[动手学深度学习]注意力机制Transformer学习笔记
动手学深度学习(视频):68 Transformer【动手学深度学习v2】_哔哩哔哩_bilibili
动手学深度学习(pdf):10.7. Transformer — 动手学深度学习 2.0.0 documentation (d2l.ai)
李沐Transformer论文逐段精读:Transformer论文逐段精读【论文精读】_哔哩哔哩_bilibili
Vaswani, A. et al. (2017) 'Attention is all you need', 31st Conference on Neural Information Processing Systems (NIPS 2017), Long Beach, CA, USA. doi: https://doi.org/10.48550/arXiv.1706.03762
目录
1. Transformer
1.1. 整体实现步骤
1.2. Transformer理念
1.3. Transformer代码实现
1.4. Transformer弊端和局限
2. Transformer论文原文学习
2.1. Abstract
2.2. Introduction
2.3. Background
2.4. Model Architecture
2.4.1. Encoder and Decoder Stacks
2.4.2. Attention
2.4.3. Position-wise Feed-Forward Networks
2.4.4. Embeddings and Softmax
2.4.5. Positional Encoding
2.5. Why Self-Attention
2.6. Training
2.6.1. Training Data and Batching
2.6.2. Hardware and Schedule
2.6.3. Optimizer
2.6.4. Regularization
2.7. Results
2.7.1. Machine Translation
2.7.2. Model Variations
2.7.3. English Constituency Parsing
2.8. Conclusion
1. Transformer
1.1. 整体实现步骤
(1)模型图
1.2. Transformer理念
(1)摒弃了传统费时的卷积和循环,仅采用注意力机制和全连接构成整个网络
(2)多使用残差连接
(3)Mask的提出
(4)李沐特地讲解了batch norm和layer norm的区别,主要是取的块不一样:
1.3. Transformer代码实现
(1)代码网址:GitHub - tensorflow/tensor2tensor: Library of deep learning models and datasets designed to make deep learning more accessible and accelerate ML research.
(2)李沐
①Encoder
class EncoderBlock(nn.Module):"""Transformer编码器块"""def __init__(self, key_size, query_size, value_size, num_hiddens,norm_shape, ffn_num_input, ffn_num_hiddens, num_heads,dropout, use_bias=False, **kwargs):super(EncoderBlock, self).__init__(**kwargs)self.attention = d2l.MultiHeadAttention(key_size, query_size, value_size, num_hiddens, num_heads, dropout,use_bias)self.addnorm1 = AddNorm(norm_shape, dropout)self.ffn = PositionWiseFFN(ffn_num_input, ffn_num_hiddens, num_hiddens)self.addnorm2 = AddNorm(norm_shape, dropout)def forward(self, X, valid_lens):Y = self.addnorm1(X, self.attention(X, X, X, valid_lens))return self.addnorm2(Y, self.ffn(Y))
②Decoder
class DecoderBlock(nn.Module):"""解码器中第i个块"""def __init__(self, key_size, query_size, value_size, num_hiddens,norm_shape, ffn_num_input, ffn_num_hiddens, num_heads,dropout, i, **kwargs):super(DecoderBlock, self).__init__(**kwargs)self.i = iself.attention1 = d2l.MultiHeadAttention(key_size, query_size, value_size, num_hiddens, num_heads, dropout)self.addnorm1 = AddNorm(norm_shape, dropout)self.attention2 = d2l.MultiHeadAttention(key_size, query_size, value_size, num_hiddens, num_heads, dropout)self.addnorm2 = AddNorm(norm_shape, dropout)self.ffn = PositionWiseFFN(ffn_num_input, ffn_num_hiddens,num_hiddens)self.addnorm3 = AddNorm(norm_shape, dropout)def forward(self, X, state):enc_outputs, enc_valid_lens = state[0], state[1]# 训练阶段,输出序列的所有词元都在同一时间处理,# 因此state[2][self.i]初始化为None。# 预测阶段,输出序列是通过词元一个接着一个解码的,# 因此state[2][self.i]包含着直到当前时间步第i个块解码的输出表示if state[2][self.i] is None:key_values = Xelse:key_values = torch.cat((state[2][self.i], X), axis=1)state[2][self.i] = key_valuesif self.training:batch_size, num_steps, _ = X.shape# dec_valid_lens的开头:(batch_size,num_steps),# 其中每一行是[1,2,...,num_steps]dec_valid_lens = torch.arange(1, num_steps + 1, device=X.device).repeat(batch_size, 1)else:dec_valid_lens = None# 自注意力X2 = self.attention1(X, key_values, key_values, dec_valid_lens)Y = self.addnorm1(X, X2)# 编码器-解码器注意力。# enc_outputs的开头:(batch_size,num_steps,num_hiddens)Y2 = self.attention2(Y, enc_outputs, enc_outputs, enc_valid_lens)Z = self.addnorm2(Y, Y2)return self.addnorm3(Z, self.ffn(Z)), state
1.4. Transformer弊端和局限
(1)位置信息表示弱
(2)长距离文本学习能力弱
2. Transformer论文原文学习
2.1. Abstract
(1)Bcakground: the sequence transduction model prevails with complicated convolution or recurrence.
(2)Purpose: they put forward a simple attention model without convolution or recurrence, which not only decreases the training time, but also increases the accuracy of translation
transduction n.转导
2.2. Introduction
①Previous model: RNN, LSTM, gated RNN
②RNN limits in parallel computing in that it is highly rely on previous data
③Factorization tricks and conditional computation have made great achievement nowadays. However, reseachers are still unable to break free from the constraints of sequential computation.
④Attention mechanism can ignore the position of words
eschew vt.避免;(有意地)避开;回避
2.3. Background
①Extended Neural GPU, ByteNet and ConvS2S try reducing the sequential mechanism. However, they still explode in distance computation.
②Self-attention, also named intra-attention has been used in "reading comprehension, abstractive summarization, textual entailment and learning task-independent sentence representations"
2.4. Model Architecture
①The Transformer model shows below:
Inputs are denoted by , then encode them to
. Lastly decode
to
(⭐input sequence and output sequence may not be of equal length).
②The model is auto-regressive
③The left of this figure is encode layer, and the right halves are decoder.
2.4.1. Encoder and Decoder Stacks
(1)Encoder
①N=6
②"Add" means adding x and sublayer(x), then using layer norm
(2)Decoder
①N=6
②Masked layer ensures can only see the previous input of its position
2.4.2. Attention
(1)Scaled Dot-Product Attention
①The model shows below:
where Q denotes queries and K denotes keys of dimension , V denotes values of dimension
②The function of Scaled Dot-Product Attention:
③They set as the dividend. They reckon when
increaces, additive attention outpeforms dot product attention.
④The more the dimension is, the less gradient the softmax is. Hence they scale the dot product.
(2) Multi-Head Attention
①The model shows below:
②The function of this block is:
where all the are parameter matrices. Additionally, h=8, and they set
(3)Applications of Attention in our Model
①Each position in the decoder participates in all positions in the input sequence
②Each position in the encoder can handle all positions in the previous layer of the encoder
③Mask all values on the right that have not yet appeared and represent them with (so when they participate in softmax layer, they always get 0)
2.4.3. Position-wise Feed-Forward Networks
① Function of fully connected feed-forward network:
where the input and output dimension , and the inner-layer dimension
2.4.4. Embeddings and Softmax
①The two embedding layers and the pre-softmax linear transformation are using the same weight matrix
②Table of different complexity for different layers:
where d denotes dimension, k denotes the size of convolutional kernal, n denotes length of sequence, and r is the size of the neighborhood in restricted self-attention
2.4.5. Positional Encoding
①Positional encodings:
where pos means position and i means dimension.
②They also tried learned positional embeddings, which got a similar result.
③Sinusoidal version allows longer sequence
2.5. Why Self-Attention
①Low computational complexity, parallelized computation and short path length are the advantages of self-attention
②分析了一堆时间空间复杂度吗?我不是很看得懂
2.6. Training
2.6.1. Training Data and Batching
They used WMT 2014 English-German dataset and WMT 2014 English-French dataset with 4.5 million sentence pairs and 36M sentences respectively.
2.6.2. Hardware and Schedule
By each step in 1 second, they trained 300,000 steps (about 3.5 days)
2.6.3. Optimizer
①Adam optimizer: β1 = 0.9, β2 = 0.98 and ϵ = 10−9
②Learning rate:
where warmup_steps = 4000 at the beginning
2.6.4. Regularization
①Dropout rate: 0.1
②Label smoothing value , which causes perplexity but increases accuracy and BLEU score
2.7. Results
2.7.1. Machine Translation
Comparison of accuracy with other models:
2.7.2. Model Variations
①Variations of Transformer
where (A) changes the number of attention heads, attention key, and dimension of value, (B) adjusts the size of attention key, (C) and (D) control model size and dropout rate, (E) replaces sinusoidal positional encoding by learned positional embeddings. These ablation studies have successfully demonstrated the superiority of Transformer.
2.7.3. English Constituency Parsing
①Comparison table of generalization performance:
2.8. Conclusion
①This is the first model which only based on attention mechanism
②Outperforms any other previous model
相关文章:
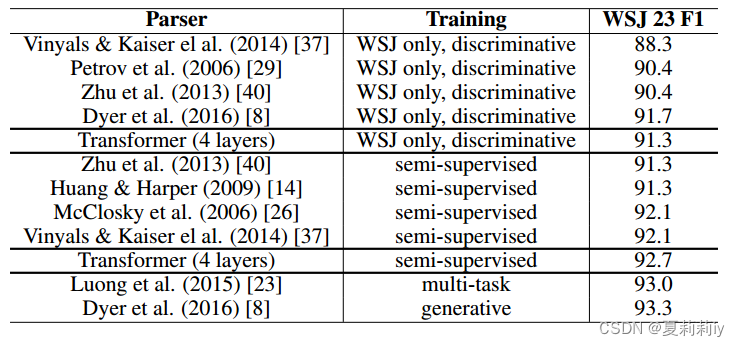
[动手学深度学习]注意力机制Transformer学习笔记
动手学深度学习(视频):68 Transformer【动手学深度学习v2】_哔哩哔哩_bilibili 动手学深度学习(pdf):10.7. Transformer — 动手学深度学习 2.0.0 documentation (d2l.ai) 李沐Transformer论文逐段精读&a…...

hadoop集群安装并配置
文章目录 1.安装JDK 环境2.系统配置2.1修改本地hosts文件2.2创建hadoop 用户2.2 设置ssh免密(使用hadoop 用户生成) 3.安装 hadoop 3.2.43.1 安装hadoop3.1.1 配置Hadoop 环境变量 3.2配置 HDFS3.2.1 配置 workers 文件3.2.2 配置hadoop-env.sh3.2.3 配置…...
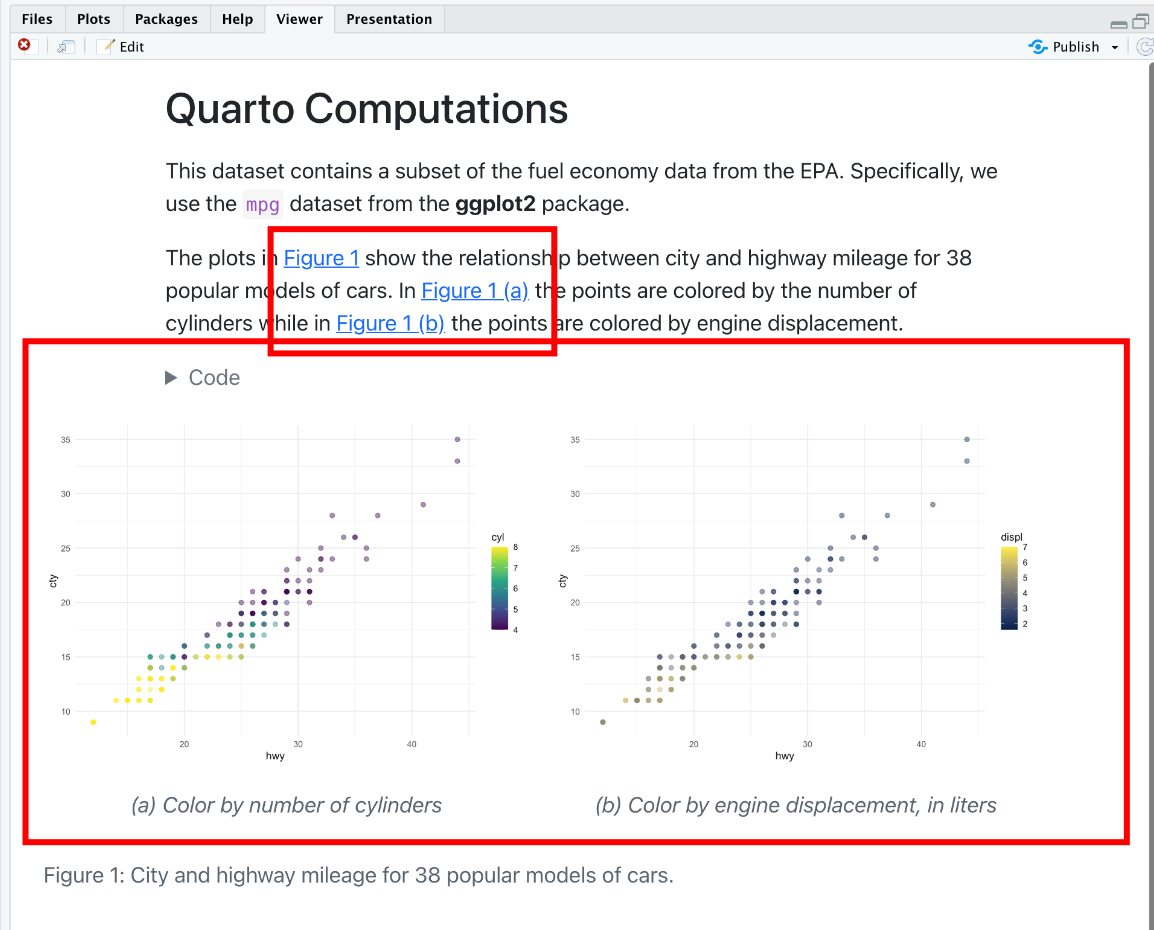
Quarto 入门教程 (3):代码框、图形、数据框设置
简介 本文是《手把手教你使用 Quarto 构建文档》第三期,前两期分别介绍了: 第一期 介绍了Quarto 构建文档的原理;可创建的文档类型;对应的参考资源分享。 第二期 介绍了如何使用 Quarto,并编译出文档(PDF…...
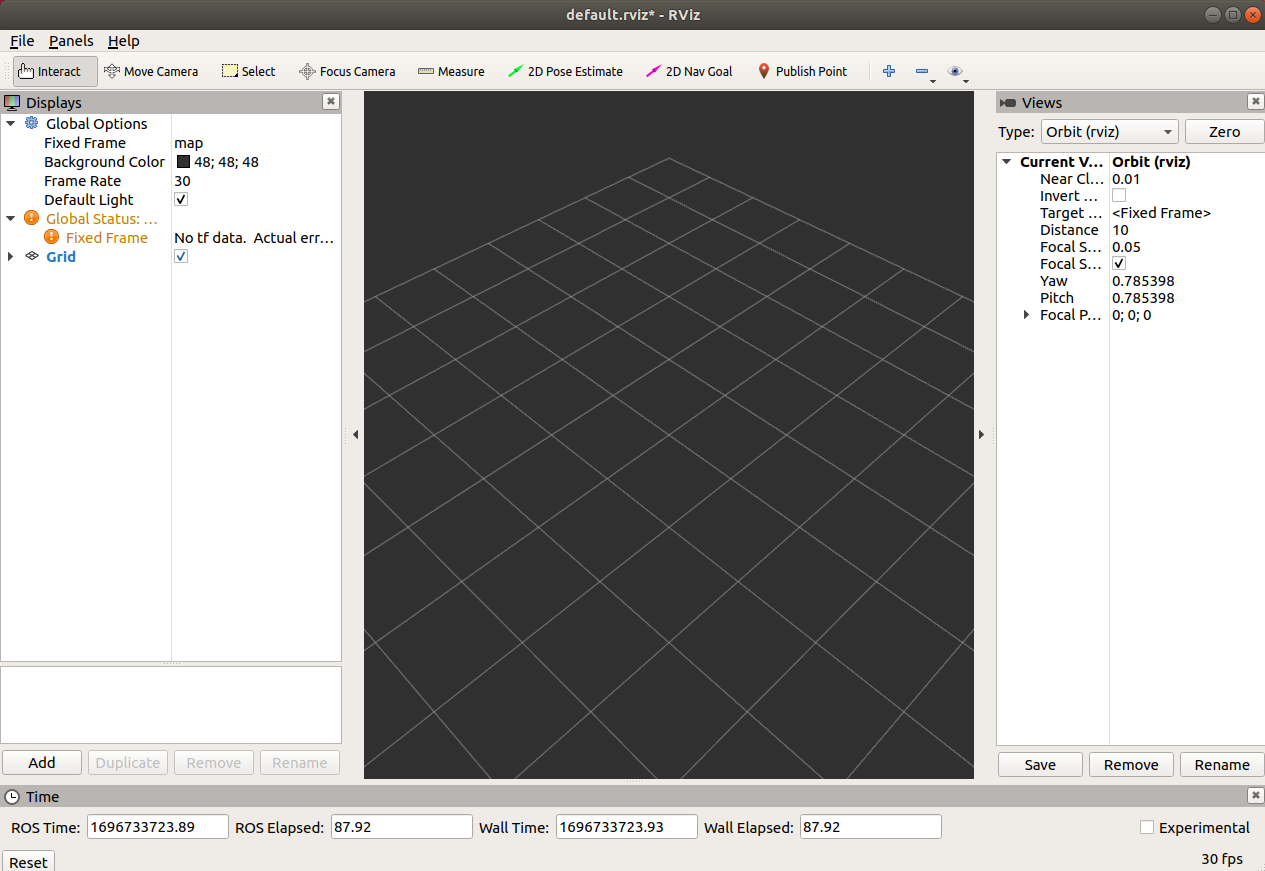
虚拟机Ubuntu18.04安装对应ROS版本详细教程!(含错误提示解决)
参考链接: Ubuntu18.04安装Ros(最新最详细亲测)_向日葵骑士Faraday的博客-CSDN博客 1.4 ROS的安装与配置_哔哩哔哩_bilibili ROS官网:http://wiki.ros.org/melodic/Installation/Ubuntu 一、检查cmake 安装ROS时会自动安装旧版的Cmake3.10.2。所以在…...

#力扣:14. 最长公共前缀@FDDLC
14. 最长公共前缀 一、Java class Solution {public String longestCommonPrefix(String[] strs) {for (int l 0; ; l) {for (int i 0; i < strs.length; i) {if (l > strs[i].length() || strs[i].charAt(l) ! strs[0].charAt(l)) return strs[0].substring(0, l);}…...

Android 13.0 解锁状态下禁止下拉状态栏功能实现
1.前言 在13.0的系统定制化开发中,在关于一些systemui下拉状态栏的定制化功能开发中,对于关于systemui的下拉状态栏 是否可以下拉做了定制,用系统属性来判断是否可以在解锁的情况下可以下拉状态栏布局,虽然11.0 12.0和13.0的关于 下拉状态栏相关分析有区别,可以通过分析相…...
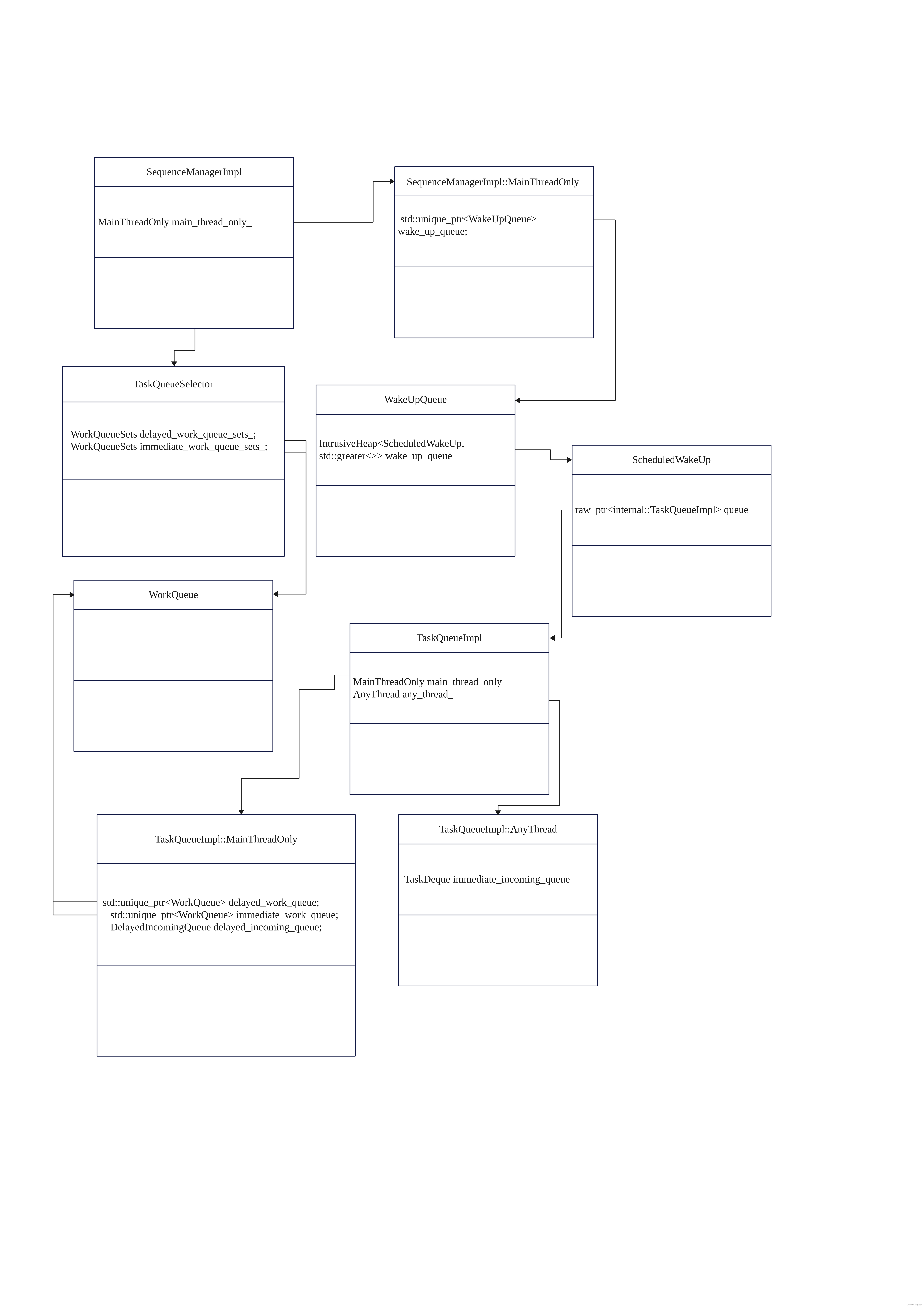
chromium线程模型(1)-普通线程实现(ui和io线程)
通过chromium 官方文档,线程和任务一节我们可以知道 ,chromium有两类线程,一类是普通线程,最典型的就是io线程和ui线程。 另一类是 线程池线程。 今天我们先分析普通线程的实现,下一篇文章分析线程池的实现。ÿ…...
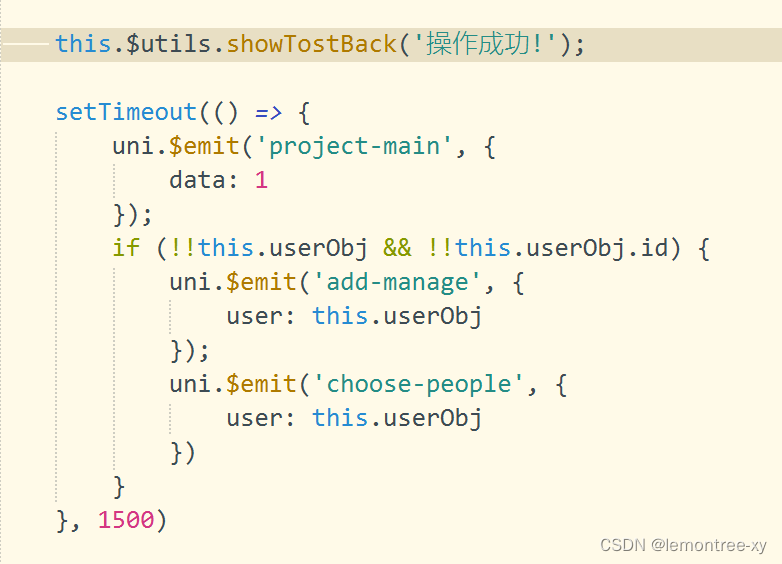
uniapp uni.showToast 一闪而过的问题
问题:在页面跳转uni.navigateBack()等操作的前或后,执行uni.showToast,即使代码中设置2000ms的显示时间,也会一闪而过。 解决:用setTimeout延后navigateBack的执行。...

代理模式介绍及具体实现(设计模式 三)
代理模式是一种结构型设计模式,它允许通过创建一个代理对象来控制对另一个对象的访问 实例介绍和实现过程 假设我们正在开发一个电子商务网站,其中有一个商品库存管理系统。我们希望在每次查询商品库存之前,先进行权限验证,以确…...

【18】c++设计模式——>适配器模式
c的适配器模式是一种结构型设计模式,他允许将一个类的接口转换成另一个客户端所期望的接口。适配器模式常用于已存在的,但不符合新需求或者规范的类的适配。 在c中实现适配器模式时,通常需要一下几个组件: 1.目标接口(…...
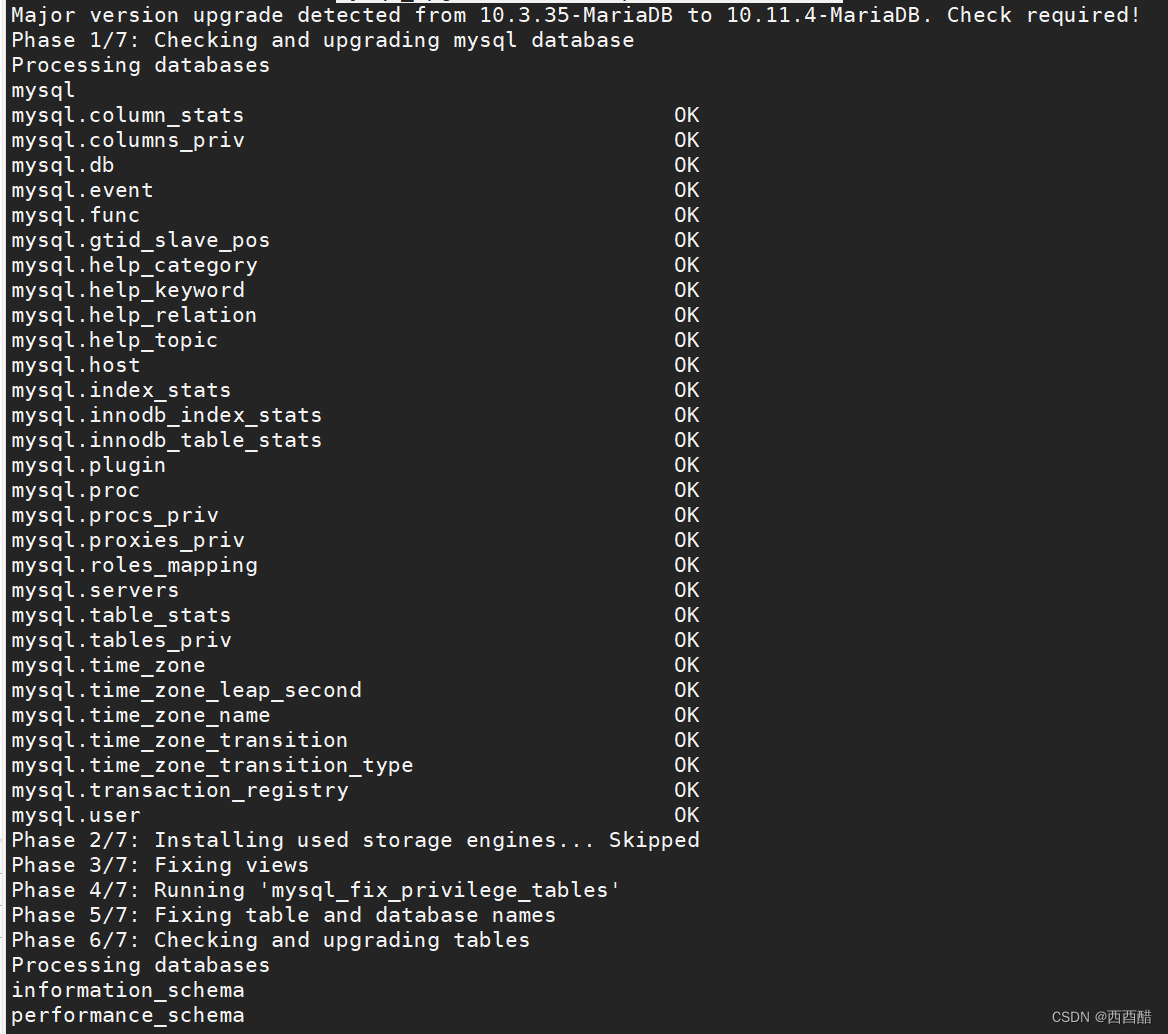
mariadb 错误日志中报错:Incorrect definition of table mysql.column_stats:
数据库错误日志出现此错误原因是因为系统表中字段类型或者数据结构有变动导致,一般是因为升级数据库版本后未同步升级系统表结构。 解决方法: 1.如果错误日志过大,直接删除。 2.执行 mysql_upgrade -u[用户名] -p[密码];,这一步…...
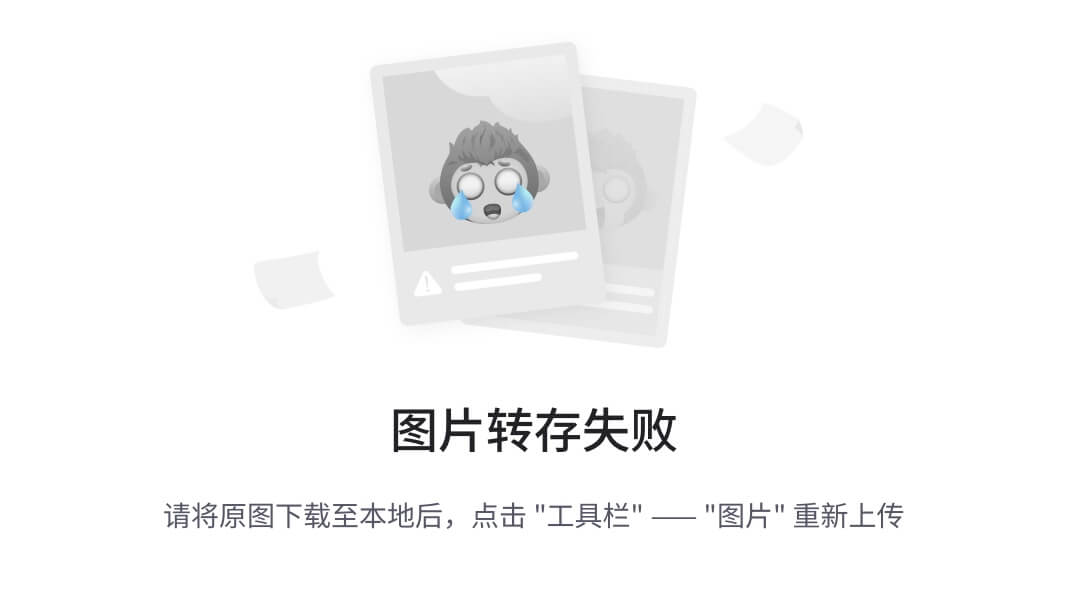
【SpringBoot】多环境配置和启动
环境分类,可以分为 本地环境、测试环境、生产环境等,通过对不同环境配置内容,来实现对不同环境做不同的事情。 SpringBoot 项目,通过 application-xxx.yml 添加不同的后缀来区分配置文件,启动时候通过后缀启动即可。 …...

跨qml通信
****Commet.qml //加载其他文件中的组件 不需要声明称Component //1.用loader.item.属性 访问属性 //2.loader.item.方法 访问方法 //3.用loader.item.方法.connect(槽)连接信号 Item { Loader{ id:loader; width: 200; …...
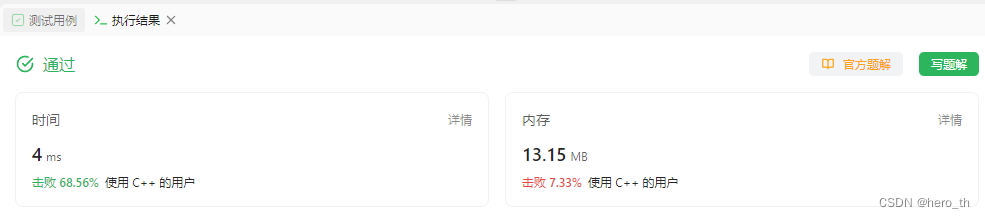
力扣-404.左叶子之和
Idea attention:先看清楚题目,题目说的是左叶子结点,不是左结点【泣不成声】 遇到像这种二叉树类型的题目呢,我们一般还是选择dfs,然后类似于前序遍历的方式加上判断条件即可 AC Code class Solution { public:void d…...
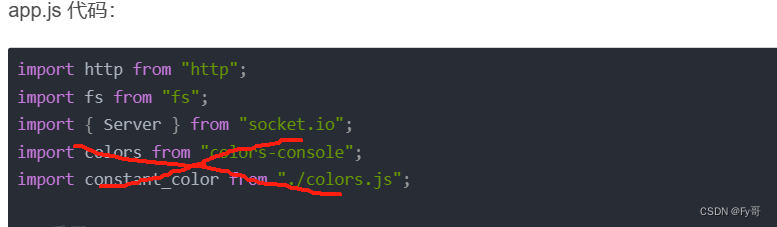
如何搭建一个 websocket
环境: NodeJssocket.io 4.7.2 安装依赖 yarn add socket.io创建服务器 引入文件 特别注意: 涉及到 colors 的代码,请采取 console.log() 打印 // 基础老三样 import http from "http"; import fs from "fs"; import { Server } from &quo…...
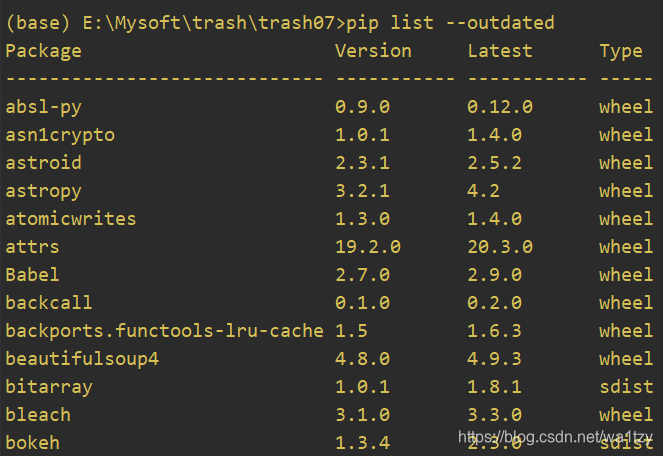
pip常用命令
TOC(pip常用命令) 1.pip 2.where pip 3.pip install --upgrade pip 4.安装 这里暂用flask库举例,安装flask库,默认安装最新版: pip install flask指定要安装flask库的版本: pip install flask版本号我们在安装第三方库时可…...
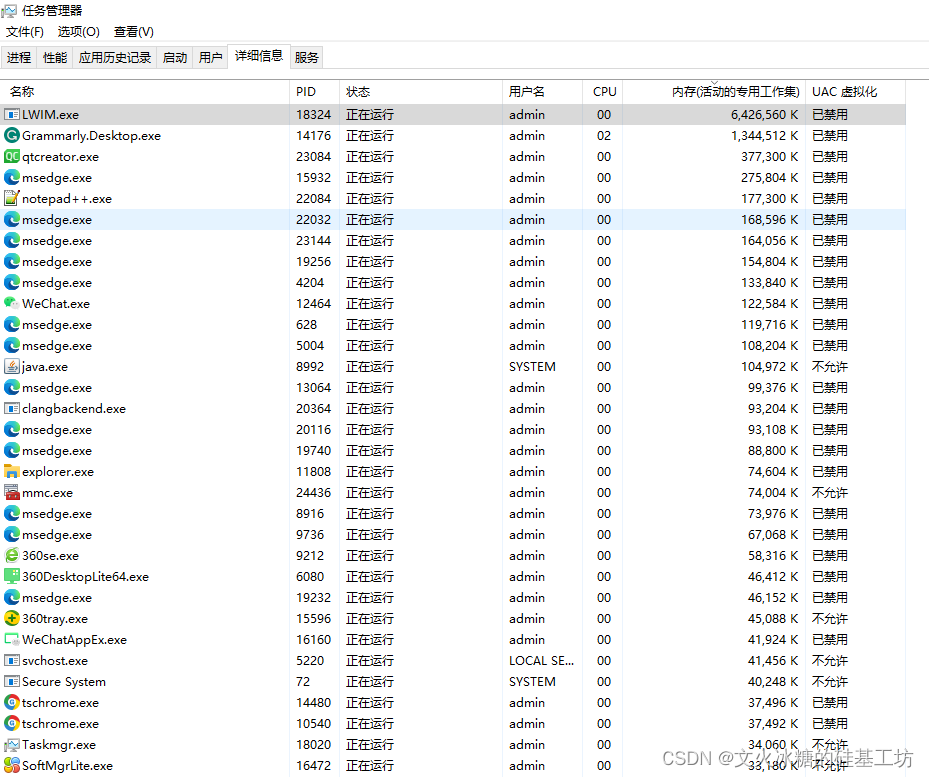
[QT编程系列-43]: Windows + QT软件内存泄露的检测方法
目录 一、如何查找Windows程序是否有内存泄露 二、如何定位Windows程序内存泄露的原因 二、Windows环境下内存监控工具的使用 2.1 内存监测工具 - Valgrind 2.2.1 Valgrind for Linux 2.2.2 Valgrind for Windows 2.2 内存监测工具 - Dr. Memory 2.2.1 特点 2.2.2 安装…...

【Java-LangChain:使用 ChatGPT API 搭建系统-5】处理输入-思维链推理
第五章,处理输入-思维链推理 在本章中,我们将专注于处理输入,即通过一系列步骤生成有用地输出。 有时,模型在回答特定问题之前需要进行详细地推理。如果您参加过我们之前的课程,您将看到许多这样的例子。有时…...
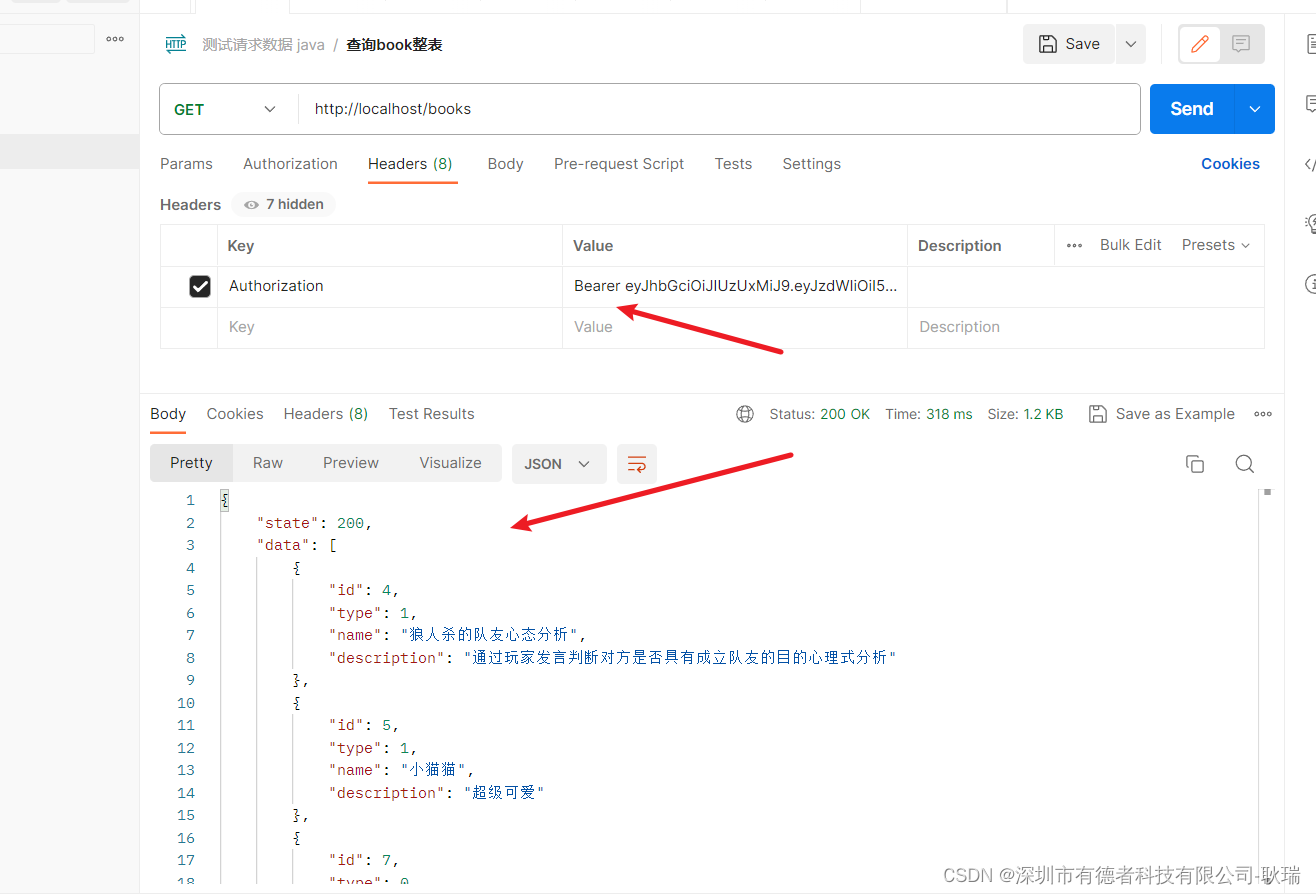
java Spring Boot RequestHeader设置请求头,当请求头中没有Authorization 直接400问题解决
我在接口中 写了一个接收请求头参数 Authorization 但是目前代理一个问题 那就是 当请求时 请求头中没有这个Authorization 就会直接因为参数不匹配 找不到指向 这里其实很简单 我们设置 value 为我们需要的字段内容 required 是否必填 我们设置为 false 就可以了 这样 没有也…...
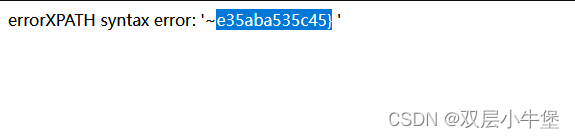
[CISCN2019 华北赛区 Day1 Web5]CyberPunk 二次报错注入
buu上 做点 首先就是打开环境 开始信息收集 发现源代码中存在?file 提示我们多半是包含 我原本去试了试 ../../etc/passwd 失败了 直接伪协议上吧 php://filter/readconvert.base64-encode/resourceindex.phpconfirm.phpsearch.phpchange.phpdelete.php 我们通过伪协议全…...
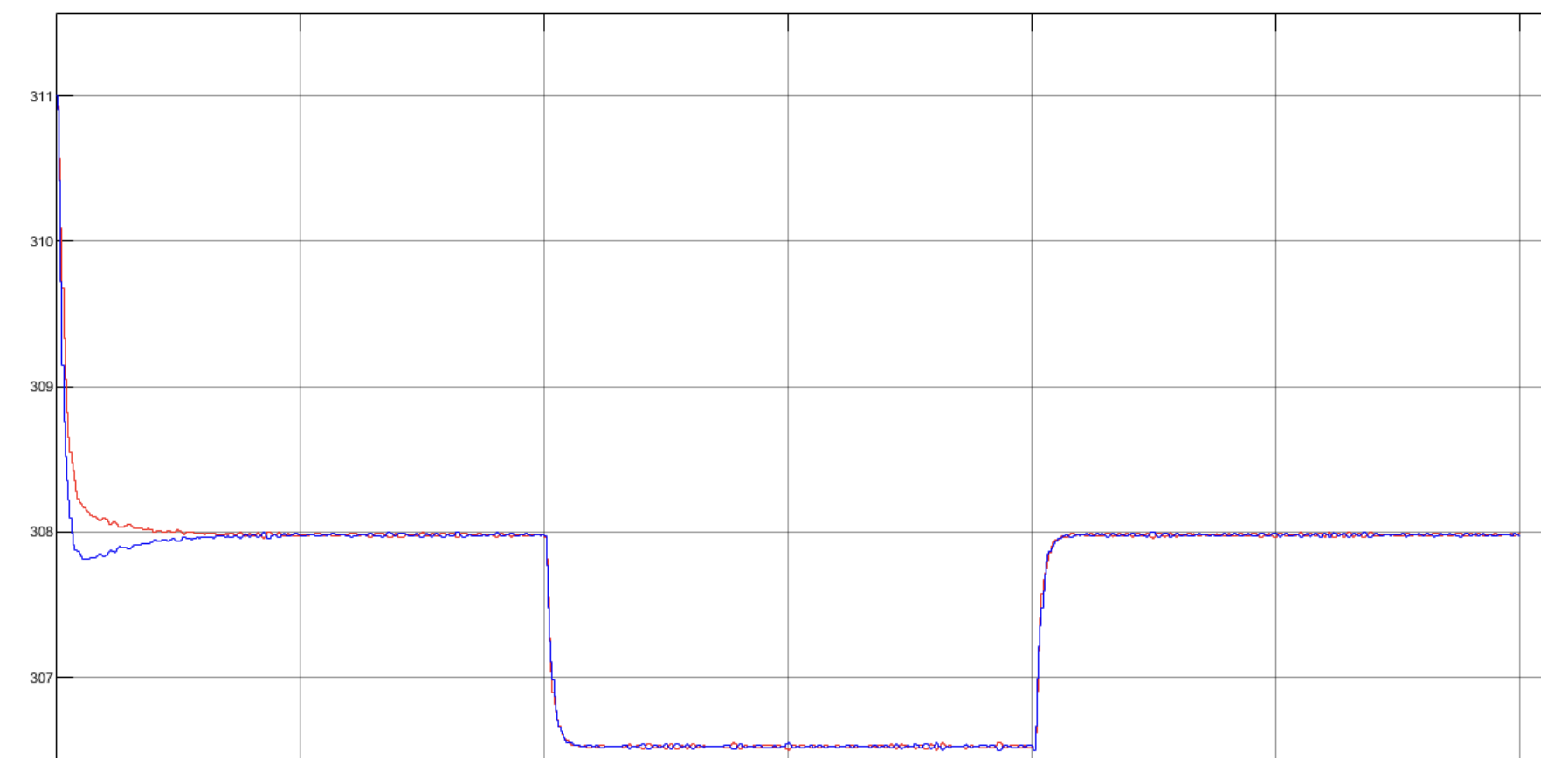
双机并联逆变器自适应虚拟阻抗下垂控制(Droop)策略Simulink仿真模型
💥💥💞💞欢迎来到本博客❤️❤️💥💥 🏆博主优势:🌞🌞🌞博客内容尽量做到思维缜密,逻辑清晰,为了方便读者。 ⛳️座右铭&a…...

8月份,誉天79名学员通过HCIE认证!
八月份,誉天又有79名学员顺利通过了HCIE认证,其中:云计算46人、Datacom14人、云服务8人、存储7人、安全3人、大数据1人。一起祝贺他们吧~...
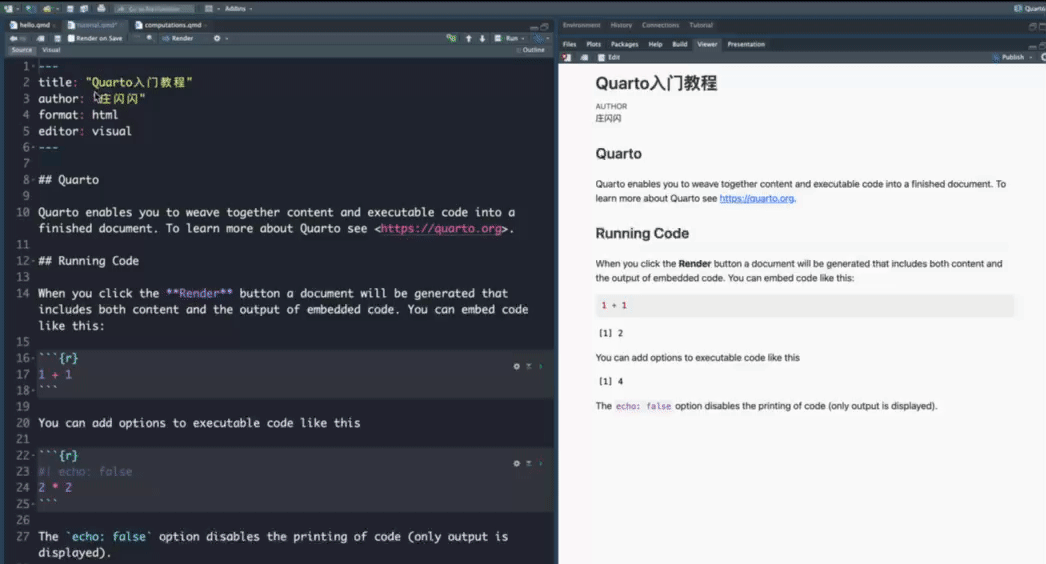
Quarto 入门教程 (2):如何使用并编译出不同文档
接着上一期内容:手把手教你使用 Quarto 构建文档 (1),本文介绍如何使用 Quarto,并编译出文档(PDF,MS Word,html)等。 安装 根据官方链接,选择适合自己电脑的 Quarto 版本并下载&am…...
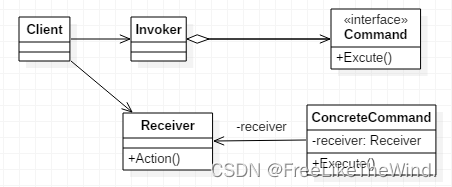
【C++设计模式之命令模式:行为型】分析及示例
简介 命令模式是一种行为型设计模式,它将请求封装成一个对象,从而使不同的请求可以被参数化、队列化或记录化。这种模式允许请求的发送者和接收者进行解耦,同时提供更高的灵活性和可扩展性。 描述 命令模式的核心思想是通过命令对象来封装…...
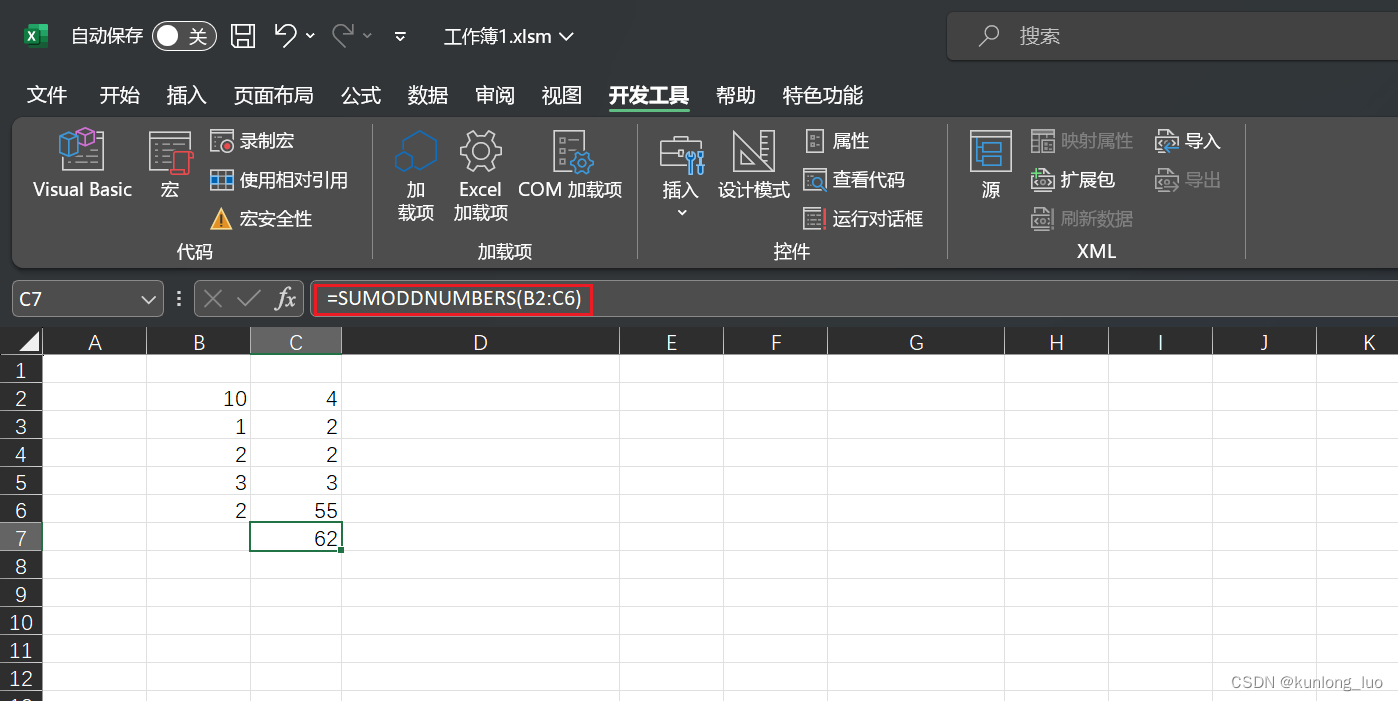
二、Excel VBA 简单使用
Excel VBA 从入门到出门一、Excel VBA 是个啥?二、Excel VBA 简单使用 👋Excel VBA 简单使用 ⚽️1. 如何在Excel中手动编写VBA代码⚽️2. 如何在 Excel 中运行 VBA 代码⚽️3. 如何在Excel中记录VBA代码⚽️4. 如何在Excel中编辑录制的VBA代码⚽️5. 如…...

获取url后面的参数
方式一 final String queryString request.getQueryString(); System.out.println(queryString); //解码 System.out.println(URLDecoder.decode(queryString, StandardCharsets.UTF_8));测试: pageSize25&pageNum1&sort%5B%27id%27%5Ddesc&sort%5B%…...
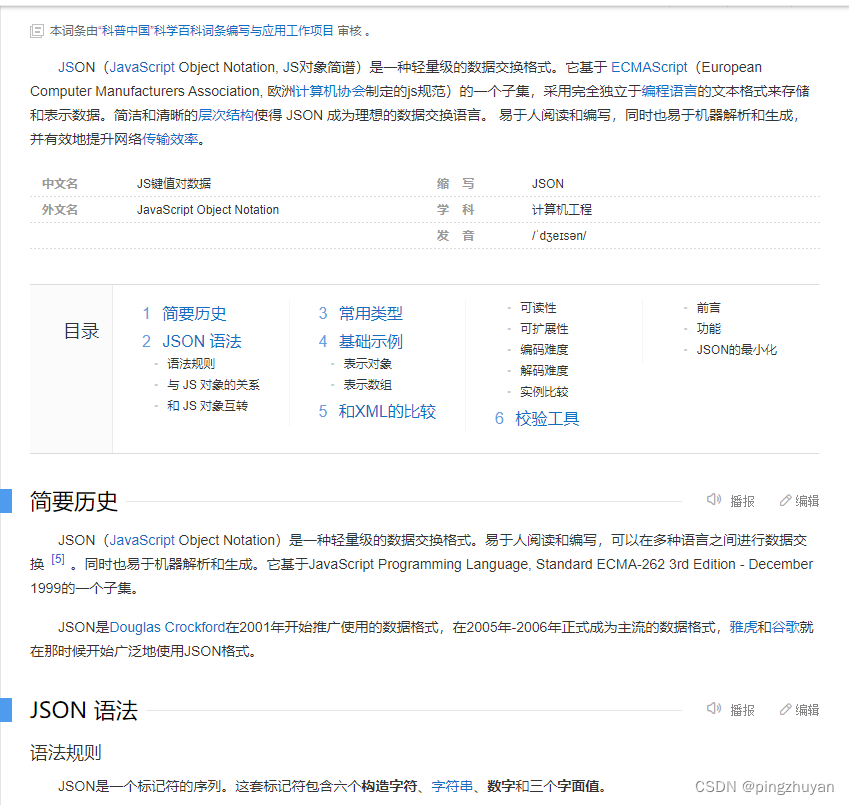
python入门篇07-数据容器(序列 集合 字典,json初识)基础(下)
全文目录,一步到位 1.前言简介1.1 专栏传送门1.1.1 上文传送门 2. python基础使用2.1 序列2.1.1 序列定义2.1.2 序列参数解释2.1.3 列表list切片2.1.4 元组tuple切片2.1.5 字符串str切片 2.2 集合定义2.2.1 set集合-基本语法2.2.2 set集合-添加元素.add()2.2.3 set集合- 移除元…...
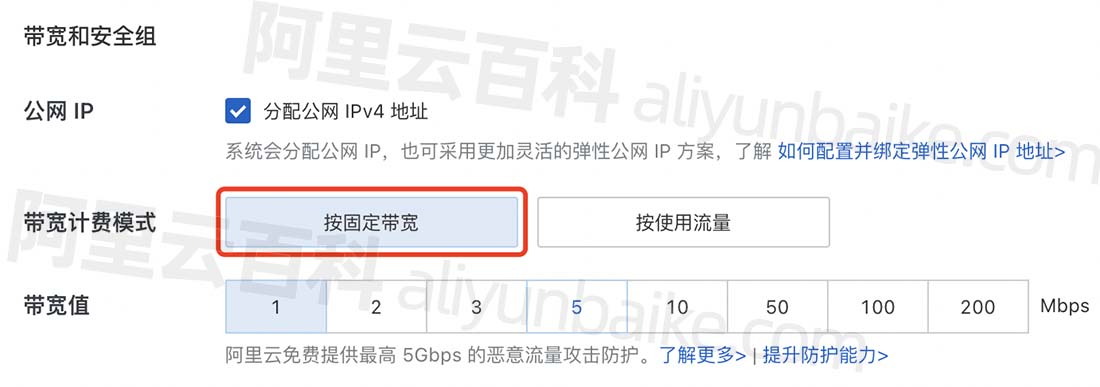
阿里云服务器公网带宽价格表“按固定带宽”计费模式
阿里云服务器公网带宽计费模式按固定带宽”计费多少钱1M?地域不同带宽价格不同,北京、杭州、深圳等大陆地域价格是23元/Mbps每月,中国香港1M带宽价格是30元一个月,美国硅谷是30元一个月,日本东京1M带宽是25元一个月&am…...

MTK的充电方案—PMIC充电
MTK目前有三套充电方案,主要如下: (1)线性充电——linering charge(PMIC充电) 充9停1,充电电流不能太大,目前最大能做到1.2A; (2)switch charge(…...
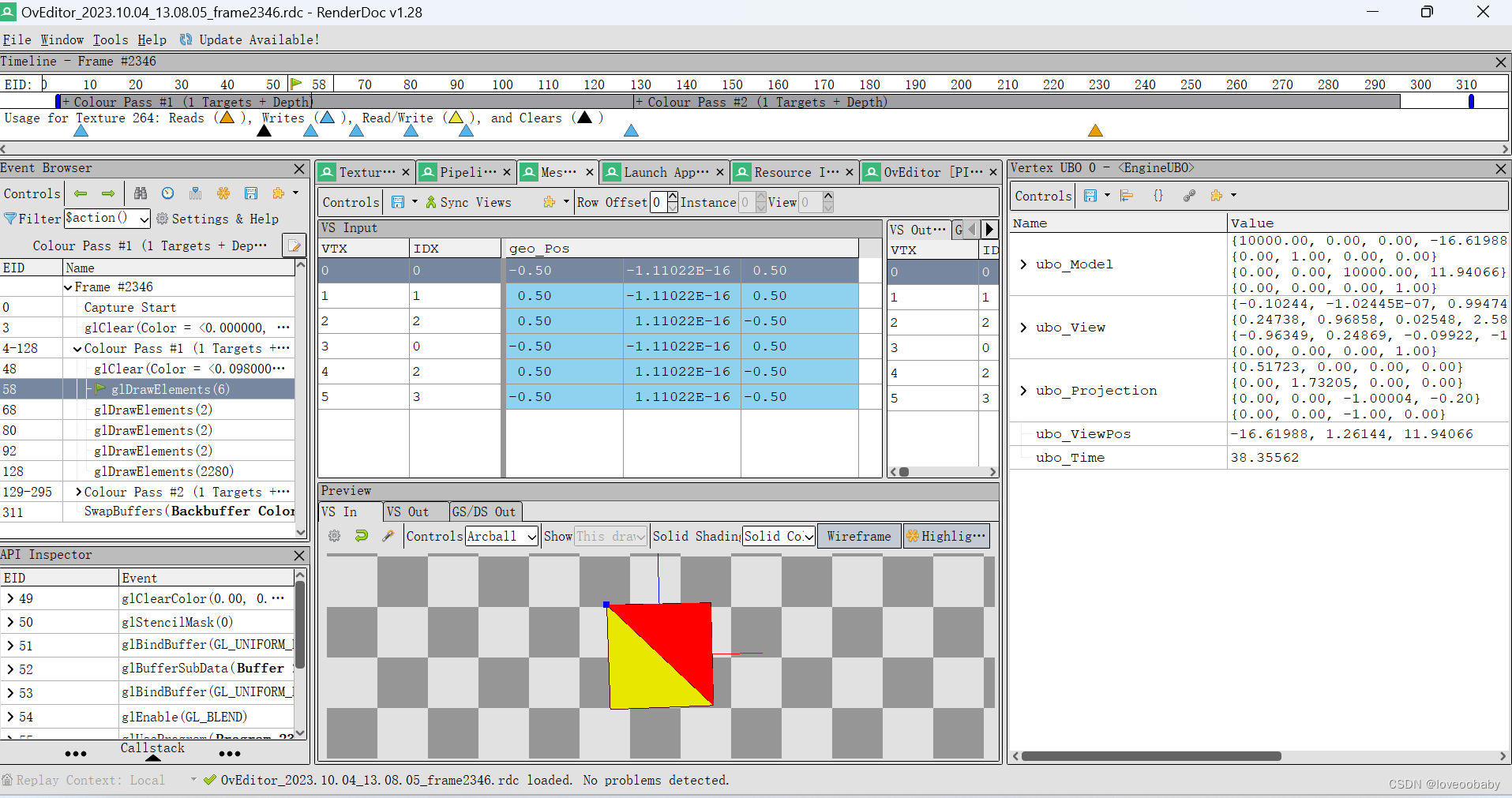
【Overload游戏引擎分析】画场景栅格的Shader分析
Overload引擎地址: GitHub - adriengivry/Overload: 3D Game engine with editor 一、栅格绘制基本原理 Overload Editor启动之后,场景视图中有栅格线,这个在很多软件中都有。刚开始我猜测它应该是通过绘制线实现的。阅读代码发现࿰…...