网站app制作平台/产品推广策划方案怎么做
目录
- 前言
- 一、场景举例
- 1.联合索引第一个字段用范围查询不走索引(分情况)
- 2.强制走指定索引
- 3.覆盖索引优化
- 4.in和or在表数据量比较大的情况会走索引,在表记录不多的情况下会选择全表扫描
- 5.like 后% 一般情况都会走索引(索引下推)
- 二、Mysql如何选择合适的索引
- 1.trace工具分析
- 三、常见sql深入优化
- 1.Order by与Group by优化
- 2.order by和group by优化总结:
- 3.Using filesort文件排序原理详解
- 四、索引设计原则
- 1、代码先行,索引后上
- 2、联合索引尽量覆盖条件
- 3、不要在小基数字段上建立索引
- 4、长字符串我们可以采用前缀索引
- 5、where与order by冲突时优先where
- 6、基于慢sql查询做优化
前言
为employees表添加10w条数据,需要等待一会(嫌时间长的话可以自己手动写一个java脚本)。下边例子都是基于10w数据演示。本章与之前的第二章有很多关联场景,建议先熟悉一下之前的博客。
第二章博客跳转地址
表结构
CREATE TABLE `employees` (`id` int(11) NOT NULL AUTO_INCREMENT,`name` varchar(24) NOT NULL DEFAULT '' COMMENT '姓名',`age` int(11) NOT NULL DEFAULT '0' COMMENT '年龄',`position` varchar(20) NOT NULL DEFAULT '' COMMENT '职位',`hire_time` timestamp NOT NULL DEFAULT CURRENT_TIMESTAMP COMMENT '入职时间',PRIMARY KEY (`id`),KEY `idx_name_age_position` (`name`,`age`,`position`) USING BTREE
) ENGINE=InnoDB AUTO_INCREMENT=100002 DEFAULT CHARSET=utf8 COMMENT='员工记录表';
插入数据语句(执行较慢建议着急的自己写个批插脚本)
DROP PROCEDURE IF EXISTS insert_emp;
DELIMITER ;;
CREATE PROCEDURE insert_emp()
BEGIN
DECLARE i INT;
SET i=1;
WHILE(i<=100000)DO
INSERT INTO employees(NAME,age,POSITION) VALUES(CONCAT('july',i),i,'dev');
SET i=i+1;
END WHILE;
END;;
DELIMITER ;
CALL insert_emp();
一、场景举例
表结构及相关索引
1.联合索引第一个字段用范围查询不走索引(分情况)
EXPLAIN SELECT * FROM employees WHERE NAME > 'july' AND age = 22 AND POSITION ='manager';
EXPLAIN SELECT * FROM employees WHERE NAME = 'july' AND age > 22 AND POSITION ='manager';
EXPLAIN SELECT * FROM employees WHERE NAME = 'july' AND age = 22 AND POSITION >'manager';
从上边三条explain结果可以看出,当联合索引中的第一个字段name为范围查询时,mysql选择了全表扫描而不是走我们的idx_name_age_position索引,为什么会这样呢?
其实mysql底层有一定的判断规则,mysql认为走全表扫描比走索引更好一些。这么说你可能不相信,请继续向下看。
2.强制走指定索引
使用FORCE INDEX关键字指定强制走哪一个索引。
EXPLAIN SELECT * FROM employees FORCE INDEX(idx_name_age_position)
WHERE NAME > 'july' AND age = 22 AND POSITION ='manager';
结合上边不走索引的执行结果,我们可以看出mysql在全表扫描时大概扫描10w多行的数据,强制走索引之后只扫描了大概5w多行数据。看到这里,那我岂不是走索引香啊。但是不能单单只看扫描行数,还要看查询耗时。
先关闭查询缓存
SET GLOBAL query_cache_size=0;
SET GLOBAL QUERY_CACHE_TYPE=0;
查询两个sql语句执行耗时
SELECT * FROM employees WHERE NAME > 'july'
SELECT * FROM employees FORCE INDEX(idx_name_age_position) WHERE NAME > 'july'
第一条执行耗时:0.199s
第二条执行耗时:0.240s
可以看出走索引查询时时间更长,全表扫描反而时间比较短。当然这里有人可能觉得执行一次不代表每次都是走索引耗时慢呢?这种情况万一是偶然呢?这里大家可以自己动手多执行几次,你会发现这两天sql语句耗时有所变化,不是差很多,但是最终结果都是第一条耗时比第二条短。
3.覆盖索引优化
如何优化这种全表扫描呢?其实之前的博客中也有讲到,那就是尽量让查询语句走覆盖索引。
EXPLAIN SELECT name,age,position FROM employees WHERE NAME > 'july'
4.in和or在表数据量比较大的情况会走索引,在表记录不多的情况下会选择全表扫描
创建一张与employees一模一样的表employees_copy
CREATE TABLE `employees_copy` (`id` int(11) NOT NULL AUTO_INCREMENT,`name` varchar(24) NOT NULL DEFAULT '' COMMENT '姓名',`age` int(11) NOT NULL DEFAULT '0' COMMENT '年龄',`position` varchar(20) NOT NULL DEFAULT '' COMMENT '职位',`hire_time` timestamp NOT NULL DEFAULT CURRENT_TIMESTAMP COMMENT '入职时间',PRIMARY KEY (`id`),KEY `idx_name_age_position` (`name`,`age`,`position`) USING BTREE
) ENGINE=InnoDB AUTO_INCREMENT=100002 DEFAULT CHARSET=utf8 COMMENT='员工记录表';
copy表中只添加几条记录,而employees表中有10w条记录。
两张表执行相同语句
employees表
EXPLAIN SELECT * FROM employees WHERE name in ('LiHua','July','Jack') AND age = 22 AND position
='manager';
employees_copy 表
EXPLAIN SELECT * FROM employees_copy WHERE name in ('LiHua','July','Jack') AND age = 22 AND position
='manager';
其实这里也可以看出为什么在表数据少时,mysql选择不走索引,因为在copy表中先去索引查询完后,还需要回表再去查一遍。一共就三条记录还有查询两遍,不如直接一下全表扫描来的快。
or 查询也是一样的道理
EXPLAIN SELECT * FROM employees WHERE (name = 'LiHua' or name = 'July') AND age = 22 AND position
='manager';
EXPLAIN SELECT * FROM employees_copy WHERE (name = 'LiHua' or name = 'July') AND age = 22 AND position
='manager';
5.like 后% 一般情况都会走索引(索引下推)
EXPLAIN SELECT * FROM employees WHERE name like 'LiHua%' AND age = 22 AND position ='manager';
mysql5.6之前版本,mysql会根据条件LiHua%
把所有的主键id查询出来,然后去主键索引去查询,然后根据查询出的数据再根据其它条件进行过滤。
索引下推:
5.6及之后的版本,首先根据条件LiHua%
把对应的主键id查询出来,然后再查出来的基础上再根据age字段和position字段的条件进行过滤,如果符合条件则把整个id拿到,否则过滤掉整个id。最后拿着得到的id集合去主键索引里查询。(减少回表次数)
索引下推会减少回表次数,对于innodb引擎的表索引下推只能用于二级索引,innodb的主键索引(聚簇索引)树叶子节点上保存的是全行数据,所以这个时候索引下推并不会起到减少查询全行数据的效果。
为什么范围查找Mysql没有用索引下推优化?
估计应该是Mysql认为范围查找过滤的结果集过大(这个就得看底层源码了解才知道了),like KK% 在绝大多数情况来看,过滤后的结果集比较小,所以这里Mysql选择给 like
KK% 用了索引下推优化,这里like后%不是一定每次都会走索引下推,有时like KK% 也不一定就会走索引下推。
二、Mysql如何选择合适的索引
1.trace工具分析
EXPLAIN select * from employees where name > 'a';
EXPLAIN select * from employees where name > 'z';
对于上面这两种 name>‘a’ 和 name>‘z’ 的执行结果,mysql最终是否选择走索引或者一张表涉及多个索引,mysql最终如何选择索引,我们可以用trace工具来一查究竟,开启trace工具会影响mysql性能,所以只能临时分析sql使用,用完之后立即关闭。
前置条件,开启trace
set session optimizer_trace="enabled=on",end_markers_in_json=on;
SELECT * FROM employees where name > 'a' order by position;
SELECT * FROM information_schema.OPTIMIZER_TRACE;
第一张表记录太多就不全部展示了。
information_schema.OPTIMIZER_TRACE这个库下的这个表是固定,也是mysql默认就有的数据库。
展开trace列原始内容
{"steps": [{"join_preparation": {"select#": 1,"steps": [{"expanded_query": "/* select#1 */ select `employees`.`id` AS `id`,`employees`.`name` AS `name`,`employees`.`age` AS `age`,`employees`.`position` AS `position`,`employees`.`hire_time` AS `hire_time` from `employees` where (`employees`.`name` > 'a') order by `employees`.`position`"}] /* steps */} /* join_preparation */},{"join_optimization": {"select#": 1,"steps": [{"condition_processing": {"condition": "WHERE","original_condition": "(`employees`.`name` > 'a')","steps": [{"transformation": "equality_propagation","resulting_condition": "(`employees`.`name` > 'a')"},{"transformation": "constant_propagation","resulting_condition": "(`employees`.`name` > 'a')"},{"transformation": "trivial_condition_removal","resulting_condition": "(`employees`.`name` > 'a')"}] /* steps */} /* condition_processing */},{"substitute_generated_columns": {} /* substitute_generated_columns */},{"table_dependencies": [{"table": "`employees`","row_may_be_null": false,"map_bit": 0,"depends_on_map_bits": [] /* depends_on_map_bits */}] /* table_dependencies */},{"ref_optimizer_key_uses": [] /* ref_optimizer_key_uses */},{"rows_estimation": [{"table": "`employees`","range_analysis": {"table_scan": {"rows": 97657,"cost": 19886} /* table_scan */,"potential_range_indexes": [{"index": "PRIMARY","usable": false,"cause": "not_applicable"},{"index": "idx_name_age_position","usable": true,"key_parts": ["name","age","position","id"] /* key_parts */}] /* potential_range_indexes */,"setup_range_conditions": [] /* setup_range_conditions */,"group_index_range": {"chosen": false,"cause": "not_group_by_or_distinct"} /* group_index_range */,"analyzing_range_alternatives": {"range_scan_alternatives": [{"index": "idx_name_age_position","ranges": ["a < name"] /* ranges */,"index_dives_for_eq_ranges": true,"rowid_ordered": false,"using_mrr": false,"index_only": false,"rows": 48828,"cost": 58595,"chosen": false,"cause": "cost"}] /* range_scan_alternatives */,"analyzing_roworder_intersect": {"usable": false,"cause": "too_few_roworder_scans"} /* analyzing_roworder_intersect */} /* analyzing_range_alternatives */} /* range_analysis */}] /* rows_estimation */},{"considered_execution_plans": [{"plan_prefix": [] /* plan_prefix */,"table": "`employees`","best_access_path": {"considered_access_paths": [{"rows_to_scan": 97657,"access_type": "scan","resulting_rows": 97657,"cost": 19884,"chosen": true,"use_tmp_table": true}] /* considered_access_paths */} /* best_access_path */,"condition_filtering_pct": 100,"rows_for_plan": 97657,"cost_for_plan": 19884,"sort_cost": 97657,"new_cost_for_plan": 117541,"chosen": true}] /* considered_execution_plans */},{"attaching_conditions_to_tables": {"original_condition": "(`employees`.`name` > 'a')","attached_conditions_computation": [] /* attached_conditions_computation */,"attached_conditions_summary": [{"table": "`employees`","attached": "(`employees`.`name` > 'a')"}] /* attached_conditions_summary */} /* attaching_conditions_to_tables */},{"clause_processing": {"clause": "ORDER BY","original_clause": "`employees`.`position`","items": [{"item": "`employees`.`position`"}] /* items */,"resulting_clause_is_simple": true,"resulting_clause": "`employees`.`position`"} /* clause_processing */},{"reconsidering_access_paths_for_index_ordering": {"clause": "ORDER BY","steps": [] /* steps */,"index_order_summary": {"table": "`employees`","index_provides_order": false,"order_direction": "undefined","index": "unknown","plan_changed": false} /* index_order_summary */} /* reconsidering_access_paths_for_index_ordering */},{"refine_plan": [{"table": "`employees`"}] /* refine_plan */}] /* steps */} /* join_optimization */},{"join_execution": {"select#": 1,"steps": [{"filesort_information": [{"direction": "asc","table": "`employees`","field": "position"}] /* filesort_information */,"filesort_priority_queue_optimization": {"usable": false,"cause": "not applicable (no LIMIT)"} /* filesort_priority_queue_optimization */,"filesort_execution": [] /* filesort_execution */,"filesort_summary": {"rows": 100001,"examined_rows": 100001,"number_of_tmp_files": 29,"sort_buffer_size": 262056,"sort_mode": "<sort_key, packed_additional_fields>"} /* filesort_summary */}] /* steps */} /* join_execution */}] /* steps */
}
相关trace中一些关键字的含义
{"steps": [{"join_preparation": { ‐第一阶段:SQL准备阶段,格式化sql},{"join_optimization": { ‐第二阶段:SQL优化阶段(比如查询条件中一些无意义的查询,where 1=1,或者优化一下查询条件使之符合最左前缀匹配等。)"select#": 1,"steps": [{"condition_processing": {"condition": "WHERE","original_condition": "(`employees`.`name` > 'a')","steps": [{"transformation": "equality_propagation","resulting_condition": "(`employees`.`name` > 'a')"},{"transformation": "constant_propagation","resulting_condition": "(`employees`.`name` > 'a')"},{"transformation": "trivial_condition_removal","resulting_condition": "(`employees`.`name` > 'a')"}] /* steps */} /* condition_processing */},{"substitute_generated_columns": {} /* substitute_generated_columns */},{"table_dependencies": [{"table": "`employees`","row_may_be_null": false,"map_bit": 0,"depends_on_map_bits": [] /* depends_on_map_bits */}] /* table_dependencies */},{"ref_optimizer_key_uses": [] /* ref_optimizer_key_uses */},{"rows_estimation": [ ‐-预估表的访问成本(走不走索引,他们的成本等){"table": "`employees`","range_analysis": { ‐-全表扫描情况(不走索引全部扫描的成本)"table_scan": {"rows": 97657, ‐-扫描行数"cost": 19886 -‐查询成本(是一个相对值,没有单位,值越大成本越高)} /* table_scan */,"potential_range_indexes": [ ‐-查询可能使用的索引{"index": "PRIMARY", ‐-主键索引"usable": false, ‐-不会走主键,所以结果为false"cause": "not_applicable"},{"index": "idx_name_age_position", ‐-辅助索引"usable": true,"key_parts": ["name","age","position","id"] /* key_parts */}] /* potential_range_indexes */,"setup_range_conditions": [] /* setup_range_conditions */,"group_index_range": {"chosen": false,"cause": "not_group_by_or_distinct"} /* group_index_range */,"analyzing_range_alternatives": { -‐分析各个索引使用成本"range_scan_alternatives": [{"index": "idx_name_age_position","ranges": ["a < name" ‐‐索引使用范围] /* ranges */,"index_dives_for_eq_ranges": true,"rowid_ordered": false, ‐‐使用该索引获取的记录是否按照主键排序"using_mrr": false,"index_only": false, ‐‐是否使用覆盖索引"rows": 48828, ‐‐索引扫描行数"cost": 58595, ‐‐索引使用成本"chosen": false, ‐‐是否选择该索引"cause": "cost"}] /* range_scan_alternatives */,"analyzing_roworder_intersect": {"usable": false,"cause": "too_few_roworder_scans"} /* analyzing_roworder_intersect */} /* analyzing_range_alternatives */} /* range_analysis */}] /* rows_estimation */},{"considered_execution_plans": [{"plan_prefix": [] /* plan_prefix */,"table": "`employees`","best_access_path": { ‐‐ 最优访问路径"considered_access_paths": [ ‐‐最终选择的访问路径{"rows_to_scan": 97657,"access_type": "scan", ‐‐访问类型:为scan,全表扫描"resulting_rows": 97657,"cost": 19884,"chosen": true, ‐‐确定选择"use_tmp_table": true}] /* considered_access_paths */} /* best_access_path */,"condition_filtering_pct": 100,"rows_for_plan": 97657,"cost_for_plan": 19884,"sort_cost": 97657,"new_cost_for_plan": 117541,"chosen": true}] /* considered_execution_plans */},{"attaching_conditions_to_tables": {"original_condition": "(`employees`.`name` > 'a')","attached_conditions_computation": [] /* attached_conditions_computation */,"attached_conditions_summary": [{"table": "`employees`","attached": "(`employees`.`name` > 'a')"}] /* attached_conditions_summary */} /* attaching_conditions_to_tables */},{"clause_processing": {"clause": "ORDER BY","original_clause": "`employees`.`position`","items": [{"item": "`employees`.`position`"}] /* items */,"resulting_clause_is_simple": true,"resulting_clause": "`employees`.`position`"} /* clause_processing */},{"reconsidering_access_paths_for_index_ordering": {"clause": "ORDER BY","steps": [] /* steps */,"index_order_summary": {"table": "`employees`","index_provides_order": false,"order_direction": "undefined","index": "unknown","plan_changed": false} /* index_order_summary */} /* reconsidering_access_paths_for_index_ordering */},{"refine_plan": [{"table": "`employees`"}] /* refine_plan */}] /* steps */} /* join_optimization */},{"join_execution": { ‐‐第三阶段:SQL执行阶段"select#": 1,"steps": [{"filesort_information": [{"direction": "asc","table": "`employees`","field": "position"}] /* filesort_information */,"filesort_priority_queue_optimization": {"usable": false,"cause": "not applicable (no LIMIT)"} /* filesort_priority_queue_optimization */,"filesort_execution": [] /* filesort_execution */,"filesort_summary": {"rows": 100001,"examined_rows": 100001,"number_of_tmp_files": 29,"sort_buffer_size": 262056,"sort_mode": "<sort_key, packed_additional_fields>"} /* filesort_summary */}] /* steps */} /* join_execution */}] /* steps */
}
结论:全表扫描的成本低于索引扫描,所以mysql最终选择全表扫描
关闭trace
set session optimizer_trace="enabled=off";
三、常见sql深入优化
1.Order by与Group by优化
employees 表相关索引信息如下:
举例1
EXPLAIN SELECT * FROM employees
WHERE NAME = 'LiLei' AND POSITION = 'dev' ORDER BY age;
分析:
利用最左前缀法则:中间字段不能断,因此查询用到了name索引,从key_len=74也能看出,age索引列用在排序过程中,因为Extra字段里没有using filesort,所以age字段也走索引了。
举例2
EXPLAIN SELECT * FROM employees WHERE NAME = 'LiLei' ORDER BY POSITION;
分析:
从explain的执行结果来看:key_len=74,查询使用了name索引,由于用了position进行排序,跳过了
age,出现了Using filesort。所以POSITION字段未走索引。
举例3
EXPLAIN SELECT * FROM employees WHERE NAME = 'LiLei' ORDER BY age,POSITION;
分析:
查找只用到索引name,age和position用于排序,也用到了索引,无Using filesort。
举例4
EXPLAIN SELECT * FROM employees WHERE NAME = 'LiLei' ORDER BY POSITION,age;
分析:
和举例3中explain的执行结果一样,但是出现了Using filesort,因为索引的创建顺序为name,age,position,但是排序的时候age和position颠倒位置了导致后边排序无法用到索引。
举例5
EXPLAIN SELECT * FROM employees WHERE NAME = 'LiLei' AND age = 18 ORDER BY POSITION,age;
分析:
与举例4对比,在Extra中并未出现Using filesort,因为age为常量,在排序中被优化,相当于在name和age都确定的情况下,按照position去排序,所以索引未颠倒,不会出现Using filesort。
举例6
EXPLAIN SELECT * FROM employees WHERE NAME = 'zhuge' ORDER BY age ASC,POSITION DESC;
分析:
虽然排序的字段列与索引顺序一样,且order by默认升序,这里position desc变成了降序,导致与索引的排序方式不同,从而产生Using filesort。Mysql8以上版本有降序索引可以支持该种查询方式(我了解是建立索引时指定字段是按照升序建立还是降序建立)。
举例7
EXPLAIN SELECT * FROM employees WHERE NAME IN ('LiLei','zhuge')
ORDER BY age,POSITION;
分析:
对于排序来说,多个相等条件也是范围查询,即在name的in查询中这一批范围里的age和position不一定是相对有序的所以order by时走的Using filesort。
举例8
EXPLAIN SELECT * FROM employees WHERE NAME > 'a' ORDER BY NAME;
分析:
按照道理该sql是可以走索引的,即在name>'a’的这个范围中,name顺序是有序的可以走索引,但是mysql确实全表扫描,可能原因就是mysql认为全表扫描比走索引要好。
EXPLAIN SELECT NAME,age,POSITION FROM employees WHERE NAME > 'a' ORDER BY NAME;
分析:
尝试用覆盖索引进行优化,果然走索引了。
2.order by和group by优化总结:
1.MySQL支持两种方式的排序filesort和index,Using index是指MySQL扫描索引本身完成排序。index效率高,filesort效率低。
2.order by满足两种情况会使用Using index。①order by语句使用索引最左前列。②使用where子句与order by子句条件列组合满足索引最左前列。
3.尽量在索引列上完成排序,遵循索引建立(索引创建的顺序)时的最左前缀法则。
4.如果order by的条件不在索引列上,就会产生Using filesort。
5.能用覆盖索引尽量用覆盖索引
6.group by与order by很类似,其实质是先排序后分组,遵照索引创建顺序的最左前缀法则。对于groupby的优化如果不需要排序的可以加上order by null禁止排序。注意,where高于having,能写在where中的限定条件就不要去having限定了。
3.Using filesort文件排序原理详解
filesort文件排序方式
- 单路排序:是一次性取出满足条件行的所有字段,然后在sort buffer中进行排序;用trace工具可以看到sort_mode信息里显示< sort_key, additional_fields >或者< sort_key,packed_additional_fields >
- 双路排序(回表排序模式):是首先根据相应的条件取出相应的排序字段和可以直接定位行数据的行 ID,然后在 sort buffer 中进行排序,排序完后需要再次取回其它需要的字段;用trace工具可以看到sort_mode信息里显示< sort_key, rowid >
MySQL 通过比较系统变量 max_length_for_sort_data(默认1024字节) 的大小和需要查询的字段总大小来判断使用哪种排序模式。
如果 字段的总长度小于max_length_for_sort_data ,那么使用 单路排序模式。如果 字段的总长度大于max_length_for_sort_data ,那么使用 双路排序模式。
验证单路排序和双路排序
首先,开启trace
set session optimizer_trace="enabled=on",end_markers_in_json=on;
查询语句
SELECT * FROM employees WHERE NAME = 'july' ORDER BY POSITION;
SELECT * FROM information_schema.OPTIMIZER_TRACE;
单路排序举例
{"steps": [{"join_preparation": {"select#": 1,"steps": [{"expanded_query": "/* select#1 */ select `employees`.`id` AS `id`,`employees`.`name` AS `name`,`employees`.`age` AS `age`,`employees`.`position` AS `position`,`employees`.`hire_time` AS `hire_time` from `employees` where (`employees`.`name` = 'july') order by `employees`.`position`"}] /* steps */} /* join_preparation */},{"join_optimization": {"select#": 1,"steps": [{"condition_processing": {"condition": "WHERE","original_condition": "(`employees`.`name` = 'july')","steps": [{"transformation": "equality_propagation","resulting_condition": "(`employees`.`name` = 'july')"},{"transformation": "constant_propagation","resulting_condition": "(`employees`.`name` = 'july')"},{"transformation": "trivial_condition_removal","resulting_condition": "(`employees`.`name` = 'july')"}] /* steps */} /* condition_processing */},{"substitute_generated_columns": {} /* substitute_generated_columns */},{"table_dependencies": [{"table": "`employees`","row_may_be_null": false,"map_bit": 0,"depends_on_map_bits": [] /* depends_on_map_bits */}] /* table_dependencies */},{"ref_optimizer_key_uses": [{"table": "`employees`","field": "name","equals": "'july'","null_rejecting": false}] /* ref_optimizer_key_uses */},{"rows_estimation": [{"table": "`employees`","range_analysis": {"table_scan": {"rows": 97656,"cost": 19886} /* table_scan */,"potential_range_indexes": [{"index": "PRIMARY","usable": false,"cause": "not_applicable"},{"index": "idx_name_age_position","usable": true,"key_parts": ["name","age","position","id"] /* key_parts */}] /* potential_range_indexes */,"setup_range_conditions": [] /* setup_range_conditions */,"group_index_range": {"chosen": false,"cause": "not_group_by_or_distinct"} /* group_index_range */,"analyzing_range_alternatives": {"range_scan_alternatives": [{"index": "idx_name_age_position","ranges": ["july <= name <= july"] /* ranges */,"index_dives_for_eq_ranges": true,"rowid_ordered": false,"using_mrr": false,"index_only": false,"rows": 1,"cost": 2.21,"chosen": true}] /* range_scan_alternatives */,"analyzing_roworder_intersect": {"usable": false,"cause": "too_few_roworder_scans"} /* analyzing_roworder_intersect */} /* analyzing_range_alternatives */,"chosen_range_access_summary": {"range_access_plan": {"type": "range_scan","index": "idx_name_age_position","rows": 1,"ranges": ["july <= name <= july"] /* ranges */} /* range_access_plan */,"rows_for_plan": 1,"cost_for_plan": 2.21,"chosen": true} /* chosen_range_access_summary */} /* range_analysis */}] /* rows_estimation */},{"considered_execution_plans": [{"plan_prefix": [] /* plan_prefix */,"table": "`employees`","best_access_path": {"considered_access_paths": [{"access_type": "ref","index": "idx_name_age_position","rows": 1,"cost": 1.2,"chosen": true},{"access_type": "range","range_details": {"used_index": "idx_name_age_position"} /* range_details */,"chosen": false,"cause": "heuristic_index_cheaper"}] /* considered_access_paths */} /* best_access_path */,"condition_filtering_pct": 100,"rows_for_plan": 1,"cost_for_plan": 1.2,"chosen": true}] /* considered_execution_plans */},{"attaching_conditions_to_tables": {"original_condition": "(`employees`.`name` = 'july')","attached_conditions_computation": [] /* attached_conditions_computation */,"attached_conditions_summary": [{"table": "`employees`","attached": null}] /* attached_conditions_summary */} /* attaching_conditions_to_tables */},{"clause_processing": {"clause": "ORDER BY","original_clause": "`employees`.`position`","items": [{"item": "`employees`.`position`"}] /* items */,"resulting_clause_is_simple": true,"resulting_clause": "`employees`.`position`"} /* clause_processing */},{"added_back_ref_condition": "((`employees`.`name` <=> 'july'))"},{"reconsidering_access_paths_for_index_ordering": {"clause": "ORDER BY","steps": [] /* steps */,"index_order_summary": {"table": "`employees`","index_provides_order": false,"order_direction": "undefined","index": "idx_name_age_position","plan_changed": false} /* index_order_summary */} /* reconsidering_access_paths_for_index_ordering */},{"refine_plan": [{"table": "`employees`","pushed_index_condition": "(`employees`.`name` <=> 'july')","table_condition_attached": null}] /* refine_plan */}] /* steps */} /* join_optimization */},{"join_execution": {"select#": 1,"steps": [{"filesort_information": [ {"direction": "asc","table": "`employees`","field": "position"}] /* filesort_information */,"filesort_priority_queue_optimization": {"usable": false,"cause": "not applicable (no LIMIT)"} /* filesort_priority_queue_optimization */,"filesort_execution": [] /* filesort_execution */,"filesort_summary": { ‐‐文件排序信息"rows": 0, -‐预计扫描行数"examined_rows": 0, -‐参与排序的行"number_of_tmp_files": 0, ‐‐使用临时文件的个数,这个值如果为0代表全部使用的sort_buffer内存排序,否则使用的
磁盘文件排序"sort_buffer_size": 262056, ‐‐排序缓存的大小,单位Byte"sort_mode": "<sort_key, packed_additional_fields>" ‐‐排序方式,这里用的单路排序} /* filesort_summary */}] /* steps */} /* join_execution */}] /* steps */
}
多路排序举例
employees表所有字段长度总和肯定大于10字节,所以设置max_length_for_sort_data为10。
set max_length_for_sort_data = 10
再次执行刚才的查询
SELECT * FROM employees WHERE NAME = 'july' ORDER BY POSITION;
SELECT * FROM information_schema.OPTIMIZER_TRACE;
{"steps": [{"join_preparation": {"select#": 1,"steps": [{"expanded_query": "/* select#1 */ select `employees`.`id` AS `id`,`employees`.`name` AS `name`,`employees`.`age` AS `age`,`employees`.`position` AS `position`,`employees`.`hire_time` AS `hire_time` from `employees` where (`employees`.`name` = 'july') order by `employees`.`position`"}] /* steps */} /* join_preparation */},{"join_optimization": {"select#": 1,"steps": [{"condition_processing": {"condition": "WHERE","original_condition": "(`employees`.`name` = 'july')","steps": [{"transformation": "equality_propagation","resulting_condition": "(`employees`.`name` = 'july')"},{"transformation": "constant_propagation","resulting_condition": "(`employees`.`name` = 'july')"},{"transformation": "trivial_condition_removal","resulting_condition": "(`employees`.`name` = 'july')"}] /* steps */} /* condition_processing */},{"substitute_generated_columns": {} /* substitute_generated_columns */},{"table_dependencies": [{"table": "`employees`","row_may_be_null": false,"map_bit": 0,"depends_on_map_bits": [] /* depends_on_map_bits */}] /* table_dependencies */},{"ref_optimizer_key_uses": [{"table": "`employees`","field": "name","equals": "'july'","null_rejecting": false}] /* ref_optimizer_key_uses */},{"rows_estimation": [{"table": "`employees`","range_analysis": {"table_scan": {"rows": 97656,"cost": 19886} /* table_scan */,"potential_range_indexes": [{"index": "PRIMARY","usable": false,"cause": "not_applicable"},{"index": "idx_name_age_position","usable": true,"key_parts": ["name","age","position","id"] /* key_parts */}] /* potential_range_indexes */,"setup_range_conditions": [] /* setup_range_conditions */,"group_index_range": {"chosen": false,"cause": "not_group_by_or_distinct"} /* group_index_range */,"analyzing_range_alternatives": {"range_scan_alternatives": [{"index": "idx_name_age_position","ranges": ["july <= name <= july"] /* ranges */,"index_dives_for_eq_ranges": true,"rowid_ordered": false,"using_mrr": false,"index_only": false,"rows": 1,"cost": 2.21,"chosen": true}] /* range_scan_alternatives */,"analyzing_roworder_intersect": {"usable": false,"cause": "too_few_roworder_scans"} /* analyzing_roworder_intersect */} /* analyzing_range_alternatives */,"chosen_range_access_summary": {"range_access_plan": {"type": "range_scan","index": "idx_name_age_position","rows": 1,"ranges": ["july <= name <= july"] /* ranges */} /* range_access_plan */,"rows_for_plan": 1,"cost_for_plan": 2.21,"chosen": true} /* chosen_range_access_summary */} /* range_analysis */}] /* rows_estimation */},{"considered_execution_plans": [{"plan_prefix": [] /* plan_prefix */,"table": "`employees`","best_access_path": {"considered_access_paths": [{"access_type": "ref","index": "idx_name_age_position","rows": 1,"cost": 1.2,"chosen": true},{"access_type": "range","range_details": {"used_index": "idx_name_age_position"} /* range_details */,"chosen": false,"cause": "heuristic_index_cheaper"}] /* considered_access_paths */} /* best_access_path */,"condition_filtering_pct": 100,"rows_for_plan": 1,"cost_for_plan": 1.2,"chosen": true}] /* considered_execution_plans */},{"attaching_conditions_to_tables": {"original_condition": "(`employees`.`name` = 'july')","attached_conditions_computation": [] /* attached_conditions_computation */,"attached_conditions_summary": [{"table": "`employees`","attached": null}] /* attached_conditions_summary */} /* attaching_conditions_to_tables */},{"clause_processing": {"clause": "ORDER BY","original_clause": "`employees`.`position`","items": [{"item": "`employees`.`position`"}] /* items */,"resulting_clause_is_simple": true,"resulting_clause": "`employees`.`position`"} /* clause_processing */},{"added_back_ref_condition": "((`employees`.`name` <=> 'july'))"},{"reconsidering_access_paths_for_index_ordering": {"clause": "ORDER BY","steps": [] /* steps */,"index_order_summary": {"table": "`employees`","index_provides_order": false,"order_direction": "undefined","index": "idx_name_age_position","plan_changed": false} /* index_order_summary */} /* reconsidering_access_paths_for_index_ordering */},{"refine_plan": [{"table": "`employees`","pushed_index_condition": "(`employees`.`name` <=> 'july')","table_condition_attached": null}] /* refine_plan */}] /* steps */} /* join_optimization */},{"join_execution": {"select#": 1,"steps": [{"filesort_information": [{"direction": "asc","table": "`employees`","field": "position"}] /* filesort_information */,"filesort_priority_queue_optimization": {"usable": false,"cause": "not applicable (no LIMIT)"} /* filesort_priority_queue_optimization */,"filesort_execution": [] /* filesort_execution */,"filesort_summary": {"rows": 0,"examined_rows": 0,"number_of_tmp_files": 0,"sort_buffer_size": 262136,"sort_mode": "<sort_key, rowid>" ‐‐‐排序方式,这里用的双路排序} /* filesort_summary */}] /* steps */} /* join_execution */}] /* steps */
}
单路排序的详细过程:
- 从索引name找到第一个满足 name = ‘july’ 条件的主键 id
- 根据主键 id 取出整行,取出所有字段的值,存入 sort_buffer 中
- 从索引name找到下一个满足 name = ‘july’ 条件的主键 id
- 重复步骤 2、3 直到不满足 name = ‘july’
- 对 sort_buffer 中的数据按照字段 position 进行排序
- 返回结果给客户端
双路排序的详细过程:
- 从索引 name 找到第一个满足 name = ‘july’ 的主键id
- 根据主键 id 取出整行,把排序字段 position 和主键 id 这两个字段放到 sort buffer 中
- 从索引 name 取下一个满足 name = ‘july’ 记录的主键 id
- 重复 3、4 直到不满足 name = ‘july’
- 对 sort_buffer 中的字段 position 和主键 id 按照字段 position 进行排序
- 遍历排序好的 id 和字段 position,按照 id 的值回到原表中取出 所有字段的值返回给客户端
对比两种排序模式:
正常在内存里排序肯定是要比在磁盘中转一下进行排序要快的。在sort buffer内存大小够用的情况下,是不会去磁盘排序的,只有超过了sort buffer大小之后才会涉及磁盘排序。
单路排序会把所有需要查询的字段都放到 sort buffer 中,而双路排序只会把主键和需要排序的字段放到 sort buffer 中进行排序,然后再通过主键回到原表查询需要的字段。这里我们可以看出来如果是单路排序,那么sort buffer里边查询的所有字段都会放进去,而双路只会放进去主键和排序的字段,这样的话,sort buffer一定的情况下,双路排序放的要排序的行就会比单路多,但是双路还是需要根据主键回表查询剩余要查询的字段。有利有弊需要自己衡量。
如果 MySQL 排序内存 sort_buffer 配置的比较小并且没有条件继续增加了,可以适当把max_length_for_sort_data 配置小点,让优化器选择使用双路排序算法,可以在sort_buffer 中一次排序更多的行,只是需要再根据主键回到原表取数据。
如果 MySQL 排序内存有条件可以配置比较大,可以适当增大 max_length_for_sort_data 的值,让优化器优先选择全字段排序(单路排序),把需要的字段放到 sort_buffer 中,这样排序后就会直接从内存里返回查询结果了。
所以,MySQL通过 max_length_for_sort_data 这个参数来控制排序,在不同场景使用不同的排序模式,从而提升排序效率。
如果全部使用sort_buffer内存排序一般情况下效率会高于磁盘文件排序,但不能因为这个就随便增大sort_buffer(默认1M),mysql很多参数设置都是做过优化的,不要轻易调整。
四、索引设计原则
1、代码先行,索引后上
一般应该等到主体业务功能开发完毕,把涉及到该表相关sql都要拿出来分析之后再建立索引。当然这样比较标准,但是正常情况下如果业务不复杂,在设计的同时那些增删改查的sql基本都能确定个差不多,在设计阶段也可以把索引设计上。前提是了解需要使用那些sql语句。
2、联合索引尽量覆盖条件
比如可以设计一个或者两三个联合索引(尽量少建单值索引),让每一个联合索引都尽量去包含sql语句里的where、order by、group by的字段,还要确保这些联合索引的字段顺序尽量满足sql查询的最左前缀原则。
3、不要在小基数字段上建立索引
索引基数是指这个字段在表里总共有多少个不同的值,比如一张表总共100万行记录,其中有个性别字段,其值不是男就是女,那么该字段的基数就是2。
如果对这种小基数字段建立索引的话,还不如全表扫描了,因为你的索引树里就包含男和女两种值,根本没法进行快速的二分查找,那用索引就没有太大的意义了。
一般建立索引,尽量使用那些基数比较大的字段,就是值比较多的字段,那么才能发挥出B+树快速二分查找的优势来。
4、长字符串我们可以采用前缀索引
尽量对字段类型较小的列设计索引,比如说什么tinyint之类的,因为字段类型较小的话,占用磁盘空间也会比较小,此时你在搜索的时候性能也会比较好一点。
当然,这个所谓的字段类型小一点的列,也不是绝对的,很多时候你就是要针对varchar(255)这种字段建立索引,哪怕多占用一些磁盘空间也是有必要的。
对于这种varchar(255)的大字段可能会比较占用磁盘空间,可以稍微优化下,比如针对这个字段的前20个字符建立索引,就是说,对这个字段里的每个值的前20个字符放在索引树里,类似于 KEYindex(name(20),age,position)。
此时你在where条件里搜索的时候,如果是根据name字段来搜索,那么此时就会先到索引树里根据name字段的前20个字符去搜索,定位到之后前20个字符的前缀匹配的部分数据之后,再回到聚簇索引提取出来完整的name字段值进行比对。
但是假如你要是order by name,那么此时你的name因为在索引树里仅仅包含了前20个字符,所以这个排序是没法用上索引的, group by也是同理。所以这里大家要对前缀索引有一个了解。
5、where与order by冲突时优先where
一般这种时候往往都是让where条件去使用索引来快速筛选出来一部分指定的数据,接着再进行排序。因为大多数情况基于索引进行where筛选往往可以最快速度筛选出你要的少部分数据,然后做排序的成本可能会小很多。
6、基于慢sql查询做优化
可以根据监控后台的一些慢sql,针对这些慢sql查询做特定的索引优化。慢sql的监控方式有很多,这里大家可以去了解了解,目的主要是获取那些慢sql然后进行分析和优化。
相关文章:

四、[mysql]索引优化-1
目录 前言一、场景举例1.联合索引第一个字段用范围查询不走索引(分情况)2.强制走指定索引3.覆盖索引优化4.in和or在表数据量比较大的情况会走索引,在表记录不多的情况下会选择全表扫描5.like 后% 一般情况都会走索引(索引下推) 二、Mysql如何选择合适的索…...

PyTorch入门学习(九):神经网络-最大池化使用
目录 一、数据准备 二、创建神经网络模型 三、可视化最大池化效果 一、数据准备 首先,需要准备一个数据集来演示最大池化层的应用。在本例中,使用了CIFAR-10数据集,这是一个包含10个不同类别图像的数据集,用于分类任务。我们使…...
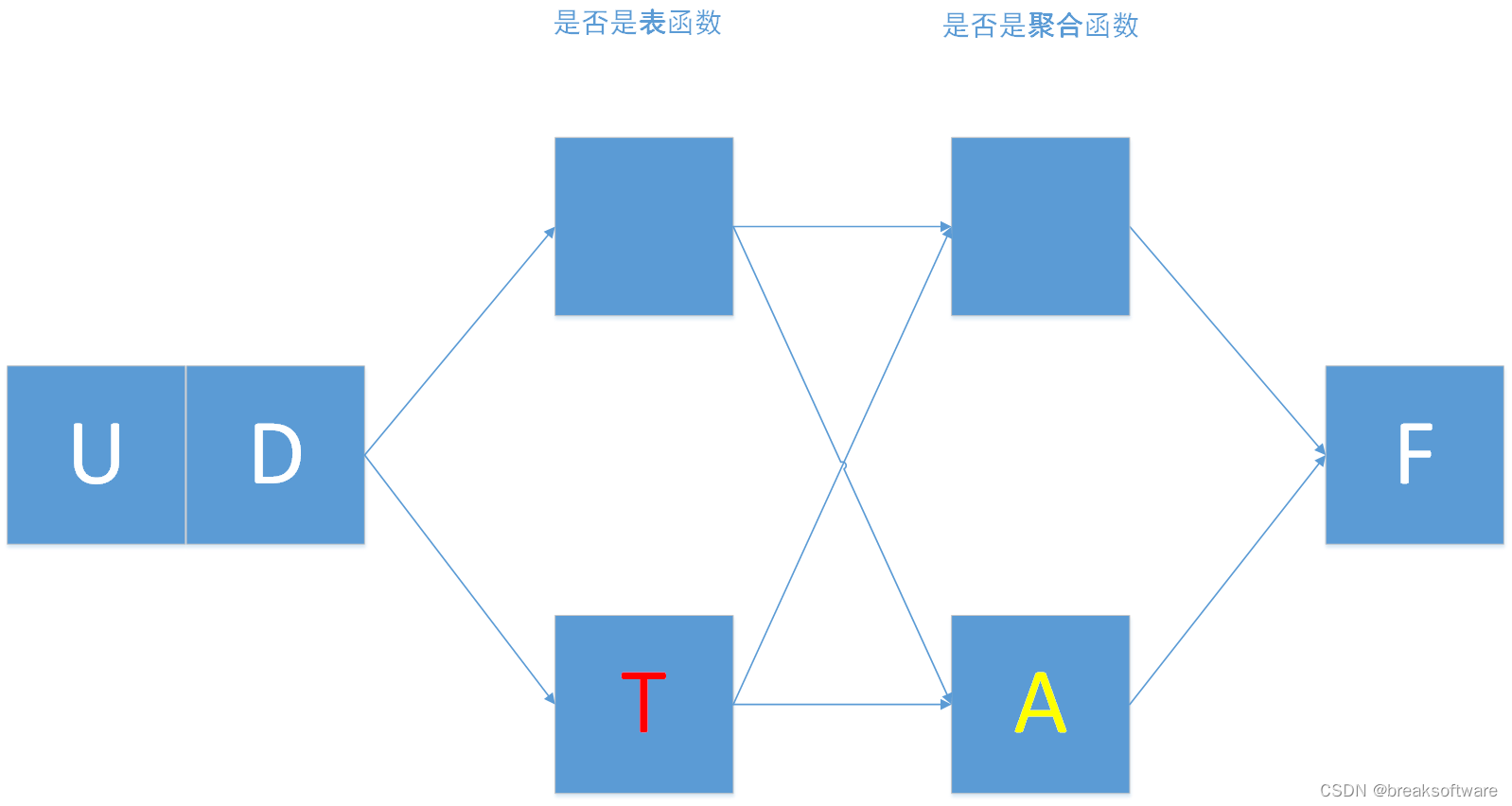
0基础学习PyFlink——用户自定义函数之UDF
大纲 标量函数入参并非表中一行(Row)入参是表中一行(Row)alias PyFlink中关于用户定义方法有: UDF:用户自定义函数。UDTF:用户自定义表值函数。UDAF:用户自定义聚合函数。UDTAF&…...

英语小作文模板(06求助+描述;07描述+建议)
06 求助描述: 题目背景及要求 第一段 第二段 第三段 翻译成中文 07 描述+建议: 题目背景及要求 第一段 第二段...

为什么感觉假期有时候比上班还累?
假期比上班还累的感觉可能由以下几个原因造成: 计划过度:在假期里,人们往往会制定各种计划,如旅游、聚会、休息等,以充分利用这段时间。然而,如果这些计划过于紧张或安排得过于紧密,就会导致身…...
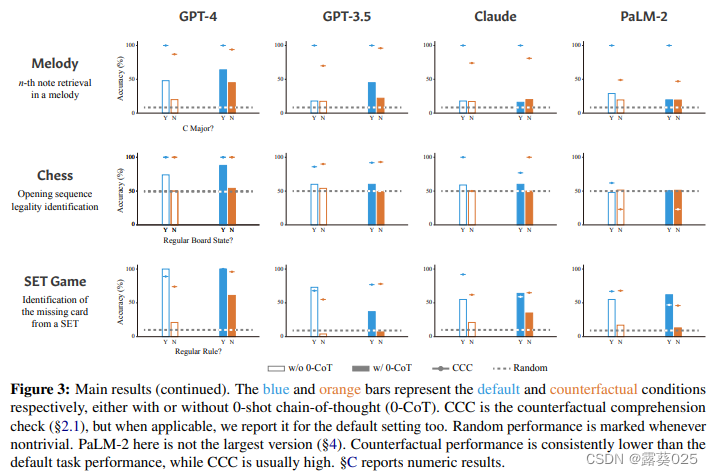
推理还是背诵?通过反事实任务探索语言模型的能力和局限性
推理还是背诵?通过反事实任务探索语言模型的能力和局限性 摘要1 引言2 反事实任务2.1 反事实理解检测 3 任务3.1 算术3.2 编程3.3 基本的句法推理3.4 带有一阶逻辑的自然语言推理3.5 空间推理3.6 绘图3.7 音乐3.8 国际象棋 4 结果5 分析5.1 反事实条件的“普遍性”5…...
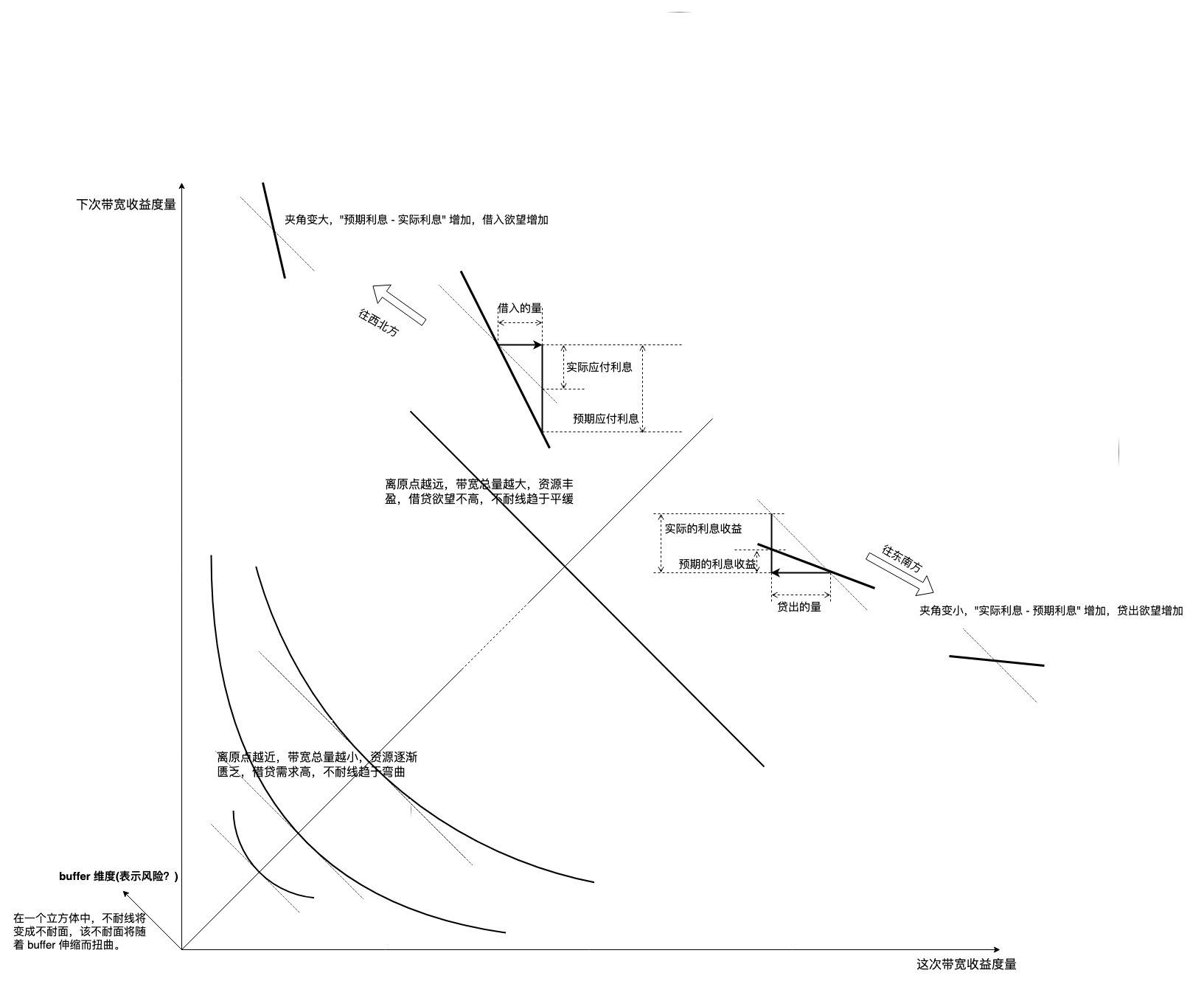
《利息理论》指导 TCP 拥塞控制
欧文费雪《利息原理》第 10 章,第 11 章对利息的几何说明是普适的,任何一个负反馈系统都能引申出新结论。给出原书图示,本文依据于此,详情参考原书: 将 burst 看作借贷是合理的,它包含成本(报文)…...

Bsdiff,Bspatch 的差分增量升级(基于Win和Linux)
目录 背景 内容 准备工作 在windows平台上 在linux平台上 正式工作 生成差分文件思路 作用差分文件思路 在保持相同目录结构进行差分增量升级 服务端(生成差分文件) 客户端(作用差分文件) 背景 像常见的Android 的linux平台,游戏,系统更新都…...
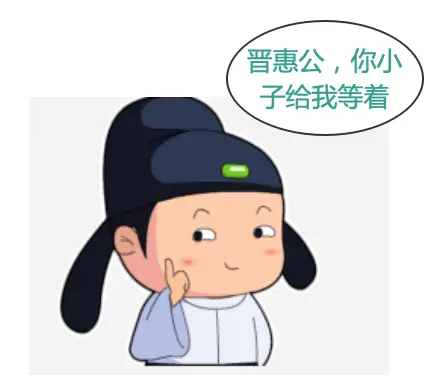
【3妹教我学历史-秦朝史】2 秦穆公-韩原之战
插: 前些天发现了一个巨牛的人工智能学习网站,通俗易懂,风趣幽默,忍不住分享一下给大家。点击跳转到网站。 坚持不懈,越努力越幸运,大家一起学习鸭~~~ 3妹:2哥,今天下班这么早&#…...

车载控制器
文章目录 车载控制器电动汽车上都有什么ECU 车载控制器 智能汽车上的控制器数量因车型和制造商而异。一般来说,现代汽车可能有50到100个电子控制单元(ECU)或控制器。这些控制器负责管理各种系统,如发动机管理、刹车、转向、空调、…...
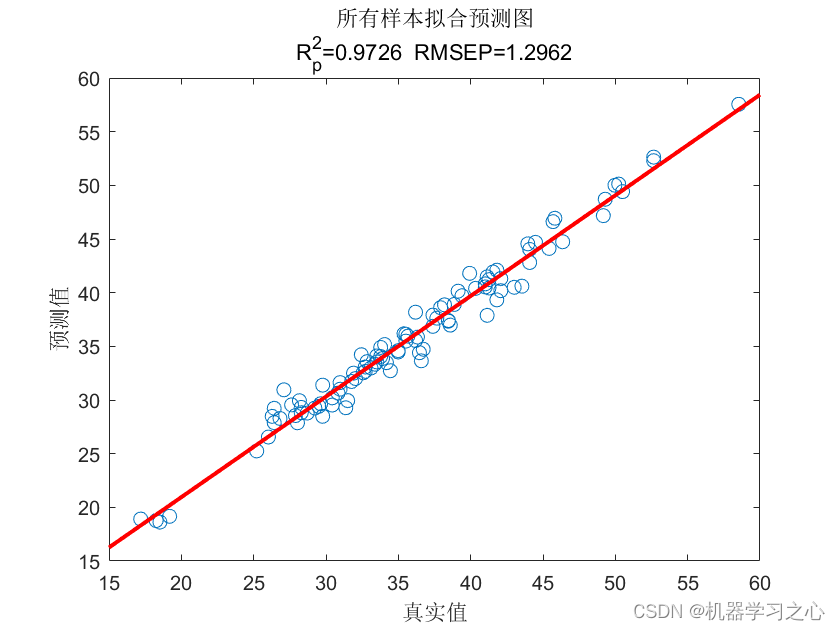
回归预测 | Matlab实现RIME-CNN-SVM霜冰优化算法优化卷积神经网络-支持向量机的多变量回归预测
回归预测 | Matlab实现RIME-CNN-SVM霜冰优化算法优化卷积神经网络-支持向量机的多变量回归预测 目录 回归预测 | Matlab实现RIME-CNN-SVM霜冰优化算法优化卷积神经网络-支持向量机的多变量回归预测效果一览基本介绍程序设计参考资料 效果一览 基本介绍 1.RIME-CNN-SVM霜冰优化算…...
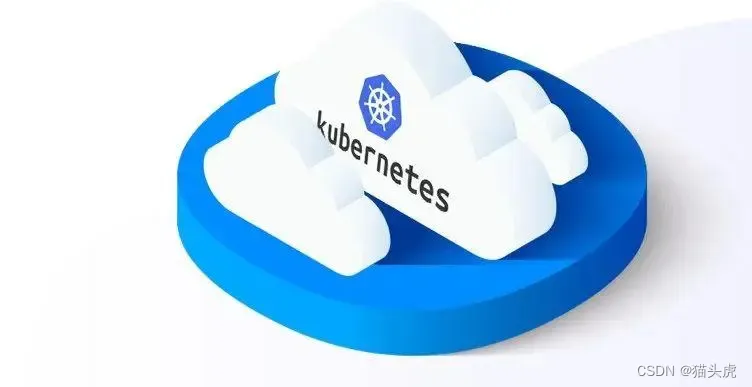
使用Jaeger进行分布式跟踪:学习如何在服务网格中使用Jaeger来监控和分析请求的跟踪信息
🌷🍁 博主猫头虎 带您 Go to New World.✨🍁 🦄 博客首页——猫头虎的博客🎐 🐳《面试题大全专栏》 文章图文并茂🦕生动形象🦖简单易学!欢迎大家来踩踩~🌺 &a…...

添加多个单元对象
开发环境: Windows 11 家庭中文版Microsoft Visual Studio Community 2019VTK-9.3.0.rc0vtk-example参考代码 demo解决问题:不同阶段添加多个单元对象。 定义一个点集和一个单元集合,单元的类型可以是点、三角形、矩形、多边形等基本图形。只…...
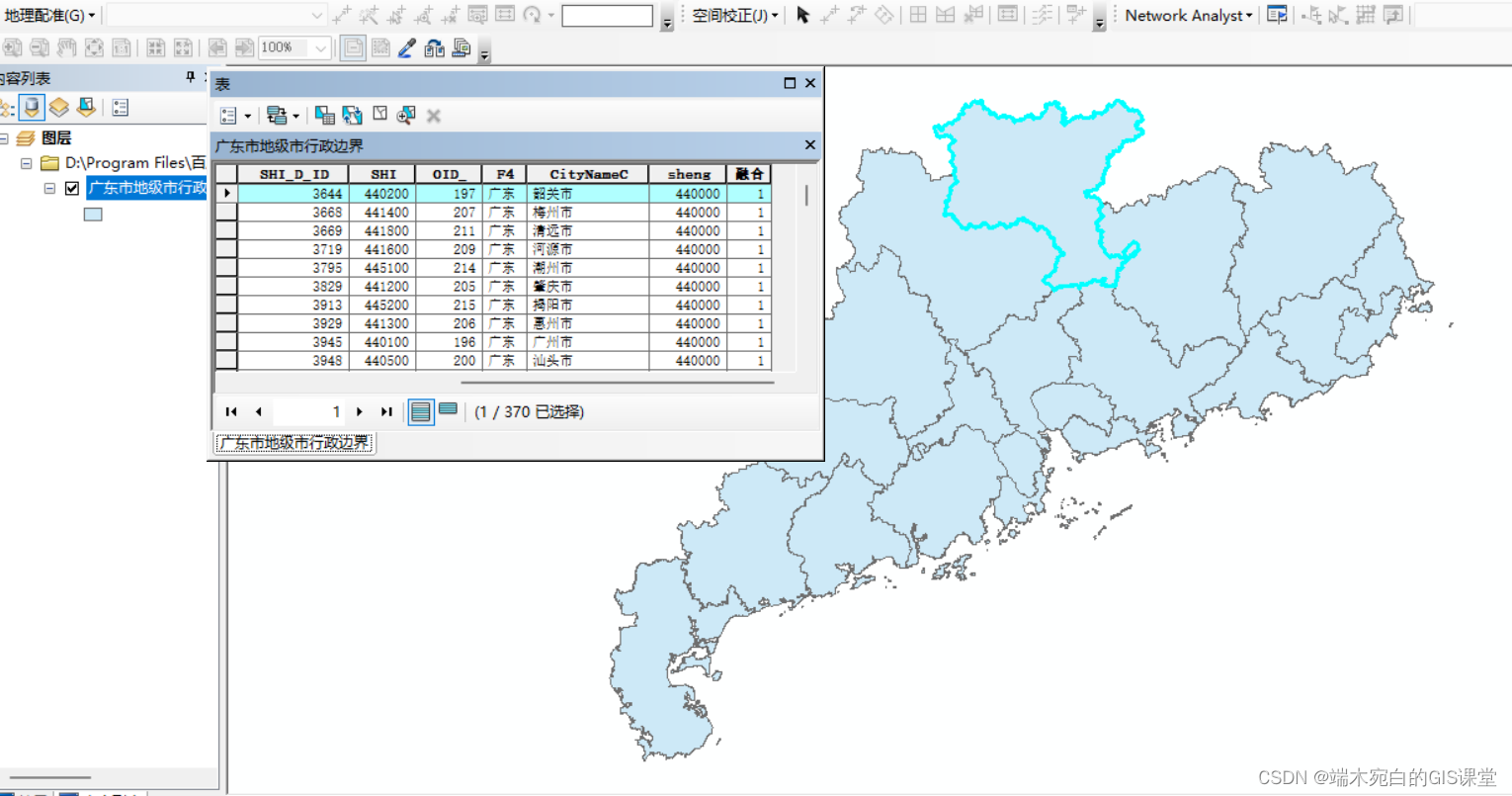
十八、模型构建器(ModelBuilder)快速提取城市建成区——批量掩膜提取夜光数据、夜光数据转面、面数据融合、要素转Excel(基于参考比较法)
一、前言 前文实现批量投影栅格、转为整型,接下来重点实现批量提取夜光数据,夜光数据转面、夜光数据面数据融合、要素转Excel。将相关结果转为Excel,接下来就是在Excel中进行阈值的确定,阈值确定无法通过批量操作,除非采用其他方式,但是那样的学习成本较高,对于参考比较…...
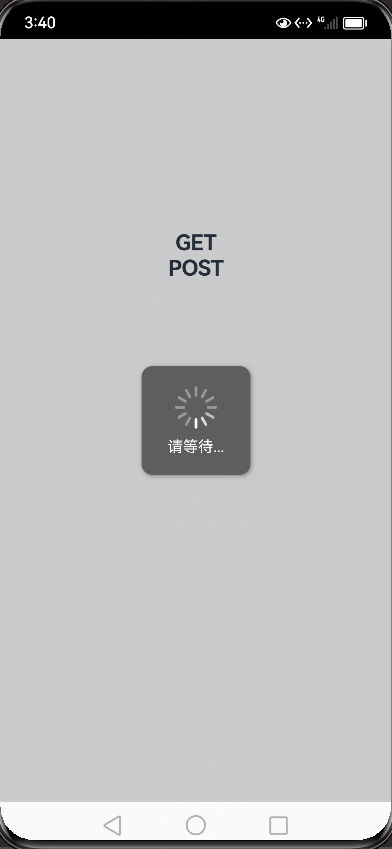
HarmonyOS开发:基于http开源一个网络请求库
前言 网络封装的目的,在于简洁,使用起来更加的方便,也易于我们进行相关动作的设置,如果,我们不封装,那么每次请求,就会重复大量的代码逻辑,如下代码,是官方给出的案例&am…...
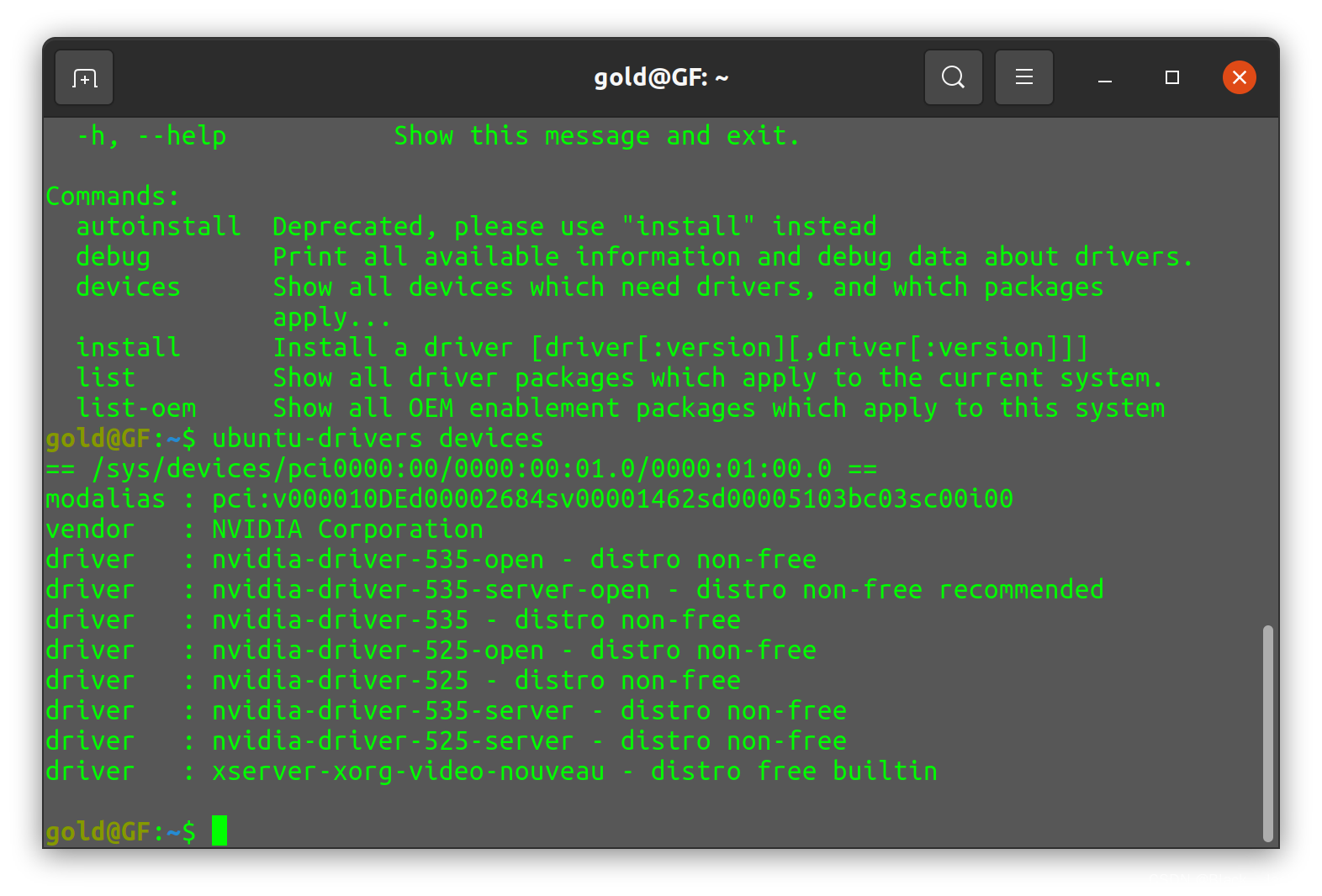
【杂记】Ubuntu20.04装系统,安装CUDA等
装20.04系统 安装系统的过程中,ROG的B660G主板,即使不关掉Secure boot也是可以的,不会影响正常安装,我这边出现问题的主要原因是使用了Ventoy制作的系统安装盘,导致每次一选择使用U盘的UEFI启动,就会跳回到…...

040-第三代软件开发-全新波形抓取算法
第三代软件开发-全新波形抓取算法 文章目录 第三代软件开发-全新波形抓取算法项目介绍全新波形抓取算法代码小解 关键字: Qt、 Qml、 抓波、 截获、 波形 项目介绍 欢迎来到我们的 QML & C 项目!这个项目结合了 QML(Qt Meta-Object …...
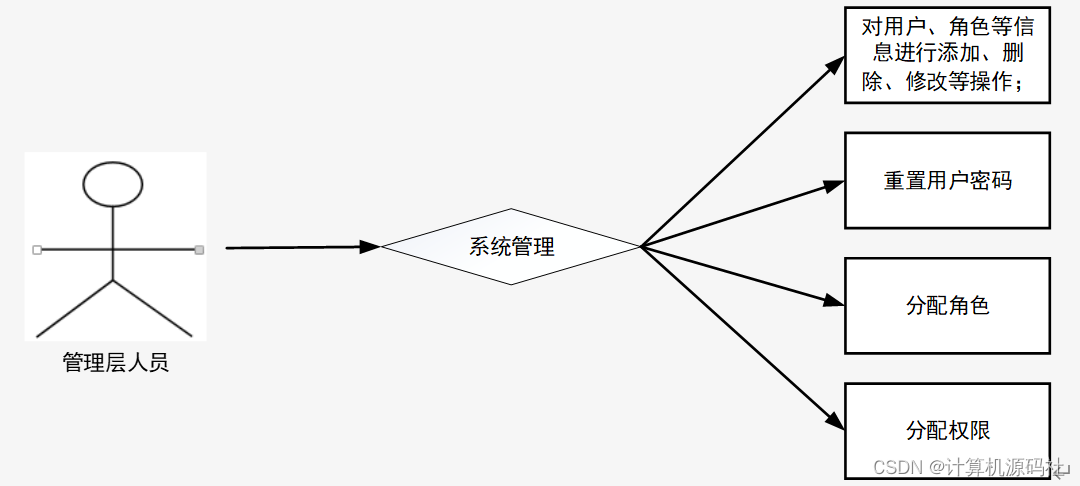
分享一个基于asp.net的供销社农产品商品销售系统的设计与实现(源码调试 lw开题报告ppt)
💕💕作者:计算机源码社 💕💕个人简介:本人七年开发经验,擅长Java、Python、PHP、.NET、微信小程序、爬虫、大数据等,大家有这一块的问题可以一起交流! 💕&…...
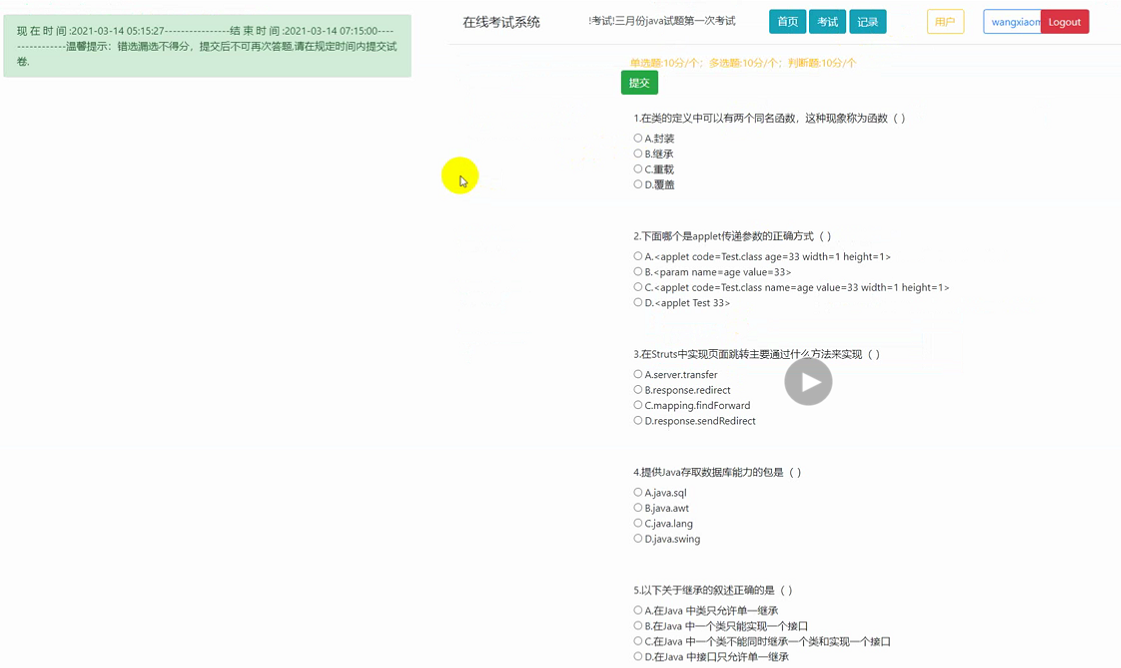
Java基于SpringBoot的线上考试系统
1 摘 要 基于 SpringBoot 的在线考试系统网站,功能模块具有课程管理、成绩管理、教师管理、学生管理、考试管理以及基本信息的管理等,通过将系统分为管理员、授课教师以及学生,从不同的身份角度来对用户提供便利,将科技与教学模式…...

flask socketio 实时传值至html上【需补充实例】
目前版本如下 Flask-Cors 4.0.0 Flask-SocketIO 5.3.6from flask_socketio import SocketIO, emit 跨域问题网上的普通方法无法解决。 参考这篇文章解决 Flask教程(十九)SocketIO - 迷途小书童的Note迷途小书童的Note (xugaoxiang.com) app Flask(__name__) socketio Sock…...
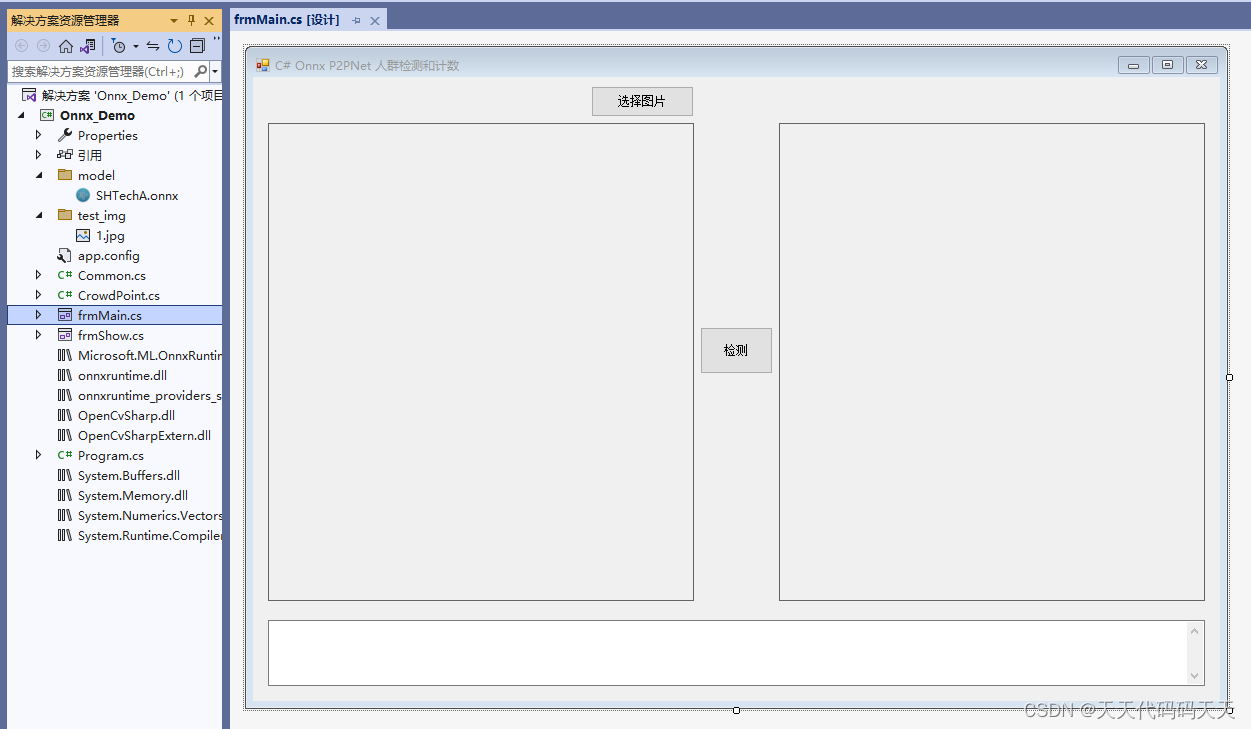
C# Onnx P2PNet 人群检测和计数
效果 项目 代码 using Microsoft.ML.OnnxRuntime; using Microsoft.ML.OnnxRuntime.Tensors; using OpenCvSharp; using System; using System.Collections.Generic; using System.Drawing; using System.Linq; using System.Text; using System.Windows.Forms;namespace Onnx…...
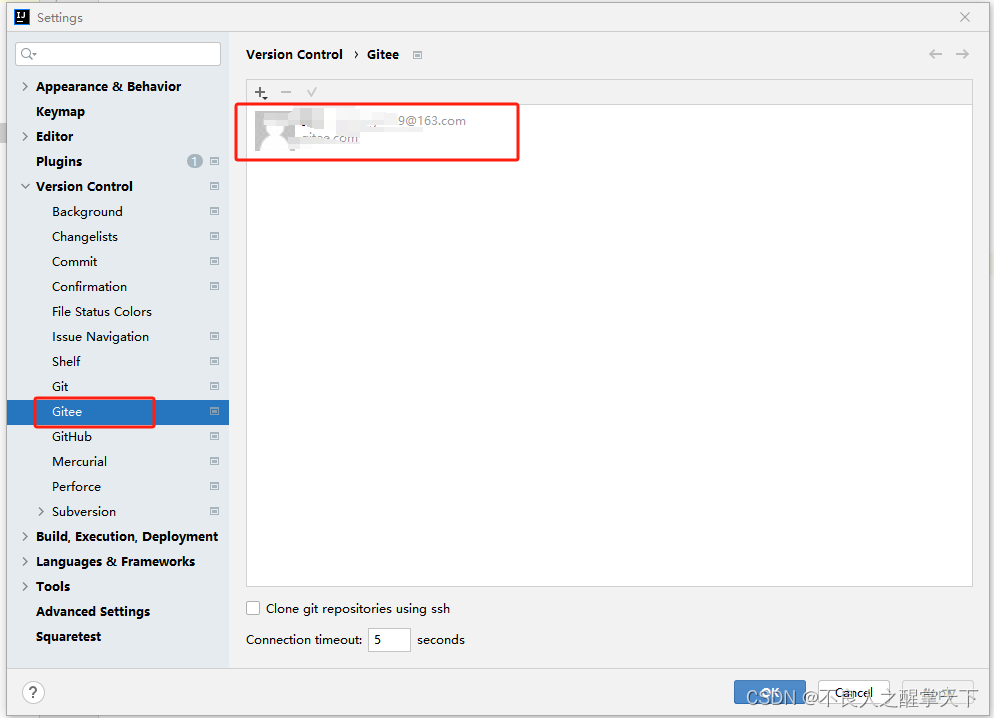
idea提交代码一直提示 log into gitee
解决idea提交代码一直提示 log into gitee问题 文章目录 打开setting->Version control->gitee,删除旧账号,重新配置账号,删除重新登录就好 打开setting->Version control->gitee,删除旧账号,重新配置账号,删除重新登…...
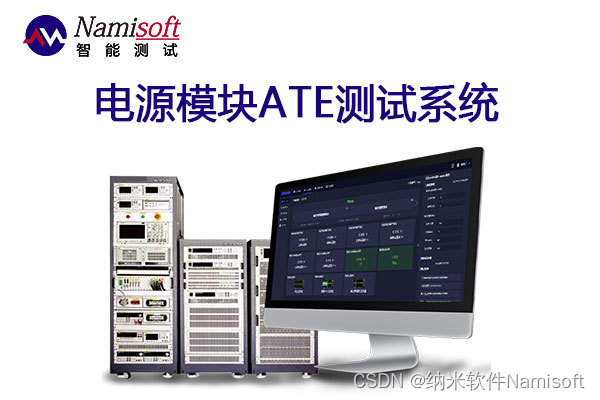
ATECLOUD如何进行电源模块各项性能指标的测试?
ATECLOUD平台进行电源模块各项性能指标的测试是通过以下步骤实现的: 连接测试设备:将测试设备与云计算服务器连接,实现数据采集和远程控制。测试设备包括示波器、电子负载、电源、万用表等,这些设备通过纳米BOX连接到云测试平台上…...

Mysql查询训练——50道题
--1.学生表 Student(SId,Sname,Sage,Ssex) --SId 学生编号,Sname 学生姓名,Sage 出生年月,Ssex 学生性别 --2.课程表 Course(CId,Cname,TId) --CId 课程编号,Cname 课程名称,TId 教师编号 --3.教师表 Teacher(TId,Tname) --TId 教师编号,Tname 教师姓名 --4.成绩表 SC(SId…...
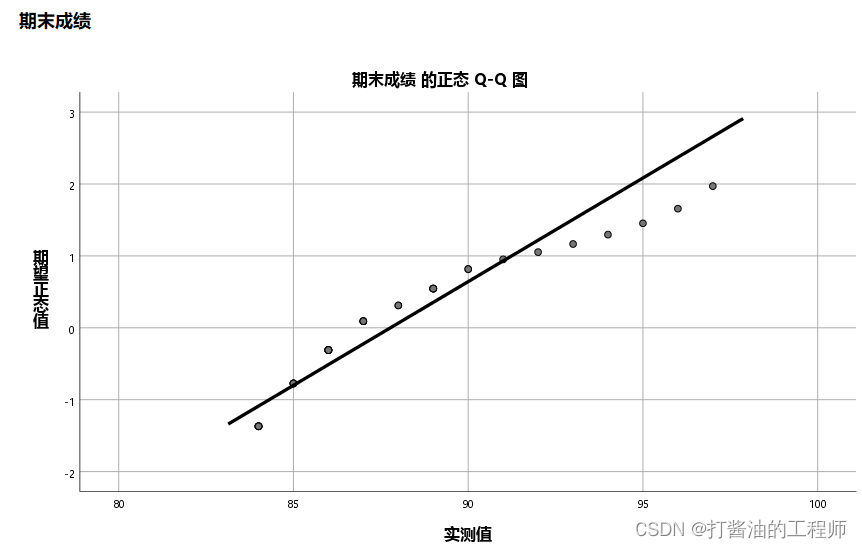
学习笔记|正态分布|图形法|偏度和峰度|非参数检验法|《小白爱上SPSS》课程:SPSS第三讲 | 正态分布怎么检验?看这篇文章就够了
目录 学习目的软件版本原始文档为什么要假设它服从正态分布呢?t检验一、图形法1、频数分布直方图解读 2、正态Q-Q图操作解读 3、正态P-P图SPSS实战操作解读 二、偏度和峰度解读: 三、非参数检验法注意事项 四、规范表达五、小结划重点 学习目的 SPSS第三讲 | 正态…...
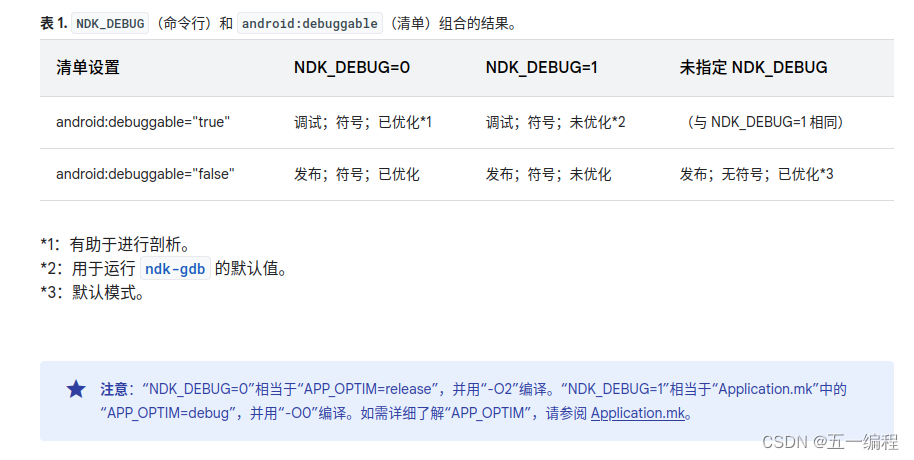
Android NDK开发详解之ndk-build 脚本
Android NDK开发详解之ndk-build 脚本 内部原理从命令行调用选项可调试 build 与发布 build要求 ndk-build 脚本使用 NDK 的基于 Make 的构建系统构建项目。我们针对 ndk-build 使用的 Android.mk 和 Application.mk 配置提供了更具体的文档。 内部原理 运行 ndk-build 脚本相…...
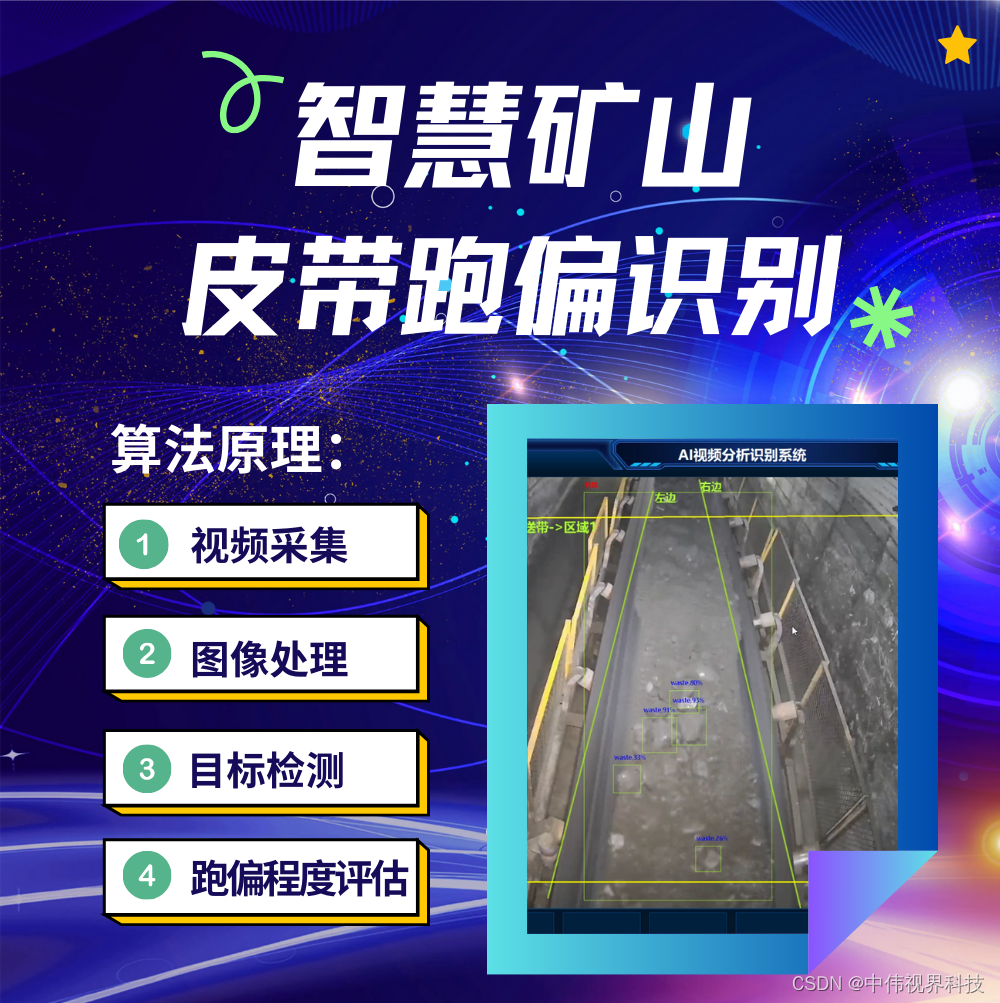
应用于智慧矿山的皮带跑偏视频分析AI算法
一、引言 随着科技的发展,人工智能技术已经在各个领域得到广泛应用。而在智慧矿山领域,皮带跑偏视频分析是其中一个重要的应用方向。本文将详细介绍皮带跑偏视频分析AI算法的原理,以期为智慧矿山的发展提供有益的参考。 二、算法原理 1. 视…...

vue3 UI组件优化之element-plus按需导入
如果不在意项目打包体积大小,正常来讲element-plus 是这样用的 import ElementPlus from element-plus //引入样式 import "element-plus/dist/index.css";app.use(ElementPlus);但是呢要是项目就用了几个弹窗提示什么的,全局引入包体积很大 …...
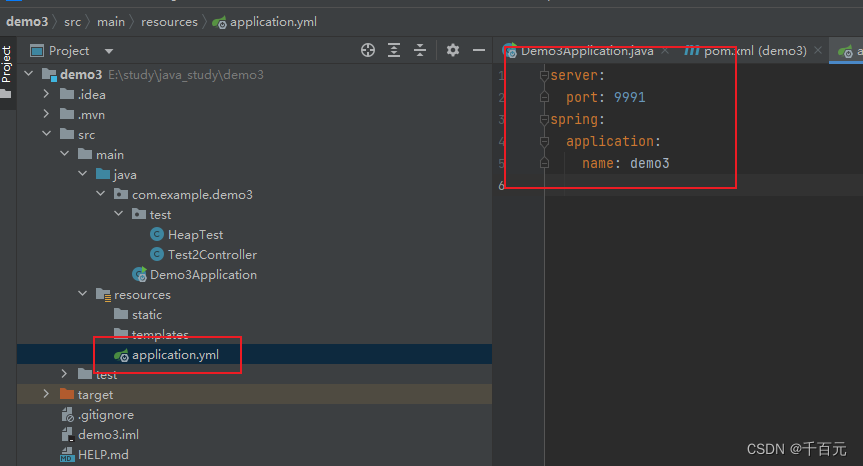
如何创建 Spring Boot 项目
如果有pom.xml有插件异常,可以先删除。 maven配置要配置好 然后yaml,再启动就行 server:port: 9991 spring:application:name: demo3参考 如何创建 Spring Boot 项目_创建springboot项目_良月初十♧的博客-CSDN博客...
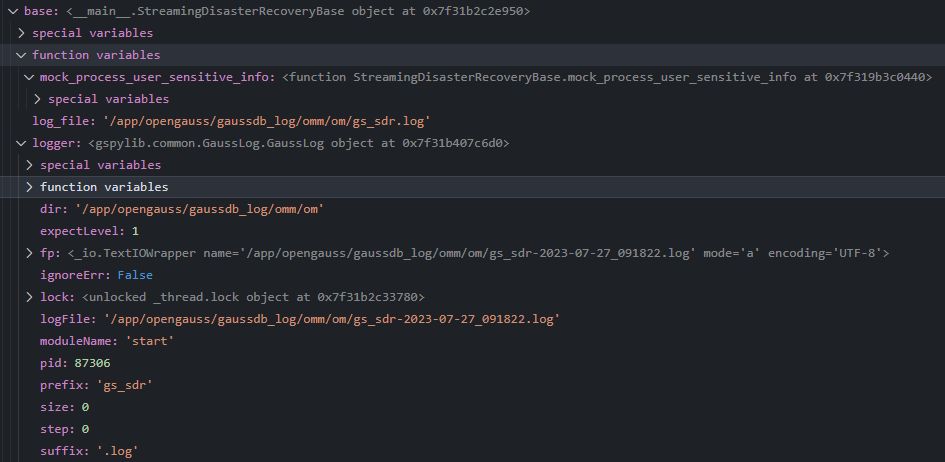
【经验分享】openGauss容灾集群搭建
gs_sdr命令代码解读 背景 openGauss推出了容灾架构,相比之前的一个集群主从架构,而容灾架构是两个集群间的数据同步。为了更深入了解其原理,本文试图通过阅读gs_sdr命令相关的代码来学习下相关的各种操作。 1.容灾搭建过程可以参考…...