PyTorch nn.CrossEntropyLoss() 交叉熵损失函数详解和要点提醒
文章目录
- 前置知识
- nn.CrossEntropyLoss() 交叉熵损失
- 参数
- 数学公式
- 带权重的公式(weight)
- 标签平滑(label_smoothing)
- 要点
- 附录
- 参考链接
前置知识
深度学习:关于损失函数的一些前置知识(PyTorch Loss)
nn.CrossEntropyLoss() 交叉熵损失
torch.nn.CrossEntropyLoss(weight=None, size_average=None, ignore_index=-100, reduce=None, reduction='mean', label_smoothing=0.0)
This criterion computes the cross entropy loss between input logits and target.
该函数计算输入 logits 和目标之间的交叉熵损失。
参数
- weight (Tensor, 可选): 一个形状为 ( C ) (C) (C) 的张量,表示每个类别的权重。如果提供了这个参数,损失函数会根据类别的权重来调整各类别的损失,适用于类别不平衡的问题。默认值是
None
。 - size_average (bool, 可选): 已弃用。如果
reduction
不是'none'
,则默认情况下损失是取平均(True
);否则,是求和(False
)。默认值是None
。 - ignore_index (int, 可选): 如果指定了这个参数,则该类别的索引会被忽略,不会对损失和梯度产生影响。默认值是
-100
。 - reduce (bool, 可选): 已弃用。请使用
reduction
参数。默认值是None
。 - reduction (str, 可选): 指定应用于输出的归约方式。可选值为
'none'
、'mean'
、'sum'
。'none'
表示不进行归约,'mean'
表示对所有样本的损失求平均,'sum'
表示对所有样本的损失求和。默认值是'mean'
。 - label_smoothing (float, 可选): 标签平滑值,范围在 [0.0, 1.0] 之间。默认值是
0.0
。标签平滑是一种正则化技术,通过在真实标签上添加一定程度的平滑来避免过拟合。
数学公式
附录部分会验证下述公式和代码的一致性。
假设有 N N N 个样本,每个样本属于 C C C 个类别之一。对于第 i i i 个样本,它的真实类别标签为 y i y_i yi,模型的输出 logits 为 x i = ( x i 1 , x i 2 , … , x i C ) \mathbf{x}_i = (x_{i1}, x_{i2}, \ldots, x_{iC}) xi=(xi1,xi2,…,xiC),其中 x i c x_{ic} xic 表示第 i i i 个样本在第 c c c 类别上的原始输出分数(logits)。
交叉熵损失的计算步骤如下:
- Softmax 函数:
对 logits 进行 softmax 操作,将其转换为概率分布:
p i c = exp ( x i c ) ∑ j = 1 C exp ( x i j ) p_{ic} = \frac{\exp(x_{ic})}{\sum_{j=1}^{C} \exp(x_{ij})} pic=∑j=1Cexp(xij)exp(xic)
其中 $ p_{ic} $ 表示第 $ i $ 个样本属于第 $ c $ 类别的预测概率。 - 负对数似然(Negative Log-Likelihood):
计算负对数似然:
ℓ i = − log ( p i y i ) \ell_i = -\log(p_{iy_i}) ℓi=−log(piyi)
其中 ℓ i \ell_i ℓi 是第 i i i 个样本的损失, p i y i p_{iy_i} piyi 表示第 i i i 个样本在真实类别 y i y_i yi 上的预测概率。 - 总损失:
计算所有样本的平均损失(reduction
参数默认为'mean'
):
L = 1 N ∑ i = 1 N ℓ i = 1 N ∑ i = 1 N − log ( p i y i ) \mathcal{L} = \frac{1}{N} \sum_{i=1}^{N} \ell_i = \frac{1}{N} \sum_{i=1}^{N} -\log(p_{iy_i}) L=N1i=1∑Nℓi=N1i=1∑N−log(piyi)
如果reduction
参数为'sum'
,总损失为所有样本损失的和:
L = ∑ i = 1 N ℓ i = ∑ i = 1 N − log ( p i y i ) \mathcal{L} = \sum_{i=1}^{N} \ell_i = \sum_{i=1}^{N} -\log(p_{iy_i}) L=i=1∑Nℓi=i=1∑N−log(piyi)
如果reduction
参数为'none'
,则返回每个样本的损失 ℓ i \ell_i ℓi 组成的张量。
L = [ ℓ 1 , ℓ 2 , … , ℓ N ] = [ − log ( p i y 1 ) , − log ( p i y 2 ) , … , − log ( p i y N ) ] \mathcal{L} = [\ell_1, \ell_2, \ldots, \ell_N] = [-\log(p_{iy_1}), -\log(p_{iy_2}), \ldots, -\log(p_{iy_N})] L=[ℓ1,ℓ2,…,ℓN]=[−log(piy1),−log(piy2),…,−log(piyN)]
带权重的公式(weight)
如果指定了类别权重 w = ( w 1 , w 2 , … , w C ) \mathbf{w} = (w_1, w_2, \ldots, w_C) w=(w1,w2,…,wC),则总损失公式为:
L = 1 N ∑ i = 1 N w y i ⋅ ℓ i = ∑ i = 1 N w y i ⋅ ( − log ( p i y i ) ) ∑ i = 1 N w y i \mathcal{L} = \frac{1}{N} \sum_{i=1}^{N} w_{y_i} \cdot \ell_i = \frac{\sum_{i=1}^{N} w_{y_i} \cdot (-\log(p_{iy_i}))}{\sum_{i=1}^{N} w_{y_i}} L=N1i=1∑Nwyi⋅ℓi=∑i=1Nwyi∑i=1Nwyi⋅(−log(piyi))
其中 w y i w_{y_i} wyi 是第 i i i 个样本真实类别的权重。
标签平滑(label_smoothing)
如果标签平滑(label smoothing)参数 α \alpha α 被启用,目标标签 y i \mathbf{y}_i yi 会被平滑处理:
y i ′ = ( 1 − α ) ⋅ y i + α C \mathbf{y}_i' = (1 - \alpha) \cdot \mathbf{y}_i + \frac{\alpha}{C} yi′=(1−α)⋅yi+Cα
其中, y i \mathbf{y}_i yi 是原始的 one-hot 编码目标标签, y i ′ \mathbf{y}_i' yi′ 是平滑后的标签。
总的损失公式会相应调整:
ℓ i = − ∑ c = 1 C y i c ′ ⋅ log ( p i c ) \ell_i = - \sum_{c=1}^{C} y_{ic}' \cdot \log(p_{ic}) ℓi=−c=1∑Cyic′⋅log(pic)
其中, y i c y_{ic} yic 是第 i i i 个样本在第 c c c 类别上的标签,为原标签 y i y_i yi 经过 one-hot 编码后 y i \mathbf{y}_i yi 中的值。对于一个 one-hot 编码标签向量, y i c y_{ic} yic 在样本属于类别 c c c 时为 1,否则为 0。
要点
nn.CrossEntropyLoss()
接受的输入是 logits,这说明分类的输出不需要提前经过 softmax。如果提前经过 softmax,则需要使用nn.NLLLoss()
(负对数似然损失)。import torch import torch.nn as nn import torch.nn.functional as F# 定义输入和目标标签 logits = torch.tensor([[2.0, 0.5], [0.5, 2.0]]) # 未经过 softmax 的 logits target = torch.tensor([0, 1]) # 目标标签# 使用 nn.CrossEntropyLoss 计算损失(接受 logits) criterion_ce = nn.CrossEntropyLoss() loss_ce = criterion_ce(logits, target)# 使用 softmax 后再使用 nn.NLLLoss 计算损失 log_probs = F.log_softmax(logits, dim=1) criterion_nll = nn.NLLLoss() loss_nll = criterion_nll(log_probs, target)print(f"Loss using nn.CrossEntropyLoss: {loss_ce.item()}") print(f"Loss using softmax + nn.NLLLoss: {loss_nll.item()}")# 验证两者是否相等 assert torch.allclose(loss_ce, loss_nll), "The losses are not equal, which indicates a mistake in the assumption." print("The losses are equal, indicating that nn.CrossEntropyLoss internally applies softmax.")
拓展: F.log_softmax()>>> Loss using nn.CrossEntropyLoss: 0.2014133334159851 >>> Loss using softmax + nn.NLLLoss: 0.2014133334159851 >>> The losses are equal, indicating that nn.CrossEntropyLoss internally applies softmax.
F.log_softmax
等价于先应用softmax
激活函数,然后对结果取对数 log()。它是将softmax
和log
这两个操作结合在一起,以提高数值稳定性和计算效率。具体的数学定义如下:
log_softmax ( x i ) = log ( softmax ( x i ) ) = log ( exp ( x i ) ∑ j exp ( x j ) ) = x i − log ( ∑ j exp ( x j ) ) \text{log\_softmax}(x_i) = \log\left(\text{softmax}(x_i)\right) = \log\left(\frac{\exp(x_i)}{\sum_j \exp(x_j)}\right) = x_i - \log\left(\sum_j \exp(x_j)\right) log_softmax(xi)=log(softmax(xi))=log(∑jexp(xj)exp(xi))=xi−log(j∑exp(xj))
在代码中,F.log_softmax
的等价操作可以用以下步骤实现:- 计算
softmax
。 - 计算
softmax
的结果的对数。
import torch import torch.nn.functional as F# 定义输入 logits logits = torch.tensor([[2.0, 1.0, 0.1], [1.0, 3.0, 0.2]])# 计算 log_softmax log_softmax_result = F.log_softmax(logits, dim=1)# 分开计算 softmax 和 log softmax_result = F.softmax(logits, dim=1) log_result = torch.log(softmax_result)print("Logits:") print(logits)print("\nLog softmax (using F.log_softmax):") print(log_softmax_result)print("\nSoftmax result:") print(softmax_result)print("\nLog of softmax result:") print(log_result)# 验证两者是否相等 assert torch.allclose(log_softmax_result, log_result), "The results are not equal." print("\nThe results are equal, indicating that F.log_softmax is equivalent to softmax followed by log.")
从结果中可以看到>>> Logits: >>> tensor([[2.0000, 1.0000, 0.1000], >>> [1.0000, 3.0000, 0.2000]])>>> Log softmax (using F.log_softmax): >>> tensor([[-0.4170, -1.4170, -2.3170], >>> [-2.1791, -0.1791, -2.9791]])>>> Softmax result: >>> tensor([[0.6590, 0.2424, 0.0986], >>> [0.1131, 0.8360, 0.0508]])>>> Log of softmax result: >>> tensor([[-0.4170, -1.4170, -2.3170], >>> [-2.1791, -0.1791, -2.9791]])>>> The results are equal, indicating that F.log_softmax is equivalent to softmax followed by log.
F.log_softmax
的结果等价于先计算 softmax 再取对数。- 计算
nn.CrossEntropyLoss()
实际上默认(reduction=‘mean’)计算的是每个样本的平均损失,已经做了归一化处理,所以不需要对得到的结果进一步除以 batch_size 或其他某个数,除非是用作 loss_weight。下面是一个简单的例子:import torch import torch.nn as nn# 定义损失函数 criterion = nn.CrossEntropyLoss()# 定义输入和目标标签 input1 = torch.tensor([[2.0, 0.5], [0.5, 2.0]], requires_grad=True) # 批量大小为 2 target1 = torch.tensor([0, 1]) # 对应的目标标签input2 = torch.tensor([[2.0, 0.5], [0.5, 2.0], [2.0, 0.5], [0.5, 2.0]], requires_grad=True) # 批量大小为 4 target2 = torch.tensor([0, 1, 0, 1]) # 对应的目标标签# 计算损失 loss1 = criterion(input1, target1) loss2 = criterion(input2, target2)print(f"Loss with batch size 2: {loss1.item()}") print(f"Loss with batch size 4: {loss2.item()}")
可以看到这里的>>> Loss with batch size 2: 0.2014133334159851 >>> Loss with batch size 4: 0.2014133334159851
input2
实际上等价于torch.cat([input1, input1], dim=0)
,target2
等价于torch.cat([target1, target1], dim=0)
,简单拓展了 batch_size 大小但最终的 Loss 没变,这也就验证了之前的说法。- 目标标签
target
期望两种格式:-
类别索引: 类别的整数索引,而不是 one-hot 编码。范围在 [ 0 , C ) [0, C) [0,C) 之间,其中 C C C 是类别数。如果指定了
ignore_index
,则该类别索引也会被接受(即便可能不在类别范围内)
使用示例:# Example of target with class indices import torch import torch.nn as nnloss = nn.CrossEntropyLoss() input = torch.randn(3, 5, requires_grad=True) target = torch.empty(3, dtype=torch.long).random_(5) output = loss(input, target) output.backward()
-
类别概率: 类别的概率分布,适用于需要每个批次项有多个类别标签的情况,如标签平滑等。
使用示例:# Example of target with class probabilities import torch import torch.nn as nnloss = nn.CrossEntropyLoss() input = torch.randn(3, 5, requires_grad=True) target = torch.randn(3, 5).softmax(dim=1) output = loss(input, target) output.backward()
The performance of this criterion is generally better when target contains class indices, as this allows for optimized computation. Consider providing target as class probabilities only when a single class label per minibatch item is too restrictive.
通常情况下,当目标为类别索引时,该函数的性能更好,因为这样可以进行优化计算。只有在每个批次项的单一类别标签过于限制时,才考虑使用类别概率。
-
附录
用于验证数学公式和函数实际运行的一致性
import torch
import torch.nn.functional as F# 假设有两个样本,每个样本有三个类别
logits = torch.tensor([[1.5, 2.0, 0.5], [1.0, 0.5, 2.5]], requires_grad=True)
targets = torch.tensor([1, 2])# 根据公式实现 softmax
def softmax(x):return torch.exp(x) / torch.exp(x).sum(dim=1, keepdim=True)# 根据公式实现 log-softmax
def log_softmax(x):return x - torch.log(torch.exp(x).sum(dim=1, keepdim=True))# 根据公式实现负对数似然损失(NLLLoss)
def nll_loss(log_probs, targets):N = log_probs.size(0)return -log_probs[range(N), targets].mean()# 根据公式实现交叉熵损失
def custom_cross_entropy(logits, targets):log_probs = log_softmax(logits)return nll_loss(log_probs, targets)# 使用 PyTorch 计算交叉熵损失
criterion = torch.nn.CrossEntropyLoss(reduction='mean')
loss_torch = criterion(logits, targets)# 使用根据公式实现的交叉熵损失
loss_custom = custom_cross_entropy(logits, targets)# 打印结果
print("PyTorch 计算的交叉熵损失:", loss_torch.item())
print("根据公式实现的交叉熵损失:", loss_custom.item())# 验证结果是否相等
assert torch.isclose(loss_torch, loss_custom), "数学公式验证失败"# 带权重的交叉熵损失
weights = torch.tensor([0.7, 0.2, 0.1])
criterion_weighted = torch.nn.CrossEntropyLoss(weight=weights, reduction='mean')
loss_weighted_torch = criterion_weighted(logits, targets)# 根据公式实现带权重的交叉熵损失
def custom_weighted_cross_entropy(logits, targets, weights):log_probs = log_softmax(logits)N = logits.size(0)weighted_loss = -log_probs[range(N), targets] * weights[targets]return weighted_loss.sum() / weights[targets].sum()loss_weighted_custom = custom_weighted_cross_entropy(logits, targets, weights)# 打印结果
print("PyTorch 计算的带权重的交叉熵损失:", loss_weighted_torch.item())
print("根据公式实现的带权重的交叉熵损失:", loss_weighted_custom.item())# 验证结果是否相等
assert torch.isclose(loss_weighted_torch, loss_weighted_custom, atol=1e-6), "带权重的数学公式验证失败"# 标签平滑的交叉熵损失
alpha = 0.1
criterion_label_smoothing = torch.nn.CrossEntropyLoss(label_smoothing=alpha, reduction='mean')
loss_label_smoothing_torch = criterion_label_smoothing(logits, targets)# 根据公式实现标签平滑的交叉熵损失
def custom_label_smoothing_cross_entropy(logits, targets, alpha):N, C = logits.size()log_probs = log_softmax(logits)one_hot = torch.zeros_like(log_probs).scatter(1, targets.view(-1, 1), 1)smooth_targets = (1 - alpha) * one_hot + alpha / Closs = - (smooth_targets * log_probs).sum(dim=1).mean()return lossloss_label_smoothing_custom = custom_label_smoothing_cross_entropy(logits, targets, alpha)# 打印结果
print("PyTorch 计算的标签平滑的交叉熵损失:", loss_label_smoothing_torch.item())
print("根据公式实现的标签平滑的交叉熵损失:", loss_label_smoothing_custom.item())# 验证结果是否相等
assert torch.isclose(loss_label_smoothing_torch, loss_label_smoothing_custom, atol=1e-6), "标签平滑的数学公式验证失败"
>>> PyTorch 计算的交叉熵损失: 0.45524317026138306
>>> 根据公式实现的交叉熵损失: 0.4552431106567383
>>> PyTorch 计算的带权重的交叉熵损失: 0.5048722624778748
>>> 根据公式实现的带权重的交叉熵损失: 0.50487220287323
>>> PyTorch 计算的标签平滑的交叉熵损失: 0.5469098091125488
>>> 根据公式实现的标签平滑的交叉熵损失: 0.5469098091125488
输出没有抛出 AssertionError,验证通过。
参考链接
CrossEntropyLoss - Docs
相关文章:

PyTorch nn.CrossEntropyLoss() 交叉熵损失函数详解和要点提醒
文章目录 前置知识nn.CrossEntropyLoss() 交叉熵损失参数数学公式带权重的公式(weight)标签平滑(label_smoothing) 要点 附录参考链接 前置知识 深度学习:关于损失函数的一些前置知识(PyTorch Loss&#x…...

DW学习笔记|数学建模task2
本章主要涉及到的知识点有: 微分方程的解法如何用 Python 解微分方程偏微分方程及其求解方法微分方程的基本案例差分方程的求解数值计算方法元胞自动机 2.1 微分方程的理论基础 微分方程是什么?如果你参加过高考,可能在高三备考中遇到过这…...
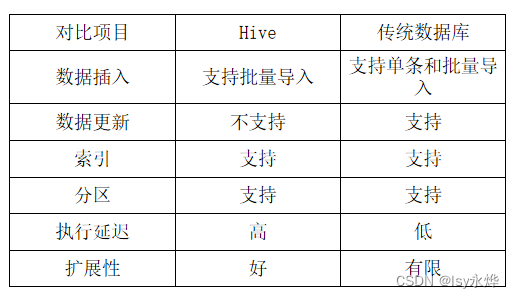
【大数据 复习】第9章 数据仓库分析工具Hive
一、概念 1.概述 (1)Hive是一个构建于Hadoop顶层的数据仓库工具。 (2)某种程度上可以看作是用户编程接口,本身不存储和处理数据。 (3)依赖分布式文件系统HDFS存储数据。 (4…...
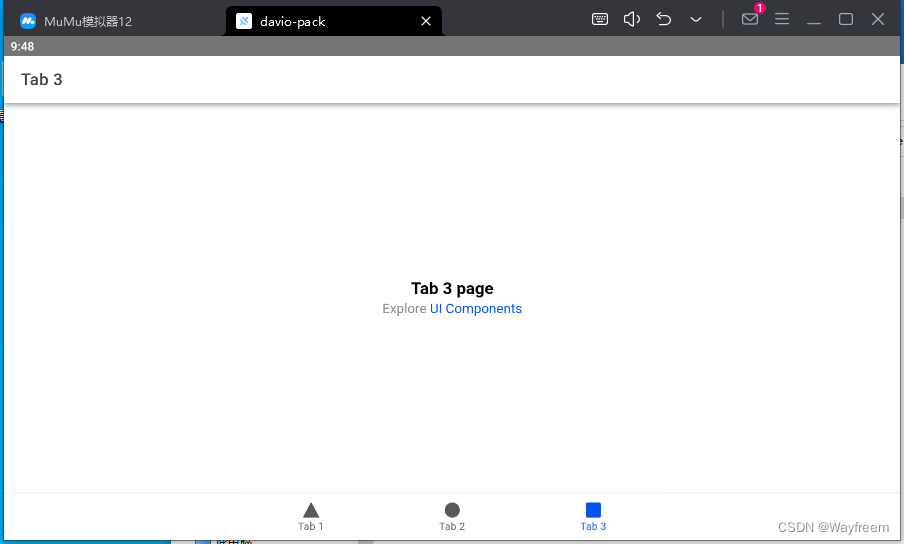
ionic7 从安装 到 项目启动最后打包成 apk
报错处理 在打包的时候遇到过几个问题,这里记录下来两个 Visual Studio Code运行ionic build出错显示ionic : 无法加载文件 ionic 项目通过 android studio 打开报错 capacitor.settings.gradle 文件不存在 说明 由于之前使用的是 ionic 3,当时打包的…...
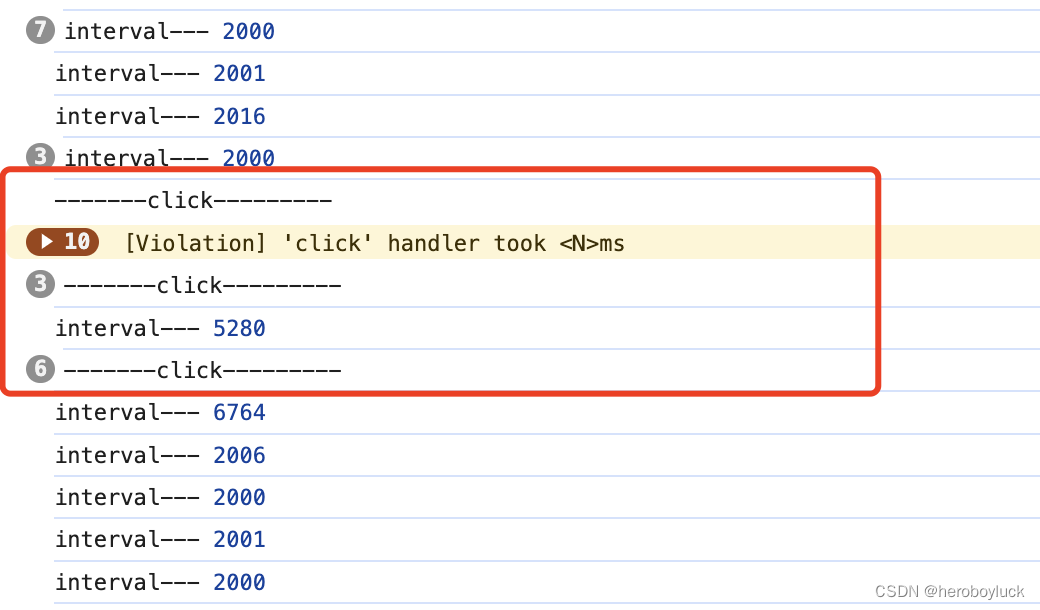
setInterval 定时任务执行时间不准验证
一般在处理定时任务的时候都使用setInterval间隔定时调用任务。 setInterval(() > {console.log("interval"); }, 2 * 1000);我们定义的是两秒执行一次,但是浏览器实际执行的间隔时间只多不少。这是由于浏览器执行 JS 是单线程模式,使用se…...

Stable Diffusion Model网站
Civitai Models | Discover Free Stable Diffusion Modelshttps://www.tjsky.net/tutorial/488https://zhuanlan.zhihu.com/p/610298913超详细的 Stable Diffusion ComfyUI 基础教程(一):安装与常用插件 - 优设网 - 学设计上优设 (uisdc.com)…...
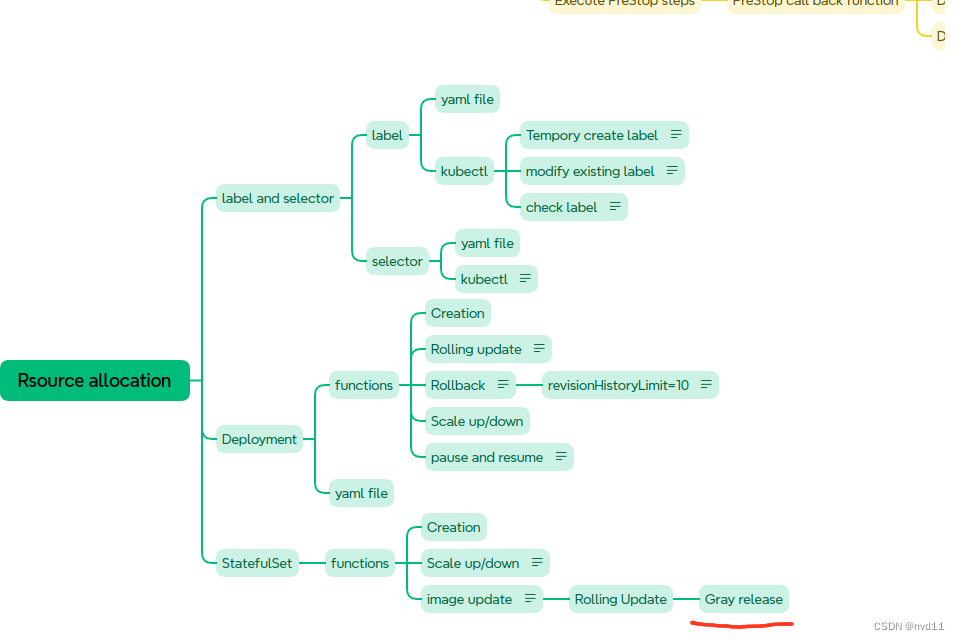
K8S - 实现statefulset 有状态service的灰度发布
什么是灰度发布 Canary Release 参考 理解 什么是 滚动更新,蓝绿部署,灰度发布 以及它们的区别 配置partition in updateStrategy/rollingUpdate 这次我为修改了 statefulset 的1个yaml file statefulsets/stateful-nginx-without-pvc.yaml: --- apiVe…...

Qt 技术博客:深入理解 Qt 中的 delete 和 deleteLater 与信号槽机制
在 Qt 开发中,内存管理和对象生命周期的处理是至关重要的一环。特别是在涉及信号和槽机制时,如何正确删除对象会直接影响应用程序的稳定性。本文将详细讨论在使用 Qt 的信号和槽机制时,delete 和 deleteLater 的工作原理,并给出最…...
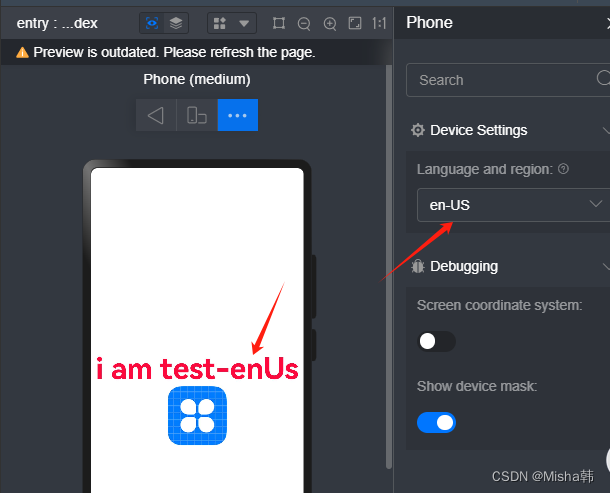
自学鸿蒙HarmonyOS的ArkTS语言<一>基本语法
一、一个ArkTs的目录结构 二、一个页面的结构 A、装饰器 Entry 装饰器 : 标记组件为入口组件,一个页面由多个自定义组件组成,但是只能有一个组件被标记 Component : 自定义组件, 仅能装饰struct关键字声明的数据结构 State:组件中的状态变量…...

【OpenGauss源码学习 —— (ALTER TABLE(列存修改列类型))】
ALTER TABLE(列存修改列类型) ATExecAlterColumnType 函数1. 检查和处理列存储表的字符集:2. 处理自动递增列的数据类型检查:3. 处理生成列的类型转换检查:4. 处理生成列的数据类型转换: build_column_defa…...
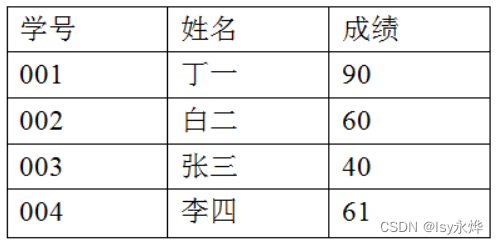
【大数据 复习】第7章 MapReduce(重中之重)
一、概念 1.MapReduce 设计就是“计算向数据靠拢”,而不是“数据向计算靠拢”,因为移动,数据需要大量的网络传输开销。 2.Hadoop MapReduce是分布式并行编程模型MapReduce的开源实现。 3.特点 (1)非共享式,…...

Zookeeper:节点
文章目录 一、节点类型二、监听器及节点删除三、创建节点四、监听节点变化五、判断节点是否存在 一、节点类型 持久(Persistent):客户端和服务器端断开连接后,创建的节点不删除。 持久化目录节点:客户端与Zookeeper断…...

生产级别的 vue
生产级别的 vue 拆分组件的标识更好的组织你的目录如何解决 props-base 设计的问题transparent component (透明组件)可减缓上述问题provide 和 inject vue-meta 在路由中的使用如何确保用户导航到某个路由自己都重新渲染?测试最佳实践如何制…...

kafka(五)spring-kafka(1)集成方法
一、集成 1、pom依赖 <!--kafka--><dependency><groupId>org.apache.kafka</groupId><artifactId>kafka-clients</artifactId></dependency><dependency><groupId>org.springframework.kafka</groupId><artif…...

Java中的设计模式深度解析
Java中的设计模式深度解析 大家好,我是免费搭建查券返利机器人省钱赚佣金就用微赚淘客系统3.0的小编,也是冬天不穿秋裤,天冷也要风度的程序猿! 在软件开发领域,设计模式是一种被广泛应用的经验总结和解决方案&#x…...
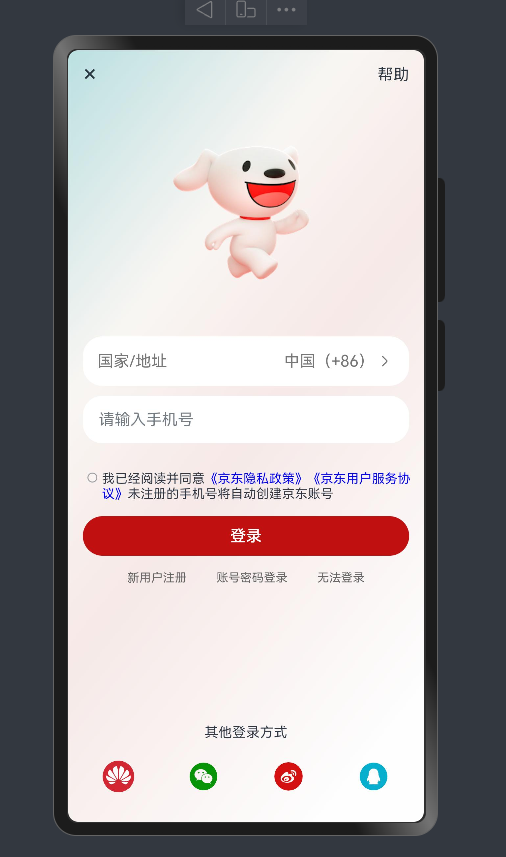
鸿蒙 HarmonyOS NEXT星河版APP应用开发—上篇
一、鸿蒙开发环境搭建 DevEco Studio安装 下载 访问官网:https://developer.huawei.com/consumer/cn/deveco-studio/选择操作系统版本后并注册登录华为账号既可下载安装包 安装 建议:软件和依赖安装目录不要使用中文字符软件安装包下载完成后࿰…...
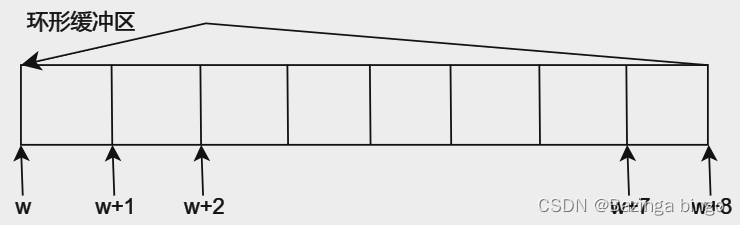
[FreeRTOS 基础知识] 互斥访问与回环队列 概念
文章目录 为什么需要互斥访问?使用队列实现互斥访问休眠和唤醒机制环形缓冲区 为什么需要互斥访问? 在裸机中,假设有两个函数(func_A, func_B)都要修改a的值(a),那么将a定义为全局变…...
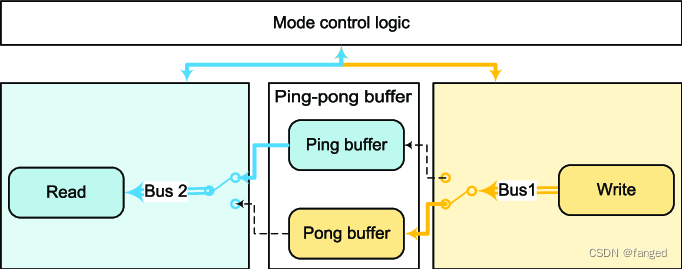
音视频的Buffer处理
最近在做安卓下UVC的一个案子。正好之前搞过ST方案的开机广告,这个也是我少数最后没搞成功的项目。当时也有点客观原因,当时ST要退出机顶盒市场,所以一切的支持都停了,当时啃他家播放器几十万行的代码,而且几乎没有文档…...

【总结】攻击 AI 模型的方法
数据投毒 污染训练数据 后门攻击 通过设计隐蔽的触发器,使得模型在正常测试时无异常,而面对触发器样本时被操纵输出。后门攻击可以看作是特殊的数据投毒,但是也可以通过修改模型参数来实现 对抗样本 只对输入做微小的改动,使模型…...
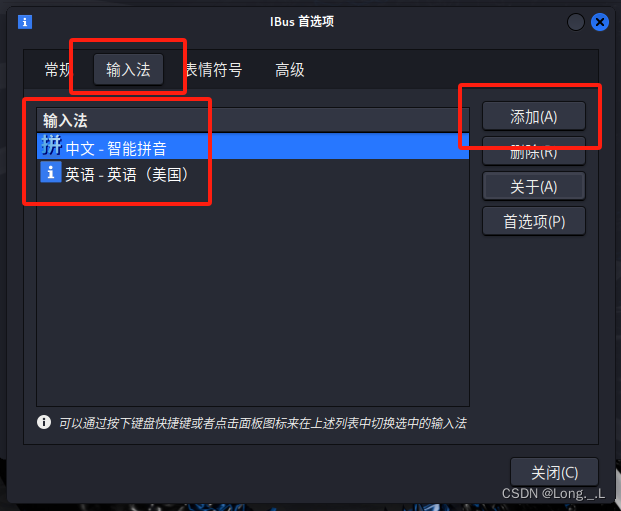
Linux配置中文环境
文章目录 前言中文语言包中文输入法中文字体 前言 在Linux系统中修改为中文环境,通常涉及以下几个步骤: 中文语言包 更新源列表: 更新系统的软件源列表和语言环境设置,确保可以安装所需的语言包。 sudo apt update sudo apt ins…...
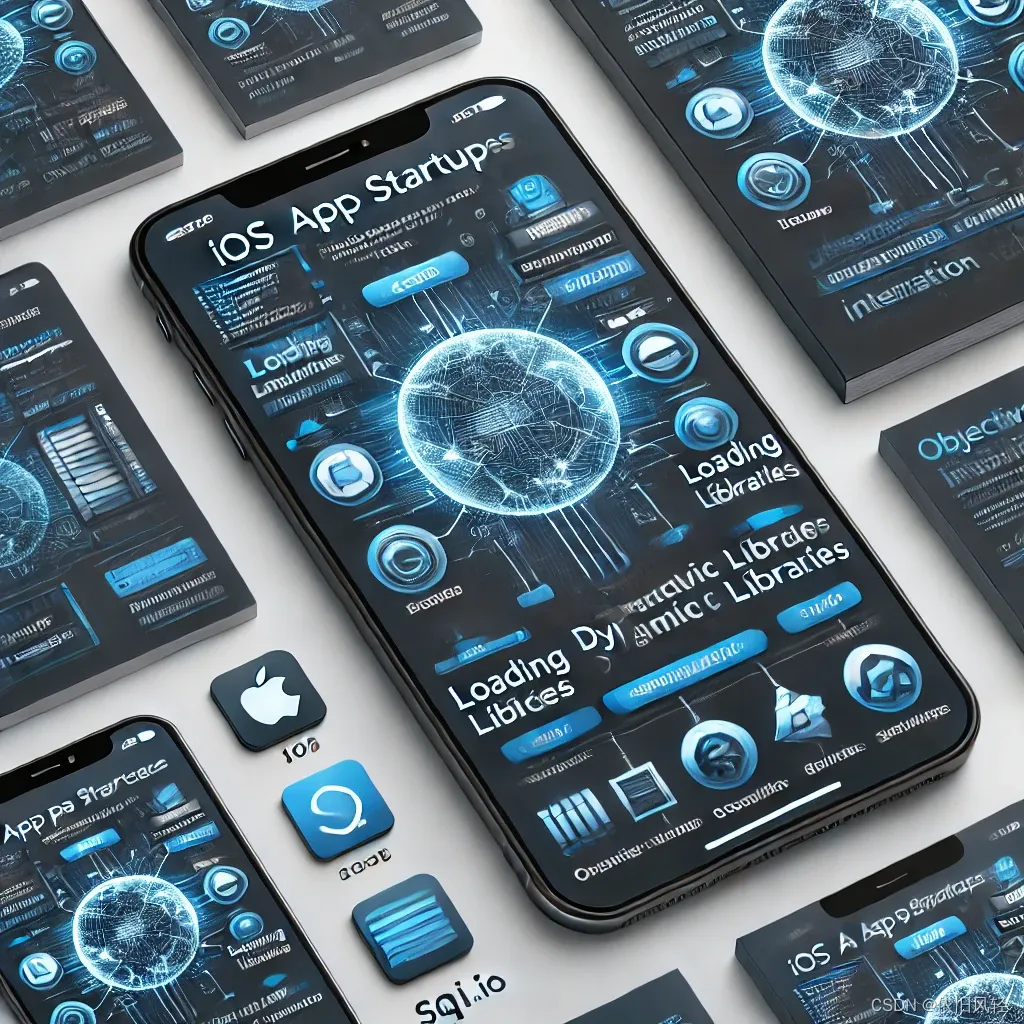
深入解析 iOS 应用启动过程:main() 函数前的四大步骤
深入解析 iOS 应用启动过程:main() 函数前的四大步骤 背景描述:使用 Objective-C 开发的 iOS 或者 MacOS 应用 在开发 iOS 应用时,我们通常会关注 main() 函数及其之后的执行逻辑,但在 main() 函数之前,系统已经为我们…...

textarea标签改写为富文本框编辑器KindEditor
下载 - KindEditor - 在线HTML编辑器 KindEditor的简单使用-CSDN博客 一、 Maven需要的依赖: 如果依赖无法下载,可以多添加几个私服地址: 在Maven框架中加入镜像私服 <mirrors><!-- mirror| Specifies a repository mirror site to…...
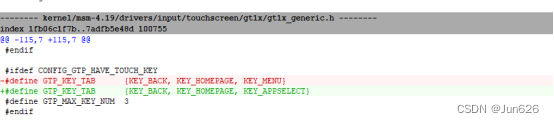
高通安卓12-Input子系统
1.Input输入子系统架构 Input Driver(Input设备驱动层)->Input core(输入子系统核心层)->Event handler(事件处理层)->User space(用户空间) 2.getevent获取Input事件的用法 getevent 指令用于获取android系统中 input 输入事件,比如获取按键上报信息、获…...

HTML 事件
HTML 事件 HTML 事件是发生在 HTML 元素上的交互瞬间,它们可以由用户的行为(如点击、按键、鼠标移动等)或浏览器自身的行为(如页面加载完成、图片加载失败等)触发。在 HTML 和 JavaScript 的交互中,事件扮演着核心角色,允许开发者创建动态和响应式的网页。 常见的 HTM…...

Mysql 官方提供的公共测试数据集 Example Databases
数据集:GitHub - datacharmer/test_db: A sample MySQL database with an integrated test suite, used to test your applications and database servers 下载 test_db: https://github.com/datacharmer/test_db/releases/download/v1.0.7/test_db-1.0.7.tar.gz …...
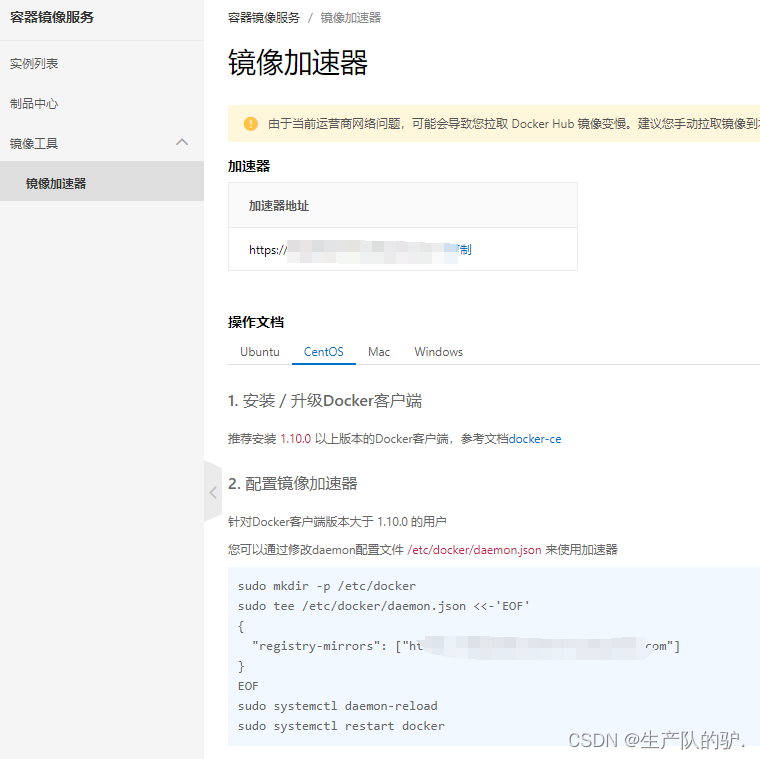
Docker 下载与安装以及配置
安装yum工具 yum install -y yum-ulits配置yum源 阿里云源 yum-config-manager --add-repo https://mirrors.aliyun.com/docker-ce/linux/centos/docker-ce.repo安装Docker 17.03后为两个版本: 社区版(Community Edition,缩写为 CE&#x…...

Java中的集合框架详解:List、Set、Map的使用场景
Java中的集合框架详解:List、Set、Map的使用场景 大家好,我是免费搭建查券返利机器人省钱赚佣金就用微赚淘客系统3.0的小编,也是冬天不穿秋裤,天冷也要风度的程序猿! 在Java编程中,集合框架是一个非常重要…...
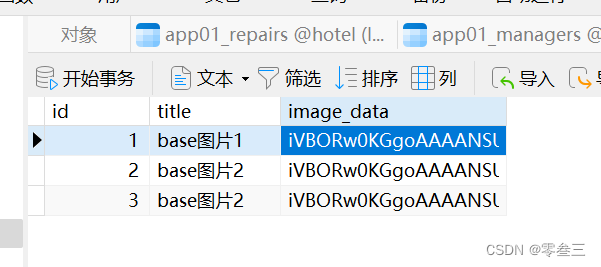
[Django学习]前端+后端两种方式处理图片流数据
方式1:数据库存放图片地址,图片存放在Django项目文件中 1.首先,我们现在models.py文件中定义模型来存放该图片数据,前端传来的数据都会存放在Django项目文件里的images文件夹下 from django.db import modelsclass Image(models.Model):title models.C…...

如何配置IOMMU或者SWIOTLB
1. 前言 这篇文章说明了如何在Linux内核中启用和配置IOMMU和SWOTLB。 当今的计算或者嵌入设备使用一种内存分区的方法进行外设的管理,如显卡、PCI设备或USB设备,都将设备映射为一段内存,用于设备的读写。 传统意义上的IOMMU用于内存映射&a…...
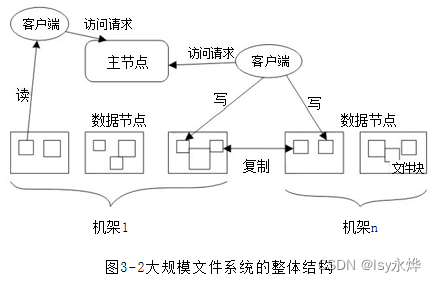
【大数据 复习】第3章 分布式文件系统HDFS(重中之重)
一、概念 1.分布式文件系统把文件分布存储到多个计算机节点上,通过网络实现、文件在多台主机上进行分布式存储的文件系统。(就是你的电脑存a,我的电脑存pple) 2.降低了硬件开销: 与之前使用多个处理器和专用高级硬件的并行化处理装…...
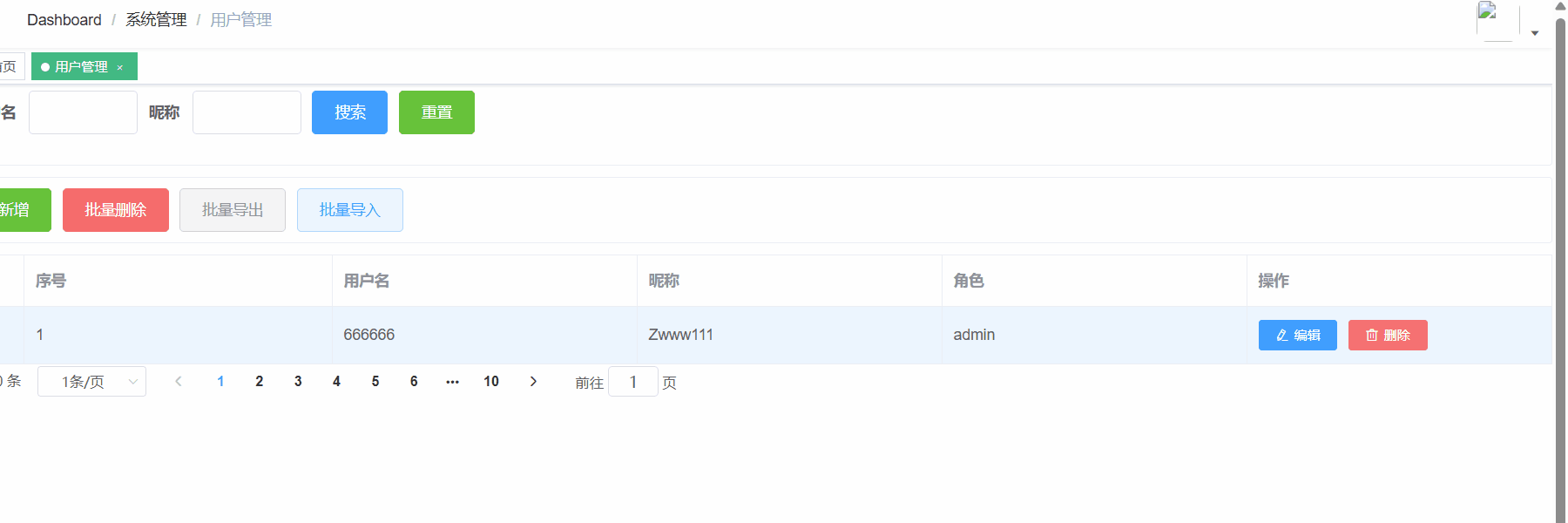
element-ui里message抖动问题
由于element默认屏蔽滚动条,导致取消时弹message时 侧边滚动栏突然回来后引起抖动问题 是由于打开弹窗时出现遮罩层dialog对话框 时引起了元素内容超出自身尺寸 对应的overflow样式内容为hidden,且新建了一个class类内容为增加17 内右边距,当…...
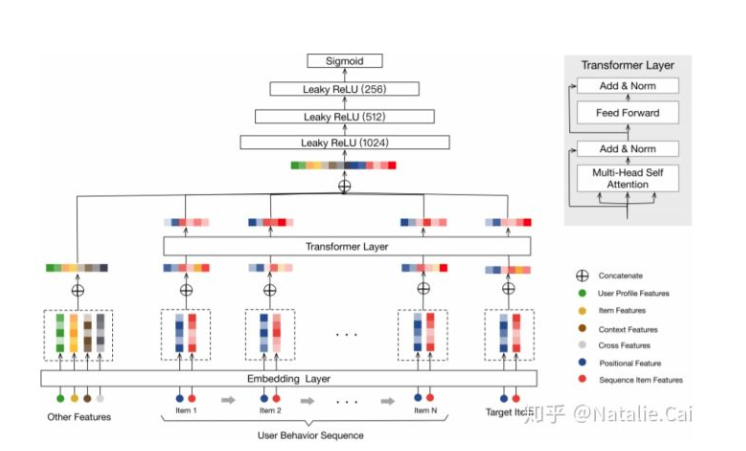
Attention系列总结-粘贴自知乎
1. 梦想做个翟老师:阿里:Behavior Sequence Transformer 解读48 赞同 7 评论文章 优点:捕捉用户行为历史序列中的顺序信息。w2v也是捕捉用户序列信息的,本质差异在于啥? 添加图片注释,不超过 140 字(可选࿰…...
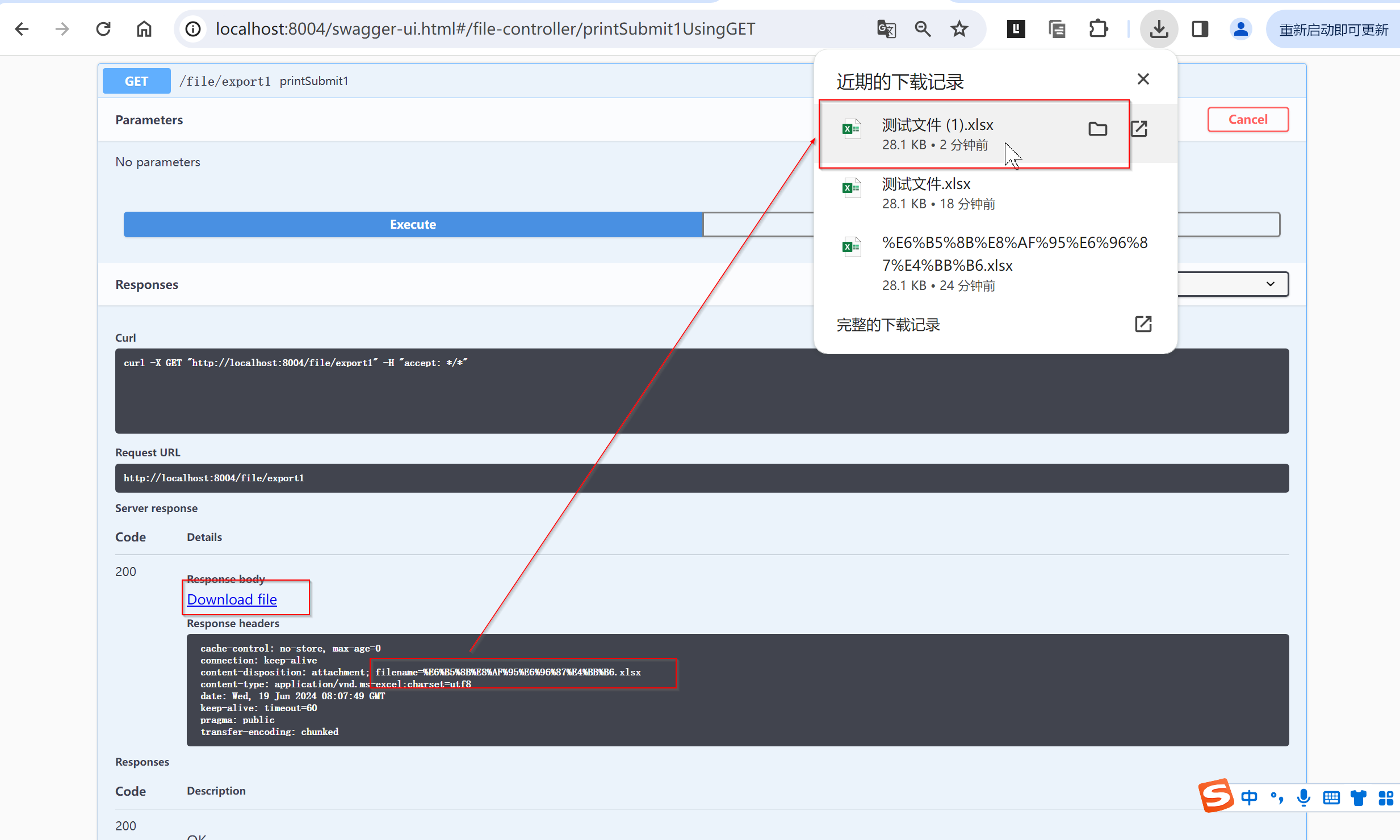
swagger下载文件名中文乱码、swagger导出文件名乱码、swagger文件导出名称乱码、解决swagger中文下载乱码bug
文章目录 一、场景描述:swagger导出文件名称乱码二、乱码原因三、解决方法3.1、方法一、在浏览器中输入地址下载3.2、方法二、swagger升级为2.10.0及以上 四、可能遇到的问题4.1、DocumentationPluginsManager.java:152 一、场景描述:swagger导出文件名称…...
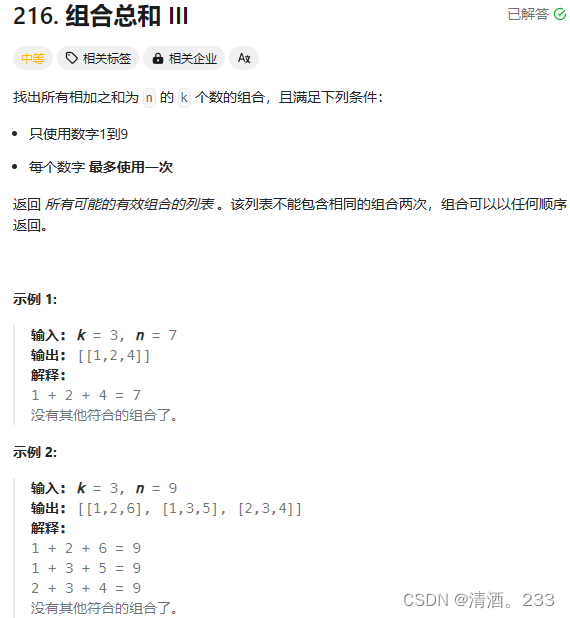
191.回溯算法:组合总和|||(力扣)
代码解决 class Solution { public:vector<vector<int>> result; // 存储所有符合条件的组合vector<int> res; // 当前组合// 回溯函数void backtracing(int k, int n, int index, int sum) {// 如果当前组合的长度等于k,且总和等于nif (res.si…...

JupyterLab使用指南(二):JupyterLab基础
第2章 JupyterLab基础 2.1 JupyterLab界面介绍 JupyterLab的用户界面非常直观和灵活。它包括文件浏览器、工作区、多标签页、命令面板和侧边栏等功能。以下是各个部分的详细介绍: 2.1.1 文件浏览器 文件浏览器位于界面左侧,用于导航和管理文件。你可…...

ubuntu18.04 + openssl + engine + pkcs11+ softhsm2 双向认证测试
安装环境 openssl 1.1.1 pkcs11-tool (由sudo apt-get install opensc 安装) libpksc11 (需源码安装apt install 只有libp11, 源码安装才有 libpksc11.so -> pkcs11.so) softhsm2 (由sudo apt-get install softhsm…...
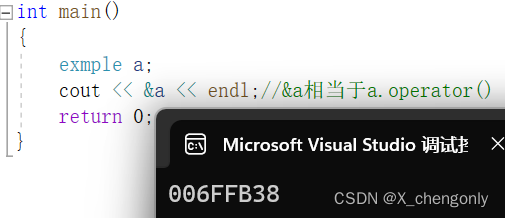
【C++】类和对象2.0
俺来写笔记了,哈哈哈,浅浅介绍类和对象的知识点! 1.类的6个默认成员函数 俺们定义一个空类: class N {}; 似乎这个类N里面什么都没有,其实不是这样子的。这个空类有6个默认的成员函数 。 默认成员函数:…...
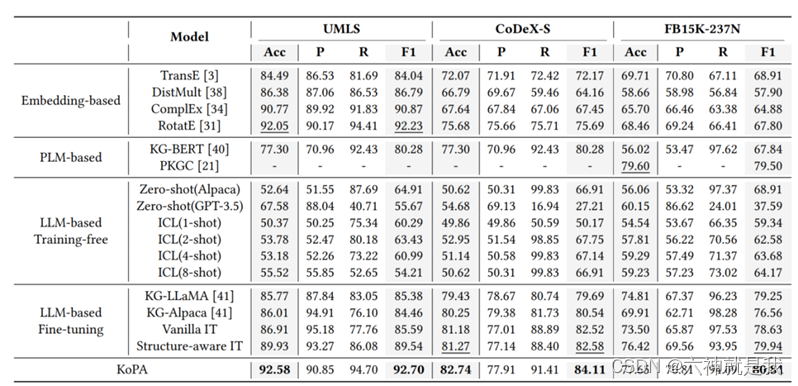
【LLM之KG】KoPA论文阅读笔记
研究背景 知识图谱补全(KGC)是通过预测知识图谱中缺失的三元组来完善知识图谱的信息。传统方法主要基于嵌入和预训练语言模型,但这些方法往往忽视了知识图谱的结构信息,导致预测效果不佳。 研究目标 本文的研究目标是探索如何将…...
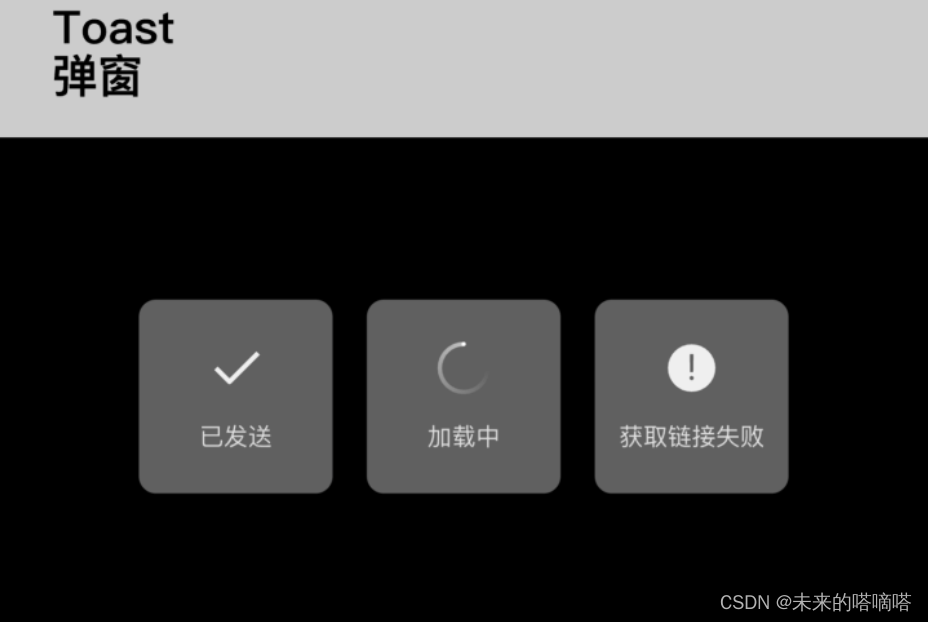
UI设计速成课:理解模态窗口与非模态窗口的区别
我们日常所说的弹性框架是非常笼统的概念。我们习惯性地称之为对话框架、浮动层和提示条。弹性框架可以分为两种:模态弹性框架和非模态弹性框架。产品需要弹性框架来传递信息,用户需要弹性框架来接受反馈,但是没有经过推敲的弹出窗口设计很容易让用户感到…...
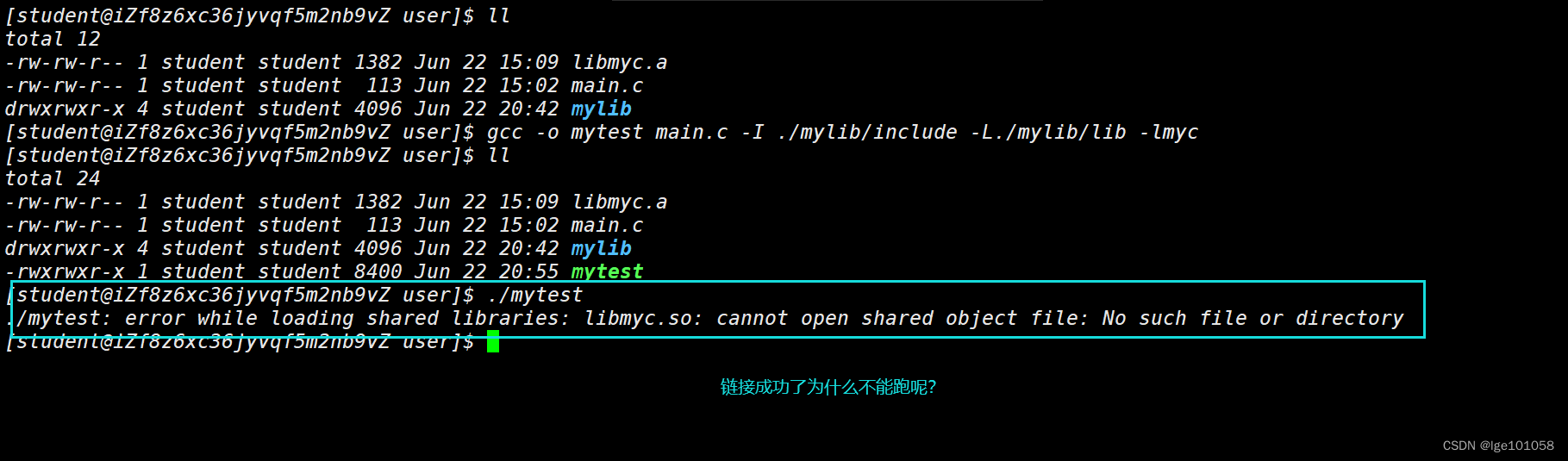
【Linux】基础IO_4
文章目录 六、基础I/O4. 动静态库 未完待续 六、基础I/O 4. 动静态库 既然我们能够成功创建静态库了,接下来我们将这个代码打包成动态库: shared: 表示生成共享库格式 fPIC:产生位置无关码(position independent code) 动态库库名规则&…...

C++模板类原理讲解
C模板类原理讲解 C模板是一种强大的编译期工具,它允许我们创建通用的、类型无关的类和函数。模板的主要目的是实现代码的重用和泛型编程。模板类的原理涉及以下几个方面: 模板的定义和实例化模板的类型参数模板特化模板的编译过程模板的优点和缺点 1.…...
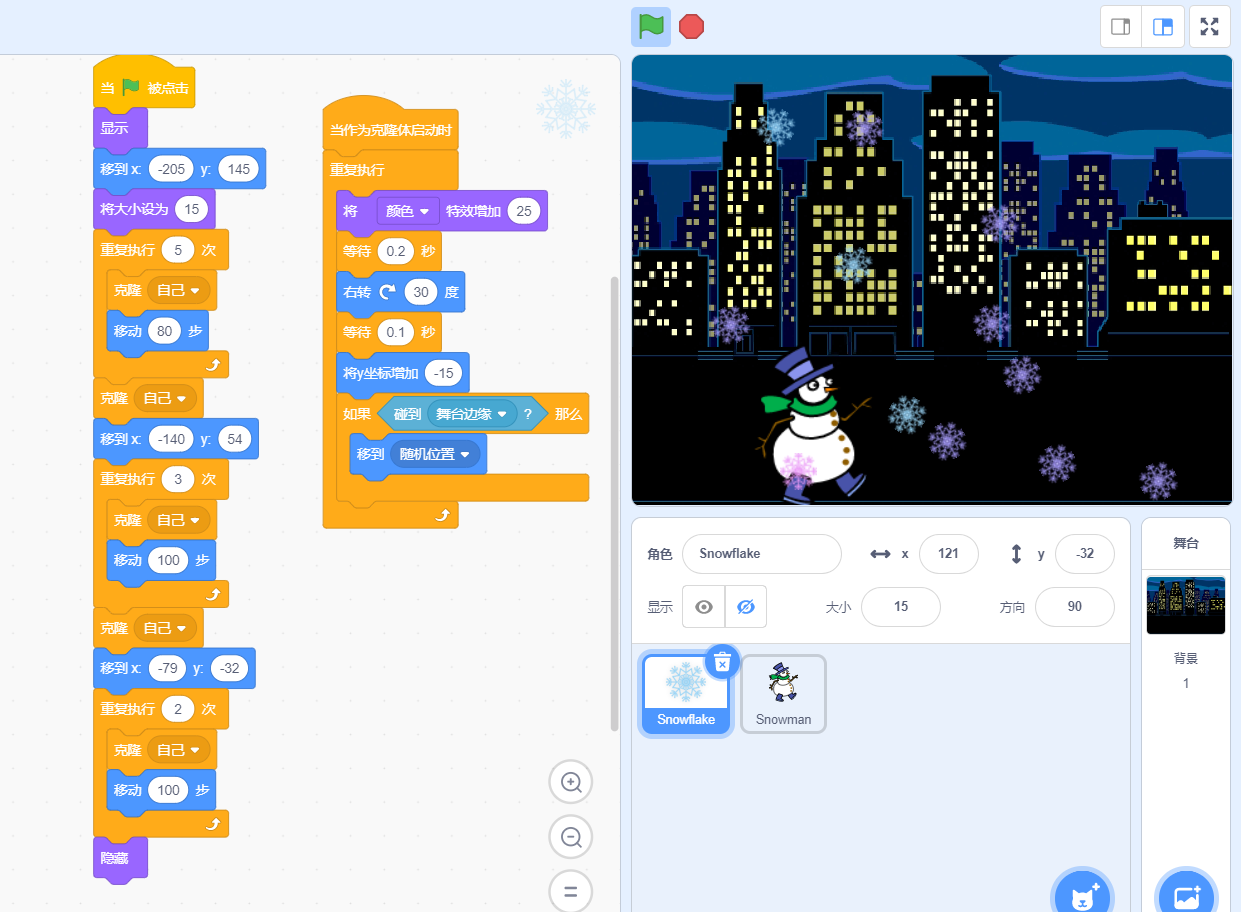
scratch编程03-反弹球
这篇文章和上一篇文章《scratch3编程02-使用克隆来编写小游戏》类似(已经完全掌握了克隆的可以忽略这篇文章),两篇文章都使用到了克隆来编写一个小游戏,这篇文章与上篇文章不同的是,本体在进行克隆操作时,不…...

postgresql数据库进阶知识
postgresql数据库进阶知识 # 如果表存在就先删除 drop table if exists student; # 创建学生表 # id serial not null 表示id自增 # id integer not null 表示id不自增 create table student (id serial not nullconstraint student_pkprimary…...
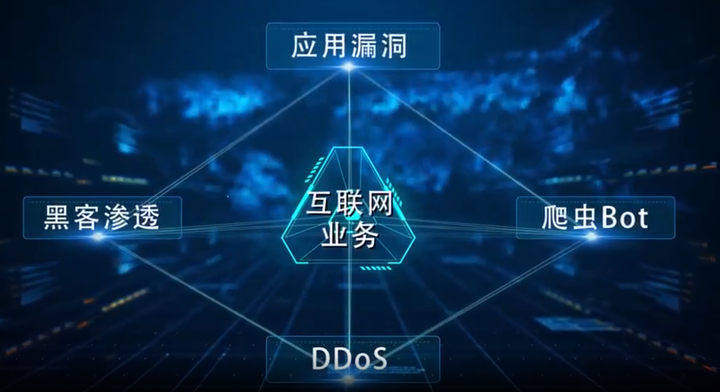
关于HTTP劫持,该如何理解、防范和应对
一、引言 HTTP劫持(HTTP Hijacking)是一种网络安全威胁,它发生在HTTP通信过程中,攻击者试图通过拦截、篡改或监控用户与服务器之间的数据流量,以达到窃取敏感信息或执行恶意操作的目的。今天我们就来详细了解HTTP劫持…...
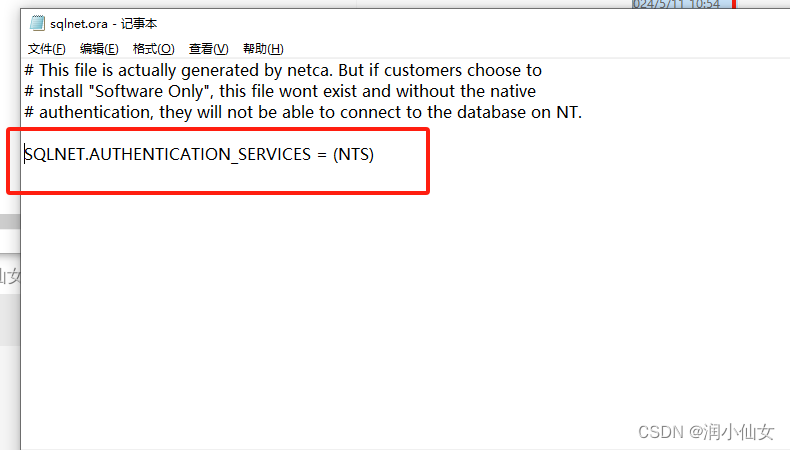
System.Data.OracleClient.OracleException:“ORA-12571: TNS: 包写入程序失败
System.Data.OracleClient.OracleException:“ORA-12571: TNS: 包写入程序失败 解决方法: 首先%oracle_home%/network/admin下的sqlnet.ora文件,把SQLNET.AUTHENTICATION_SERVICES (NTS)加个 # 注释掉就好了...
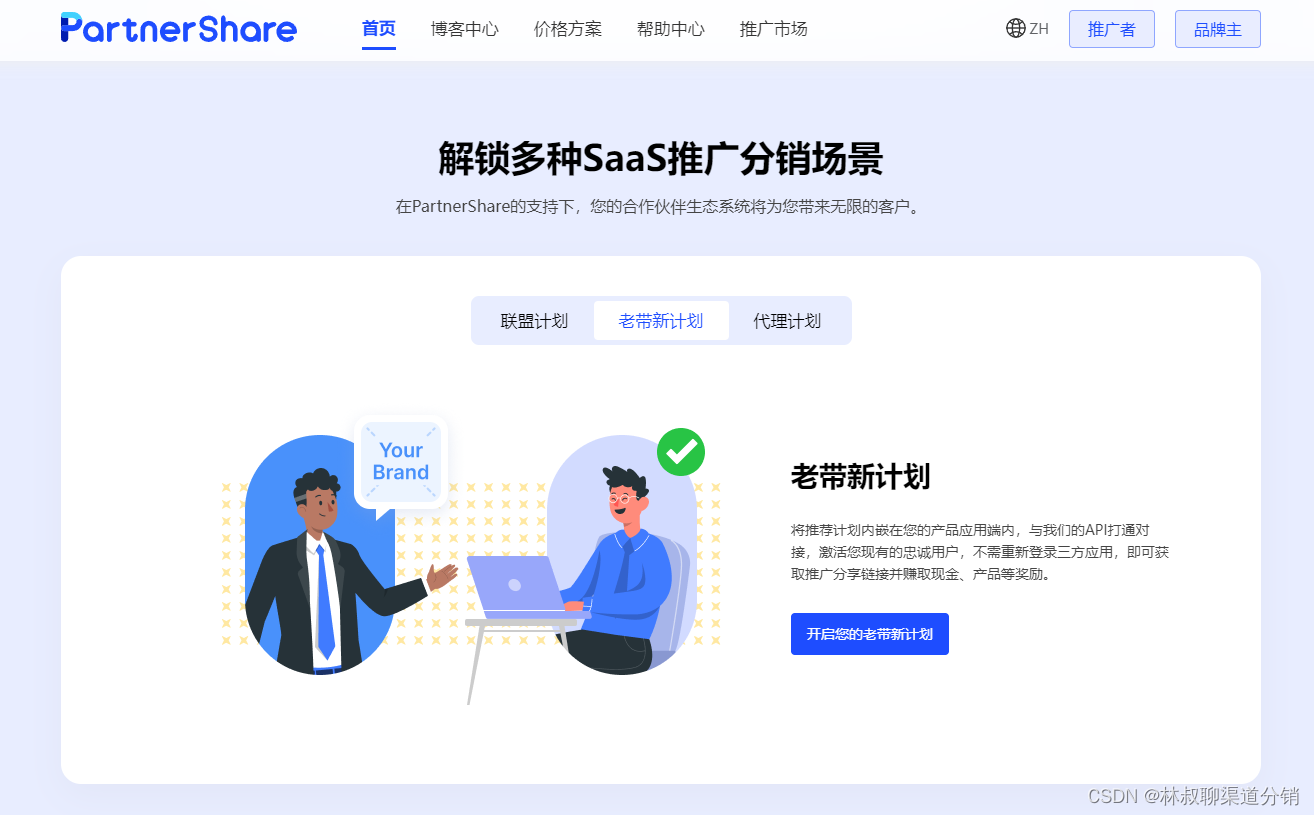
saas产品运营案例 | 联盟营销计划如何帮助企业提高销售额?
在当今数字化时代,SaaS(软件即服务)产品已成为企业提高效率、降低成本的重要工具。然而,面对激烈的市场竞争,如何有效地推广SaaS产品、提高销售额,成为许多企业面临的挑战。林叔将以ClickFunnels为例&#…...
模式分解算法-满足3NF的无损且保持函数依赖的分解算法、满足BCNF的无损连接分解算法
一、引言 1、对指定的关系模式,若范式级别较低,为第一范式或第二范式,由于存在数据冗余或更新异常问题,在实际中一般是不可用的,关系模式的规范化就是将满足低一级的关系模式分解为若干满足高一级范式的关系模式的集合…...
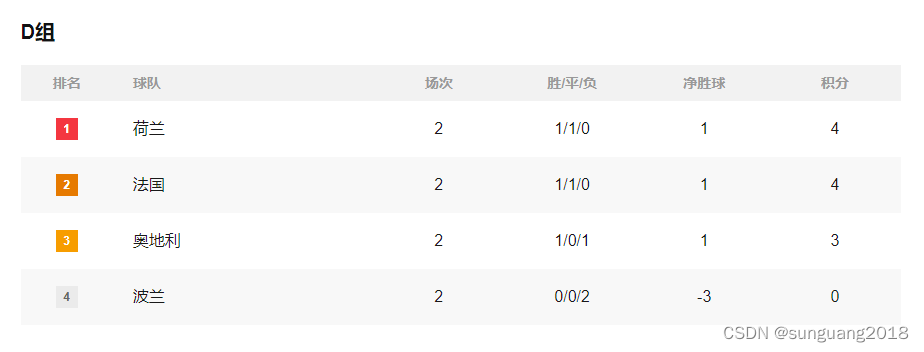
荷兰与法国战平,双方能携手出现?
就在昨天晚上,荷兰队经历了90分钟的鏖战,最终0-0与法国队握手言和。此役,哈维-西蒙斯为荷兰队打进一球,但进球被判无效。从目前的积分形势来看,双方基本上确定携手晋级16强赛。本场比赛,荷兰队后卫内森-阿克…...
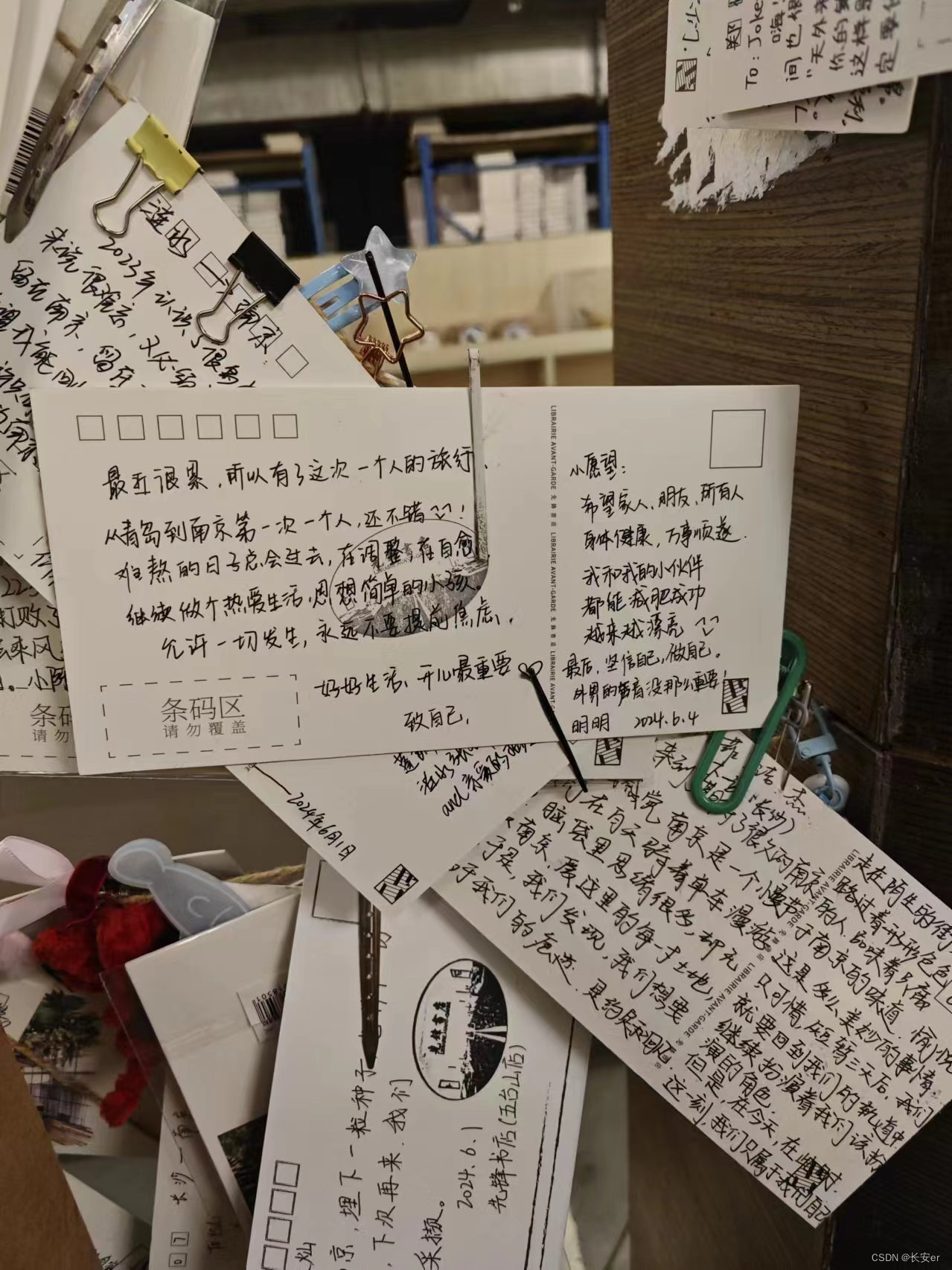
数据可视化实验二:回归分析、判别分析与聚类分析
目录 一、使用回归分析方法分析某病毒是否与温度呈线性关系 1.1 代码实现 1.2 线性回归结果 1.3 相关系数验证 二、使用判别分析方法预测某病毒在一定的温度下是否可以存活,分别使用三种判别方法,包括Fish判别、贝叶斯判别、LDA 2.1 数据集展示&am…...
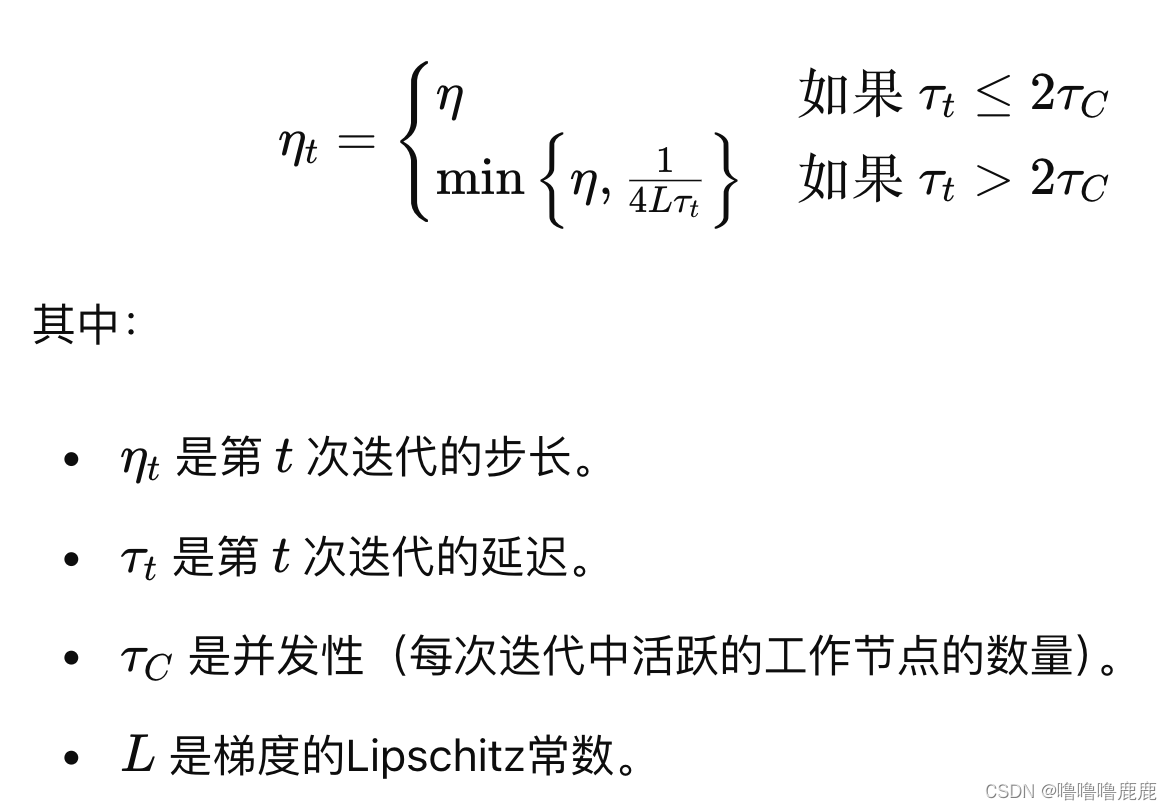
FL论文专栏|设备异构、异步联邦
论文:Asynchronous Federated Optimization(12th Annual Workshop on Optimization for Machine Learning) 链接 实现Server的异步更新。每次Server广播全局Model的时候附带一个时间戳,Client跑完之后上传将时间戳和Model同时带回…...