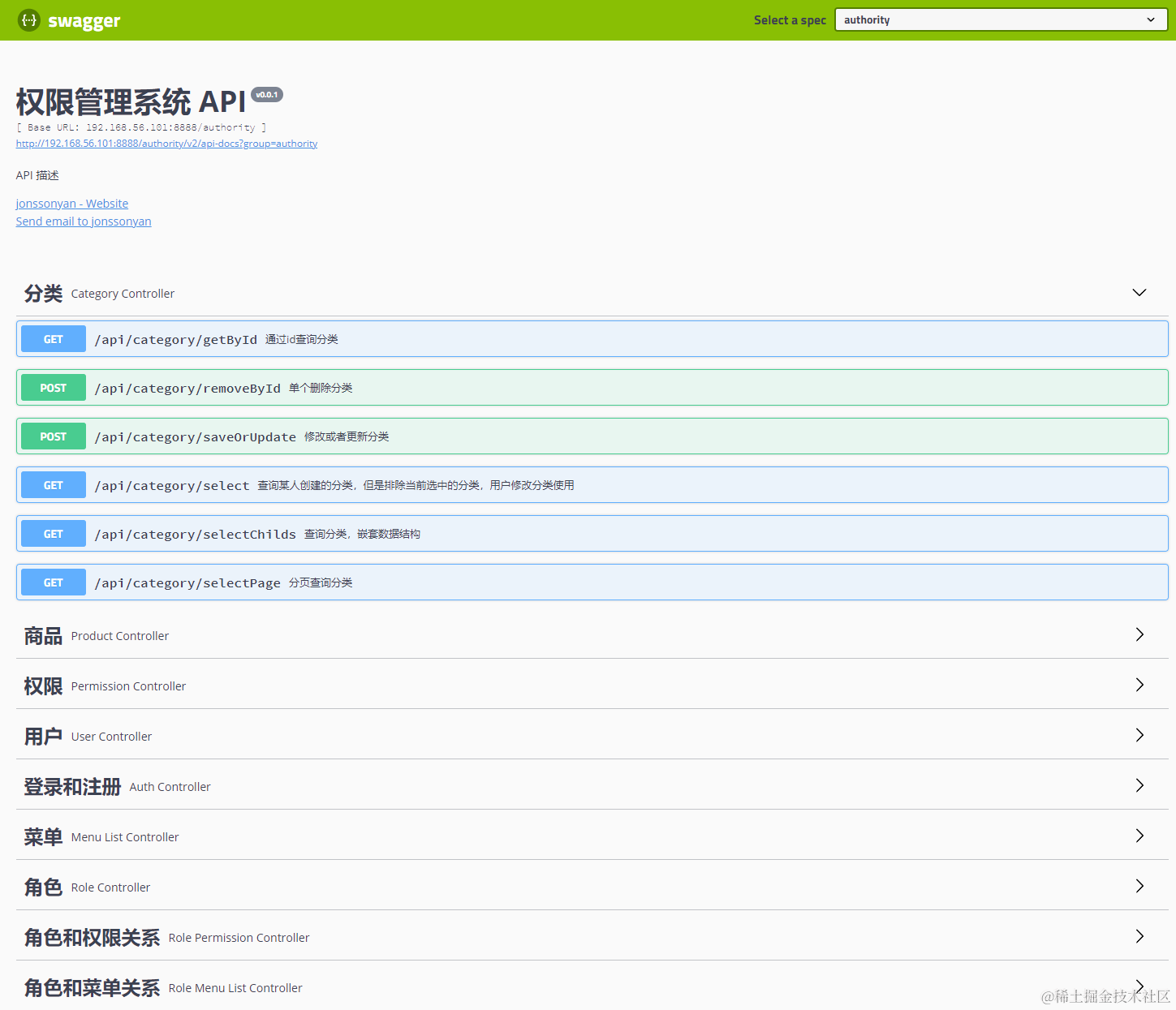
基于 SpringBoot 和 Vue.js 的权限管理系统部署教程
大家后,我是 jonssonyan 在上一篇文章我介绍了我的新项目——基于 SpringBoot 和 Vue.js 的权限管理系统,本文主要介绍该系统的部署 部署教程 这里使用 Docker 进行部署,Docker 基于容器技术,它可以占用更少的资源,…...
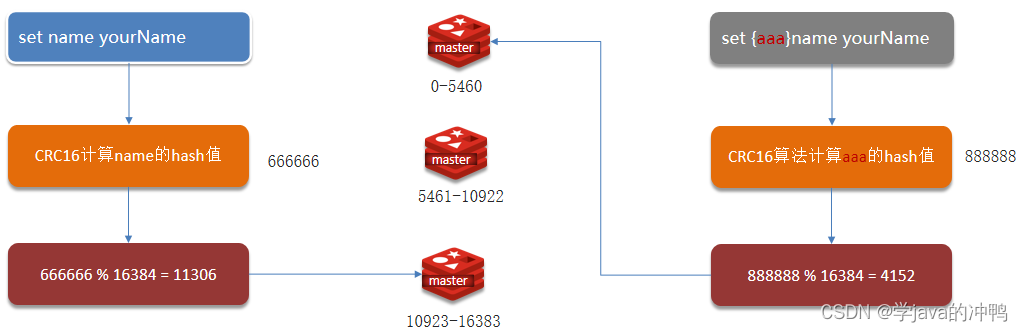
Redis篇之集群
一、主从复制 1.实现主从作用 单节点Redis的并发能力是有上限的,要进一步提高Redis的并发能力,就需要搭建主从集群,实现读写分离。主节点用来写的操作,从节点用来读操作,并且主节点发生写操作后,会把数据同…...

JUnit 5 注解总结与解析
前言 大家好,我是chowley,通过前篇的JUnit实践,我对这个框架产生了好奇,除了断言判断,它还有哪些用处呢?下面来总结一下它的常见注解及作用。 正文 在Java单元测试中,JUnit是一种常用的测试框…...
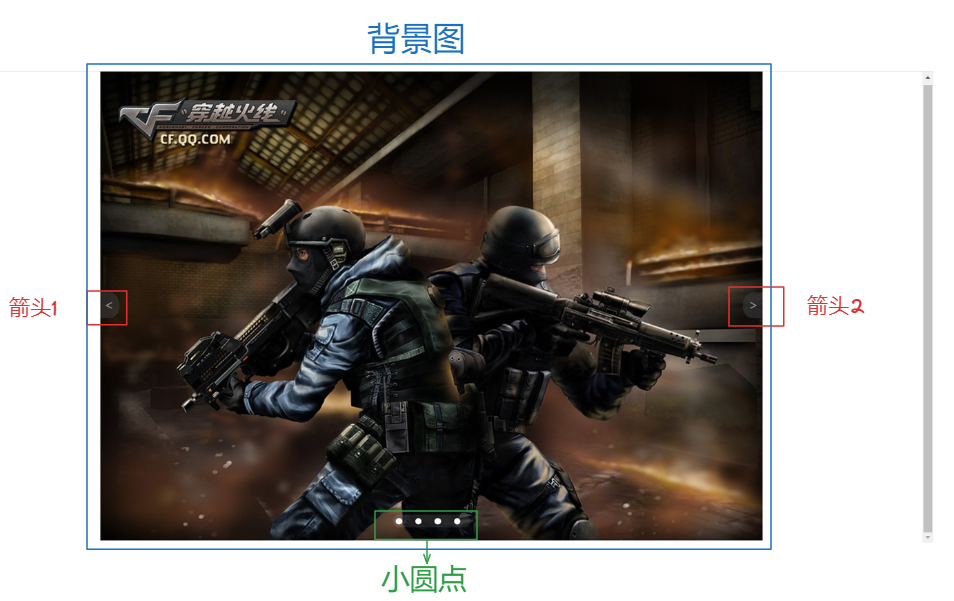
CSS综合案例4
CSS综合案例4 1. 综合案例 我们来做一个静态的轮播图。 2. 分析思路 首先需要加载一张背景图进去需要4个小圆点,设置样式,并用定位和平移调整位置添加两个箭头,也是需要用定位和位移进行调整位置 3. 代码演示 html文件 <!DOCTYPE htm…...
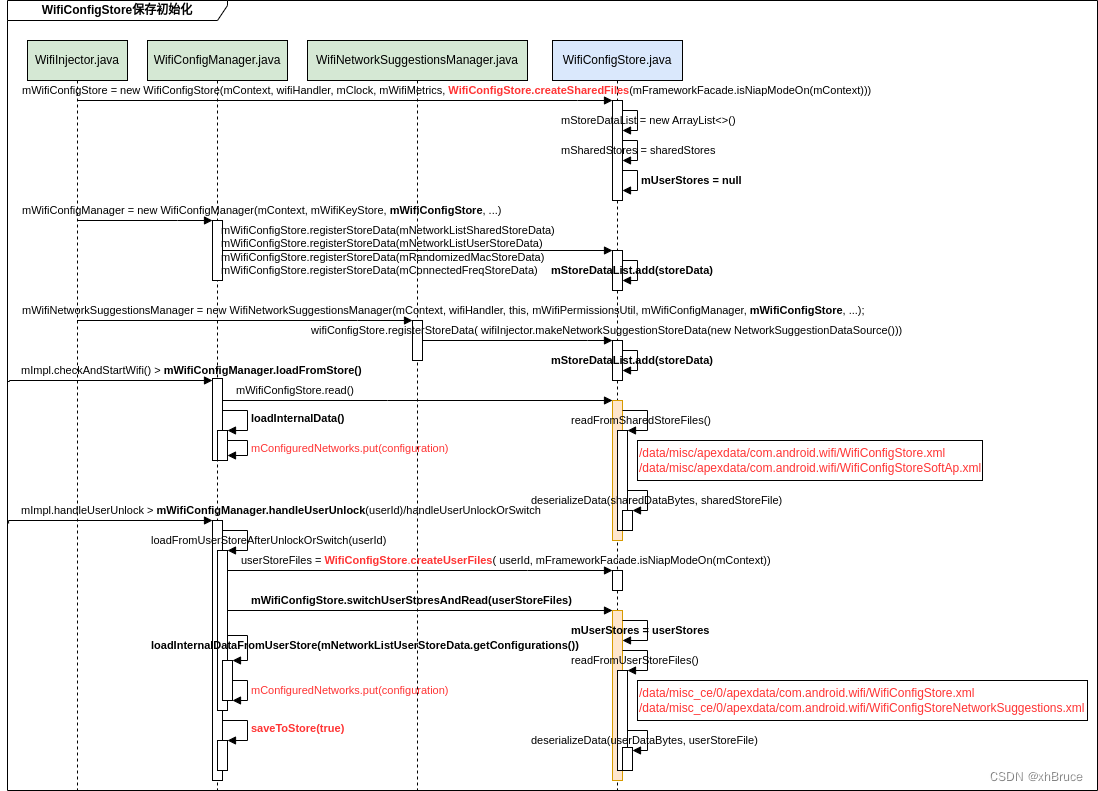
WifiConfigStore初始化读取-Android13
WifiConfigStore初始化读取 1、StoreData创建并注册2、WifiConfigStore读取2.1 文件读取流程2.2 时序图2.3 日志 1、StoreData创建并注册 packages/modules/Wifi/service/java/com/android/server/wifi/WifiConfigManager.java mWifiConfigStore.registerStoreData(mNetworkL…...

【Spring源码解读!底层原理进阶】【下】探寻Spring内部:BeanFactory和ApplicationContext实现原理揭秘✨
🎉🎉欢迎光临🎉🎉 🏅我是苏泽,一位对技术充满热情的探索者和分享者。🚀🚀 🌟特别推荐给大家我的最新专栏《Spring 狂野之旅:底层原理高级进阶》 🚀…...
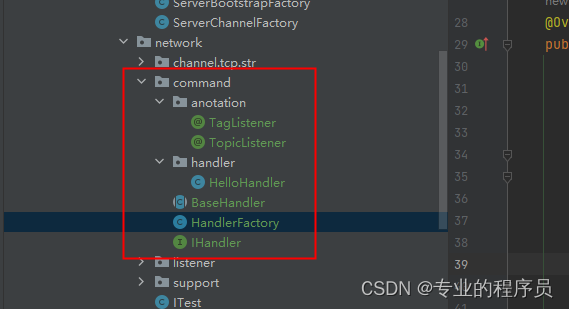
从零开始手写mmo游戏从框架到爆炸(六)— 消息处理工厂
就好像门牌号一样,我们需要把消息路由到对应的楼栋和楼层,总不能像菜鸟一样让大家都来自己找数据吧。 首先这里我们参考了rabbitmq中的topic与tag模型,topic对应类,tag对应方法。 新增一个模块,专门记录路由eternity-…...

Go基础学习笔记-知识点
学习笔记记录了我在学习官方文档过程中记的要点,可以参考学习。 go build *.go 文件 编译 go run *.go 执行 go mod init 生成依赖管理文件 gofmt -w *.go 格式换名称的大小写用来控制方法的可见域主方法及包命名规范 package main //注意package的命名࿰…...
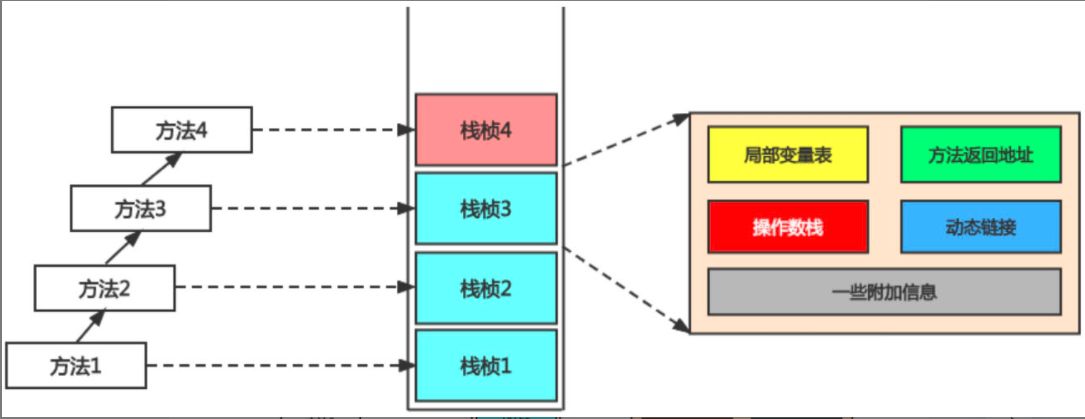
jvm几个常见面试题整理
1. Full GC触发机制有如下5种情况。 (1)调用System.gc()时,系统建议执行Full GC,但是不必然执行。(2)老年代空间不足。(3)方法区空间不足。(4)老年代的最大可用连续空间小于历次晋升到老年代对象的平均大小就会进行Full GC。(5)由Eden区、S0(From)区向S…...

ReentrantLock 和 公平锁
ReentrantLock 和 公平锁 一、基本介绍 ReentrantLock(重入锁) 是一个独占式锁,具有和synchronize的监视器锁基本相同的行为和语意。但和synchronized相比,它更加的灵活、强大、增加了轮询、超时、中断等高级功能以及可以创建公平和非公平锁。Reentran…...
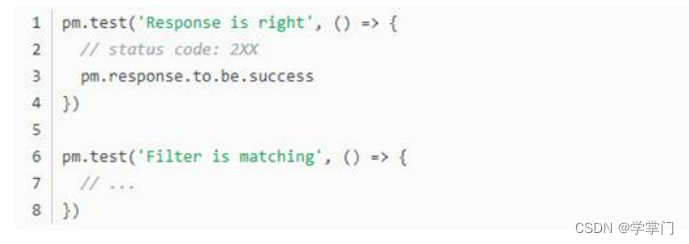
使用Postman做API自动化测试
Postman最基本的功能用来重放请求,并且配合良好的response格式化工具。 高级点的用法可以使用Postman生成各个语言的脚本,还可以抓包,认证,传输文件。 仅仅做到这些还不能够满足一个系统的开发,或者说过于琐碎&#…...
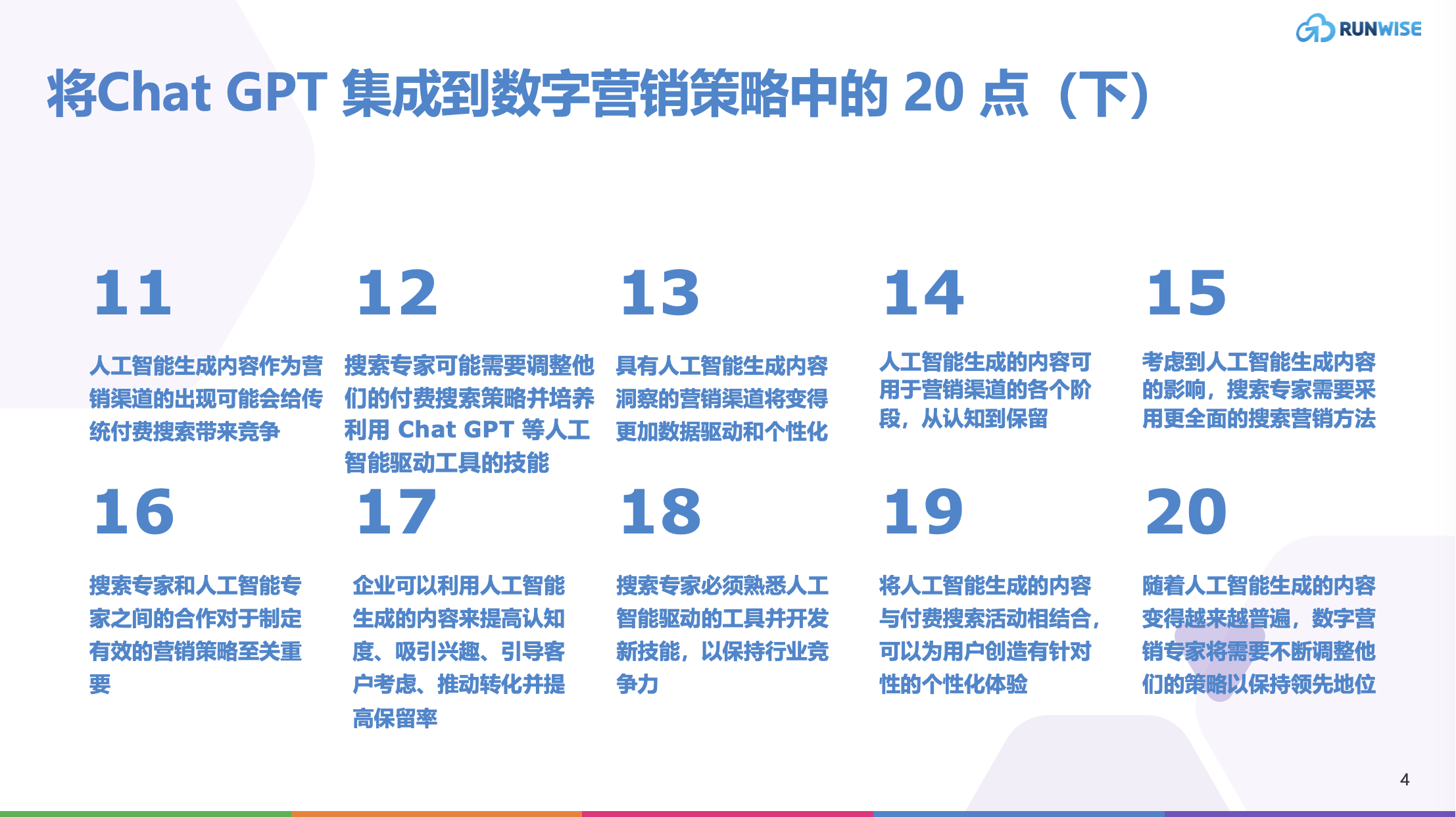
入门指南|Chat GPT 的兴起:它如何改变数字营销格局?
随着数字营销的不断发展,支持数字营销的技术也在不断发展。OpenAI 的 ChatGPT 是一项备受关注的突破性工具。凭借其先进的自然语言处理能力,ChatGPT 已被证明是全球营销人员的宝贵资产。在这份入门指南中,我们将探讨Chat GPT对数字营销专家及…...
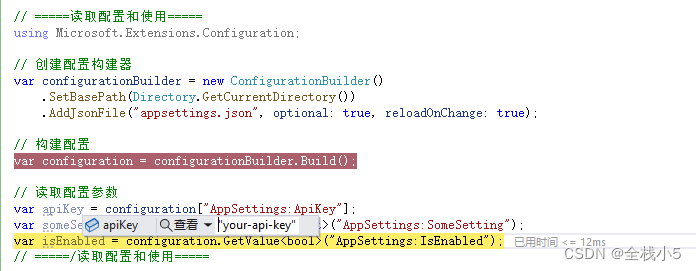
【C#】.net core 6.0 创建默认Web应用,以及默认结构讲解,适合初学者
欢迎来到《小5讲堂》 大家好,我是全栈小5。 这是《C#》系列文章,每篇文章将以博主理解的角度展开讲解, 特别是针对知识点的概念进行叙说,大部分文章将会对这些概念进行实际例子验证,以此达到加深对知识点的理解和掌握。…...

Linux中的numactl命令指南
假设我们想控制线程如何被分配到处理器核心,或者选择我们想分配数据的位置,那么numactl命令就适合此类任务。在这篇文章中,我们讨论了如何使用numactl命令执行此类操作。 目录: 介绍语法命令总结参考文献 简介 现代处理器采用…...
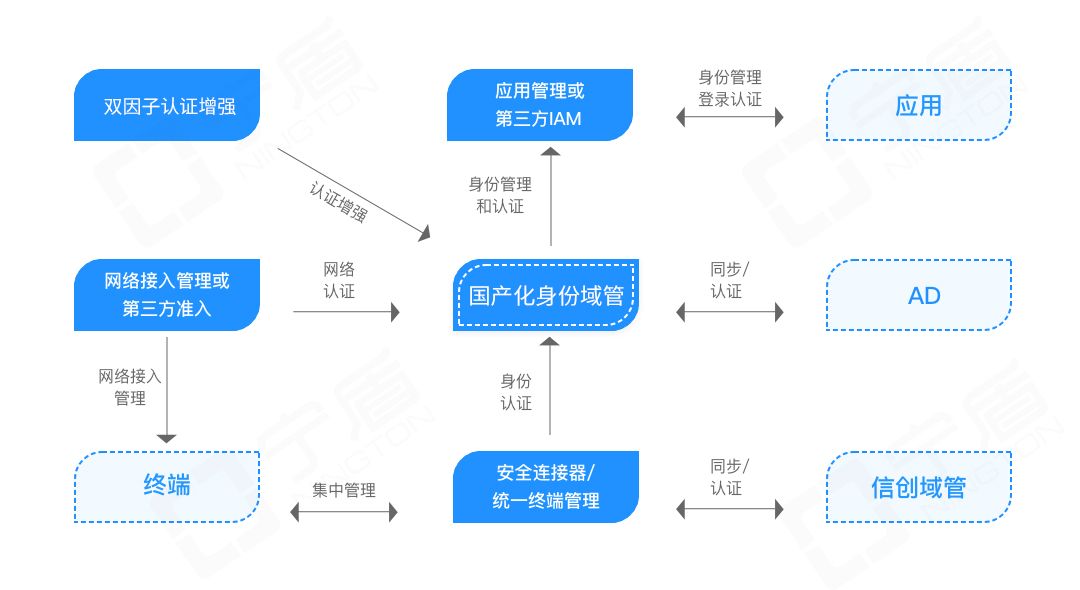
AD域国产替代方案,助力某金融企业麒麟信创电脑实现“真替真用”
近期收到不少企业客户反馈采购的信创PC电脑用不起来,影响信创改造的进度。例如,某金融企业积极响应国产化信创替代战略,购置了一批麒麟操作系统电脑。分发使用中发现了如下问题: • 当前麒麟操作系统电脑无法做到统一身份认证&…...
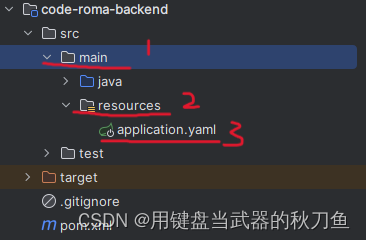
抽象springBoot报错
Failed to configure a DataSource: url attribute is not specified and no embedded datasource could be configured. 中文翻译:无法配置DataSource:未指定“url”属性,并且无法配置嵌入数据源。 DataSource 翻译:数据源 得…...

Linux的打包压缩与解压缩---tar、xz、zip、unzip
最近突然用到了许久不用的压缩解压缩命令,真的陌生, 哈哈,记录一下,后续就不用搜索了。 tar的打包 tar -cvf 压缩有的文件名称 需要压缩的文件或文件夹tar -cvf virtualbox.tar virtualbox/ tar -zcvf virtualbox.tar virtualbo…...
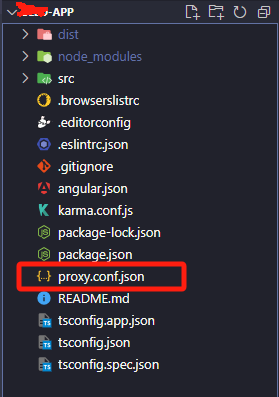
在angular12中proxy.conf.json中配置详解
一、proxy.conf.json文件的目录 二、proxy.conf.json文件中的配置 "/xxx/api": {"target": "地址/api","secure": false,"logLevel": "debug","changeOrigin": true,"pathRewrite": {"…...

PyTorch 中音频信号处理库torchaudio的详细介绍
torchaudio 是 PyTorch 深度学习框架的一部分,是 PyTorch 中处理音频信号的库,专门用于处理和分析音频数据。它提供了丰富的音频信号处理工具、特征提取功能以及与深度学习模型结合的接口,使得在 PyTorch 中进行音频相关的机器学习和深度学习…...

OpenAI研究揭示:ChatGPT对生物武器制造影响有限
### OpenAI研究揭示:ChatGPT对生物武器制造影响有限 在最近的一项引人注目的研究中,OpenAI探索了其旗舰人工智能产品GPT-4在辅助制造生物武器方面的潜力。尽管公众对人工智能可能带来的潜在风险表示担忧,但OpenAI的发现却意味着这种担忧可能…...
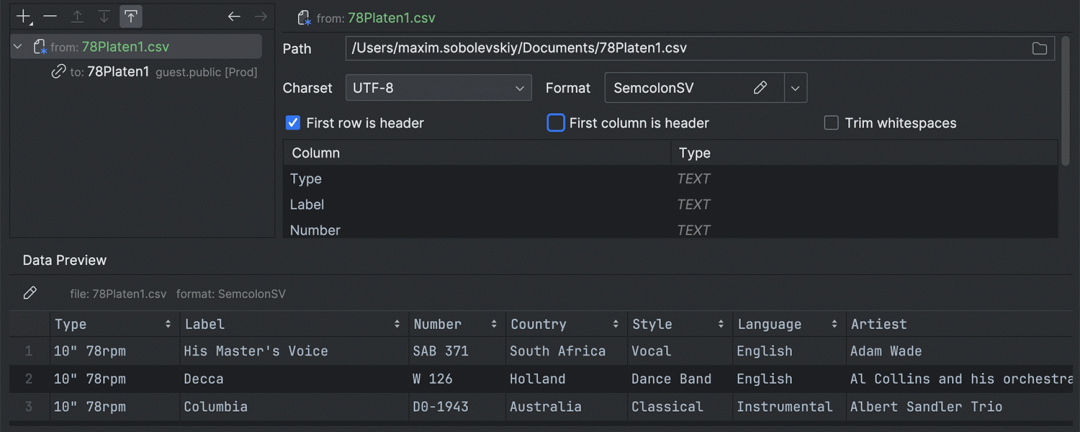
IntelliJ IDEA 2023.3发布,AI 助手出世,新特性杀麻了!!
目录 关键亮点 对 Java 21 功能的完全支持 调试器中的 Run to Cursor(运行到光标)嵌入选项 带有编辑操作的浮动工具栏 用户体验优化 Default(默认)工具窗口布局选项 默认颜色编码编辑器标签页 适用于 macOS 的新产品图标 Speed Sear…...
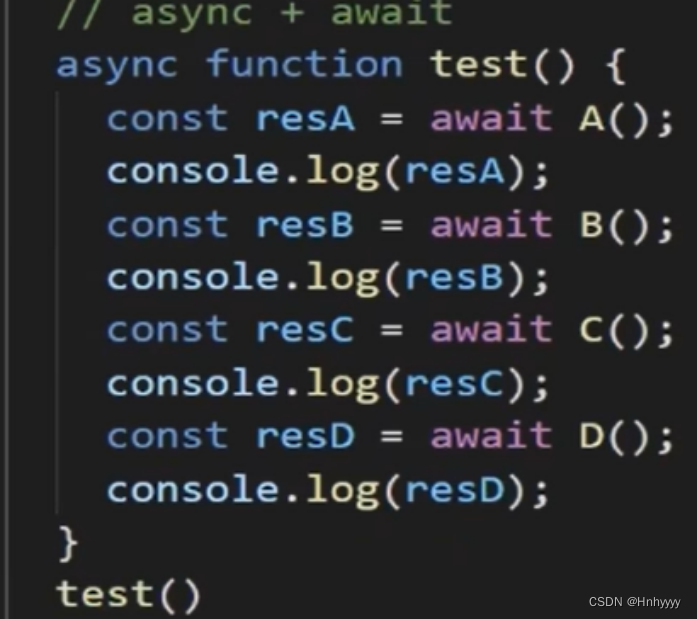
async 与 await(JavaScript)
目录捏 前言一、async二、await三、使用方法总结 前言 async / await 是 ES2017(ES8) 提出的基于 Promise 解决异步的最终方案。上一篇文章介绍了 回调地狱 与 Promise(JavaScript),因为 Promise 的编程模型依然充斥着大量的 then 方法&#…...
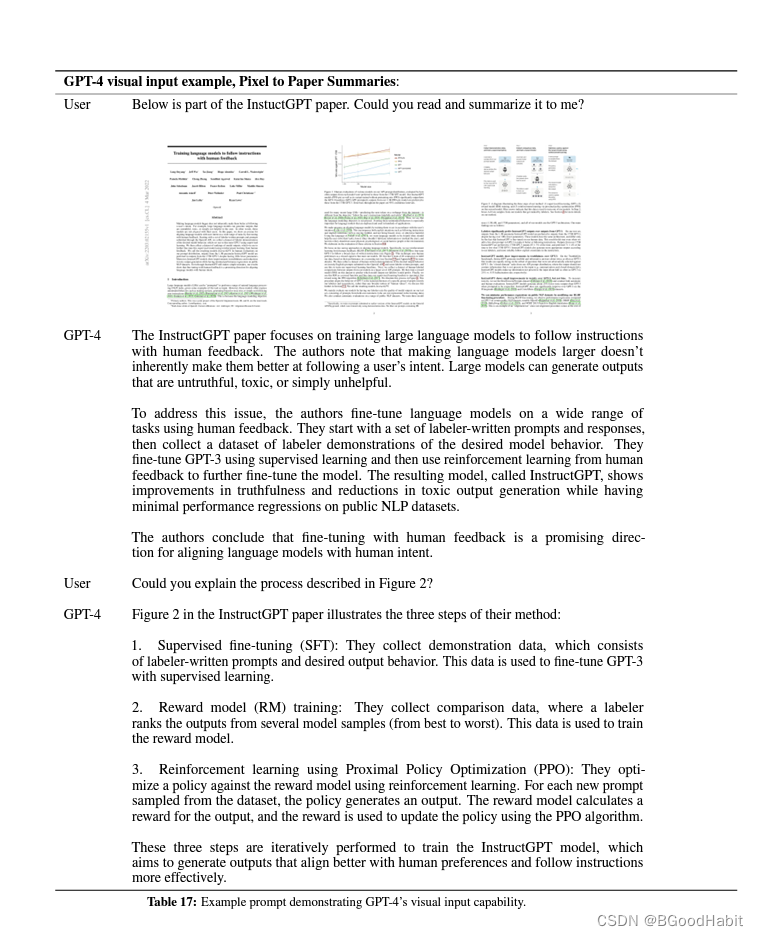
GPT-1, GPT-2, GPT-3, GPT-3.5, GPT-4论文内容解读
目录 1 ChatGPT概述1.1 what is chatGPT1.2 How does ChatGPT work1.3 The applications of ChatGPT1.3 The limitations of ChatGPT 2 算法原理2.1 GPT-12.1.1 Unsupervised pre-training2.1.2 Supervised fine-tuning2.1.3 语料2.1.4 分析 2.2 GPT-22.3 GPT-32.4 InstructGPT…...
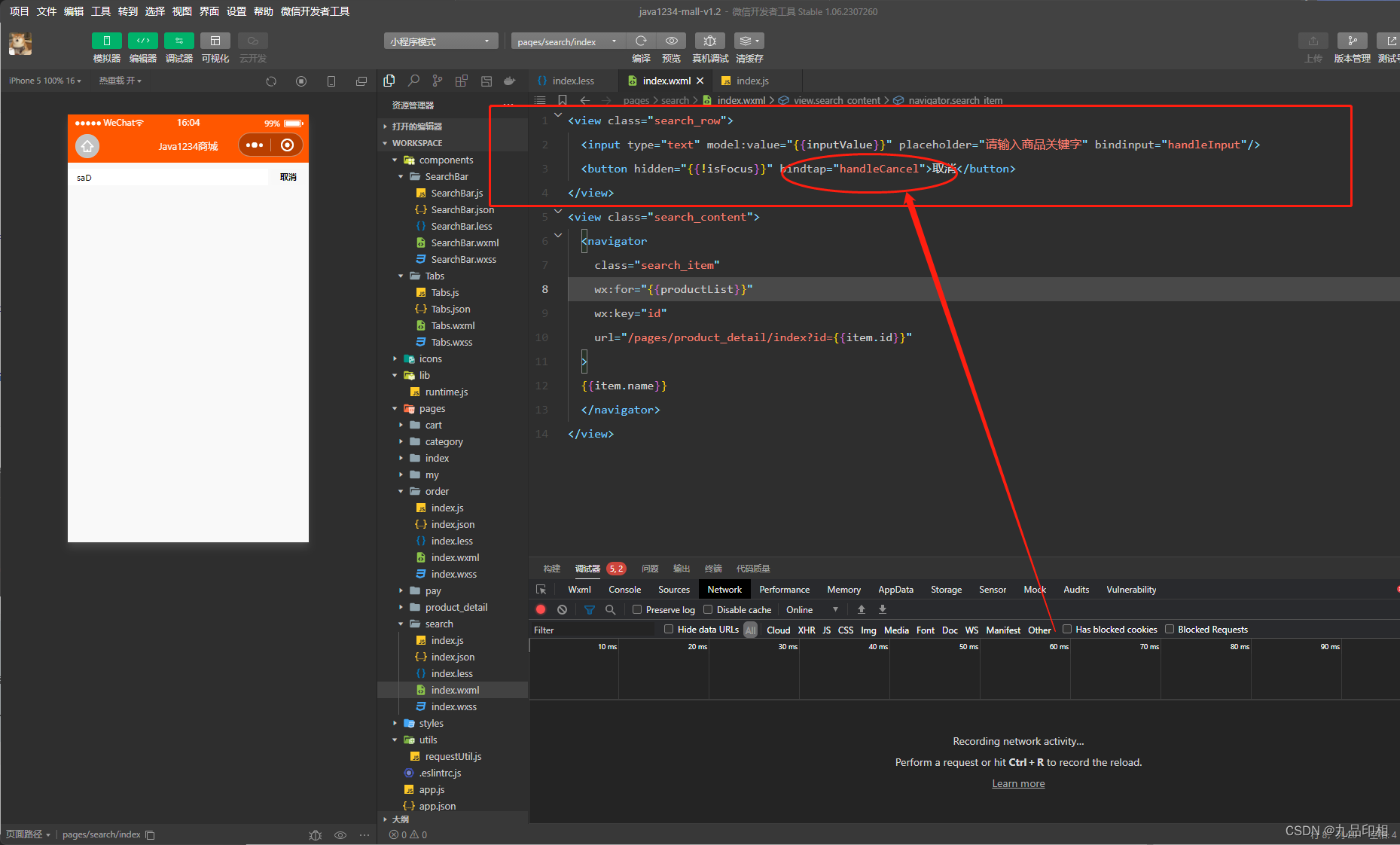
第62讲商品搜索动态实现以及性能优化
商品搜索后端动态获取数据 后端动态获取数据: /*** 商品搜索* param q* return*/GetMapping("/search")public R search(String q){List<Product> productList productService.list(new QueryWrapper<Product>().like("name", q)…...
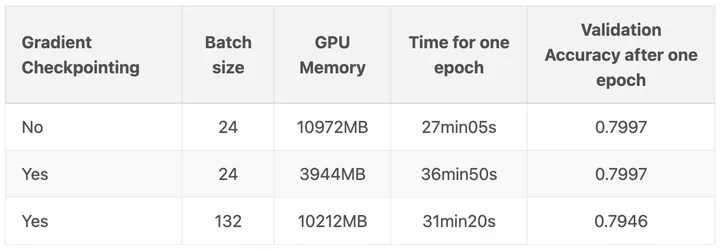
我的PyTorch模型比内存还大,怎么训练呀?
原文:我的PyTorch模型比内存还大,怎么训练呀? - 知乎 看了一篇比较老(21年4月文章)的不大可能训练优化方案,保存起来以后研究一下。 随着深度学习的飞速发展,模型越来越臃肿,哦不&a…...
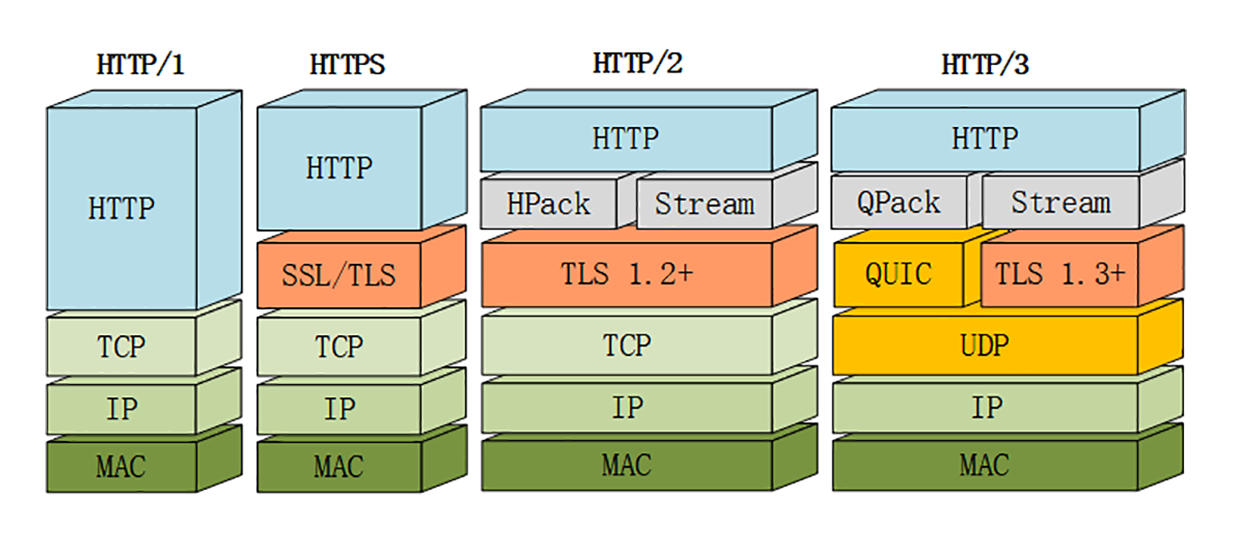
HTTP协议笔记
HTTP协议笔记 参考: (建议精读)HTTP灵魂之问,巩固你的 HTTP 知识体系 《透视 HTTP 协议》——chrono 目录: 1、说说你对HTTP的了解吧。 1. HTTP状态码。 2. HTTP请求头和响应头,其中包括cookie、跨域响…...
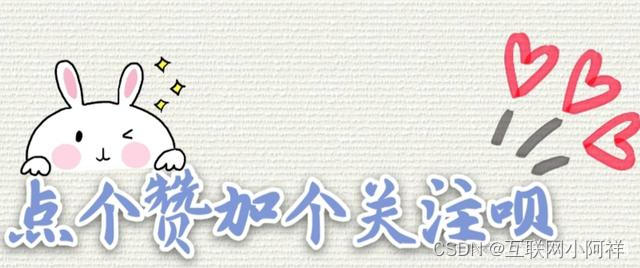
零基础学Python之网络编程
1.什么是socket 官方定义: 套接字(socket)是一个抽象层,应用程序可以通过它发送或接收数据,可对其进行像对文件一样的打开、读写和关闭等操作。套接字允许应用程序将I/O插入到网络中,并与网络中的其他应用…...
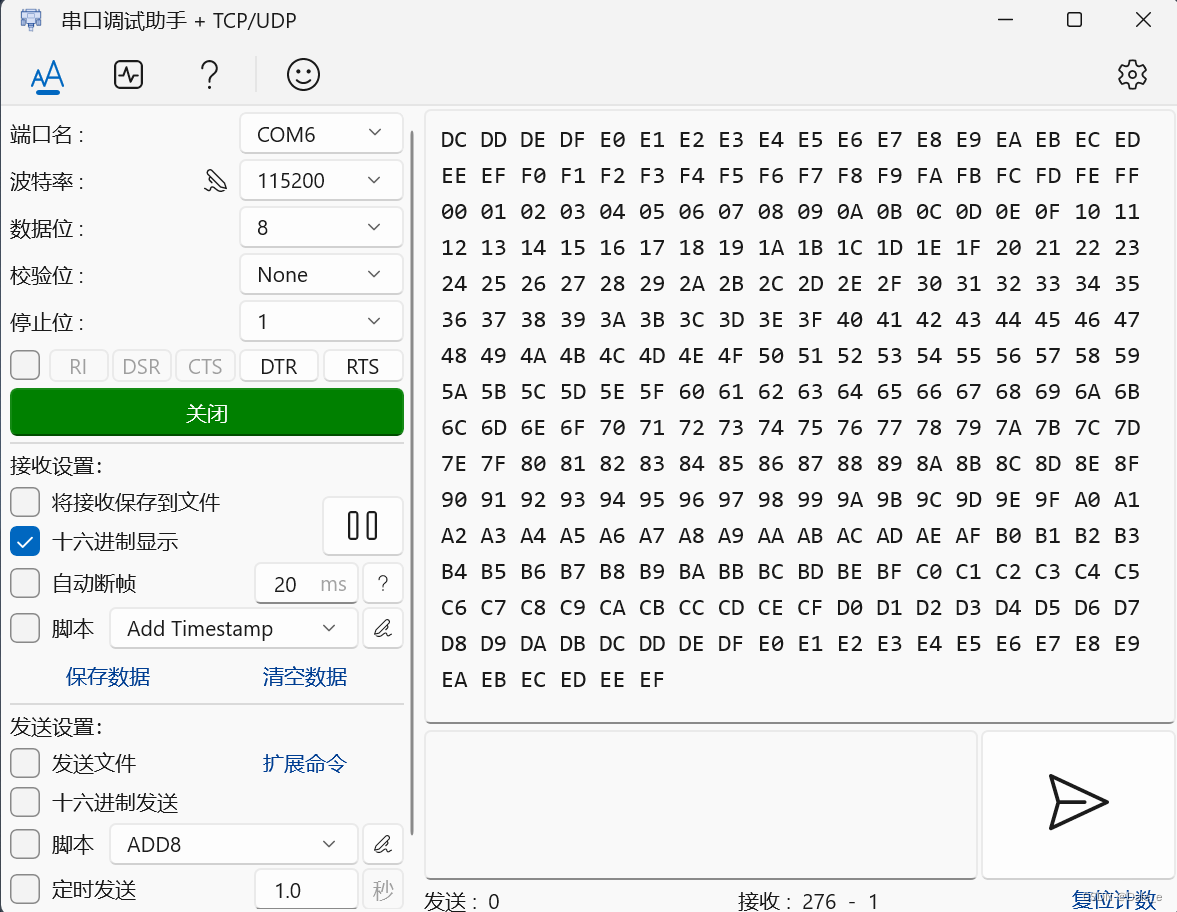
09 AB 10串口通信发送原理
通用异步收发传输器( Universal Asynchronous Receiver/Transmitter, UART)是一种异步收发传输器,其在数据发送时将并行数据转换成串行数据来传输, 在数据接收时将接收到的串行数据转换成并行数据, 可以实现…...