OpenMMlab导出MaskFormer/Mask2Former模型并用onnxruntime和tensorrt推理
onnxruntime推理
使用mmdeploy导出onnx模型:
from mmdeploy.apis import torch2onnx
from mmdeploy.backend.sdk.export_info import export2SDK# img = './bus.jpg'
# work_dir = './work_dir/onnx/maskformer'
# save_file = './end2end.onnx'
# deploy_cfg = './configs/mmdet/panoptic-seg/panoptic-seg_maskformer_onnxruntime_dynamic.py'
# model_cfg = '../mmdetection-3.3.0/configs/maskformer/maskformer_r50_ms-16xb1-75e_coco.py'
# model_checkpoint = '../checkpoints/maskformer_r50_ms-16xb1-75e_coco_20230116_095226-baacd858.pth'
# device = 'cpu'img = './bus.jpg'
work_dir = './work_dir/onnx/mask2former'
save_file = './end2end.onnx'
deploy_cfg = './configs/mmdet/panoptic-seg/panoptic-seg_maskformer_onnxruntime_dynamic.py'
model_cfg = '../mmdetection-3.3.0/configs/mask2former/mask2former_r50_8xb2-lsj-50e_coco.py'
model_checkpoint = '../checkpoints/mask2former_r50_8xb2-lsj-50e_coco_20220506_191028-41b088b6.pth'
device = 'cpu'# 1. convert model to onnx
torch2onnx(img, work_dir, save_file, deploy_cfg, model_cfg, model_checkpoint, device)# 2. extract pipeline info for sdk use (dump-info)
export2SDK(deploy_cfg, model_cfg, work_dir, pth=model_checkpoint, device=device)
自行编写python推理脚本,目前SDK尚未支持:
import cv2
import numpy as np
import onnxruntime
# import torch
# import torch.nn.functional as Fnum_classes = 133
num_things_classes = 80
object_mask_thr = 0.8
iou_thr = 0.8
INSTANCE_OFFSET = 1000
resize_shape = (1333, 800)
palette = [ ]
for i in range(num_classes):palette.append((np.random.randint(0, 256), np.random.randint(0, 256), np.random.randint(0, 256)))def resize_keep_ratio(image, img_scale):h, w = image.shape[0], image.shape[1]max_long_edge = max(img_scale)max_short_edge = min(img_scale)scale_factor = min(max_long_edge / max(h, w), max_short_edge / min(h, w))scale_w = int(w * float(scale_factor ) + 0.5)scale_h = int(h * float(scale_factor ) + 0.5)img_new = cv2.resize(image, (scale_w, scale_h))return img_newdef draw_binary_masks(img, binary_masks, colors, alphas=0.8):binary_masks = binary_masks.astype('uint8') * 255binary_mask_len = binary_masks.shape[0]alphas = [alphas] * binary_mask_lenfor binary_mask, color, alpha in zip(binary_masks, colors, alphas):binary_mask_complement = cv2.bitwise_not(binary_mask)rgb = np.zeros_like(img)rgb[...] = colorrgb = cv2.bitwise_and(rgb, rgb, mask=binary_mask)img_complement = cv2.bitwise_and(img, img, mask=binary_mask_complement)rgb = rgb + img_complementimg = cv2.addWeighted(img, 1 - alpha, rgb, alpha, 0)cv2.imwrite("output.jpg", img)if __name__=="__main__":image = cv2.imread('E:/vscode_workspace/mmdeploy-1.3.1/bus.jpg')image_resize = resize_keep_ratio(image, resize_shape) input = image_resize[:, :, ::-1].transpose(2, 0, 1).astype(dtype=np.float32) #BGR2RGB和HWC2CHWinput[0,:] = (input[0,:] - 123.675) / 58.395 input[1,:] = (input[1,:] - 116.28) / 57.12input[2,:] = (input[2,:] - 103.53) / 57.375input = np.expand_dims(input, axis=0)import ctypesctypes.CDLL('E:/vscode_workspace/mmdeploy-1.3.1/mmdeploy/lib/onnxruntime.dll')session_options = onnxruntime.SessionOptions()session_options.register_custom_ops_library('E:/vscode_workspace/mmdeploy-1.3.1/mmdeploy/lib/mmdeploy_onnxruntime_ops.dll') onnx_session = onnxruntime.InferenceSession('E:/vscode_workspace/mmdeploy-1.3.1/work_dir/onnx/mask2former/end2end.onnx', session_options, providers=['CPUExecutionProvider'])input_name = []for node in onnx_session.get_inputs():input_name.append(node.name)output_name=[]for node in onnx_session.get_outputs():output_name.append(node.name)inputs = {}for name in input_name:inputs[name] = inputoutputs = onnx_session.run(None, inputs)batch_cls_logits = outputs[0]batch_mask_logits = outputs[1]mask_pred_results = batch_mask_logits[0][:, :image.shape[0], :image.shape[1]]#mask_pred = F.interpolate(mask_pred_results[:, None], size=(image.shape[0], image.shape[1]), mode='bilinear', align_corners=False)[:, 0]mask_pred = np.zeros((mask_pred_results.shape[0], image.shape[0], image.shape[1]))for i in range(mask_pred_results.shape[0]):mask_pred[i] = cv2.resize(mask_pred_results[i], dsize=(image.shape[1], image.shape[0]), interpolation=cv2.INTER_LINEAR)mask_cls = batch_cls_logits[0]#scores, labels = F.softmax(torch.Tensor(mask_cls), dim=-1).max(-1)scores = np.array([np.exp(mask_cls[i]) / np.exp(mask_cls[i]).sum() for i in range(mask_cls.shape[0])]).max(-1)labels = np.array([np.exp(mask_cls[i]) / np.exp(mask_cls[i]).sum() for i in range(mask_cls.shape[0])]).argmax(-1)#mask_pred = mask_pred.sigmoid()mask_pred = 1/ (1 + np.exp(-mask_pred))#keep = labels.ne(num_classes) & (scores > object_mask_thr)keep = np.not_equal(labels, num_classes) & (scores > object_mask_thr)cur_scores = scores[keep]cur_classes = labels[keep]cur_masks = mask_pred[keep]#cur_prob_masks = cur_scores.view(-1, 1, 1) * cur_maskscur_prob_masks = cur_scores.reshape(-1, 1, 1) * cur_masksh, w = cur_masks.shape[-2:]panoptic_seg = np.full((h, w), num_classes, dtype=np.int32)cur_mask_ids = cur_prob_masks.argmax(0)instance_id = 1for k in range(cur_classes.shape[0]):pred_class = int(cur_classes[k].item())isthing = pred_class < num_things_classesmask = cur_mask_ids == kmask_area = mask.sum().item()original_area = (cur_masks[k] >= 0.5).sum().item()if mask_area > 0 and original_area > 0:if mask_area / original_area < iou_thr:continueif not isthing:panoptic_seg[mask] = pred_classelse:panoptic_seg[mask] = (pred_class + instance_id * INSTANCE_OFFSET)instance_id += 1ids = np.unique(panoptic_seg)[::-1]ids = ids[ids != num_classes]labels = np.array([id % INSTANCE_OFFSET for id in ids], dtype=np.int64)segms = (panoptic_seg[None] == ids[:, None, None])colors = [palette[label] for label in labels]draw_binary_masks(image, segms, colors)
tensorrt推理
使用mmdeploy导出engine模型:
from mmdeploy.apis import torch2onnx
from mmdeploy.backend.tensorrt.onnx2tensorrt import onnx2tensorrt
from mmdeploy.backend.sdk.export_info import export2SDK
import os# img = 'bus.jpg'
# work_dir = './work_dir/trt/maskformer'
# save_file = './end2end.onnx'
# deploy_cfg = './configs/mmdet/panoptic-seg/panoptic-seg_maskformer_tensorrt_static-1067x800.py'
# model_cfg = '../mmdetection-3.3.0/configs/maskformer/maskformer_r50_ms-16xb1-75e_coco.py'
# model_checkpoint = '../checkpoints/maskformer_r50_ms-16xb1-75e_coco_20230116_095226-baacd858.pth'
# device = 'cuda'img = 'bus.jpg'
work_dir = './work_dir/trt/mask2former'
save_file = './end2end.onnx'
deploy_cfg = './configs/mmdet/panoptic-seg/panoptic-seg_maskformer_tensorrt_static-1088x800.py'
model_cfg = '../mmdetection-3.3.0/configs/mask2former/mask2former_r50_8xb2-lsj-50e_coco.py'
model_checkpoint = '../checkpoints/mask2former_r50_8xb2-lsj-50e_coco_20220506_191028-41b088b6.pth'
device = 'cuda'# 1. convert model to IR(onnx)
torch2onnx(img, work_dir, save_file, deploy_cfg, model_cfg, model_checkpoint, device)# 2. convert IR to tensorrt
onnx_model = os.path.join(work_dir, save_file)
save_file = 'end2end.engine'
model_id = 0
device = 'cuda'
onnx2tensorrt(work_dir, save_file, model_id, deploy_cfg, onnx_model, device)# 3. extract pipeline info for sdk use (dump-info)
export2SDK(deploy_cfg, model_cfg, work_dir, pth=model_checkpoint, device=device)
自行编写python推理脚本,目前SDK尚未支持:
maskformer
import cv2
import ctypes
import numpy as np
import tensorrt as trt
import pycuda.autoinit
import pycuda.driver as cuda num_classes = 133
num_things_classes = 80
object_mask_thr = 0.8
iou_thr = 0.8
INSTANCE_OFFSET = 1000
resize_shape = (1333, 800)
palette = [ ]
for i in range(num_classes):palette.append((np.random.randint(0, 256), np.random.randint(0, 256), np.random.randint(0, 256)))def resize_keep_ratio(image, img_scale):h, w = image.shape[0], image.shape[1]max_long_edge = max(img_scale)max_short_edge = min(img_scale)scale_factor = min(max_long_edge / max(h, w), max_short_edge / min(h, w))scale_w = int(w * float(scale_factor ) + 0.5)scale_h = int(h * float(scale_factor ) + 0.5)img_new = cv2.resize(image, (scale_w, scale_h))return img_newdef draw_binary_masks(img, binary_masks, colors, alphas=0.8):binary_masks = binary_masks.astype('uint8') * 255binary_mask_len = binary_masks.shape[0]alphas = [alphas] * binary_mask_lenfor binary_mask, color, alpha in zip(binary_masks, colors, alphas):binary_mask_complement = cv2.bitwise_not(binary_mask)rgb = np.zeros_like(img)rgb[...] = colorrgb = cv2.bitwise_and(rgb, rgb, mask=binary_mask)img_complement = cv2.bitwise_and(img, img, mask=binary_mask_complement)rgb = rgb + img_complementimg = cv2.addWeighted(img, 1 - alpha, rgb, alpha, 0)cv2.imwrite("output.jpg", img)if __name__=="__main__":logger = trt.Logger(trt.Logger.WARNING)ctypes.CDLL('E:/vscode_workspace/mmdeploy-1.3.1/mmdeploy/lib/mmdeploy_tensorrt_ops.dll')with open("E:/vscode_workspace/mmdeploy-1.3.1/work_dir/trt/maskformer/end2end.engine", "rb") as f, trt.Runtime(logger) as runtime:engine = runtime.deserialize_cuda_engine(f.read())context = engine.create_execution_context()h_input = cuda.pagelocked_empty(trt.volume(context.get_binding_shape(0)), dtype=np.float32)h_output0 = cuda.pagelocked_empty(trt.volume(context.get_binding_shape(1)), dtype=np.float32)h_output1 = cuda.pagelocked_empty(trt.volume(context.get_binding_shape(2)), dtype=np.float32)d_input = cuda.mem_alloc(h_input.nbytes)d_output0 = cuda.mem_alloc(h_output0.nbytes)d_output1 = cuda.mem_alloc(h_output1.nbytes)stream = cuda.Stream()image = cv2.imread('E:/vscode_workspace/mmdeploy-1.3.1/bus.jpg')image_resize = resize_keep_ratio(image, resize_shape) input = image_resize[:, :, ::-1].transpose(2, 0, 1).astype(dtype=np.float32) #BGR2RGB和HWC2CHWinput[0,:] = (input[0,:] - 123.675) / 58.395 input[1,:] = (input[1,:] - 116.28) / 57.12input[2,:] = (input[2,:] - 103.53) / 57.375h_input = input.flatten()with engine.create_execution_context() as context:cuda.memcpy_htod_async(d_input, h_input, stream)context.execute_async_v2(bindings=[int(d_input), int(d_output0), int(d_output1)], stream_handle=stream.handle)cuda.memcpy_dtoh_async(h_output0, d_output0, stream)cuda.memcpy_dtoh_async(h_output1, d_output1, stream)stream.synchronize() batch_cls_logits = h_output0.reshape(context.get_binding_shape(1))batch_mask_logits = h_output1.reshape(context.get_binding_shape(2))mask_pred_results = batch_mask_logits[0][:, :image.shape[0], :image.shape[1]]#mask_pred = F.interpolate(mask_pred_results[:, None], size=(image.shape[0], image.shape[1]), mode='bilinear', align_corners=False)[:, 0]mask_pred = np.zeros((mask_pred_results.shape[0], image.shape[0], image.shape[1]))for i in range(mask_pred_results.shape[0]):mask_pred[i] = cv2.resize(mask_pred_results[i], dsize=(image.shape[1], image.shape[0]), interpolation=cv2.INTER_LINEAR)mask_cls = batch_cls_logits[0]#scores, labels = F.softmax(torch.Tensor(mask_cls), dim=-1).max(-1)scores = np.array([np.exp(mask_cls[i]) / np.exp(mask_cls[i]).sum() for i in range(mask_cls.shape[0])]).max(-1)labels = np.array([np.exp(mask_cls[i]) / np.exp(mask_cls[i]).sum() for i in range(mask_cls.shape[0])]).argmax(-1)#mask_pred = mask_pred.sigmoid()mask_pred = 1/ (1 + np.exp(-mask_pred))#keep = labels.ne(num_classes) & (scores > object_mask_thr)keep = np.not_equal(labels, num_classes) & (scores > object_mask_thr)cur_scores = scores[keep]cur_classes = labels[keep]cur_masks = mask_pred[keep]#cur_prob_masks = cur_scores.view(-1, 1, 1) * cur_maskscur_prob_masks = cur_scores.reshape(-1, 1, 1) * cur_masksh, w = cur_masks.shape[-2:]panoptic_seg = np.full((h, w), num_classes, dtype=np.int32)cur_mask_ids = cur_prob_masks.argmax(0)instance_id = 1for k in range(cur_classes.shape[0]):pred_class = int(cur_classes[k].item())isthing = pred_class < num_things_classesmask = cur_mask_ids == kmask_area = mask.sum().item()original_area = (cur_masks[k] >= 0.5).sum().item()if mask_area > 0 and original_area > 0:if mask_area / original_area < iou_thr:continueif not isthing:panoptic_seg[mask] = pred_classelse:panoptic_seg[mask] = (pred_class + instance_id * INSTANCE_OFFSET)instance_id += 1ids = np.unique(panoptic_seg)[::-1]ids = ids[ids != num_classes]labels = np.array([id % INSTANCE_OFFSET for id in ids], dtype=np.int64)segms = (panoptic_seg[None] == ids[:, None, None])max_label = int(max(labels) if len(labels) > 0 else 0)colors = [palette[label] for label in labels]draw_binary_masks(image, segms, colors)
mask2former
import cv2
import ctypes
import numpy as np
import tensorrt as trt
import pycuda.autoinit
import pycuda.driver as cuda num_classes = 133
num_things_classes = 80
object_mask_thr = 0.8
iou_thr = 0.8
INSTANCE_OFFSET = 1000
resize_shape = (1333, 800)
palette = [ ]
for i in range(num_classes):palette.append((np.random.randint(0, 256), np.random.randint(0, 256), np.random.randint(0, 256)))def resize_keep_ratio(image, img_scale):h, w = image.shape[0], image.shape[1]max_long_edge = max(img_scale)max_short_edge = min(img_scale)scale_factor = min(max_long_edge / max(h, w), max_short_edge / min(h, w))scale_w = int(w * float(scale_factor ) + 0.5)scale_h = int(h * float(scale_factor ) + 0.5)img_new = cv2.resize(image, (scale_w, scale_h))return img_newdef draw_binary_masks(img, binary_masks, colors, alphas=0.8):binary_masks = binary_masks.astype('uint8') * 255binary_mask_len = binary_masks.shape[0]alphas = [alphas] * binary_mask_lenfor binary_mask, color, alpha in zip(binary_masks, colors, alphas):binary_mask_complement = cv2.bitwise_not(binary_mask)rgb = np.zeros_like(img)rgb[...] = colorrgb = cv2.bitwise_and(rgb, rgb, mask=binary_mask)img_complement = cv2.bitwise_and(img, img, mask=binary_mask_complement)rgb = rgb + img_complementimg = cv2.addWeighted(img, 1 - alpha, rgb, alpha, 0)cv2.imwrite("output.jpg", img)if __name__=="__main__":logger = trt.Logger(trt.Logger.WARNING)ctypes.CDLL('E:/vscode_workspace/mmdeploy-1.3.1/mmdeploy/lib/mmdeploy_tensorrt_ops.dll')with open("E:/vscode_workspace/mmdeploy-1.3.1/work_dir/trt/mask2former/end2end.engine", "rb") as f, trt.Runtime(logger) as runtime:engine = runtime.deserialize_cuda_engine(f.read())context = engine.create_execution_context()h_input = cuda.pagelocked_empty(trt.volume(context.get_binding_shape(0)), dtype=np.float32)h_output0 = cuda.pagelocked_empty(trt.volume(context.get_binding_shape(1)), dtype=np.float32)h_output1 = cuda.pagelocked_empty(trt.volume(context.get_binding_shape(2)), dtype=np.float32)d_input = cuda.mem_alloc(h_input.nbytes)d_output0 = cuda.mem_alloc(h_output0.nbytes)d_output1 = cuda.mem_alloc(h_output1.nbytes)stream = cuda.Stream()image = cv2.imread('E:/vscode_workspace/mmdeploy-1.3.1/bus.jpg')image_resize = resize_keep_ratio(image, resize_shape) scale = (image.shape[0]/image_resize.shape[0], image.shape[1]/image_resize.shape[1])pad_shape = (np.ceil(image_resize.shape[1]/32)*32, np.ceil(image_resize.shape[0]/32)*32) pad_x, pad_y = int(pad_shape[0]-image_resize.shape[1]), int(pad_shape[1]-image_resize.shape[0])image_pad = cv2.copyMakeBorder(image_resize, 0, pad_y, 0, pad_x, cv2.BORDER_CONSTANT, value=0)input = image_pad[:, :, ::-1].transpose(2, 0, 1).astype(dtype=np.float32) #BGR2RGB和HWC2CHW input[0,:] = (input[0,:] - 123.675) / 58.395 input[1,:] = (input[1,:] - 116.28) / 57.12input[2,:] = (input[2,:] - 103.53) / 57.375h_input = input.flatten()with engine.create_execution_context() as context:cuda.memcpy_htod_async(d_input, h_input, stream)context.execute_async_v2(bindings=[int(d_input), int(d_output0), int(d_output1)], stream_handle=stream.handle)cuda.memcpy_dtoh_async(h_output0, d_output0, stream)cuda.memcpy_dtoh_async(h_output1, d_output1, stream)stream.synchronize() batch_cls_logits = h_output0.reshape(context.get_binding_shape(1))batch_mask_logits = h_output1.reshape(context.get_binding_shape(2))mask_pred_results = batch_mask_logits[0][:, :image.shape[0], :image.shape[1]]#mask_pred = F.interpolate(mask_pred_results[:, None], size=(image.shape[0], image.shape[1]), mode='bilinear', align_corners=False)[:, 0]mask_pred = np.zeros((mask_pred_results.shape[0], image.shape[0], image.shape[1]))for i in range(mask_pred_results.shape[0]):mask_pred[i] = cv2.resize(mask_pred_results[i], dsize=(image.shape[1], image.shape[0]), interpolation=cv2.INTER_LINEAR)mask_cls = batch_cls_logits[0]#scores, labels = F.softmax(torch.Tensor(mask_cls), dim=-1).max(-1)scores = np.array([np.exp(mask_cls[i]) / np.exp(mask_cls[i]).sum() for i in range(mask_cls.shape[0])]).max(-1)labels = np.array([np.exp(mask_cls[i]) / np.exp(mask_cls[i]).sum() for i in range(mask_cls.shape[0])]).argmax(-1)#mask_pred = mask_pred.sigmoid()mask_pred = 1/ (1 + np.exp(-mask_pred))#keep = labels.ne(num_classes) & (scores > object_mask_thr)keep = np.not_equal(labels, num_classes) & (scores > object_mask_thr)cur_scores = scores[keep]cur_classes = labels[keep]cur_masks = mask_pred[keep]#cur_prob_masks = cur_scores.view(-1, 1, 1) * cur_maskscur_prob_masks = cur_scores.reshape(-1, 1, 1) * cur_masksh, w = cur_masks.shape[-2:]panoptic_seg = np.full((h, w), num_classes, dtype=np.int32)cur_mask_ids = cur_prob_masks.argmax(0)instance_id = 1for k in range(cur_classes.shape[0]):pred_class = int(cur_classes[k].item())isthing = pred_class < num_things_classesmask = cur_mask_ids == kmask_area = mask.sum().item()original_area = (cur_masks[k] >= 0.5).sum().item()if mask_area > 0 and original_area > 0:if mask_area / original_area < iou_thr:continueif not isthing:panoptic_seg[mask] = pred_classelse:panoptic_seg[mask] = (pred_class + instance_id * INSTANCE_OFFSET)instance_id += 1ids = np.unique(panoptic_seg)[::-1]ids = ids[ids != num_classes]labels = np.array([id % INSTANCE_OFFSET for id in ids], dtype=np.int64)segms = (panoptic_seg[None] == ids[:, None, None])max_label = int(max(labels) if len(labels) > 0 else 0)colors = [palette[label] for label in labels]draw_binary_masks(image, segms, colors)
推理结果:
相关文章:
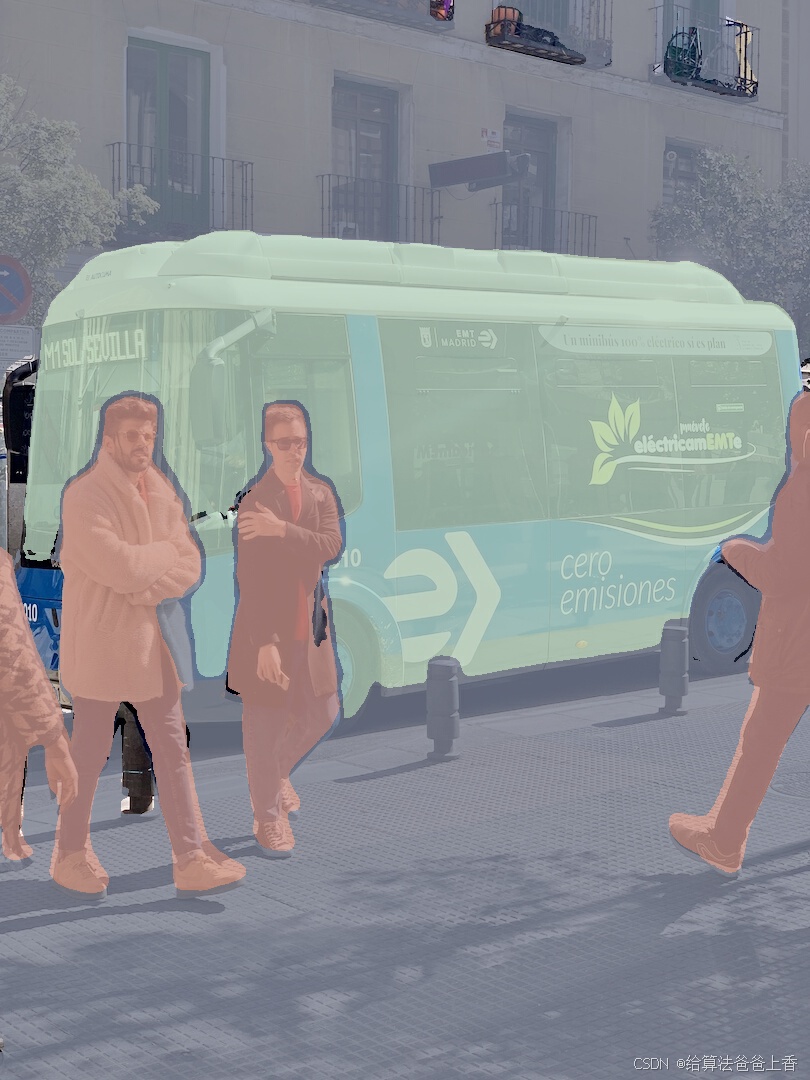
OpenMMlab导出MaskFormer/Mask2Former模型并用onnxruntime和tensorrt推理
onnxruntime推理 使用mmdeploy导出onnx模型: from mmdeploy.apis import torch2onnx from mmdeploy.backend.sdk.export_info import export2SDK# img ./bus.jpg # work_dir ./work_dir/onnx/maskformer # save_file ./end2end.onnx # deploy_cfg ./configs/m…...

若依微服务中配置 MySQL + DM 多数据源
文章目录 1、导入 MySQL 和达梦(DM)依赖2、在 application-druid.yml 中配置达梦(DM)数据源3、在 DruidConfig 类中配置多数据源信息4、在 Service 层或方法级别切换数据源4.1 在 Service 类上切换到从库数据源4.2 在方法级别切换…...

一些前端组件介绍
wangEditor : 一款开源 Web 富文本编辑器,可用于 jQuery Vue React等 https://www.wangeditor.com/ Handsontable:一款前端可编辑电子表格https://blog.csdn.net/carcarrot/article/details/108492356mitt:Mitt 是一个在 Vue.js 应…...
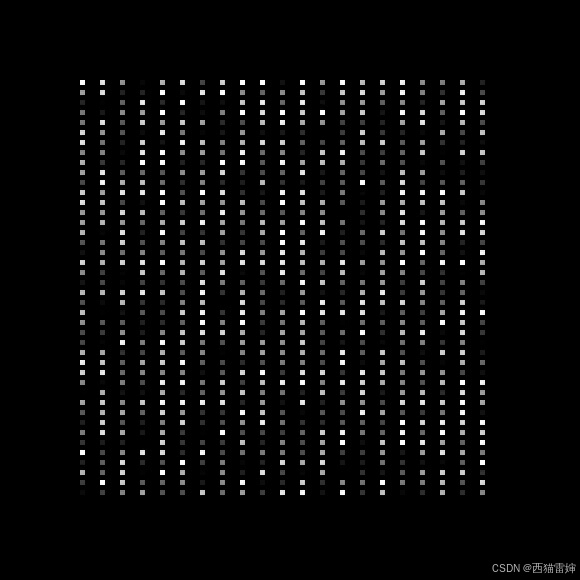
python学opencv|读取图像(九)用numpy创建黑白相间灰度图
【1】引言 前述学习过程中,掌握了用numpy创建矩阵数据,把所有像素点的BGR取值设置为0,然后创建纯黑灰度图的方法,具体链接为: python学opencv|读取图像(八)用numpy创建纯黑灰度图-CSDN博客 在…...

AtCoder Beginner Contest 383
C - Humidifier 3 Description 一个 h w h \times w hw 的网格,每个格子可能是墙、空地或者城堡。 一个格子是好的,当且仅当从至少一个城堡出发,走不超过 d d d 步能到达。(只能上下左右走,不能穿墙)&…...

20. 内置模块
一、random模块 random 模块用来创建随机数的模块。 random.random() # 随机生成一个大于0且小于1之间的小数 random.randint(a, b) # 随机生成一个大于等于a小于等于b的随机整数 random.uniform(a, b) …...

《知识拓展 · 统一建模语言UML》
📢 大家好,我是 【战神刘玉栋】,有10多年的研发经验,致力于前后端技术栈的知识沉淀和传播。 💗 🌻 CSDN入驻不久,希望大家多多支持,后续会继续提升文章质量,绝不滥竽充数…...
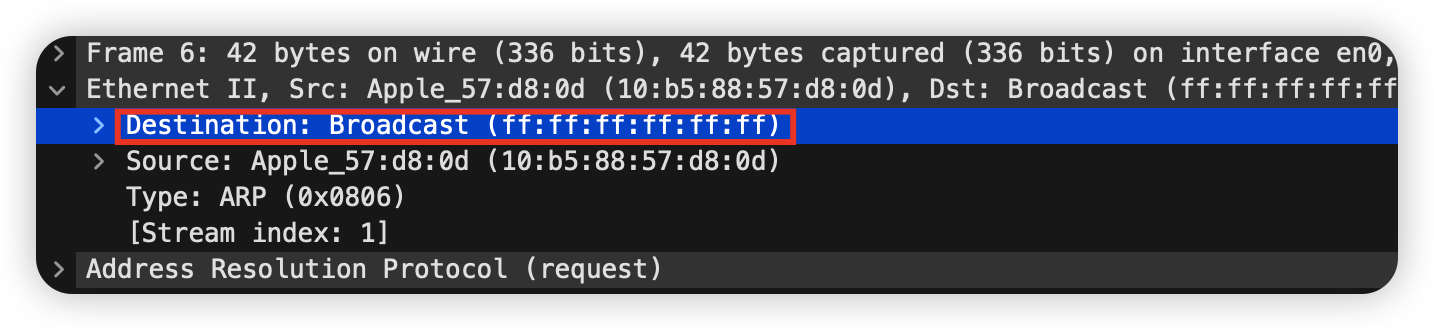
计算机网络-Wireshark探索ARP
使用工具 Wiresharkarp: To inspect and clear the cache used by the ARP protocol on your computer.curl(MacOS)ifconfig(MacOS or Linux): to inspect the state of your computer’s network interface.route/netstat: To inspect the routes used by your computer.Brows…...
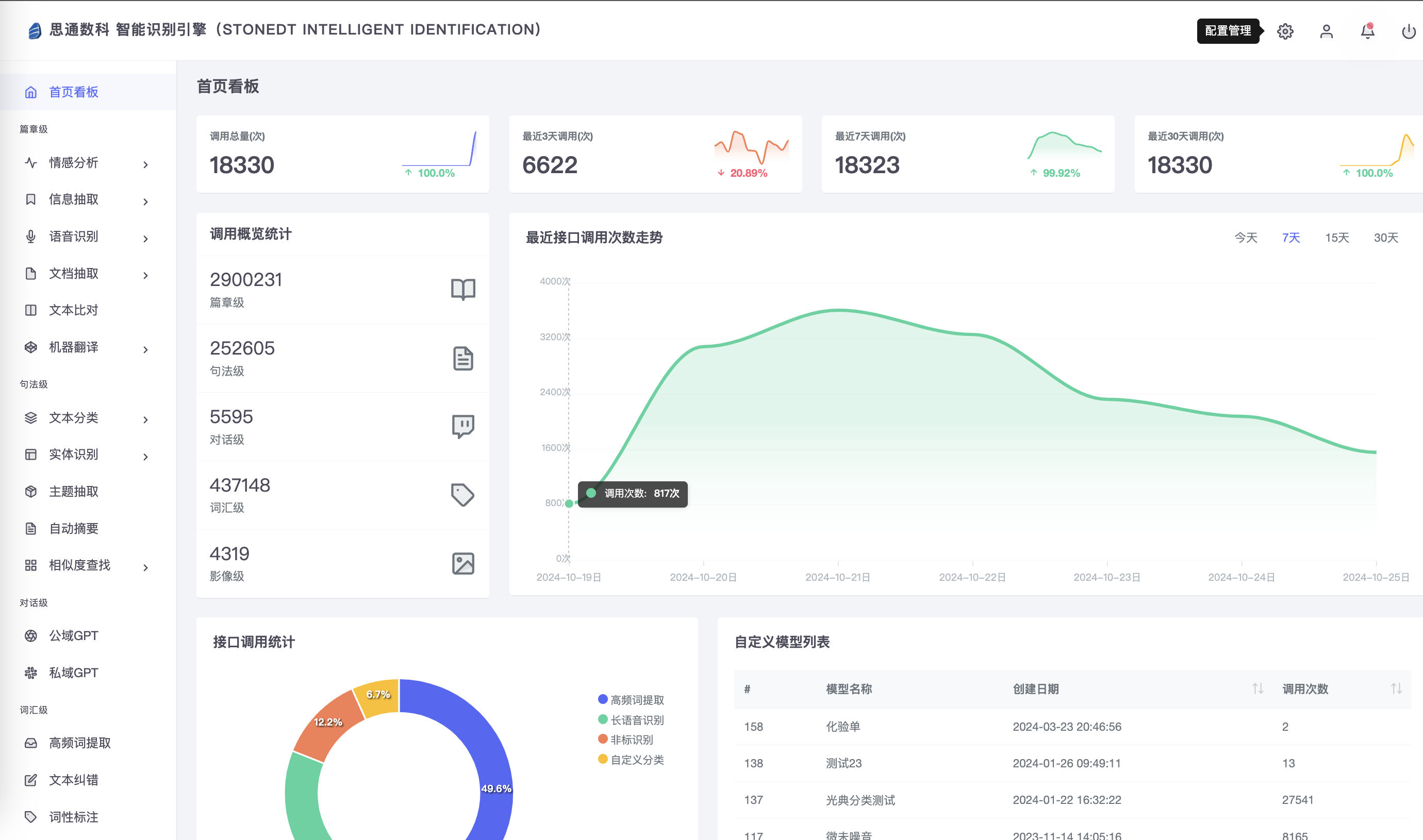
减少30%人工处理时间,AI OCR与表格识别助力医疗化验单快速处理
在医疗行业,化验单作为重要的诊断依据和数据来源,涉及大量的文字和表格信息,传统的手工输入和数据处理方式不仅繁琐,而且容易出错,给医院的运营效率和数据准确性带来较大挑战。随着人工智能技术的快速发展,…...
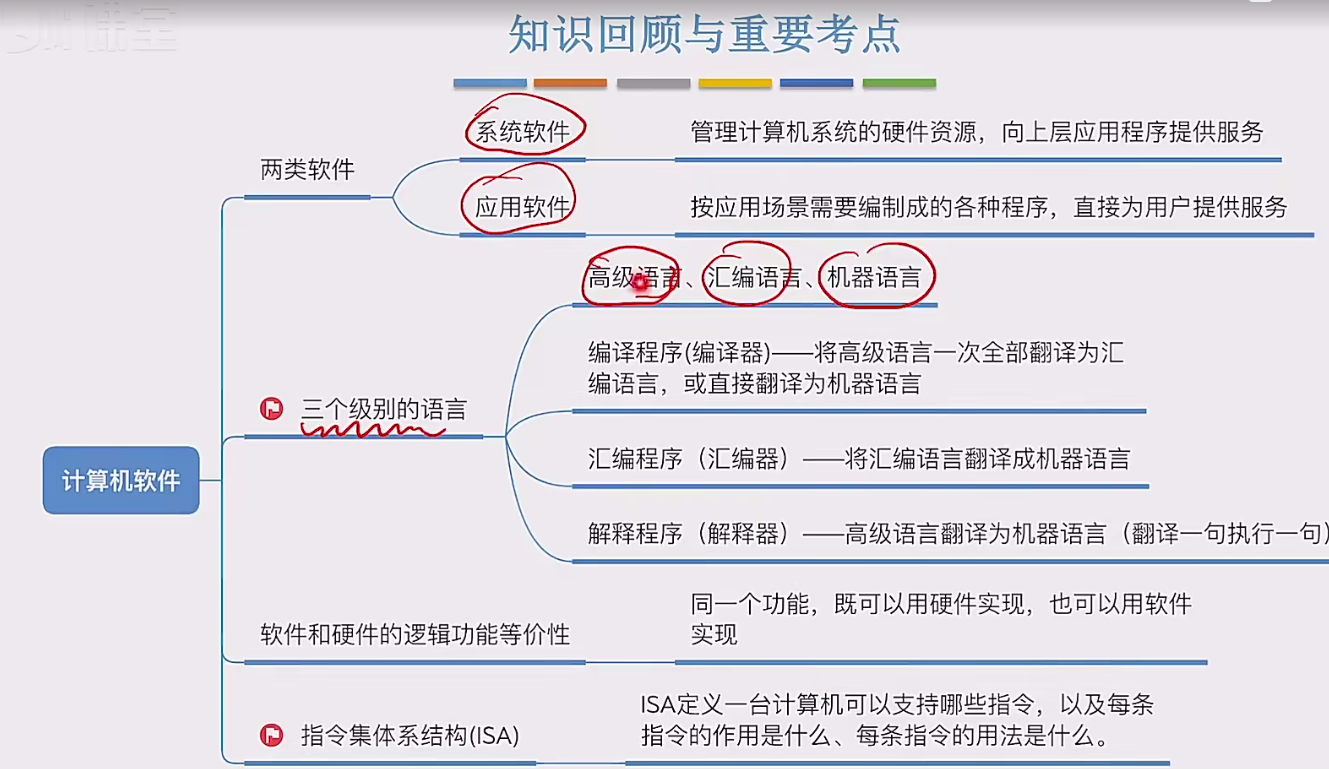
1.2.3计算机软件
一个完整的计算机系统由硬件和软件组成,用户使用软件,而软件运行在硬件之上,软件进一步的划分为两类:应用软件和系统软件。普通用户通常只会跟应用软件打交道。应用软件是为了解决用户的某种特定的需求而研发出来的。除了每个人都…...

二、uni-forms
避坑指南:uni-forms表单在uni-app中的实践经验-CSDN博客...
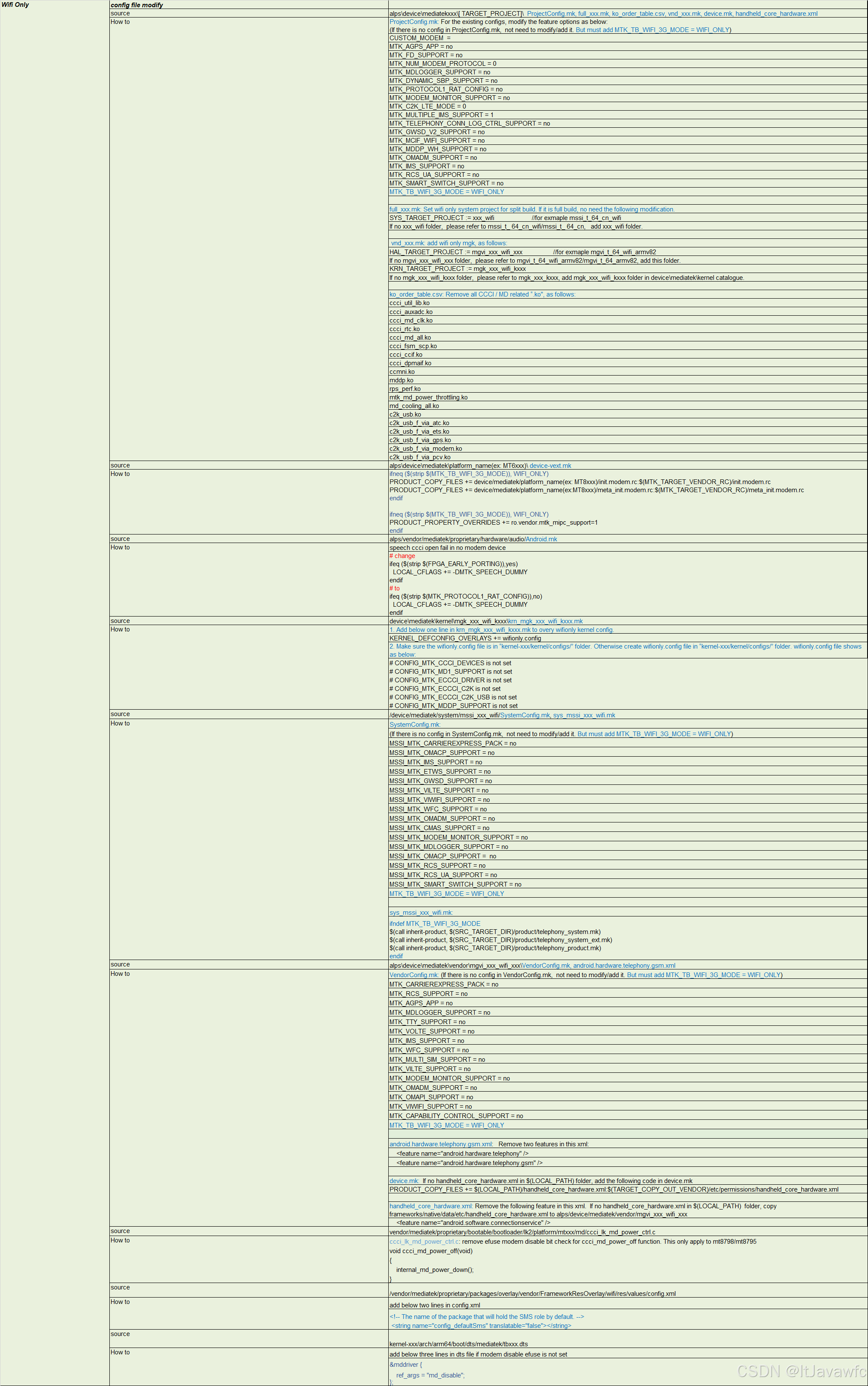
Android13开机向导
文章目录 前言需求-场景第三方资料说明需求思路按照平台 思路 从配置上去 feature换个思路,去feature。SimMissingActivity 判断跳过逻辑SetupWizardUtils 判断SIM 、 hasSystemFeature FEATURE_TELEPHONYPackageManager.FEATURE_TELEPHONYApplicationPackageManage…...

软件测试丨Appium 源码分析与定制
在本文中,我们将深入Appium的源码,探索它的底层架构、定制化使用方法和给软件测试带来的优势。我们将详细介绍这些技术如何解决实际问题,并与大家分享一些实用的案例,以帮助读者更好地理解和应用这一技术。 Appium简介 什么是App…...
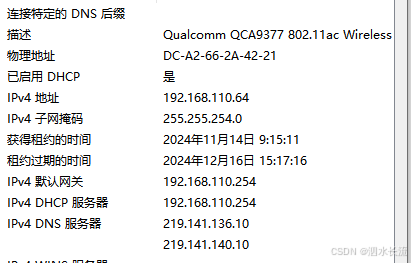
1.网络知识-IP与子网掩码的关系及计算实例
IP与子网掩码 说实话,之前没有注意过,今天我打开自己的办公地电脑,看到我的网络配置如下: 我看到我的子网掩码是255.255.254.0,我就奇怪了,我经常见到的子网掩码都是255.255.255.0啊?难道公司配…...

Android中Gradle常用配置
前言 本文记录了一些常用的gradle配置,基本上都是平时开发中可能会使用到的,如果有新内容会不定时更新,附官网 1.依赖库版本写法 不推荐写法: dependencies {compile com.example.code.abc:def:2. // 不推荐的写法 }这样写虽然可…...
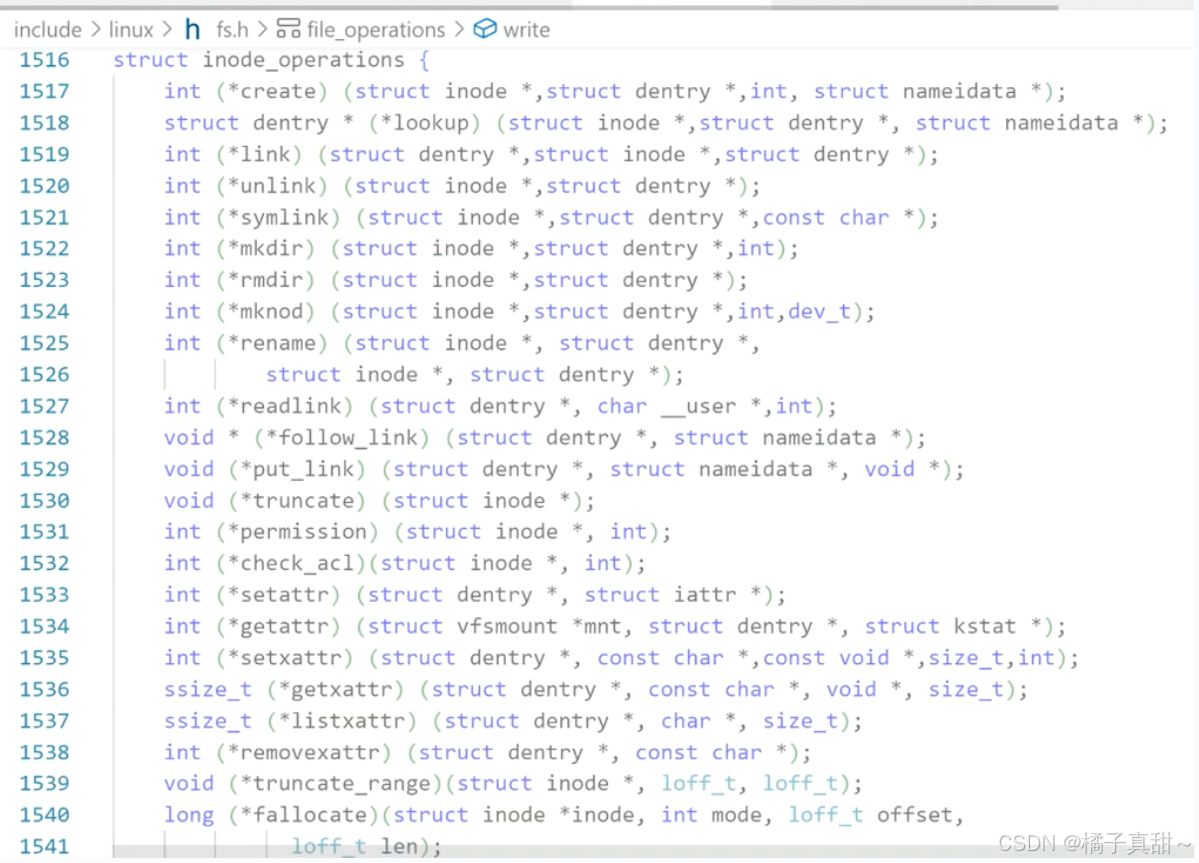
Linux操作系统3-文件与IO操作2(文件描述符fd与文件重定向)
上篇文章:Linux操作系统3-文件与IO操作1(从C语言IO操作到系统调用)-CSDN博客 本篇代码Gitee仓库:myLerningCode 橘子真甜/Linux操作系统与网络编程学习 - 码云 - 开源中国 (gitee.com) 本篇重点:文件描述符fd与文件重定向 目录 一. 文件描述…...

k8s调度策略
调度策略 binpack(装箱策略) Binpacking策略(又称装箱问题)是一种优化算法,用于将物品有效地放入容器(或“箱子”)中,使得所使用的容器数量最少,Kubernetes等集群管理系…...
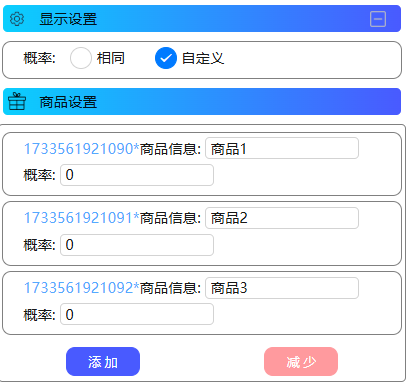
uniapp中父组件传参到子组件页面渲染不生效问题处理实战记录
上篇文件介绍了,父组件数据更新正常但是页面渲染不生效的问题,详情可以看下:uniapp中父组件数组更新后与页面渲染数组不一致实战记录 本文在此基础上由于新增需求衍生出新的问题.本文只记录一下解决思路. 下面说下新增需求方便理解场景: 商品信息设置中添加抽奖概率设置…...
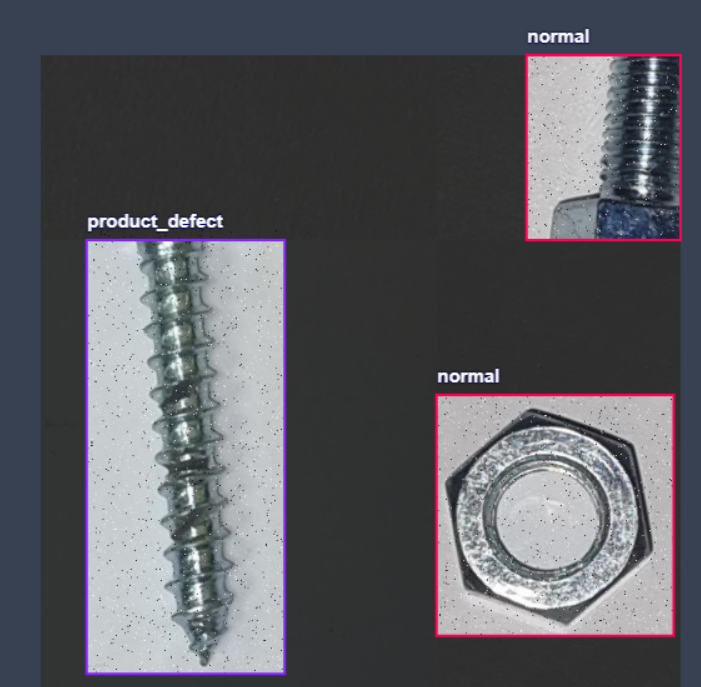
螺丝螺帽缺陷检测识别数据集,支持yolo,coco,voc三种格式的标记,一共3081张图片
螺丝螺帽缺陷检测识别数据集,支持yolo,coco,voc三种格式的标记,一共3081张图片 3081总图像数 数据集分割 训练组90% 2781图片 有效集7% 220图片 测试集3% 80图片 预处理…...
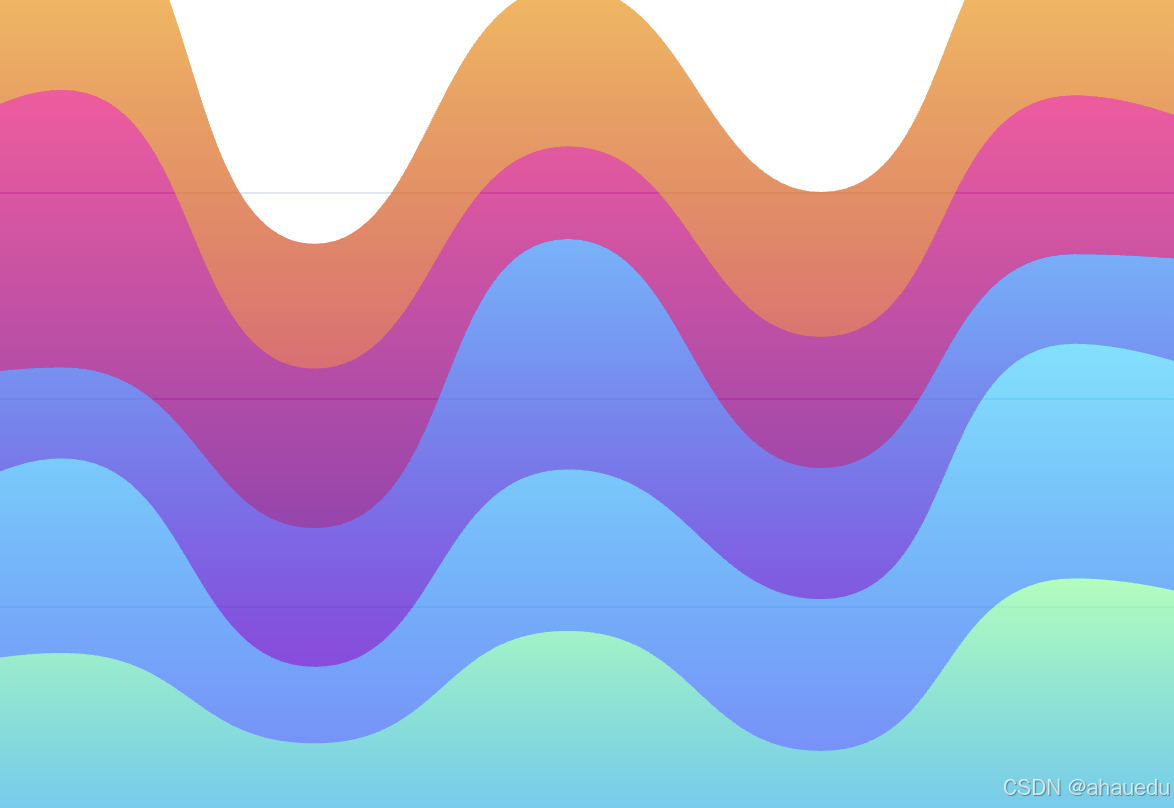
一个简单带颜色的Map
越简单 越实用。越少设计,越易懂。 需求背景: 创建方法,声明一个hashset, 元素为 {“#DE3200”, “#FA8C00”, “#027B00”, “#27B600”, “#5EB600”} 。 对应的key为 key1 、key2、key3、key4、key5。 封装该方法,…...

kubeadm安装K8s集群之基础环境配置
系列文章目录 1.kubeadm安装K8s集群之基础环境配置 2.kubeadm安装K8s集群之高可用组件keepalivednginx 3.kubeadm安装K8s集群之master节点加入 4.kubeadm安装K8s集群之worker1节点加入 kubeadm安装K8s集群基础环境配置 1.首先确保所有机器可以通信,然后配置主机host…...
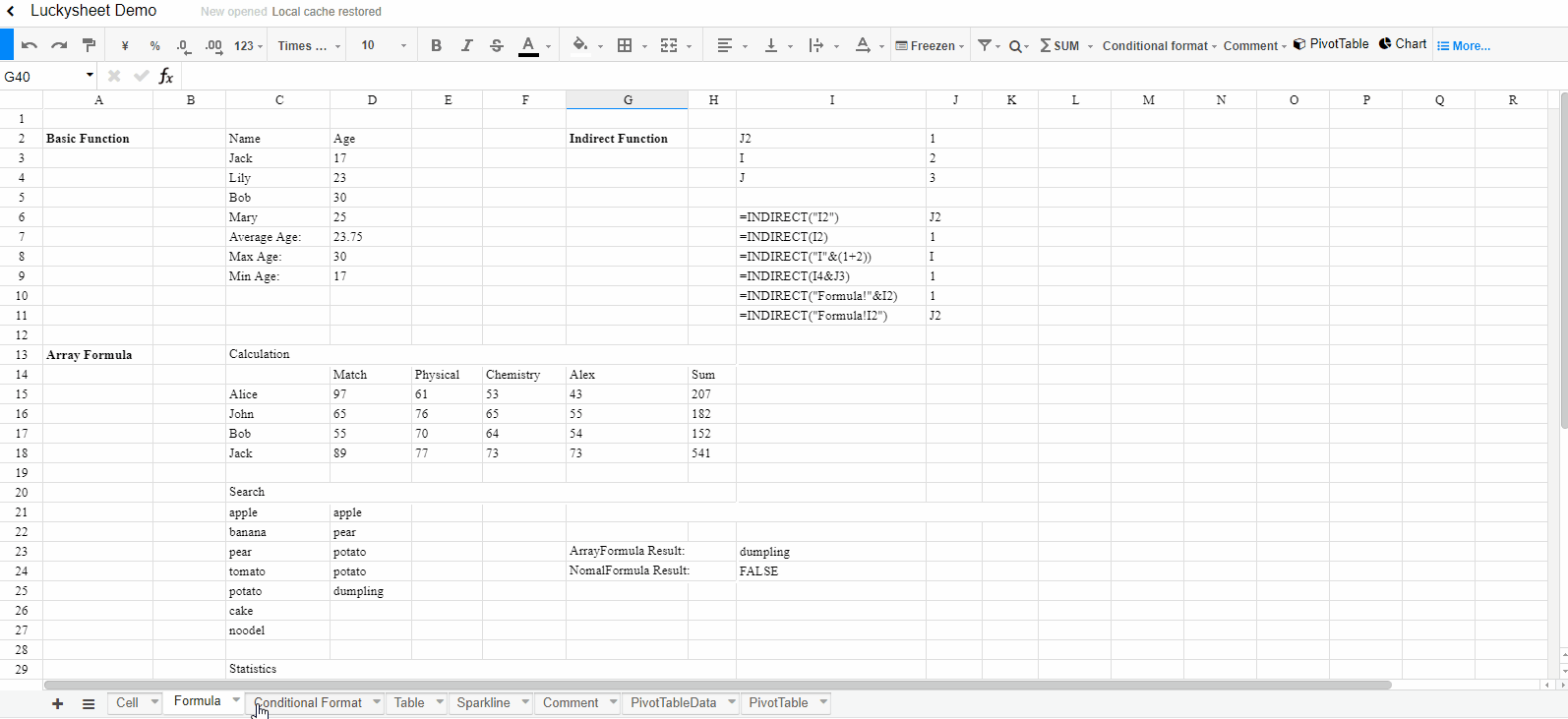
前端实现在线预览excel文件
在前端开发中,经常会遇到需要在线预览各种文件的需求。本文将介绍如何使用前端技术实现在线预览 Excel 文件的功能。 一、基于微软office服务的excel预览 获取要预览的 Excel 文件的 URL(例如存储在 OneDrive 或 SharePoint 上的文件)。 使…...
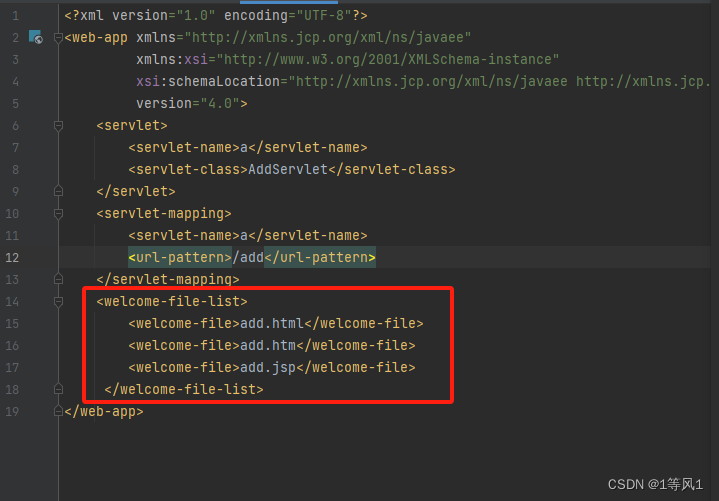
关于idea-Java-servlet-Tomcat-Web开发中出现404NOT FOUND问题的解决
在做web项目时,第一次使用servlet开发链接前端和后端的操作,果不其然,遇到了诸多问题,而遇到最多的就是运行项目打开页面时出现404NOT FOUND的情况。因为这个问题我也是鼓捣了好久,上网查了许多资料才最终解决…...
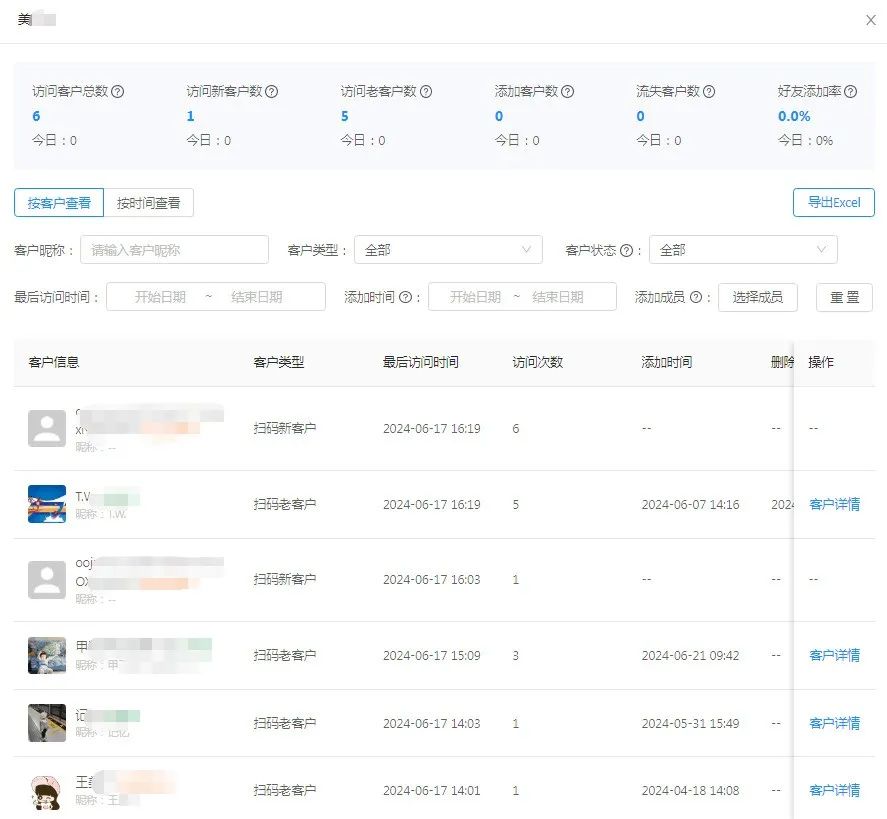
SCRM私域流量管理工具助力企业微信电商转型升级
内容概要 在当今数字化时代,SCRM(社交客户关系管理)私域流量管理工具正逐渐成为企业转型的重要助力。尤其是在电商领域,企业微信的兴起为许多公司打开了新的销售渠道,通过SCRM系统的高效整合,企业能够更加…...

三相异步电动机为什么能够旋转?
三相异步电动机,作为一种广泛应用于工业、农业及其他领域的电动机,其工作原理的理解对于工程技术人员以及相关从业者来说至关重要。 一、三相异步电动机的基本结构 三相异步电动机主要由定子、转子和机壳组成。定子是电动机的静止部分,包含…...
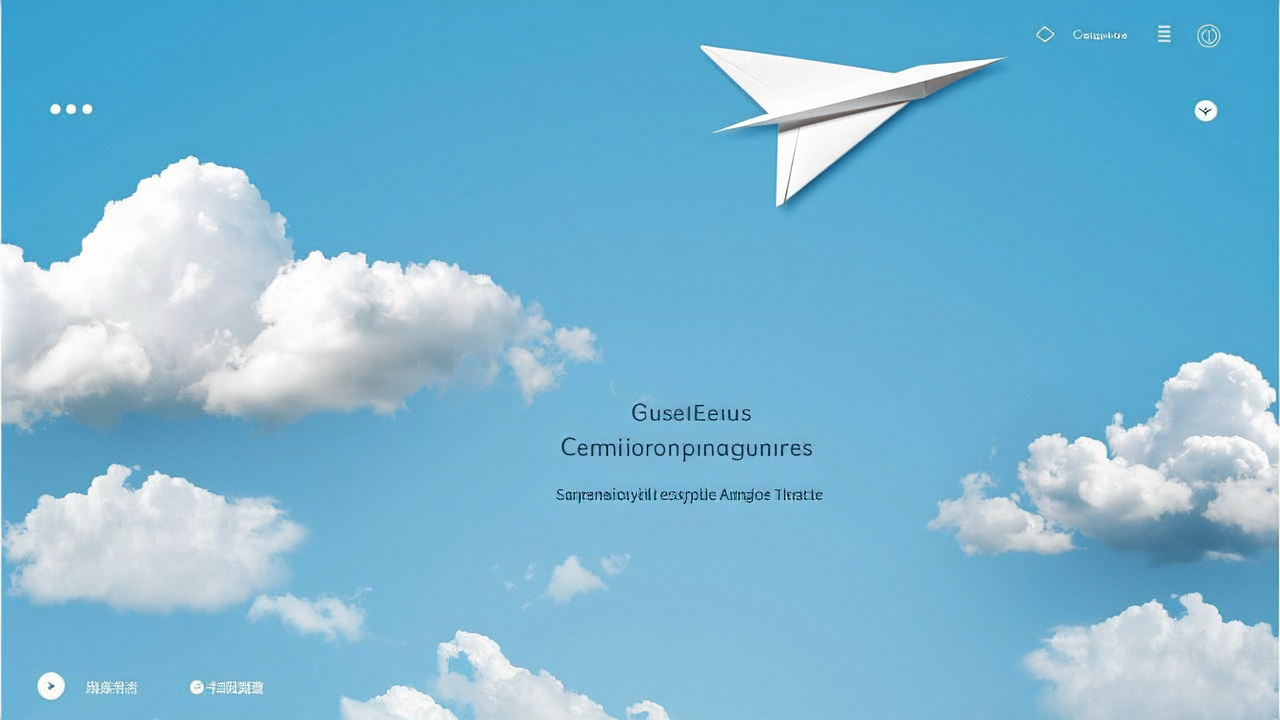
优化移动端H5:常见问题与解决方案
移动端H5开发中的“坑”与解决方案 本文介绍了开发中遇到的几个关于移动端H5开发中的小问题,以及解决的方法。 一、iOS滑动不流畅问题 在iOS设备上,H5页面的滑动效果有时会出现不流畅的情况,特别是在页面高度超过一屏时。这通常是由于iOS的…...

TM1不藏私系列——#10. TM1快速运算的秘密武器-Feeder
与其他BI产品对比,TM1的快速运算能力一骑绝尘。 但是在多维度的数据组合下,TM1是依据什么进行运算的呢? 今天将和大家一同了解TM1快速运算的秘密武器-Feeder。 上期我们提到通过配置维度中的元素权重,可以在合并层级加总计算。除…...

【Python】【Conda 】Conda vs venv:Python开发者的虚拟环境选择指南
目录 引言一、概述1.1 Conda 虚拟环境1.2 Python venv 虚拟环境 二、安装与设置2.1 安装 Conda 虚拟环境2.2 安装 Python venv 虚拟环境 三、依赖管理3.1 Conda 依赖管理3.2 Python venv 依赖管理 四、适用场景五、性能与资源占用5.1 Conda 性能与资源占用5.2 Python venv 性能…...

【从0学英语】06.时态 - 一般过去时
一般过去时(Past Simple Tense)是表达过去发生的动作、状态或事实的核心时态。这一时态都扮演着不可或缺的角色,本篇文章将全面讲解一般过去时的定义、结构、用法以及常见的动词变化,通过例句和详细的解释帮你理解这一时态。 文章…...
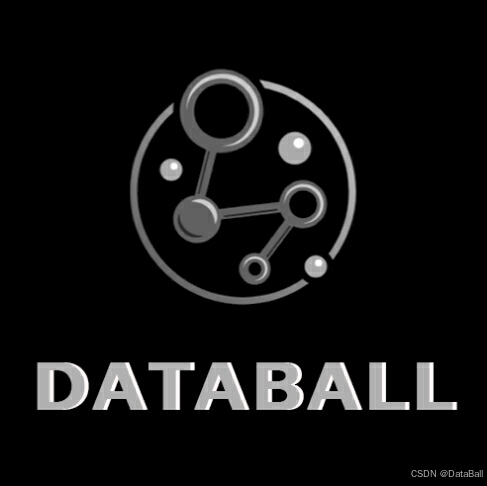
获取cpu序列号-python实现
DataBall 助力快速掌握数据集的信息和使用方式,会员享有 百种数据集,持续增加中。 需要更多数据资源和技术解决方案,知识星球: “DataBall - X 数据球(free)” -------------------------------------------------------------…...