双色球预测算法(Java),——森林机器学习、时间序列
最近AI很火,老想着利用AI的什么算法,干点什么有意义的事情。其中之一便想到了双色球,然后让AI给我预测,结果基本都是简单使用随机算法列出了几个数字。
额,,,,咋说呢,双色球确实是随机的,但是,如果只是随机,我用你AI干嘛,直接写个随机数就行了嘛。
于是乎,问了下市面上的一些预测算法,给出了俩,一个是:森林机器学习,一个是时间序列。
然后,我让它给我把这俩算法写出来,给是给了,但是,,,无力吐槽。
于是,在我和它的共同配合下,这俩算法的java版诞生了,仅供参考:
森林机器学习:
package com.ruoyi.web.controller.test;
import java.io.BufferedReader;
import java.io.FileInputStream;
import java.io.InputStreamReader;
import java.nio.charset.StandardCharsets;
import java.util.ArrayList;
import java.util.HashSet;
import java.util.List;
import java.util.Set;import lombok.val;
import org.apache.commons.csv.CSVFormat;
import org.apache.commons.csv.CSVParser;
import org.apache.commons.csv.CSVRecord;import weka.classifiers.Classifier;
import weka.classifiers.trees.RandomForest;
import weka.core.Attribute;
import weka.core.DenseInstance;
import weka.core.Instances;public class LotteryPredictor {public static void main(String[] args) throws Exception {String csvFilePath = "D:\\12.csv"; // 请替换为你的CSV文件的绝对路径// Step 1: Read historical data from CSVList<int[]> historicalData = readCSV(csvFilePath);// Step 2: Prepare data for WekaInstances trainingData = prepareTrainingData(historicalData);// Step 3: Train RandomForest modelClassifier model = new RandomForest();model.buildClassifier(trainingData);// Step 4: Make a predictionint[] prediction = predictNextNumbers(model, trainingData);// Output the predictionSystem.out.println("Predicted numbers: ");for (int num : prediction) {System.out.print(num + " ");}}private static List<int[]> readCSV(String csvFilePath) throws Exception {List<int[]> data = new ArrayList<>();try (BufferedReader reader = new BufferedReader(new InputStreamReader(new FileInputStream(csvFilePath), StandardCharsets.UTF_8))) {CSVParser csvParser = new CSVParser(reader, CSVFormat.DEFAULT.withDelimiter(',').withTrim());for (CSVRecord record : csvParser) {if(record.size() == 1) {val rec = record.get(0).split(","); // Remove non-numeric charactersint[] row = new int[rec.length];for (int i = 0; i < rec.length; i++) {String value = rec[i].replaceAll("[^0-9]", ""); // Remove non-numeric charactersif (!value.isEmpty()) {row[i] = Integer.parseInt(value);}}data.add(row);}else {int[] row = new int[record.size()];for (int i = 0; i < record.size(); i++) {String value = record.get(i).replaceAll("[^0-9]", ""); // Remove non-numeric charactersif (!value.isEmpty()) {row[i] = Integer.parseInt(value);}}data.add(row);}}}return data;}private static Instances prepareTrainingData(List<int[]> historicalData) {// Define attributesArrayList<Attribute> attributes = new ArrayList<>();for (int i = 0; i < historicalData.get(0).length; i++) {attributes.add(new Attribute("num" + (i + 1)));}// Create datasetInstances dataset = new Instances("LotteryData", attributes, historicalData.size());dataset.setClassIndex(dataset.numAttributes() - 1);// Add datafor (int[] row : historicalData) {dataset.add(new DenseInstance(1.0, toDoubleArray(row)));}return dataset;}private static double[] toDoubleArray(int[] intArray) {double[] doubleArray = new double[intArray.length];for (int i = 0; i < intArray.length; i++) {doubleArray[i] = intArray[i];}return doubleArray;}private static int[] predictNextNumbers(Classifier model, Instances trainingData) throws Exception {int numAttributes = trainingData.numAttributes();Set<Integer> predictedNumbers = new HashSet<>();while (predictedNumbers.size() < numAttributes) {DenseInstance instance = new DenseInstance(numAttributes);instance.setDataset(trainingData);for (int i = 0; i < numAttributes; i++) {instance.setValue(i, Math.random() * 33 + 1); // Random values for prediction}double prediction = model.classifyInstance(instance);int predictedNumber = (int) Math.round(prediction);// Ensure the predicted number is within the valid range and not a duplicateif (predictedNumber >= 1 && predictedNumber <= 33) {predictedNumbers.add(predictedNumber);}}int[] predictionArray = new int[numAttributes];int index = 0;for (int num : predictedNumbers) {predictionArray[index++] = num;}return predictionArray;}
}
时间序列算法:
package com.ruoyi.web.controller.test;
import lombok.val;
import org.apache.commons.csv.CSVFormat;
import org.apache.commons.csv.CSVParser;
import org.apache.commons.csv.CSVRecord;
import org.deeplearning4j.nn.api.Model;
import org.deeplearning4j.nn.conf.MultiLayerConfiguration;
import org.deeplearning4j.nn.conf.NeuralNetConfiguration;
import org.deeplearning4j.nn.conf.layers.DenseLayer;
import org.deeplearning4j.nn.conf.layers.OutputLayer;
import org.deeplearning4j.nn.multilayer.MultiLayerNetwork;
import org.deeplearning4j.optimize.listeners.ScoreIterationListener;
import org.nd4j.linalg.activations.Activation;
import org.nd4j.linalg.api.ndarray.INDArray;
import org.nd4j.linalg.dataset.DataSet;
import org.nd4j.linalg.dataset.api.preprocessor.NormalizerMinMaxScaler;
import org.nd4j.linalg.factory.Nd4j;
import org.nd4j.linalg.learning.config.Adam;
import org.nd4j.linalg.lossfunctions.LossFunctions;import java.io.BufferedReader;
import java.io.FileInputStream;
import java.io.InputStreamReader;
import java.nio.charset.StandardCharsets;
import java.util.ArrayList;
import java.util.HashSet;
import java.util.List;
import java.util.Set;public class LotteryPredictor3 {public static void main(String[] args) throws Exception {String csvFilePath = "D:\\12.csv"; // 请替换为你的CSV文件的绝对路径// Step 1: Read historical data from CSVList<int[]> historicalData = readCSV(csvFilePath);// Step 2: Prepare data for time series analysisdouble[][] timeSeriesData = prepareTimeSeriesData(historicalData);// Step 3: Train neural network modelMultiLayerNetwork model = trainModel(timeSeriesData);// Step 4: Make a predictionint[] redBallPrediction = predictRedBalls(model, timeSeriesData);int blueBallPrediction = predictBlueBall(model, timeSeriesData);// Output the predictionSystem.out.println("Predicted numbers: ");for (int num : redBallPrediction) {System.out.print(num + " ");}System.out.println("Blue ball: " + blueBallPrediction);}private static List<int[]> readCSV(String csvFilePath) throws Exception {List<int[]> data = new ArrayList<>();try (BufferedReader reader = new BufferedReader(new InputStreamReader(new FileInputStream(csvFilePath), StandardCharsets.UTF_8))) {CSVParser csvParser = new CSVParser(reader, CSVFormat.DEFAULT.withDelimiter(',').withTrim());for (CSVRecord record : csvParser) {if(record.size() == 1) {val rec = record.get(0).split(","); // Remove non-numeric charactersint[] row = new int[rec.length];for (int i = 0; i < rec.length; i++) {String value = rec[i].replaceAll("[^0-9]", ""); // Remove non-numeric charactersif (!value.isEmpty()) {row[i] = Integer.parseInt(value);}}data.add(row);}else {int[] row = new int[record.size()];for (int i = 0; i < record.size(); i++) {String value = record.get(i).replaceAll("[^0-9]", ""); // Remove non-numeric charactersif (!value.isEmpty()) {row[i] = Integer.parseInt(value);}}data.add(row);}}}return data;}private static double[][] prepareTimeSeriesData(List<int[]> historicalData) {// Flatten the historical data into a 2D arraydouble[][] timeSeriesData = new double[historicalData.size()][];for (int i = 0; i < historicalData.size(); i++) {timeSeriesData[i] = new double[historicalData.get(i).length];for (int j = 0; j < historicalData.get(i).length; j++) {timeSeriesData[i][j] = historicalData.get(i)[j];}}return timeSeriesData;}private static MultiLayerNetwork trainModel(double[][] timeSeriesData) {int numInputs = timeSeriesData[0].length;int numOutputs = numInputs;int numHiddenNodes = 10;MultiLayerConfiguration conf = new NeuralNetConfiguration.Builder().updater(new Adam(0.01)).list().layer(0, new DenseLayer.Builder().nIn(numInputs).nOut(numHiddenNodes).activation(Activation.RELU).build()).layer(1, new OutputLayer.Builder(LossFunctions.LossFunction.MSE).activation(Activation.IDENTITY).nIn(numHiddenNodes).nOut(numOutputs).build()).build();MultiLayerNetwork model = new MultiLayerNetwork(conf);model.init();model.setListeners(new ScoreIterationListener(10));// Prepare the dataINDArray input = Nd4j.create(timeSeriesData);INDArray output = Nd4j.create(timeSeriesData);DataSet dataSet = new DataSet(input, output);// Normalize the dataNormalizerMinMaxScaler scaler = new NormalizerMinMaxScaler(0, 1);scaler.fit(dataSet);scaler.transform(dataSet);// Train the modelfor (int i = 0; i < 2000; i++) {model.fit(dataSet);}return model;}private static int[] predictRedBalls(MultiLayerNetwork model, double[][] timeSeriesData) {INDArray input = Nd4j.create(timeSeriesData);INDArray output = model.output(input);double[] lastPrediction = output.getRow(output.rows() - 1).toDoubleVector();Set<Integer> predictedNumbers = new HashSet<>();for (double num : lastPrediction) {int scaledNum = (int) Math.round(num * 32) + 1; // Scale back to 1-33 rangeif (scaledNum >= 1 && scaledNum <= 33) {predictedNumbers.add(scaledNum);}if (predictedNumbers.size() == 6) {break;}}// Ensure we have exactly 6 unique numberswhile (predictedNumbers.size() < 6) {int randomNum = (int) (Math.random() * 33) + 1;predictedNumbers.add(randomNum);}int[] predictionArray = new int[6];int index = 0;for (int num : predictedNumbers) {predictionArray[index++] = num;}return predictionArray;}private static int predictBlueBall(MultiLayerNetwork model, double[][] timeSeriesData) {INDArray input = Nd4j.create(timeSeriesData);INDArray output = model.output(input);double lastPrediction = output.getDouble(output.rows() - 1);// Predict blue ball numberint blueBallPrediction = (int) Math.round(lastPrediction * 15) + 1; // Scale back to 1-16 rangeif (blueBallPrediction < 1) blueBallPrediction = 1;if (blueBallPrediction > 16) blueBallPrediction = 16;return blueBallPrediction;}
}
对比了下,时间序列的相对容易让人相信,机器学习,不知道咋评价,大家可以试试。
相关文章:
双色球预测算法(Java),——森林机器学习、时间序列
最近AI很火,老想着利用AI的什么算法,干点什么有意义的事情。其中之一便想到了双色球,然后让AI给我预测,结果基本都是简单使用随机算法列出了几个数字。 额,,,,咋说呢,双…...
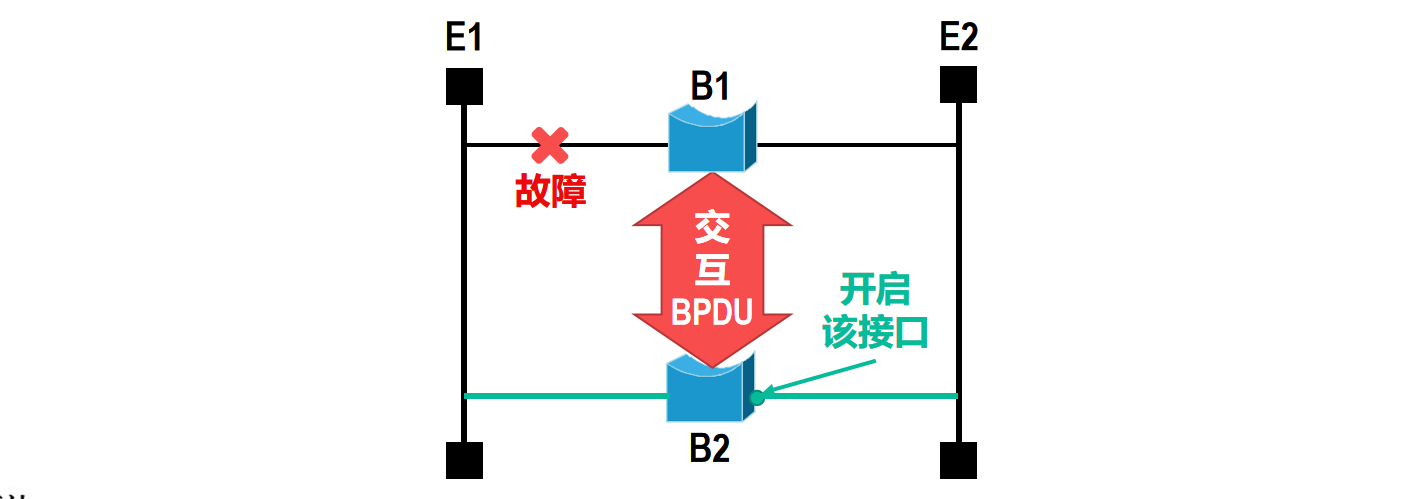
【计算机网络篇】数据链路层(11)在数据链路层扩展以太网
文章目录 🍔使用网桥在数据链路层扩展以太网🥚网桥的主要结构和基本工作原理🎈网桥的主要结构🔎网桥转发帧的例子🔎网桥丢弃帧的例子🔎网桥转发广播帧的例子 🥚透明网桥🔎透明网桥的…...
Ubuntu20.04 使用scrapy-splash爬取动态网页
我们要先安装splash服务,使用dock安装,如果dock没有安装,请参考我的上一篇博文: 按照官方文档:https://splash.readthedocs.io/en/stable/install.html 1.下载splash sudo docker pull scrapinghub/splash2.安装scrapy…...
Function:控制继电器上下电,上电后adb登录,copy配置文件
import serial import time import datetime import subprocess import osdef append_to_txt(file_path, content):if os.path.exists(file_path):with open(file_path, a) as file: # 使用 a 模式打开文件进行追加file.write(content \n) # 追加内容,并换行else…...
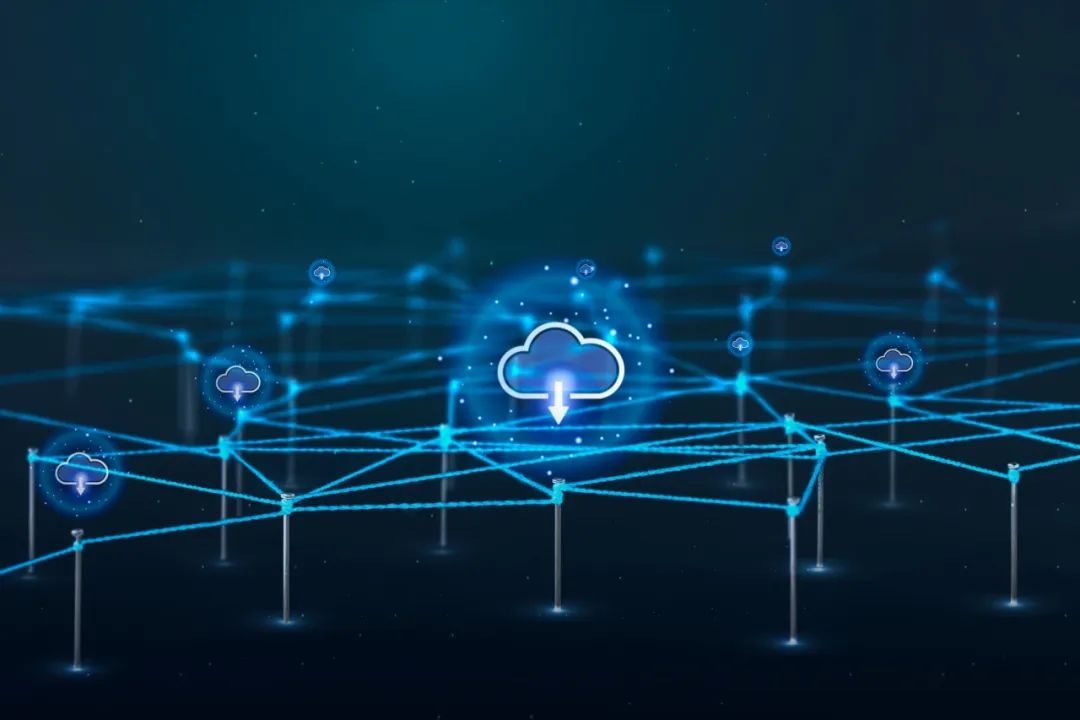
香港电讯高可用网络助力企业变革金融计算
客户背景 客户是一家金融行业知名的量化私募对冲基金公司,专注于股票、期权、期货、债券等主要投资市场,在量化私募管理深耕多年,目前资管规模已达数百亿级,在国内多个城市均设有办公地点。 客户需求 由于客户业务倚重量化技术…...
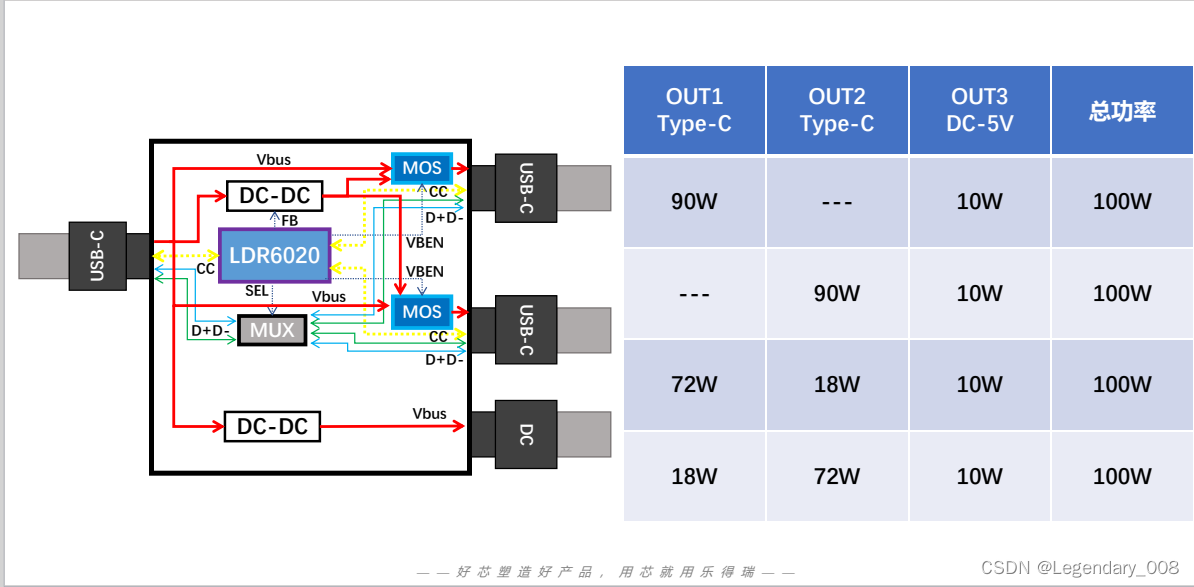
LDR6020一拖二快充线:多设备充电新选择
随着科技的快速发展,我们的日常生活中越来越多地依赖于智能设备。然而,每当手机、平板或其他移动设备电量告急时,我们总是需要寻找合适的充电线进行充电。为了解决这一痛点,市场上出现了一款备受瞩目的新产品——LDR6020一拖二快充…...
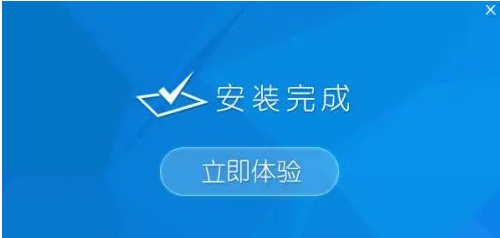
电脑ffmpeg.dll丢失原因解析,找不到ffmpeg.dll的5种解决方法
在数字化时代,多媒体文件的处理已经成为我们日常生活和工作中不可或缺的一部分。在计算机使用过程中,丢失ffmpeg.dll文件是一个特定但常见的问题,尤其是对于那些经常处理视频编解码任务的用户来说。下面小编讲全面分析ffmpeg.dll丢失原因以及…...
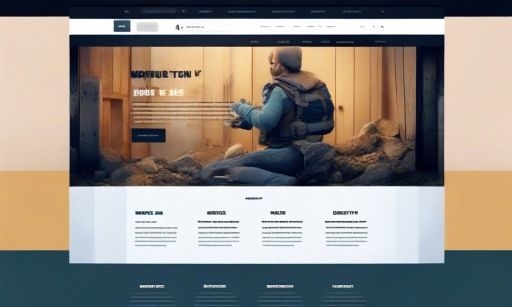
手机网站制作软件是哪些
手机网站制作软件是一种用于设计、开发和创建适用于移动设备的网站的软件工具。随着移动互联网时代的到来,越来越多的用户开始使用手机浏览网页和进行在线交流,因此,手机网站制作软件也逐渐成为了市场上的热门工具。 1. Adobe Dreamweaver&am…...
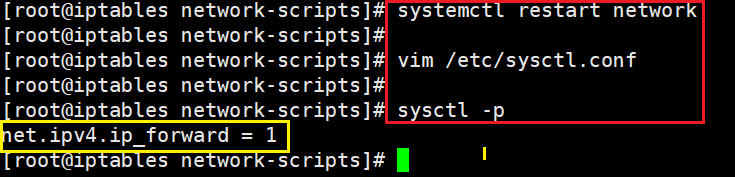
【Kubernetes项目部署】k8s集群+高可用、负载均衡+防火墙
项目架构图 (1)部署 kubernetes 集群 详见:http://t.csdnimg.cn/RLveS (2) 在 Kubernetes 环境中,通过yaml文件的方式,创建2个Nginx Pod分别放置在两个不同的节点上; Pod使用hostP…...
IPC工业电脑的现状、发展未来与破局策略
文章目录 全球工业电脑市场概况1.1 市场规模与增长1.2 区域分布与主要市场 工业电脑的技术发展与应用2.1 技术趋势与创新2.2 应用领域扩展2.3 工业自动化与智能化 竞争格局与市场参与者3.1 主要企业与市场竞争3.2 国内外竞争对比3.3 市场集中度与竞争策略 未来发展趋势与市场预…...
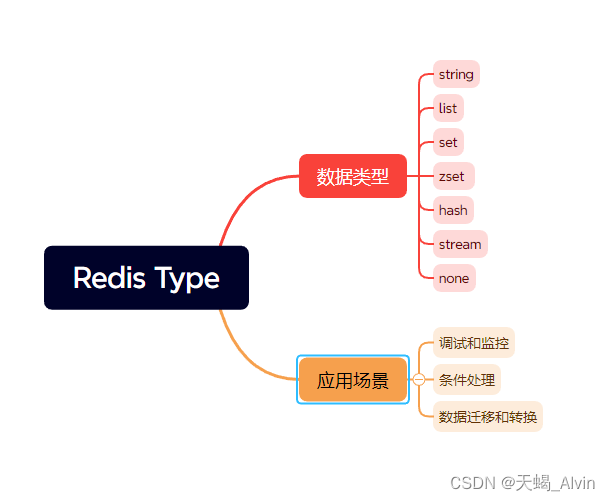
深入了解Redis的TYPE命令
Redis作为一个高性能的内存数据库,支持多种数据结构。在管理和操作Redis数据库时,了解键对应的数据类型是至关重要的。本文将深入探讨Redis的TYPE命令,它用于返回存储在指定键中的值的数据类型。 什么是TYPE命令? TYPE命令用于查…...
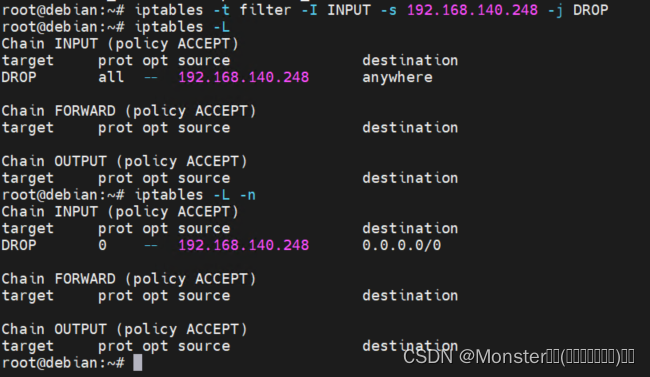
iptables(3)规则管理
简介 上一篇文章中,我们已经介绍了怎样使用iptables命令查看规则,那么这篇文章我们就来介绍一下,怎样管理规则,即对iptables进行”增、删、改”操作。 注意:在进行iptables实验时,请务必在个人的测试机上进行,不要再有任何业务的机器上进行测试。 在进行测试前,为保障…...
关于addEventListener的使用和注意项
一、addEventListener基本理解 addEventListener 是一个 JavaScript DOM 方法,用于向指定元素添加事件监听器。它接受三个参数: 事件类型:一个字符串,表示要监听的事件类型,如 ‘click’、‘mouseover’、‘keydown’…...
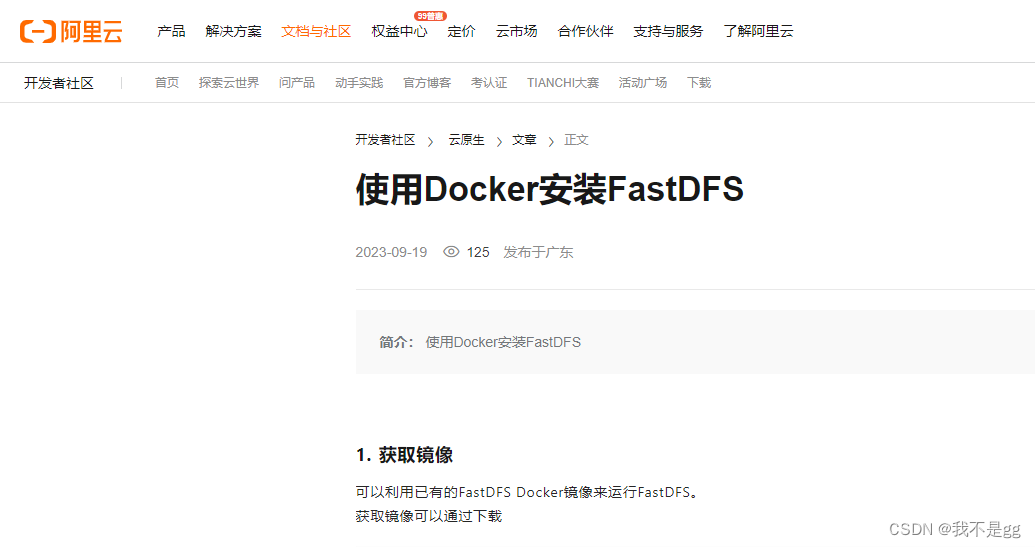
分享一下,如何搭建个人网站的步骤
在这段充满探索与创造的奇妙旅途中,我就像一位耐心的建筑师,在数字世界的荒原上精心雕琢,两周的时光缓缓流淌。每天,我与代码共舞,手执HTML、CSS与JavaScript这三大构建魔杖,一砖一瓦地筑起了梦想中的网络城…...
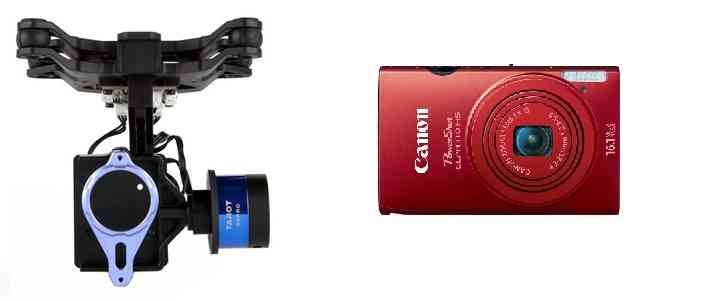
(7)摄像机和云台
文章目录 前言 1 云台 2 带有MAVLink接口的摄像机 3 相机控制和地理标签 4 视频质量差的常见修复方法 5 详细主题 前言 Copter、Plane 和 Rover 最多支持 3 轴云台,包括自动瞄准感兴趣区域(ROI)的相机和自动触发相机快门等先进功能。按…...
MicroBlaze IP核中的外设接口和缓冲器接口介绍
MicroBlaze IP核是Xilinx公司提供的一个嵌入式软核处理器,广泛应用于FPGA设计中。在MicroBlaze IP核中,外设接口和缓冲器接口是处理器与外部设备和内存交互的关键部分。 1 外设接口 MicroBlaze处理器中的AXI4 内存映射外设接口AXI4是一种在Xilinx FPGA设…...
Java数据结构与算法(完全背包)
前言: 完全背包问题是背包问题的一个变种,与0/1背包问题不同,在完全背包问题中,每种物品可以被选取多次。问题描述如下: 给定 n 件物品,每件物品有一个重量 wi和一个价值 vi,以及一个背包,它能…...
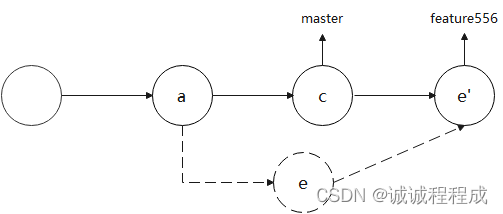
git merge(3个模式) 与 git rebase 图文详解区别
目录 1 git merge1.1 模式一:fast-forward(–ff)1.2 模式二:non-Fast-forward(–no-ff)1.3 模式三:fast-forward only(–ff-only) 2 git rebase3 区别 1 git merge git merge有好几种不同的模式 默认情况下你直接使用 git merge 命令&#x…...
Eclipse 工作空间:深入解析与高效使用
Eclipse 工作空间:深入解析与高效使用 Eclipse 是一款广受欢迎的集成开发环境(IDE),它为各种编程语言提供了强大的开发工具。在 Eclipse 中,工作空间(Workspace)是一个核心概念,它代表了一个项目的集合,这些项目共享相同的配置和设置。本文将深入探讨 Eclipse 工作空…...
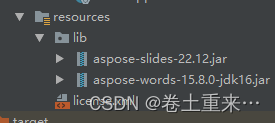
Aspose将doc,ppt转成pdf
1.需要引入的jar包 链接: https://pan.baidu.com/s/1t3wqq7KrHi50K9KX3-Eb9A?pwdu4se 提取码: u4se <dependency><groupId>com.aspose</groupId><artifactId>aspose-words-jdk16</artifactId><version>15.8.0</version><scop…...
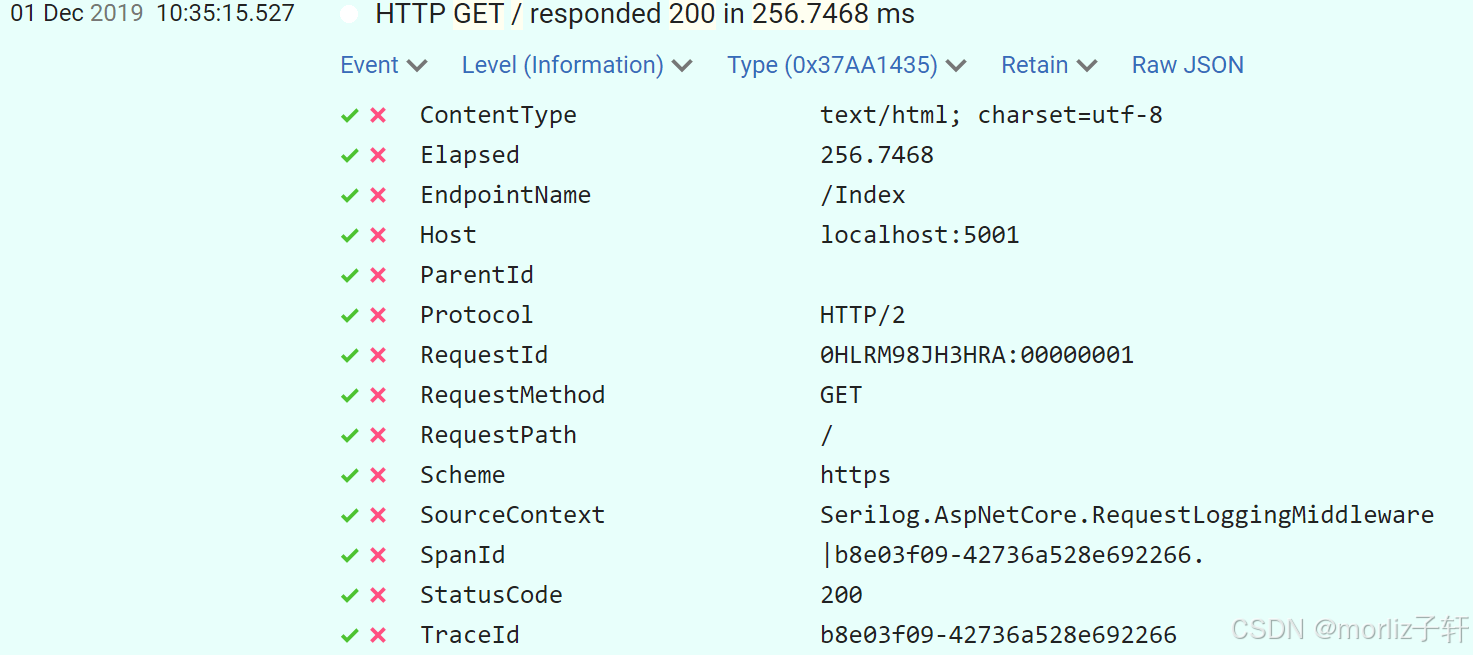
深入浅出Asp.Net Core MVC应用开发系列-AspNetCore中的日志记录
ASP.NET Core 是一个跨平台的开源框架,用于在 Windows、macOS 或 Linux 上生成基于云的新式 Web 应用。 ASP.NET Core 中的日志记录 .NET 通过 ILogger API 支持高性能结构化日志记录,以帮助监视应用程序行为和诊断问题。 可以通过配置不同的记录提供程…...
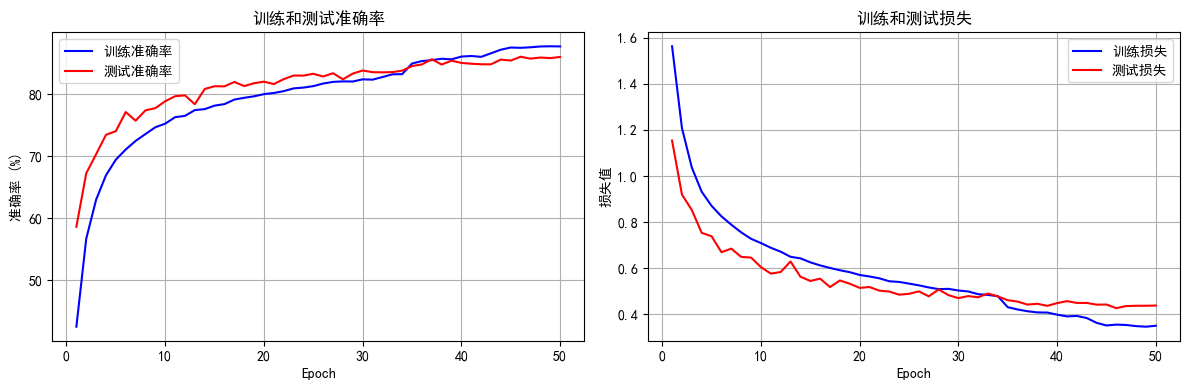
python打卡day49
知识点回顾: 通道注意力模块复习空间注意力模块CBAM的定义 作业:尝试对今天的模型检查参数数目,并用tensorboard查看训练过程 import torch import torch.nn as nn# 定义通道注意力 class ChannelAttention(nn.Module):def __init__(self,…...
Spring Boot 实现流式响应(兼容 2.7.x)
在实际开发中,我们可能会遇到一些流式数据处理的场景,比如接收来自上游接口的 Server-Sent Events(SSE) 或 流式 JSON 内容,并将其原样中转给前端页面或客户端。这种情况下,传统的 RestTemplate 缓存机制会…...
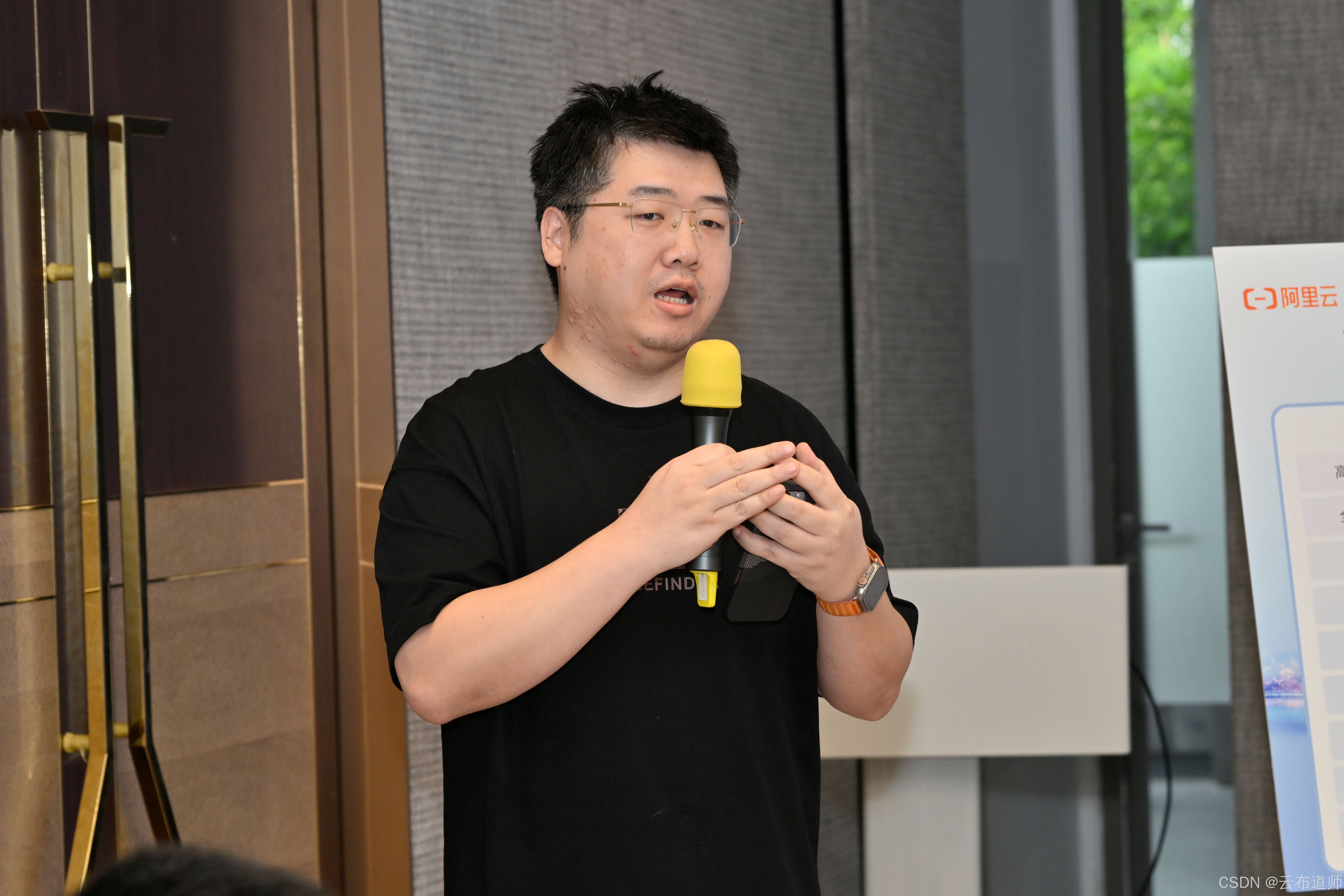
云启出海,智联未来|阿里云网络「企业出海」系列客户沙龙上海站圆满落地
借阿里云中企出海大会的东风,以**「云启出海,智联未来|打造安全可靠的出海云网络引擎」为主题的阿里云企业出海客户沙龙云网络&安全专场于5.28日下午在上海顺利举办,现场吸引了来自携程、小红书、米哈游、哔哩哔哩、波克城市、…...
深入浅出:JavaScript 中的 `window.crypto.getRandomValues()` 方法
深入浅出:JavaScript 中的 window.crypto.getRandomValues() 方法 在现代 Web 开发中,随机数的生成看似简单,却隐藏着许多玄机。无论是生成密码、加密密钥,还是创建安全令牌,随机数的质量直接关系到系统的安全性。Jav…...
JVM垃圾回收机制全解析
Java虚拟机(JVM)中的垃圾收集器(Garbage Collector,简称GC)是用于自动管理内存的机制。它负责识别和清除不再被程序使用的对象,从而释放内存空间,避免内存泄漏和内存溢出等问题。垃圾收集器在Ja…...
大语言模型如何处理长文本?常用文本分割技术详解
为什么需要文本分割? 引言:为什么需要文本分割?一、基础文本分割方法1. 按段落分割(Paragraph Splitting)2. 按句子分割(Sentence Splitting)二、高级文本分割策略3. 重叠分割(Sliding Window)4. 递归分割(Recursive Splitting)三、生产级工具推荐5. 使用LangChain的…...
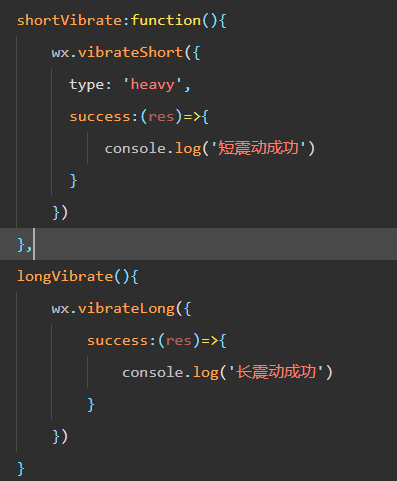
微信小程序 - 手机震动
一、界面 <button type"primary" bindtap"shortVibrate">短震动</button> <button type"primary" bindtap"longVibrate">长震动</button> 二、js逻辑代码 注:文档 https://developers.weixin.qq…...
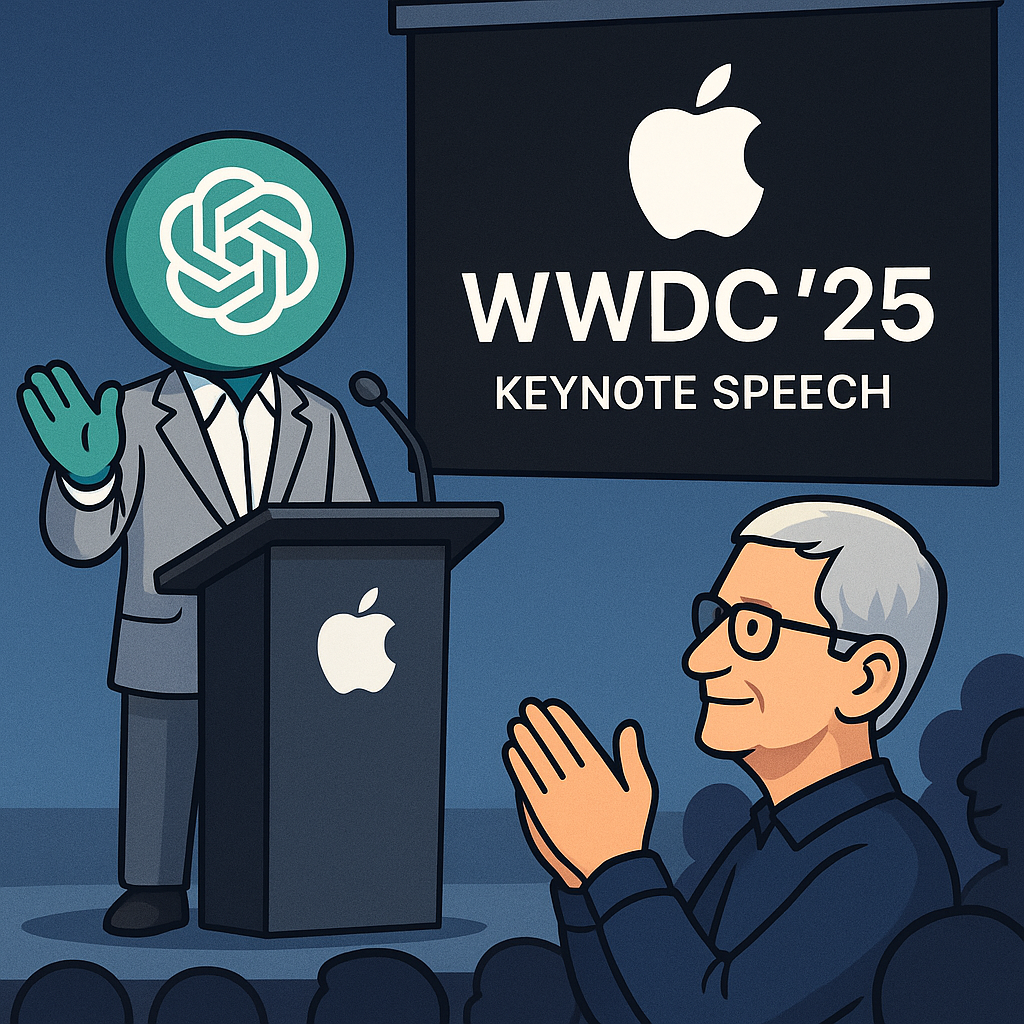
对WWDC 2025 Keynote 内容的预测
借助我们以往对苹果公司发展路径的深入研究经验,以及大语言模型的分析能力,我们系统梳理了多年来苹果 WWDC 主题演讲的规律。在 WWDC 2025 即将揭幕之际,我们让 ChatGPT 对今年的 Keynote 内容进行了一个初步预测,聊作存档。等到明…...
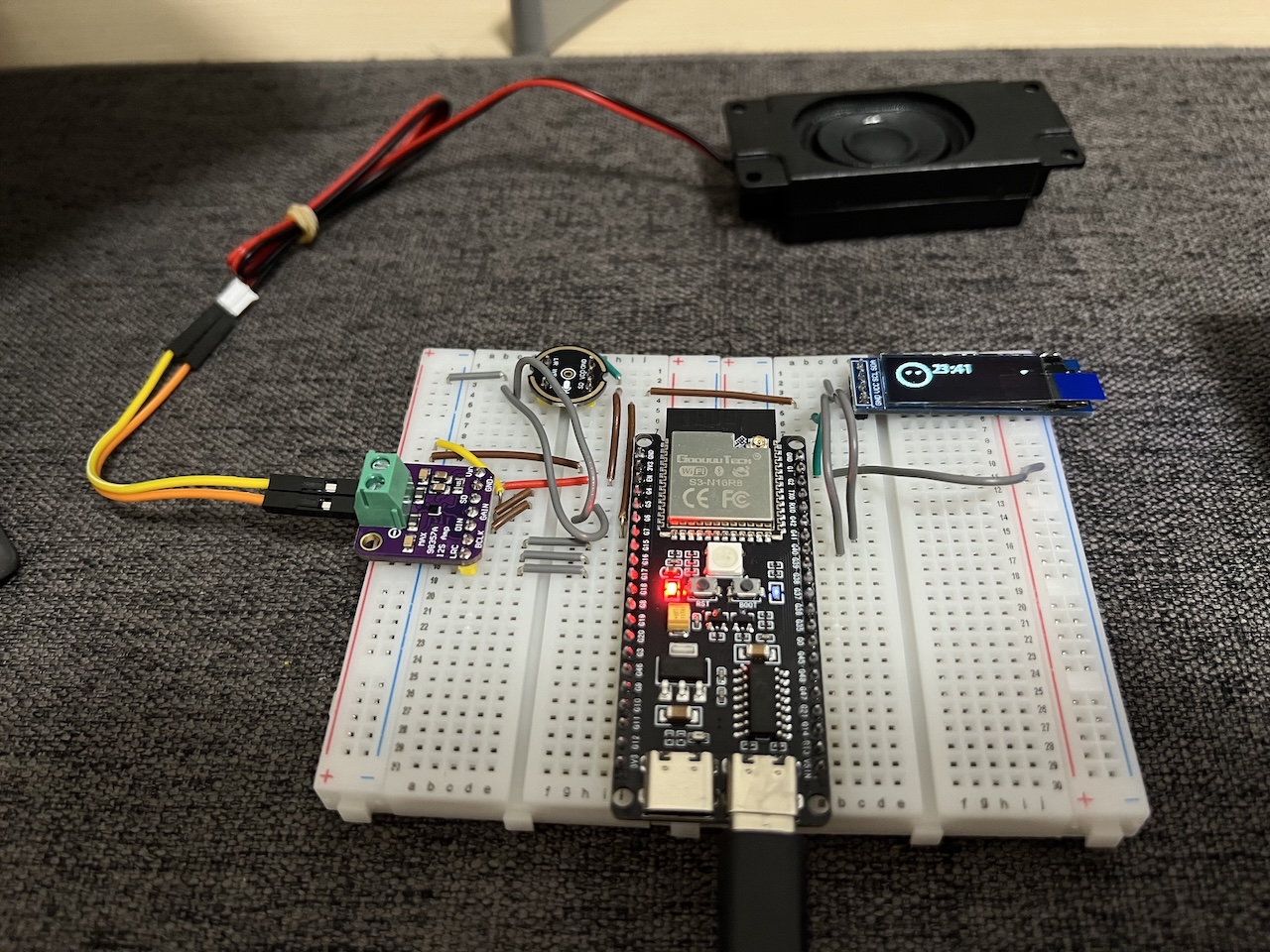
DIY|Mac 搭建 ESP-IDF 开发环境及编译小智 AI
前一阵子在百度 AI 开发者大会上,看到基于小智 AI DIY 玩具的演示,感觉有点意思,想着自己也来试试。 如果只是想烧录现成的固件,乐鑫官方除了提供了 Windows 版本的 Flash 下载工具 之外,还提供了基于网页版的 ESP LA…...