Constitutional AI
用中文以结构树的方式列出这篇讲稿的知识点: Although you can use a reward model to eliminate the need for human evaluation during RLHF fine tuning, the human effort required to produce the trained reward model in the first place is huge. The labeled data set used to train the reward model typically requires large teams of labelers, sometimes many thousands of people to evaluate many prompts each. This work requires a lot of time and other resources which can be important limiting factors. As the number of models and use cases increases, human effort becomes a limited resource. Methods to scale human feedback are an active area of research. One idea to overcome these limitations is to scale through model self supervision. Constitutional AI is one approach of scale supervision. First proposed in 2022 by researchers at Anthropic, Constitutional AI is a method for training models using a set of rules and principles that govern the model's behavior. Together with a set of sample prompts, these form the constitution. You then train the model to self critique and revise its responses to comply with those principles. Constitutional AI is useful not only for scaling feedback, it can also help address some unintended consequences of RLHF. For example, depending on how the prompt is structured, an aligned model may end up revealing harmful information as it tries to provide the most helpful response it can. As an example, imagine you ask the model to give you instructions on how to hack your neighbor's WiFi. Because this model has been aligned to prioritize helpfulness, it actually tells you about an app that lets you do this, even though this activity is illegal. Providing the model with a set of constitutional principles can help the model balance these competing interests and minimize the harm. Here are some example rules from the research paper that Constitutional AI I asks LLMs to follow. For example, you can tell the model to choose the response that is the most helpful, honest, and harmless. But you can play some bounds on this, asking the model to prioritize harmlessness by assessing whether it's response encourages illegal, unethical, or immoral activity. Note that you don't have to use the rules from the paper, you can define your own set of rules that is best suited for your domain and use case. When implementing the Constitutional AI method, you train your model in two distinct phases. In the first stage, you carry out supervised learning, to start your prompt the model in ways that try to get it to generate harmful responses, this process is called red teaming. You then ask the model to critique its own harmful responses according to the constitutional principles and revise them to comply with those rules. Once done, you'll fine-tune the model using the pairs of red team prompts and the revised constitutional responses. Let's look at an example of how one of these prompt completion pairs is generated. Let's return to the WiFi hacking problem. As you saw earlier, this model gives you a harmful response as it tries to maximize its helpfulness. To mitigate this, you augment the prompt using the harmful completion and a set of predefined instructions that ask the model to critique its response. Using the rules outlined in the Constitution, the model detects the problems in its response. In this case, it correctly acknowledges that hacking into someone's WiFi is illegal. Lastly, you put all the parts together and ask the model to write a new response that removes all of the harmful or illegal content. The model generates a new answer that puts the constitutional principles into practice and does not include the reference to the illegal app. The original red team prompt, and this final constitutional response can then be used as training data. You'll build up a data set of many examples like this to create a fine-tuned NLM that has learned how to generate constitutional responses. The second part of the process performs reinforcement learning. This stage is similar to RLHF, except that instead of human feedback, we now use feedback generated by a model. This is sometimes referred to as reinforcement learning from AI feedback or RLAIF. Here you use the fine-tuned model from the previous step to generate a set of responses to your prompt. You then ask the model which of the responses is preferred according to the constitutional principles. The result is a model generated preference dataset that you can use to train a reward model. With this reward model, you can now fine-tune your model further using a reinforcement learning algorithm like PPO, as discussed earlier. Aligning models is a very important topic and an active area of research. The foundations of RLHF that you've explored in this lesson will allow you to follow along as the field evolves. I'm really excited to see what new discoveries researchers make in this area. I encourage you to keep an eye out for any new methods and best practices that emerge in the coming months and years
- RLHF
- Use of reward model to eliminate need for human evaluation
- Large human effort required to produce trained reward model
- Large teams of labelers needed for labeled data set used to train reward model
- Human effort becomes limited resource as number of models and use cases increases
- Methods to scale human feedback an active area of research
- Large human effort required to produce trained reward model
- Constitutional AI as approach to scale through model self supervision
- Method for training models using set of rules and principles that govern model's behavior and form constitution
- Train model to self critique and revise responses to comply with principles
- Can help address unintended consequences of RLHF, such as revealing harmful information
- Example constitutional principles/rules:
- Choose most helpful, honest, and harmless response
- Prioritize harmlessness by assessing whether response encourages illegal, unethical, or immoral activity
- Can define own set of rules suited for domain/use case
- Train model using two distinct phases:
- Supervised learning to generate harmful responses and critique and revise them according to constitutional principles (red teaming)
- Reinforcement learning using feedback generated by model to train reward model
- Use of reward model to eliminate need for human evaluation
- Fine-tuned NLM
- Reinforcement learning algorithms (PPO)
- 深度强化学习 (Deep Reinforcement Learning)
- 奖励模型 (Reward Model)
- 人工评估 (Human Evaluation)
- 训练奖励模型的数据集 (Labeled Dataset)
- 大规模标签队伍 (Large Teams of Labelers)
- 自我监督 (Self Supervision)
- 宪法型人工智能 (Constitutional AI)
- 宪法中的规则和原则 (Rules and Principles in the Constitution)
- RLHF的意外后果 (Unintended Consequences of RLHF)
- 宪法中的规则示例 (Example Rules in the Constitution)
- 监督学习 (Supervised Learning)
- 红队测试 (Red Teaming)
- 奖励模型的训练 (Training of Reward Model)
- 强化学习 (Reinforcement Learning)
- AI反馈的强化学习 (RLAIF)
- 使用奖励模型消除RLHF微调过程中人工评估的需求
- 为训练奖励模型需要大量的人力资源
- 通过模型自我监督来扩展人类反馈的方法
- 宪法AI是一种扩展反馈的方法,通过一组规则和原则来训练模型的行为
- 使用宪法AI能够避免RLHF的一些意外后果
- 宪法AI的规则可以根据领域和用例的需要进行定义和调整
- 使用宪法AI的方法进行训练分为两个阶段:第一阶段进行有监督学习,第二阶段进行强化学习
- 在强化学习阶段,使用奖励模型进行模型反馈,称为RLAIF
- 定期关注领域内新的方法和最佳实践
相关文章:
Constitutional AI
用中文以结构树的方式列出这篇讲稿的知识点: Although you can use a reward model to eliminate the need for human evaluation during RLHF fine tuning, the human effort required to produce the trained reward model in the first place is huge. The label…...
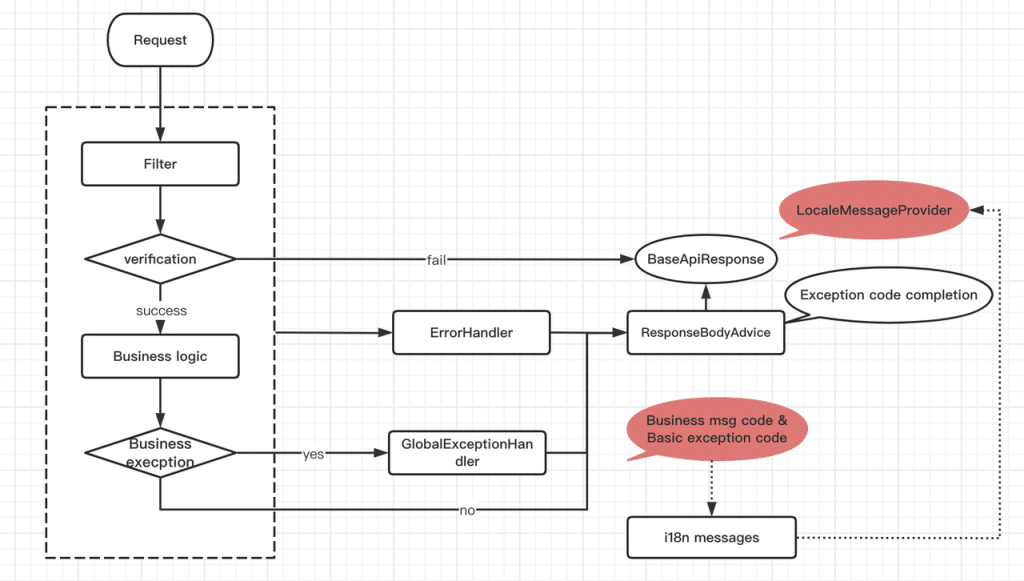
TDengine 资深研发整理:基于 SpringBoot 多语言实现 API 返回消息国际化
作为一款在 Java 开发社区中广受欢迎的技术框架,SpringBoot 在开发者和企业的具体实践中应用广泛。具体来说,它是一个用于构建基于 Java 的 Web 应用程序和微服务的框架,通过简化开发流程、提供约定大于配置的原则以及集成大量常用库和组件&a…...
数据结构-冒泡排序Java实现
目录 一、引言二、算法步骤三、原理演示四、代码实战五、结论 一、引言 冒泡排序是一种基础的比较排序算法,它的思想很简单:重复地遍历待排序的元素列表,比较相邻元素,如果它们的顺序不正确,则交换它们。这个过程不断重…...
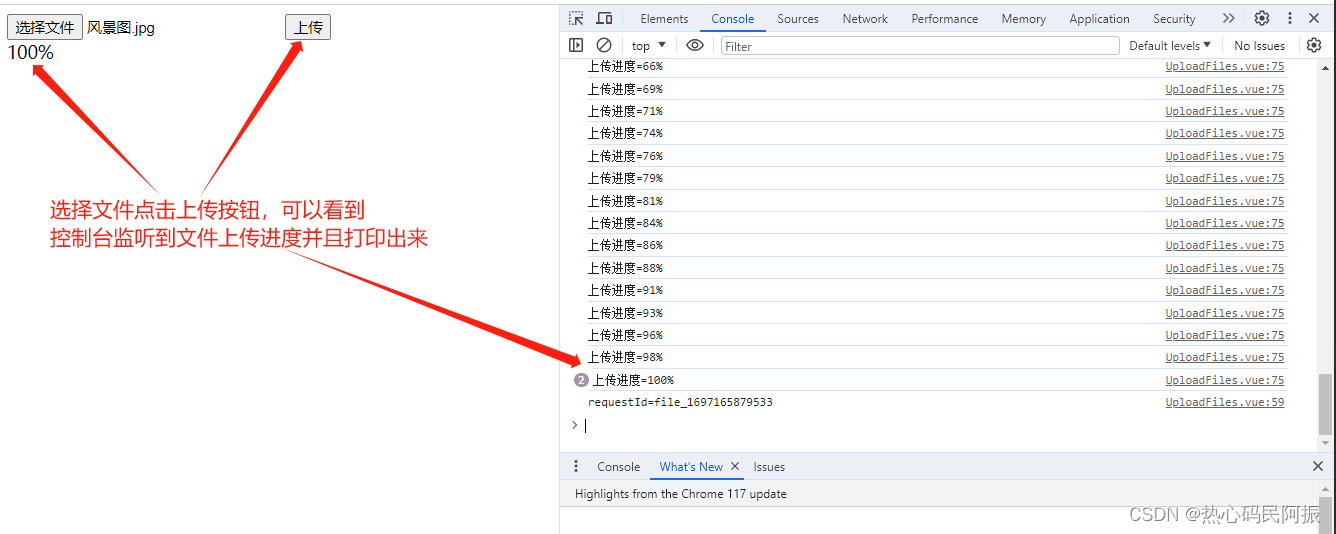
完整教程:Java+Vue+Websocket实现OSS文件上传进度条功能
引言 文件上传是Web应用开发中常见的需求之一,而实时显示文件上传的进度条可以提升用户体验。本教程将介绍如何使用Java后端和Vue前端实现文件上传进度条功能,借助阿里云的OSS服务进行文件上传。 技术栈 后端:Java、Spring Boot 、WebSock…...

【微服务 SpringCloud】实用篇 · 服务拆分和远程调用
微服务(2) 文章目录 微服务(2)1. 服务拆分原则2. 服务拆分示例1.2.1 导入demo工程1.2.2 导入Sql语句 3. 实现远程调用案例1.3.1 案例需求:1.3.2 注册RestTemplate1.3.3 实现远程调用1.3.4 查看效果 4. 提供者与消费者 …...

Linux 下I/O操作
一、文件IO 文件 IO 是 Linux 系统提供的接口,针对文件和磁盘进行操作,不带缓存机制;标准IO是C 语言函数库里的标准 I/O 模型,在 stdio.h 中定义,通过缓冲区操作文件,带缓存机制。 标准 IO 和文件 IO 常…...
C#内映射lua表
都是通过同一个方法得到的 例如得到List List<int> list LuaMgr.GetInstance().Global.Get<List<int>>("testList"); 只要把Get的泛型换成对应的类型即可 得到Dictionnary Dictionary<string, int> dic2 LuaMgr.GetInstance().Global…...
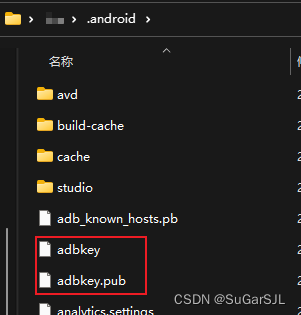
android studio检测不到真机
我的情况是: 以前能检测到,有一天我使用无线调试,发现调试有问题,想改为USB调试,但是半天没反应,我就点了手机上的撤销USB调试授权,然后就G了。 解决办法: 我这个情况比较简单&…...
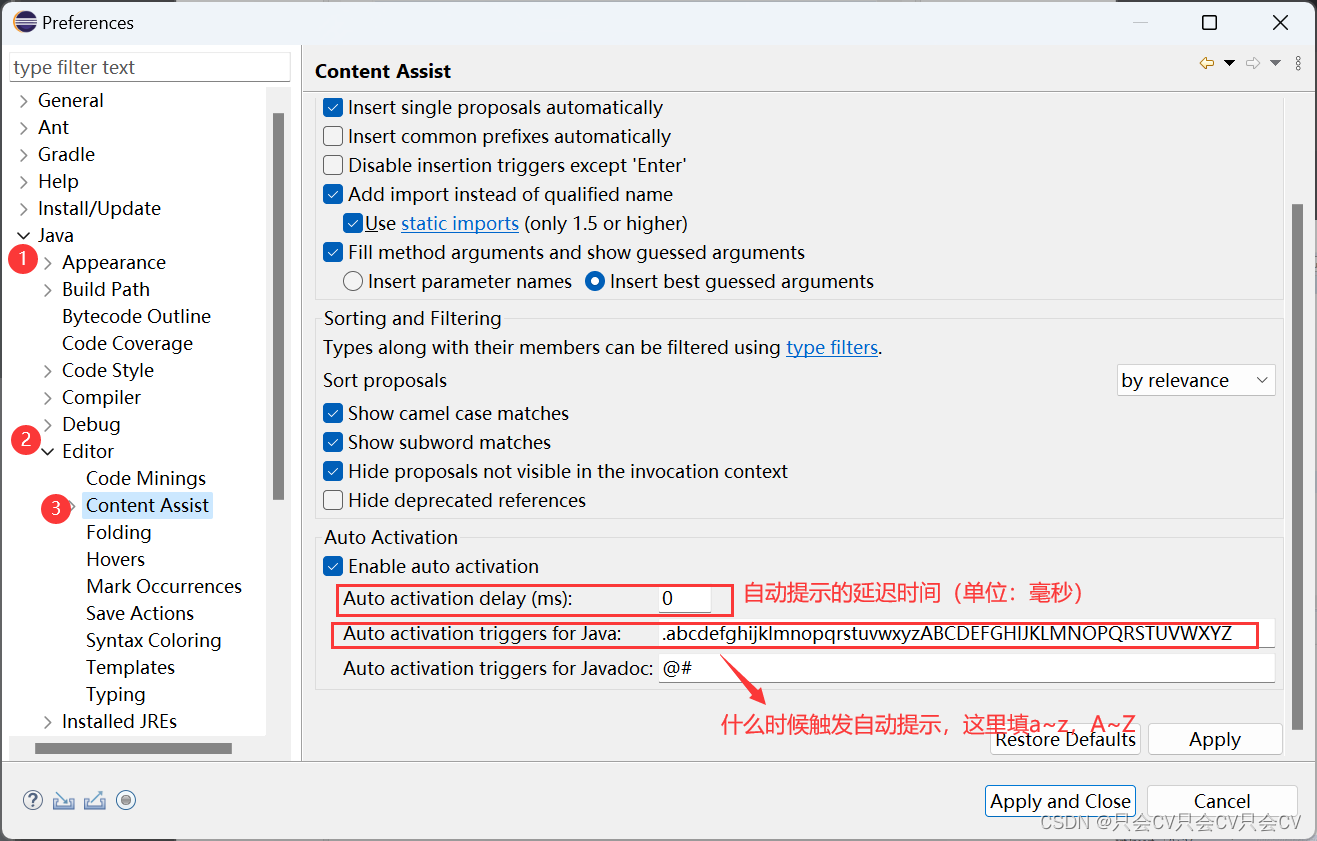
【Eclipse】设置自动提示
前言: eclipse默认有个快捷键:alt /就可以弹出自动提示,但是这样也太麻烦啦!每次都需要手动按这个快捷键,下面给大家介绍的是:如何设置敲的过程中就会出现自动提示的教程! 先按路线找到需要的页…...

单片机TDL的功能、应用与技术特点 | 百能云芯
在现代电子领域中,单片机(Microcontroller)是一种至关重要的电子元件,广泛应用于各种应用中。TDL(Time Division Multiplexing,时分多路复用)是一种数据传输技术,结合单片机的应用&a…...
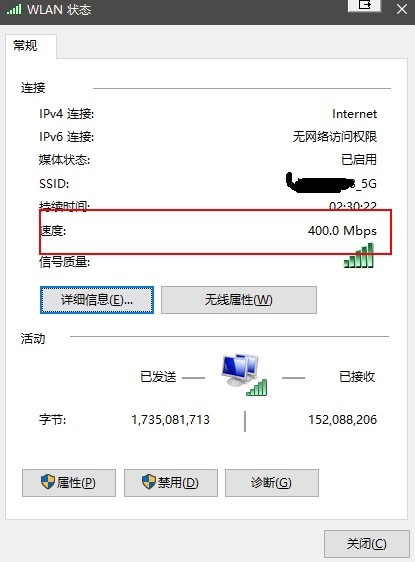
解决笔记本无线网络5G比2.4还慢的奇怪问题
环境:笔记本Dell XPS15 9570,内置无线网卡Killer Wireless-n/a/ac 1535 Wireless Network Adapter,系统win10家庭版,路由器H3C Magic R2Pro千兆版 因为笔记本用的不多,一直没怎么注意网络速度,直到最近因为…...
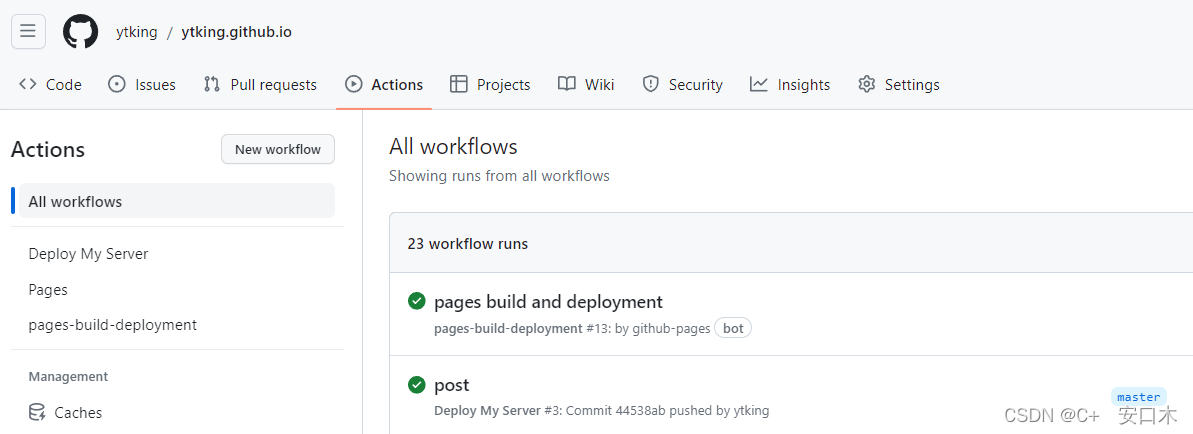
GitHub Action 通过SSH 自动部署到云服务器上
准备 正式开始之前,你需要掌握 GitHub Action 的基础语法: workflow (工作流程):持续集成一次运行的过程,就是一个 workflow。name: 工作流的名称。on: 指定次工作流的触发器。push 表示只要有人将更改推…...
【AOP系列】7.数据校验
在Java中,我们可以使用Spring AOP(面向切面编程)和自定义注解来做数据校验。以下是一个简单的示例: 首先,我们创建一个自定义注解,用于标记需要进行数据校验的方法: import java.lang.annotat…...
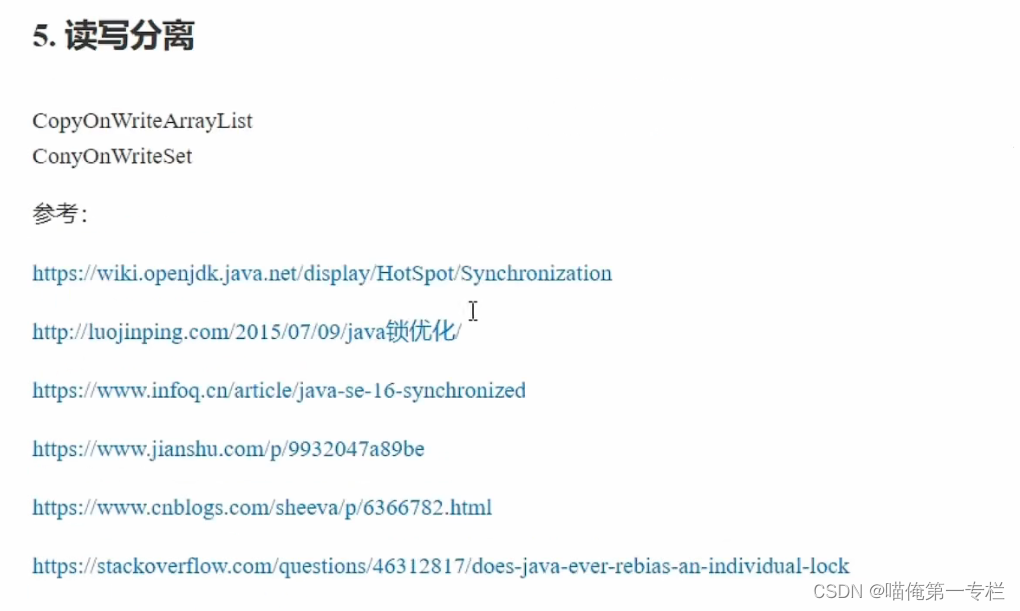
黑马JVM总结(三十七)
(1)synchronized-轻量级锁-无竞争 (2)synchronized-轻量级锁-锁膨胀 重量级锁就是我们前面介绍过的Monitor enter (3)synchronized-重量级锁-自旋 (4)synchronized-偏向锁 轻量级锁…...
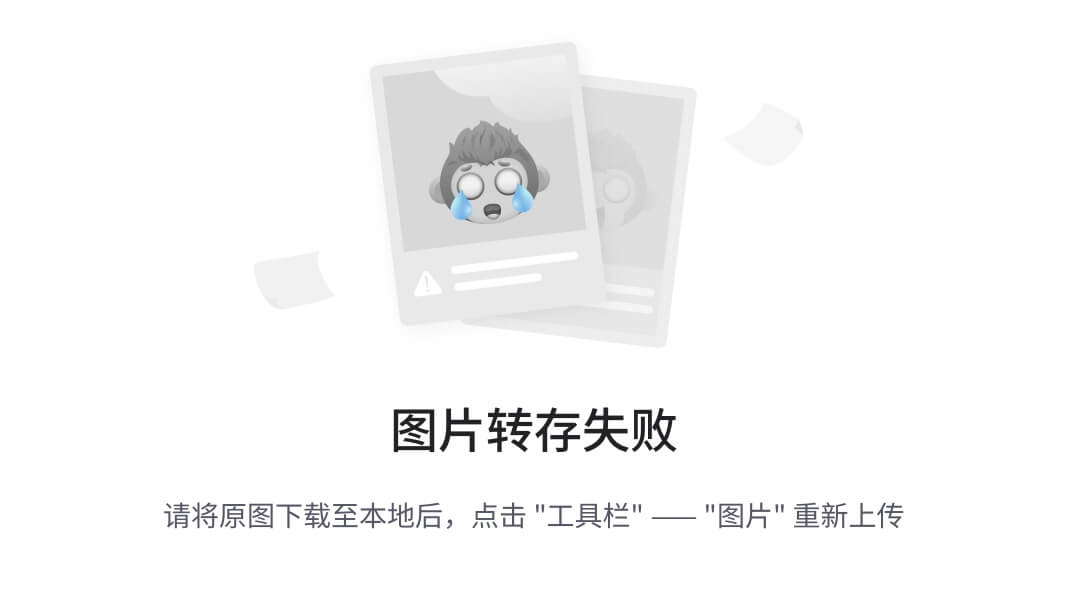
企业如何通过媒体宣传扩大自身影响力
传媒如春雨,润物细无声,大家好,我是51媒体网胡老师。 企业可以通过媒体宣传来扩大自身的影响力。可以通过以下的方法。 1. 制定媒体宣传战略: - 首先,制定一份清晰的媒体宣传战略,明确您的宣传目标、目标…...
处理vue直接引入图片地址时显示不出来的问题 src=“[object Module]“
在webpack中使用vue-loader编译template之后,发现图片加载不出来了,开发人员工具中显示src“[object Module]” 这是因为当vue-loader编译template块之后,会将所有的资源url转换为webpack模块请求 这是因为vue使用的是commonjs语法规范&…...
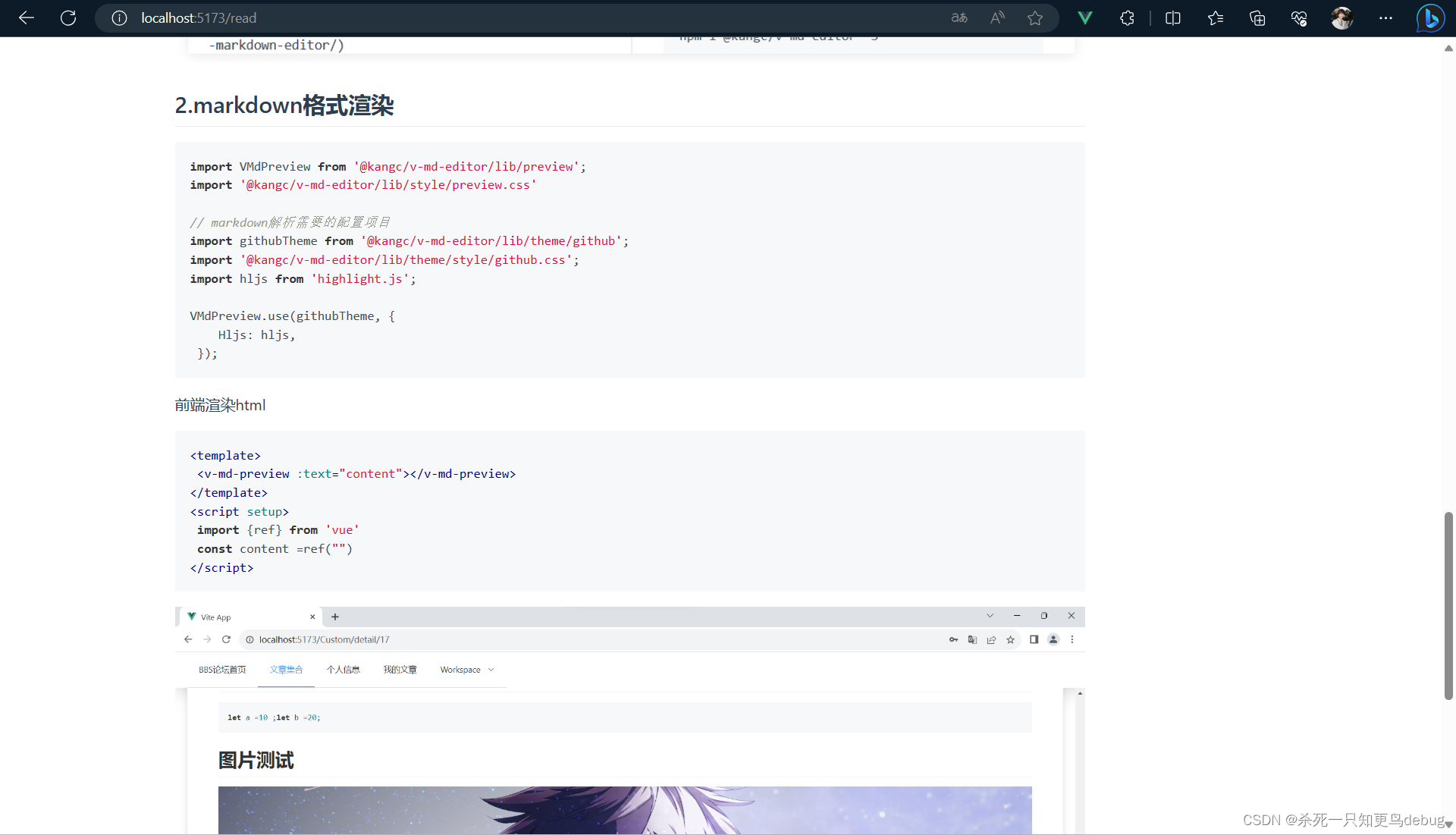
vue3 v-md-editor markdown编辑器(VMdEditor)和预览组件(VMdPreview )的使用
vue3 v-md-editor markdown编辑器和预览组件的使用 概述安装支持vue3版本使用1.使用markdown编辑器 VMdEditor2.markdown文本格式前端渲染 VMdPreview 例子效果代码部分 完整代码 概述 v-md-editor 是基于 Vue 开发的 markdown 编辑器组件 轻量版编辑器 轻量版编辑器左侧编辑…...
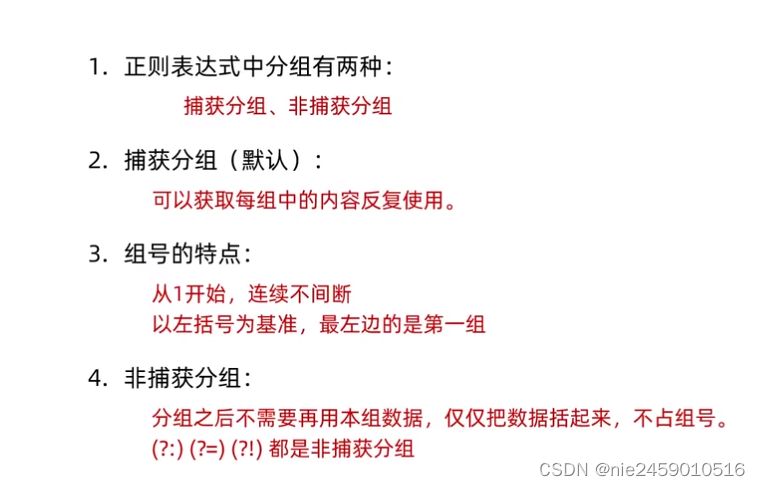
java正则表达式 及应用场景爬虫,捕获分组非捕获分组
正则表达式 通常用于校验 比如说qq号 看输入的是否符合规则就可以用这个 public class regex {public static void main(String[] args) {//正则表达式判断qq号是否正确//规则 6位及20位以内 0不能再开头 必须全是数子String qq"1234567890";System.out.println(qq…...

基于 Debian 稳定分支发行版的Zephix 7 发布
Zephix 是一个基于 Debian 稳定版的实时 Linux 操作系统。它可以完全从可移动媒介上运行,而不触及用户系统磁盘上存储的任何文件。 Zephix 是一个基于 Debian 稳定版的实时 Linux 操作系统。它可以完全从可移动媒介上运行,而不触及用户系统磁盘上存储的…...
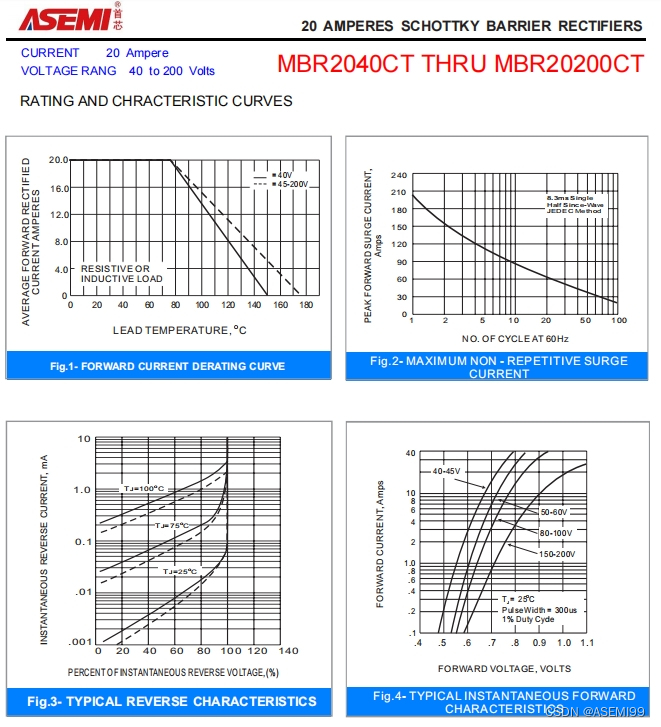
MBR20100CT-ASEMI肖特基MBR20100CT参数、规格、尺寸
编辑:ll MBR20100CT-ASEMI肖特基MBR20100CT参数、规格、尺寸 型号:MBR20100CT 品牌:ASEMI 芯片个数:2 封装:TO-220 恢复时间:>50ns 工作温度:-65C~175C 浪涌电流:…...
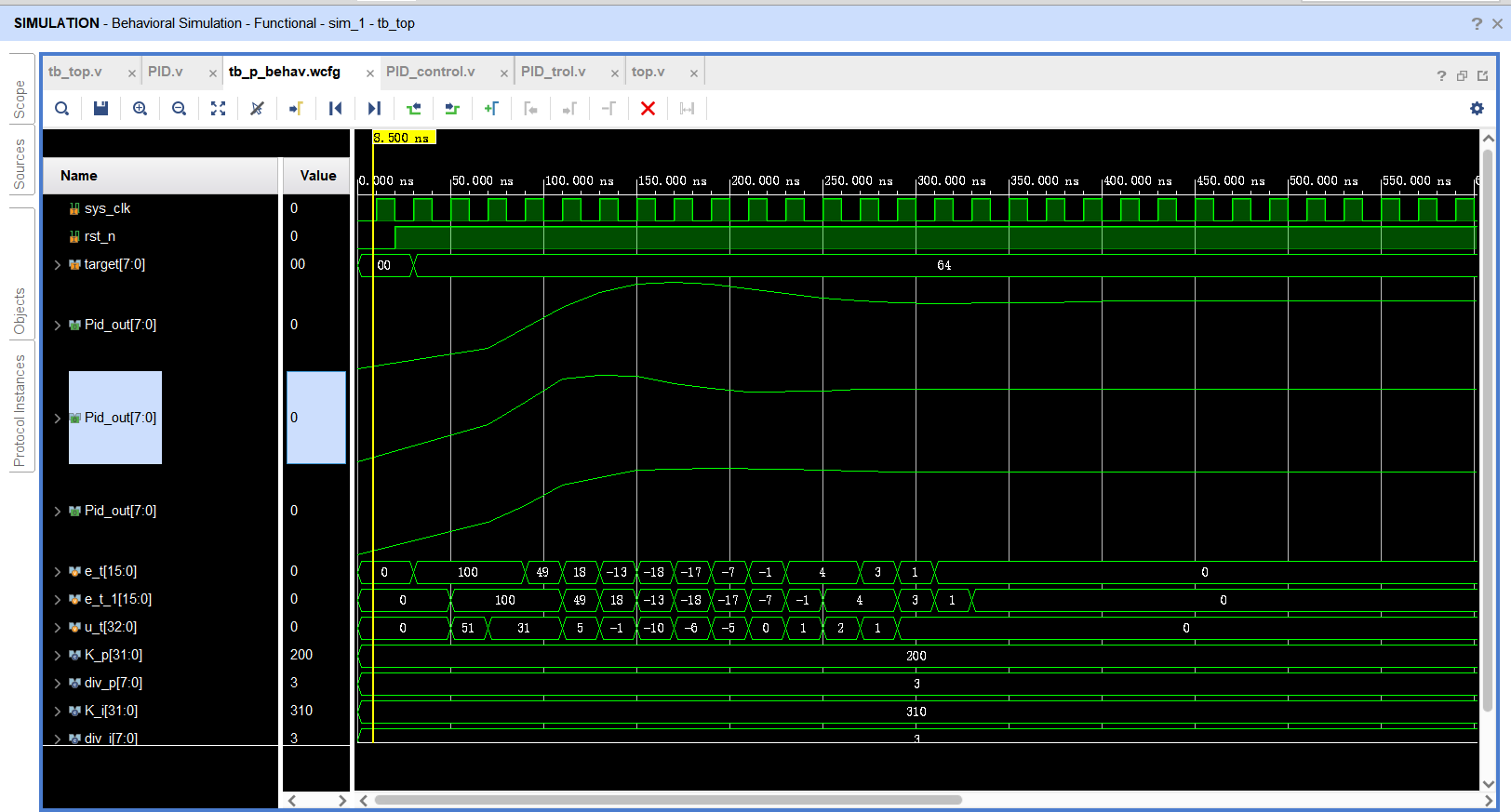
基于FPGA的PID算法学习———实现PID比例控制算法
基于FPGA的PID算法学习 前言一、PID算法分析二、PID仿真分析1. PID代码2.PI代码3.P代码4.顶层5.测试文件6.仿真波形 总结 前言 学习内容:参考网站: PID算法控制 PID即:Proportional(比例)、Integral(积分&…...
Python爬虫实战:研究feedparser库相关技术
1. 引言 1.1 研究背景与意义 在当今信息爆炸的时代,互联网上存在着海量的信息资源。RSS(Really Simple Syndication)作为一种标准化的信息聚合技术,被广泛用于网站内容的发布和订阅。通过 RSS,用户可以方便地获取网站更新的内容,而无需频繁访问各个网站。 然而,互联网…...
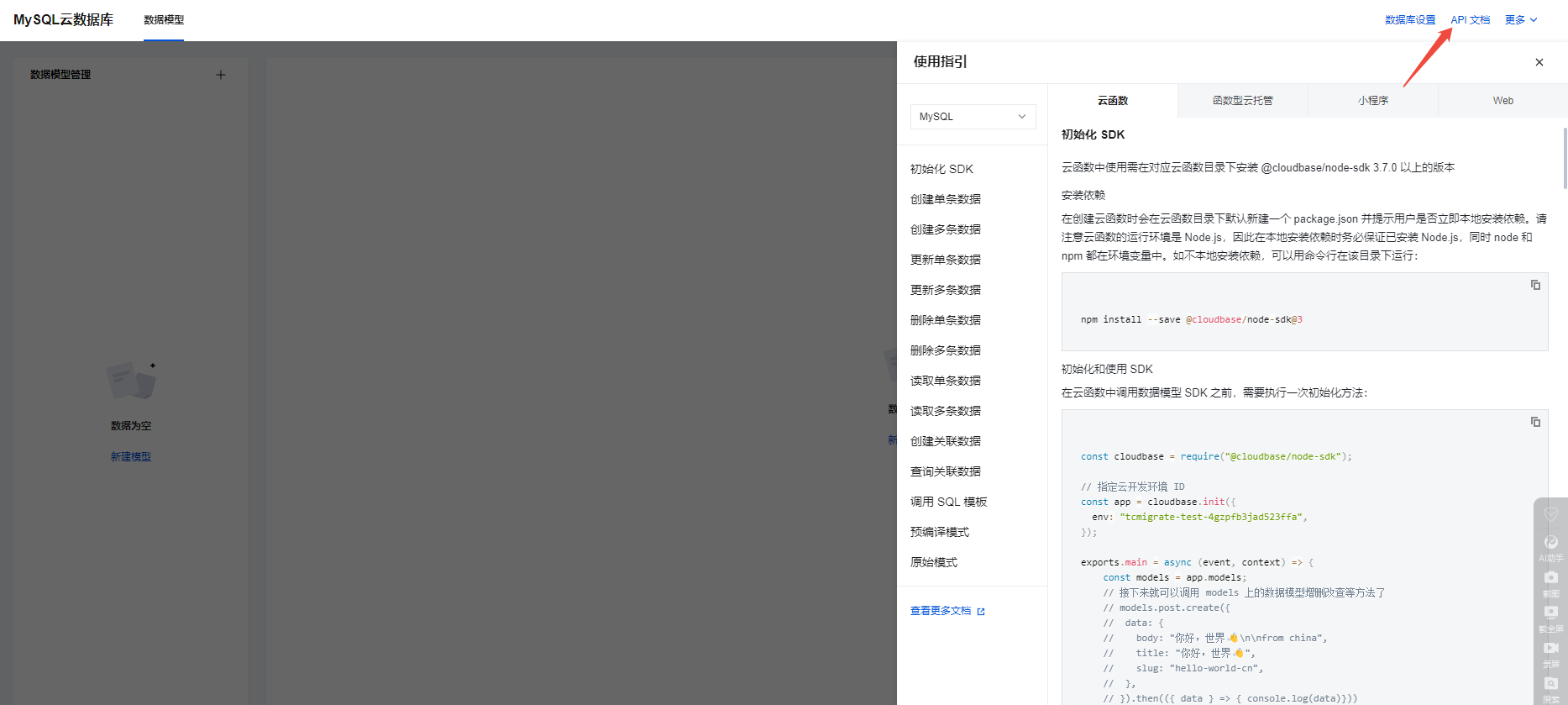
微信小程序云开发平台MySQL的连接方式
注:微信小程序云开发平台指的是腾讯云开发 先给结论:微信小程序云开发平台的MySQL,无法通过获取数据库连接信息的方式进行连接,连接只能通过云开发的SDK连接,具体要参考官方文档: 为什么? 因为…...
Java + Spring Boot + Mybatis 实现批量插入
在 Java 中使用 Spring Boot 和 MyBatis 实现批量插入可以通过以下步骤完成。这里提供两种常用方法:使用 MyBatis 的 <foreach> 标签和批处理模式(ExecutorType.BATCH)。 方法一:使用 XML 的 <foreach> 标签ÿ…...
CRMEB 中 PHP 短信扩展开发:涵盖一号通、阿里云、腾讯云、创蓝
目前已有一号通短信、阿里云短信、腾讯云短信扩展 扩展入口文件 文件目录 crmeb\services\sms\Sms.php 默认驱动类型为:一号通 namespace crmeb\services\sms;use crmeb\basic\BaseManager; use crmeb\services\AccessTokenServeService; use crmeb\services\sms\…...
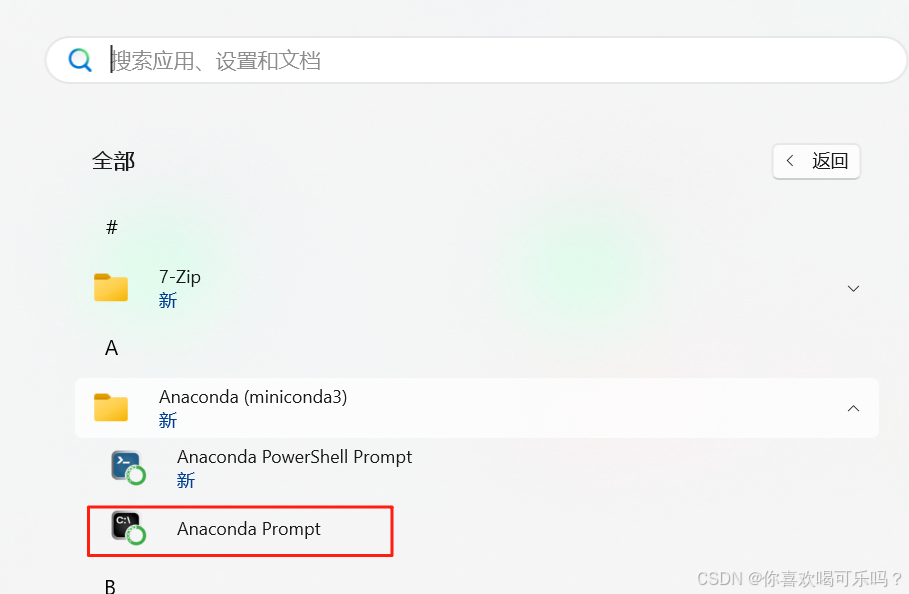
Windows安装Miniconda
一、下载 https://www.anaconda.com/download/success 二、安装 三、配置镜像源 Anaconda/Miniconda pip 配置清华镜像源_anaconda配置清华源-CSDN博客 四、常用操作命令 Anaconda/Miniconda 基本操作命令_miniconda创建环境命令-CSDN博客...
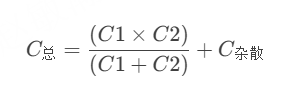
STM32---外部32.768K晶振(LSE)无法起振问题
晶振是否起振主要就检查两个1、晶振与MCU是否兼容;2、晶振的负载电容是否匹配 目录 一、判断晶振与MCU是否兼容 二、判断负载电容是否匹配 1. 晶振负载电容(CL)与匹配电容(CL1、CL2)的关系 2. 如何选择 CL1 和 CL…...
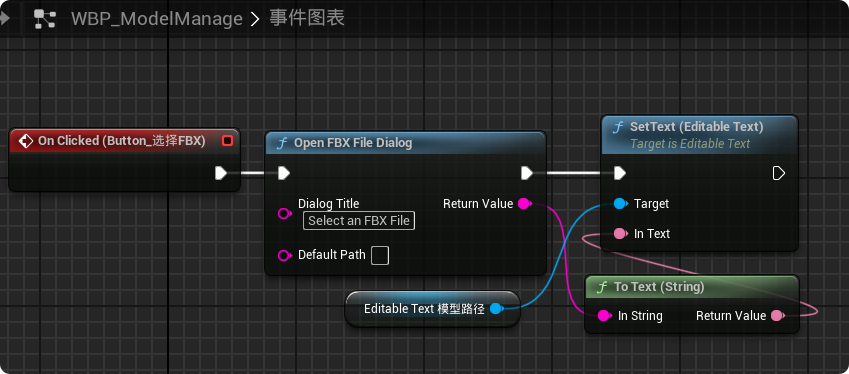
【UE5 C++】通过文件对话框获取选择文件的路径
目录 效果 步骤 源码 效果 步骤 1. 在“xxx.Build.cs”中添加需要使用的模块 ,这里主要使用“DesktopPlatform”模块 2. 添加后闭UE编辑器,右键点击 .uproject 文件,选择 "Generate Visual Studio project files",重…...
Pydantic + Function Calling的结合
1、Pydantic Pydantic 是一个 Python 库,用于数据验证和设置管理,通过 Python 类型注解强制执行数据类型。它广泛用于 API 开发(如 FastAPI)、配置管理和数据解析,核心功能包括: 数据验证:通过…...
GeoServer发布PostgreSQL图层后WFS查询无主键字段
在使用 GeoServer(版本 2.22.2) 发布 PostgreSQL(PostGIS)中的表为地图服务时,常常会遇到一个小问题: WFS 查询中,主键字段(如 id)莫名其妙地消失了! 即使你在…...