超详细||YOLOv8基础教程(环境搭建,训练,测试,部署看一篇就够)(在推理视频中添加FPS信息)
一、YOLOv8环境搭建
这篇文章将跳过基础的深度学习环境的搭建,如果没有完成的可以看我的这篇博客:超详细||深度学习环境搭建记录cuda+anaconda+pytorch+pycharm-CSDN博客
1. 在github上下载源码:
GitHub - ultralytics/ultralytics: NEW - YOLOv8 🚀 in PyTorch > ONNX > OpenVINO > CoreML > TFLite
2. 安装ultralytics(YOLOv8改名为ultralytics)
这里有两种方式安装ultralytics
- 直接使用CLI
pip install ultralytics
- 使用requirements.txt安装,这种方法是在上面下载的源码处安装,方便对yolov8进行改进
cd ultralytics
pip install -r requirements.txt
3. 安装wandb
pip install wandb
登录自己的wandb账号
wandb login
二、开始训练
1. 构建数据集
数据集要严格按照下面的目录格式,image的格式为jpg,label的格式为txt,对应的image和label的名字要一致
Dataset└─images└─train└─val└─labels└─train└─val
2. 创建一个dataset.yaml文件
更换自己的image train和image val的地址,labels地址不用,它会自动索引
将classes改为自己的类别,从0开始
path: ../datasets/coco128 # dataset root dir
train: images/train2017 # train images (relative to 'path') 128 images
val: images/train2017 # val images (relative to 'path') 128 images
test: # test images (optional)# Classes
names:0: person1: bicycle2: car3: motorcycle4: airplane5: bus6: train7: truck8: boat
3. 新建一个train.py,修改相关参数,运行即可开始训练
from ultralytics import YOLOif __name__ == '__main__':# Load a modelmodel = YOLO(r'\ultralytics\detection\yolov8n\yolov8n.yaml') # 不使用预训练权重训练# model = YOLO(r'yolov8p.yaml').load("yolov8n.pt") # 使用预训练权重训练# Trainparameters ----------------------------------------------------------------------------------------------model.train(data=r'\ultralytics\detection\dataset\appledata.yaml',epochs= 30 , # (int) number of epochs to train forpatience= 50 , # (int) epochs to wait for no observable improvement for early stopping of trainingbatch= 8 , # (int) number of images per batch (-1 for AutoBatch)imgsz= 320 , # (int) size of input images as integer or w,hsave= True , # (bool) save train checkpoints and predict resultssave_period= -1, # (int) Save checkpoint every x epochs (disabled if < 1)cache= False , # (bool) True/ram, disk or False. Use cache for data loadingdevice= 0 , # (int | str | list, optional) device to run on, i.e. cuda device=0 or device=0,1,2,3 or device=cpuworkers= 16 , # (int) number of worker threads for data loading (per RANK if DDP)project= 'result', # (str, optional) project namename= 'yolov8n' ,# (str, optional) experiment name, results saved to 'project/name' directoryexist_ok= False , # (bool) whether to overwrite existing experimentpretrained= False , # (bool | str) whether to use a pretrained model (bool) or a model to load weights from (str)optimizer= 'SGD', # (str) optimizer to use, choices=[SGD, Adam, Adamax, AdamW, NAdam, RAdam, RMSProp, auto]verbose= True ,# (bool) whether to print verbose outputseed= 0 , # (int) random seed for reproducibilitydeterministic= True , # (bool) whether to enable deterministic modesingle_cls= True , # (bool) train multi-class data as single-classrect= False ,# (bool) rectangular training if mode='train' or rectangular validation if mode='val'cos_lr= False , # (bool) use cosine learning rate schedulerclose_mosaic= 0, # (int) disable mosaic augmentation for final epochsresume= False , # (bool) resume training from last checkpointamp= False, # (bool) Automatic Mixed Precision (AMP) training, choices=[True, False], True runs AMP checkfraction= 1.0 , # (float) dataset fraction to train on (default is 1.0, all images in train set)profile= False, # (bool) profile ONNX and TensorRT speeds during training for loggers# Segmentationoverlap_mask= True , # (bool) masks should overlap during training (segment train only)mask_ratio= 4, # (int) mask downsample ratio (segment train only)# Classificationdropout= 0.0, # (float) use dropout regularization (classify train only)# Hyperparameters ----------------------------------------------------------------------------------------------lr0=0.01, # (float) initial learning rate (i.e. SGD=1E-2, Adam=1E-3)lrf=0.01, # (float) final learning rate (lr0 * lrf)momentum=0.937, # (float) SGD momentum/Adam beta1weight_decay=0.0005, # (float) optimizer weight decay 5e-4warmup_epochs=3.0, # (float) warmup epochs (fractions ok)warmup_momentum=0.8, # (float) warmup initial momentumwarmup_bias_lr=0.1, # (float) warmup initial bias lrbox=7.5, # (float) box loss gaincls=0.5, # (float) cls loss gain (scale with pixels)dfl=1.5, # (float) dfl loss gainpose=12.0, # (float) pose loss gainkobj=1.0, # (float) keypoint obj loss gainlabel_smoothing=0.0, # (float) label smoothing (fraction)nbs=64, # (int) nominal batch sizehsv_h=0.015, # (float) image HSV-Hue augmentation (fraction)hsv_s=0.7, # (float) image HSV-Saturation augmentation (fraction)hsv_v=0.4, # (float) image HSV-Value augmentation (fraction)degrees=0.0, # (float) image rotation (+/- deg)translate=0.1, # (float) image translation (+/- fraction)scale=0.5, # (float) image scale (+/- gain)shear=0.0, # (float) image shear (+/- deg)perspective=0.0, # (float) image perspective (+/- fraction), range 0-0.001flipud=0.0, # (float) image flip up-down (probability)fliplr=0.5, # (float) image flip left-right (probability)mosaic=1.0, # (float) image mosaic (probability)mixup=0.0, # (float) image mixup (probability)copy_paste=0.0, # (float) segment copy-paste (probability))
三、测试与验证
1. 新建一个test.py, 这个可以打印网路信息,参数量以及FLOPs,还有每一层网络的信息
from ultralytics import YOLOif __name__ == '__main__':# Load a modelmodel = YOLO(r'\ultralytics\detection\yolov8n\yolov8n.yaml') # build a new model from YAMLmodel.info()
2. 新建一个val.py,这个可以打印模型在验证集上的结果,如mAP,推理速度等
from ultralytics import YOLOif __name__ == '__main__':# Load a modelmodel = YOLO(r'\ultralytics\detection\yolov8n\result\yolov8n4\weights\best.pt') # build a new model from YAML# Validate the modelmodel.val(val=True, # (bool) validate/test during trainingdata=r'\ultralytics\detection\dataset\appledata.yaml',split='val', # (str) dataset split to use for validation, i.e. 'val', 'test' or 'train'batch=1, # 测试速度时一般设置为 1 ,设置越大速度越快。 (int) number of images per batch (-1 for AutoBatch)imgsz=320, # (int) size of input images as integer or w,hdevice=0, # (int | str | list, optional) device to run on, i.e. cuda device=0 or device=0,1,2,3 or device=cpuworkers=8, # (int) number of worker threads for data loading (per RANK if DDP)save_json=False, # (bool) save results to JSON filesave_hybrid=False, # (bool) save hybrid version of labels (labels + additional predictions)conf=0.001, # (float, optional) object confidence threshold for detection (default 0.25 predict, 0.001 val)iou=0.7, # (float) intersection over union (IoU) threshold for NMSproject='val', # (str, optional) project namename='', # (str, optional) experiment name, results saved to 'project/name' directorymax_det=300, # (int) maximum number of detections per imagehalf=True, # (bool) use half precision (FP16)dnn=False, # (bool) use OpenCV DNN for ONNX inferenceplots=True, # (bool) save plots during train/val)
3. 新建一个predict.py,这个可以根据训练好的权重文件进行推理,权重文件格式支持pt,onnx等,支持图片,视频,摄像头等进行推理
from ultralytics import YOLOif __name__ == '__main__':# Load a modelmodel = YOLO(r'\deploy\yolov8n.pt') # pretrained YOLOv8n modelmodel.predict(source=r'\deploy\output_video.mp4',save=False, # save predict resultsimgsz=320, # (int) size of input images as integer or w,hconf=0.25, # object confidence threshold for detection (default 0.25 predict, 0.001 val)iou=0.7, # # intersection over union (IoU) threshold for NMSshow=True, # show results if possibleproject='', # (str, optional) project namename='', # (str, optional) experiment name, results saved to 'project/name' directorysave_txt=False, # save results as .txt filesave_conf=True, # save results with confidence scoressave_crop=False, # save cropped images with resultsshow_labels=True, # show object labels in plotsshow_conf=True, # show object confidence scores in plotsvid_stride=1, # video frame-rate strideline_width=1, # bounding box thickness (pixels)visualize=False, # visualize model featuresaugment=False, # apply image augmentation to prediction sourcesagnostic_nms=False, # class-agnostic NMSretina_masks=False, # use high-resolution segmentation masksboxes=True, # Show boxes in segmentation predictions)
四、onnx模型部署
1. 新建一个export.py,将pt文件转化为onnx文件
from ultralytics import YOLO# Load a model
model = YOLO('/ultralytics/weight file/yolov8n.pt') # load a custom trained model# Export the model
model.export(format='onnx')
2. 将onnx文件添加到之前提到的predict.py中,进行推理。
3. 如果想在推理的视频中添加FPS信息,请把ultralytics/engine/predictor.py替换为下面的代码。
# Ultralytics YOLO 🚀, AGPL-3.0 license
"""
Run prediction on images, videos, directories, globs, YouTube, webcam, streams, etc.Usage - sources:$ yolo mode=predict model=yolov8n.pt source=0 # webcamimg.jpg # imagevid.mp4 # videoscreen # screenshotpath/ # directorylist.txt # list of imageslist.streams # list of streams'path/*.jpg' # glob'https://youtu.be/Zgi9g1ksQHc' # YouTube'rtsp://example.com/media.mp4' # RTSP, RTMP, HTTP streamUsage - formats:$ yolo mode=predict model=yolov8n.pt # PyTorchyolov8n.torchscript # TorchScriptyolov8n.onnx # ONNX Runtime or OpenCV DNN with dnn=Trueyolov8n_openvino_model # OpenVINOyolov8n.engine # TensorRTyolov8n.mlmodel # CoreML (macOS-only)yolov8n_saved_model # TensorFlow SavedModelyolov8n.pb # TensorFlow GraphDefyolov8n.tflite # TensorFlow Liteyolov8n_edgetpu.tflite # TensorFlow Edge TPUyolov8n_paddle_model # PaddlePaddle
"""
import platform
from pathlib import Pathimport cv2
import numpy as np
import torchfrom ultralytics.cfg import get_cfg
from ultralytics.data import load_inference_source
from ultralytics.data.augment import LetterBox, classify_transforms
from ultralytics.nn.autobackend import AutoBackend
from ultralytics.utils import DEFAULT_CFG, LOGGER, MACOS, SETTINGS, WINDOWS, callbacks, colorstr, ops
from ultralytics.utils.checks import check_imgsz, check_imshow
from ultralytics.utils.files import increment_path
from ultralytics.utils.torch_utils import select_device, smart_inference_modeSTREAM_WARNING = """WARNING ⚠️ stream/video/webcam/dir predict source will accumulate results in RAM unless `stream=True` is passed,causing potential out-of-memory errors for large sources or long-running streams/videos.Usage:results = model(source=..., stream=True) # generator of Results objectsfor r in results:boxes = r.boxes # Boxes object for bbox outputsmasks = r.masks # Masks object for segment masks outputsprobs = r.probs # Class probabilities for classification outputs
"""class BasePredictor:"""BasePredictorA base class for creating predictors.Attributes:args (SimpleNamespace): Configuration for the predictor.save_dir (Path): Directory to save results.done_warmup (bool): Whether the predictor has finished setup.model (nn.Module): Model used for prediction.data (dict): Data configuration.device (torch.device): Device used for prediction.dataset (Dataset): Dataset used for prediction.vid_path (str): Path to video file.vid_writer (cv2.VideoWriter): Video writer for saving video output.data_path (str): Path to data."""def __init__(self, cfg=DEFAULT_CFG, overrides=None, _callbacks=None):"""Initializes the BasePredictor class.Args:cfg (str, optional): Path to a configuration file. Defaults to DEFAULT_CFG.overrides (dict, optional): Configuration overrides. Defaults to None."""self.args = get_cfg(cfg, overrides)self.save_dir = self.get_save_dir()if self.args.conf is None:self.args.conf = 0.25 # default conf=0.25self.done_warmup = Falseif self.args.show:self.args.show = check_imshow(warn=True)# Usable if setup is doneself.model = Noneself.data = self.args.data # data_dictself.imgsz = Noneself.device = Noneself.dataset = Noneself.vid_path, self.vid_writer = None, Noneself.plotted_img = Noneself.data_path = Noneself.source_type = Noneself.batch = Noneself.results = Noneself.transforms = Noneself.callbacks = _callbacks or callbacks.get_default_callbacks()self.txt_path = Nonecallbacks.add_integration_callbacks(self)def get_save_dir(self):project = self.args.project or Path(SETTINGS['runs_dir']) / self.args.taskname = self.args.name or f'{self.args.mode}'return increment_path(Path(project) / name, exist_ok=self.args.exist_ok)def preprocess(self, im):"""Prepares input image before inference.Args:im (torch.Tensor | List(np.ndarray)): BCHW for tensor, [(HWC) x B] for list."""not_tensor = not isinstance(im, torch.Tensor)if not_tensor:im = np.stack(self.pre_transform(im))im = im[..., ::-1].transpose((0, 3, 1, 2)) # BGR to RGB, BHWC to BCHW, (n, 3, h, w)im = np.ascontiguousarray(im) # contiguousim = torch.from_numpy(im)img = im.to(self.device)img = img.half() if self.model.fp16 else img.float() # uint8 to fp16/32if not_tensor:img /= 255 # 0 - 255 to 0.0 - 1.0return imgdef inference(self, im, *args, **kwargs):visualize = increment_path(self.save_dir / Path(self.batch[0][0]).stem,mkdir=True) if self.args.visualize and (not self.source_type.tensor) else Falsereturn self.model(im, augment=self.args.augment, visualize=visualize)def pre_transform(self, im):"""Pre-transform input image before inference.Args:im (List(np.ndarray)): (N, 3, h, w) for tensor, [(h, w, 3) x N] for list.Return: A list of transformed imgs."""same_shapes = all(x.shape == im[0].shape for x in im)auto = same_shapes and self.model.ptreturn [LetterBox(self.imgsz, auto=auto, stride=self.model.stride)(image=x) for x in im]def write_results(self, idx, results, batch):"""Write inference results to a file or directory."""p, im, _ = batchlog_string = ''if len(im.shape) == 3:im = im[None] # expand for batch dimif self.source_type.webcam or self.source_type.from_img or self.source_type.tensor: # batch_size >= 1log_string += f'{idx}: 'frame = self.dataset.countelse:frame = getattr(self.dataset, 'frame', 0)self.data_path = pself.txt_path = str(self.save_dir / 'labels' / p.stem) + ('' if self.dataset.mode == 'image' else f'_{frame}')log_string += '%gx%g ' % im.shape[2:] # print stringresult = results[idx]log_string += result.verbose()if self.args.save or self.args.show: # Add bbox to imageplot_args = {'line_width': self.args.line_width,'boxes': self.args.boxes,'conf': self.args.show_conf,'labels': self.args.show_labels}if not self.args.retina_masks:plot_args['im_gpu'] = im[idx]self.plotted_img = result.plot(**plot_args)# Writeif self.args.save_txt:result.save_txt(f'{self.txt_path}.txt', save_conf=self.args.save_conf)if self.args.save_crop:result.save_crop(save_dir=self.save_dir / 'crops',file_name=self.data_path.stem + ('' if self.dataset.mode == 'image' else f'_{frame}'))return log_stringdef postprocess(self, preds, img, orig_imgs):"""Post-processes predictions for an image and returns them."""return predsdef __call__(self, source=None, model=None, stream=False, *args, **kwargs):"""Performs inference on an image or stream."""self.stream = streamif stream:return self.stream_inference(source, model, *args, **kwargs)else:return list(self.stream_inference(source, model, *args, **kwargs)) # merge list of Result into onedef predict_cli(self, source=None, model=None):"""Method used for CLI prediction. It uses always generator as outputs as not required by CLI mode."""gen = self.stream_inference(source, model)for _ in gen: # running CLI inference without accumulating any outputs (do not modify)passdef setup_source(self, source):"""Sets up source and inference mode."""self.imgsz = check_imgsz(self.args.imgsz, stride=self.model.stride, min_dim=2) # check image sizeself.transforms = getattr(self.model.model, 'transforms', classify_transforms(self.imgsz[0])) if self.args.task == 'classify' else Noneself.dataset = load_inference_source(source=source, imgsz=self.imgsz, vid_stride=self.args.vid_stride)self.source_type = self.dataset.source_typeif not getattr(self, 'stream', True) and (self.dataset.mode == 'stream' or # streamslen(self.dataset) > 1000 or # imagesany(getattr(self.dataset, 'video_flag', [False]))): # videosLOGGER.warning(STREAM_WARNING)self.vid_path, self.vid_writer = [None] * self.dataset.bs, [None] * self.dataset.bs@smart_inference_mode()def stream_inference(self, source=None, model=None, *args, **kwargs):"""Streams real-time inference on camera feed and saves results to file."""if self.args.verbose:LOGGER.info('')# Setup modelif not self.model:self.setup_model(model)# Setup source every time predict is calledself.setup_source(source if source is not None else self.args.source)# Check if save_dir/ label file existsif self.args.save or self.args.save_txt:(self.save_dir / 'labels' if self.args.save_txt else self.save_dir).mkdir(parents=True, exist_ok=True)# Warmup modelif not self.done_warmup:self.model.warmup(imgsz=(1 if self.model.pt or self.model.triton else self.dataset.bs, 3, *self.imgsz))self.done_warmup = Trueself.seen, self.windows, self.batch, self.profilers = 0, [], None, (ops.Profile(), ops.Profile(), ops.Profile())self.run_callbacks('on_predict_start')for batch in self.dataset:self.run_callbacks('on_predict_batch_start')self.batch = batchpath, im0s, vid_cap, s = batch# Preprocesswith self.profilers[0]:im = self.preprocess(im0s)# Inferencewith self.profilers[1]:preds = self.inference(im, *args, **kwargs)# Postprocesswith self.profilers[2]:self.results = self.postprocess(preds, im, im0s)self.run_callbacks('on_predict_postprocess_end')# Visualize, save, write resultsn = len(im0s)for i in range(n):self.seen += 1self.results[i].speed = {'preprocess': self.profilers[0].dt * 1E3 / n,'inference': self.profilers[1].dt * 1E3 / n,'postprocess': self.profilers[2].dt * 1E3 / n}p, im0 = path[i], None if self.source_type.tensor else im0s[i].copy()p = Path(p)if self.args.verbose or self.args.save or self.args.save_txt or self.args.show:s += self.write_results(i, self.results, (p, im, im0))if self.args.save or self.args.save_txt:self.results[i].save_dir = self.save_dir.__str__()if self.args.show and self.plotted_img is not None:self.show(p)if self.args.save and self.plotted_img is not None:self.save_preds(vid_cap, i, str(self.save_dir / p.name))self.run_callbacks('on_predict_batch_end')yield from self.results# Print time (inference-not only)if self.args.verbose:LOGGER.info(f'{s}{(self.profilers[0].dt+self.profilers[1].dt+self.profilers[2].dt) * 1E3:.2f}ms')# Release assetsif isinstance(self.vid_writer[-1], cv2.VideoWriter):self.vid_writer[-1].release() # release final video writer# Print resultsif self.args.verbose and self.seen:t = tuple(x.t / self.seen * 1E3 for x in self.profilers) # speeds per imageLOGGER.info(f'Speed: %.2fms preprocess, %.2fms inference, %.2fms postprocess per image at shape 'f'{(1, 3, *im.shape[2:])}' % t)if self.args.save or self.args.save_txt or self.args.save_crop:nl = len(list(self.save_dir.glob('labels/*.txt'))) # number of labelss = f"\n{nl} label{'s' * (nl > 1)} saved to {self.save_dir / 'labels'}" if self.args.save_txt else ''LOGGER.info(f"Results saved to {colorstr('bold', self.save_dir)}{s}")self.run_callbacks('on_predict_end')def setup_model(self, model, verbose=True):"""Initialize YOLO model with given parameters and set it to evaluation mode."""self.model = AutoBackend(model or self.args.model,device=select_device(self.args.device, verbose=verbose),dnn=self.args.dnn,data=self.args.data,fp16=self.args.half,fuse=True,verbose=verbose)self.device = self.model.device # update deviceself.args.half = self.model.fp16 # update halfself.model.eval()def show(self, p):"""Display an image in a window using OpenCV imshow()."""im0 = self.plotted_img#--------------------后添加-----------------------------str_FPS = "FPS: %.2f" % (1. / (self.profilers[0].dt + self.profilers[1].dt + self.profilers[2].dt))cv2.putText(im0, str_FPS, (50, 50), cv2.FONT_HERSHEY_COMPLEX, 1, (0, 255, 0), 3)# --------------------后添加-----------------------------if platform.system() == 'Linux' and p not in self.windows:self.windows.append(p)cv2.namedWindow(str(p), cv2.WINDOW_NORMAL | cv2.WINDOW_KEEPRATIO) # allow window resize (Linux)cv2.resizeWindow(str(p), im0.shape[1], im0.shape[0])cv2.imshow(str(p), im0)cv2.waitKey(500 if self.batch[3].startswith('image') else 1) # 1 milliseconddef save_preds(self, vid_cap, idx, save_path):"""Save video predictions as mp4 at specified path."""im0 = self.plotted_img# Save imgsif self.dataset.mode == 'image':cv2.imwrite(save_path, im0)else: # 'video' or 'stream'if self.vid_path[idx] != save_path: # new videoself.vid_path[idx] = save_pathif isinstance(self.vid_writer[idx], cv2.VideoWriter):self.vid_writer[idx].release() # release previous video writerif vid_cap: # videofps = int(vid_cap.get(cv2.CAP_PROP_FPS)) # integer required, floats produce error in MP4 codecw = int(vid_cap.get(cv2.CAP_PROP_FRAME_WIDTH))h = int(vid_cap.get(cv2.CAP_PROP_FRAME_HEIGHT))else: # streamfps, w, h = 30, im0.shape[1], im0.shape[0]suffix = '.mp4' if MACOS else '.avi' if WINDOWS else '.avi'fourcc = 'avc1' if MACOS else 'WMV2' if WINDOWS else 'MJPG'save_path = str(Path(save_path).with_suffix(suffix))self.vid_writer[idx] = cv2.VideoWriter(save_path, cv2.VideoWriter_fourcc(*fourcc), fps, (w, h))self.vid_writer[idx].write(im0)def run_callbacks(self, event: str):"""Runs all registered callbacks for a specific event."""for callback in self.callbacks.get(event, []):callback(self)def add_callback(self, event: str, func):"""Add callback"""self.callbacks[event].append(func)
相关文章:
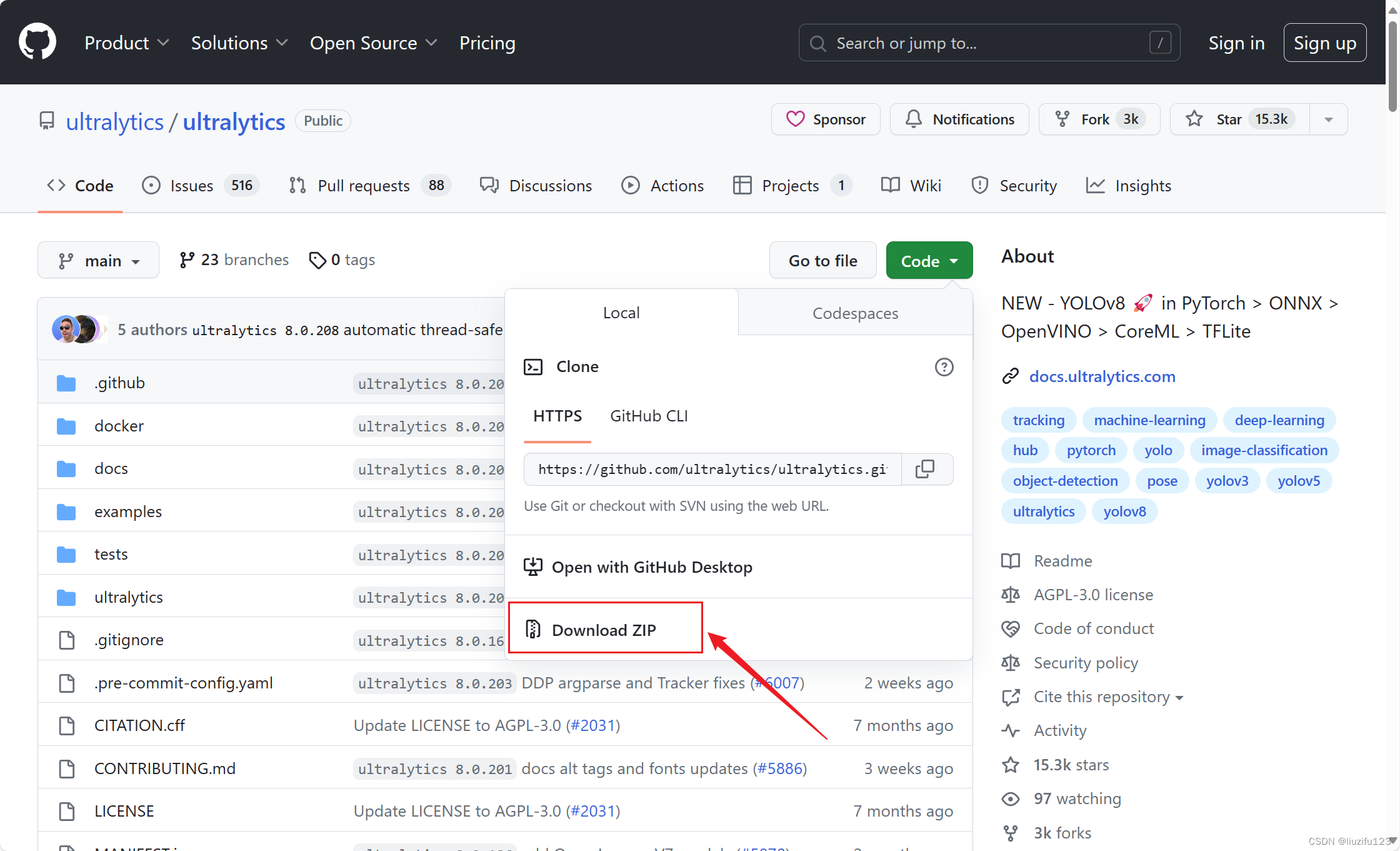
超详细||YOLOv8基础教程(环境搭建,训练,测试,部署看一篇就够)(在推理视频中添加FPS信息)
一、YOLOv8环境搭建 这篇文章将跳过基础的深度学习环境的搭建,如果没有完成的可以看我的这篇博客:超详细||深度学习环境搭建记录cudaanacondapytorchpycharm-CSDN博客 1. 在github上下载源码: GitHub - ultralytics/ultralytics: NEW - YO…...

LeetCode171. Excel Sheet Column Number
文章目录 一、题目二、题解 一、题目 Given a string columnTitle that represents the column title as appears in an Excel sheet, return its corresponding column number. For example: A -> 1 B -> 2 C -> 3 … Z -> 26 AA -> 27 AB -> 28 … Exa…...
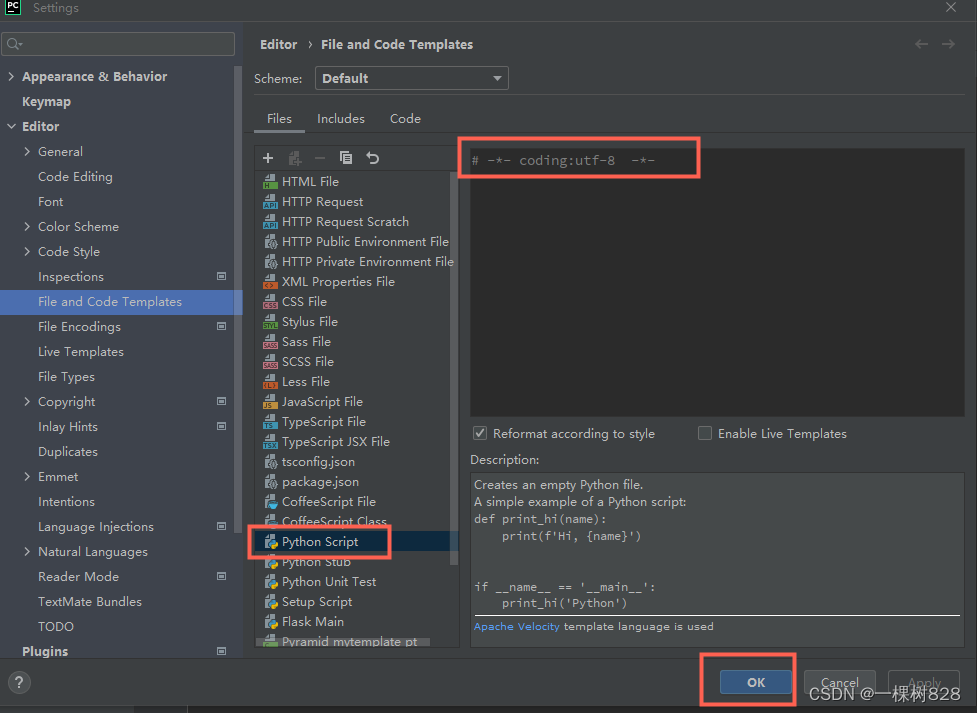
pycharm创建py文件,自动带# -*- coding:utf-8 -*-
File–Settings...
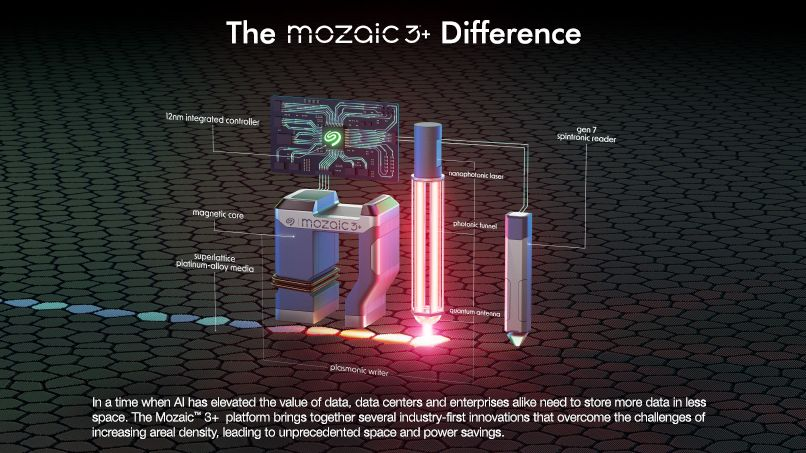
希捷与索尼集团合作生产HAMR写头激光二极管
最近有报道指出,希捷(Seagate)在生产其采用热辅助磁记录(HAMR)技术的大容量硬盘时,并非所有组件都在内部制造。根据日经新闻的一份新报告,希捷已与索尼集团合作,由索尼为其HAMR写头生…...
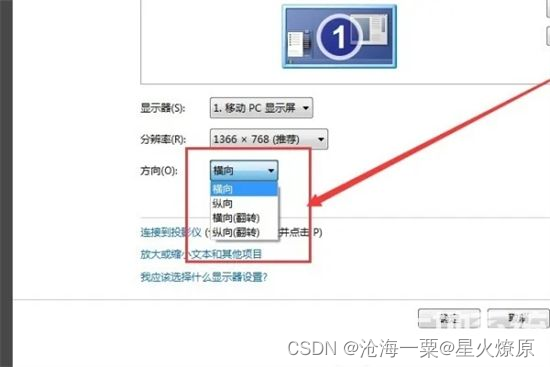
电脑竖屏显示了怎么回复原状
电脑屏幕变成这样 怎么恢复原状? 1、登录系统 2、在桌面上空白点击鼠标右键 3、在右键菜单中选择“屏幕分辨率”,左键点击打开 4、在窗口中“方向”位置选择“横向” 5、保存设置win7桌面即可恢复到正常状态...
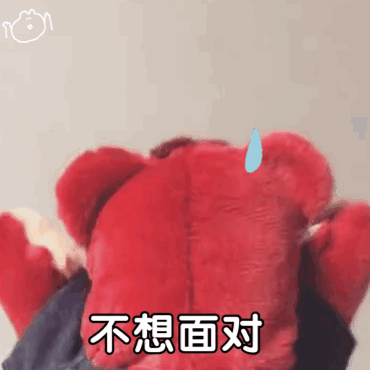
Elasticsearch从入门到精通
目录 🧂1.简单介绍 🥓2.安装与下载 🌭3.安装启动es 🍿4.安装启动kibana 🥞5.初步检索 🧈6.进阶检索 🫓7.Elasticsearch整合 1.简单介绍🚗🚗🚗 Elat…...
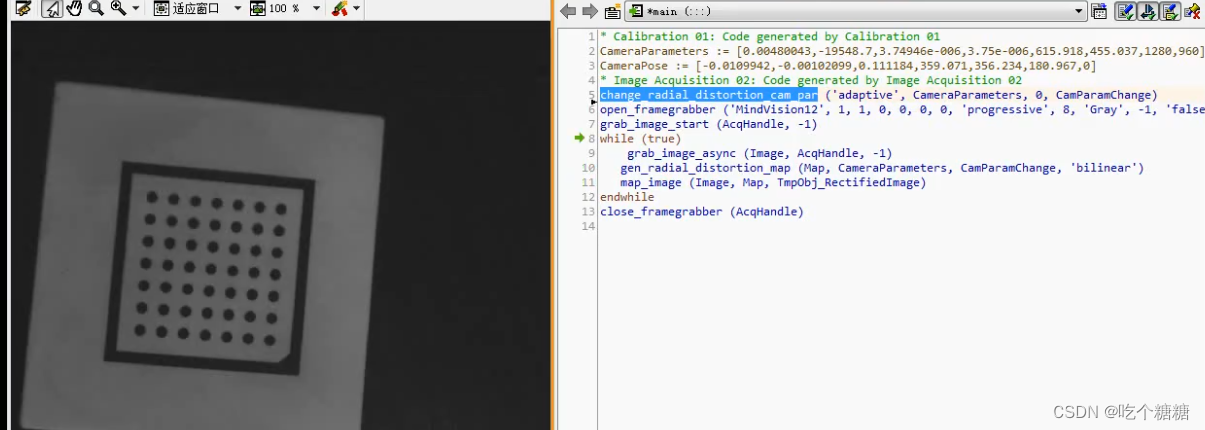
Halcon 相机标定
文章目录 算子单相机标定单相机标定畸变的矫正 算子 gen_caltab 生成标定文件 gen_caltab(::XNum,YNum,MarkDist,DiameterRatio,CalTabDescrFile,CalTabPSFile :) 算子来制作一个标定板XNum 每行黑色标志圆点的数量。YNum 每列黑色标志圆点的数…...

【JavaScript】深浅拷贝
JavaScript中复制对象有深拷贝和浅拷贝两种方式 浅拷贝:浅拷贝只复制对象的第一层属性,而不复制嵌套对象的引用。这意味着如果原始对象包含嵌套对象,浅拷贝后的对象仍然会共享这些嵌套对象的引用。可以使用`Object.assign()`或展开运算符(`...`)来进行浅拷贝。 // 浅拷贝示…...

CH32V3xx RT-Thread RS485实现modbus rtu master
目录 1、串口配置1.1 串口初始化1.2 uart DMA 初始化1.1.3 发送函数2、agile modbus3、应用测试4、遇到的问题本文通过ch32v3xx的串口 + RS485收发器实现modbus rtu master设备。此工程中移植的RT-Thread Nano系统,详情可参看本专栏前几篇文章。 1、串口配置 串口使用重映射后…...

当网站遭到DDOS攻击怎么办?
一般网站进行上线后会经常遭到攻击,有一些攻击不怎么容易被发现,当大规模的攻击来临时,会给企业造成巨大的损失,使网站的业务下线并且访问异常,出现很多的问题。 其中DDOS攻击是最高频的一种网络攻击方式,那…...

ES6中的数组解构赋值【详解】
文章目录 1.数组的解构赋值1.1 基本用法1.2 默认值1.3更多对象解构赋值 1.数组的解构赋值 1.1 基本用法 ES6允许按照一定的模式,从数组和对象中提取值,对变量进行赋值,这被称为结构。 let [a, b, c] [1, 2, 3];如果解构不成功,…...

error An unexpected error occurred: “https://registry.npm.taobao.org
背景: 想使用yarn命令结果报错 问题原因: 原来证书到期了 http://registry.npm.taobao.org/ 把这个放到浏览器搜索的时候自动换成https://registry.npmmirror.com/ 方案: npm cache clean --forcenpm config set registry https://registry…...

react中commit工作流程
整个React工作流程可以分为两大阶段: Render阶段 Schecule Reconcile Commit阶段 注意,Render阶段是在内存中运行的,这意味者可以被打断,而commit阶段一旦开始同步执行直到完成。 Renderer工作的阶段被称为commit阶段。commit阶…...
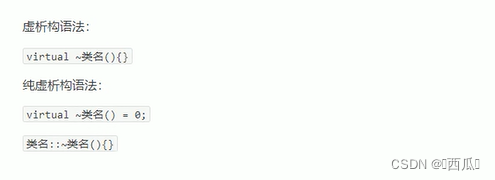
C++类和对象-多态->多态的基本语法、多态的原理剖析、纯虚函数和抽象类、虚析构和纯虚析构
#include<iostream> using namespace std; //多态 //动物类 class Animal { public: //Speak函数就是虚函数 //函数前面加上virtual关键字,变成虚函数,那么编译器在编译的时候就不能确定函数调用了。 virtual void speak() { …...

QShortcut
一、QShortcut简介 QShortcut是Qt框架中提供的一个类,用于创建和管理键盘快捷键。它允许开发者为应用程序定义一组快捷键组合,当用户按下这些组合键时,可以触发相应的动作或事件。QShortcut的使用使得用户能够更加方便、快捷地操作应用程序&…...
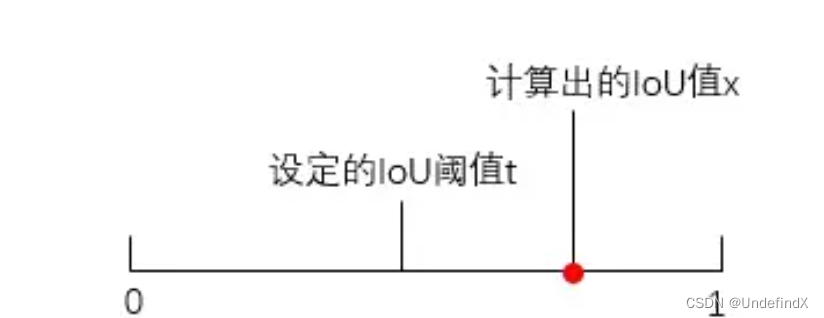
浅谈语义分割、图像分类与目标检测中的TP、TN、FP、FN
语义分割 TP:正确地预测出了正类,即原本是正类,识别的也是正类 TN:正确地预测出了负类,即原本是负类,识别的也是负类 FP:错误地预测为了正类,即原本是负类,识别的是正类…...
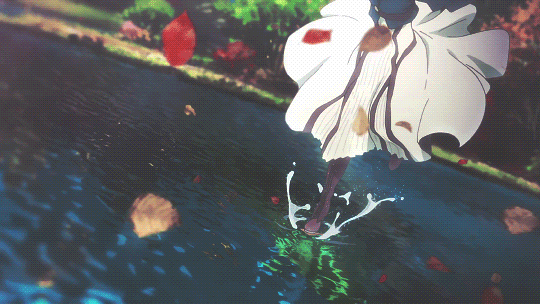
Python基础教程:解构
嗨喽~大家好呀,这里是魔王呐 ❤ ~! python更多源码/资料/解答/教程等 点击此处跳转文末名片免费获取 我们提到了字典的.items()方法会返回键值对元组的键值对元组列表: dic {key: 1, dsb: alex} print(dic.items())输出的内容为: dict_it…...
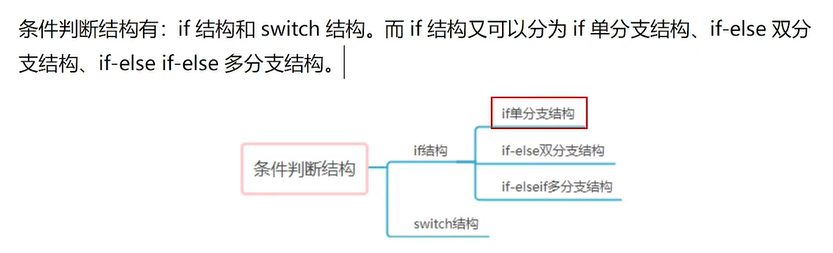
Java 学习和实践笔记(12)
这个就比较有意思了!所有的事情,拆分完之后,都有且只有这三种状态流程! //TIP To <b>Run</b> code, press <shortcut actionId"Run"/> or // click the <icon src"AllIcons.Actions.Execute&…...
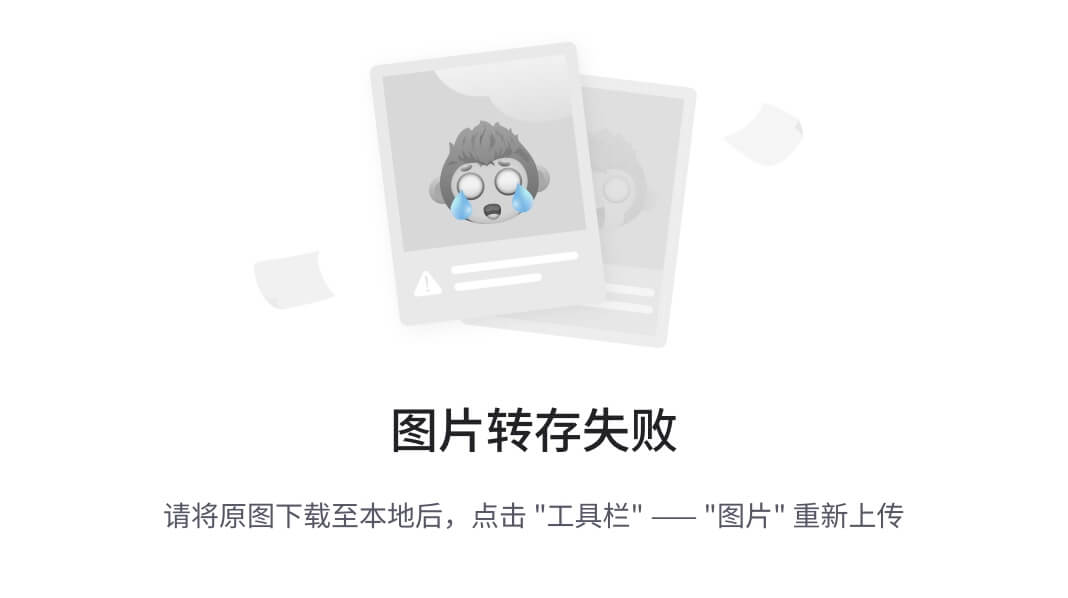
学习数据结构和算法的第9天
题目讲解 移除元素 给你一个数组nums和一个值 val,你需要 原地 移除所有数值等于 val的元素,并返回移除后数组的新长度。 不要使用额外的数组空间,你必须仅使用0(1)额外空间并 原地 修改输入数组。 元素的顺序可以改变。你不需要…...
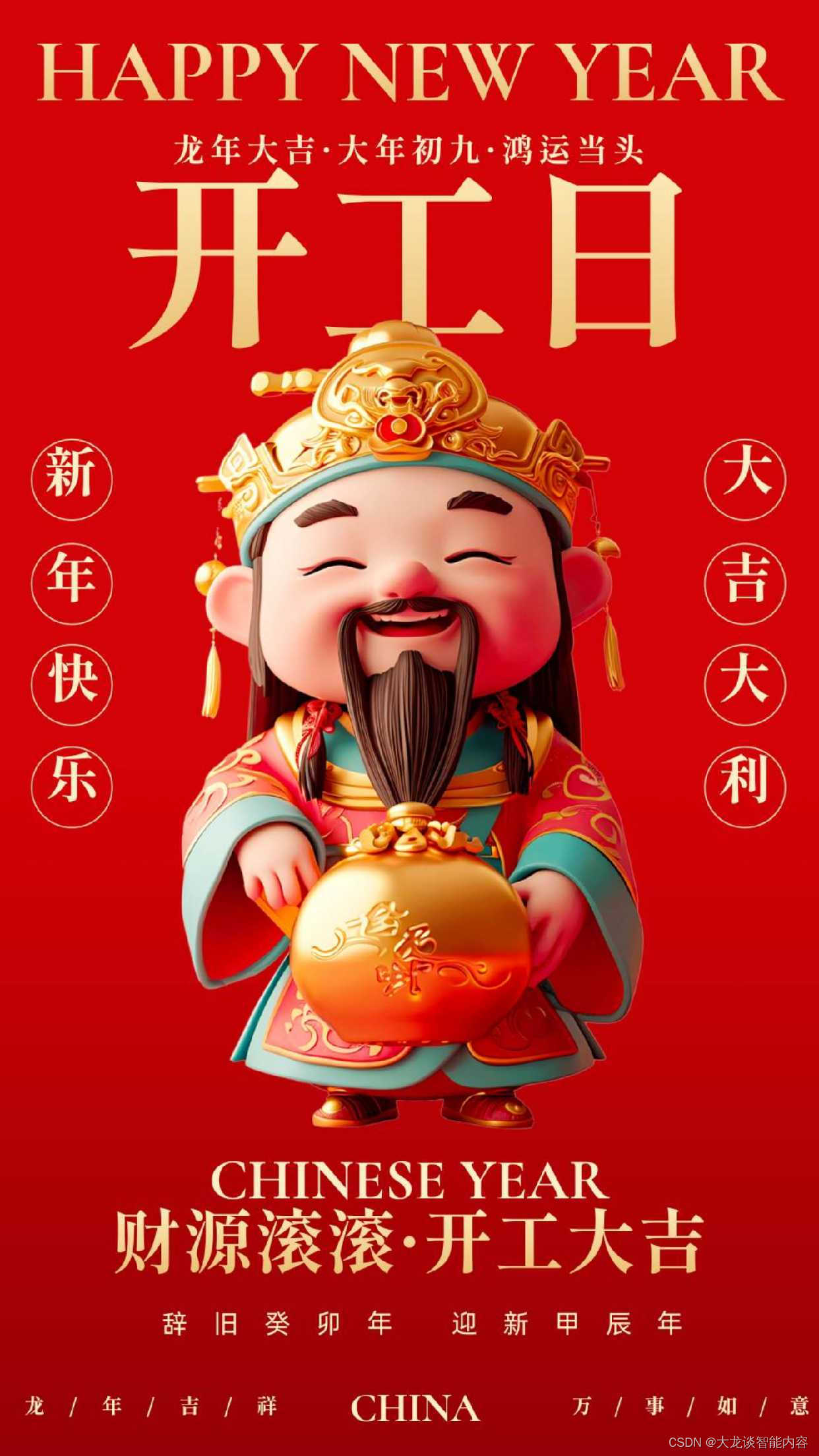
大龙谈智能内容 - 开工大吉
今天是2024年2月18日,开工第一天。 祝关注“大龙谈智能内容”的朋友龙年如龙飞天,事业步步高升!...
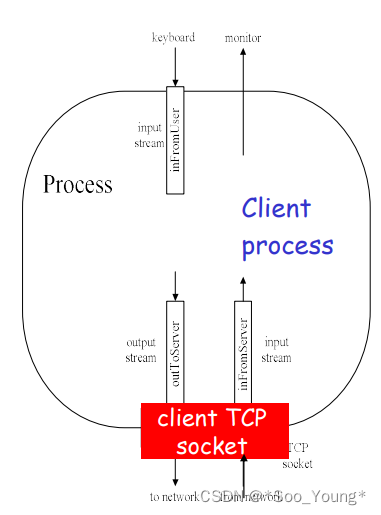
中科大计网学习记录笔记(十二):TCP 套接字编程
前前言:大家看到这一章节的时候一定不要跳过,虽然标题是编程,但实际上是对 socket 的运行机制做了详细的讨论,对理解 TCP 有很大的帮助;但是由于本节涉及到了大量的编程知识,对于一些朋友来说不是很好理解&…...
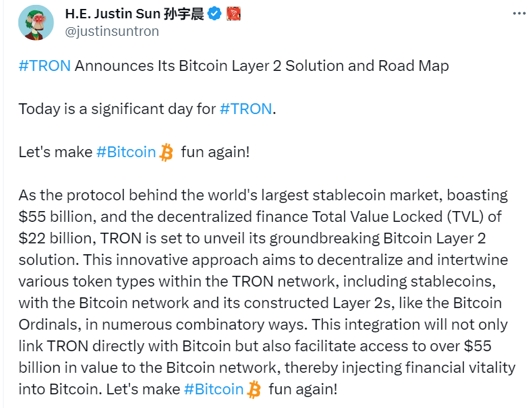
落实三大阶段目标,TRON全方位打通与BTC生态互联
2月15日,波场TRON创始人、火币HTX全球顾问委员会委员孙宇晨在X平台发布公告表示,波场TRON已正式公布比特币第二层解决方案及路线图,围绕打通比特币与波场TRON网络的跨链连接、投资开发用户友好的钱包和工具,同时与多个比特币第二层协议进行合作等重点,全方位拥抱比特币发展机遇…...
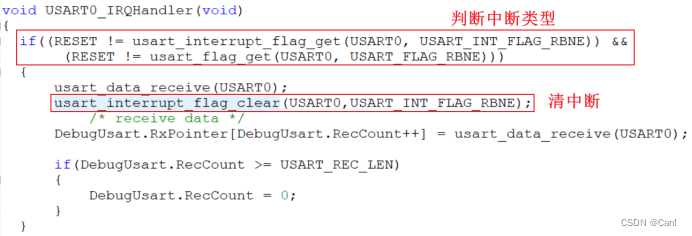
MCU中断控制
目录 一、中断相关基础知识 1、NVIC:嵌套向量中断控制器 2、可屏蔽中断和不可屏蔽中断的区别 3、中断优先级 4、常见特殊中断 二、中断相关寄存器 三、中断使用步骤: 一、中断相关基础知识 1、NVIC:嵌套向量中断控制器 (1) 它是内核的…...
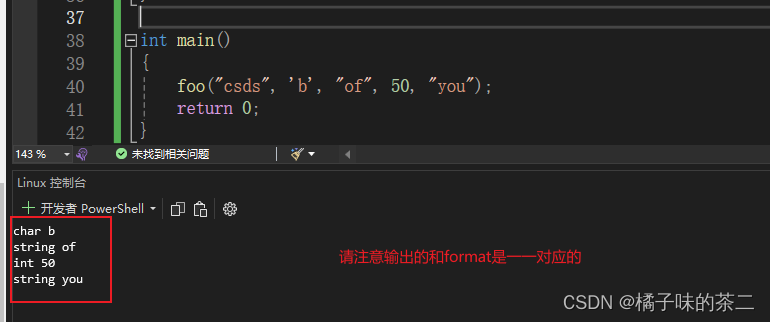
C语言中的可变参数
目录 可变参数函数原理与分析总结 实现方案1、 va_start 宏2、 va_arg 宏3、 va_end 宏 应用举例举例1:提前已知所有参数类型的简单情况举例2:通过固定参数,来动态确定可变参数类型的复杂情况 可变参数函数 在C语言中,有这样的一…...
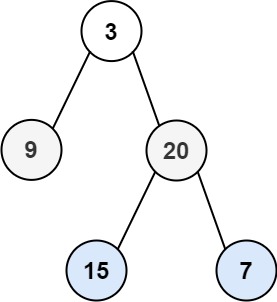
Leetcode-103. 二叉树的锯齿形层序遍历
这个年和树过不去啦啦啦! 题目: 给你二叉树的根节点 root ,返回其节点值的 锯齿形层序遍历 。(即先从左往右,再从右往左进行下一层遍历,以此类推,层与层之间交替进行)。 示例 1&…...

vs code“无法与远程服务器建立连接:XHR failed.”解决办法
获取到 commit id 的方式参考: vscode通过ssh链接服务器卡在downloading with wget - 知乎 关于下载 vscode-server-linux-x64.tar.gz,浏览器打开: https://vscode.download.prss.microsoft.com/dbazure/download/stable/你的commit id/vs…...
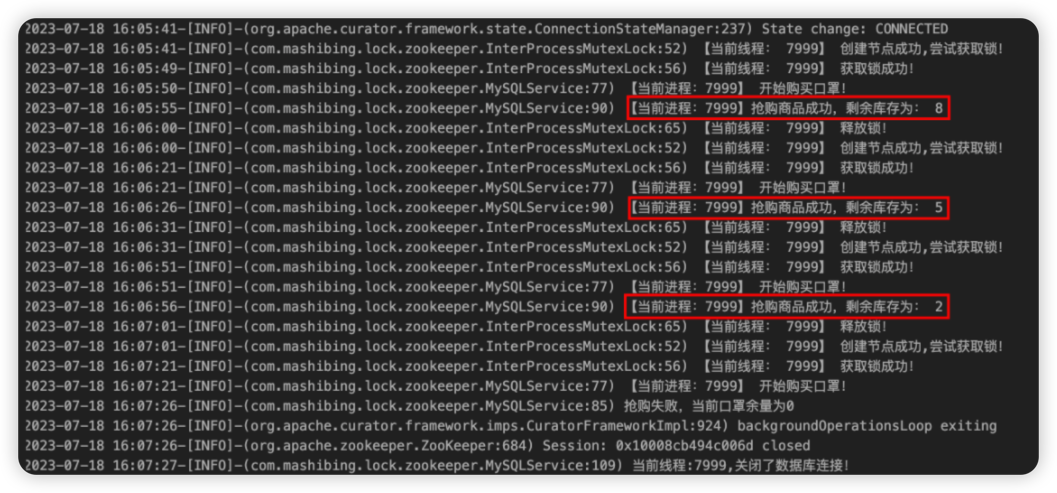
第五节 zookeeper集群与分布式锁_2
1.分布式锁概述 1.1 什么是分布式锁 1)要介绍分布式锁,首先要提到与分布式锁相对应的是线程锁。 线程锁:主要用来给方法、代码块加锁。当某个方法或代码使用锁,在同一时刻仅有一个线程执行该方法或该代码段。 线程锁只在同一J…...

Shell脚本——提取目录名和文件名
目录 一、${} 1.${var##*/} 2.${var##*.} 3.${var#*.} 4.${var%/*} 5.${var%%.*} 6.总结 二、basename和dirname 1.basename 2.dirname 在许多场景下,我们都需要对文件名称或者文件所在的目录进行操作,已达到我们业务目的。通常的操作是由路径…...
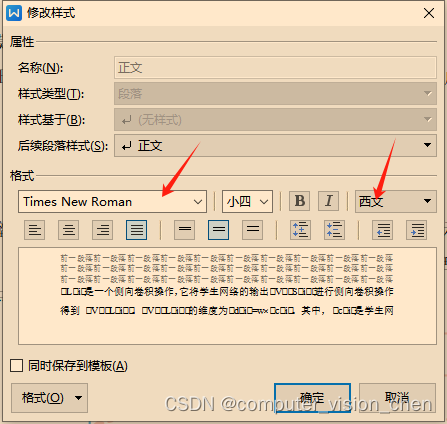
wps使用方法(包括:插入倒三角符号,字母上面加横线,将word中的所有英文设置为time new roman)
倒三角符号 字母上面加横线 将word中的所有英文设置为time new roman ctrla选中全文...
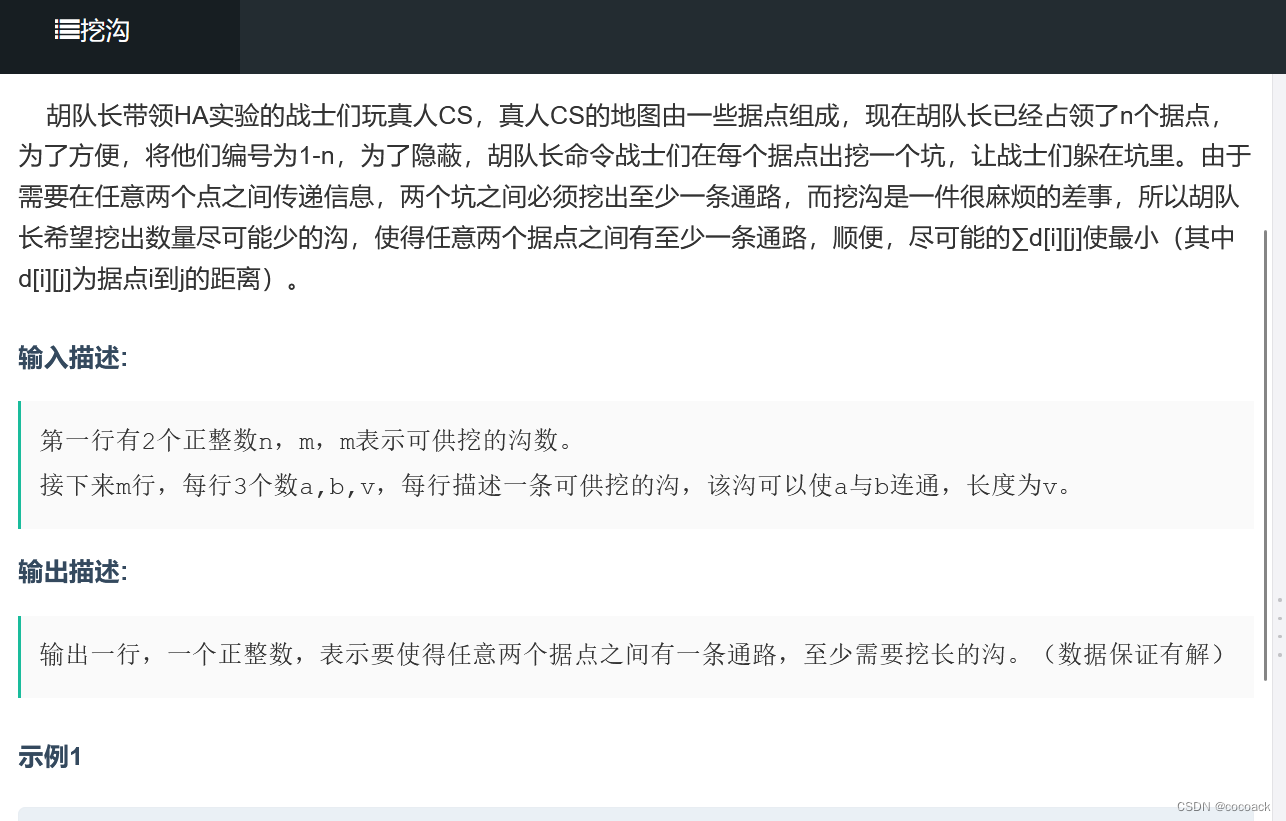
备战蓝桥杯---图论之最小生成树
首先,什么是最小生成树? 他就是无向图G中的所有生成树中树枝权值总和最小的。 如何求? 我们不妨采用以下的贪心策略: Prim算法(复杂度:(nm)logm): 我们对于把上述的点看成两个集…...
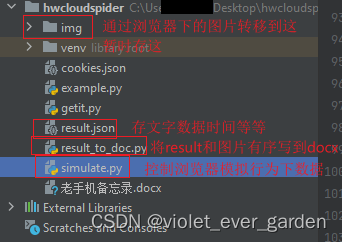
爬虫-华为云空间备忘录导出到docx-selenium控制浏览器行为-python数据处理
背景适用情况介绍 老的荣耀手机属于华为云系统,家里人换了新荣耀手机属于荣耀云系统无法通过云空间将备忘录转移到新手机,不想让他们一个一个搞,于是整了一晚上想办法爬取下来。从网页抓取下来,然后存到docx文档中(包…...

网络安全的新防线:主动进攻,预防为先
进攻性安全(Offensive security)是指一系列主动安全策略,这些策略与恶意行为者在现实世界的攻击中使用的策略相同,区别在于其目的是加强而非损害网络安全。常见的进攻性安全方法包括红队、渗透测试和漏洞评估。 进攻性安全行动通常…...
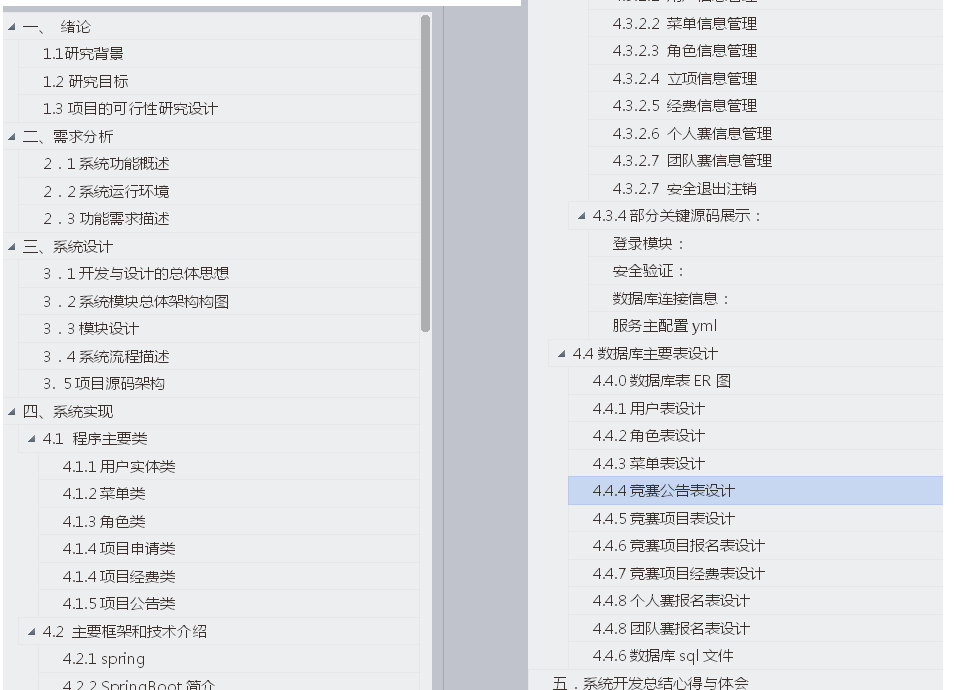
基于java springboot+mybatis学生学科竞赛管理管理系统设计和实现
基于java springbootmybatis学生学科竞赛管理管理系统设计和实现 🍅 作者主页 央顺技术团队 🍅 欢迎点赞 👍 收藏 ⭐留言 📝 🍅 文末获取源码联系方式 📝 🍅 查看下方微信号获取联系方式 承接各…...
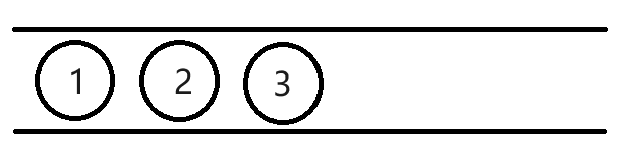
秒懂百科,C++如此简单丨第二十一天:栈和队列
目录 前言 Everyday English 栈(Stack) 图文解释 实现添加删除元素 实现查看清空栈 完整代码 运行示例 栈的选择题 队列(Queue) 图文解释 队列的基本用法 完整代码 运行结果 队列的好处 结尾 前言 今天我们将…...

STM32-开发环境之STM32CubeMX
目录 STM32CubeMX介绍 STM32CubeMX特性 应用场景 其他事项 STM32CubeMX介绍 STM32CubeMX是ST公司(意法半导体)推出的一款图形化工具,也是配置和初始化C代码生成器。它主要服务于STM32微控制器的配置和开发。 STM32CubeMX特性 1.直观选…...
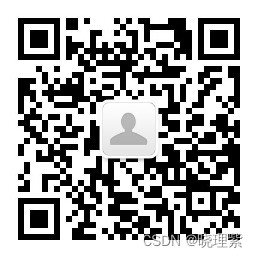
[晓理紫]CCF系列会议截稿时间订阅
CCF系列会议截稿时间订阅 VX 关注{晓理紫},每日更新最新CCF系列会议信息,如感兴趣,请转发给有需要的同学,谢谢支持!! 如果你感觉对你有所帮助,请关注我,每日准时为你推送最新CCF会议…...
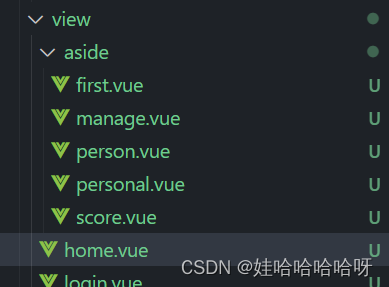
重复导航到当前位置引起的。Vue Router 提供了一种机制,阻止重复导航到相同的路由路径。
代码: <!-- 侧边栏 --><el-col :span"12" :style"{ width: 200px }"><el-menu default-active"first" class"el-menu-vertical-demo" select"handleMenuSelect"><el-menu-item index"…...

如何在 Angular 中使用 Flex 布局
介绍 Flex Layout 是一个组件引擎,允许您使用 CSS Flexbox 创建页面布局,并提供一组指令供您在模板中使用。 该库是用纯 TypeScript 编写的,因此不需要外部样式表。它还提供了一种在不同断点上指定不同指令以创建响应式布局的方法。 在本教…...
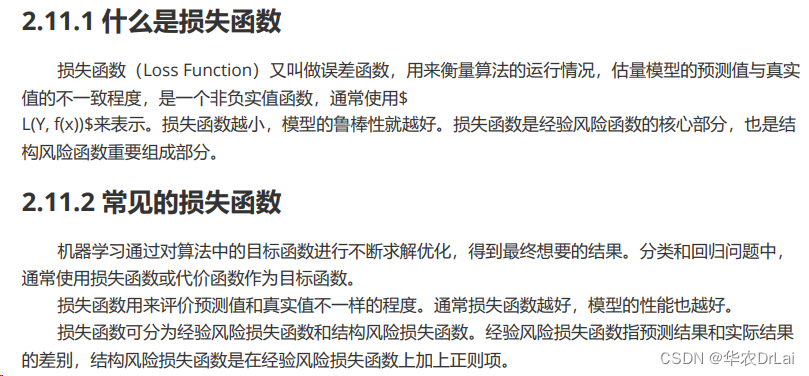
通俗的讲解什么是机器学习之损失函数
想象一下,你在玩一个靶心射击的游戏,你的目标是尽可能让箭簇命中靶心。在这个游戏中,损失函数可以看作是测量你的箭簇与靶心距离的规则。损失函数的值越小,意味着你的箭簇离靶心越近,你的射击技能越好。 在机器学习中…...
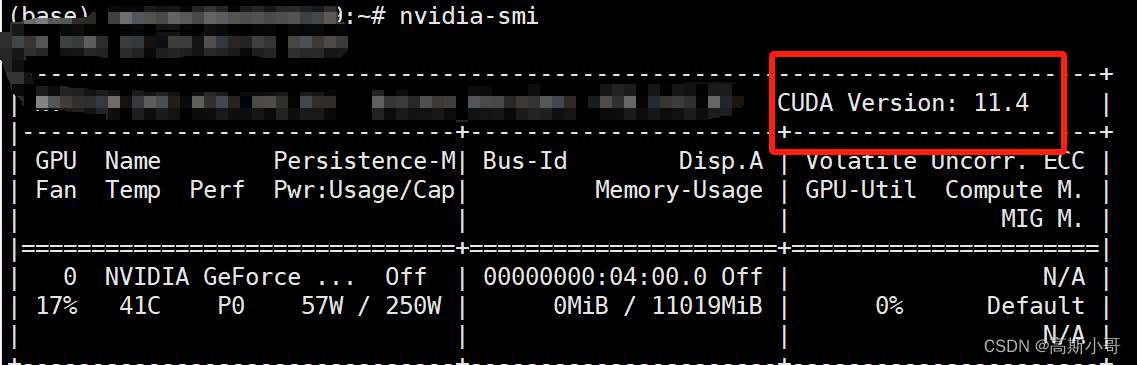
快速搭建PyTorch环境:Miniconda一步到位
快速搭建PyTorch环境:Miniconda一步到位 🌵文章目录🌵 🌳一、为何选择Miniconda搭建PyTorch环境?🌳🌳二、Miniconda安装指南:轻松上手🌳🌳三、PyTorch与Minic…...
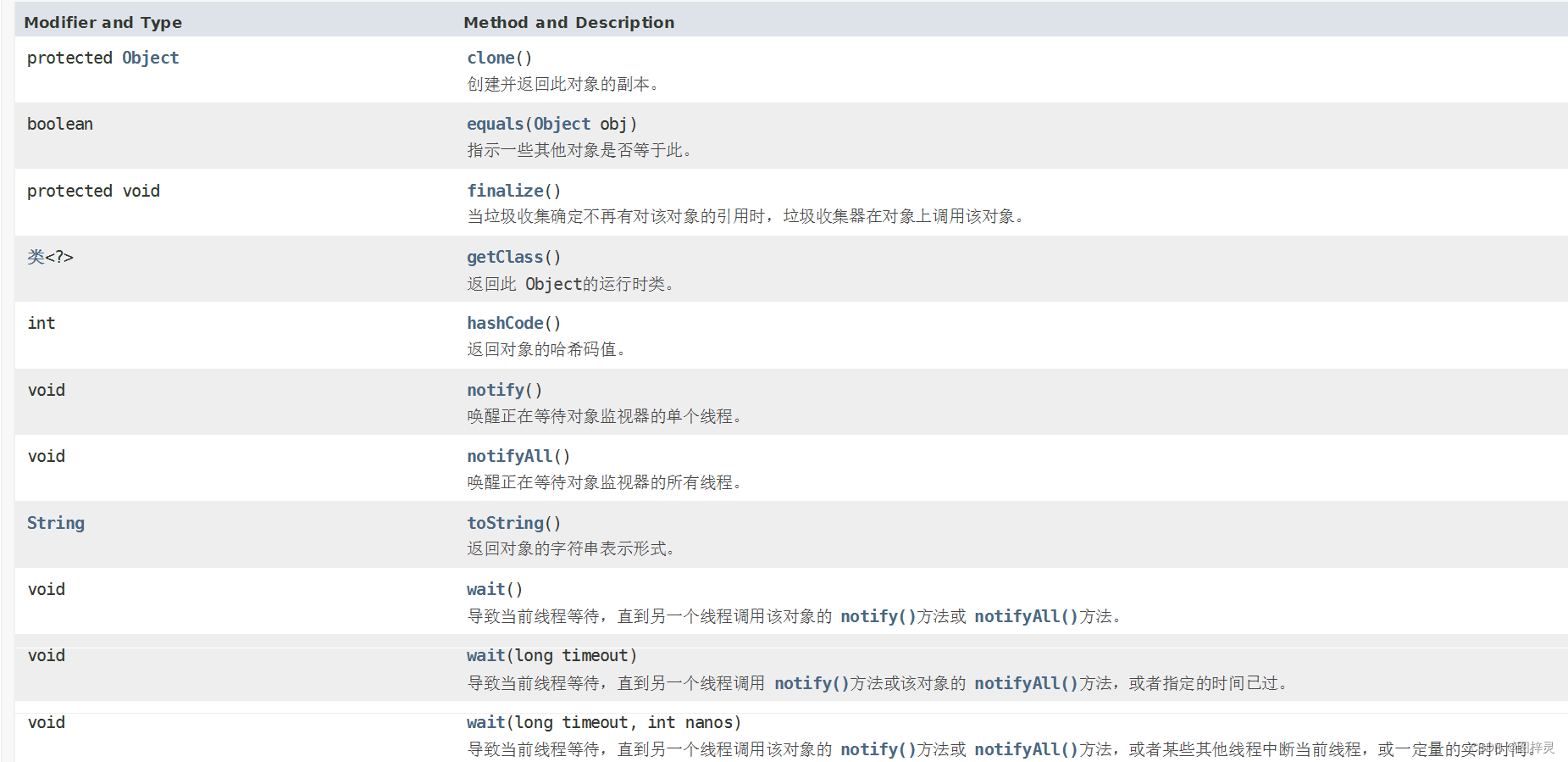
图灵日记之java奇妙历险记--抽象类和接口
目录 抽象类概念抽象类语法 接口概念规则使用特性实现多个接口接口的继承接口使用实例Clonable接口和深拷贝抽象类和接口的区别 Object类 抽象类 概念 在面向对象的概念中,所有对象都是通过类来描述的,但是反过来,并不是所有的类都是用来描绘对象的,如果一个类中没有包含足够…...

批量给元素添加进场动画;获取文本光标位置;项目国际化
批量给元素添加进场动画 api及参数参考:https://juejin.cn/post/7310977323484971071 简单实现: addAnimationClass(){//交叉观察器if (window?.IntersectionObserver) {//获取所有需要添加进场动画的元素,放到一个数组let items [...do…...
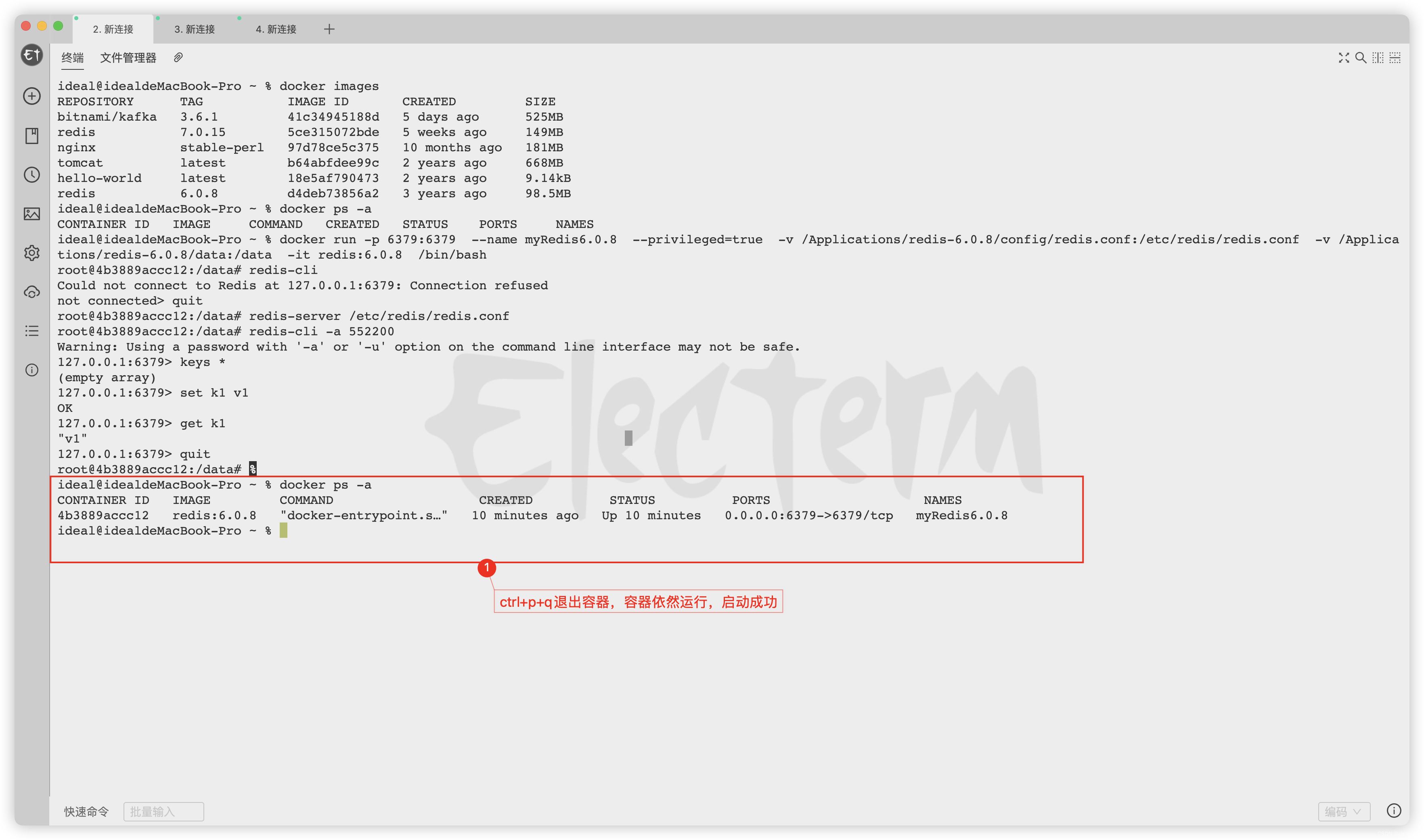
解决:docker创建Redis容器成功,但无法启动Redis容器、也无报错提示
解决:docker创建Redis容器成功,但无法启动Redis容器、也无报错提示 一问题描述:1.docker若是直接简单使用run命令,但不挂载容器数据卷等参数,则可以启动Redis容器2.docker复杂使用run命令,使用指定redis.co…...
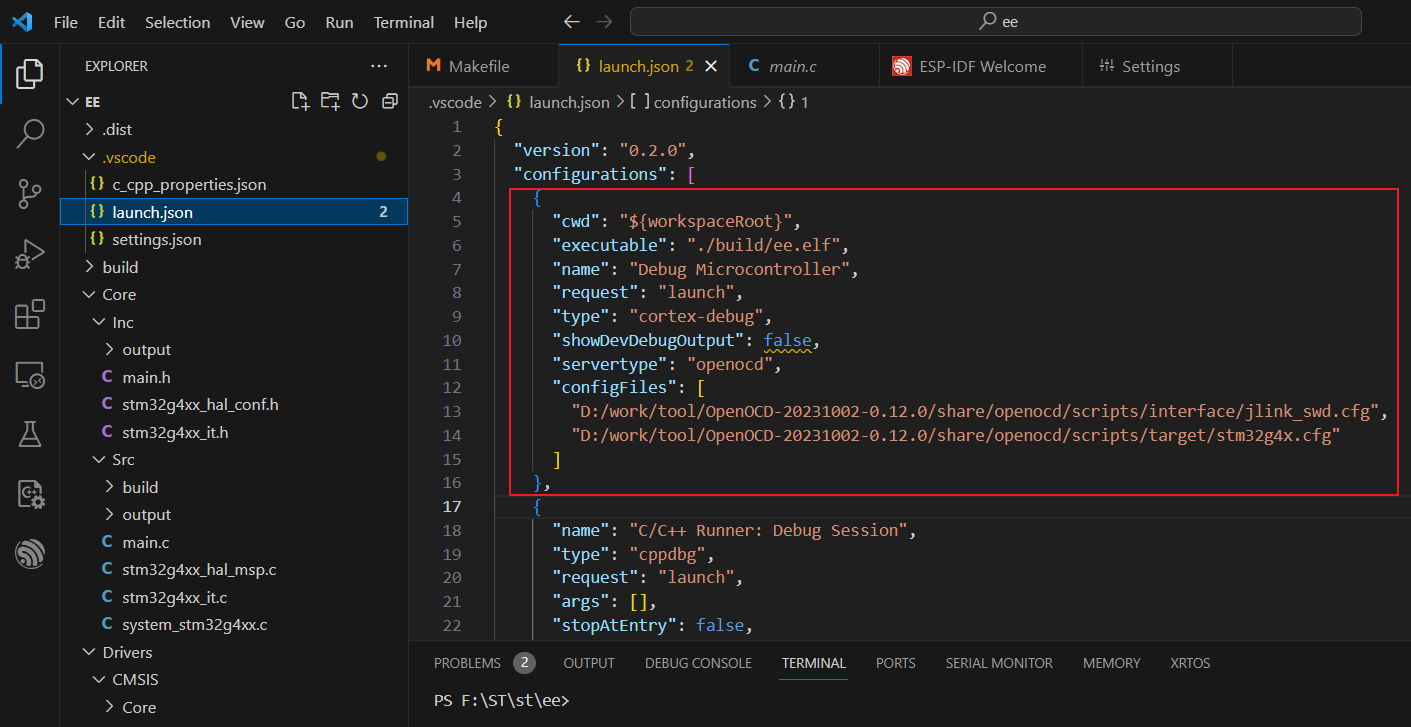
Jlink+OpenOCD+STM32 Vscode 下载和调试环境搭建
对于 Mingw 的安装比较困难,国内的网无法正常在线下载组件, 需要手动下载 x86_64-8.1.0-release-posix-seh-rt_v6-rev0.7z 版本的软件包,添加环境变量,并将 mingw32-make.exe 名字改成 make.exe。 对于 OpenOCD,需要…...
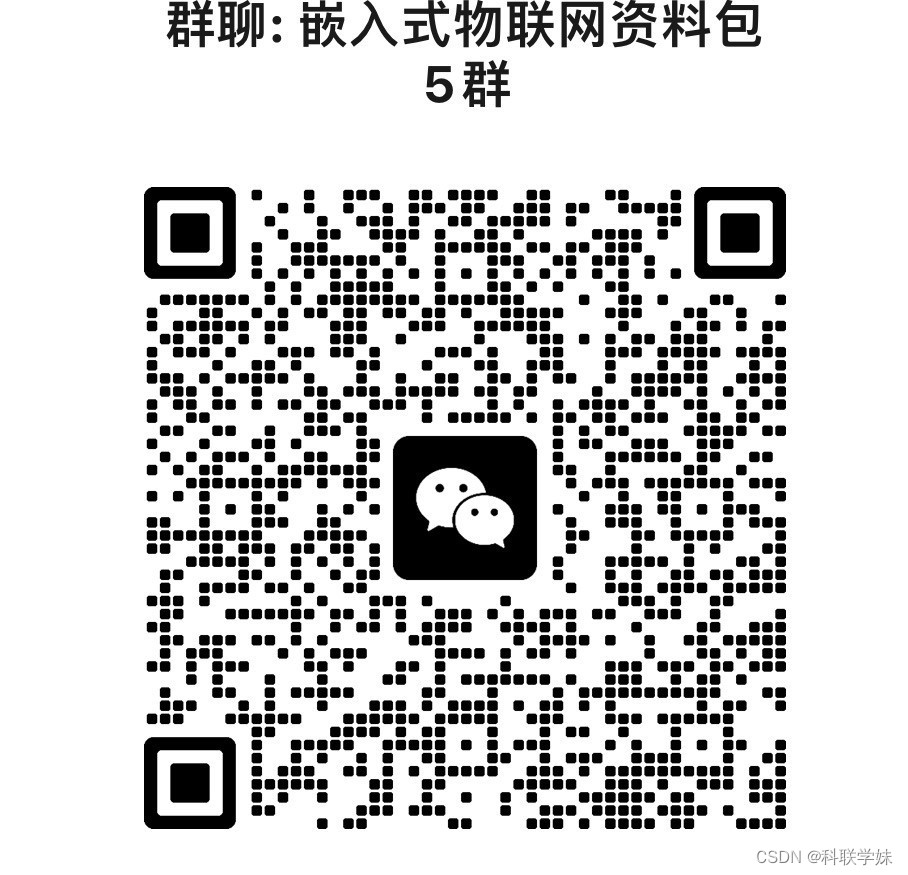
单片机在物联网中的应用
单片机,这个小巧的电子设备,可能听起来有点技术性,但它实际上是物联网世界中的一个超级英雄。简单来说,单片机就像是各种智能设备的大脑,它能让设备“思考”和“行动”。由于其体积小、成本低、功耗低、易于编程等特点…...
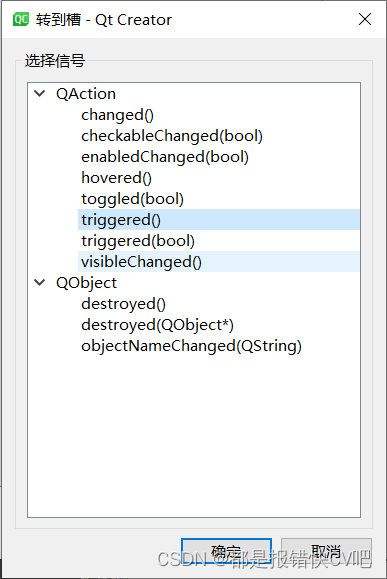
16.Qt 工具栏生成
目录 前言: 技能: 内容: 1. 界面添加 2. 信号槽 功能实现 参考: 前言: 基于QMainWindow,生成菜单下面的工具栏,可以当作菜单功能的快捷键,也可以完成新的功能 直接在UI文件中…...

【Linux内核】从0开始入门Linux Kernel源码
🌈 博客个人主页:Chris在Coding 🎥 本文所属专栏:[Linux内核] ❤️ 前置学习专栏:[Linux学习]从0到1 ⏰ 我们仍在旅途 目录 …...
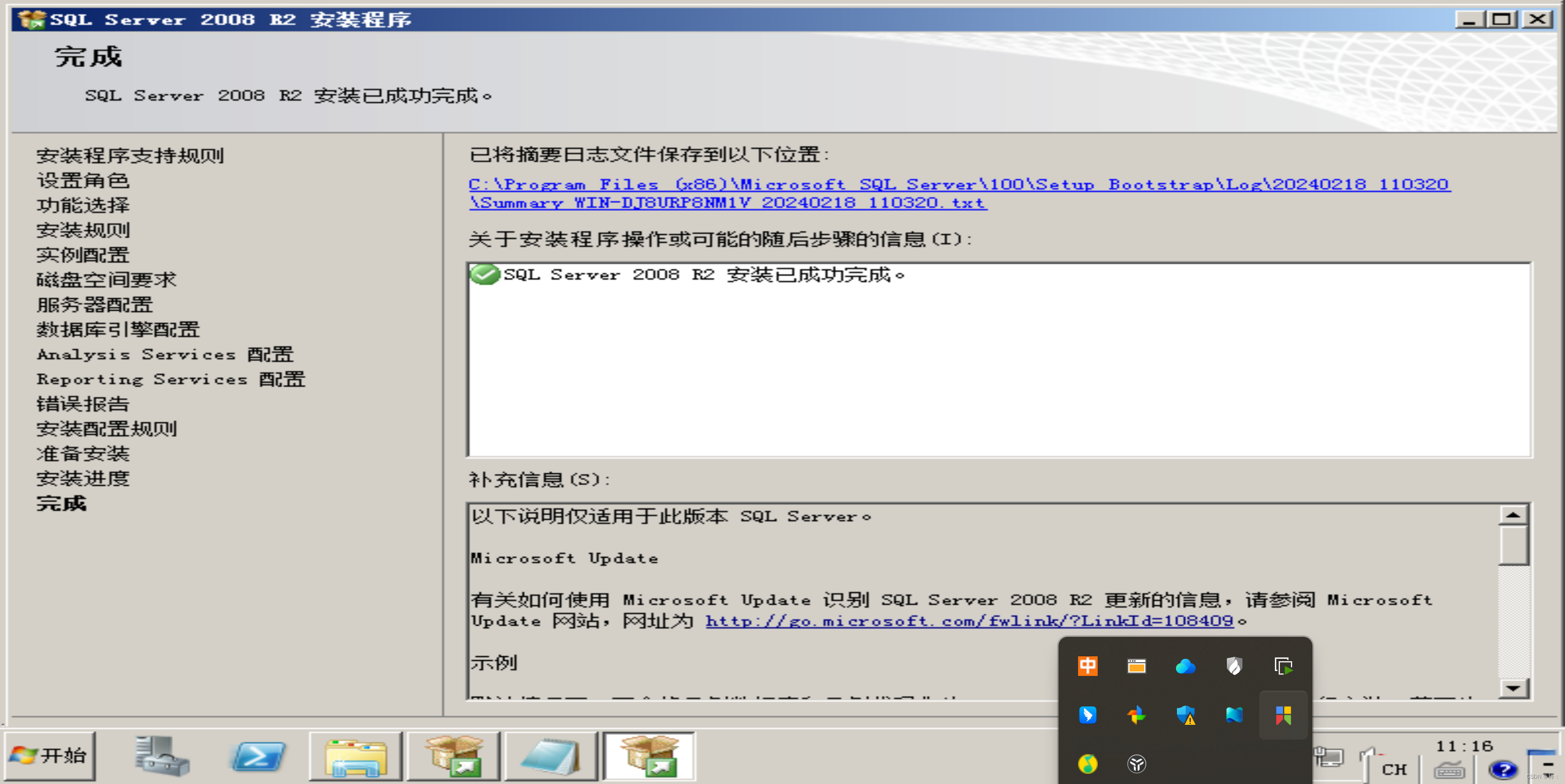
SQL Service 2008 的安装与配置
点击添加当前用户...
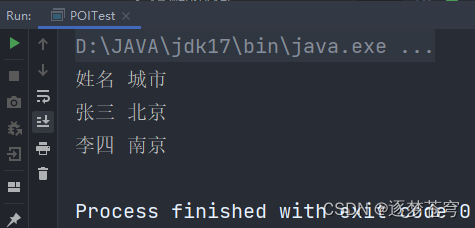
Apache POI | Java操作Excel文件
目录 1、介绍 2、代码示例 2.1、将数据写入Excel文件 2.2、读取Excel文件中的数据 🍃作者介绍:双非本科大三网络工程专业在读,阿里云专家博主,专注于Java领域学习,擅长web应用开发、数据结构和算法,初步…...

vue 学习definproperty方法
definproperty方法是Vue很重要的一个底层方法,掌握他的原理很重要,下面通过代码说明问题: <!DOCTYPE html> <html><head><meta charset"UTF-8" /><title>回顾Object.defineproperty方法</title&…...