神经网络代码实现(用手写数字识别数据集实验)
目录
一、前言
二、神经网络架构
三、算法实现
1、导入包
2、实现类
3、训练函数
4、权重参数矩阵初始化
5、参数矩阵变换向量
6、向量变换权重参数矩阵
7、进行梯度下降
7.1、损失函数
7.1.1、前向传播
7.2、反向传播
8、预测函数
四、完整代码
五、手写数字识别
一、前言
读者需要了解神经网络的基础知识,可以参考神经网络(深度学习,计算机视觉,得分函数,损失函数,前向传播,反向传播,激活函数)
本文为大家详细的描述了,实现神经网络的逻辑,代码。并且用手写识别来实验,结果基本实现了神经网络的要求。
二、神经网络架构
想一想:
1.输入数据:特征值(手写数字识别是像素点,784个特征)
2.W1,W2,W3矩阵的形状
3.前向传播
4.激活函数(用Sigmoid)
5.反向传播
6.偏置项
7.损失()
8.得出W1,W2,W3对损失有多大影响,公式如下:
算法流程(简便版):
三、算法实现
1、导入包
import numpy as np
from Neural_Network_Lab.utils.features import prepare_for_training
from Neural_Network_Lab.utils.hypothesis import sigmoid,sigmoid_gradient
这里utils包用来封装数据预处理,和Sigmoid函数
"""Add polynomial features to the features set"""import numpy as np
from .normalize import normalizedef generate_polynomials(dataset, polynomial_degree, normalize_data=False):"""变换方法:x1, x2, x1^2, x2^2, x1*x2, x1*x2^2, etc."""features_split = np.array_split(dataset, 2, axis=1)dataset_1 = features_split[0]dataset_2 = features_split[1](num_examples_1, num_features_1) = dataset_1.shape(num_examples_2, num_features_2) = dataset_2.shapeif num_examples_1 != num_examples_2:raise ValueError('Can not generate polynomials for two sets with different number of rows')if num_features_1 == 0 and num_features_2 == 0:raise ValueError('Can not generate polynomials for two sets with no columns')if num_features_1 == 0:dataset_1 = dataset_2elif num_features_2 == 0:dataset_2 = dataset_1num_features = num_features_1 if num_features_1 < num_examples_2 else num_features_2dataset_1 = dataset_1[:, :num_features]dataset_2 = dataset_2[:, :num_features]polynomials = np.empty((num_examples_1, 0))for i in range(1, polynomial_degree + 1):for j in range(i + 1):polynomial_feature = (dataset_1 ** (i - j)) * (dataset_2 ** j)polynomials = np.concatenate((polynomials, polynomial_feature), axis=1)if normalize_data:polynomials = normalize(polynomials)[0]return polynomials
import numpy as npdef generate_sinusoids(dataset, sinusoid_degree):"""sin(x)."""num_examples = dataset.shape[0]sinusoids = np.empty((num_examples, 0))for degree in range(1, sinusoid_degree + 1):sinusoid_features = np.sin(degree * dataset)sinusoids = np.concatenate((sinusoids, sinusoid_features), axis=1)return sinusoids
"""Normalize features"""import numpy as npdef normalize(features):features_normalized = np.copy(features).astype(float)# 计算均值features_mean = np.mean(features, 0)# 计算标准差features_deviation = np.std(features, 0)# 标准化操作if features.shape[0] > 1:features_normalized -= features_mean# 防止除以0features_deviation[features_deviation == 0] = 1features_normalized /= features_deviationreturn features_normalized, features_mean, features_deviation
数据预处理:
"""Prepares the dataset for training"""import numpy as np
from .normalize import normalize
from .generate_sinusoids import generate_sinusoids
from .generate_polynomials import generate_polynomialsdef prepare_for_training(data, polynomial_degree=0, sinusoid_degree=0, normalize_data=True):# 计算样本总数num_examples = data.shape[0]data_processed = np.copy(data)# 预处理features_mean = 0features_deviation = 0data_normalized = data_processedif normalize_data:(data_normalized,features_mean,features_deviation) = normalize(data_processed)data_processed = data_normalized# 特征变换sinusoidalif sinusoid_degree > 0:sinusoids = generate_sinusoids(data_normalized, sinusoid_degree)data_processed = np.concatenate((data_processed, sinusoids), axis=1)# 特征变换polynomialif polynomial_degree > 0:polynomials = generate_polynomials(data_normalized, polynomial_degree, normalize_data)data_processed = np.concatenate((data_processed, polynomials), axis=1)# 加一列1data_processed = np.hstack((np.ones((num_examples, 1)), data_processed))return data_processed, features_mean, features_deviation
Sigmoid函数:
import numpy as npdef sigmoid(matrix):"""Applies sigmoid function to NumPy matrix"""return 1 / (1 + np.exp(-matrix))
2、实现类
多层感知机 初始化:数据,标签,网络层次(用列表表示如三层[784,25,10]表示输入层784个神经元,25个隐藏层神经元,10个输出层神经元),数据是否标准化处理。
class MultilayerPerceptron:def __init__(self,data,labels,layers,normalize_data=False):data_processed = prepare_for_training(data,normalize_data=normalize_data)[0]self.data = data_processedself.labels = labelsself.layers = layers # [ 784 ,25 ,10]self.normalize_data = normalize_dataself.thetas = MultilayerPerceptron.thetas_init(layers)
3、训练函数
输入迭代次数,学习率,进行梯度下降算法,更新权重参数矩阵,得到最终的权重参数矩阵,和损失值。矩阵不好进行更新操作,可以把它拉成向量。
def train(self,max_ietrations = 1000,alpha = 0.1):#方便矩阵更新 拉长 把矩阵拉成向量unrolled_theta = MultilayerPerceptron.thetas_unroll(self.thetas)(optimized_theta, cost_history) = MultilayerPerceptron.gradient_descent(self.data,self.labels,unrolled_theta,self.layers,max_ietrations,alpha)self.thetas = MultilayerPerceptron.thetas_roll(optimized_theta,self.layers)return self.thetas,cost_history
4、权重参数矩阵初始化
根据网络层次可以确定,矩阵的大小,用字典存储。
@staticmethoddef thetas_init(layers):num_layers = len(layers)thetas = {} #用字典形式 key:表示第几层 vlues:权重参数矩阵for layer_index in range(num_layers-1):'''会执行两次: 得到两组参数矩阵 25 * 785 , 10 * 26'''in_count = layers[layer_index]out_count = layers[layer_index+1]#初始化 初始值小#这里需要考虑偏置项,偏置的个数与输出的个数一样thetas[layer_index]=np.random.rand(out_count,in_count+1) * 0.05 #加一列输入特征return thetas
5、参数矩阵变换向量
将权重参数矩阵变换成向量
@staticmethoddef thetas_unroll(thetas):#拼接成一个向量num_theta_layers = len(thetas)unrolled_theta = np.array([])for theta_layer_index in range(num_theta_layers):unrolled_theta = np.hstack((unrolled_theta,thetas[theta_layer_index].flatten()))return unrolled_theta
6、向量变换权重参数矩阵
后边前向传播时需要进行矩阵乘法,需要变换回来
@staticmethoddef thetas_roll(unrolled_theta,layers):num_layers = len(layers)thetas = {}unrolled_shift = 0for layer_index in range(num_layers - 1):in_count = layers[layer_index]out_count = layers[layer_index + 1]thetas_width = in_count + 1thetas_height = out_countthetas_volume = thetas_width * thetas_heightstart_index = unrolled_shiftend_index =unrolled_shift + thetas_volumelayer_theta_unrolled = unrolled_theta[start_index:end_index]thetas[layer_index] = layer_theta_unrolled.reshape((thetas_height,thetas_width))unrolled_shift = unrolled_shift + thetas_volumereturn thetas
7、进行梯度下降
1. 损失函数,计算损失值
2. 计算梯度值
3. 更新参数
那么得先要实现损失函数,计算损失值。
7.1、损失函数
实现损失函数,得到损失值得要实现前向传播走一次
7.1.1、前向传播
@staticmethoddef feedforword_propagation(data,thetas,layers):num_layers = len(layers)num_examples = data.shape[0]in_layer_activation = data #输入层#逐层计算 隐藏层for layer_index in range(num_layers - 1):theta = thetas[layer_index]out_layer_activation = sigmoid(np.dot(in_layer_activation,theta.T)) #输出层# 正常计算之后是num_examples * 25 ,但是要考虑偏置项 变成num_examples * 26out_layer_activation = np.hstack((np.ones((num_examples,1)),out_layer_activation))in_layer_activation = out_layer_activation#返回输出层结果,不要偏置项return in_layer_activation[:,1:]
损失函数:
@staticmethoddef cost_function(data,labels,thetas,layers):num_layers = len(layers)num_examples = data.shape[0]num_labels = layers[-1]#前向传播走一次predictions = MultilayerPerceptron.feedforword_propagation(data,thetas,layers)#制作标签,每一个样本的标签都是one-dotbitwise_labels = np.zeros((num_examples,num_labels))for example_index in range(num_examples):bitwise_labels[example_index][labels[example_index][0]] = 1#咱们的预测值是概率值y= 7 [0,0,0,0,0,0,1,0,0,0] 在正确值的位置上概率越大越好 在错误值的位置上概率越小越好bit_set_cost = np.sum(np.log(predictions[bitwise_labels == 1]))bit_not_set_cost = np.sum(np.log(1 - predictions[bitwise_labels == 0]))cost = (-1/num_examples) * (bit_set_cost+bit_not_set_cost)return cost
7.2、反向传播
在梯度下降的过程中,要实现参数矩阵的更新,必须要实现反向传播。利用上述的公式,进行运算即可得到。
@staticmethoddef back_propagation(data,labels,thetas,layers):num_layers = len(layers)(num_examples,num_features) = data.shapenum_label_types = layers[-1]deltas = {} # 算出每一层对结果的影响#初始化for layer_index in range(num_layers - 1):in_count = layers[layer_index]out_count = layers[layer_index + 1]deltas[layer_index] = np.zeros((out_count,in_count+1)) #25 * 785 10 *26for example_index in range(num_examples):layers_inputs = {}layers_activations = {}layers_activation = data[example_index,:].reshape((num_features,1))layers_activations[0] = layers_activation#逐层计算for layer_index in range(num_layers - 1):layer_theta = thetas[layer_index] #得到当前的权重参数值 : 25 *785 10 *26layer_input = np.dot(layer_theta,layers_activation) # 第一次 得到 25 * 1 第二次: 10 * 1layers_activation = np.vstack((np.array([[1]]),sigmoid(layer_input))) #完成激活函数,加上一个偏置参数layers_inputs[layer_index+1] = layer_input # 后一层计算结果layers_activations[layer_index +1] = layers_activation # 后一层完成激活的结果output_layer_activation = layers_activation[1:,:]#计算输出层和结果的差异delta = {}#标签处理bitwise_label = np.zeros((num_label_types,1))bitwise_label[labels[example_index][0]] = 1#计算输出结果和真实值之间的差异delta[num_layers-1] = output_layer_activation - bitwise_label #输出层#遍历 L,L-1,L-2...2for layer_index in range(num_layers - 2,0,-1):layer_theta = thetas[layer_index]next_delta = delta[layer_index+1]layer_input = layers_inputs[layer_index]layer_input = np.vstack((np.array((1)),layer_input))#按照公式计算delta[layer_index] = np.dot(layer_theta.T,next_delta)*sigmoid(layer_input)#过滤掉偏置参数delta[layer_index] = delta[layer_index][1:,:]#计算梯度值for layer_index in range(num_layers-1):layer_delta = np.dot(delta[layer_index+1],layers_activations[layer_index].T) #微调矩阵deltas[layer_index] = deltas[layer_index] + layer_delta #第一次25 * 785 第二次 10 * 26for layer_index in range(num_layers-1):deltas[layer_index] = deltas[layer_index] * (1/num_examples) #公式return deltas
实现一次梯度下降:
@staticmethoddef gradient_step(data,labels,optimized_theta,layers):theta = MultilayerPerceptron.thetas_roll(optimized_theta,layers)#反向传播BPthetas_rolled_gradinets = MultilayerPerceptron.back_propagation(data,labels,theta,layers)thetas_unrolled_gradinets = MultilayerPerceptron.thetas_unroll(thetas_rolled_gradinets)return thetas_unrolled_gradinets
实现梯度下降:
@staticmethoddef gradient_descent(data,labels,unrolled_theta,layers,max_ietrations,alpha):#1. 计算损失值#2. 计算梯度值#3. 更新参数optimized_theta = unrolled_theta #最好的theta值cost_history = [] #损失值的记录for i in range(max_ietrations):if i % 10 == 0 :print("当前迭代次数:",i)cost = MultilayerPerceptron.cost_function(data,labels,MultilayerPerceptron.thetas_roll(optimized_theta,layers),layers)cost_history.append(cost)theta_gradient = MultilayerPerceptron.gradient_step(data,labels,optimized_theta,layers)optimized_theta = optimized_theta - alpha * theta_gradientreturn optimized_theta,cost_history
8、预测函数
输入测试数据,前向传播走一次,得到预测值
def predict(self,data):data_processed = prepare_for_training(data,normalize_data = self.normalize_data)[0]num_examples = data_processed.shape[0]predictions = MultilayerPerceptron.feedforword_propagation(data_processed,self.thetas,self.layers)return np.argmax(predictions,axis=1).reshape((num_examples,1))
四、完整代码
import numpy as np
from Neural_Network_Lab.utils.features import prepare_for_training
from Neural_Network_Lab.utils.hypothesis import sigmoid,sigmoid_gradientclass MultilayerPerceptron:def __init__(self,data,labels,layers,normalize_data=False):data_processed = prepare_for_training(data,normalize_data=normalize_data)[0]self.data = data_processedself.labels = labelsself.layers = layers # [ 784 ,25 ,10]self.normalize_data = normalize_dataself.thetas = MultilayerPerceptron.thetas_init(layers)def predict(self,data):data_processed = prepare_for_training(data,normalize_data = self.normalize_data)[0]num_examples = data_processed.shape[0]predictions = MultilayerPerceptron.feedforword_propagation(data_processed,self.thetas,self.layers)return np.argmax(predictions,axis=1).reshape((num_examples,1))def train(self,max_ietrations = 1000,alpha = 0.1):#方便矩阵更新 拉长 把矩阵拉成向量unrolled_theta = MultilayerPerceptron.thetas_unroll(self.thetas)(optimized_theta, cost_history) = MultilayerPerceptron.gradient_descent(self.data,self.labels,unrolled_theta,self.layers,max_ietrations,alpha)self.thetas = MultilayerPerceptron.thetas_roll(optimized_theta,self.layers)return self.thetas,cost_history@staticmethoddef gradient_descent(data,labels,unrolled_theta,layers,max_ietrations,alpha):#1. 计算损失值#2. 计算梯度值#3. 更新参数optimized_theta = unrolled_theta #最好的theta值cost_history = [] #损失值的记录for i in range(max_ietrations):if i % 10 == 0 :print("当前迭代次数:",i)cost = MultilayerPerceptron.cost_function(data,labels,MultilayerPerceptron.thetas_roll(optimized_theta,layers),layers)cost_history.append(cost)theta_gradient = MultilayerPerceptron.gradient_step(data,labels,optimized_theta,layers)optimized_theta = optimized_theta - alpha * theta_gradientreturn optimized_theta,cost_history@staticmethoddef gradient_step(data,labels,optimized_theta,layers):theta = MultilayerPerceptron.thetas_roll(optimized_theta,layers)#反向传播BPthetas_rolled_gradinets = MultilayerPerceptron.back_propagation(data,labels,theta,layers)thetas_unrolled_gradinets = MultilayerPerceptron.thetas_unroll(thetas_rolled_gradinets)return thetas_unrolled_gradinets@staticmethoddef back_propagation(data,labels,thetas,layers):num_layers = len(layers)(num_examples,num_features) = data.shapenum_label_types = layers[-1]deltas = {} # 算出每一层对结果的影响#初始化for layer_index in range(num_layers - 1):in_count = layers[layer_index]out_count = layers[layer_index + 1]deltas[layer_index] = np.zeros((out_count,in_count+1)) #25 * 785 10 *26for example_index in range(num_examples):layers_inputs = {}layers_activations = {}layers_activation = data[example_index,:].reshape((num_features,1))layers_activations[0] = layers_activation#逐层计算for layer_index in range(num_layers - 1):layer_theta = thetas[layer_index] #得到当前的权重参数值 : 25 *785 10 *26layer_input = np.dot(layer_theta,layers_activation) # 第一次 得到 25 * 1 第二次: 10 * 1layers_activation = np.vstack((np.array([[1]]),sigmoid(layer_input))) #完成激活函数,加上一个偏置参数layers_inputs[layer_index+1] = layer_input # 后一层计算结果layers_activations[layer_index +1] = layers_activation # 后一层完成激活的结果output_layer_activation = layers_activation[1:,:]#计算输出层和结果的差异delta = {}#标签处理bitwise_label = np.zeros((num_label_types,1))bitwise_label[labels[example_index][0]] = 1#计算输出结果和真实值之间的差异delta[num_layers-1] = output_layer_activation - bitwise_label #输出层#遍历 L,L-1,L-2...2for layer_index in range(num_layers - 2,0,-1):layer_theta = thetas[layer_index]next_delta = delta[layer_index+1]layer_input = layers_inputs[layer_index]layer_input = np.vstack((np.array((1)),layer_input))#按照公式计算delta[layer_index] = np.dot(layer_theta.T,next_delta)*sigmoid(layer_input)#过滤掉偏置参数delta[layer_index] = delta[layer_index][1:,:]#计算梯度值for layer_index in range(num_layers-1):layer_delta = np.dot(delta[layer_index+1],layers_activations[layer_index].T) #微调矩阵deltas[layer_index] = deltas[layer_index] + layer_delta #第一次25 * 785 第二次 10 * 26for layer_index in range(num_layers-1):deltas[layer_index] = deltas[layer_index] * (1/num_examples)return deltas@staticmethoddef cost_function(data,labels,thetas,layers):num_layers = len(layers)num_examples = data.shape[0]num_labels = layers[-1]#前向传播走一次predictions = MultilayerPerceptron.feedforword_propagation(data,thetas,layers)#制作标签,每一个样本的标签都是one-dotbitwise_labels = np.zeros((num_examples,num_labels))for example_index in range(num_examples):bitwise_labels[example_index][labels[example_index][0]] = 1#咱们的预测值是概率值y= 7 [0,0,0,0,0,0,1,0,0,0] 在正确值的位置上概率越大越好 在错误值的位置上概率越小越好bit_set_cost = np.sum(np.log(predictions[bitwise_labels == 1]))bit_not_set_cost = np.sum(np.log(1 - predictions[bitwise_labels == 0]))cost = (-1/num_examples) * (bit_set_cost+bit_not_set_cost)return cost@staticmethoddef feedforword_propagation(data,thetas,layers):num_layers = len(layers)num_examples = data.shape[0]in_layer_activation = data #输入层#逐层计算 隐藏层for layer_index in range(num_layers - 1):theta = thetas[layer_index]out_layer_activation = sigmoid(np.dot(in_layer_activation,theta.T)) #输出层# 正常计算之后是num_examples * 25 ,但是要考虑偏置项 变成num_examples * 26out_layer_activation = np.hstack((np.ones((num_examples,1)),out_layer_activation))in_layer_activation = out_layer_activation#返回输出层结果,不要偏置项return in_layer_activation[:,1:]@staticmethoddef thetas_roll(unrolled_theta,layers):num_layers = len(layers)thetas = {}unrolled_shift = 0for layer_index in range(num_layers - 1):in_count = layers[layer_index]out_count = layers[layer_index + 1]thetas_width = in_count + 1thetas_height = out_countthetas_volume = thetas_width * thetas_heightstart_index = unrolled_shiftend_index =unrolled_shift + thetas_volumelayer_theta_unrolled = unrolled_theta[start_index:end_index]thetas[layer_index] = layer_theta_unrolled.reshape((thetas_height,thetas_width))unrolled_shift = unrolled_shift + thetas_volumereturn thetas@staticmethoddef thetas_unroll(thetas):#拼接成一个向量num_theta_layers = len(thetas)unrolled_theta = np.array([])for theta_layer_index in range(num_theta_layers):unrolled_theta = np.hstack((unrolled_theta,thetas[theta_layer_index].flatten()))return unrolled_theta@staticmethoddef thetas_init(layers):num_layers = len(layers)thetas = {} #用字典形式 key:表示第几层 vlues:权重参数矩阵for layer_index in range(num_layers-1):'''会执行两次: 得到两组参数矩阵 25 * 785 , 10 * 26'''in_count = layers[layer_index]out_count = layers[layer_index+1]#初始化 初始值小#这里需要考虑偏置项,偏置的个数与输出的个数一样thetas[layer_index]=np.random.rand(out_count,in_count+1) * 0.05 #加一列输入特征return thetas
五、手写数字识别
数据集(读者可以找找下载,我就不放链接了>_<):
共一万个样本,第一列为标签值,一列表示像素点的值共28*28共784个像素点。
import numpy as np
import pandas as pd
import matplotlib.pyplot as plt
import matplotlib.image as mping
import math
from Neural_Network_Lab.Multilayer_Perceptron import MultilayerPerceptrondata = pd.read_csv('../Neural_Network_Lab/data/mnist-demo.csv')
#展示数据
numbers_to_display = 25
num_cells = math.ceil(math.sqrt(numbers_to_display))
plt.figure(figsize=(10,10))
for plot_index in range(numbers_to_display):digit = data[plot_index:plot_index+1].valuesdigit_label = digit[0][0]digit_pixels = digit[0][1:]image_size = int(math.sqrt(digit_pixels.shape[0]))frame = digit_pixels.reshape((image_size,image_size))plt.subplot(num_cells,num_cells,plot_index+1)plt.imshow(frame,cmap = 'Greys')plt.title(digit_label)
plt.subplots_adjust(wspace=0.5,hspace=0.5)
plt.show()train_data = data.sample(frac= 0.8)
test_data = data.drop(train_data.index)train_data = train_data.values
test_data = test_data.valuesnum_training_examples = 8000X_train = train_data[:num_training_examples,1:]
y_train = train_data[:num_training_examples,[0]]X_test = test_data[:,1:]
y_test = test_data[:,[0]]layers = [784,25,10]
normalize_data = True
max_iteration = 500
alpha = 0.1multilayerperceptron = MultilayerPerceptron(X_train,y_train,layers,normalize_data)
(thetas,cost_history) = multilayerperceptron.train(max_iteration,alpha)
plt.plot(range(len(cost_history)),cost_history)
plt.xlabel('Grident steps')
plt.ylabel('cost')
plt.show()y_train_predictions = multilayerperceptron.predict(X_train)
y_test_predictions = multilayerperceptron.predict(X_test)train_p = np.sum((y_train_predictions == y_train) / y_train.shape[0] * 100)
test_p = np.sum((y_test_predictions == y_test) / y_test.shape[0] * 100)print("训练集准确率:",train_p)
print("测试集准确率:",test_p)numbers_to_display = 64
num_cells = math.ceil(math.sqrt(numbers_to_display))
plt.figure(figsize=(15,15))
for plot_index in range(numbers_to_display):digit_label = y_test[plot_index,0]digit_pixels = X_test[plot_index,:]predicted_label = y_test_predictions[plot_index][0]image_size = int(math.sqrt(digit_pixels.shape[0]))frame = digit_pixels.reshape((image_size,image_size))plt.subplot(num_cells,num_cells,plot_index+1)color_map = 'Greens' if predicted_label == digit_label else 'Reds'plt.imshow(frame,cmap = color_map)plt.title(predicted_label)plt.tick_params(axis='both',which='both',bottom=False,left=False,labelbottom=False)plt.subplots_adjust(wspace=0.5,hspace=0.5)
plt.show()
训练集8000个,测试集2000个,迭代次数500次
这里准确率不高,读者可以自行调整参数,改变迭代次数,网络层次都可以哦。
相关文章:
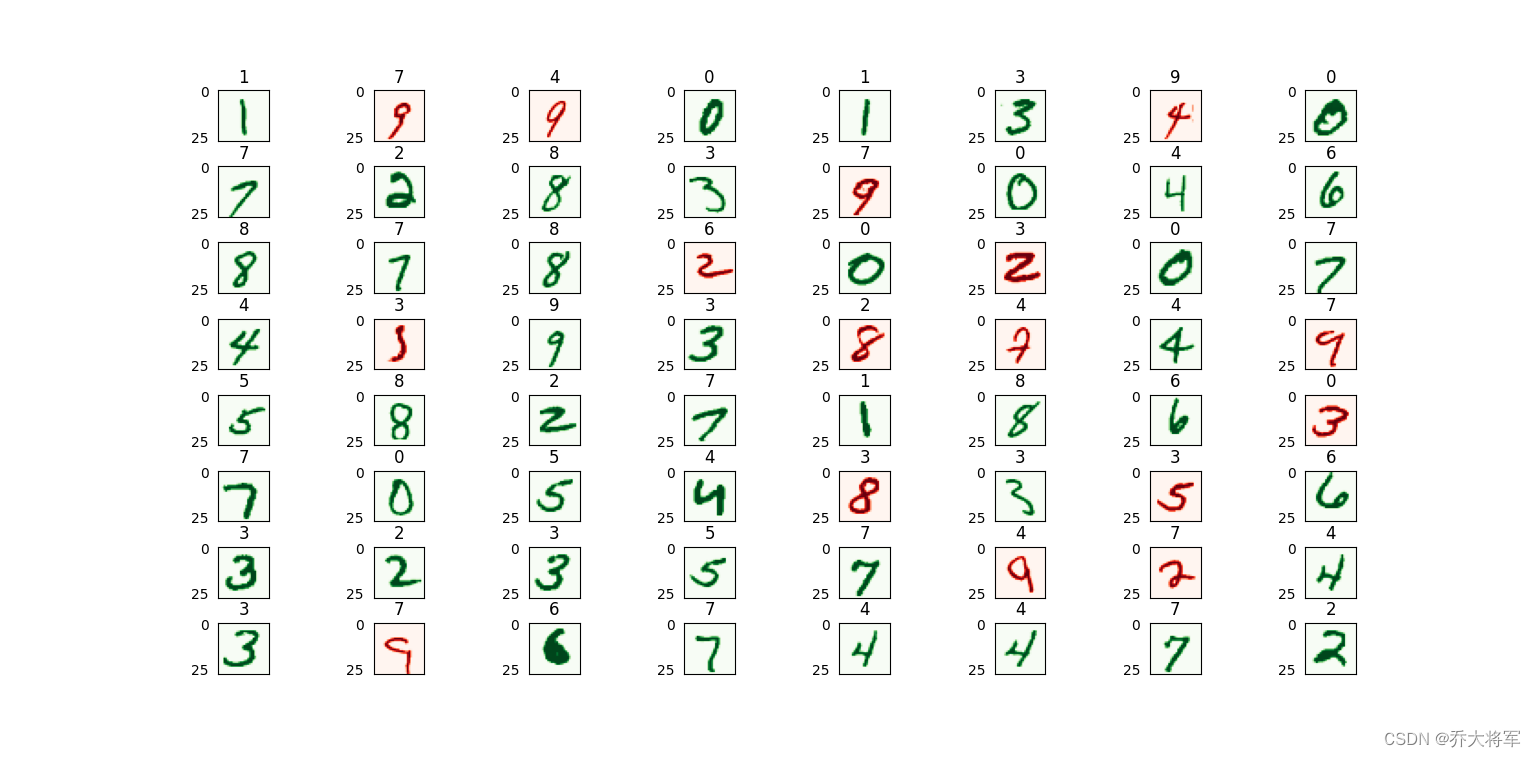
神经网络代码实现(用手写数字识别数据集实验)
目录 一、前言 二、神经网络架构 三、算法实现 1、导入包 2、实现类 3、训练函数 4、权重参数矩阵初始化 5、参数矩阵变换向量 6、向量变换权重参数矩阵 7、进行梯度下降 7.1、损失函数 7.1.1、前向传播 7.2、反向传播 8、预测函数 四、完整代码 五、手写数字识别 一、前言 …...
菜鸟笔记-Python函数-linspace
linspace 是 NumPy 库中的一个函数,用于生成具有指定数量的等间距样本的数组。它的名字来源于“linear space”(线性空间),因为它在指定的范围内均匀地生成数值。 linspace 函数的基本语法如下: numpy.linspace(star…...
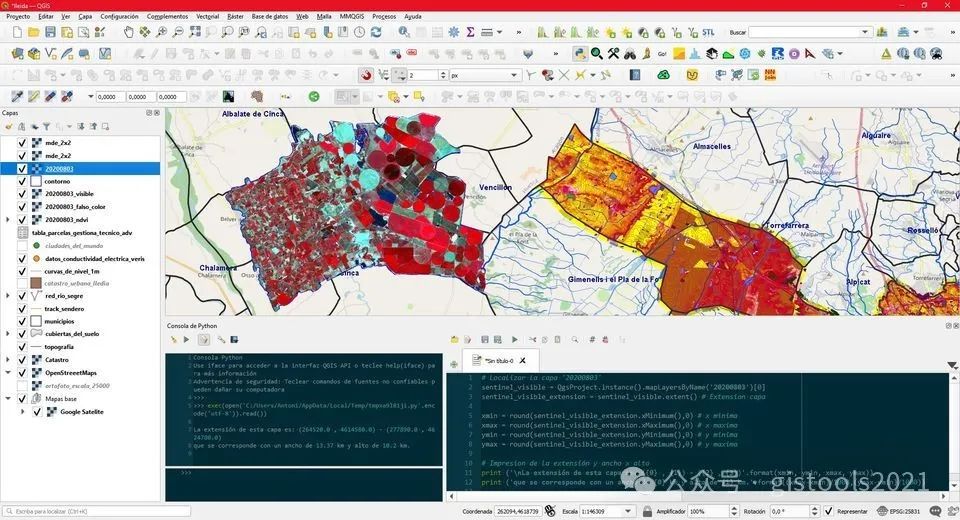
为什么我们应该使用QGIS
QGIS地理信息系统是免费的开源软件,已成为创建地图和分析空间数据的强大工具。在本文中,我们将探讨 QGIS 为地图公司带来的诸多好处,以及为什么使用它可以促进您的业务成功。使用QGIS的好处: 1. 免费开源软件,但从长远…...
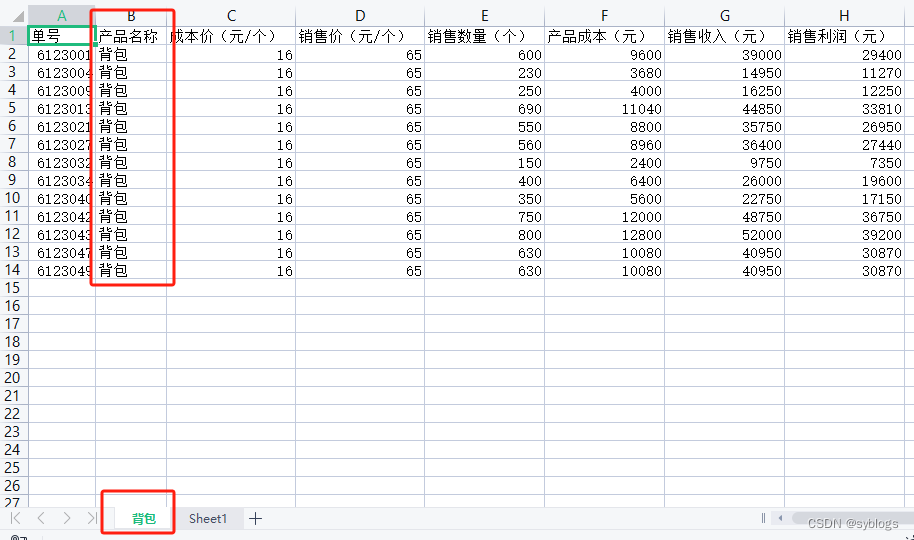
用Python实现办公自动化(自动化处理Excel工作簿)
自动化处理Excel工作簿 (一)批量生产产品出货清单 以“出货统计表”为例, 需求:将出货记录按照出货日期分类整理成多张出货清单 “出货统计表数据案例” “产品出货清单模板” 1.提取出货统计表的数据 “Python程序代码” # 使用…...
BaseDao入门使用
目录 一、什么是BaseDao?BaseDao的优点:BaseDao用来做什么操作? 二、BaseDao封装增删改查 案例演示:1、java与数据库进行连接2、连接后可对其进行操作(增、删、改)返回影响行数3、查询 查询一个字段(返回一…...
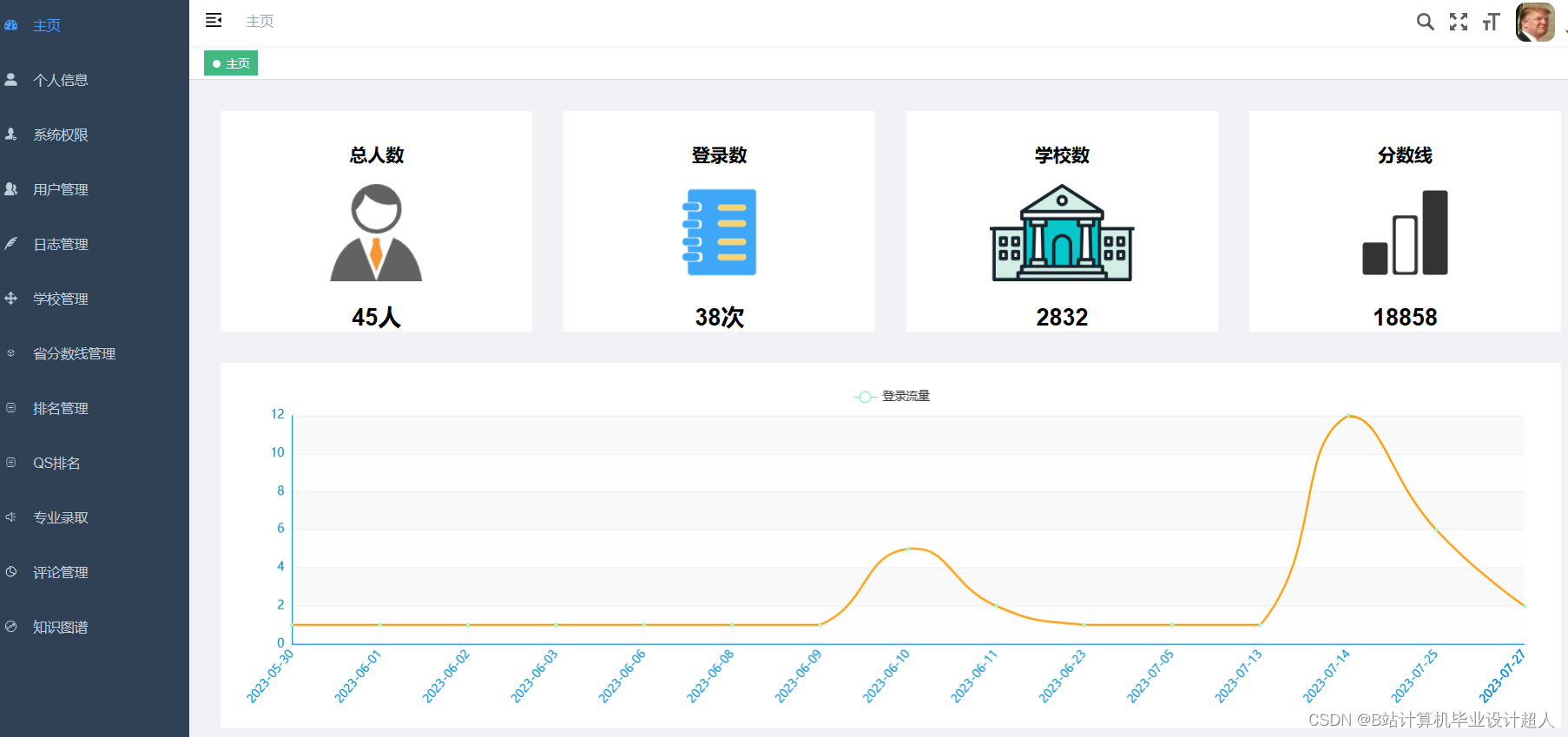
计算机毕业设计Python+Spark知识图谱高考志愿推荐系统 高考数据分析 高考可视化 高考大数据 大数据毕业设计 机器学习 深度学习 人工智能
学院(全称): 专业(全称): 姓名 学号 年级 班级 设计(论文) 题目 基于Spark的高考志愿推荐系统设计与实现 指导教师姓名 职称 拟…...
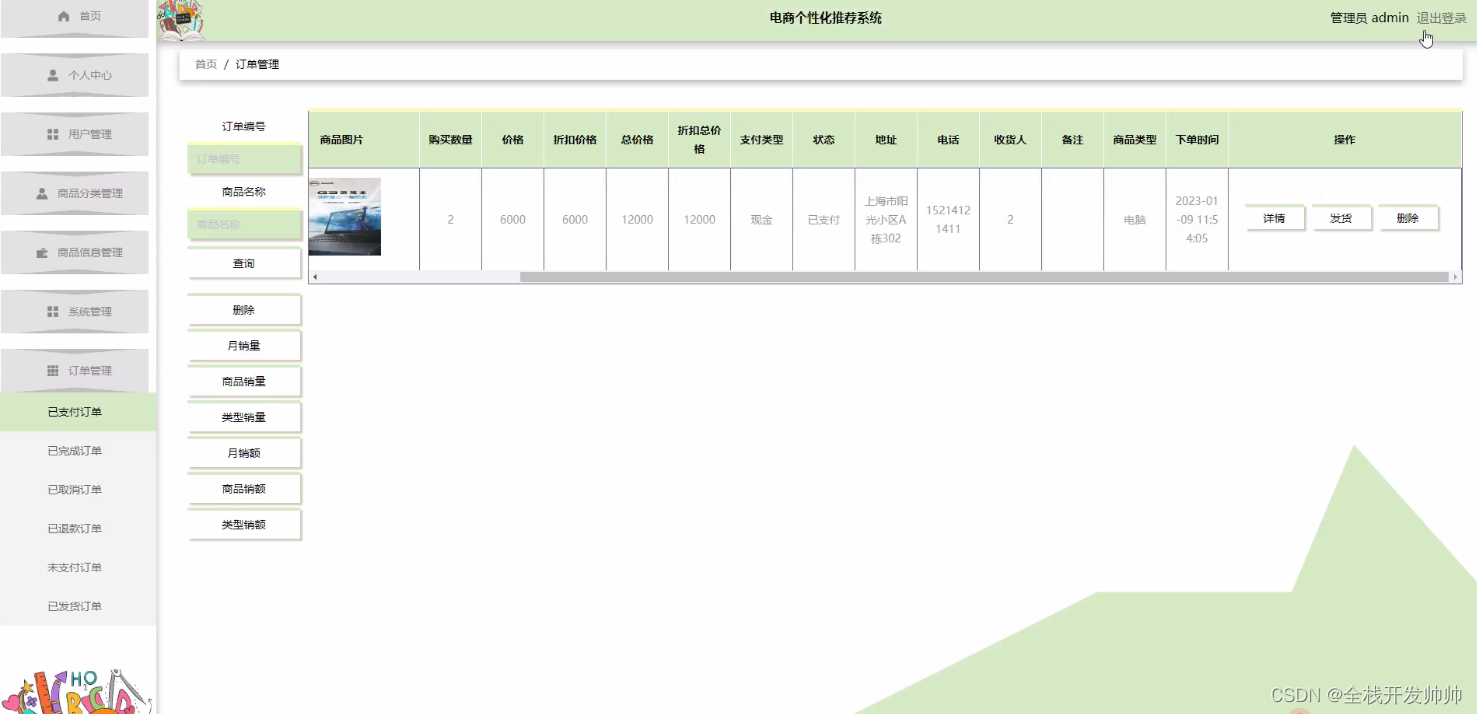
基于java+springboot+vue实现的电商个性化推荐系统(文末源码+Lw+ppt)23-389
摘 要 伴随着我国社会的发展,人民生活质量日益提高。于是对电商个性化推荐进行规范而严格是十分有必要的,所以许许多多的信息管理系统应运而生。此时单靠人力应对这些事务就显得有些力不从心了。所以本论文将设计一套电商个性化推荐系统,帮…...
论文阅读,The Lattice Boltzmann Method: Principles and Practice(六)(1)
目录 一、流体模拟方法概述 二、传统的Navier-Stokes求解器 2.1 有限差分 2.2 有限体积法 2.3 有限元法 三、基于粒子的求解器 3.1 动力学理论 3.2 分子动力学 3.3 格子气体模型 3.4 耗散粒子动力学 3.5 多粒子碰撞动力学 3.6 直接模拟蒙特卡罗方法 3.7 平滑粒子流…...
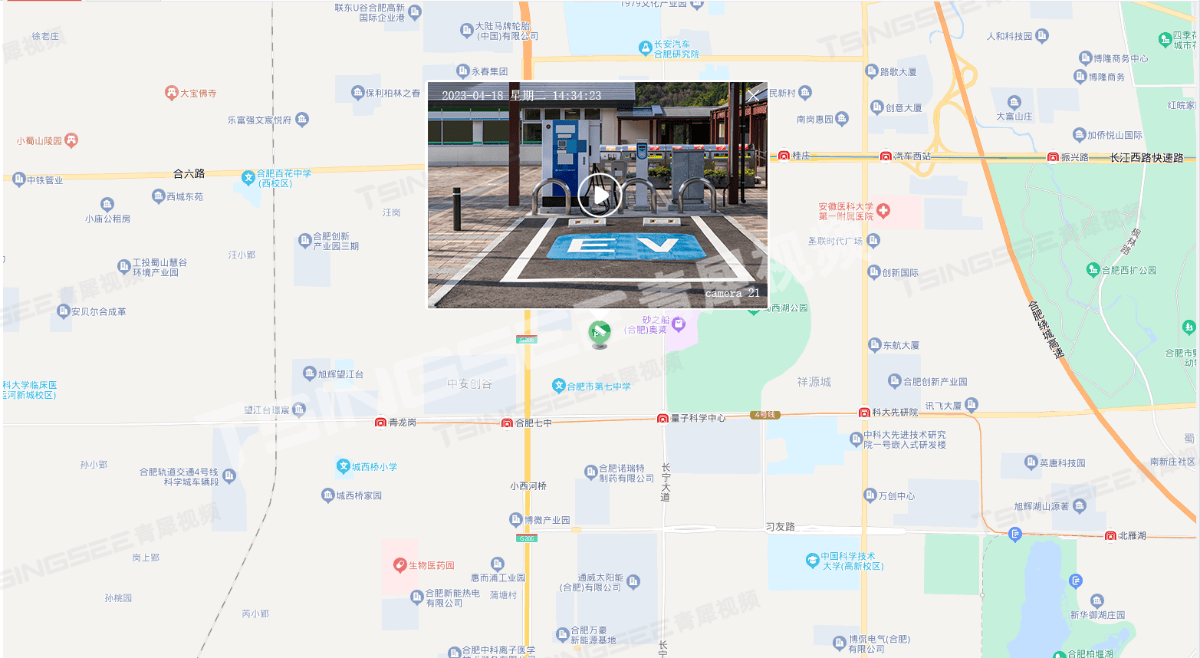
新能源充电桩站场视频汇聚系统建设方案及技术特点分析
随着新能源汽车的普及,充电桩作为新能源汽车的基础设施,其安全性和可靠性越来越受到人们的关注。为了更好地保障充电桩的安全运行与站场管理,TSINGSEE青犀&触角云推出了一套新能源汽车充电桩视频汇聚管理与视频监控方案。 方案采用高清摄…...
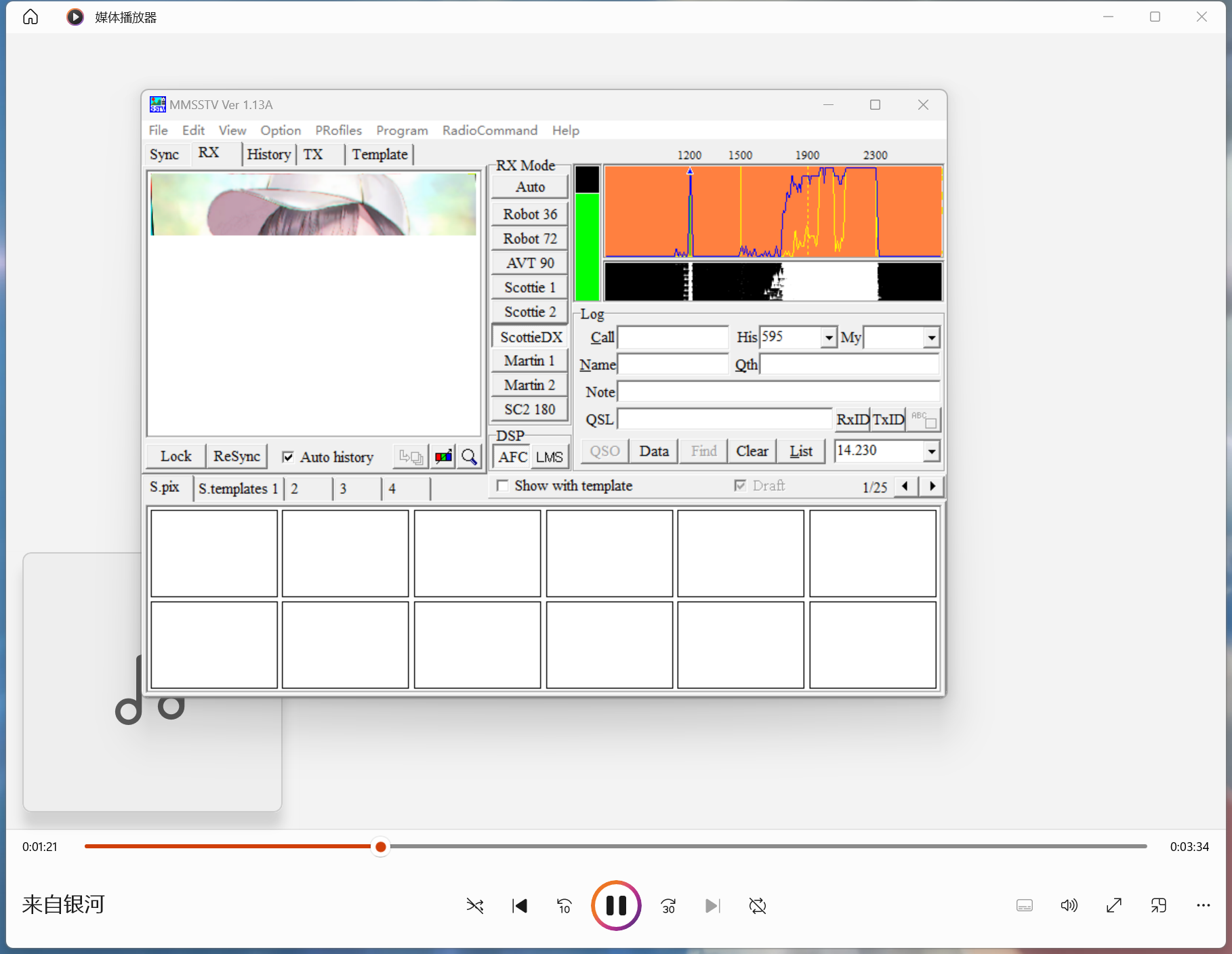
三、音频隐写[Audacity、deepsound、dtmf2num、MMSSTV、虚拟声卡、MP3Stego]
工具 1.Audacity 下载:https://www.audacityteam.org/download/windows/ 使用: 删除:先用左键长按拖着选中内容,然后选择软件最上方菜单栏的编辑,然后选择“删除”,最后点击文件的导出音频就能成功导出…...
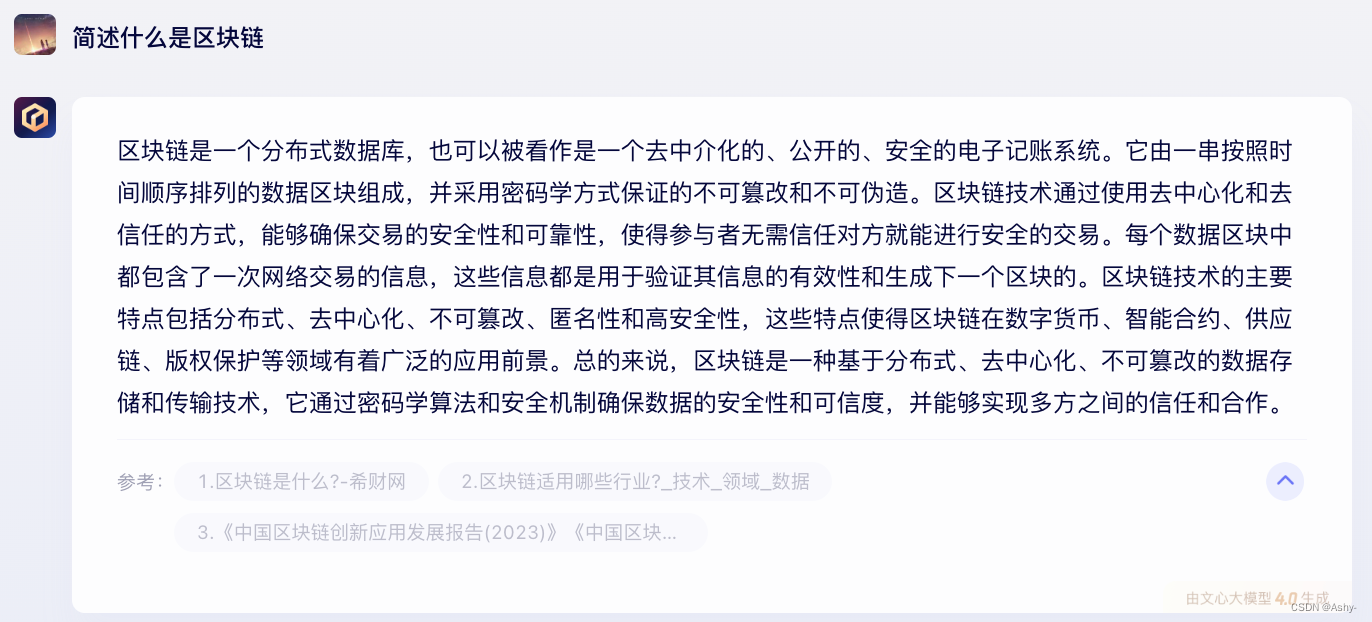
二、Web3 学习(区块链)
区块链基础知识 一、基础知识1. 区块链可以做什么?2. 区块链的三个特点 二、区块链的类型概括1. PoW2. PoS3. 私有链和联盟链 三、智能合约1. 什么是智能合约2. 如何使用智能合约 四、困境1. 三难选择的基本要素2. 这真的是一个三难选择吗? 五、比特币1. 什么是比特…...
Linux内网提权
一、SUID提权 前提条件: (1)SUID仅对二进制有效(2)执行者对于该程序需要有x的可执行权限(3)本权限仅在程序的执行过程中有效 1、设置SUID权限:(root权限) …...
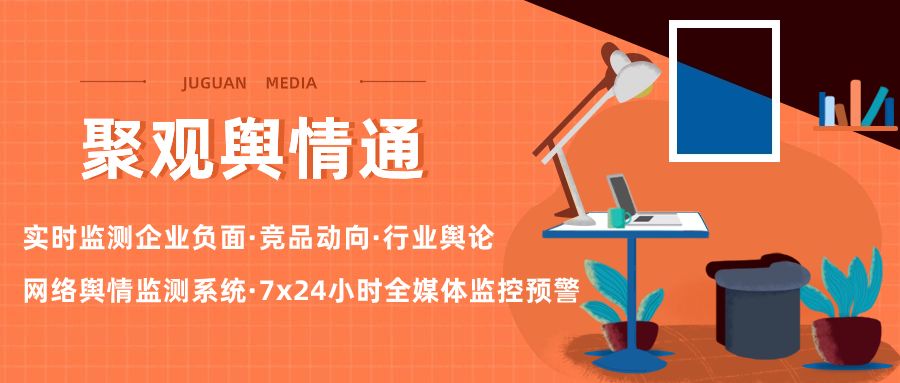
聚观早报 | 抖音独立商城App上线;阿里云联发科合作
聚观早报每日整理最值得关注的行业重点事件,帮助大家及时了解最新行业动态,每日读报,就读聚观365资讯简报。 整理丨Cutie 3月29日消息 抖音独立商城App上线 阿里云联发科合作 苹果WWDC24官宣 恒大汽车2023年营收财报 亚马逊投资Anthro…...

第十四届蓝桥杯省赛C++ B组所有题目以及题解(C++)【编程题均通过100%测试数据】
第一题《日期统计》【枚举】 【问题描述】 小蓝现在有一个长度为100的数组,数组中的每个元素的值都在0到9的范围之内。数组中的元素从左至右如下所示: 5 6 8 6 9 1 6 1 2 4 9 1 9 8 2 3 6 4 7 7 5 9 5 0 3 8 7 5 8 1 5 8 6 1 8 3 0 3 7 9 2 7 0 5 8 8 …...
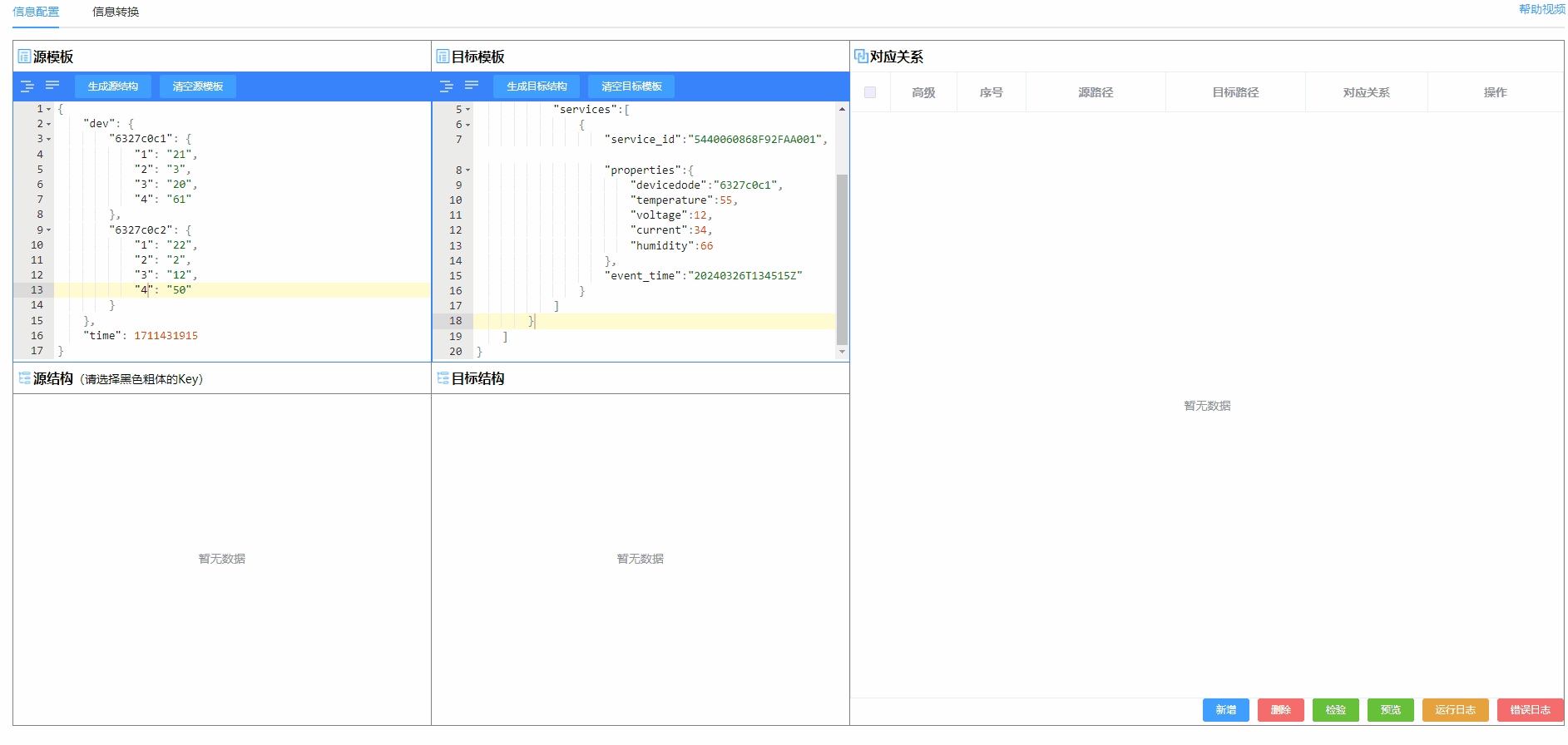
【御控物联】 IOT异构数据JSON转化(场景案例一)
文章目录 前言技术资料 前言 随着物联网、大数据、智能制造技术的不断发展,越来越多的企业正在进行工厂的智能化转型升级。转型升级第一步往往是设备的智能化改造,助力设备数据快速上云,实现设备数据共享和场景互联。然而,在生产…...
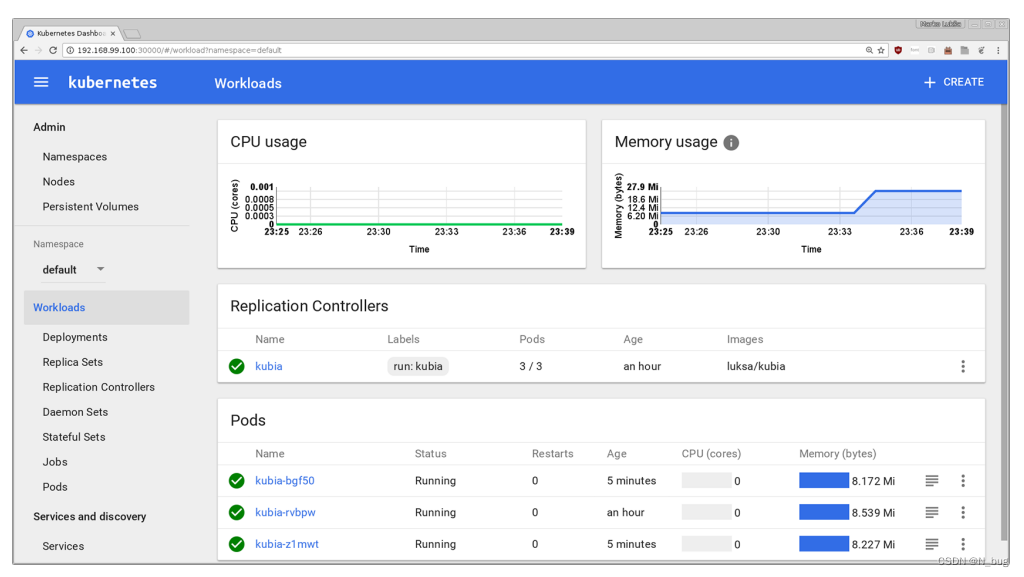
Kubernetes-running app on kube
Docker 安装Docker 首先,您需要在Linux机器上安装Docker。如果您不使用Linux,则需要启动一个Linux虚拟机(VM)并在该虚拟机中运行Docker。如果你使用的是Mac或Windows系统,并按照指令安装Docker, Docker将为你建立一个虚拟机,并在…...
简述如何系统地学习Python
随着人工智能、大数据和云计算等技术的快速发展,编程已经成为了当今社会中不可或缺的一项技能。Python作为一种高级编程语言,因其简洁明了的语法、强大的功能和广泛的应用领域,成为了许多初学者和专业人士的首选。那么,如何系统地…...
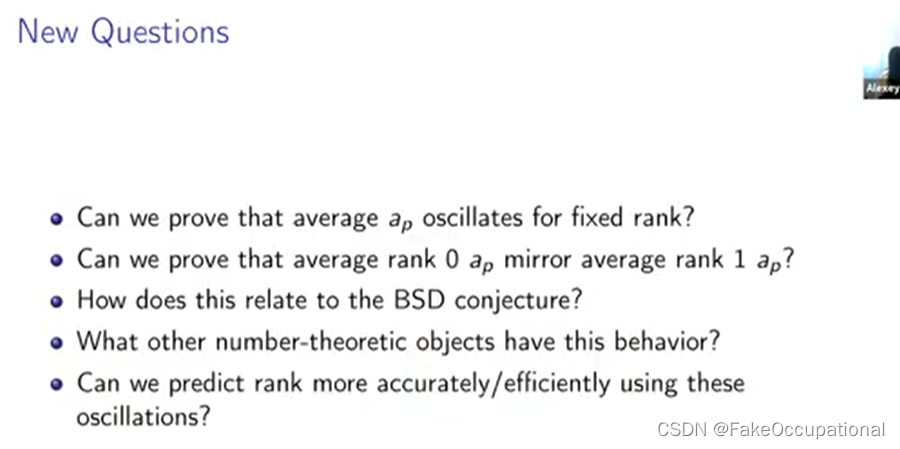
bsd猜想 Murmuration of Eliptic Curves(笔记)
BSD Alexey Pozdnyakov (University of Connecticut) YUTUBE视频, B站搬运地址新生代女数学家Nina Zubrilina得到椭圆曲线椋鸟群飞模式精确公式与证明 Arithmetic Geometry算术几何 希尔伯特第十问题 希尔伯特第十问题(Hilbert’s Tenth Problem&#…...

小米汽车正式发布:开启智能电动新篇章
随着科技的不断进步,汽车产业正经历着前所未有的变革。智能电动汽车作为这一变革的重要方向,正吸引着越来越多的目光。在这个充满机遇和挑战的时代,小米汽车凭借其卓越的技术实力和深厚的市场底蕴,终于迈出了坚实的一步。今天&…...
线性代数笔记25--复数矩阵、快速傅里叶变换
1. 复数矩阵 复向量 Z [ z 1 z 2 z 3 z 4 ⋯ ] Z\begin{bmatrix} z_1\\z_2\\z_3\\z_4\\ \cdots \end{bmatrix} Z z1z2z3z4⋯ 复向量的模长 ∣ z ∣ z ‾ ⊤ z [ z ‾ 1 z ‾ 2 z ‾ 3 ] [ z 1 z 2 z 3 ] \lvert z\rvert\overline z^{\top}z \begin{bmatrix…...
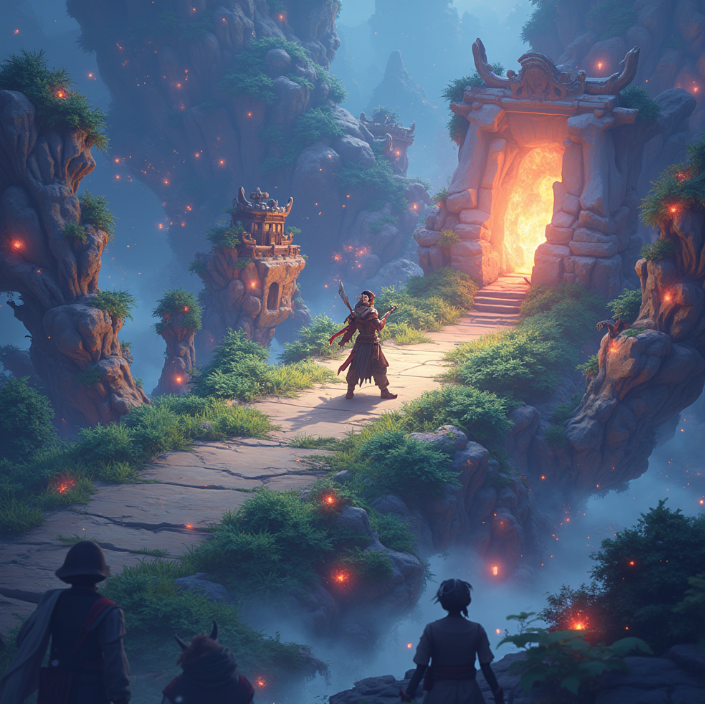
手游刚开服就被攻击怎么办?如何防御DDoS?
开服初期是手游最脆弱的阶段,极易成为DDoS攻击的目标。一旦遭遇攻击,可能导致服务器瘫痪、玩家流失,甚至造成巨大经济损失。本文为开发者提供一套简洁有效的应急与防御方案,帮助快速应对并构建长期防护体系。 一、遭遇攻击的紧急应…...
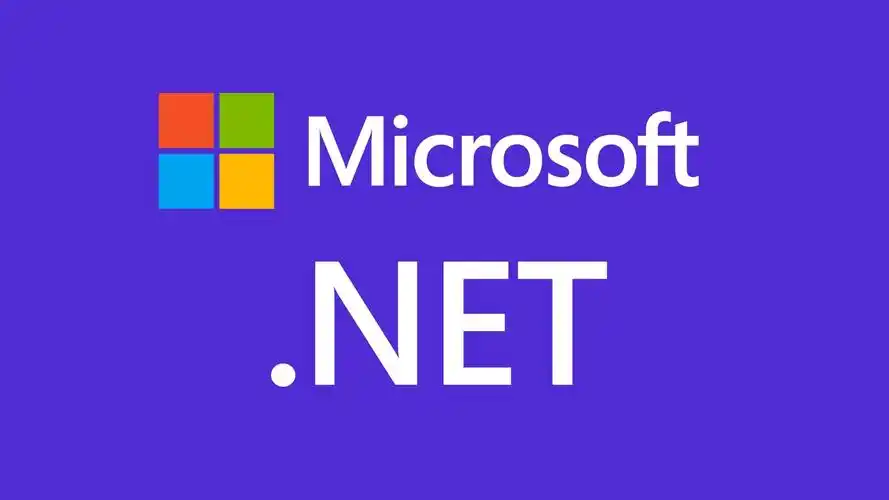
.Net框架,除了EF还有很多很多......
文章目录 1. 引言2. Dapper2.1 概述与设计原理2.2 核心功能与代码示例基本查询多映射查询存储过程调用 2.3 性能优化原理2.4 适用场景 3. NHibernate3.1 概述与架构设计3.2 映射配置示例Fluent映射XML映射 3.3 查询示例HQL查询Criteria APILINQ提供程序 3.4 高级特性3.5 适用场…...
Neo4j 集群管理:原理、技术与最佳实践深度解析
Neo4j 的集群技术是其企业级高可用性、可扩展性和容错能力的核心。通过深入分析官方文档,本文将系统阐述其集群管理的核心原理、关键技术、实用技巧和行业最佳实践。 Neo4j 的 Causal Clustering 架构提供了一个强大而灵活的基石,用于构建高可用、可扩展且一致的图数据库服务…...
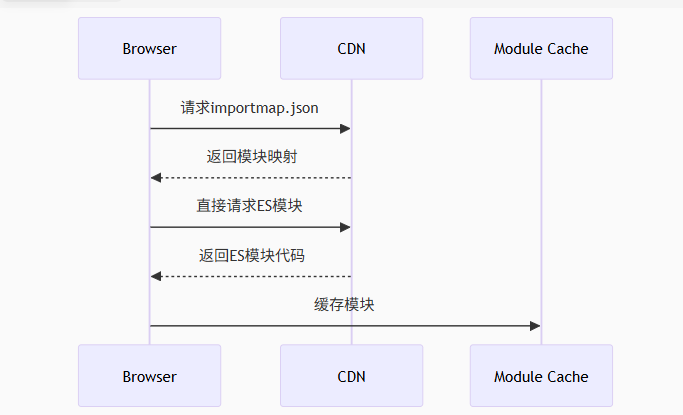
Module Federation 和 Native Federation 的比较
前言 Module Federation 是 Webpack 5 引入的微前端架构方案,允许不同独立构建的应用在运行时动态共享模块。 Native Federation 是 Angular 官方基于 Module Federation 理念实现的专为 Angular 优化的微前端方案。 概念解析 Module Federation (模块联邦) Modul…...
Unit 1 深度强化学习简介
Deep RL Course ——Unit 1 Introduction 从理论和实践层面深入学习深度强化学习。学会使用知名的深度强化学习库,例如 Stable Baselines3、RL Baselines3 Zoo、Sample Factory 和 CleanRL。在独特的环境中训练智能体,比如 SnowballFight、Huggy the Do…...
高防服务器能够抵御哪些网络攻击呢?
高防服务器作为一种有着高度防御能力的服务器,可以帮助网站应对分布式拒绝服务攻击,有效识别和清理一些恶意的网络流量,为用户提供安全且稳定的网络环境,那么,高防服务器一般都可以抵御哪些网络攻击呢?下面…...
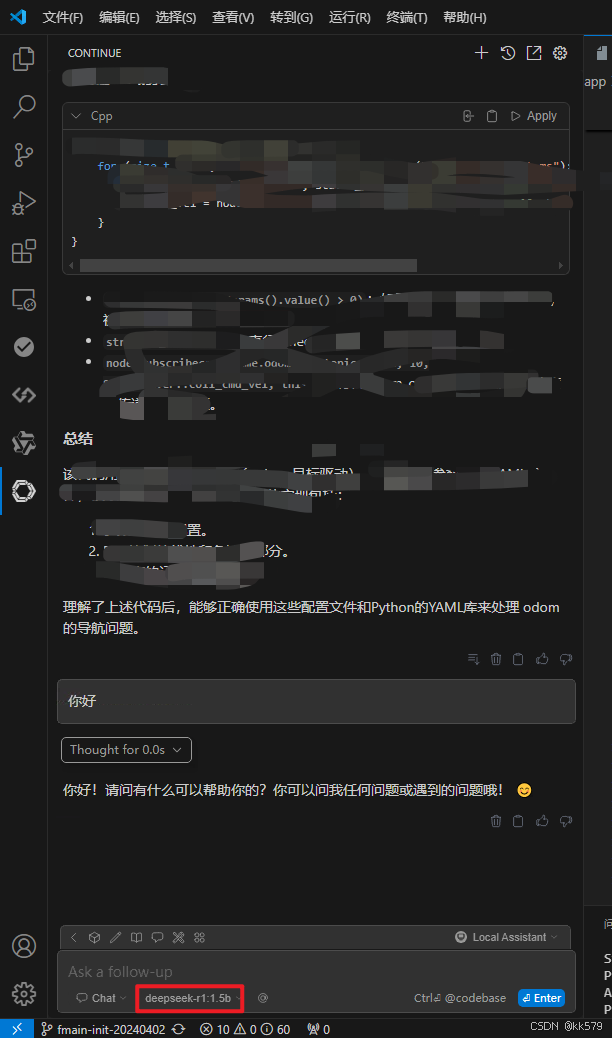
保姆级教程:在无网络无显卡的Windows电脑的vscode本地部署deepseek
文章目录 1 前言2 部署流程2.1 准备工作2.2 Ollama2.2.1 使用有网络的电脑下载Ollama2.2.2 安装Ollama(有网络的电脑)2.2.3 安装Ollama(无网络的电脑)2.2.4 安装验证2.2.5 修改大模型安装位置2.2.6 下载Deepseek模型 2.3 将deepse…...

视觉slam十四讲实践部分记录——ch2、ch3
ch2 一、使用g++编译.cpp为可执行文件并运行(P30) g++ helloSLAM.cpp ./a.out运行 二、使用cmake编译 mkdir build cd build cmake .. makeCMakeCache.txt 文件仍然指向旧的目录。这表明在源代码目录中可能还存在旧的 CMakeCache.txt 文件,或者在构建过程中仍然引用了旧的路…...
C++课设:简易日历程序(支持传统节假日 + 二十四节气 + 个人纪念日管理)
名人说:路漫漫其修远兮,吾将上下而求索。—— 屈原《离骚》 创作者:Code_流苏(CSDN)(一个喜欢古诗词和编程的Coder😊) 专栏介绍:《编程项目实战》 目录 一、为什么要开发一个日历程序?1. 深入理解时间算法2. 练习面向对象设计3. 学习数据结构应用二、核心算法深度解析…...
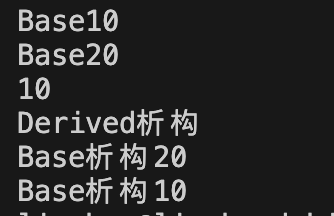
c++第七天 继承与派生2
这一篇文章主要内容是 派生类构造函数与析构函数 在派生类中重写基类成员 以及多继承 第一部分:派生类构造函数与析构函数 当创建一个派生类对象时,基类成员是如何初始化的? 1.当派生类对象创建的时候,基类成员的初始化顺序 …...