Dataset for Stable Diffusion
1.Dataset for Stable Diffusion
笔记来源:
1.Flickr8k数据集处理
2.处理Flickr8k数据集
3.Github:pytorch-stable-diffusion
4.Flickr 8k Dataset
5.dataset_flickr8k.json
1.1 Dataset
采用Flicker8k数据集,该数据集有两个文件,第一个文件为Flicker8k_Dataset (全部为图片),第二个文件为Flickr8k.token.txt (含两列image_id和caption),其中一个image_id对应5个caption (sentence)
![]() | ![]() |
![]() | ![]() |
1.2 Dataset description file
数据集文本描述文件:dataset_flickr8k.json
文件格式如下:
{“images”: [ {“sentids”: [ ],“imgid”: 0,“sentences”:[{“tokens”:[ ]}, {“tokens”:[ ], “raw”: “…”, “imgid”:0, “sentid”:0}, …, “split”: “train”, “filename”: …jpg}, {“sentids”…} ], “dataset”: “flickr8k”}
参数 | 解释 |
---|---|
“sentids”:[0,1,2,3,4] | caption 的 id 范围(一个image对应5个caption,所以sentids从0到4) |
“imgid”:0 | image 的 id(从0到7999共8000张image) |
“sentences”:[ ] | 包含一张照片的5个caption |
“tokens”:[ ] | 每个caption分割为单个word |
“raw”: " " | 每个token连接起来的caption |
“imgid”: 0 | 与caption相匹配的image的id |
“sentid”: 0 | imag0对应的具体的caption的id |
“split”:" " | 将该image和对应caption划分到训练集or验证集or测试集 |
“filename”:“…jpg” | image具体名称 |
dataset_flickr8k.json
1.3 Process Datasets
下面代码引用自:Flickr8k数据集处理(仅作学习使用)
import json
import os
import random
from collections import Counter, defaultdict
from matplotlib import pyplot as plt
from PIL import Image
from argparse import Namespace
import numpy as np
import torch
import torch.nn as nn
from torch.nn.utils.rnn import pack_padded_sequence
from torch.utils.data import Dataset
import torchvision
import torchvision.transforms as transformsdef create_dataset(dataset='flickr8k', captions_per_image=5, min_word_count=5, max_len=30):"""Parameters:dataset: Name of the datasetcaptions_per_image: Number of captions per imagemin_word_count: Only consider words that appear at least this many times in the dataset (excluding the test set)max_len: Maximum number of words in a caption. Captions longer than this will be truncated.Output:A vocabulary file: vocab.jsonThree dataset files: train_data.json, val_data.json, test_data.json"""# Paths for reading data and saving processed data# Path to the dataset JSON fileflickr_json_path = ".../sd/data/dataset_flickr8k.json"# Folder containing imagesimage_folder = ".../sd/data/Flicker8k_Dataset"# Folder to save processed results# The % operator is used to format the string by replacing %s with the value of the dataset variable.# For example, if dataset is "flickr8k", the resulting output_folder will be# /home/wxy/Documents/PycharmProjects/pytorch-stable-diffusion/sd/data/flickr8k.output_folder = ".../sd/data/%s" % dataset# Ensure output directory existsos.makedirs(output_folder, exist_ok=True)print(f"Output folder: {output_folder}")# Read the dataset JSON filewith open(file=flickr_json_path, mode="r") as j:data = json.load(fp=j)# Initialize containers for image paths, captions, and vocabulary# Dictionary to store image pathsimage_paths = defaultdict(list)# Dictionary to store image captionsimage_captions = defaultdict(list)# Count the number of elements, then count and return a dictionary# key:element value:the number of elements.vocab = Counter()# read from file dataset_flickr8k.jsonfor img in data["images"]: # Iterate over each image in the datasetsplit = img["split"] # Determine the split (train, val, or test) for the imagecaptions = []for c in img["sentences"]: # Iterate over each caption for the image# Update word frequency count, excluding test set dataif split != "test": # Only update vocabulary for train/val splits# c['tokens'] is a list, The number of occurrences of each word in the list is increased by onevocab.update(c['tokens']) # Update vocabulary with words in the caption# Only consider captions that are within the maximum lengthif len(c["tokens"]) <= max_len:captions.append(c["tokens"]) # Add the caption to the list if it meets the length requirementif len(captions) == 0: # Skip images with no valid captionscontinue# Construct the full image path/home/wxy/Documents/PycharmProjects/pytorch-stable-diffusion# image_folder + image_name# ./Flicker8k_Dataset/img['filename']path = os.path.join(image_folder, img['filename'])# Save the full image path and its captions in the respective dictionariesimage_paths[split].append(path)image_captions[split].append(captions)'''After the above steps, we have:- vocab(a dict) keys:words、values: counts of all words- image_paths: (a dict) keys "train", "val", and "test"; values: lists of absolute image paths- image_captions: (a dict) keys: "train", "val", and "test"; values: lists of captions'''/home/wxy/Documents/PycharmProjects/pytorch-stable-diffusion........
我们通过dataset_flickr8k.json文件把数据集转化为三个词典
dict | key | value |
---|---|---|
vacab | word | frequency of words in all captions |
image_path | “train”、“val”、“test” | lists of absolute image path |
image_captions | “train”、“val”、“test” | lists of captions |
我们通过Debug打印其中的内容
print(vocab)
print(image_paths["train"][1])
print(image_captions["train"][1])
def create_dataset(dataset='flickr8k', captions_per_image=5, min_word_count=5, max_len=30):"""Parameters:dataset: Name of the datasetcaptions_per_image: Number of captions per imagemin_word_count: Only consider words that appear at least this many times in the dataset (excluding the test set)max_len: Maximum number of words in a caption. Captions longer than this will be truncated.Output:A vocabulary file: vocab.jsonThree dataset files: train_data.json, val_data.json, test_data.json"""........# Create the vocabulary, adding placeholders for special tokens# Add placeholders<pad>, unregistered word identifiers<unk>, sentence beginning and end identifiers<start><end>words = [w for w in vocab.keys() if vocab[w] > min_word_count] # Filter words by minimum countvocab = {k: v + 1 for v, k in enumerate(words)} # Create the vocabulary with indices# Add special tokens to the vocabularyvocab['<pad>'] = 0vocab['<unk>'] = len(vocab)vocab['<start>'] = len(vocab)vocab['<end>'] = len(vocab)# Save the vocabulary to a filewith open(os.path.join(output_folder, 'vocab.json'), "w") as fw:json.dump(vocab, fw)# Process each dataset split (train, val, test)# Iterate over each split: split = "train" 、 split = "val" 和 split = "test"for split in image_paths:# List of image paths for the splitimgpaths = image_paths[split] # type(imgpaths)=list# List of captions for the splitimcaps = image_captions[split] # type(imcaps)=list# store result that converting words of caption to their respective indices in the vocabularyenc_captions = []for i, path in enumerate(imgpaths):# Check if the image can be openedimg = Image.open(path)# Ensure each image has the required number of captionsif len(imcaps[i]) < captions_per_image:filled_num = captions_per_image - len(imcaps[i])# Repeat captions if neededcaptions = imcaps[i] + [random.choice(imcaps[i]) for _ in range(0, filled_num)]else:# Randomly sample captions if there are more than neededcaptions = random.sample(imcaps[i], k=captions_per_image)assert len(captions) == captions_per_imagefor j, c in enumerate(captions):# Encode each caption by converting words to their respective indices in the vocabularyenc_c = [vocab['<start>']] + [vocab.get(word, vocab['<unk>']) for word in c] + [vocab["<end>"]]enc_captions.append(enc_c)assert len(imgpaths) * captions_per_image == len(enc_captions)data = {"IMAGES": imgpaths,"CAPTIONS": enc_captions}# Save the processed dataset for the current split (train,val,test)with open(os.path.join(output_folder, split + "_data.json"), 'w') as fw:json.dump(data, fw)create_dataset()
经过create_dataset函数,我们得到如下图的文件
四个文件的详细内容见下表
![]() | ![]() |
![]() | ![]() |
![]() | ![]() |
![]() | ![]() |
生成vocab.json的关键代码
首先统计所有caption中word出现至少大于5次的word,而后给这些word依次赋予一个下标
# Create the vocabulary, adding placeholders for special tokens# Add placeholders<pad>, unregistered word identifiers<unk>, sentence beginning and end identifiers<start><end># Create a list of words from the vocabulary that have a frequency higher than 'min_word_count'# min_word_count: Only consider words that appear at least this many times in the dataset (excluding the test set)words = [w for w in vocab.keys() if vocab[w] > min_word_count] # Filter words by minimum count# assign an index to each word, starting from 1 (indices start from 0, so add 1)vocab = {k: v + 1 for v, k in enumerate(words)} # Create the vocabulary with indices
最终生成vocab.json
生成 [“split”]_data.json 的关键
读入文件dataset_flickr8k.json,并创建两个字典,第一个字典放置每张image的绝对路径,第二个字典放置描述image的caption,根据vocab将token换为下标保存,根据文件dataset_flickr8k.json中不同的split,这image的绝对路径和相应caption保存在不同文件中(train_data.json、test_data.json、val_data.json)
dataset_flickr8k.json
train_data.json
从vocab中获取token的下标得到CAPTION的编码
for j, c in enumerate(captions):# Encode each caption by converting words to their respective indices in the vocabularyenc_c = [vocab['<start>']] + [vocab.get(word, vocab['<unk>']) for word in c] + [vocab["<end>"]]enc_captions.append(enc_c)
尝试使用上面生成的测试集文件test_data.json和vocab.json输出某张image以及对应的caption
下面代码引用自:Flickr8k数据集处理(仅作学习使用)
'''
test
1.Iterates over the 5 captions for 下面代码引用自:[Flickr8k数据集处理](https://blog.csdn.net/weixin_48981284/article/details/134676813)(仅作学习使用)the 250th image.
2.Retrieves the word indices for each caption.
3.Converts the word indices to words using vocab_idx2word.
4.Joins the words to form complete sentences.
5.Prints each caption.
'''
import json
from PIL import Image
from matplotlib import pyplot as plt
# Load the vocabulary from the JSON file
with open('.../sd/data/flickr8k/vocab.json', 'r') as f:vocab = json.load(f) # Load the vocabulary from the JSON file into a dictionary
# Create a dictionary to map indices to words
vocab_idx2word = {idx: word for word, idx in vocab.items()}
# Load the test data from the JSON file
with open('.../sd/data/flickr8k/test_data.json', 'r') as f:data = json.load(f) # Load the test data from the JSON file into a dictionary
# Open and display the 250th image in the test set
# Open the image at index 250 in the 'IMAGES' list
content_img = Image.open(data['IMAGES'][250])
plt.figure(figsize=(6, 6))
plt.subplot(1,1,1)
plt.imshow(content_img)
plt.title('Image')
plt.axis('off')
plt.show()
# Print the lengths of the data, image list, and caption list
# Print the number of keys in the dataset dictionary (should be 2: 'IMAGES' and 'CAPTIONS')
print(len(data))
print(len(data['IMAGES'])) # Print the number of images in the 'IMAGES' list
print(len(data["CAPTIONS"])) # Print the number of captions in the 'CAPTIONS' list
# Display the captions for the 300th image
# Iterate over the 5 captions associated with the 300th image
for i in range(5):# Get the word indices for the i-th caption of the 300th imageword_indices = data['CAPTIONS'][250 * 5 + i]# Convert indices to words and join them to form a captionprint(''.join([vocab_idx2word[idx] for idx in word_indices]))
data 的 key 有两个 IMAGES 和 CAPTIONS
测试集image有1000张,每张对应5个caption,共5000个caption
第250张图片的5个caption如下图
1.4 Dataloader
下面代码引用自:Flickr8k数据集处理(仅作学习使用)
import json
import os
import random
from collections import Counter, defaultdict
from PIL import Image
import torch
from torch.utils.data import Dataset
from torch.utils import data
import torchvision.transforms as transformsclass ImageTextDataset(Dataset):"""Pytorch Dataset class to generate data batches using torch DataLoader"""def __init__(self, dataset_path, vocab_path, split, captions_per_image=5, max_len=30, transform=None):"""Parameters:dataset_path: Path to the JSON file containing the datasetvocab_path: Path to the JSON file containing the vocabularysplit: The dataset split, which can be "train", "val", or "test"captions_per_image: Number of captions per imagemax_len: Maximum number of words per captiontransform: Image transformation methods"""self.split = split# Validate that the split is one of the allowed valuesassert self.split in {"train", "val", "test"}# Store captions per imageself.cpi = captions_per_image# Store maximum caption lengthself.max_len = max_len# Load the datasetwith open(dataset_path, "r") as f:self.data = json.load(f)# Load the vocabularywith open(vocab_path, "r") as f:self.vocab = json.load(f)# Store the image transformation methodsself.transform = transform# Number of captions in the dataset# Calculate the size of the datasetself.dataset_size = len(self.data["CAPTIONS"])def __getitem__(self, i):"""Retrieve the i-th sample from the dataset"""# Get [i // self.cpi]-th image corresponding to the i-th sample (each image has multiple captions)img = Image.open(self.data['IMAGES'][i // self.cpi]).convert("RGB")# Apply image transformation if providedif self.transform is not None:# Apply the transformation to the imageimg = self.transform(img)# Get the length of the captioncaplen = len(self.data["CAPTIONS"][i])# Pad the caption if its length is less than max_lenpad_caps = [self.vocab['<pad>']] * (self.max_len + 2 - caplen)# Convert the caption to a tensor and pad itcaption = torch.LongTensor(self.data["CAPTIONS"][i] + pad_caps)return img, caption, caplen # Return the image, caption, and caption lengthdef __len__(self):return self.dataset_size # Number of samples in the datasetdef make_train_val(data_dir, vocab_path, batch_size, workers=4):"""Create DataLoader objects for training, validation, and testing sets.Parameters:data_dir: Directory where the dataset JSON files are locatedvocab_path: Path to the vocabulary JSON filebatch_size: Number of samples per batchworkers: Number of subprocesses to use for data loading (default is 4)Returns:train_loader: DataLoader for the training setval_loader: DataLoader for the validation settest_loader: DataLoader for the test set"""# Define transformation for training settrain_tx = transforms.Compose([transforms.Resize(256), # Resize images to 256x256transforms.ToTensor(), # Convert image to PyTorch tensortransforms.Normalize(mean=[0.485, 0.456, 0.406], std=[0.229, 0.224, 0.225]) # Normalize using ImageNet mean and std])val_tx = transforms.Compose([transforms.Resize(256),transforms.ToTensor(),transforms.Normalize([0.485, 0.456, 0.406], [0.229, 0.224, 0.225])])# Create dataset objects for training, validation, and test setstrain_set = ImageTextDataset(dataset_path=os.path.join(data_dir, "train_data.json"), vocab_path=vocab_path,split="train", transform=train_tx)vaild_set = ImageTextDataset(dataset_path=os.path.join(data_dir, "val_data.json"), vocab_path=vocab_path,split="val", transform=val_tx)test_set = ImageTextDataset(dataset_path=os.path.join(data_dir, "test_data.json"), vocab_path=vocab_path,split="test", transform=val_tx)# Create DataLoader for training set with data shufflingtrain_loder = data.DataLoader(dataset=train_set, batch_size=batch_size, shuffer=True,num_workers=workers, pin_memory=True)# Create DataLoader for validation set without data shufflingval_loder = data.DataLoader(dataset=vaild_set, batch_size=batch_size, shuffer=False,num_workers=workers, pin_memory=True, drop_last=False)# Create DataLoader for test set without data shufflingtest_loder = data.DataLoader(dataset=test_set, batch_size=batch_size, shuffer=False,num_workers=workers, pin_memory=True, drop_last=False)return train_loder, val_loder, test_loder
创建好train_loader后,接下来我们就可以着手开始训练SD了!
相关文章:
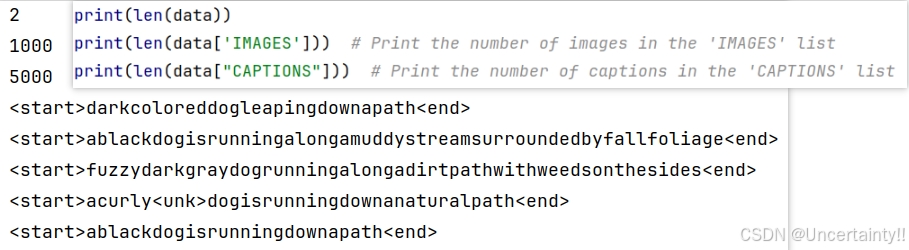
Dataset for Stable Diffusion
1.Dataset for Stable Diffusion 笔记来源: 1.Flickr8k数据集处理 2.处理Flickr8k数据集 3.Github:pytorch-stable-diffusion 4.Flickr 8k Dataset 5.dataset_flickr8k.json 1.1 Dataset 采用Flicker8k数据集,该数据集有两个文件ÿ…...
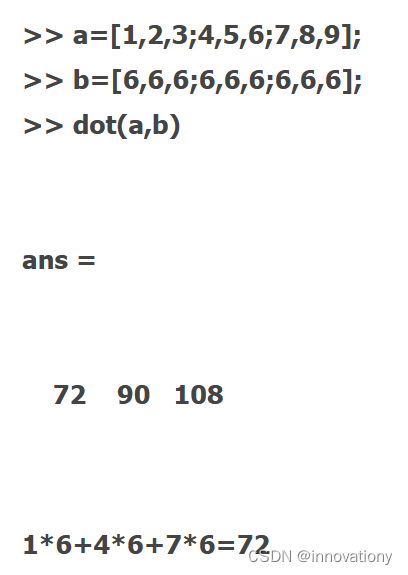
近期matlab学习笔记,学习是一个记录,反复的过程
近期matlab学习笔记,学习是一个记录,反复的过程 matlab的mlx文件在运行的时候,不需要在文件夹路径下,也能运行,但是需要调用子函数时,就需要在文件所在路径下运行 那就先运行子函数,把路径换过来…...
Elasticsearch7.5.2 常用rest api与elasticsearch库
目录 一、rest api 1. 新建索引 2. 删除索引 3. 插入单条数据 4. 更新单条数据 5. 删除单条数据 6. 查询数据 二、python elasticsearch库 1. 新建索引 一、rest api 1. 新建索引 请求方式:PUT 请求URL:http://ip/(your_index_nam…...
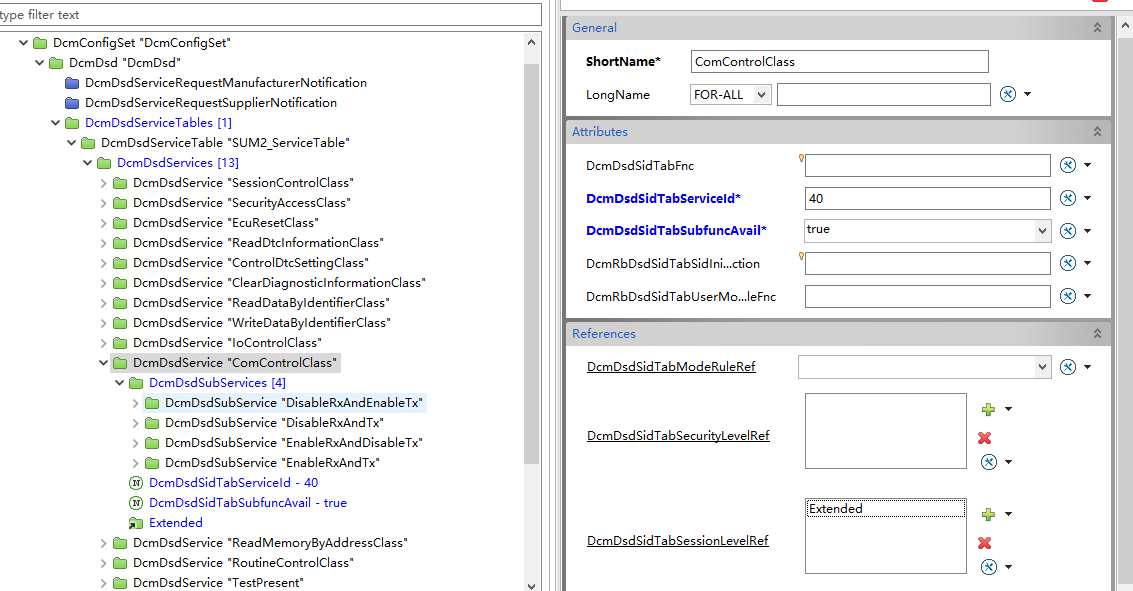
Autosar Dcm配置-0x28服务ComControl-基于ETAS软件
文章目录 前言DcmDcmDsdDcmDspBswMBswMModeRequestPortBswMModeConditionBswMLogicalExpressionBswMActionBswMActionListBswMRule总结前言 0x28服务主要用来控制非诊断报文的通讯,一般在刷写预编程过程中,用来禁止APP的通信报文,可以减少总线负载率,提高刷写成功率。本文…...
平安养老险厦门分公司:提升金融服务,发挥金融力量
为向社会公众普及金融保险知识,传递消费者权益保护理念,平安养老保险股份有限公司厦门分公司(以下简称“分公司”)积极开展“78保险公众宣传日”系列教育宣传活动。分公司紧扣“保险,让每一步前行更有底气”主题&#…...
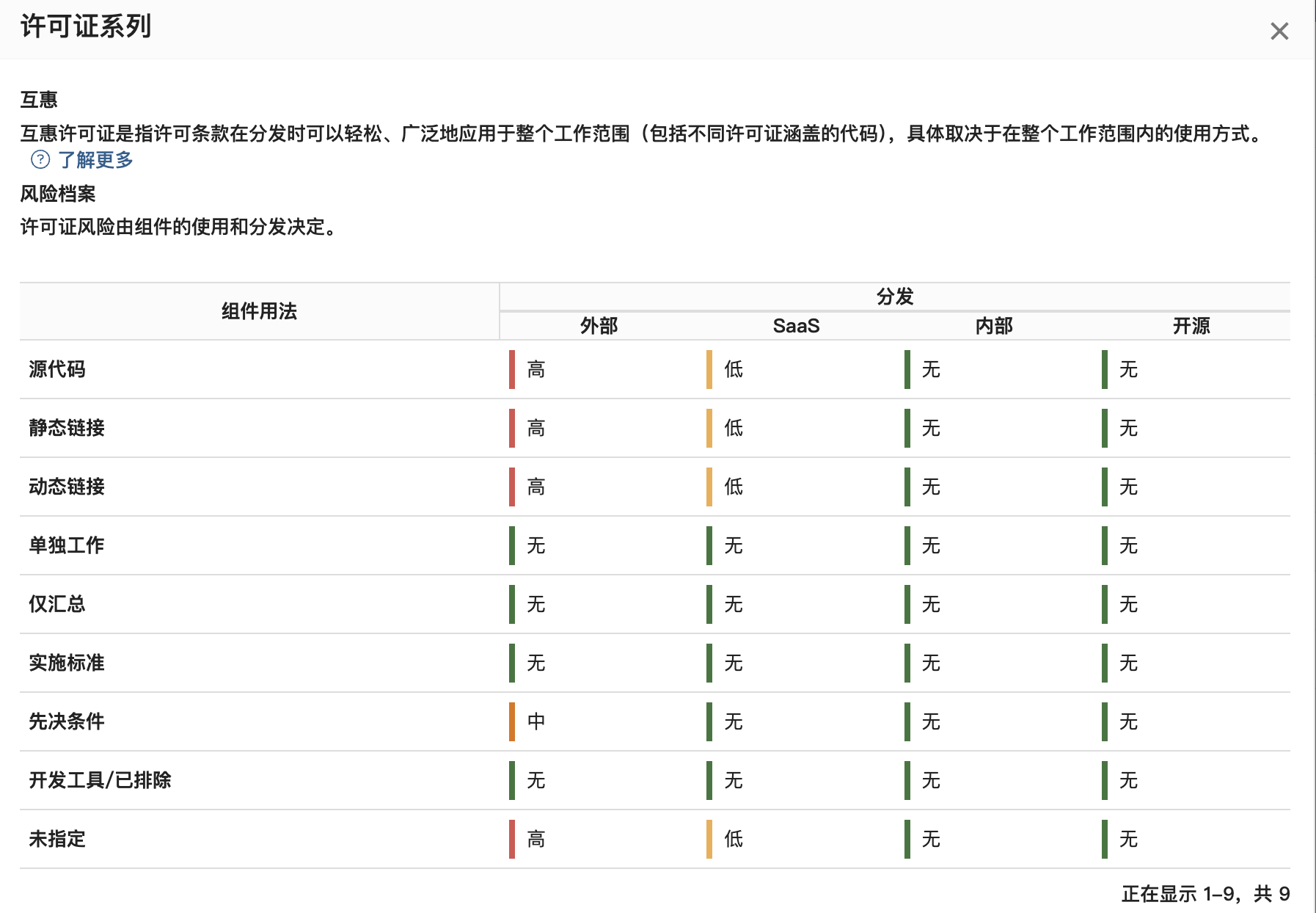
【开源合规】开源许可证风险场景详细解读
文章目录 前言关于BlackDuck许可证风险对比图弱互惠型许可证举个例子具体示例LGPL系列LGPL-2.0-onlyLGPL-2.0-or-laterLGPL-2.1-onlyLGPL-2.1-or-laterLGPL-3.0-onlyLGPL-3.0-or-laterMPL系列MPL-1.0MPL-1.1MPL-2.0EPL系列EPL-1.0EPL-2.0互惠型许可证GPL系列GPL-1.0GPL-2.0GPL-…...
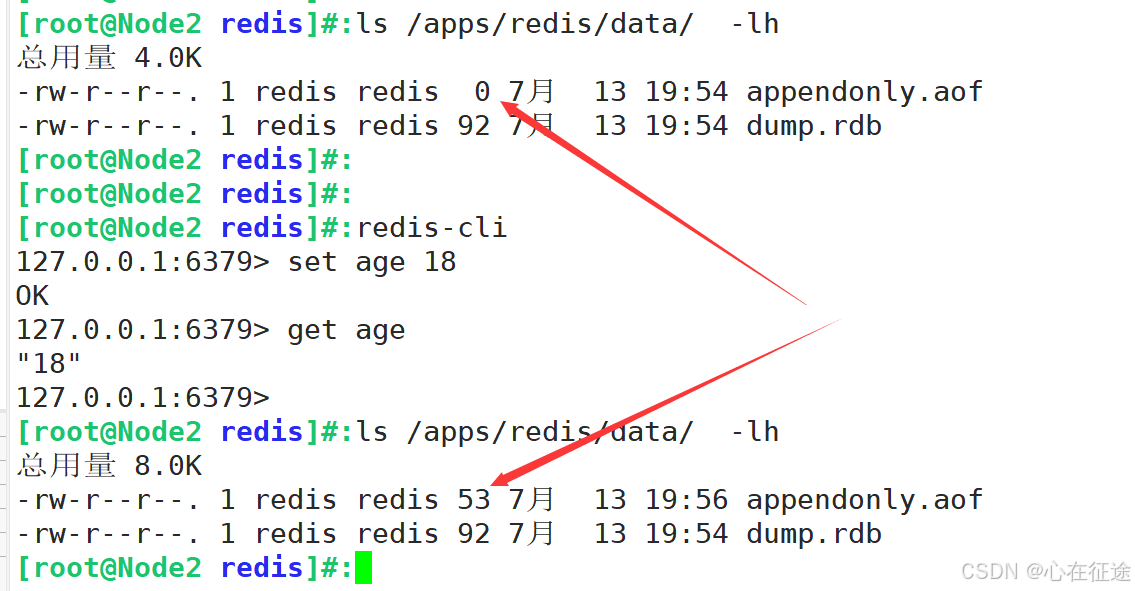
Redis持久化RDB,AOF
目 录 CONFIG动态修改配置 慢查询 持久化 在上一篇主要对redis的了解入门,安装,以及基础配置,多实例的实现:redis的安装看我上一篇: Redis安装部署与使用,多实例 redis是挡在MySQL前面的,运行在内存…...
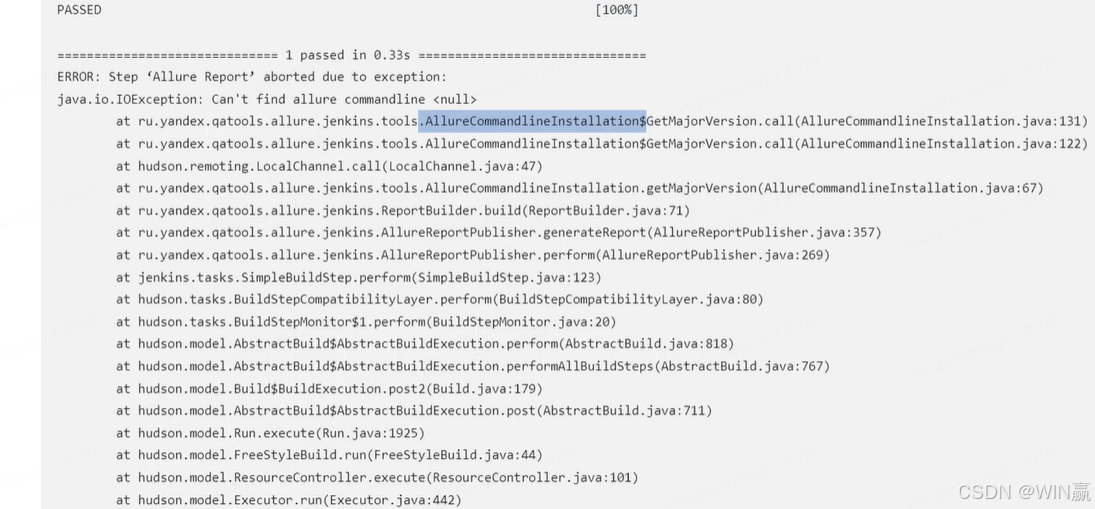
【持续集成_03课_Linux部署Sonar+Gogs+Jenkins】
一、通过虚拟机搭建Linux环境-CnetOS 1、安装virtualbox,和Vmware是一样的,只是box更轻量级 1)需要注意内存选择,4G 2、启动完成后,需要获取服务器IP地址 命令 ip add 服务器IP地址 通过本地的工具,进…...
mvcc 速读
MVCC(Multi-Version Concurrency Control,多版本并发控制)是MySQL中一种用于实现数据库并发控制的方法,尤其在InnoDB存储引擎中得到了广泛应用。它的主要作用是提高数据库在高并发场景下的性能,并确保数据的一致性。 …...
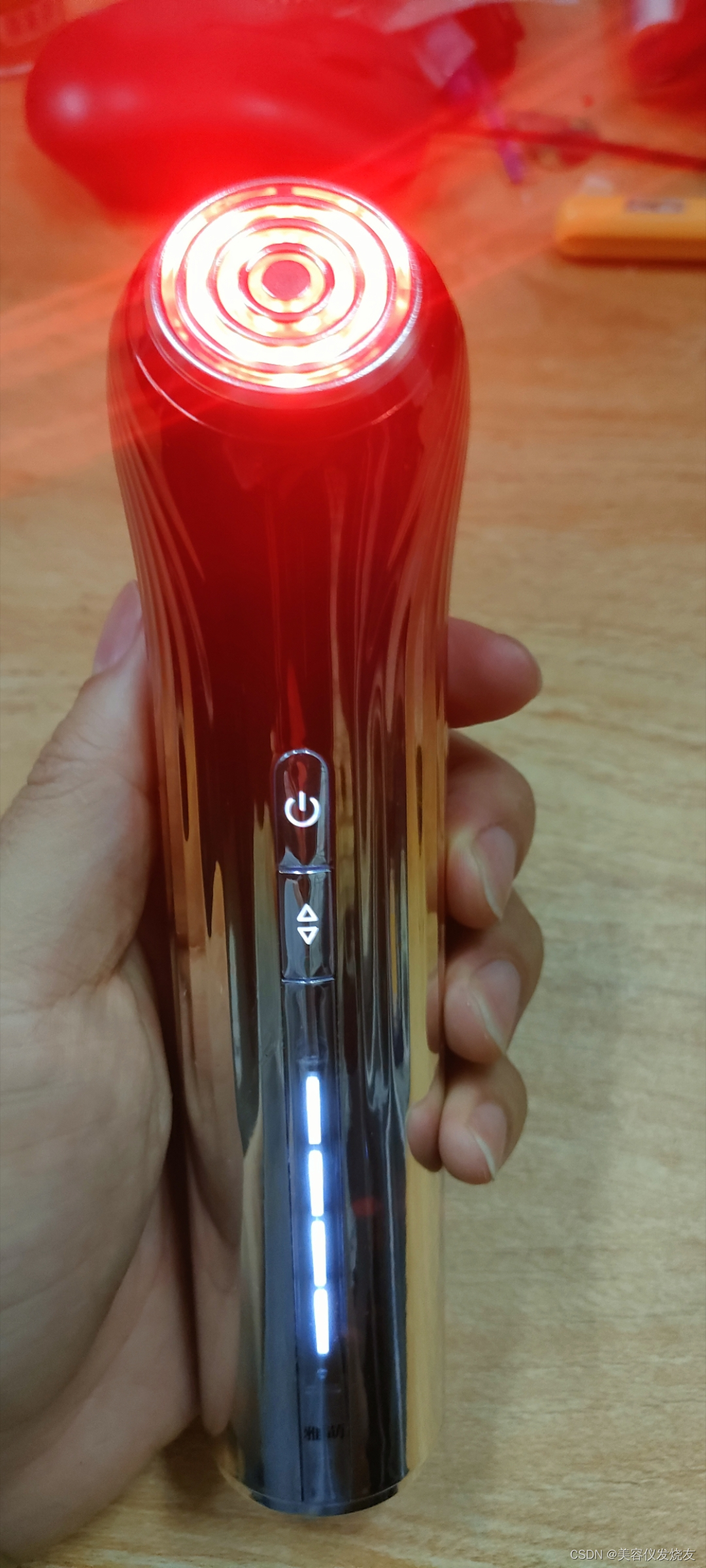
美容仪维修过程记录
近期维修的家用射频美容仪,发一些维修过程的拆机图片...
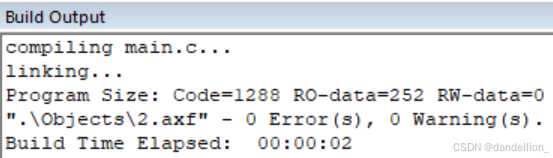
STM32入门开发操作记录(一)——新建工程
目录 一、课程准备1. 课程资料2. 配件清单3. 根目录 二、环境搭建三、新建工程1. 载入器件支持包2. 添加模块3. ST配置4. 外观设置5. 主函数文件 一、课程准备 1. 课程资料 本记录操作流程参考自b站视频BV1th411z7snSTM32入门教程-2023版 细致讲解 中文字幕,课程资…...
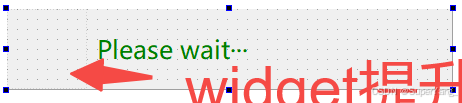
QT实现自定义带有提示信息的透明环形进度条
1. 概述 做界面开发的童鞋可能都会遇到这样的需求,就是有一些界面点击了之后比较耗时的操作,需要界面给出一个环形进度条的进度反馈信息. 如何来实现这样的需求呢,话不多说,上效果 透明进度条 2. 代码实现 waitfeedbackprogressba…...
金币程序题
昨天,小孩问了我一个python编程竞赛题,我看了一下题目,是一个数列编程的问题,我在想,小学五年级的学生能搞得懂吗?反正我家小孩是没有搞懂,不知道别人家的小孩能不能搞明白。所以我花了一点时间…...
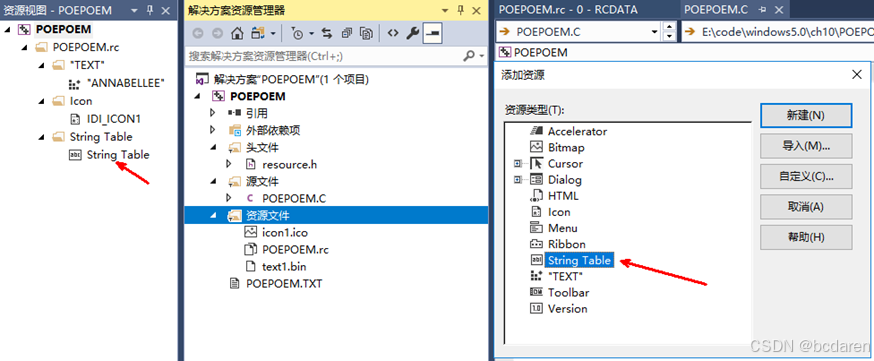
《Windows API每日一练》9.13资源-鼠标位图和字符串
鼠标指针位图(Mouse Cursor Bitmap)是用于表示鼠标指针外观的图像。在 Windows 窗口编程中,可以使用自定义的鼠标指针位图来改变鼠标的外观,并提供更加个性化的用户体验。 ■以下是一些与鼠标指针位图相关的要点: ●…...

【保姆级教程】CenterNet的目标检测、3D检测、关键点检测使用教程
一、代码下载 仓库地址:https://github.com/xingyizhou/CenterNet?tab=readme-ov-file 二、目标检测 2.1 下载预训练权重 下载预训练权重ctdet_coco_dla_2x.pth放到models文件夹下 下载链接:https://drive.google.com/file/d/18Q3fzzAsha_3Qid6mn4jcIFPeOGUaj1d/edit …...
thinkphp:数据库复合查询-OR的使用
完整代码 $data[info] db::table(po_headers_all)->alias(ph) //设置wip_jobs_all的别名->join([vendors > ve], ph.vendor_codeve.vendor_code)->field(ph.po_num,ph.status,ph.vendor_code,ve.vendor_name,ph.po_all_amount,ph.note,ph.order_date,ph.need_dat…...
网络安全那些梗
网络安全领域的梗往往以幽默、讽刺或夸张的方式反映了该领域的某些现象、挑战或误解。以下是一些网络安全相关的梗: 关掉服务器是最有效的安全方法:这个梗源自一个笑话,讲述了一位程序员因误解妻子的话而只买了一个包子回家,随后被…...
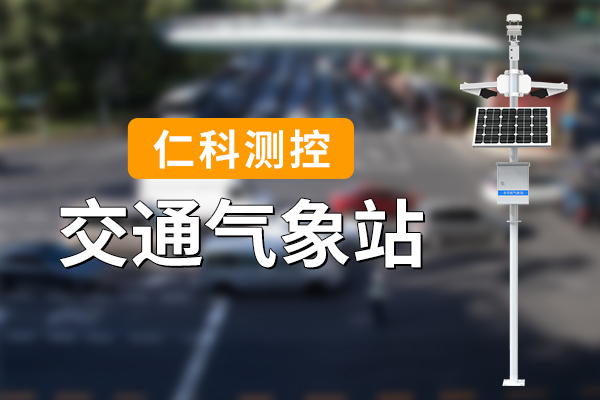
交通气象站:保障道路安全的智慧之眼
随着社会的快速发展,交通运输日益繁忙,道路安全成为公众关注的焦点。在这个背景下,交通气象站作为保障道路安全的重要设施,正发挥着越来越重要的作用。它们不仅为交通管理部门提供及时、准确的气象信息,也为广大驾驶员…...
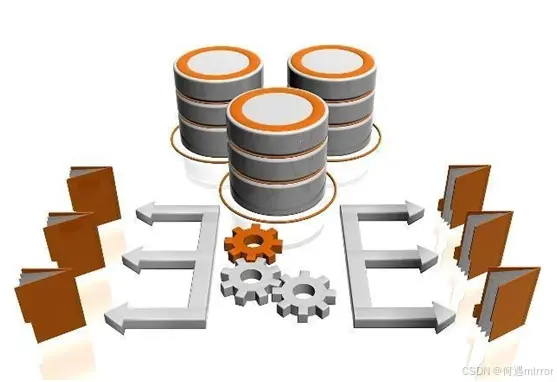
【分库】分库的核心原则
目录 分库的核心原则 前言 分区透明性与一致性保证 弹性伸缩性与容错性设计 数据安全与访问控制机制 分库的核心原则 前言 在设计和实施分库策略时,遵循一系列核心原则是至关重要的,以确保系统不仅能够在当前规模下高效运行,还能够随着…...

【Linux】软件管理工具 yum
文章目录 概念搜索:yum list安装:yum install卸载:yum remove 概念 在Linux下安装软件,可以下载到程序的源代码,进行编译得到可执行程序,另外这些软件还有依赖其它工具的问题,还得下载编译这些依…...
conda相比python好处
Conda 作为 Python 的环境和包管理工具,相比原生 Python 生态(如 pip 虚拟环境)有许多独特优势,尤其在多项目管理、依赖处理和跨平台兼容性等方面表现更优。以下是 Conda 的核心好处: 一、一站式环境管理:…...
Golang dig框架与GraphQL的完美结合
将 Go 的 Dig 依赖注入框架与 GraphQL 结合使用,可以显著提升应用程序的可维护性、可测试性以及灵活性。 Dig 是一个强大的依赖注入容器,能够帮助开发者更好地管理复杂的依赖关系,而 GraphQL 则是一种用于 API 的查询语言,能够提…...
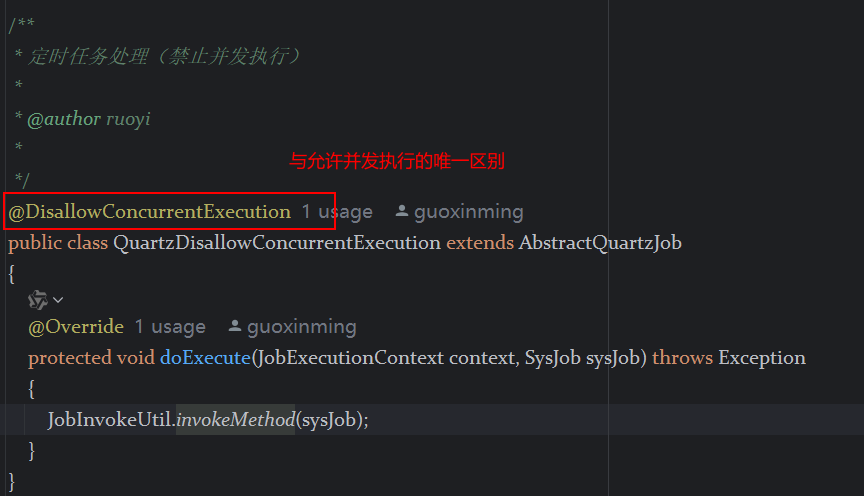
定时器任务——若依源码分析
分析util包下面的工具类schedule utils: ScheduleUtils 是若依中用于与 Quartz 框架交互的工具类,封装了定时任务的 创建、更新、暂停、删除等核心逻辑。 createScheduleJob createScheduleJob 用于将任务注册到 Quartz,先构建任务的 JobD…...
Java - Mysql数据类型对应
Mysql数据类型java数据类型备注整型INT/INTEGERint / java.lang.Integer–BIGINTlong/java.lang.Long–––浮点型FLOATfloat/java.lang.FloatDOUBLEdouble/java.lang.Double–DECIMAL/NUMERICjava.math.BigDecimal字符串型CHARjava.lang.String固定长度字符串VARCHARjava.lang…...
工程地质软件市场:发展现状、趋势与策略建议
一、引言 在工程建设领域,准确把握地质条件是确保项目顺利推进和安全运营的关键。工程地质软件作为处理、分析、模拟和展示工程地质数据的重要工具,正发挥着日益重要的作用。它凭借强大的数据处理能力、三维建模功能、空间分析工具和可视化展示手段&…...
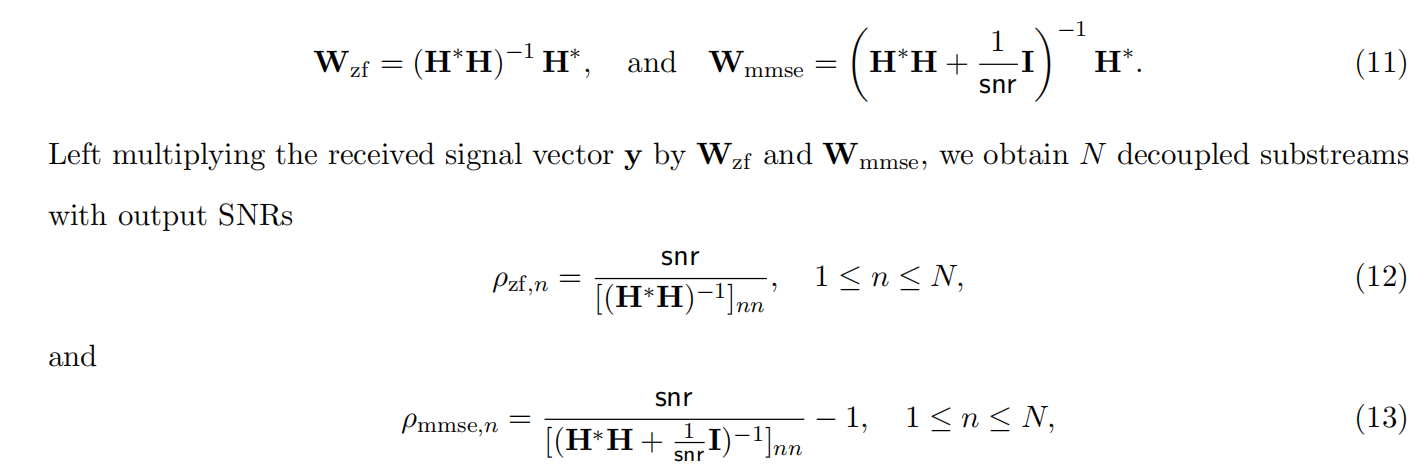
均衡后的SNRSINR
本文主要摘自参考文献中的前两篇,相关文献中经常会出现MIMO检测后的SINR不过一直没有找到相关数学推到过程,其中文献[1]中给出了相关原理在此仅做记录。 1. 系统模型 复信道模型 n t n_t nt 根发送天线, n r n_r nr 根接收天线的 MIMO 系…...
代理篇12|深入理解 Vite中的Proxy接口代理配置
在前端开发中,常常会遇到 跨域请求接口 的情况。为了解决这个问题,Vite 和 Webpack 都提供了 proxy 代理功能,用于将本地开发请求转发到后端服务器。 什么是代理(proxy)? 代理是在开发过程中,前端项目通过开发服务器,将指定的请求“转发”到真实的后端服务器,从而绕…...
rnn判断string中第一次出现a的下标
# coding:utf8 import torch import torch.nn as nn import numpy as np import random import json""" 基于pytorch的网络编写 实现一个RNN网络完成多分类任务 判断字符 a 第一次出现在字符串中的位置 """class TorchModel(nn.Module):def __in…...

SiFli 52把Imagie图片,Font字体资源放在指定位置,编译成指定img.bin和font.bin的问题
分区配置 (ptab.json) img 属性介绍: img 属性指定分区存放的 image 名称,指定的 image 名称必须是当前工程生成的 binary 。 如果 binary 有多个文件,则以 proj_name:binary_name 格式指定文件名, proj_name 为工程 名&…...
Linux离线(zip方式)安装docker
目录 基础信息操作系统信息docker信息 安装实例安装步骤示例 遇到的问题问题1:修改默认工作路径启动失败问题2 找不到对应组 基础信息 操作系统信息 OS版本:CentOS 7 64位 内核版本:3.10.0 相关命令: uname -rcat /etc/os-rele…...