计算方法实验9:Romberg积分求解速度、位移
任务
- 输出质点的轨迹 ( x ( t ) , y ( t ) ) , t ∈ { 0.1 , 0.2 , 0.3 , . . . , 10 } (x(t), y(t)), t\in \{0.1, 0.2, 0.3, ..., 10\} (x(t),y(t)),t∈{0.1,0.2,0.3,...,10},并在二维平面中画出该轨迹.
- 请比较M分别取4, 8, 12, 16, 20 时,Romberg积分达到要求精度的比例(达到误差要求的次数/调用总次数),分析该比例随M 的变化。
算法
现在要用数值方法求 ∫ a b f ( x ) d x \int_{a}^{b} f(x) \, dx ∫abf(x)dx,设 h = b − a n h=\frac{b-a}{n} h=nb−a,已知:
复化梯形积分 T n ( f ) = h [ 1 2 f ( a ) + ∑ i = 1 n − 1 f ( a + i h ) + 1 2 f ( b ) ] T_{n}\left(f\right)=h\left[\frac{1}{2}f\left(a\right)+\sum_{i=1}^{n-1}f\left(a+ih\right)+\frac{1}{2}f\left(b\right)\right] Tn(f)=h[21f(a)+∑i=1n−1f(a+ih)+21f(b)]、
复化Simpson积分 S n ( f ) = h 3 [ f ( a ) + 4 ∑ i = 0 m − 1 f ( x 2 i + 1 ) + 2 ∑ i = 1 m − 1 f ( x 2 i ) + f ( b ) ] S_{n}\left(f\right)=\frac{h}{3}\left[f\left(a\right)+4\sum_{i=0}^{m-1}f\left(x_{2i+1}\right)+2\sum_{i=1}^{m-1}f\left(x_{2i}\right)+f\left(b\right)\right] Sn(f)=3h[f(a)+4∑i=0m−1f(x2i+1)+2∑i=1m−1f(x2i)+f(b)].
将 ( T n ( f ) − T 2 n ( f ) ) ( T_n( f) - T_{2n}( f) ) (Tn(f)−T2n(f)) 作 为 T 2 n ( f ) T_{2n}(f) T2n(f)的修正值补充到 I ( f ) I(f) I(f),即
I ( f ) ≈ T 2 n ( f ) + 1 3 ( T 2 n ( f ) − T n ( f ) ) = 4 3 T 2 n − 1 3 T n = S n I(f)\approx T_{2n}(f)+\frac{1}{3}\left(T_{2n}\left(f\right)-T_{n}\left(f\right)\right)=\frac{4}{3}T_{2n}-\frac{1}{3}T_{n}=S_{n} I(f)≈T2n(f)+31(T2n(f)−Tn(f))=34T2n−31Tn=Sn
其结果是将复化梯形求积公式组合成复化 Simpson 求积公式, 截断误差由 O ( h 2 ) O(h^2) O(h2)提高到 O ( h 4 ) O(h^4) O(h4),这种手段称为外推算法,该算法在不增加计算量的前提下提高了误差的精度.不妨对 S 2 n ( f ) , S n ( f ) S_{2n}(f),S_n(f) S2n(f),Sn(f)再作一次线性组合:
I ( f ) − S n ( f ) = − f ( 4 ) ( ξ ) 180 h 4 ( b − a ) ≈ d h 4 I\left(f\right)-S_{n}\left(f\right)=-\frac{f^{\left(4\right)}\left(\xi\right)}{180}h^{4}\left(b-a\right)\approx dh^{4} I(f)−Sn(f)=−180f(4)(ξ)h4(b−a)≈dh4
I ( f ) − S 2 n ( f ) = − f ( 4 ) ( η ) 180 ( h 2 ) 4 ( b − a ) ≈ d ( h 2 ) 4 I(f)-S_{2n}(f)=-\frac{f^{(4)}(\eta)}{180}\left(\frac{h}{2}\right)^{4}(b-a)\approx d\left(\frac{h}{2}\right)^{4} I(f)−S2n(f)=−180f(4)(η)(2h)4(b−a)≈d(2h)4
I ( f ) ≈ S 2 n ( f ) + 1 15 ( S 2 n ( f ) − S n ( f ) ) = C n ( f ) I\left(f\right)\approx S_{2n}\left(f\right)+\frac{1}{15}\left(S_{2n}\left(f\right)-S_{n}\left(f\right)\right)=C_{n}\left(f\right) I(f)≈S2n(f)+151(S2n(f)−Sn(f))=Cn(f)
复化 Simpson 公式组成复化 Cotes 公式,其截断误差是 O ( h 6 ) . O(h^6). O(h6).同理对 Cotes公式进行线性组合:
I ( f ) − C 2 n ( f ) = e ( h 2 ) 6 I ( f ) − C n ( f ) = e h 6 I\left(f\right)-C_{2n}\left(f\right)=e\left(\frac{h}{2}\right)^{6}\\I\left(f\right)-C_{n}\left(f\right)=eh^{6} I(f)−C2n(f)=e(2h)6I(f)−Cn(f)=eh6
得到具有 7 次代数精度和截断误差是 O ( h 8 ) O(h^8) O(h8)的 Romberg 公式:
R n ( f ) = C 2 n ( f ) + 1 63 ( C 2 n ( f ) − C n ( f ) ) R_{n}\left(f\right)=C_{2n}\left(f\right)+\frac{1}{63}\left(C_{2n}\left(f\right)-C_{n}\left(f\right)\right) Rn(f)=C2n(f)+631(C2n(f)−Cn(f))
为了便于在计算机上实现 Romberg 算法,将 T n , S n , C n , R n , ⋯ T_n,S_n,C_n,R_n,\cdots Tn,Sn,Cn,Rn,⋯统一用 R k , j R_{k,j} Rk,j 表示,列标 j = 1 , 2 , 3 , 4 j=1,2,3,4 j=1,2,3,4分别表示梯形、Simpson、Cotes 、Romberg积分,行标 k k k表示步长 h k = h 2 k − 1 h_k=\frac h{2^{k-1}} hk=2k−1h,得到Romberg 计算公式:
R k , j = R k , j − 1 + R k , j − 1 − R k − 1 , j − 1 4 j − 1 − 1 , k = 2 , 3 , ⋯ R_{k,j}=R_{k,j-1}+\frac{R_{k,j-1}-R_{k-1,j-1}}{4^{j-1}-1},k=2,3,\cdots Rk,j=Rk,j−1+4j−1−1Rk,j−1−Rk−1,j−1,k=2,3,⋯
对每一个 k , j k,j k,j从 2 做到 k k k,一直做到 ∣ R k , k − R k − 1 , k − 1 ∣ |R_k,k-R_{k-1,k-1}| ∣Rk,k−Rk−1,k−1∣小于给定控制精度时停止计算.
注:下面代码中数组下标从0开始.
代码
C++实现Romberg积分运算
#include<bits/stdc++.h>
using namespace std;int satisfiedCount;long double ax(long double t);
long double ay(long double t);
long double romberg(function<long double(long double)> f, long double a, long double b, long double eps, int maxIter, bool isX);// Perform the Romberg integrationint main()
{long double eps = 1e-6, proportion;int maxIter;satisfiedCount = 0;maxIter = 4;cout << "maxIter = " << maxIter << endl;for (long double t = 0.1; t <= 10; t += 0.1) { long double vx = romberg(ax, 0, t, eps, maxIter, 0);long double vy = romberg(ay, 0, t, eps, maxIter, 0);long double x = romberg([&](long double t) { return vx; }, 0, t, eps, maxIter, 1);long double y = romberg([&](long double t) { return vy; }, 0, t, eps, maxIter, 0);cout << fixed << setprecision(1) << "At t = " << t << ", vx = " << setprecision(6) << vx << ", vy = " << setprecision(6) << vy << ", " << "(x, y) = (" << setprecision(6) << x << ", " << setprecision(6) << y << ")" << endl;}proportion = (long double)satisfiedCount / 100;cout << "At maxIter = " << maxIter << ", proportion of times the error requirement of x was satisfied: " << proportion << endl;satisfiedCount = 0;maxIter = 8;cout << "maxIter = " << maxIter << endl;ofstream outFile("trajectory.txt");for (long double t = 0.1; t <= 10; t += 0.1) {long double vx = romberg(ax, 0, t, eps, maxIter, 0);long double vy = romberg(ay, 0, t, eps, maxIter, 0);long double x = romberg([&](long double t) { return vx; }, 0, t, eps, maxIter, 1);long double y = romberg([&](long double t) { return vy; }, 0, t, eps, maxIter, 0);cout << fixed << setprecision(1) << "At t = " << t << ", vx = " << setprecision(6) << vx << ", vy = " << setprecision(6) << vy << ", " << "(x, y) = (" << setprecision(6) << x << ", " << setprecision(6) << y << ")" << endl;outFile << fixed << setprecision(6) << x << " " << y << "\n";//把坐标写入文件,方便画轨迹}proportion = (long double)satisfiedCount / 100;cout << "At maxIter = " << maxIter << ", proportion of times the error requirement of x was satisfied: " << proportion << endl;satisfiedCount = 0;maxIter = 12;cout << "maxIter = " << maxIter << endl;for (long double t = 0.1; t <= 10; t += 0.1) {long double vx = romberg(ax, 0, t, eps, maxIter, 0);long double vy = romberg(ay, 0, t, eps, maxIter, 0);long double x = romberg([&](long double t) { return vx; }, 0, t, eps, maxIter, 1);long double y = romberg([&](long double t) { return vy; }, 0, t, eps, maxIter, 0);cout << fixed << setprecision(1) << "At t = " << t << ", vx = " << setprecision(6) << vx << ", vy = " << setprecision(6) << vy << ", " << "(x, y) = (" << setprecision(6) << x << ", " << setprecision(6) << y << ")" << endl;}proportion = (long double)satisfiedCount / 100;cout << "At maxIter = " << maxIter << ", proportion of times the error requirement of x was satisfied: " << proportion << endl;satisfiedCount = 0;maxIter = 16;cout << "maxIter = " << maxIter << endl;for (long double t = 0.1; t <= 10; t += 0.1) {long double vx = romberg(ax, 0, t, eps, maxIter, 0);long double vy = romberg(ay, 0, t, eps, maxIter, 0);long double x = romberg([&](long double t) { return vx; }, 0, t, eps, maxIter, 1);long double y = romberg([&](long double t) { return vy; }, 0, t, eps, maxIter, 0);cout << fixed << setprecision(1) << "At t = " << t << ", vx = " << setprecision(6) << vx << ", vy = " << setprecision(6) << vy << ", " << "(x, y) = (" << setprecision(6) << x << ", " << setprecision(6) << y << ")" << endl;}proportion = (long double)satisfiedCount / 100;cout << "At maxIter = " << maxIter << ", proportion of times the error requirement of x was satisfied: " << proportion << endl;satisfiedCount = 0;maxIter = 20;cout << "maxIter = " << maxIter << endl;for (long double t = 0.1; t < 10.1; t += 0.1) {long double vx = romberg(ax, 0, t, eps, maxIter, 0);long double vy = romberg(ay, 0, t, eps, maxIter, 0);long double x = romberg([&](long double t) { return vx; }, 0, t, eps, maxIter, 1);long double y = romberg([&](long double t) { return vy; }, 0, t, eps, maxIter, 0);cout << fixed << setprecision(1) << "At t = " << t << ", vx = " << setprecision(6) << vx << ", vy = " << setprecision(6) << vy << ", " << "(x, y) = (" << setprecision(6) << x << ", " << setprecision(6) << y << ")" << endl;}proportion = (long double)satisfiedCount / 100;cout << "At maxIter = " << maxIter << ", proportion of times the error requirement of x was satisfied: " << proportion << endl;return 0;
}long double ax(long double t)
{return sin(t) / (1 + sqrt(t));
}long double ay(long double t)
{return log(t + 1) / (t + 1);
}// Perform the Romberg integration
long double romberg(function<long double(long double)> f, long double a, long double b, long double eps, int maxIter, bool isX) {long double h[maxIter], r[maxIter][maxIter];h[0] = b - a;r[0][0] = 0.5 * h[0] * (f(a) + f(b));for (int i = 1; i < maxIter; i++) {h[i] = 0.5 * h[i-1];long double sum = 0;for (int k = 0; k < pow(2, i-1); k++)sum += f(a + (2*k+1) * h[i]);r[i][0] = 0.5 * r[i-1][0] + h[i] * sum;for (int j = 1; j <= i; j++)r[i][j] = r[i][j-1] + (r[i][j-1] - r[i-1][j-1]) / (pow(4, j) - 1);if (i > 1 && fabs(r[i][i] - r[i-1][i-1]) < eps){if(isX)satisfiedCount++;return r[i][i];}}return r[maxIter-1][maxIter-1];
}
python可视化运动轨迹
import matplotlib.pyplot as pltwith open('trajectory.txt', 'r') as file:lines = file.readlines()x, y = zip(*[(float(line.split()[0]), float(line.split()[1])) for line in lines])plt.plot(x, y, 'o-')
plt.xlabel('X')
plt.ylabel('Y')
plt.title('Plot of points with smooth curve')
plt.show()
结果
部分运算结果
轨迹可视化结果
相关文章:
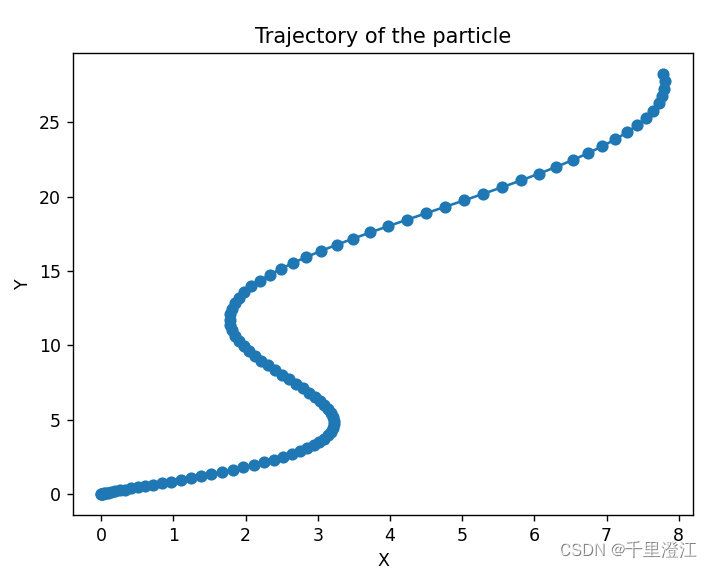
计算方法实验9:Romberg积分求解速度、位移
任务 输出质点的轨迹 ( x ( t ) , y ( t ) ) , t ∈ { 0.1 , 0.2 , 0.3 , . . . , 10 } (x(t), y(t)), t\in \{0.1, 0.2, 0.3, ..., 10\} (x(t),y(t)),t∈{0.1,0.2,0.3,...,10},并在二维平面中画出该轨迹.请比较M分别取4, 8, 12, 16, 20 时,Romberg积分达…...

设计模式有哪些基本原则
目录 开闭原则(Open Closed Principle) 里氏替换原则(Liskov Substitution principle) 单一职责原则(Single Responsibility Principle,SRP)...
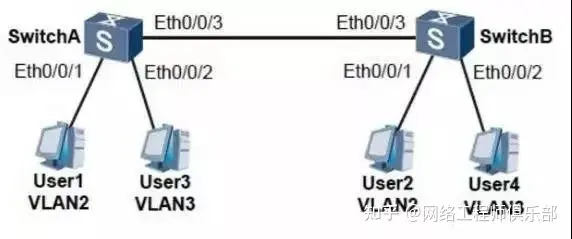
别再出错了!华为交换机到底如何配置access、trunk、hybird端口?
号主:老杨丨11年资深网络工程师,更多网工提升干货,请关注公众号:网络工程师俱乐部 下午好,我的网工朋友。 我们都知道,网络工程师的工作离不开对交换机的熟练操作。华为交换机的配置,绝对是考验…...

OceanBase 分布式数据库【信创/国产化】- OceanBase 平台产品 - 迁移评估工具 OMA
本心、输入输出、结果 文章目录 OceanBase 分布式数据库【信创/国产化】- OceanBase 平台产品 - 迁移评估工具 OMA前言OceanBase 数据更新架构OceanBase 平台产品 - 迁移评估工具 OMA兼容性评估性能评估导出 OceanBase 数据库对象和 SQL 语句OceanBase 分布式数据库【信创/国产…...
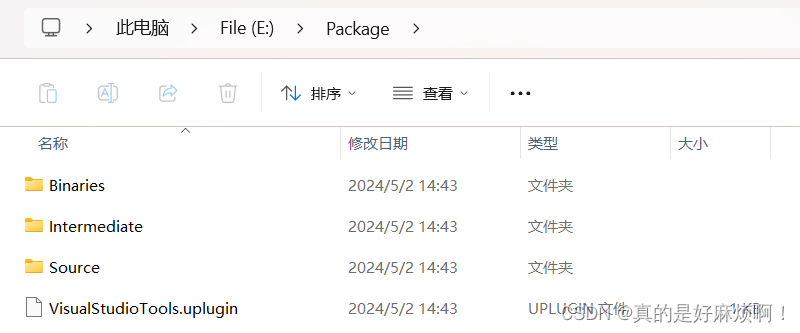
UE5入门学习笔记(六)——编译低版本插件
对于有些低版本的插件,可以通过此方法自己编译到高版本而无需等待插件作者更新 使用工具:如图所示 步骤1:打开cmd,并使用cd命令切换到此目录 步骤2:输入如下指令 RunUAT.bat BuildPlugin -Plugin“路径1” -Package“…...

MySQL全局锁、表级锁、行锁、死锁、索引选择
文章目录 全局锁表级锁表锁元数据锁 MDL 如何安全的给小表添加字段1. 理解和监控长事务2. 使用NOWAIT和WAIT语法示例 3. 选择合适的时间窗口4. 分阶段执行5. 使用在线DDL工具 行锁死锁普通索引和唯一索引的选择索引基础业务场景分析性能考量实践建议索引及其选择机制索引选择错…...
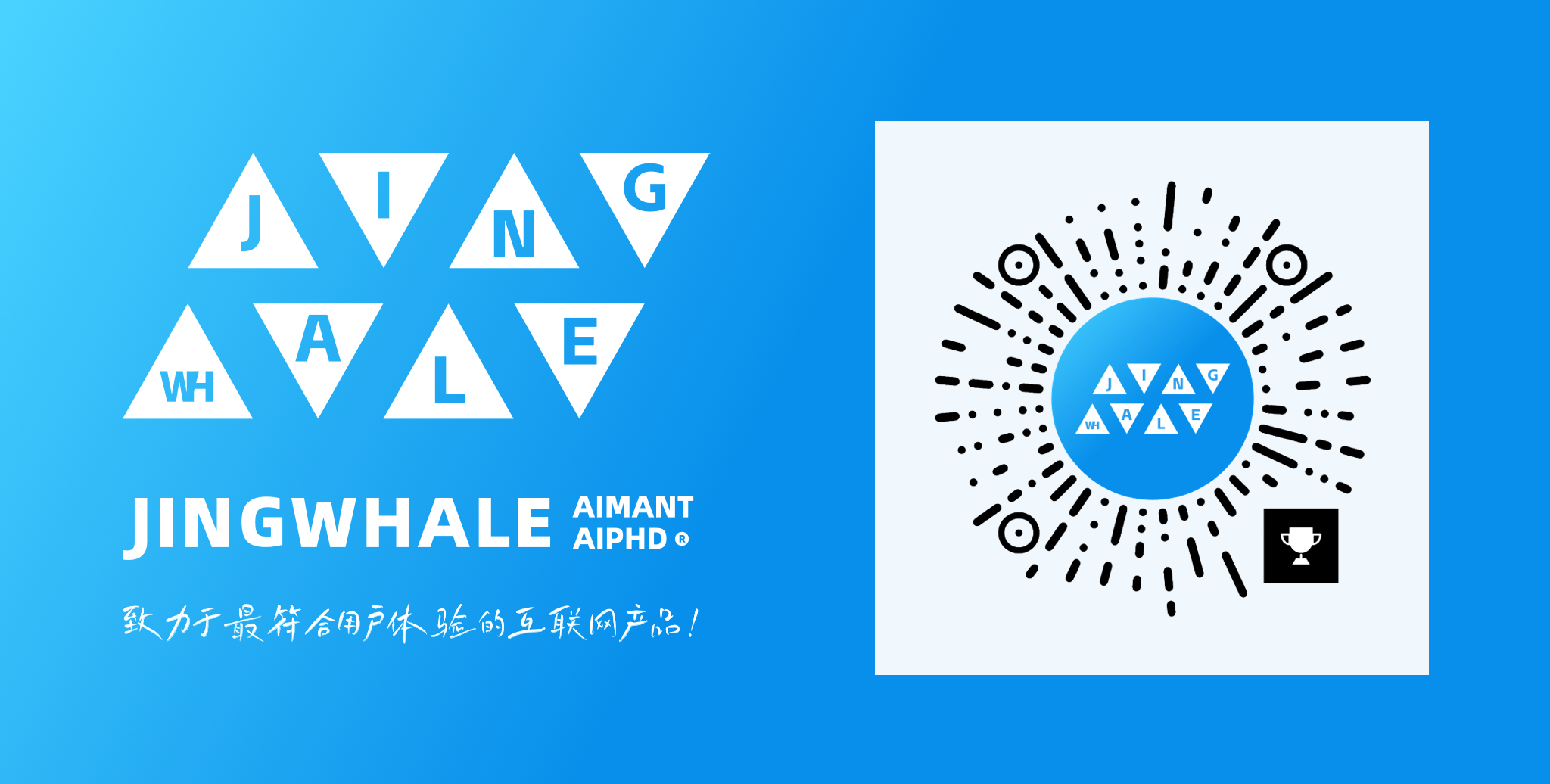
深入解析算法效率核心:时间与空间复杂度概览及优化策略
算法复杂度,即时间复杂度与空间复杂度,衡量算法运行时资源消耗。时间复杂度反映执行时间随数据规模增长的关系,空间复杂度表明额外内存需求。优化策略,如选择合适数据结构、算法改进、循环展开等,对于提升程序效率、减…...
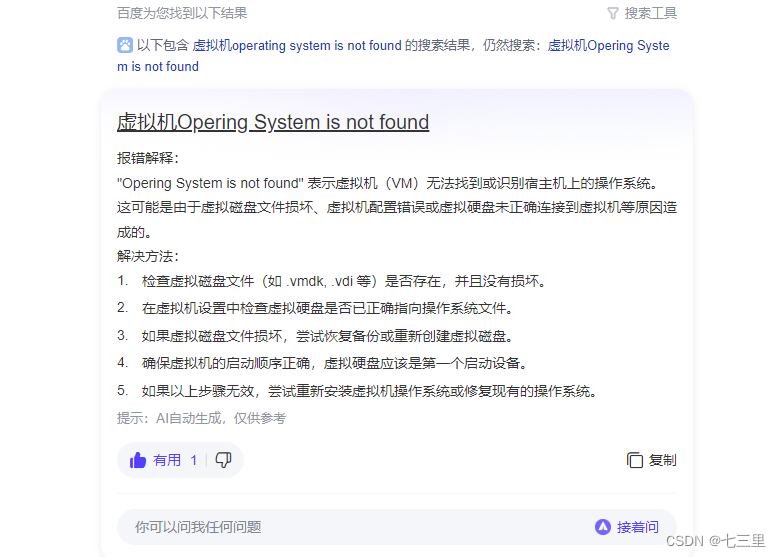
虚拟机装CentOS镜像
起先,是先安装一个VM虚拟机,再去官方网站之类的下载一些镜像,常见镜像有CentOS镜像,ubantu镜像,好像还有一个树莓还是什么的,软件这块,日新月异,更新太快,好久没碰&#…...
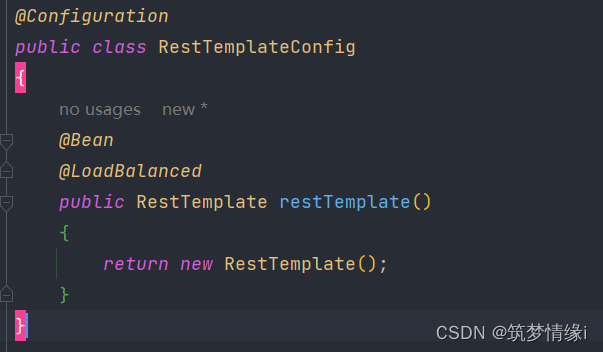
SpringCloud 集成consul,消费者报I/O error on GET request for...
创建消费者微服务,去调用生产者微服务的请求过程中,出现以下错误: 报错原因 因为在使用SpringCloudAlibaba中的Nacos框架时,自动整合了SpringCloud中的Ribbon框架中的负载均衡,因为微服务提供者有两个,在消…...

pytest的测试标记marks
引用打标的marks文档 Python的pytest框架(5)--测试标记(Markers)_pytest执行指定的marker-CSDN博客 https://www.cnblogs.com/pipile/p/12696226.html 给用例自定义打标签的代码示例 #coding:utf-8 import pytest pytest.mark.smoke def test_1():print("smoke的测试用…...
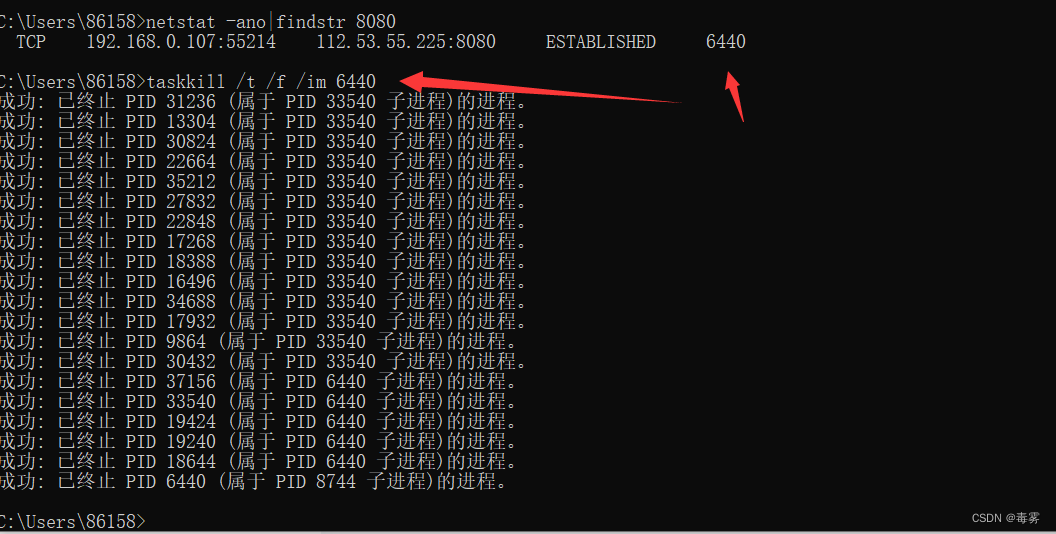
端口占用解决方法
1、查询端口 打开cmd命令提示符窗口,输入以下指令查询所有端口 netstat -ano //查询所有端口 netstat -ano|findstr 8080 //查询指定端口 2、杀死进程 taskkill /t /f /im 进程号(PID)...
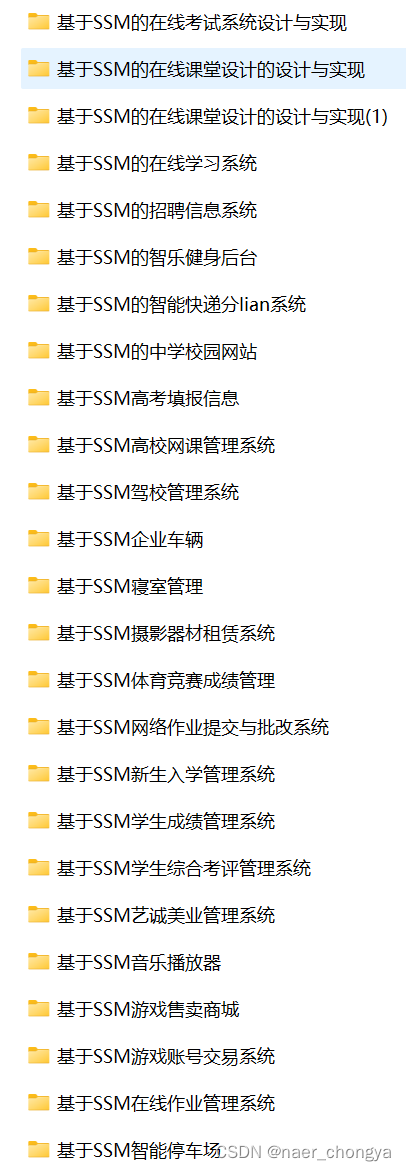
Java毕设之基于springboot的医护人员排班系统
运行环境 开发语言:java 框架:springboot,vue JDK版本:JDK1.8 数据库:mysql5.7(推荐5.7,8.0也可以) 数据库工具:Navicat11 开发软件:idea/eclipse(推荐idea) 系统详细实现 医护类型管理 医护人员排班系统的系统管理员可以对医护类型添加修改删除以及…...
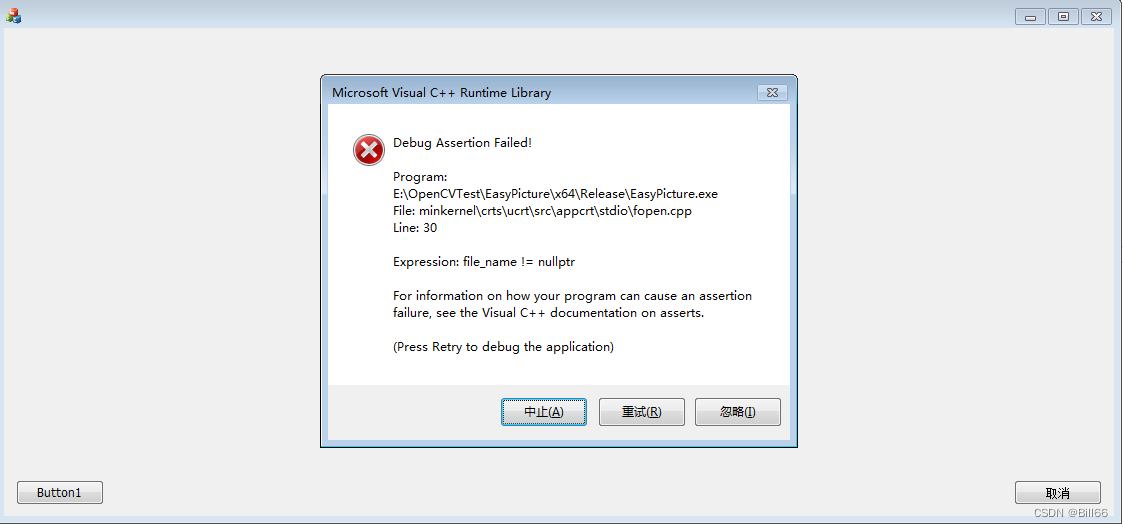
OpenCV4.8 VS2019 MFC编程出现的诡异现象
OpenCV4.8及OpenCV4.4 VS2019MFC编程在调用imred()函数时,debug X64试运行没问题。 release X64试运行时出现下面错误。 void CEasyPictureDlg::OnBnClickedOpen() {CFileDialog fdlg(TRUE, NULL, 0, OFN_HIDEREADONLY | OFN_OVERWRITEPROMP…...
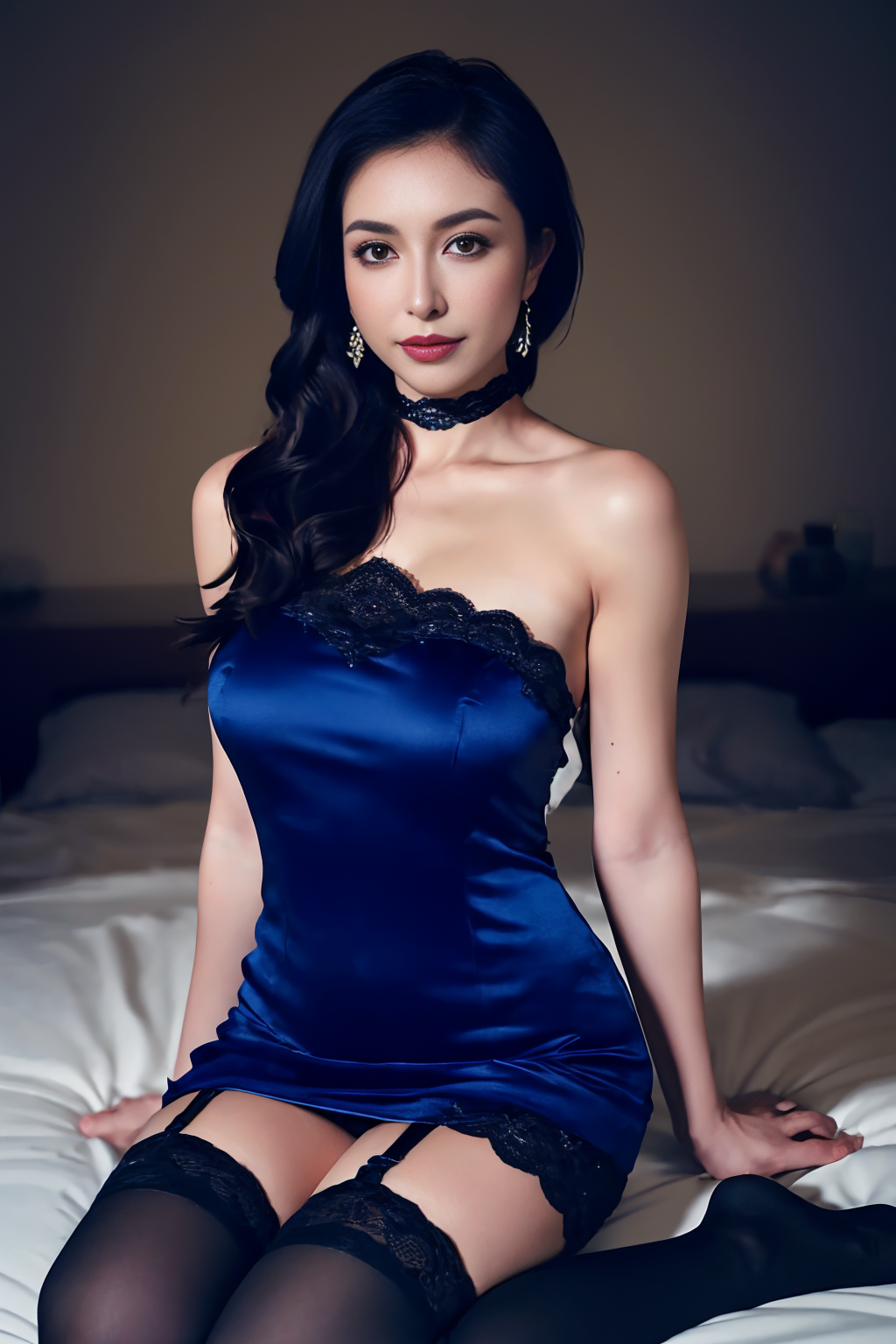
游戏辅助 -- 三种分析角色坐标方法(CE、xdbg、龙龙遍历工具)
所用工具下载地址: https://pan.quark.cn/s/d54e7cdc55e6 在上次课程中,我们成功获取了人物对象的基址:[[[0xd75db8]1C]28],而人物血量的地址则是基址再加上偏移量278。 接下来,我们需要执行以下步骤来进一步操作&a…...
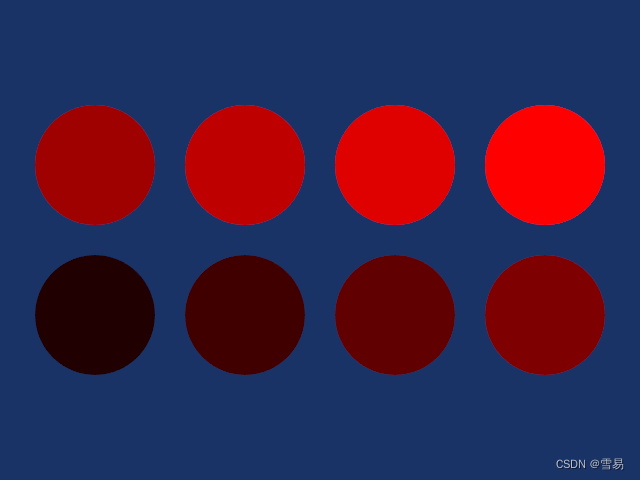
【VTKExamples::Rendering】第一期 TestAmbientSpheres(环境照明系数)
很高兴在雪易的CSDN遇见你 VTK技术爱好者 QQ:870202403 公众号:VTK忠粉 前言 本文分享VTK样例TestAmbientShperes,介绍环境照明系数对Actor颜色的影响,希望对各位小伙伴有所帮助! 感谢各位小伙伴的点赞+关注,小易会继续努力分享,一起进步! 你的点赞就是我的动…...

代码随想录leetcode200题之栈与队列
目录 1 介绍2 训练3 参考 1 介绍 本博客用来记录代码随想录leetcode200题中栈与队列部分的题目。 2 训练 题目1:232. 用栈实现队列 C代码如下, #include <stack>class MyQueue { private:stack<int> a;stack<int> b; //辅助栈 pu…...
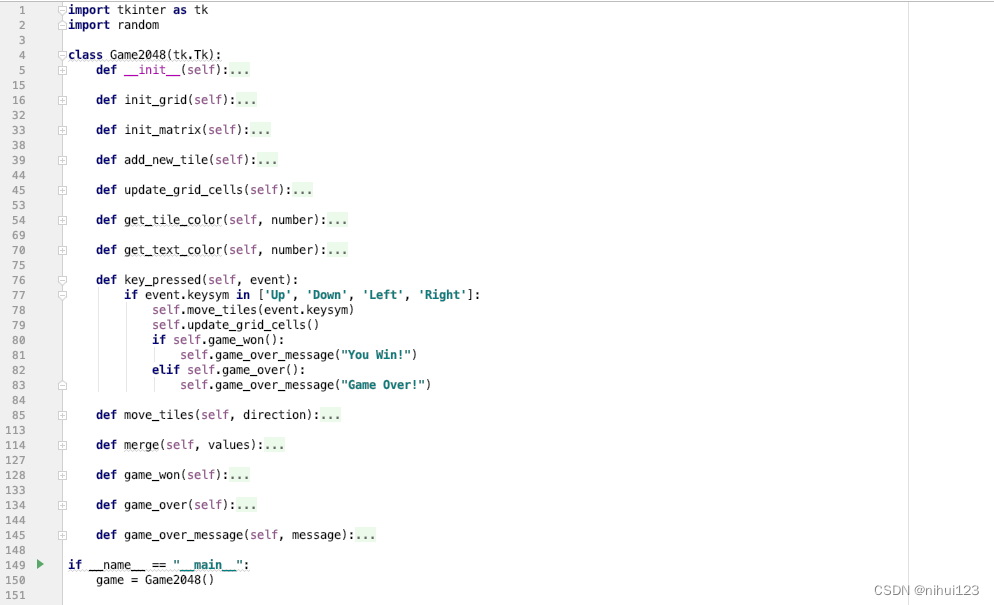
使用Python实现2048小游戏
使用Python实现2048小游戏源码分享。实现效果如下所示。 实现效果图 游戏开始效果图 游戏结束效果图 部分源码截图 下载链接 基于如下的运行环境。运行需要安装tkinter /Library/Frameworks/Python.framework/Versions/3.7/bin/python/bin/python /Users/nihui/Documents/P…...
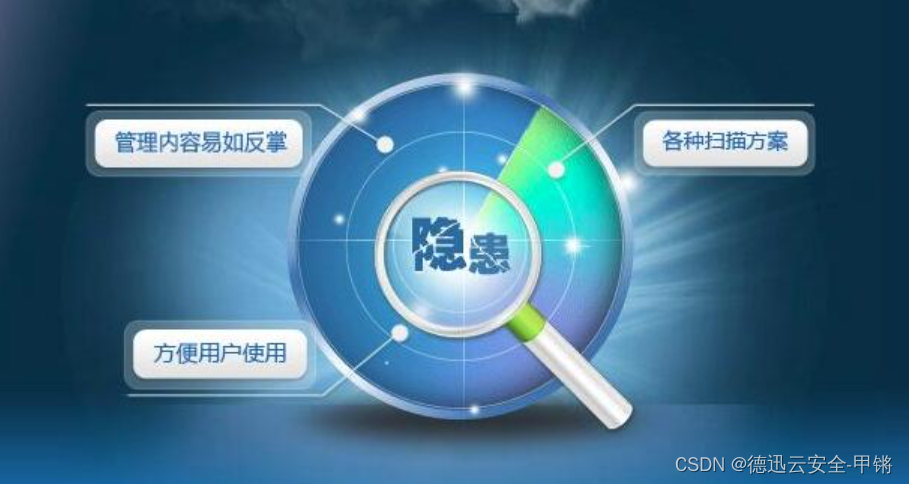
漏洞管理是如何在攻击者之前识别漏洞从而帮助人们阻止攻击的
漏洞管理 是主动查找、评估和缓解组织 IT 环境中的安全漏洞、弱点、差距、错误配置和错误的过程。该过程通常扩展到整个 IT 环境,包括网络、应用程序、系统、基础设施、软件和第三方服务等。鉴于所涉及的高成本,组织根本无法承受网络攻击和数据泄露。如果…...
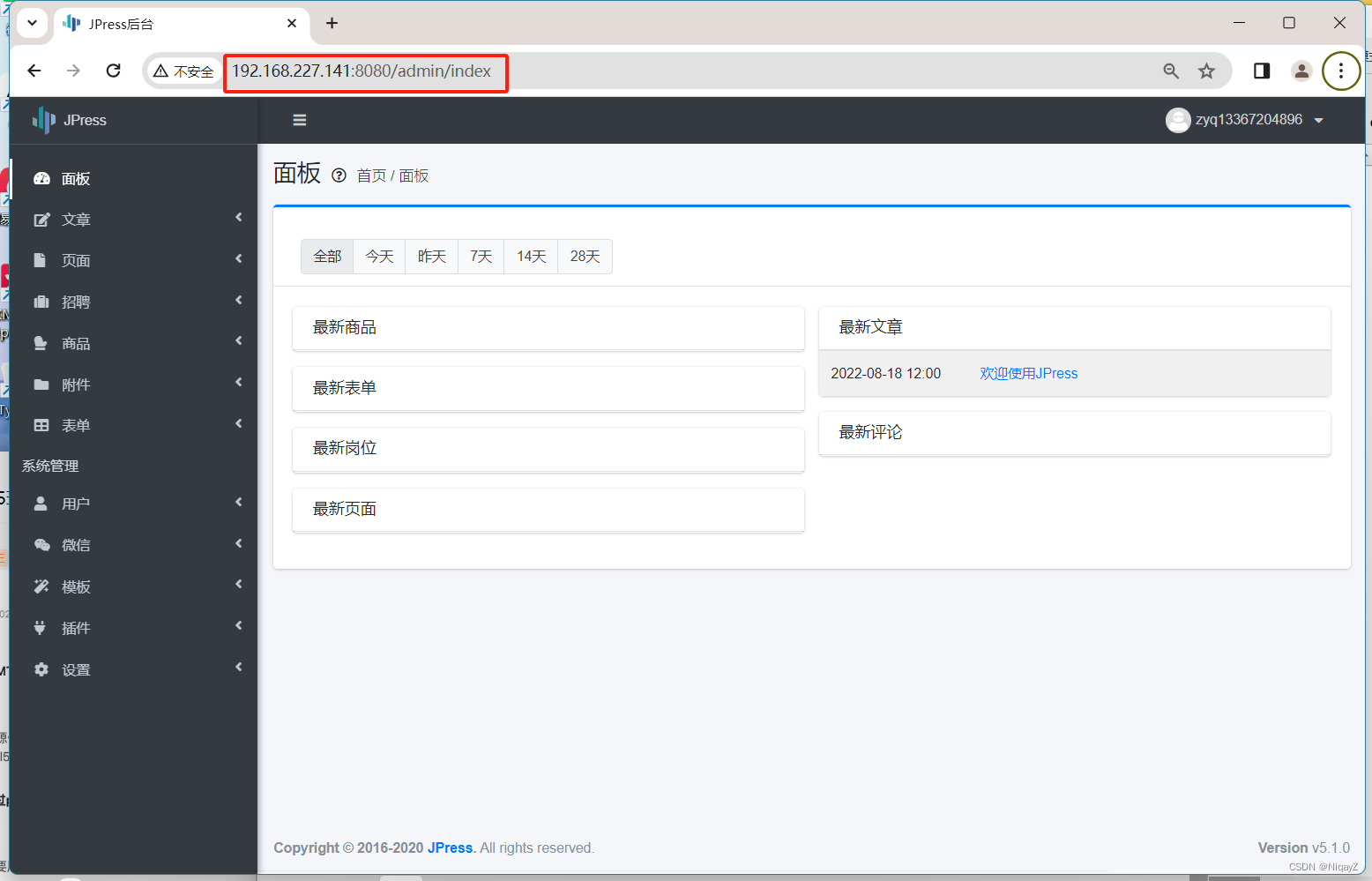
LNMT部署jpress
LNMT部署jpress 环境要求: MySQL版本5.6/5.7 tomcat版本9.0.65 源码安装MySQL5.7版 //源码安装MySQL5.7版1关闭防火墙 2创建mysql用户 3上传mysql5.7包(https://downloads.mysql.com/archives/get/p/23/file/mysql-5.7.30-linux-glibc2.12-x86_64.tar.g…...

汽车软件研发工具链丨怿星科技新产品重磅发布
“创新引领未来”聚焦汽车软件新基建,4月27日下午,怿星科技2024新产品发布会在北京圆满举行!智能汽车领域的企业代表、知名大企业负责人、投资机构代表、研究机构代表齐聚现场,线上直播同步开启,共同见证怿星科技从单点…...

Faiss原理及使用总结
Faiss(Facebook AI Similarity Search)是一个用于高效相似性搜索和密集向量聚类的库。 一、原理 向量表示与相似度度量:在Faiss中,数据通常被表示为高维向量,这些向量可以来自深度学习模型的特征提取,也可…...
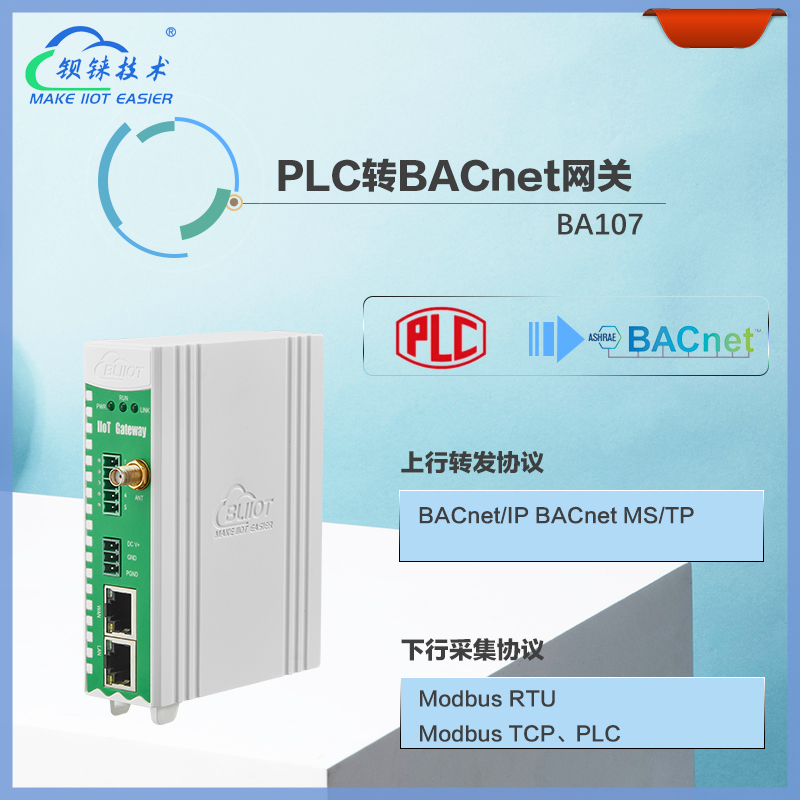
跨越智能建筑桥梁:西门子PLC无缝对接BACnet楼宇自动化系统化
智能楼宇每一个环节的互联互通都至关重要,而PLC(可编程逻辑控制器)作为自动化领域的基石,其与BACnet协议的融合无疑成为了构建智能楼宇神经系统的关键节点。今天,让我们深入探讨如何利用先进的PLC转BACnet协议网关&…...
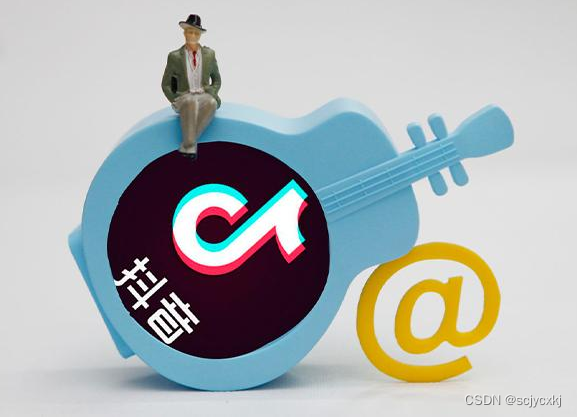
景源畅信电商:抖音小店有哪些比较热门的宣传方法?
抖音小店的热门宣传方法,是许多商家关注的焦点。在数字化营销时代,有效的宣传手段不仅能提升品牌知名度,还能吸引潜在消费者,促进销售。以下是针对抖音小店热门宣传方法的详细阐述: 一、短视频内容营销 作为抖音的核心…...

兄弟DCP-7057激光打印机报错误代码EC检修及分析
故障描述: 兄弟DCP-7057激光打印机屏幕显示无法打印EC关闭电源,然后重新打开打印机。 故障检修及分析: 1、定影单元风扇的插线连接不良 检查定影单元风扇的插线连接并重新连接; 2、定影单元风扇故障 更换定影单元风扇;…...
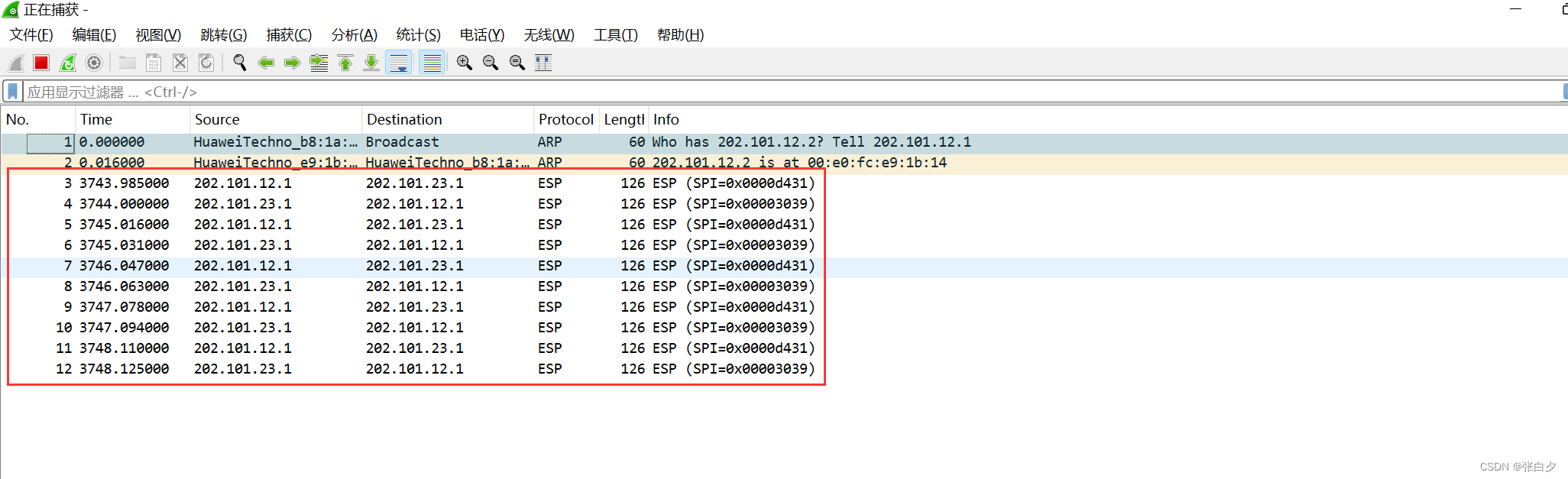
【华为】IPSec VPN手动配置
【华为】IPSec VPN手动配置 拓扑配置ISP - 2AR1NAT - Easy IPIPSec VPN AR3NATIPsec VPN PC检验 配置文档AR1AR2 拓扑 配置 配置步骤 1、配置IP地址,ISP 路由器用 Lo0 模拟互联网 2、漳州和福州两个出口路由器配置默认路由指向ISP路由器 3、进行 IPsec VPN配置&…...

面试题分享之Java集合篇(三)
注意:文章若有错误的地方,欢迎评论区里面指正 🍭 系列文章目录 面试题分享之Java基础篇(二)面试题分享之Java基础篇(三) 面试题分享之Java集合篇(一)、 面试题分享之Ja…...
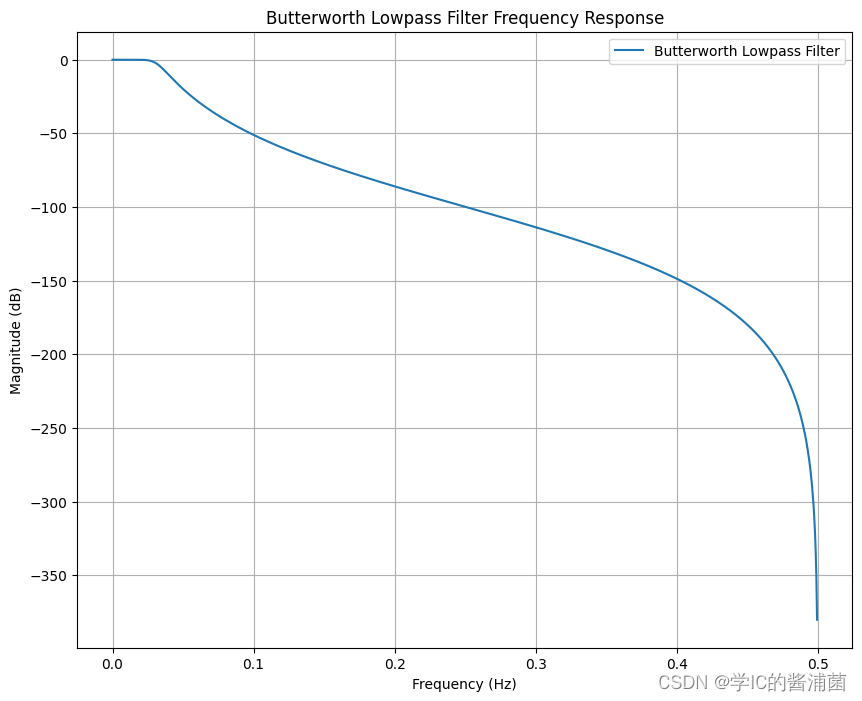
【python】模拟巴特沃斯滤波器
巴特沃斯滤波器(Butterworth Filter),以其设计者斯蒂芬巴特沃斯(Stephen Butterworth)的名字命名,是一种具有平滑频率响应的滤波器。这种滤波器在频域中具有非常平坦的无波纹响应,直到它达到截止…...

面试题:简述Go的垃圾回收机制
Go的GC(Garbage Collection, 垃圾回收)机制主要是用来自动释放不再被程序使用的内存,以防止内存泄漏。Go的垃圾回收是并发的,也就是说,它在主程序运行的同时进行垃圾回收。 1. 标记清除(Mark and Sweep) Go的垃圾回收器主要使用的是标记清除…...
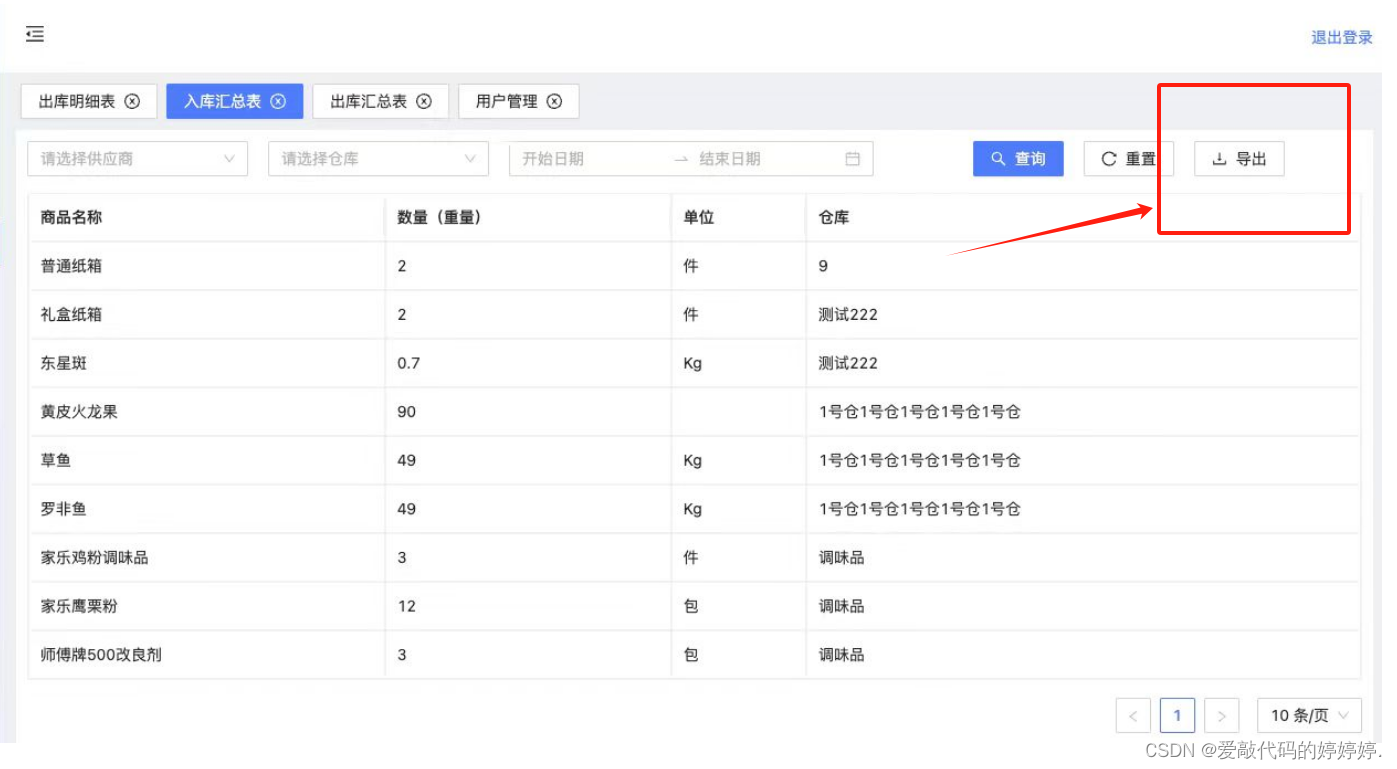
Vue、React实现excel导出功能(三种实现方式保姆级讲解)
第一种:后端返回文件流,前端转换并导出(常用,通常公司都是用这种方式) 第二种:纯后端导出(需要了解) 第三种:纯前端导出(不建议使用,数据处理放…...
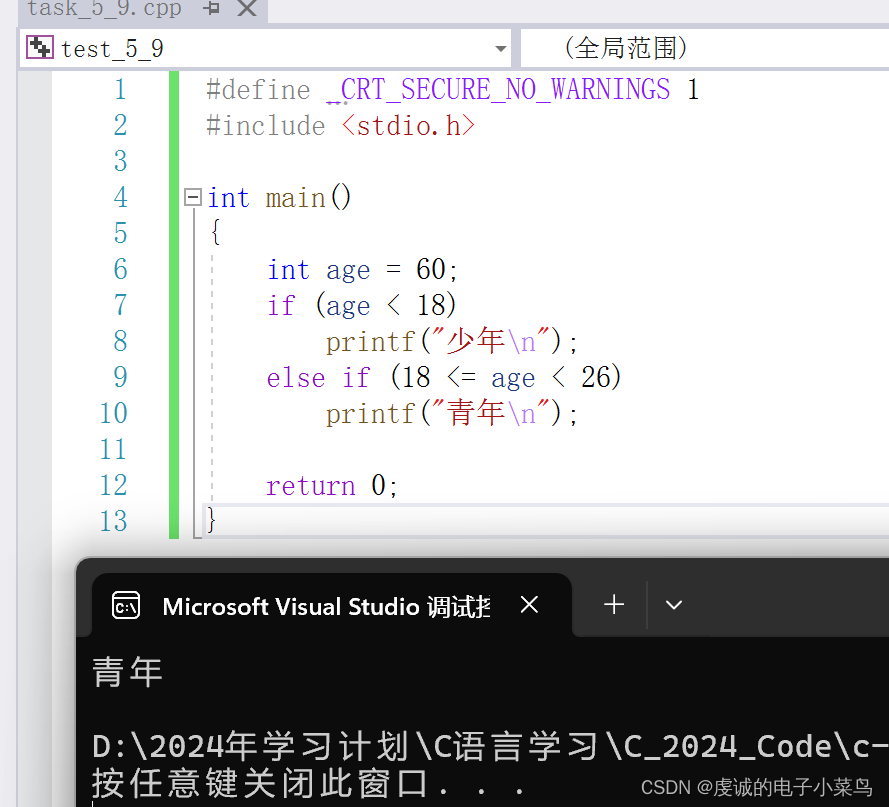
初识C语言——第十六天
C语言中的语句结构类型:顺序/选择/循环 分支语句 if else switch 循环语句 while for do whlie goto语句 代码练习:找两个整数的最大公约数和最小公倍数 #define _CRT_SECURE_NO_WARNINGS #include <stdio.h>//int main() //{ // int age 60; // if (ag…...