架构以及架构中的组件
架构以及架构中的组件
- Transform
Transform
以下的代码包含:
- 标准化的示例
- 残差化的示例
# huggingface
# transformers# https://www.bilibili.com/video/BV1At4y1W75x?spm_id_from=333.999.0.0import copy
import math
from collections import namedtupleimport numpy as np
import torch
import torch.nn as nn
import torch.nn.functional as F
from torch.autograd import VariableHypothesis = namedtuple('Hypothesis', ['value', 'score'])def clones(module, n):return nn.ModuleList([copy.deepcopy(module) for _ in range(n)])"""
实现x 的标准化处理(标准化的作用:使x符合正太分布)
"""
class LayerNorm(nn.Module):def __init__(self, feature, eps=1e-6):""":param feature: self-attention 的 x 的大小:param eps:"""super(LayerNorm, self).__init__()self.a_2 = nn.Parameter(torch.ones(feature))self.b_2 = nn.Parameter(torch.zeros(feature))self.eps = epsdef forward(self, x):mean = x.mean(-1, keepdim=True)std = x.std(-1, keepdim=True)return self.a_2 * (x - mean) / (std + self.eps) + self.b_2"""
残差化的示例
"""
class SublayerConnection(nn.Module):"""这不仅仅做了残差,这是把残差和 layernorm 一起给做了"""def __init__(self, size, dropout=0.1):super(SublayerConnection, self).__init__()# 第一步做 layernorm 这是类的实例化的一种方法self.layer_norm = LayerNorm(size)# 第二步做 dropoutself.dropout = nn.Dropout(p=dropout)def forward(self, x, sublayer):""":param x: 就是self-attention的输入:param sublayer: self-attention层:return:"""return self.dropout(self.layer_norm(x + sublayer(x)))class FeatEmbedding(nn.Module):def __init__(self, d_feat, d_model, dropout):super(FeatEmbedding, self).__init__()self.video_embeddings = nn.Sequential(LayerNorm(d_feat),nn.Dropout(dropout),nn.Linear(d_feat, d_model))def forward(self, x):return self.video_embeddings(x)class TextEmbedding(nn.Module):def __init__(self, vocab_size, d_model):super(TextEmbedding, self).__init__()self.d_model = d_modelself.embed = nn.Embedding(vocab_size, d_model)def forward(self, x):return self.embed(x) * math.sqrt(self.d_model)"""
给一个词向量添加位置编码的示例
"""
class PositionalEncoding(nn.Module):def __init__(self, dim, dropout, max_len=5000):if dim % 2 != 0:raise ValueError("Cannot use sin/cos positional encoding with ""odd dim (got dim={:d})".format(dim))pe = torch.zeros(max_len, dim)position = torch.arange(0, max_len).unsqueeze(1)div_term = torch.exp((torch.arange(0, dim, 2, dtype=torch.float) *-(math.log(10000.0) / dim)))pe[:, 0::2] = torch.sin(position.float() * div_term)pe[:, 1::2] = torch.cos(position.float() * div_term)pe = pe.unsqueeze(1)super(PositionalEncoding, self).__init__()self.register_buffer('pe', pe)self.drop_out = nn.Dropout(p=dropout)self.dim = dimdef forward(self, emb, step=None):emb = emb * math.sqrt(self.dim)if step is None:emb = emb + self.pe[:emb.size(0)]else:emb = emb + self.pe[step]emb = self.drop_out(emb)return emb"""
自注意力机制的实现示例
"""
def self_attention(query, key, value, dropout=None, mask=None):d_k = query.size(-1)scores = torch.matmul(query, key.transpose(-2, -1)) / math.sqrt(d_k)# mask的操作在QK之后,softmax之前if mask is not None:mask.cuda()scores = scores.masked_fill(mask == 0, -1e9)self_attn = F.softmax(scores, dim=-1)if dropout is not None:self_attn = dropout(self_attn)return torch.matmul(self_attn, value), self_attn"""
多头--注意力机制的实现示例
"""
class MultiHeadAttention(nn.Module):def __init__(self, head, d_model, dropout=0.1):super(MultiHeadAttention, self).__init__()assert (d_model % head == 0)self.d_k = d_model // headself.head = headself.d_model = d_modelself.linear_query = nn.Linear(d_model, d_model)self.linear_key = nn.Linear(d_model, d_model)self.linear_value = nn.Linear(d_model, d_model)self.linear_out = nn.Linear(d_model, d_model)self.dropout = nn.Dropout(p=dropout)self.attn = Nonedef forward(self, query, key, value, mask=None):if mask is not None:# 多头注意力机制的线性变换层是4维,是把query[batch, frame_num, d_model]变成[batch, -1, head, d_k]# 再1,2维交换变成[batch, head, -1, d_k], 所以mask要在第一维添加一维,与后面的self attention计算维度一样mask = mask.unsqueeze(1)n_batch = query.size(0)# if self.head == 1:# x, self.attn = self_attention(query, key, value, dropout=self.dropout, mask=mask)# else:# query = self.linear_query(query).view(n_batch, -1, self.head, self.d_k).transpose(1, 2) # [b, 8, 32, 64]# key = self.linear_key(key).view(n_batch, -1, self.head, self.d_k).transpose(1, 2) # [b, 8, 28, 64]# value = self.linear_value(value).view(n_batch, -1, self.head, self.d_k).transpose(1, 2) # [b, 8, 28, 64]## x, self.attn = self_attention(query, key, value, dropout=self.dropout, mask=mask)# # 变为三维, 或者说是concat head# x = x.transpose(1, 2).contiguous().view(n_batch, -1, self.head * self.d_k)query = self.linear_query(query).view(n_batch, -1, self.head, self.d_k).transpose(1, 2) # [b, 8, 32, 64]key = self.linear_key(key).view(n_batch, -1, self.head, self.d_k).transpose(1, 2) # [b, 8, 28, 64]value = self.linear_value(value).view(n_batch, -1, self.head, self.d_k).transpose(1, 2) # [b, 8, 28, 64]x, self.attn = self_attention(query, key, value, dropout=self.dropout, mask=mask)# 变为三维, 或者说是concat headx = x.transpose(1, 2).contiguous().view(n_batch, -1, self.head * self.d_k)return self.linear_out(x)class PositionWiseFeedForward(nn.Module):def __init__(self, d_model, d_ff, dropout=0.1):super(PositionWiseFeedForward, self).__init__()self.w_1 = nn.Linear(d_model, d_ff)self.w_2 = nn.Linear(d_ff, d_model)self.layer_norm = nn.LayerNorm(d_model, eps=1e-6)self.dropout_1 = nn.Dropout(dropout)self.relu = nn.ReLU()self.dropout_2 = nn.Dropout(dropout)def forward(self, x):inter = self.dropout_1(self.relu(self.w_1(self.layer_norm(x))))output = self.dropout_2(self.w_2(inter))return outputclass EncoderLayer(nn.Module):def __init__(self, size, attn, feed_forward, dropout=0.1):super(EncoderLayer, self).__init__()self.attn = attnself.feed_forward = feed_forwardself.sublayer_connection = clones(SublayerConnection(size, dropout), 2)def forward(self, x, mask):x = self.sublayer_connection[0](x, lambda x: self.attn(x, x, x, mask))return self.sublayer_connection[1](x, self.feed_forward)class EncoderLayerNoAttention(nn.Module):def __init__(self, size, attn, feed_forward, dropout=0.1):super(EncoderLayerNoAttention, self).__init__()self.attn = attnself.feed_forward = feed_forwardself.sublayer_connection = clones(SublayerConnection(size, dropout), 2)def forward(self, x, mask):return self.sublayer_connection[1](x, self.feed_forward)class DecoderLayer(nn.Module):def __init__(self, size, attn, feed_forward, sublayer_num, dropout=0.1):super(DecoderLayer, self).__init__()self.attn = attnself.feed_forward = feed_forwardself.sublayer_connection = clones(SublayerConnection(size, dropout), sublayer_num)def forward(self, x, memory, src_mask, trg_mask, r2l_memory=None, r2l_trg_mask=None):x = self.sublayer_connection[0](x, lambda x: self.attn(x, x, x, trg_mask))x = self.sublayer_connection[1](x, lambda x: self.attn(x, memory, memory, src_mask))if r2l_memory is not None:x = self.sublayer_connection[-2](x, lambda x: self.attn(x, r2l_memory, r2l_memory, r2l_trg_mask))return self.sublayer_connection[-1](x, self.feed_forward)class Encoder(nn.Module):def __init__(self, n, encoder_layer):super(Encoder, self).__init__()self.encoder_layer = clones(encoder_layer, n)def forward(self, x, src_mask):for layer in self.encoder_layer:x = layer(x, src_mask)return xclass R2L_Decoder(nn.Module):def __init__(self, n, decoder_layer):super(R2L_Decoder, self).__init__()self.decoder_layer = clones(decoder_layer, n)def forward(self, x, memory, src_mask, r2l_trg_mask):for layer in self.decoder_layer:x = layer(x, memory, src_mask, r2l_trg_mask)return xclass L2R_Decoder(nn.Module):def __init__(self, n, decoder_layer):super(L2R_Decoder, self).__init__()self.decoder_layer = clones(decoder_layer, n)def forward(self, x, memory, src_mask, trg_mask, r2l_memory, r2l_trg_mask):for layer in self.decoder_layer:x = layer(x, memory, src_mask, trg_mask, r2l_memory, r2l_trg_mask)return xdef pad_mask(src, r2l_trg, trg, pad_idx):if isinstance(src, tuple):if len(src) == 4:src_image_mask = (src[0][:, :, 0] != pad_idx).unsqueeze(1)src_motion_mask = (src[1][:, :, 0] != pad_idx).unsqueeze(1)src_object_mask = (src[2][:, :, 0] != pad_idx).unsqueeze(1)src_rel_mask = (src[3][:, :, 0] != pad_idx).unsqueeze(1)enc_src_mask = (src_image_mask, src_motion_mask, src_object_mask, src_rel_mask)dec_src_mask_1 = src_image_mask & src_motion_maskdec_src_mask_2 = src_image_mask & src_motion_mask & src_object_mask & src_rel_maskdec_src_mask = (dec_src_mask_1, dec_src_mask_2)src_mask = (enc_src_mask, dec_src_mask)if len(src) == 3:src_image_mask = (src[0][:, :, 0] != pad_idx).unsqueeze(1)src_motion_mask = (src[1][:, :, 0] != pad_idx).unsqueeze(1)src_object_mask = (src[2][:, :, 0] != pad_idx).unsqueeze(1)enc_src_mask = (src_image_mask, src_motion_mask, src_object_mask)dec_src_mask = src_image_mask & src_motion_masksrc_mask = (enc_src_mask, dec_src_mask)if len(src) == 2:src_image_mask = (src[0][:, :, 0] != pad_idx).unsqueeze(1)src_motion_mask = (src[1][:, :, 0] != pad_idx).unsqueeze(1)enc_src_mask = (src_image_mask, src_motion_mask)dec_src_mask = src_image_mask & src_motion_masksrc_mask = (enc_src_mask, dec_src_mask)else:src_mask = (src[:, :, 0] != pad_idx).unsqueeze(1)if trg is not None:if isinstance(src_mask, tuple):trg_mask = (trg != pad_idx).unsqueeze(1) & subsequent_mask(trg.size(1)).type_as(src_image_mask.data)r2l_pad_mask = (r2l_trg != pad_idx).unsqueeze(1).type_as(src_image_mask.data)r2l_trg_mask = r2l_pad_mask & subsequent_mask(r2l_trg.size(1)).type_as(src_image_mask.data)return src_mask, r2l_pad_mask, r2l_trg_mask, trg_maskelse:trg_mask = (trg != pad_idx).unsqueeze(1) & subsequent_mask(trg.size(1)).type_as(src_mask.data)r2l_pad_mask = (r2l_trg != pad_idx).unsqueeze(1).type_as(src_mask.data)r2l_trg_mask = r2l_pad_mask & subsequent_mask(r2l_trg.size(1)).type_as(src_mask.data)return src_mask, r2l_pad_mask, r2l_trg_mask, trg_mask # src_mask[batch, 1, lens] trg_mask[batch, 1, lens]else:return src_maskdef subsequent_mask(size):"""Mask out subsequent positions."""attn_shape = (1, size, size)mask = np.triu(np.ones(attn_shape), k=1).astype('uint8')return (torch.from_numpy(mask) == 0).cuda()class Generator(nn.Module):def __init__(self, d_model, vocab_size):super(Generator, self).__init__()self.linear = nn.Linear(d_model, vocab_size)def forward(self, x):return F.log_softmax(self.linear(x), dim=-1)class ABDTransformer(nn.Module):def __init__(self, vocab, d_feat, d_model, d_ff, n_heads, n_layers, dropout, feature_mode,device='cuda', n_heads_big=128):super(ABDTransformer, self).__init__()self.vocab = vocabself.device = deviceself.feature_mode = feature_modec = copy.deepcopy# attn_no_heads = MultiHeadAttention(1, d_model, dropout)attn = MultiHeadAttention(n_heads, d_model, dropout)attn_big = MultiHeadAttention(n_heads_big, d_model, dropout)# attn_big2 = MultiHeadAttention(10, d_model, dropout)feed_forward = PositionWiseFeedForward(d_model, d_ff)if feature_mode == 'one':self.src_embed = FeatEmbedding(d_feat, d_model, dropout)elif feature_mode == 'two':self.image_src_embed = FeatEmbedding(d_feat[0], d_model, dropout)self.motion_src_embed = FeatEmbedding(d_feat[1], d_model, dropout)elif feature_mode == 'three':self.image_src_embed = FeatEmbedding(d_feat[0], d_model, dropout)self.motion_src_embed = FeatEmbedding(d_feat[1], d_model, dropout)self.object_src_embed = FeatEmbedding(d_feat[2], d_model, dropout)elif feature_mode == 'four':self.image_src_embed = FeatEmbedding(d_feat[0], d_model, dropout)self.motion_src_embed = FeatEmbedding(d_feat[1], d_model, dropout)self.object_src_embed = FeatEmbedding(d_feat[2], d_model, dropout)self.rel_src_embed = FeatEmbedding(d_feat[3], d_model, dropout)self.trg_embed = TextEmbedding(vocab.n_vocabs, d_model)self.pos_embed = PositionalEncoding(d_model, dropout)# self.encoder_no_heads = Encoder(n_layers, EncoderLayer(d_model, c(attn_no_heads), c(feed_forward), dropout))self.encoder = Encoder(n_layers, EncoderLayer(d_model, c(attn), c(feed_forward), dropout))self.encoder_big = Encoder(n_layers, EncoderLayer(d_model, c(attn_big), c(feed_forward), dropout))# self.encoder_big2 = Encoder(n_layers, EncoderLayer(d_model, c(attn_big2), c(feed_forward), dropout))self.encoder_no_attention = Encoder(n_layers,EncoderLayerNoAttention(d_model, c(attn), c(feed_forward), dropout))self.r2l_decoder = R2L_Decoder(n_layers, DecoderLayer(d_model, c(attn), c(feed_forward),sublayer_num=3, dropout=dropout))self.l2r_decoder = L2R_Decoder(n_layers, DecoderLayer(d_model, c(attn), c(feed_forward),sublayer_num=4, dropout=dropout))self.generator = Generator(d_model, vocab.n_vocabs)def encode(self, src, src_mask, feature_mode_two=False):if self.feature_mode == 'two':x1 = self.image_src_embed(src[0])x1 = self.pos_embed(x1)x1 = self.encoder_big(x1, src_mask[0])x2 = self.motion_src_embed(src[1])x2 = self.pos_embed(x2)x2 = self.encoder_big(x2, src_mask[1])return x1 + x2if feature_mode_two:x1 = self.image_src_embed(src[0])x1 = self.pos_embed(x1)x1 = self.encoder_big(x1, src_mask[0])x2 = self.motion_src_embed(src[1])x2 = self.pos_embed(x2)x2 = self.encoder_big(x2, src_mask[1])return x1 + x2if self.feature_mode == 'one':x = self.src_embed(src)x = self.pos_embed(x)return self.encoder(x, src_mask)elif self.feature_mode == 'two':x1 = self.image_src_embed(src[0])x1 = self.pos_embed(x1)x1 = self.encoder_big(x1, src_mask[0])x2 = self.motion_src_embed(src[1])x2 = self.pos_embed(x2)x2 = self.encoder_big(x2, src_mask[1])return x1 + x2elif self.feature_mode == 'three':x1 = self.image_src_embed(src[0])x1 = self.pos_embed(x1)x1 = self.encoder(x1, src_mask[0])x2 = self.motion_src_embed(src[1])x2 = self.pos_embed(x2)x2 = self.encoder(x2, src_mask[1])x3 = self.object_src_embed(src[2])x3 = self.pos_embed(x3)x3 = self.encoder(x3, src_mask[2])return x1 + x2 + x3elif self.feature_mode == 'four':x1 = self.image_src_embed(src[0])x1 = self.pos_embed(x1)x1 = self.encoder(x1, src_mask[0])x2 = self.motion_src_embed(src[1])x2 = self.pos_embed(x2)x2 = self.encoder(x2, src_mask[1])x3 = self.object_src_embed(src[2])# x3 = self.pos_embed(x3)x3 = self.encoder(x3, src_mask[2])# x3 = self.encoder_no_attention(x3, src_mask[2])x4 = self.rel_src_embed(src[3])# x4 = self.pos_embed(x4)# x4 = self.encoder_no_# heads(x4, src_mask[3])x4 = self.encoder_no_attention(x4, src_mask[3])# x4 = self.encoder(x4, src_mask[3])return x1 + x2 + x3 + x4def r2l_decode(self, r2l_trg, memory, src_mask, r2l_trg_mask):x = self.trg_embed(r2l_trg)x = self.pos_embed(x)return self.r2l_decoder(x, memory, src_mask, r2l_trg_mask)def l2r_decode(self, trg, memory, src_mask, trg_mask, r2l_memory, r2l_trg_mask):x = self.trg_embed(trg)x = self.pos_embed(x)return self.l2r_decoder(x, memory, src_mask, trg_mask, r2l_memory, r2l_trg_mask)def forward(self, src, r2l_trg, trg, mask):src_mask, r2l_pad_mask, r2l_trg_mask, trg_mask = maskif self.feature_mode == 'one':encoding_outputs = self.encode(src, src_mask)r2l_outputs = self.r2l_decode(r2l_trg, encoding_outputs, src_mask, r2l_trg_mask)l2r_outputs = self.l2r_decode(trg, encoding_outputs, src_mask, trg_mask, r2l_outputs, r2l_pad_mask)elif self.feature_mode == 'two' or 'three' or 'four':enc_src_mask, dec_src_mask = src_maskr2l_encoding_outputs = self.encode(src, enc_src_mask, feature_mode_two=True)encoding_outputs = self.encode(src, enc_src_mask)r2l_outputs = self.r2l_decode(r2l_trg, r2l_encoding_outputs, dec_src_mask[0], r2l_trg_mask)l2r_outputs = self.l2r_decode(trg, encoding_outputs, dec_src_mask[1], trg_mask, r2l_outputs, r2l_pad_mask)# r2l_outputs = self.r2l_decode(r2l_trg, encoding_outputs, dec_src_mask, r2l_trg_mask)# l2r_outputs = self.l2r_decode(trg, encoding_outputs, dec_src_mask, trg_mask, None, None)else:raise "没有输出"r2l_pred = self.generator(r2l_outputs)l2r_pred = self.generator(l2r_outputs)return r2l_pred, l2r_preddef greedy_decode(self, batch_size, src_mask, memory, max_len):eos_idx = self.vocab.word2idx['<S>']r2l_hidden = Nonewith torch.no_grad():output = torch.ones(batch_size, 1).fill_(eos_idx).long().cuda()for i in range(max_len + 2 - 1):trg_mask = subsequent_mask(output.size(1))dec_out = self.r2l_decode(output, memory, src_mask, trg_mask) # batch, len, d_modelr2l_hidden = dec_outpred = self.generator(dec_out) # batch, len, n_vocabsnext_word = pred[:, -1].max(dim=-1)[1].unsqueeze(1) # pred[:, -1]([batch, n_vocabs])output = torch.cat([output, next_word], dim=-1)return r2l_hidden, output# beam search 必用的def r2l_beam_search_decode(self, batch_size, src, src_mask, model_encodings, beam_size, max_len):end_symbol = self.vocab.word2idx['<S>']start_symbol = self.vocab.word2idx['<S>']r2l_outputs = None# 1.1 Setup Src"src has shape (batch_size, sent_len)""src_mask has shape (batch_size, 1, sent_len)"# src_mask = (src[:, :, 0] != self.vocab.word2idx['<PAD>']).unsqueeze(-2) # TODO Untested"model_encodings has shape (batch_size, sentence_len, d_model)"# model_encodings = self.encode(src, src_mask)# 1.2 Setup Tgt Hypothesis Tracking"hypothesis is List(4 bt)[(cur beam_sz, dec_sent_len)], init: List(4 bt)[(1 init_beam_sz, dec_sent_len)]""hypotheses[i] is shape (cur beam_sz, dec_sent_len)"hypotheses = [copy.deepcopy(torch.full((1, 1), start_symbol, dtype=torch.long,device=self.device)) for _ in range(batch_size)]"List after init: List 4 bt of List of len max_len_completed, init: List of len 4 bt of []"completed_hypotheses = [copy.deepcopy([]) for _ in range(batch_size)]"List len batch_sz of shape (cur beam_sz), init: List(4 bt)[(1 init_beam_sz)]""hyp_scores[i] is shape (cur beam_sz)"hyp_scores = [copy.deepcopy(torch.full((1,), 0, dtype=torch.float, device=self.device))for _ in range(batch_size)] # probs are log_probs must be init at 0.# 2. Iterate: Generate one char at a time until maxlenfor iter in range(max_len + 1):if all([len(completed_hypotheses[i]) == beam_size for i in range(batch_size)]):break# 2.1 Setup the batch. Since we use beam search, each batch has a variable number (called cur_beam_size)# between 0 and beam_size of hypotheses live at any moment. We decode all hypotheses for all batches at# the same time, so we must copy the src_encodings, src_mask, etc the appropriate number fo times for# the number of hypotheses for each example. We keep track of the number of live hypotheses for each example.# We run all hypotheses for all examples together through the decoder and log-softmax,# and then use `torch.split` to get the appropriate number of hypotheses for each example in the end.cur_beam_sizes, last_tokens, model_encodings_l, src_mask_l = [], [], [], []for i in range(batch_size):if hypotheses[i] is None:cur_beam_sizes += [0]continuecur_beam_size, decoded_len = hypotheses[i].shapecur_beam_sizes += [cur_beam_size]last_tokens += [hypotheses[i]]model_encodings_l += [model_encodings[i:i + 1]] * cur_beam_sizesrc_mask_l += [src_mask[i:i + 1]] * cur_beam_size"shape (sum(4 bt * cur_beam_sz_i), 1 dec_sent_len, 128 d_model)"model_encodings_cur = torch.cat(model_encodings_l, dim=0)src_mask_cur = torch.cat(src_mask_l, dim=0)y_tm1 = torch.cat(last_tokens, dim=0)"shape (sum(4 bt * cur_beam_sz_i), 1 dec_sent_len, 128 d_model)"if self.feature_mode == 'one':out = self.r2l_decode(Variable(y_tm1).to(self.device), model_encodings_cur, src_mask_cur,Variable(subsequent_mask(y_tm1.size(-1)).type_as(src.data)).to(self.device))elif self.feature_mode == 'two' or 'three' or 'four':out = self.r2l_decode(Variable(y_tm1).to(self.device), model_encodings_cur, src_mask_cur,Variable(subsequent_mask(y_tm1.size(-1)).type_as(src[0].data)).to(self.device))r2l_outputs = out"shape (sum(4 bt * cur_beam_sz_i), 1 dec_sent_len, 50002 vocab_sz)"log_prob = self.generator(out[:, -1, :]).unsqueeze(1)"shape (sum(4 bt * cur_beam_sz_i), 1 dec_sent_len, 50002 vocab_sz)"_, decoded_len, vocab_sz = log_prob.shape# log_prob = log_prob.reshape(batch_size, cur_beam_size, decoded_len, vocab_sz)"shape List(4 bt)[(cur_beam_sz_i, dec_sent_len, 50002 vocab_sz)]""log_prob[i] is (cur_beam_sz_i, dec_sent_len, 50002 vocab_sz)"log_prob = torch.split(log_prob, cur_beam_sizes, dim=0)# 2.2 Now we process each example in the batch. Note that the example may have already finished processing before# other examples (no more hypotheses to try), in which case we continuenew_hypotheses, new_hyp_scores = [], []for i in range(batch_size):if hypotheses[i] is None or len(completed_hypotheses[i]) >= beam_size:new_hypotheses += [None]new_hyp_scores += [None]continue# 2.2.1 We compute the cumulative scores for each live hypotheses for the example# hyp_scores is the old scores for the previous stage, and `log_prob` are the new probs for# this stage. Since they are log probs, we sum them instaed of multiplying them.# The .view(-1) forces all the hypotheses into one dimension. The shape of this dimension is# cur_beam_sz * vocab_sz (ex: 5 * 50002). So after getting the topk from it, we can recover the# generating sentence and the next word using: ix // vocab_sz, ix % vocab_sz.cur_beam_sz_i, dec_sent_len, vocab_sz = log_prob[i].shape"shape (vocab_sz,)"cumulative_hyp_scores_i = (hyp_scores[i].unsqueeze(-1).unsqueeze(-1).expand((cur_beam_sz_i, 1, vocab_sz)) + log_prob[i]).view(-1)# 2.2.2 We get the topk values in cumulative_hyp_scores_i and compute the current (generating) sentence# and the next word using: ix // vocab_sz, ix % vocab_sz."shape (cur_beam_sz,)"live_hyp_num_i = beam_size - len(completed_hypotheses[i])"shape (cur_beam_sz,). Vals are between 0 and 50002 vocab_sz"top_cand_hyp_scores, top_cand_hyp_pos = torch.topk(cumulative_hyp_scores_i, k=live_hyp_num_i)"shape (cur_beam_sz,). prev_hyp_ids vals are 0 <= val < cur_beam_sz. hyp_word_ids vals are 0 <= val < vocab_len"prev_hyp_ids, hyp_word_ids = top_cand_hyp_pos // self.vocab.n_vocabs, \top_cand_hyp_pos % self.vocab.n_vocabs# 2.2.3 For each of the topk words, we append the new word to the current (generating) sentence# We add this to new_hypotheses_i and add its corresponding total score to new_hyp_scores_inew_hypotheses_i, new_hyp_scores_i = [], [] # Removed live_hyp_ids_i, which is used in the LSTM decoder to track live hypothesis idsfor prev_hyp_id, hyp_word_id, cand_new_hyp_score in zip(prev_hyp_ids, hyp_word_ids,top_cand_hyp_scores):prev_hyp_id, hyp_word_id, cand_new_hyp_score = \prev_hyp_id.item(), hyp_word_id.item(), cand_new_hyp_score.item()new_hyp_sent = torch.cat((hypotheses[i][prev_hyp_id], torch.tensor([hyp_word_id], device=self.device)))if hyp_word_id == end_symbol:completed_hypotheses[i].append(Hypothesis(value=[self.vocab.idx2word[a.item()] for a in new_hyp_sent[1:-1]],score=cand_new_hyp_score))else:new_hypotheses_i.append(new_hyp_sent.unsqueeze(-1))new_hyp_scores_i.append(cand_new_hyp_score)# 2.2.4 We may find that the hypotheses_i for some example in the batch# is empty - we have fully processed that example. We use None as a sentinel in this case.# Above, the loops gracefully handle None examples.if len(new_hypotheses_i) > 0:hypotheses_i = torch.cat(new_hypotheses_i, dim=-1).transpose(0, -1).to(self.device)hyp_scores_i = torch.tensor(new_hyp_scores_i, dtype=torch.float, device=self.device)else:hypotheses_i, hyp_scores_i = None, Nonenew_hypotheses += [hypotheses_i]new_hyp_scores += [hyp_scores_i]# print(new_hypotheses, new_hyp_scores)hypotheses, hyp_scores = new_hypotheses, new_hyp_scores# 2.3 Finally, we do some postprocessing to get our final generated candidate sentences.# Sometimes, we may get to max_len of a sentence and still not generate the </s> end token.# In this case, the partial sentence we have generated will not be added to the completed_hypotheses# automatically, and we have to manually add it in. We add in as many as necessary so that there are# `beam_size` completed hypotheses for each example.# Finally, we sort each completed hypothesis by score.for i in range(batch_size):hyps_to_add = beam_size - len(completed_hypotheses[i])if hyps_to_add > 0:scores, ix = torch.topk(hyp_scores[i], k=hyps_to_add)for score, id in zip(scores, ix):completed_hypotheses[i].append(Hypothesis(value=[self.vocab.idx2word[a.item()] for a in hypotheses[i][id][1:]],score=score))completed_hypotheses[i].sort(key=lambda hyp: hyp.score, reverse=True)return r2l_outputs, completed_hypothesesdef beam_search_decode(self, src, beam_size, max_len):"""An Implementation of Beam Search for the Transformer Model.Beam search is performed in a batched manner. Each example in a batch generates `beam_size` hypotheses.We return a list (len: batch_size) of list (len: beam_size) of Hypothesis, which contain our output decoded sentencesand their scores.:param src: shape (sent_len, batch_size). Each val is 0 < val < len(vocab_dec). The input tokens to the decoder.:param max_len: the maximum length to decode:param beam_size: the beam size to use:return completed_hypotheses: A List of length batch_size, each containing a List of beam_size Hypothesis objects.Hypothesis is a named Tuple, its first entry is "value" and is a List of strings which contains the translated word(one string is one word token). The second entry is "score" and it is the log-prob score for this translated sentence.Note: Below I note "4 bt", "5 beam_size" as the shapes of objects. 4, 5 are default values. Actual values may differ."""# 1. Setupstart_symbol = self.vocab.word2idx['<S>']end_symbol = self.vocab.word2idx['<S>']# 1.1 Setup Src"src has shape (batch_size, sent_len)""src_mask has shape (batch_size, 1, sent_len)"# src_mask = (src[:, :, 0] != self.vocab.word2idx['<PAD>']).unsqueeze(-2) # TODO Untestedsrc_mask = pad_mask(src, r2l_trg=None, trg=None, pad_idx=self.vocab.word2idx['<PAD>'])"model_encodings has shape (batch_size, sentence_len, d_model)"if self.feature_mode == 'one':batch_size = src.shape[0]model_encodings = self.encode(src, src_mask)r2l_memory, r2l_completed_hypotheses = self.r2l_beam_search_decode(batch_size, src, src_mask,model_encodings=model_encodings,beam_size=beam_size, max_len=max_len)elif self.feature_mode == 'two' or 'three' or 'four':batch_size = src[0].shape[0]enc_src_mask = src_mask[0]dec_src_mask = src_mask[1]r2l_model_encodings = self.encode(src, enc_src_mask, feature_mode_two=True)# model_encodings = r2l_model_encodingsmodel_encodings = self.encode(src, enc_src_mask)r2l_memory, r2l_completed_hypotheses = self.r2l_beam_search_decode(batch_size, src, dec_src_mask[0],model_encodings=r2l_model_encodings,beam_size=beam_size, max_len=max_len)# 1.2 Setup r2l target output# r2l_memory, r2l_completed_hypotheses = self.r2l_beam_search_decode(batch_size, src, src_mask,# model_encodings=model_encodings,# beam_size=1, max_len=max_len)# r2l_memory, r2l_completed_hypotheses = self.greedy_decode(batch_size, src_mask, model_encodings, max_len)# beam_r2l_memory = [copy.deepcopy(r2l_memory) for _ in range(beam_size)]# 1.3 Setup Tgt Hypothesis Tracking"hypothesis is List(4 bt)[(cur beam_sz, dec_sent_len)], init: List(4 bt)[(1 init_beam_sz, dec_sent_len)]""hypotheses[i] is shape (cur beam_sz, dec_sent_len)"hypotheses = [copy.deepcopy(torch.full((1, 1), start_symbol, dtype=torch.long,device=self.device)) for _ in range(batch_size)]"List after init: List 4 bt of List of len max_len_completed, init: List of len 4 bt of []"completed_hypotheses = [copy.deepcopy([]) for _ in range(batch_size)]"List len batch_sz of shape (cur beam_sz), init: List(4 bt)[(1 init_beam_sz)]""hyp_scores[i] is shape (cur beam_sz)"hyp_scores = [copy.deepcopy(torch.full((1,), 0, dtype=torch.float, device=self.device))for _ in range(batch_size)] # probs are log_probs must be init at 0.# 2. Iterate: Generate one char at a time until maxlenfor iter in range(max_len + 1):if all([len(completed_hypotheses[i]) == beam_size for i in range(batch_size)]):break# 2.1 Setup the batch. Since we use beam search, each batch has a variable number (called cur_beam_size)# between 0 and beam_size of hypotheses live at any moment. We decode all hypotheses for all batches at# the same time, so we must copy the src_encodings, src_mask, etc the appropriate number fo times for# the number of hypotheses for each example. We keep track of the number of live hypotheses for each example.# We run all hypotheses for all examples together through the decoder and log-softmax,# and then use `torch.split` to get the appropriate number of hypotheses for each example in the end.cur_beam_sizes, last_tokens, model_encodings_l, src_mask_l, r2l_memory_l = [], [], [], [], []for i in range(batch_size):if hypotheses[i] is None:cur_beam_sizes += [0]continuecur_beam_size, decoded_len = hypotheses[i].shapecur_beam_sizes += [cur_beam_size]last_tokens += [hypotheses[i]]model_encodings_l += [model_encodings[i:i + 1]] * cur_beam_sizeif self.feature_mode == 'one':src_mask_l += [src_mask[i:i + 1]] * cur_beam_sizeelif self.feature_mode == 'two' or 'three' or 'four':src_mask_l += [dec_src_mask[1][i:i + 1]] * cur_beam_sizer2l_memory_l += [r2l_memory[i: i + 1]] * cur_beam_size"shape (sum(4 bt * cur_beam_sz_i), 1 dec_sent_len, 128 d_model)"model_encodings_cur = torch.cat(model_encodings_l, dim=0)src_mask_cur = torch.cat(src_mask_l, dim=0)y_tm1 = torch.cat(last_tokens, dim=0)r2l_memory_cur = torch.cat(r2l_memory_l, dim=0)"shape (sum(4 bt * cur_beam_sz_i), 1 dec_sent_len, 128 d_model)"if self.feature_mode == 'one':out = self.l2r_decode(Variable(y_tm1).to(self.device), model_encodings_cur, src_mask_cur,Variable(subsequent_mask(y_tm1.size(-1)).type_as(src.data)).to(self.device),r2l_memory_cur, r2l_trg_mask=None)elif self.feature_mode == 'two' or 'three' or 'four':out = self.l2r_decode(Variable(y_tm1).to(self.device), model_encodings_cur, src_mask_cur,Variable(subsequent_mask(y_tm1.size(-1)).type_as(src[0].data)).to(self.device),r2l_memory_cur, r2l_trg_mask=None)"shape (sum(4 bt * cur_beam_sz_i), 1 dec_sent_len, 50002 vocab_sz)"log_prob = self.generator(out[:, -1, :]).unsqueeze(1)"shape (sum(4 bt * cur_beam_sz_i), 1 dec_sent_len, 50002 vocab_sz)"_, decoded_len, vocab_sz = log_prob.shape# log_prob = log_prob.reshape(batch_size, cur_beam_size, decoded_len, vocab_sz)"shape List(4 bt)[(cur_beam_sz_i, dec_sent_len, 50002 vocab_sz)]""log_prob[i] is (cur_beam_sz_i, dec_sent_len, 50002 vocab_sz)"log_prob = torch.split(log_prob, cur_beam_sizes, dim=0)# 2.2 Now we process each example in the batch. Note that the example may have already finished processing before# other examples (no more hypotheses to try), in which case we continuenew_hypotheses, new_hyp_scores = [], []for i in range(batch_size):if hypotheses[i] is None or len(completed_hypotheses[i]) >= beam_size:new_hypotheses += [None]new_hyp_scores += [None]continue# 2.2.1 We compute the cumulative scores for each live hypotheses for the example# hyp_scores is the old scores for the previous stage, and `log_prob` are the new probs for# this stage. Since they are log probs, we sum them instaed of multiplying them.# The .view(-1) forces all the hypotheses into one dimension. The shape of this dimension is# cur_beam_sz * vocab_sz (ex: 5 * 50002). So after getting the topk from it, we can recover the# generating sentence and the next word using: ix // vocab_sz, ix % vocab_sz.cur_beam_sz_i, dec_sent_len, vocab_sz = log_prob[i].shape"shape (vocab_sz,)"cumulative_hyp_scores_i = (hyp_scores[i].unsqueeze(-1).unsqueeze(-1).expand((cur_beam_sz_i, 1, vocab_sz)) + log_prob[i]).view(-1)# 2.2.2 We get the topk values in cumulative_hyp_scores_i and compute the current (generating) sentence# and the next word using: ix // vocab_sz, ix % vocab_sz."shape (cur_beam_sz,)"live_hyp_num_i = beam_size - len(completed_hypotheses[i])"shape (cur_beam_sz,). Vals are between 0 and 50002 vocab_sz"top_cand_hyp_scores, top_cand_hyp_pos = torch.topk(cumulative_hyp_scores_i, k=live_hyp_num_i)"shape (cur_beam_sz,). prev_hyp_ids vals are 0 <= val < cur_beam_sz. hyp_word_ids vals are 0 <= val < vocab_len"prev_hyp_ids, hyp_word_ids = top_cand_hyp_pos // self.vocab.n_vocabs, \top_cand_hyp_pos % self.vocab.n_vocabs# 2.2.3 For each of the topk words, we append the new word to the current (generating) sentence# We add this to new_hypotheses_i and add its corresponding total score to new_hyp_scores_inew_hypotheses_i, new_hyp_scores_i = [], [] # Removed live_hyp_ids_i, which is used in the LSTM decoder to track live hypothesis idsfor prev_hyp_id, hyp_word_id, cand_new_hyp_score in zip(prev_hyp_ids, hyp_word_ids,top_cand_hyp_scores):prev_hyp_id, hyp_word_id, cand_new_hyp_score = \prev_hyp_id.item(), hyp_word_id.item(), cand_new_hyp_score.item()new_hyp_sent = torch.cat((hypotheses[i][prev_hyp_id], torch.tensor([hyp_word_id], device=self.device)))if hyp_word_id == end_symbol:completed_hypotheses[i].append(Hypothesis(value=[self.vocab.idx2word[a.item()] for a in new_hyp_sent[1:-1]],score=cand_new_hyp_score))else:new_hypotheses_i.append(new_hyp_sent.unsqueeze(-1))new_hyp_scores_i.append(cand_new_hyp_score)# 2.2.4 We may find that the hypotheses_i for some example in the batch# is empty - we have fully processed that example. We use None as a sentinel in this case.# Above, the loops gracefully handle None examples.if len(new_hypotheses_i) > 0:hypotheses_i = torch.cat(new_hypotheses_i, dim=-1).transpose(0, -1).to(self.device)hyp_scores_i = torch.tensor(new_hyp_scores_i, dtype=torch.float, device=self.device)else:hypotheses_i, hyp_scores_i = None, Nonenew_hypotheses += [hypotheses_i]new_hyp_scores += [hyp_scores_i]# print(new_hypotheses, new_hyp_scores)hypotheses, hyp_scores = new_hypotheses, new_hyp_scores# 2.3 Finally, we do some postprocessing to get our final generated candidate sentences.# Sometimes, we may get to max_len of a sentence and still not generate the </s> end token.# In this case, the partial sentence we have generated will not be added to the completed_hypotheses# automatically, and we have to manually add it in. We add in as many as necessary so that there are# `beam_size` completed hypotheses for each example.# Finally, we sort each completed hypothesis by score.for i in range(batch_size):hyps_to_add = beam_size - len(completed_hypotheses[i])if hyps_to_add > 0:scores, ix = torch.topk(hyp_scores[i], k=hyps_to_add)for score, id in zip(scores, ix):completed_hypotheses[i].append(Hypothesis(value=[self.vocab.idx2word[a.item()] for a in hypotheses[i][id][1:]],score=score))completed_hypotheses[i].sort(key=lambda hyp: hyp.score, reverse=True)# print('completed_hypotheses', completed_hypotheses)return r2l_completed_hypotheses, completed_hypotheses
相关文章:

架构以及架构中的组件
架构以及架构中的组件 Transform Transform 以下的代码包含: 标准化的示例残差化的示例 # huggingface # transformers# https://www.bilibili.com/video/BV1At4y1W75x?spm_id_from333.999.0.0import copy import math from collections import namedtupleimport …...
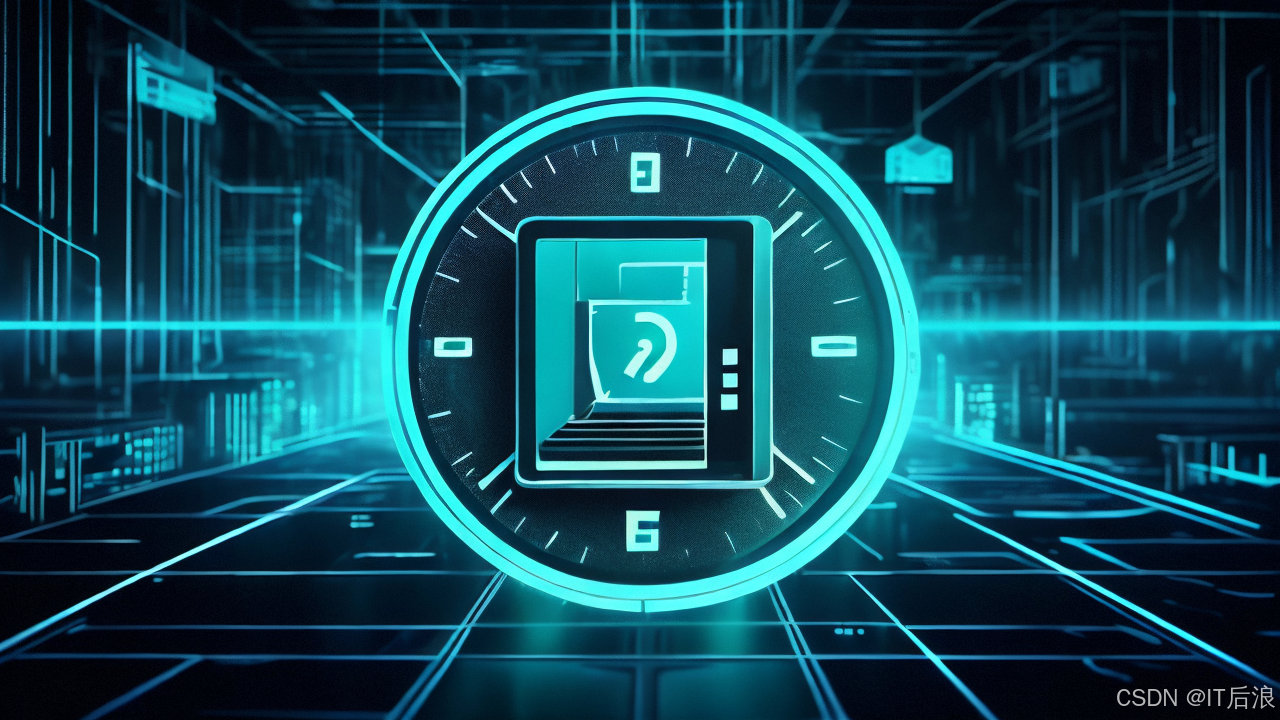
Docker启动PostgreSql并设置时间与主机同步
在 Docker 中启动 PostgreSql 时,需要配置容器的时间与主机同步。可以通过在 Dockerfile 或者 Docker Compose 文件中设置容器的时区,或者使用宿主机的时间来同步容器的时间。这样可以确保容器中的 PostgreSql 与主机的时间保持一致,避免在使…...
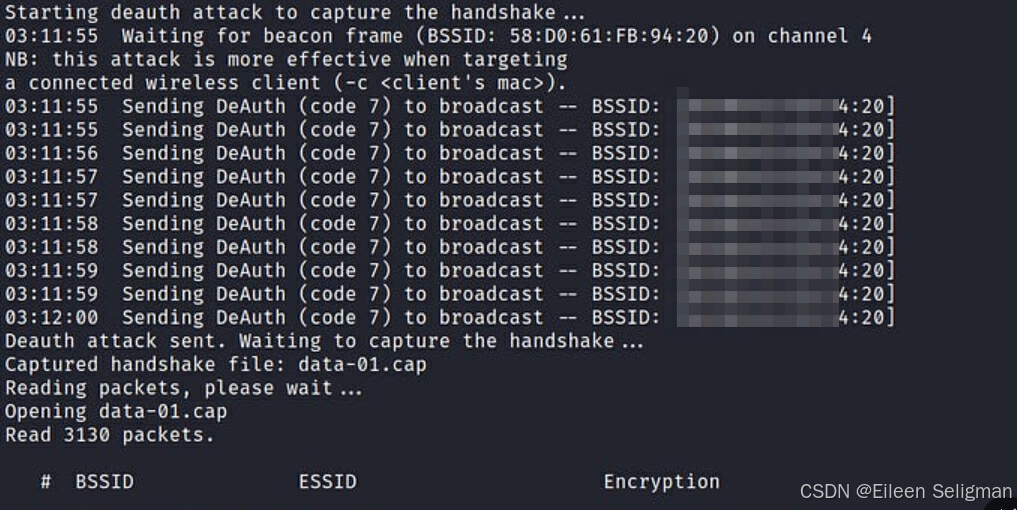
提升无线网络安全:用Python脚本发现并修复WiFi安全问题
文章目录 概要环境准备技术细节3.1 实现原理3.2 创建python文件3.3 插入内容3.4 运行python脚本 加固建议4.1 选择强密码4.2 定期更换密码4.3 启用网络加密4.4 关闭WPS4.5 隐藏SSID4.6 限制连接设备 小结 概要 在本文中,我们将介绍并展示如何使用Python脚本来测试本…...
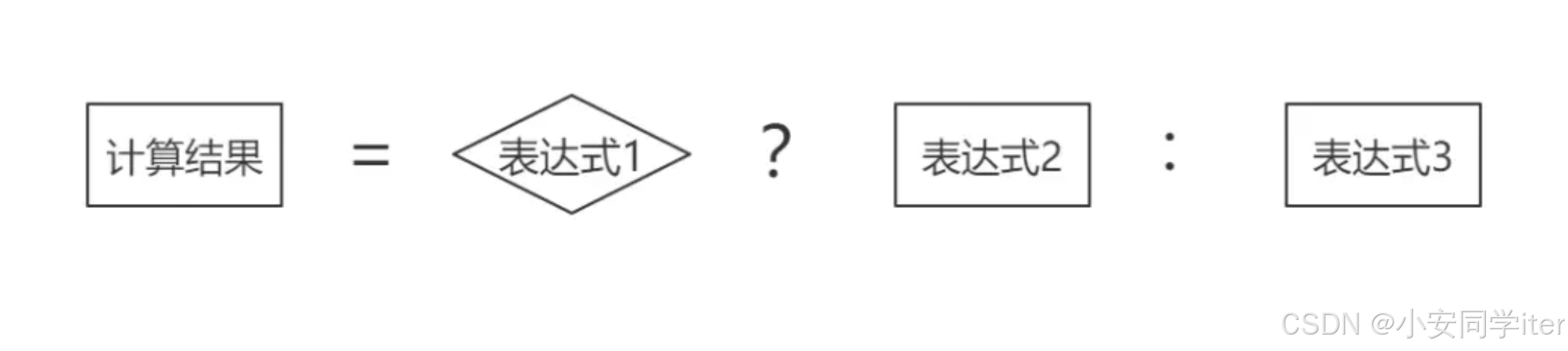
#三元运算符(python/java/c)
引入:什么是三元运算符呢?无疑其操作元有三个,一个是条件表达式,剩余两个为值,条件表达式为真时运算取第一个值,为假时取第二个值。 一 Python true_expression if condition else false_expressi…...

探索Python自然语言处理的新篇章:jionlp库介绍
探索Python自然语言处理的新篇章:jionlp库介绍 1. 背景:为什么选择jionlp? 在Python的生态中,自然语言处理(NLP)是一个活跃且不断发展的领域。jionlp是一个专注于中文自然语言处理的库,它提供了…...
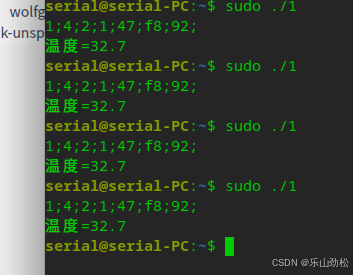
Deepin系统,中盛科技温湿度模块读温度纯c程序(备份)
#include <stdio.h> #include <fcntl.h> #include <unistd.h> #include <termios.h>int main() {int fd;struct termios options;// 打开串口设备fd open("/dev/ttyMP0", O_RDWR | O_NOCTTY|O_NDELAY); //O_NDELAY:打开设备不阻塞//O_NOCTT…...

文件包含漏洞: 函数,实例[pikachu_file_inclusion_local]
文件包含 文件包含是一种较为常见技术,允许程序员在不同的脚本或程序中重用代码或调用文件 主要作用和用途: 代码重用:通过将通用函数或代码段放入单独的文件中,可以在多个脚本中包含这些文件,避免重复编写相同代码。…...

学习计划2024下半年
基础: 学习《算法第4版》,学习leetcode上的面试经典150题,使用C完成;再看一般《深入理解计算机系统》语言: 学习go语言,并且用它写一个小软件(还没想好什么),写一个pingtool程序编程思想: 阅读经…...

RabbitMQ的学习和模拟实现|sqlite轻量级数据库的介绍和简单使用
SQLite3 项目仓库:https://github.com/ffengc/HareMQ SQLite3 什么是SQLite为什么需要用SQLite官方文档封装Helper进行一些实验 什么是SQLite SQLite是一个进程内的轻量级数据库,它实现了自给自足的、无服务器的、零配置的、事务性的 SQL数据库引擎…...
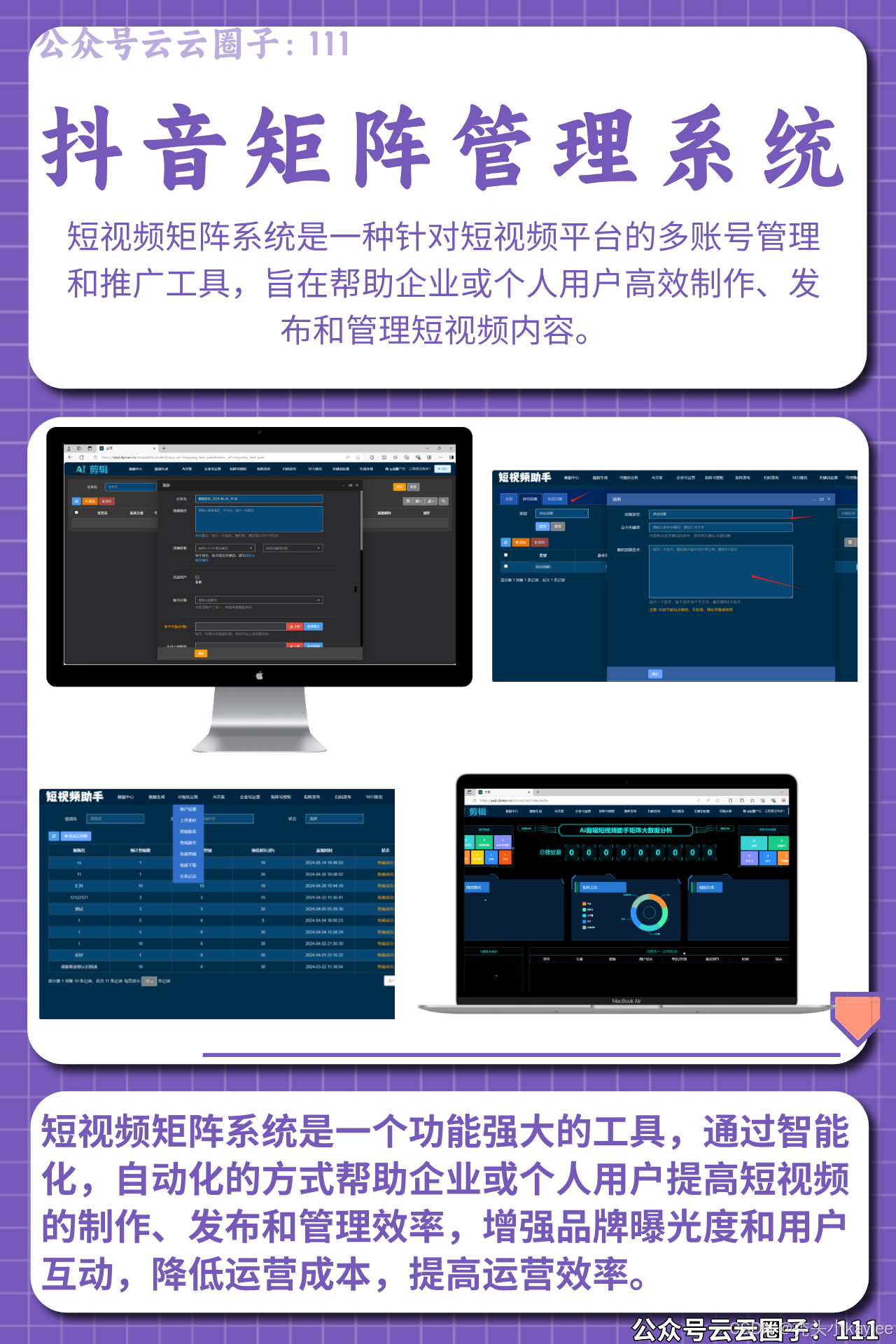
AI批量剪辑,批量发布大模型矩阵系统搭建开发
目录 前言 一、AI矩阵系统功能 二、AI批量剪辑可以解决什么问题? 总结: 前言 基于ai生成或剪辑视频的原理,利用ai将原视频进行混剪,生成新的视频素材。ai会将剪辑好的视频加上标题,批量发布到各个自媒体账号上。这…...
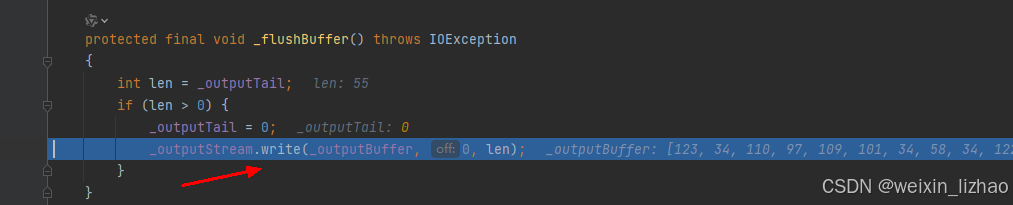
SpringMVC源码深度解析(中)
接上一遍博客《SpringMVC源码深度解析(上)》继续聊。最后聊到了SpringMVC的九大组建的初始化,以 HandlerMapping为例,SpringMVC提供了三个实现了,分别是:BeanNameUrlHandlerMapping、RequestMappingHandlerMapping、RouterFunctio…...

Mojo模型动态批处理:智能预测的终极武器
标题:Mojo模型动态批处理:智能预测的终极武器 在机器学习领域,模型的灵活性和可扩展性是至关重要的。Mojo模型(Model-as-a-Service)提供了一种将机器学习模型部署为服务的方式,允许开发者和数据科学家轻松…...
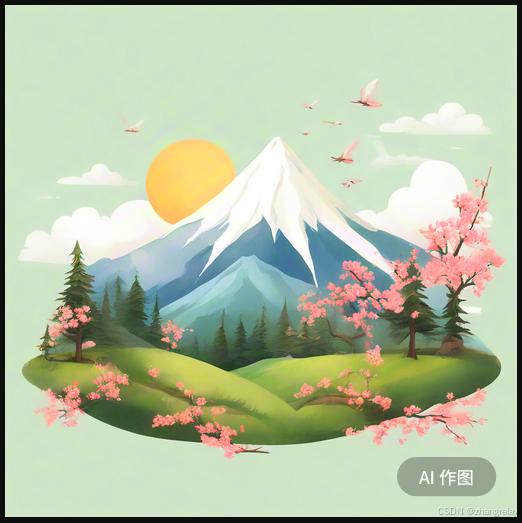
人、智能、机器人……
在遥远的未来之城,智能时代如同晨曦般照亮了每一个角落,万物互联,机器智能与人类智慧交织成一幅前所未有的图景。这座城市,既是科技的盛宴,也是人性与情感深刻反思的舞台。 寓言:《智光与心影》 在智能之…...
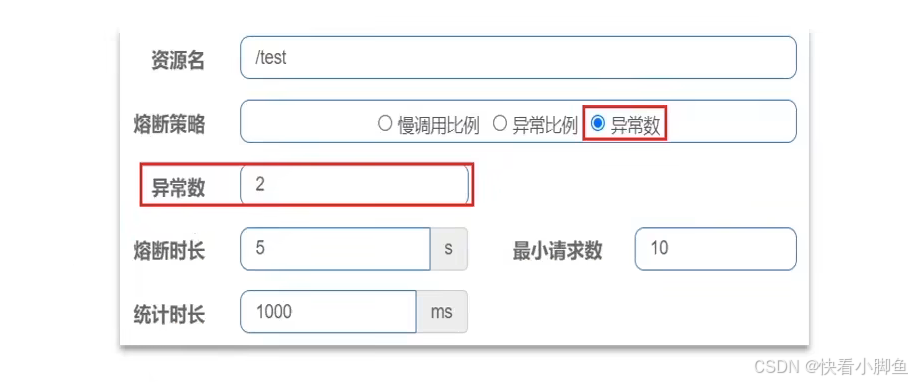
SpringCloud------Sentinel(微服务保护)
目录 雪崩问题 处理方式!!!技术选型 Sentinel 启动命令使用步骤引入依赖配置控制台地址 访问微服务触发监控 限流规则------故障预防流控模式流控效果 FeignClient整合Sentinel线程隔离-------故障处理线程池隔离和信号量隔离编辑 两种方式优缺点设置方式 熔断降级-----…...

【无标题】Elasticsearch for windows
一、windows安装Elasticsearch 1、Elasticsearch:用于存储数据、计算和搜索; 2、Logstash/Beats:用于数据搜集 3、Kibana:用于数据可视化 以上三个被称为ELK,常用语日志搜集、系统监控和状态分析 Elasticsearch安…...
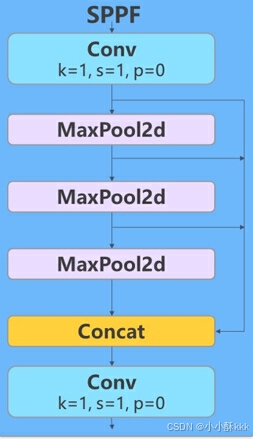
Yolo-World网络模型结构及原理分析(一)——YOLO检测器
文章目录 概要一、整体架构分析二、详细结构分析YOLO检测器1. Backbone2. Head3.各模块的过程和作用Conv卷积模块C2F模块BottleNeck模块SPPF模块Upsampling模块Concat模块 概要 尽管YOLO(You Only Look Once)系列的对象检测器在效率和实用性方面表现出色…...
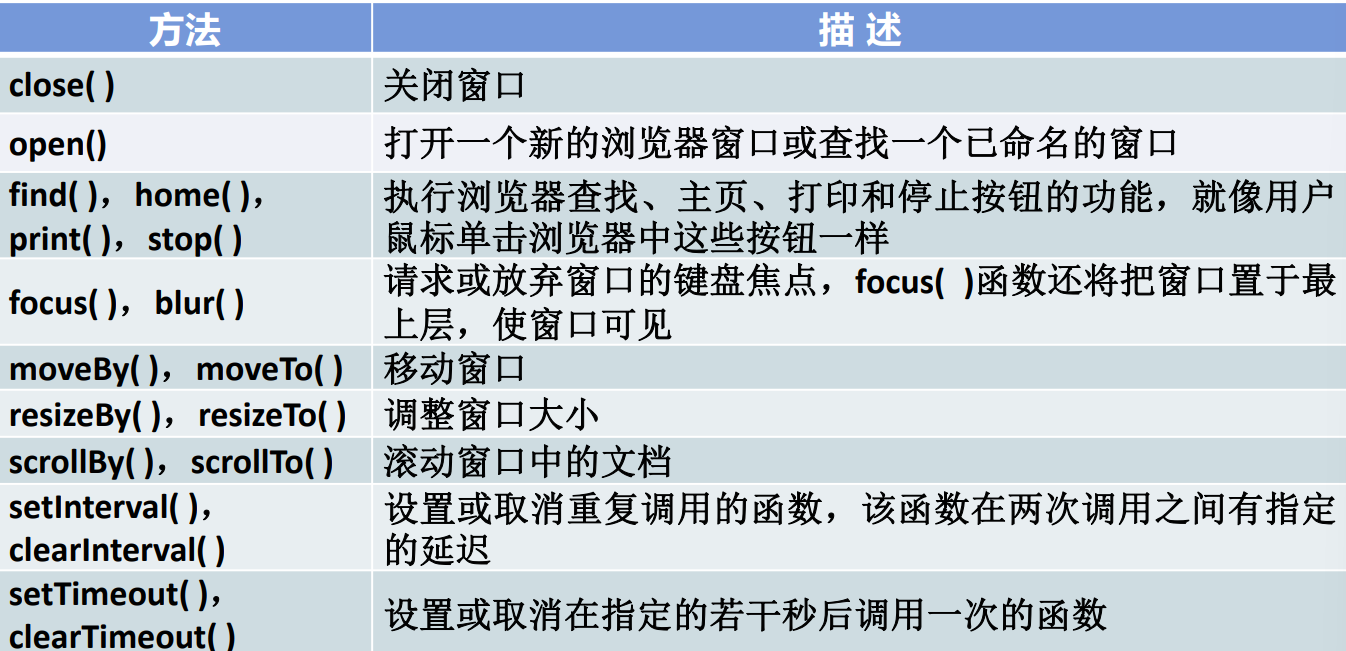
WEB前端06-BOM对象
BOM浏览器对象模型 浏览器对象模型:将浏览器的各个组成部分封装成对象。是用于描述浏览器中对象与对象之间层次关系的模型,提供了独立于页面内容、并能够与浏览器窗口进行交互的对象结构。 组成部分 Window:浏览器窗口对象 Navigator&…...

Android11 framework 禁止三方应用开机自启动
Android11应用自启动限制 大纲 Android11应用自启动限制分析验证猜想:Android11 AOSP是否自带禁止三方应用监听BOOT_COMPLETED方案禁止执行非系统应用监听到BOOT_COMPLETED后的代码逻辑在执行启动时判断其启动的广播接收器一棍子打死方案(慎用&#…...
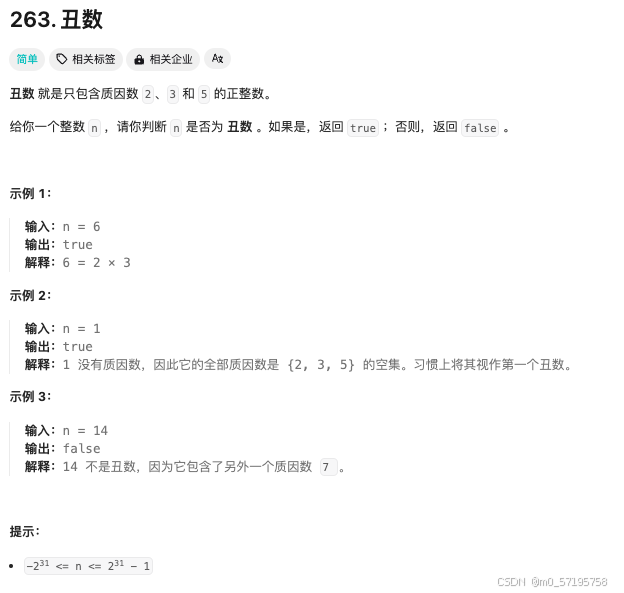
Java | Leetcode Java题解之第263题丑数
题目: 题解: class Solution {public boolean isUgly(int n) {if (n < 0) {return false;}int[] factors {2, 3, 5};for (int factor : factors) {while (n % factor 0) {n / factor;}}return n 1;} }...

将AWS RDS MySQL实例从存储未加密改为加密的方案
问题描述: 因为AWS RDS官方文档【1】中已经明确说明,MySQL RDS的存储为EBS卷,用KMS进行RDS加密有如下限制: 您只能在创建RDS的时候,选择加密。对于已经创建的RDS实例,您无法将为加密的实例,直…...

nginx的配置:TLSv1 TLSv1.1 被暴露不安全
要在 Nginx 配置中禁用不安全的 SSL 协议(如 TLSv1 和 TLSv1.1),并仅启用更安全的协议(如 TLSv1.2 和 TLSv1.3),您可以更新您的 Nginx 配置文件。下面是一个示例配置: # 位于 Nginx 配置文件 (…...

揭开黑箱:目标检测中可解释性的重要性与实现
揭开黑箱:目标检测中可解释性的重要性与实现 在深度学习的目标检测任务中,模型的准确性虽然重要,但模型的决策过程是否透明也同样关键。可解释性(Explainability)是指模型能够为其预测结果提供清晰、可理解的解释。本…...
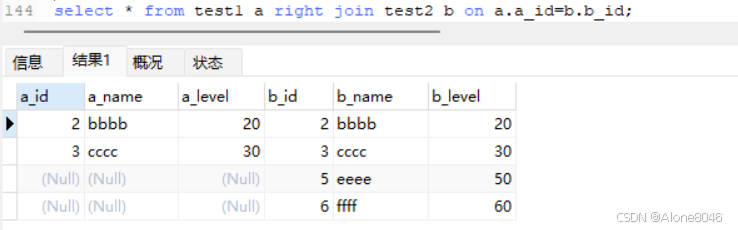
Mysql高价语句
一.高级语法的查询语句 1.排序语法(默认的排序方式就是升序)。 升序ASC:select * from test01 order by name; 降序DESC:select * from test01 order by name desc; 多个列排序:以多个列作为排序,只有第一…...

ArcGIS Pro SDK (九)几何 6 包络
ArcGIS Pro SDK (九)几何 6 包络 文章目录 ArcGIS Pro SDK (九)几何 6 包络1 构造包络2 构造包络 - 从 JSON 字符串3 合并两个包络4 与两个包络相交5 展开包络6 更新包络的坐标 环境:Visual Studio 2022 .NET6 ArcGI…...
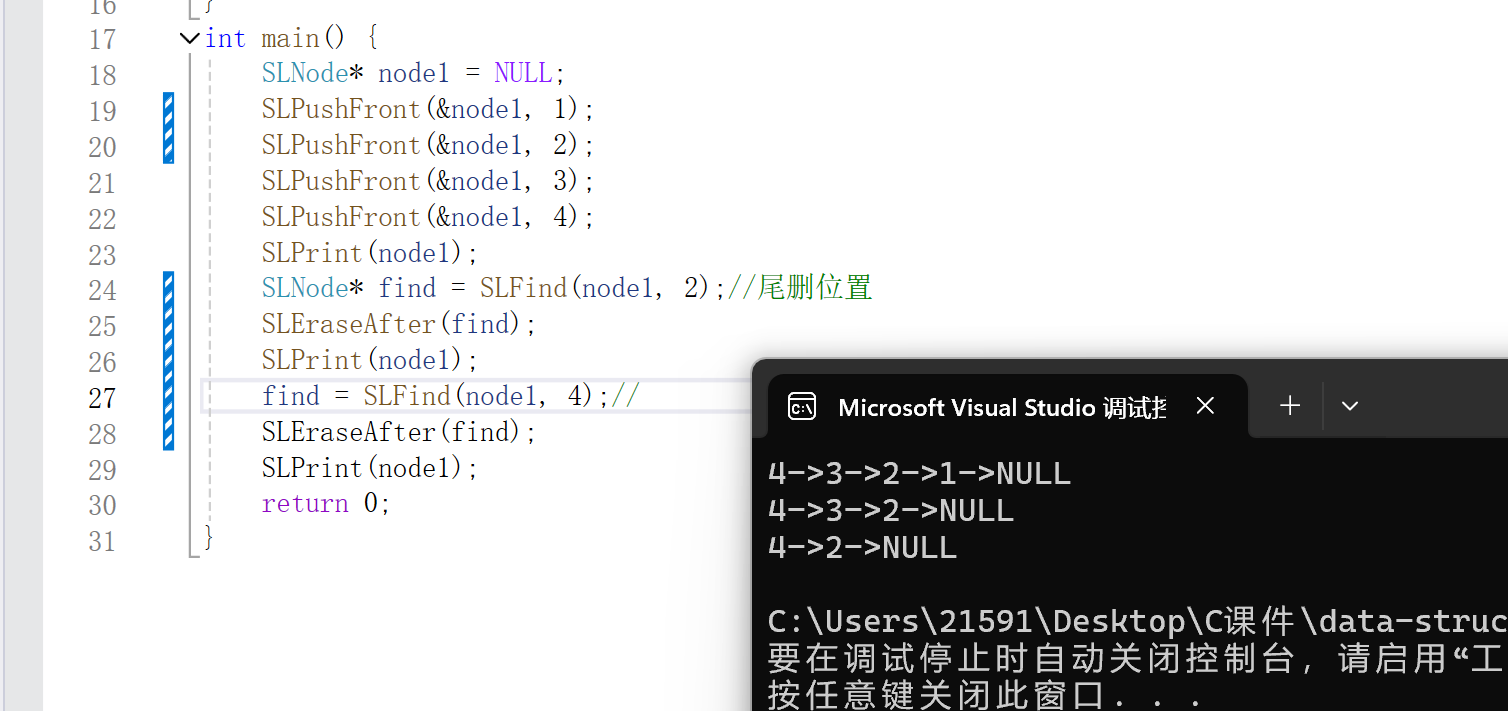
单链表<数据结构 C版>
目录 概念 链表的单个结点 链表的打印操作 新结点的申请 尾部插入 头部插入 尾部删除 头部删除 查找 在指定位置之前插入数据 在任意位置之后插入数据 测试运行一下: 删除pos结点 删除pos之后结点 销毁链表 概念 单链表是一种在物理存储结构上非连续、非顺序…...
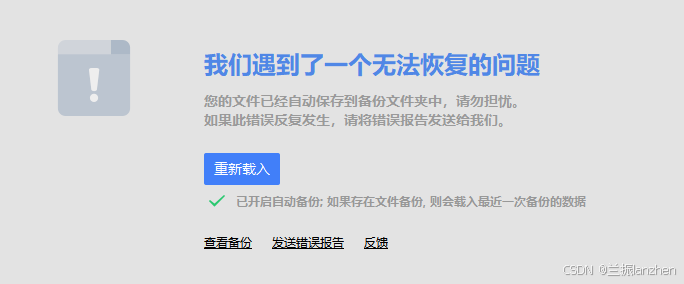
监控电脑进程,避免程序在打开前就已经在运行
文章目录 一、文章的目的(适用于windows)二、处理方式三、进程查看的内容在窗口端的演示四、附上代码例子四、通过os.kill的方式,再回到原来的表格时,会出现如下错误提示: 一、文章的目的(适用于windows&am…...

【MySQL进阶篇】存储对象:视图、存储过程及触发器
一、视图 1、介绍 视图(view)是一种虚拟存在的表。视图中的数据并不在数据库中实际存在,行和列数据来定义视图的查询中使用的表(基表),并且是在使用视图时动态生成的。 通俗的讲,视图只保存了…...
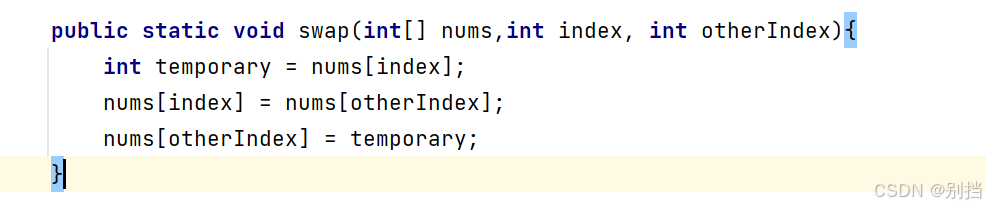
算法day05 master公式估算递归时间复杂度 归并排序 小和问题 堆排序
2.认识O(NlogN)的排序_哔哩哔哩_bilibili master公式 有这样一个数组:【0,4,2,3,3,1,2】;假设实现了这样一个sort()排序方法, 将数组二分成左右两等分,使用so…...
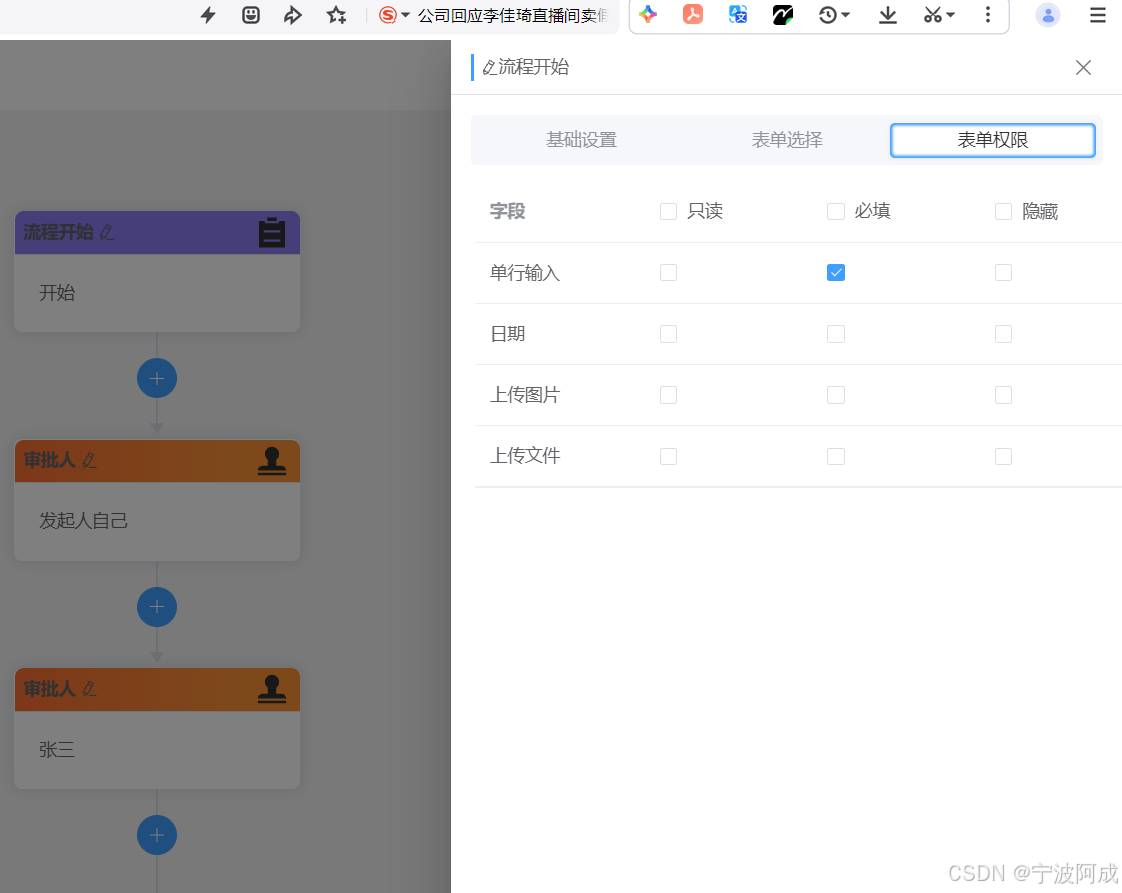
基于jeecgboot-vue3的Flowable流程仿钉钉流程设计器-支持VForm3表单的选择与支持
因为这个项目license问题无法开源,更多技术支持与服务请加入我的知识星球。 1、初始化的时候加载表单 /** 查询表单列表 */ const getFormList () > {listForm().then(res > formOptions.value res.result.records) } 2、开始节点的修改,增加表…...
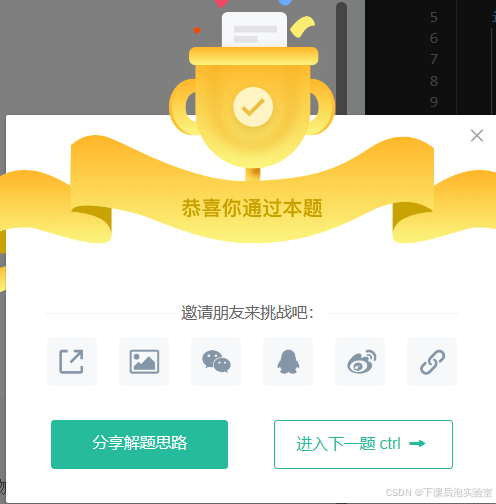
【刷题汇总 -- 压缩字符串(一)、chika和蜜柑、 01背包】
C日常刷题积累 今日刷题汇总 - day0181、压缩字符串(一)1.1、题目1.2、思路1.3、程序实现 2、chika和蜜柑2.1、题目2.2、思路2.3、程序实现 3、 01背包3.1、题目3.2、思路3.3、程序实现 -- dp 4、题目链接 今日刷题汇总 - day018 1、压缩字符串(一) 1.1、题目 1.2、思路 读完…...
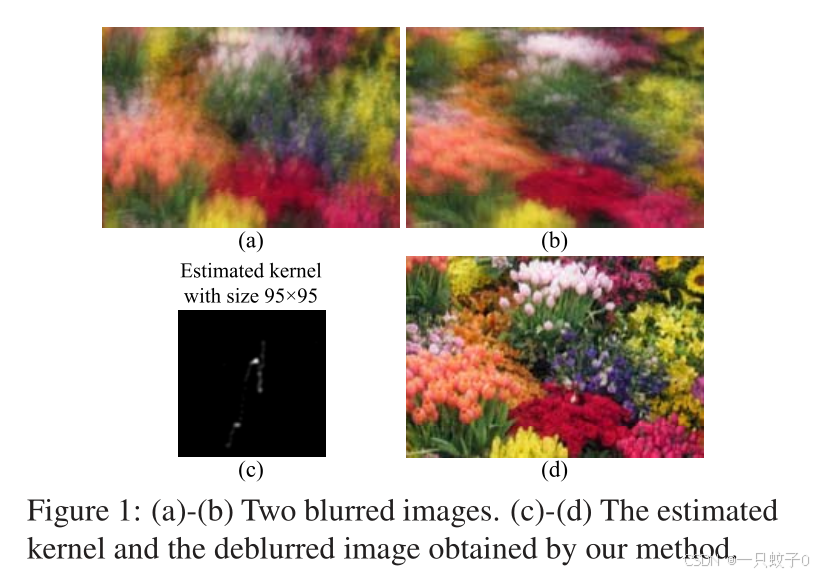
《Exploring Aligned Complementary Image Pair for Blind Motion Deblurring》
这篇论文的标题《Exploring Aligned Complementary Image Pair for Blind Motion Deblurring》可以翻译为《探索对齐的互补图像对用于盲运动去模糊》。从标题可以推断,论文的焦点在于开发一种算法或技术,利用成对的图像来解决运动模糊问题,特别是在不知道模糊核(即造成模糊…...
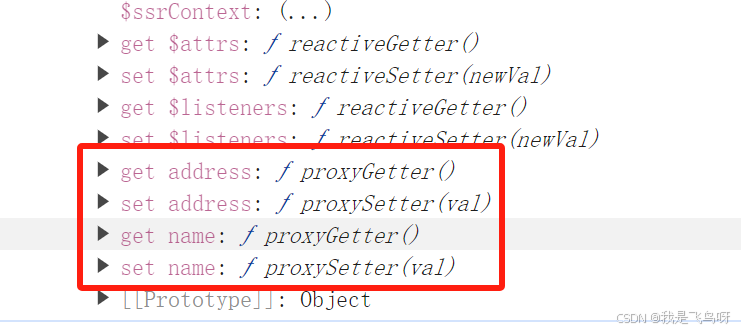
vue2学习笔记9 - 通过观察vue实例中的data,理解Vue中的数据代理
接着上一节,学一学vue中的数据代理。学vue这几天,最大的感受就是,名词众多,听得发懵。。不过,深入理解之后,其实说得都是一回事。 在Vue中,数据代理是指在实例化Vue对象时,将data对…...
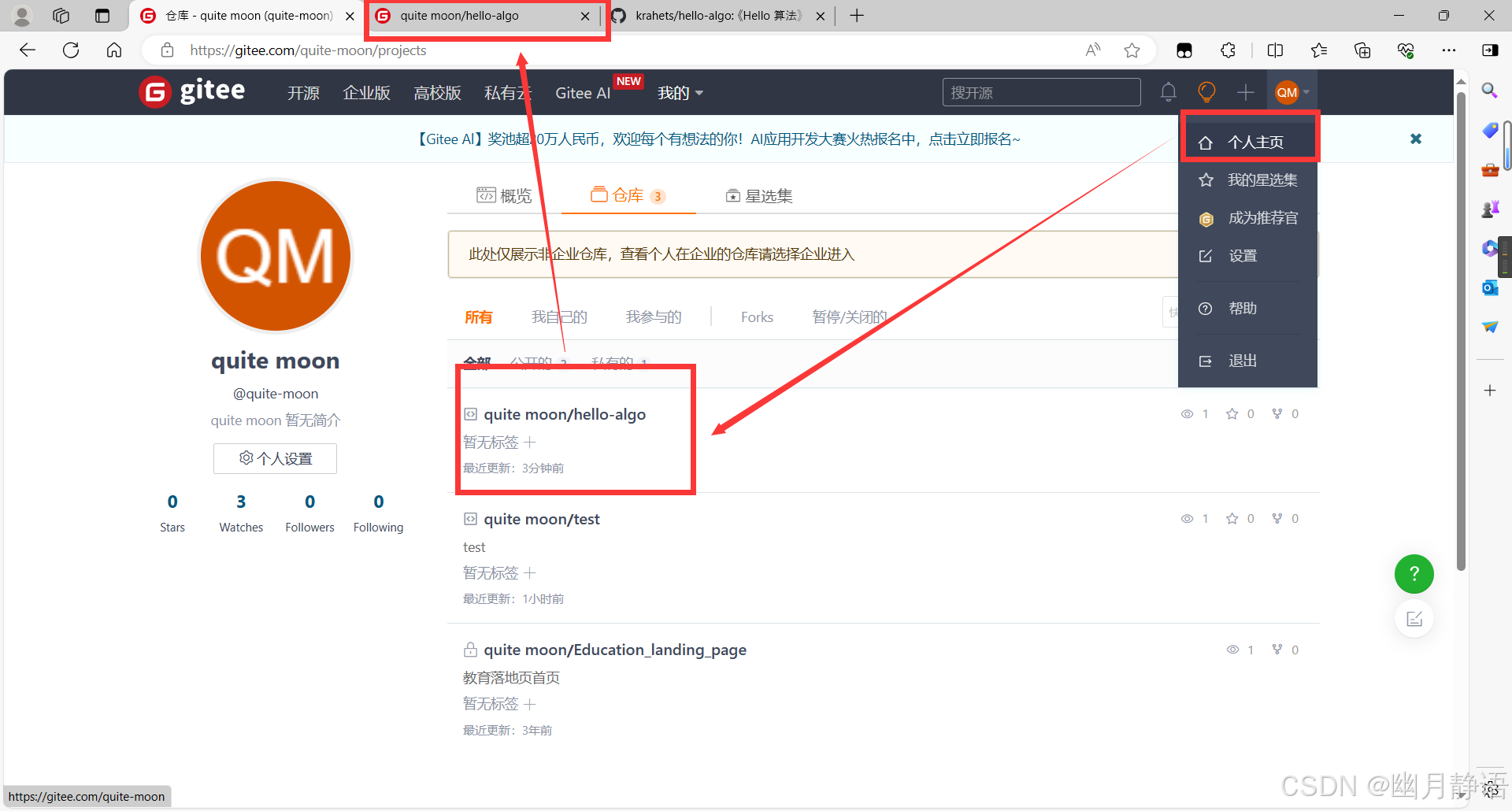
04 Git与远程仓库
第4章:Git与远程仓库 一、Gitee介绍及创建仓库 一)获取远程仓库 使用在线的代码托管平台,如Gitee(码云)、GitHub等 自行搭建Git代码托管平台,如GitLab 二)Gitee创建仓库 gitee官…...
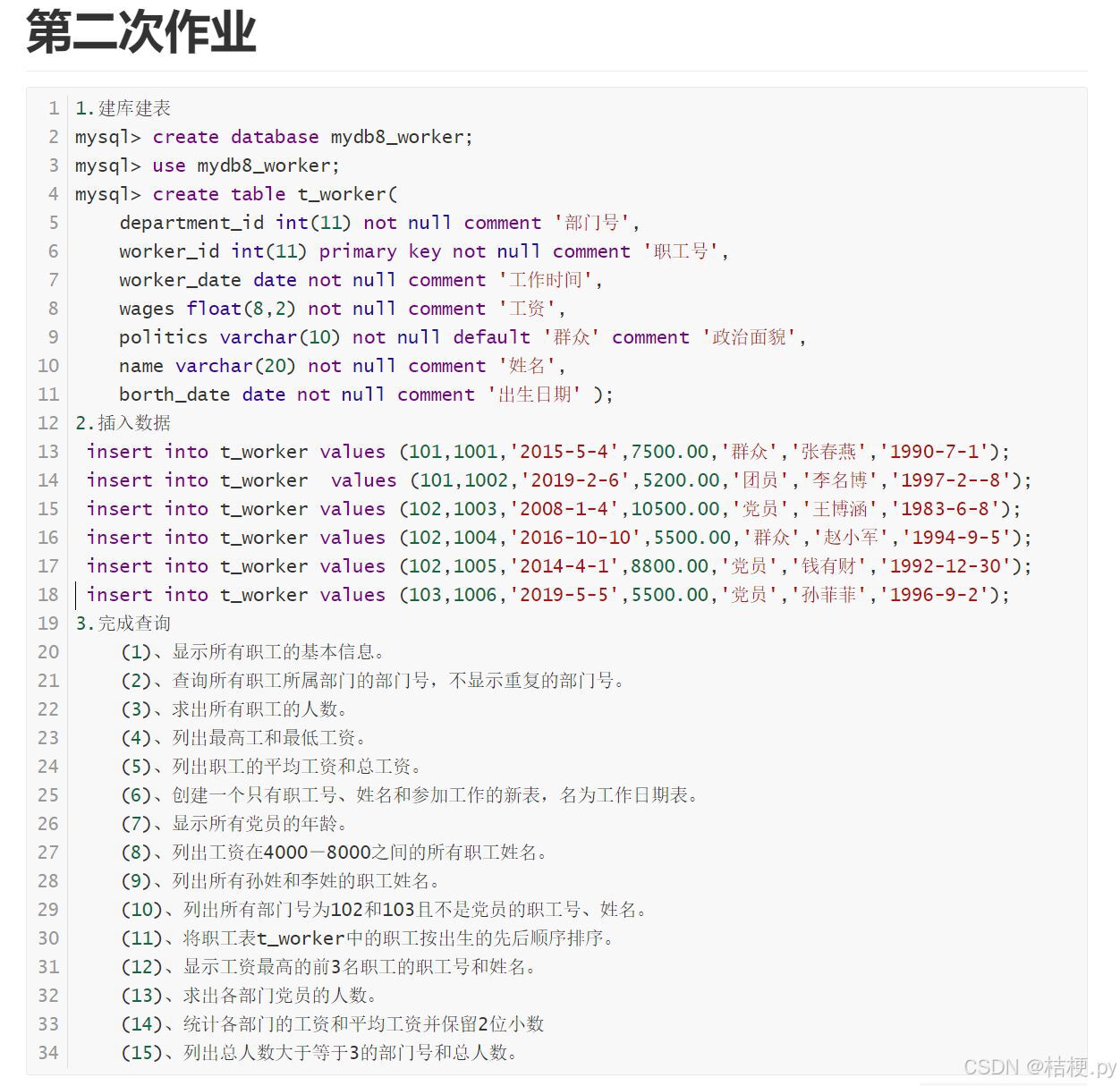
数据库之表的查询
一.新建表: mysql> create table t_worker(-> department_id int(11) not null comment部门号,-> worker_id int(11) primary key not null comment职工号,-> worker_date date not null comment工作时间,-> wages float(8,2) not null comment工资,…...
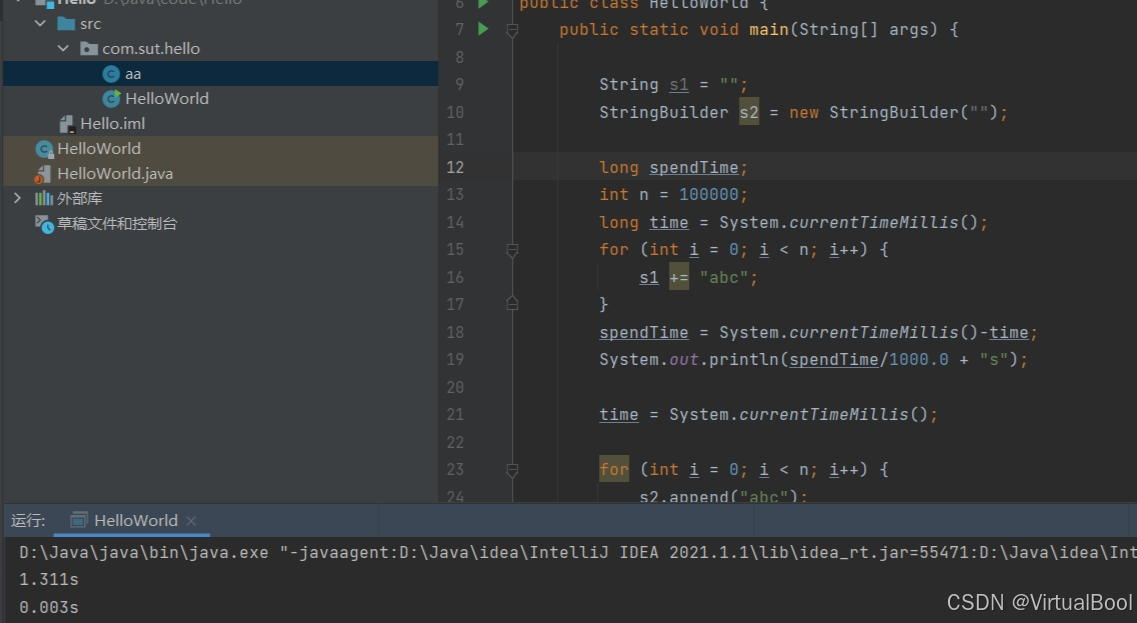
String 和StringBuilder字符串操作快慢的举例比较
System.currentTimeMillis(); //当前时间与1970年1月1日午夜UTC之间的毫秒差。public class HelloWorld {public static void main(String[] args) {String s1 "";StringBuilder s2 new StringBuilder("");long time System.currentTimeMillis();long s…...
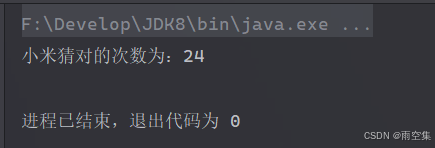
Java代码基础算法练习-竞猜卡片值-2024.07.22
任务描述: 小米和小王玩竞猜游戏:准备7张卡片包含数字2、3、4、5、6、7、8,从中抽出2张(有 顺序之分,抽2、3跟抽3、2是两种情况),猜2张卡片的和,如果是奇数,则猜对。小米…...
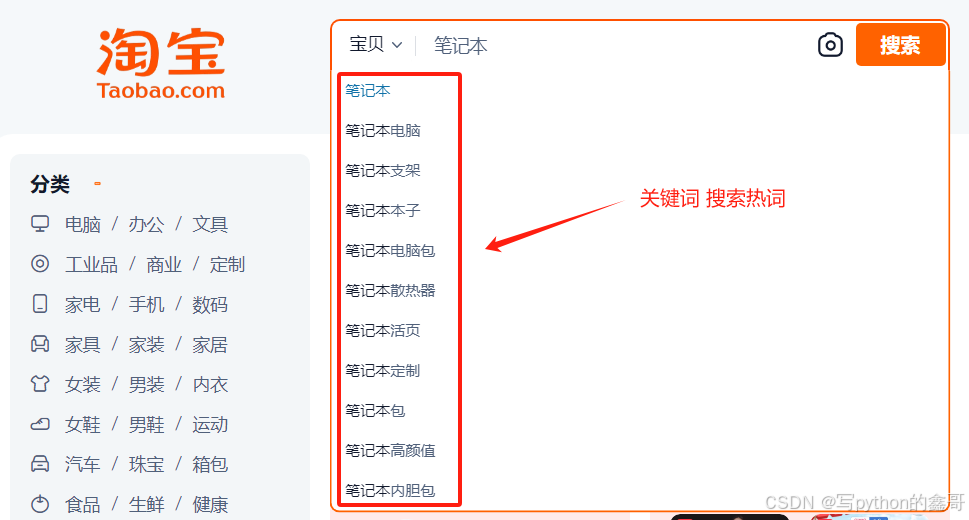
Python爬虫-淘宝搜索热词数据
前言 本文是该专栏的第70篇,后面会持续分享python爬虫干货知识,记得关注。 在本专栏之前,笔者有详细针对“亚马逊Amazon搜索热词”数据采集的详细介绍,对此感兴趣的同学,可以往前翻阅《Python爬虫-某跨境电商(AM)搜索热词》进行查看。 而在本文,笔者将以淘宝为例,获取…...
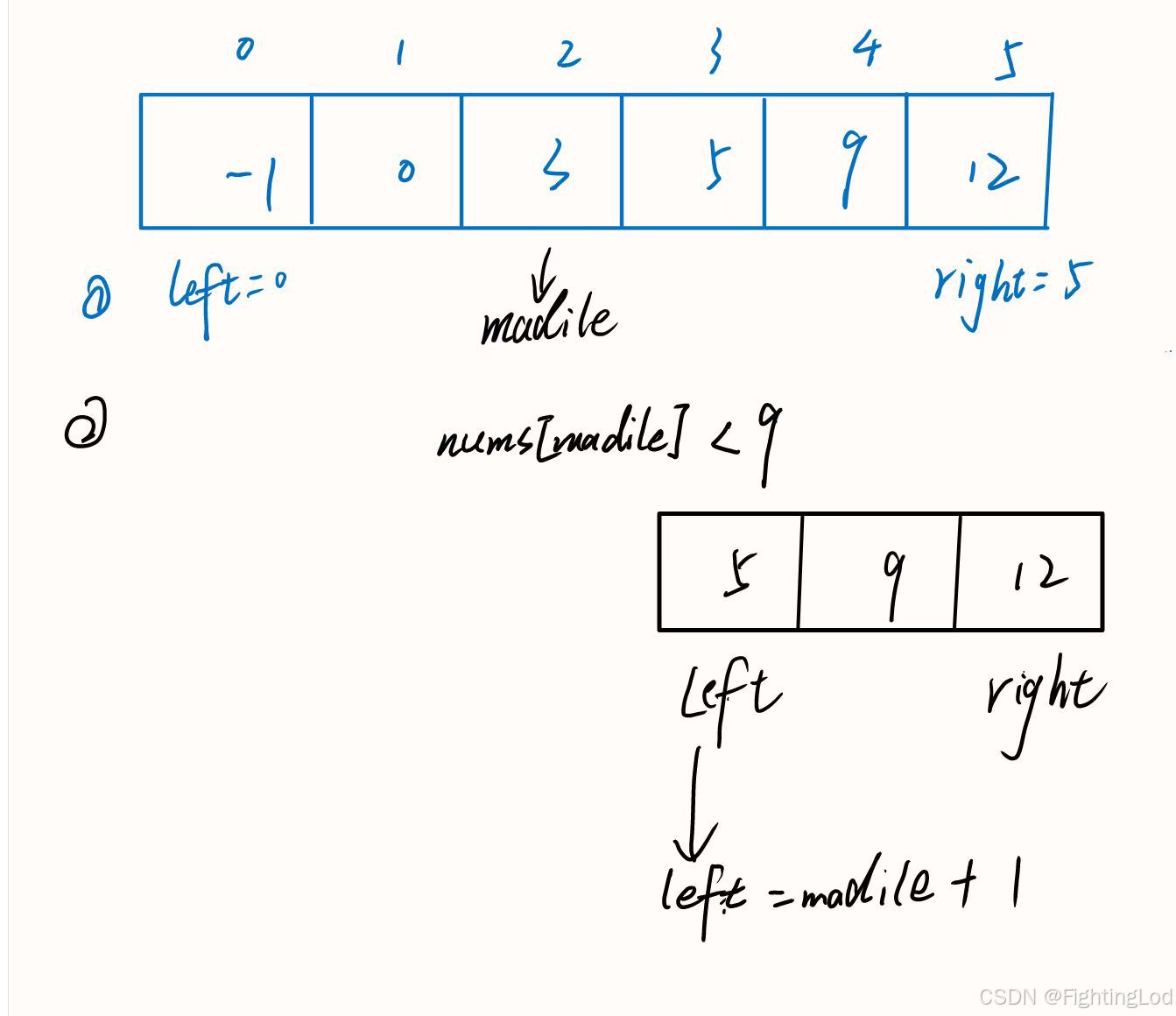
Leetcode二分搜索法浅析
文章目录 1.二分搜索法1.1什么是二分搜索法?1.2解法思路1.3扩展 1.二分搜索法 题目原文: 给定一个 n 个元素有序的(升序)整型数组 nums 和一个目标值 target ,写一个函数搜索 nums 中的 target,如果目标值…...
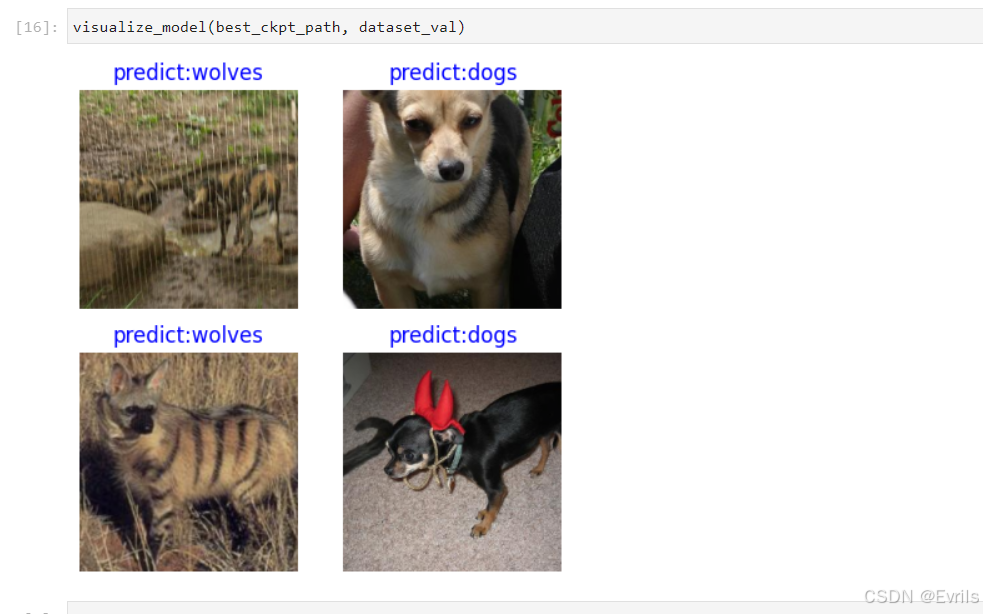
昇思25天学习打卡营第24天|ResNet50迁移学习
课程打卡凭证 迁移学习 迁移学习是机器学习中一个重要的技术,通过在一个任务上训练的模型来改善在另一个相关任务上的表现。在深度学习中,迁移学习通常涉及在一个大型数据集(如ImageNet)上预训练的模型上进行微调,以便…...

Shell 构建flutter + Navtive 生成IPA
具体实现: #1. 在工程的根目录下,建立文件夹build_iOS文件,在此文件下建立build_iOS.sh的文件,把以下内容copy进sh文件;build_iOS.sh 就是第5步之后整个的脚本内容。 #2. 进入build_iOS.sh 文件的目录; #3. 在build_iOS 文件夹配置打包的DEVELOPExportOptionsPlist…...
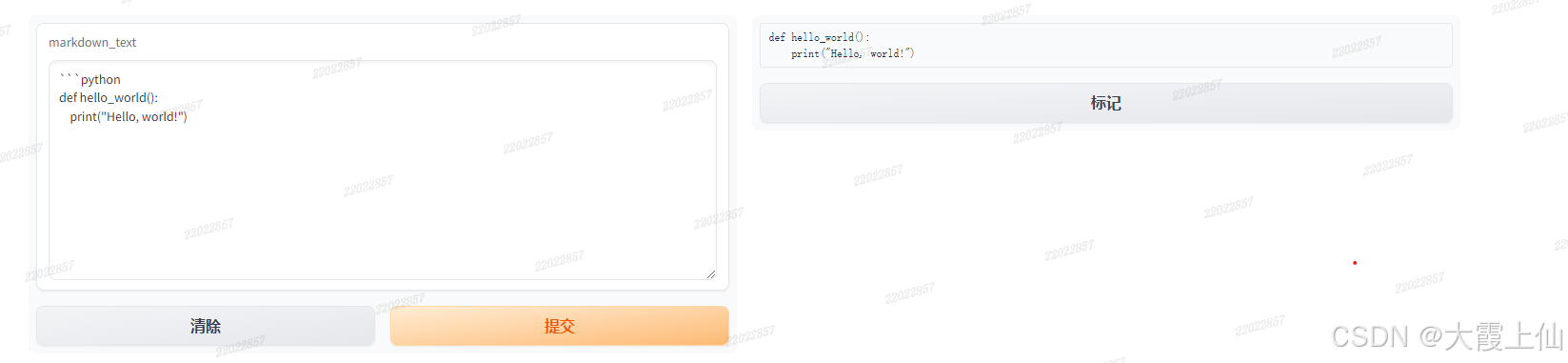
python gradio 的输出展示组件
HTML:展示HTML内容,适用于富文本或网页布局。JSON:以JSON格式展示数据,便于查看结构化数据。KeyValues:以键值对形式展示数据。Label:展示文本标签,适用于简单的文本输出。Markdown:…...
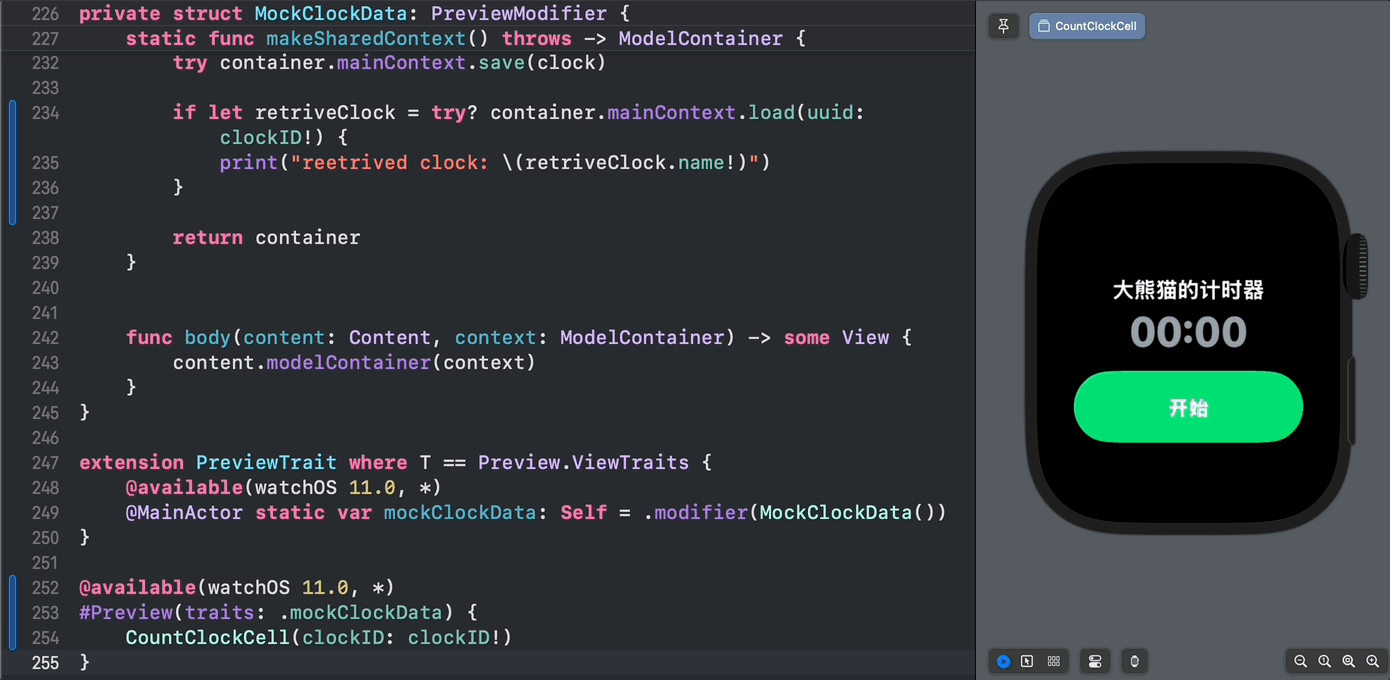
SwiftUI 6.0(Xcode 16)新 PreviewModifier 协议让预览调试如虎添翼
概览 用 SwiftUI 框架开发过应用的小伙伴们都知道,SwiftUI 中的视图由各种属性和绑定“扑朔迷离”的缠绕在一起,自成体系。 想要在 Xcode 预览中泰然处之的调试 SwiftUI 视图有时并不是件容易的事。其中,最让人秃头码农们头疼的恐怕就要数如…...
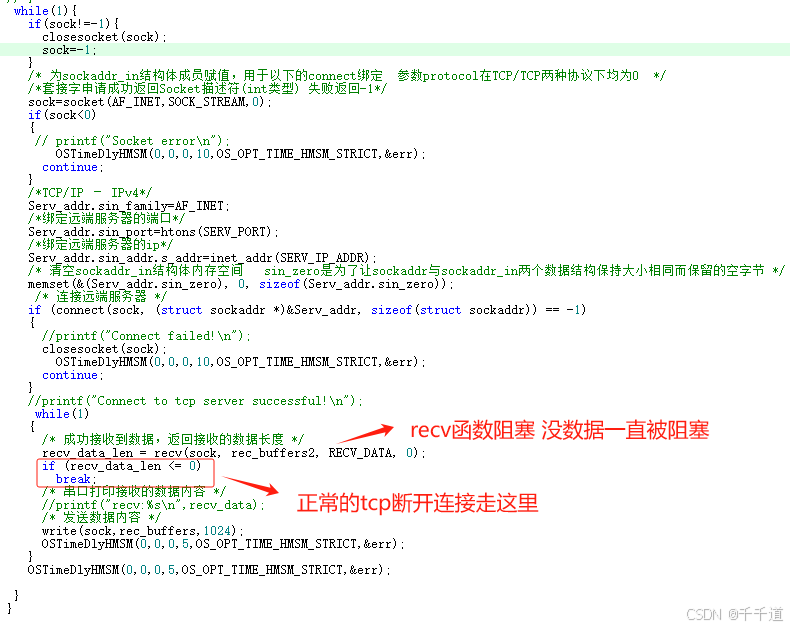
STM32被拔网线 LWIP的TCP无法重连解决方案
目录 一、问题描述 二、项目构成 三、问题解决 1.问题代码 2.解决思路 3.核心代码: 四、完整代码 1.监测网口插入拔出任务 2.TCP任务 3.创建tcp任务 4.删除tcp任务 五、总结 一、问题描述 最近遇到一个问题,就是我的stm32设备作为tcp客户端…...

Linux下开放指定端口
比如需要开放82端口: #查询是否开通 firewall-cmd --query-port82/tcp#开放端口82 firewall-cmd --zonepublic --add-port82/tcp --permanent#重新加载防火墙 firewall-cmd --reload...
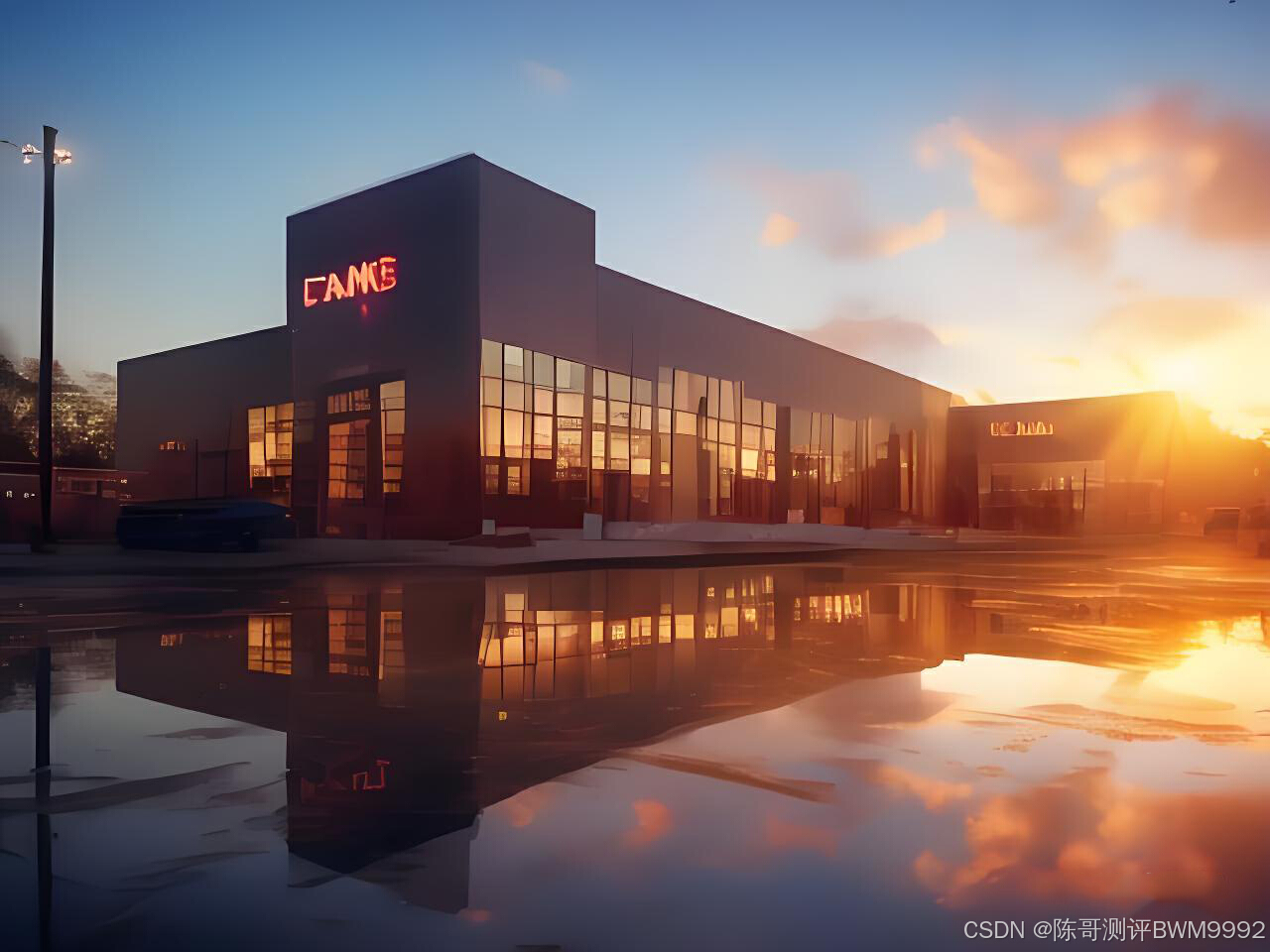
亚马逊测评行为的识别与防范:教你如何搭建安全的测评环境
亚马逊平台以其严格的内部系统和精密的买家信息对比机制而闻名。一旦发现买家存在不当评价行为,系统会立即展开深入的调查,追溯其所有的购买和评价记录。如果确认该买家存在补评价的行为,那么他/她之前留下的所有评价都可能会被系统自动删除。…...
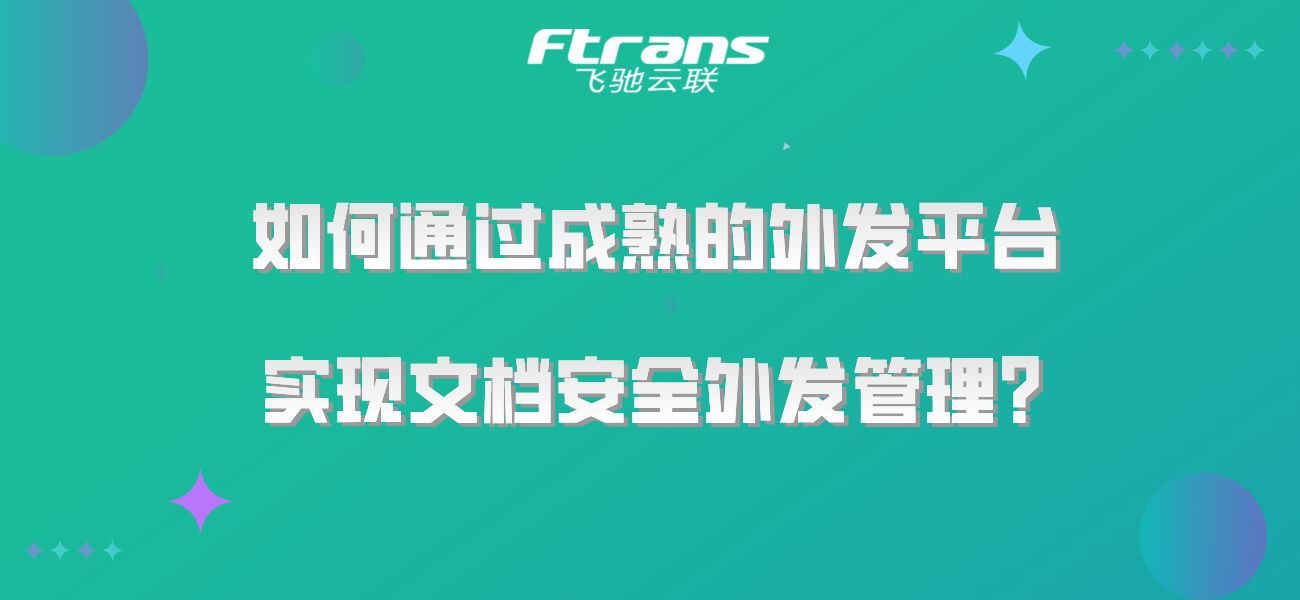
如何通过成熟的外发平台,实现文档安全外发管理?
文档安全外发管理是企业信息安全管理的重要组成部分,它涉及到企业向外发送的文件,需要进行严格的控制和管理,防止敏感或机密信息的泄露。以下是一些关键考虑因素: 文件外发的挑战:企业在文件外发时面临的主要挑战包括…...
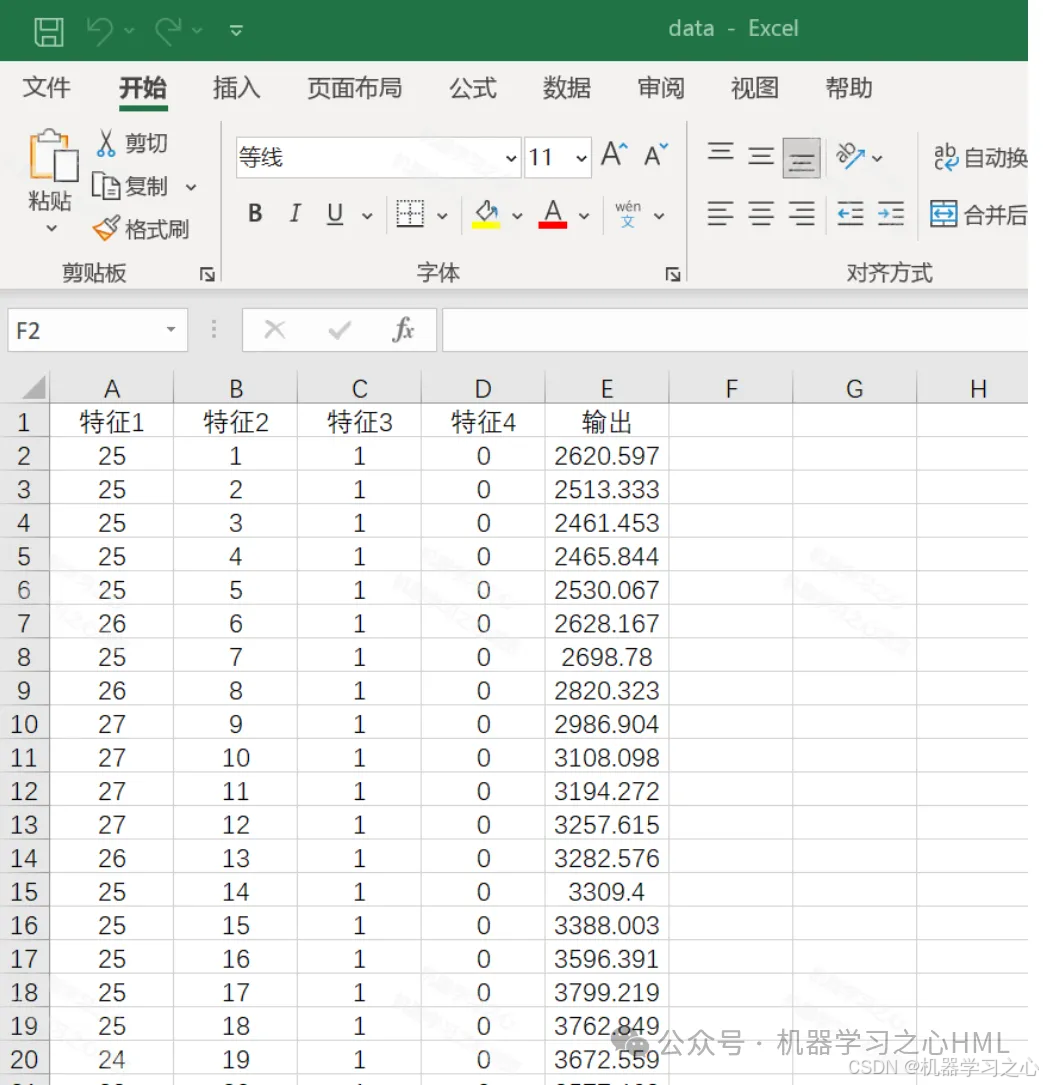
SCI一区级 | Matlab实现SSA-CNN-GRU-Multihead-Attention多变量时间序列预测
目录 效果一览基本介绍程序设计参考资料 效果一览 基本介绍 1.【SCI一区级】Matlab实现SSA-CNN-GRU-Multihead-Attention麻雀算法优化卷积门控循环单元融合多头注意力机制多变量时间序列预测,要求Matlab2023版以上; 2.输入多个特征,输出单个…...
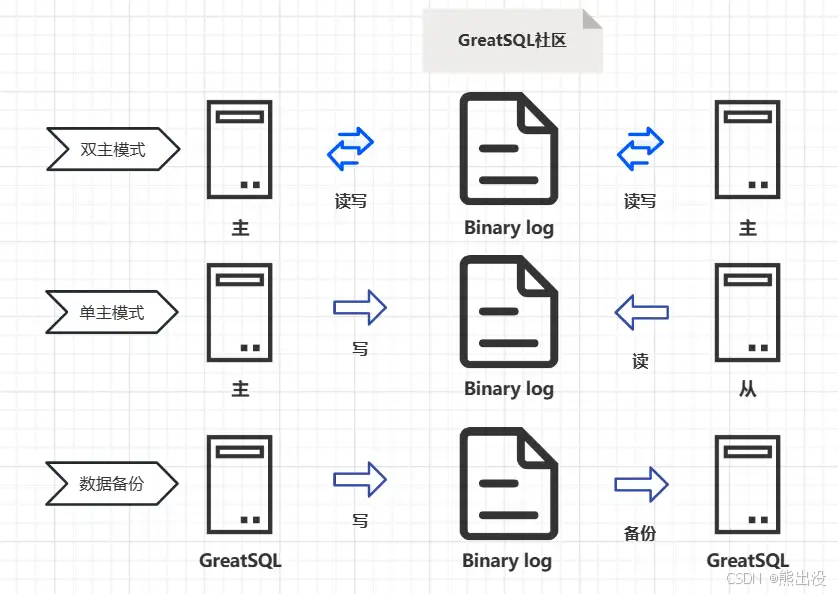
Mysql中的几种常见日志
引言 本文是对Mysql中几种常见日志及其作用的介绍 一、error log(错误日志) MySQL 中的 error log(错误日志)是一种非常重要的日志类型,它记录了 MySQL 服务器在启动、运行及关闭过程中遇到的所有重要事件、错误信…...
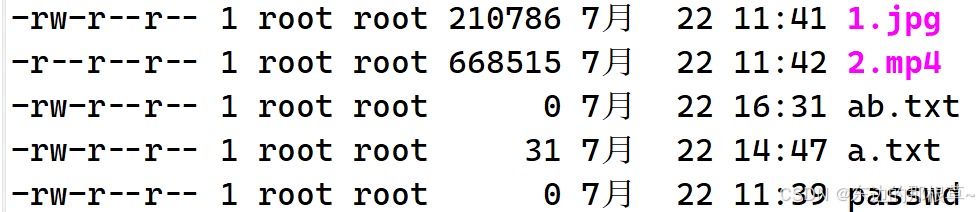
2024年7月22日(nfs samba)
一、webserver 服务器:作用是发布nginx的web项目 1、安装nginx(只下载不安装) [rootweb_server ~]# yum -y install --downloadonly --downloaddir./soft/ nginx 2、配置一个本地的nginx仓库 [rootweb_server ~]# yum -y install createrepo…...

黑龙江网络安全等级保护测评策略概述
一、简介 黑龙江省网络安全等级保护测评策略是为了保障信息系统安全稳定运行,根据《网络安全法》和相关国家标准制定的综合性安全评估和加固过程。该策略不仅要求企业和机构明确自身信息系统的安全等级,还指导其实施相应的技术防护与管理措施࿰…...