Child Mind Institute - Detect Sleep States(2023年第一次Kaggle拿到了银牌总结)
感谢
感谢艾兄(大佬带队)、rich师弟(师弟通过这次比赛机械转码成功、耐心学习)、张同学(也很有耐心的在学习),感谢开源方案(开源就是银牌),在此基础上一个月不到收获到了很多,运气很好。这个是我们比赛的总结:
我们队Kaggle CMI银牌方案,欢迎感兴趣的伙伴upvote:https://www.kaggle.com/competitions/child-mind-institute-detect-sleep-states/discussion/459610
计划 (系统>结果,稳健>取巧)
团队计划表,每个人做的那部分工作,避免重复,方便交流,提高效率,这个工作表起了很大的作用。
具体方案
75th Place Detailed Solution - Spec2DCNN + CenterNet + Transformer + NMS
First of all, I would like to thank @tubotubo for sharing your high-quality code, and also thank my teammates @liruiqi577 @brickcoder @xtzhou for their contributions in the competition. Here, I am going to share our team’s “snore like thunder” solution from the following aspects:
- Data preprocessing
- Feature Engineering
- Model
- Post Processing
- Model Ensemble
1. Data preprocessing
We made EDA and readed open discussions found that there are 4 types of data anomalies:
- Some series have a high missing rate and some of them do not even have any event labels;
- In some series , there are no event annotations in the middle and tail (possibly because the collection activity has stopped);
- The sleep record is incomplete (a period of sleep is only marked with onset or wakeup).
- There are outliers in the enmo value.
To this end, we have some attempts, such as:
- Eliminate series with high missing rates;
- Cut the tail of the series without event labels;
- Upper clip enmo to 1.
But the above methods didn't completely work. In the end, our preprocessing method was:
We split the dataset group by series into 5 folds. For each fold, we eliminate series with a label missing rate of 100% in the training dataset while without performing any data preprocessing on the validation set. This is done to avoid introducing noise to the training set, and to ensure that the evaluation results of the validation set are more biased towards the real data distribution, which improve our LB score + 0.006.
Part of our experiments as below:
Experiment | Fold0 | Public (single fold) | Private (5-fold) |
---|---|---|---|
No preprocess missing data | 0.751 | 0.718 | 0.744 |
Eliminate unlabeled data at the end of train_series & series with missing rate >80% | 0.739 | 0.709 | 0.741 |
Drop train series which don’t have any event labels | 0.752 | 0.724 | 0.749 |
2. Feature Engineering
- Sensor features: After smoothing the enmo and anglez features, a first-order difference is made to obtain the absolute value. Then replace the original enmo and anglez features with these features, which improve our LB score + 0.01.
train_series['enmo_abs_diff'] = train_series['enmo'].diff().abs()
train_series['enmo'] = train_series['enmo_abs_diff'].rolling(window=5, center=True, min_periods=1).mean()
train_series['anglez_abs_diff'] = train_series['anglez'].diff().abs()
train_series['anglez'] = train_series['anglez_abs_diff'].rolling(window=5, center=True, min_periods=1).mean()
- Time features: sin and cos hour.
In addition, we also made the following features based on open notebooks and our EDA, such as: differential features with different orders, rolling window statistical features, interactive features of enmo and anglez (such as anglez's differential abs * enmo, etc.), anglez_rad_sin/cos, dayofweek/is_weekend (I find that children have different sleeping habits on weekdays and weekends). But strangely enough, too much feature engineering didn’t bring us much benefit.
Experiment | Fold0 | Public (5-fold) | Private (5-fold) |
---|---|---|---|
anglez + enmo + hour_sin + hour_cos | 0.763 | 0.731 | 0.768 |
anglez_abs_diff + enmo_abs_diff + hour_sin + hour_cos | 0.771 | 0.741 | 0.781 |
3. Model
We used 4 models:
- CNNSpectrogram + Spec2DCNN + UNet1DDecoder;
- PANNsFeatureExtractor + Spec2DCNN + UNet1DDecoder.
- PANNsFeatureExtractor + CenterNet + UNet1DDecoder.
- TransformerAutoModel (xsmall, downsample_rate=8).
Parameter Tunning: Add more kernel_size 8 for CNNSpectrogram can gain +0.002 online.
Multi-Task Learning Objectives: sleep status, onset, wake.
Loss Function: For Spec2DCNN and TransformerAutoModel, we use BCE, but with multi-task target weighting, sleep:onset:wake = 0.5:1:1. The purpose of this is to allow the model to focus on learning the last two columns. We tried to train only for the onset and wake columns, but the score was not good. The reason is speculated that the positive samples in these two columns are sparse, and MTL needs to be used to transfer the information from positive samples in the sleep status to the prediction of sleep activity events. Also, I tried KL Loss but it didn't work that well.
self.loss_fn = nn.BCEWithLogitsLoss(pos_weight=torch.tensor([0.5,1.,1.]))
At the same time, we adjusted epoch to 70 and added early stopping with patience=15. The early stopping criterion is the AP of the validation dataset, not the loss of the validation set. batch_size=32.
Experiment | Fold0 | Public (single fold) | Private (5-fold) |
---|---|---|---|
earlystop by val_loss | 0.750 | 0.697 | 0.742 |
earlystop by val_score | 0.751 | 0.718 | 0.744 |
loss_wgt = 1:1:1 | 0.752 | 0.724 | 0.749 |
loss_wgt = 0.5:1:1 | 0.755 | 0.723 | 0.753 |
Note: we used the model_weight.pth with the best offline val_score to submit our LB instead of using the best_model.pth with the best offline val_loss。
4. Post Processing
Our post-processing mainly includes:
- find_peaks(): scipy.signal.find_peaks;
- NMS: This task can be treated as object detection. [onset, wakeup] is regarded as a bounding boxes, and score is the confident of the box. Therefore, I used a time-series NMS. Using NMS can eliminate redundant boxes with high IOU, which increase our AP.
def apply_nms(dets_arr, thresh):x1 = dets_arr[:, 0]x2 = dets_arr[:, 1]scores = dets_arr[:, 2]areas = x2 - x1order = scores.argsort()[::-1]keep = []while order.size > 0:i = order[0]keep.append(i)xx1 = np.maximum(x1[i], x1[order[1:]])xx2 = np.minimum(x2[i], x2[order[1:]])inter = np.maximum(0.0, xx2 - xx1 + 1)ovr = inter / (areas[i] + areas[order[1:]] - inter)inds = np.where(ovr <= thresh)[0]order = order[inds + 1]dets_nms_arr = dets_arr[keep,:]onset_steps = dets_nms_arr[:, 0].tolist()wakeup_steps = dets_nms_arr[:, 1].tolist()nms_save_steps = np.unique(onset_steps + wakeup_steps).tolist()return nms_save_steps
In addition, we set score_th=0.005 (If it is set too low, a large number of events will be detected and cause online scoring errors, so it is fixed at 0.005 here), and use optuna to simultaneously search the parameter distance in find_peaks and the parameter iou_threshold of NMS. Finally, when distance=72 and iou_threshold=0.995, the best performance is achieved.
import optunadef objective(trial):score_th = 0.005 # trial.suggest_float('score_th', 0.003, 0.006)distance = trial.suggest_int('distance', 20, 80)thresh = trial.suggest_float('thresh', 0.75, 1.)# find peakval_pred_df = post_process_for_seg(keys=keys,preds=preds[:, :, [1, 2]],score_th=score_th,distance=distance,)# nmsval_pred_df = val_pred_df.to_pandas()nms_pred_dfs = NMS_prediction(val_pred_df, thresh, verbose=False)score = event_detection_ap(valid_event_df.to_pandas(), nms_pred_dfs)return -scorestudy = optuna.create_study()
study.optimize(objective, n_trials=100)
print('Best hyperparameters: ', study.best_params)
print('Best score: ', study.best_value)
Experiment | Fold0 | Pubic (5-fold) | Private (5-fold) |
---|---|---|---|
find_peak | - | 0.745 | 0.787 |
find_peak+NMS+optuna | - | 0.746 | 0.789 |
5. Model Ensemble
Finally, we average the output probabilities of the following models and then feed into the post processing methods to detect events. By the way, I tried post-processing the detection events for each model and then concating them, but this resulted in too many detections. Even with NMS, I didn't get a better score.
The number of ensemble models: 4 (types of models) * 5 (fold number) = 20.
Experiment | Fold0 | Pubic (5-fold) | Private (5-fold) |
---|---|---|---|
model1: CNNSpectrogram + Spec2DCNN + UNet1DDecoder | 0.77209 | 0.743 | 0.784 |
model2: PANNsFeatureExtractor + Spec2DCNN + UNet1DDecoder | 0.777 | 0.743 | 0.782 |
model3: PANNsFeatureExtractor + CenterNet + UNet1DDecoder | 0.75968 | 0.634 | 0.68 |
model4: TransformerAutoModel | 0.74680 | - | - |
model1 + model2(1:1) | - | 0.746 | 0.789 |
model1 + model2+model3(1:1:0.4) | - | 0.75 | 0.786 |
model1 + model2+model3+model4(1:1:0.4:0.2) | 0.752 | 0.787 |
Unfortunately, we only considered CenterNet and Transformer to model ensemble with a tentative attitude on the last day, but surprisingly found that a low-CV-scoring model still has a probability of improving final performance as long as it is heterogeneous compared with your previous models. But we didn’t have more opportunities to submit more, which was a profound lesson for me.
Thoughts not done:
-
Data Augmentation: Shift the time within the batch to increase more time diversity and reduce dependence on hour features.
-
Model: Try more models. Although we try transformer and it didn’t work for us. I am veryyy looking forward to the solutions from top-ranking players.
Thanks again to Kaggle and all Kaggle players. This was a good competition and we learned a lot from it. If you think our solution is useful for you, welcome to upvote and discuss with us.
In addition, this is my first 🥈 silver medal. Thank you everyone for letting me learn a lot. I will continue to work hard. :)
相关文章:
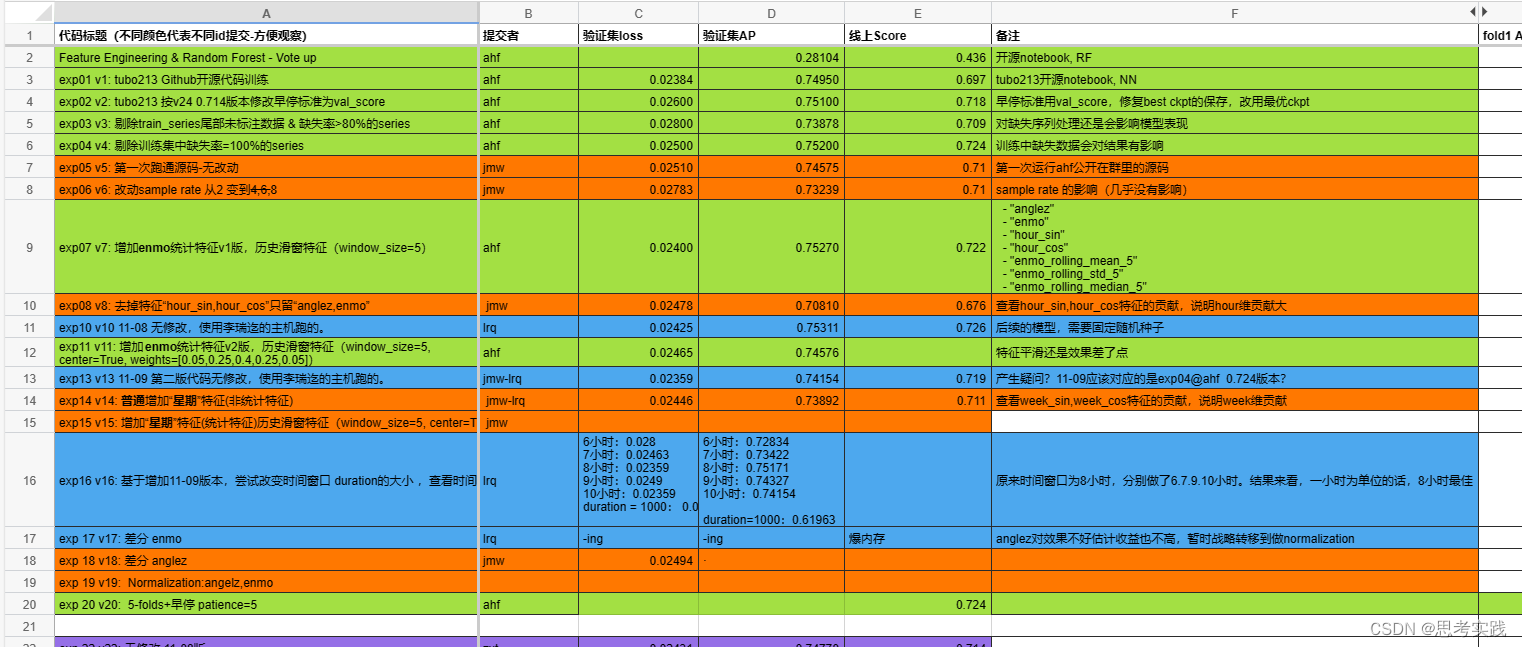
Child Mind Institute - Detect Sleep States(2023年第一次Kaggle拿到了银牌总结)
感谢 感谢艾兄(大佬带队)、rich师弟(师弟通过这次比赛机械转码成功、耐心学习)、张同学(也很有耐心的在学习),感谢开源方案(开源就是银牌),在此基础上一个月…...
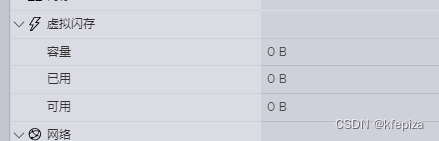
Esxi7Esxi8设置VMFSL虚拟闪存的大小
Esxi7Esxi8设置VMFSL虚拟闪存的大小 ESXi7,8 默认安装会分配一个 VMFSL(VMFS-L)(Local VMFS)很大空间(120G), 感觉很浪费, 实际给 8G 就可以了, 最少 6G , 经实验,给2G没法安装 . Esxi7是虚拟闪存的 修改的方法是: 在安装时修改 设置 autoPartitionOSDataSize8192 在cdromBoo…...
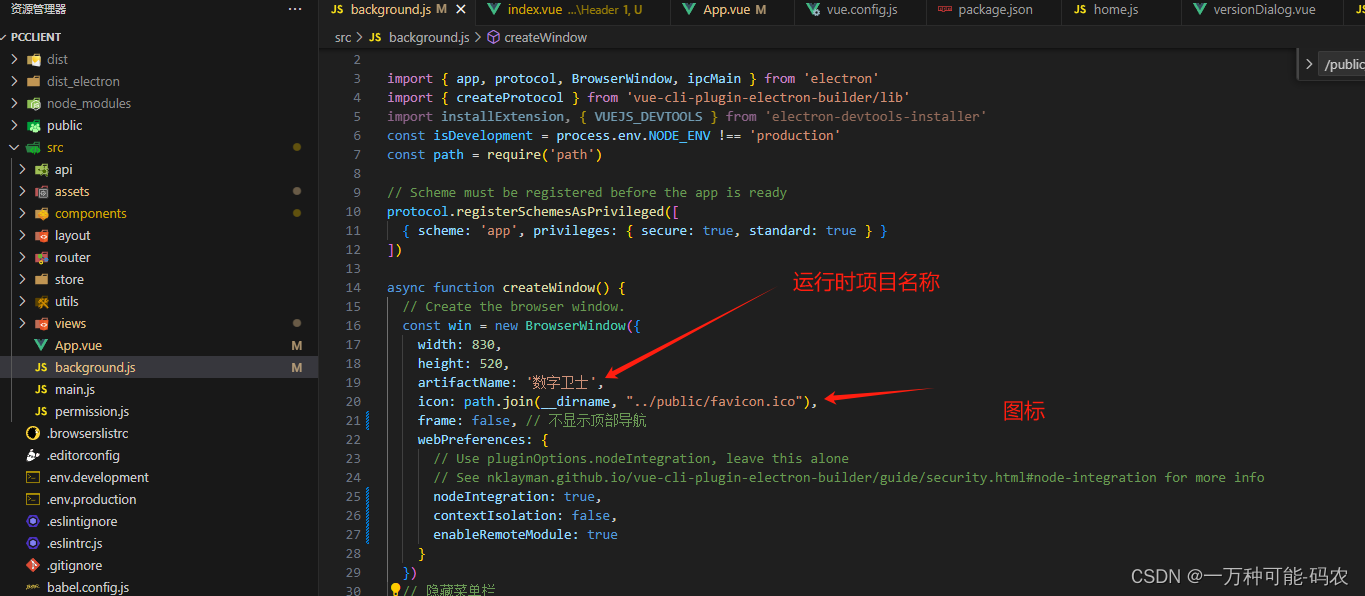
vue2+electron桌面端一体机应用
vue2+electron项目 前言:公司有一个项目需要用Vue转成exe,首先我使用vue-cli脚手架搭建vue2项目,然后安装electron 安装electron 这一步骤可以省略,安装electron-builder时会自动安装electron npm i electron 安装electron-builder vue add electron-builder 项目中多出…...
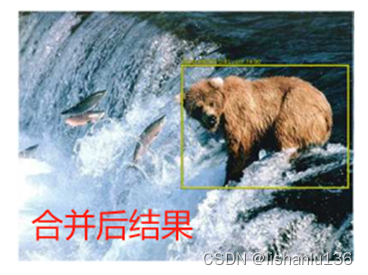
目标检测——OverFeat算法解读
论文:OverFeat: Integrated Recognition, Localization and Detection using Convolutional Networks 作者:Pierre Sermanet, David Eigen, Xiang Zhang, Michael Mathieu, Rob Fergus, Yann LeCun 链接:https://arxiv.org/abs/1312.6229 文章…...
vue获取主机id和IP地址
获取主机id和IP地址 在vue.config.js const os require(“os”); function getNetworkIp() { let needHost “”; // 打开的host try { // 获得网络接口列表 let network os.networkInterfaces(); for (let dev in network) { let iface network[dev]; for (let i 0; i …...
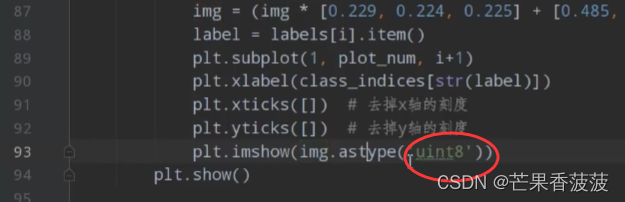
在pytorch中自定义dataset读取数据
这篇是我对哔哩哔哩up主 霹雳吧啦Wz 的视频的文字版学习笔记 感谢他对知识的分享 有关我们数据读取预训练 以及如何将它打包成一个一个batch输入我们的网络的 首先我们来看一下之前我们在讲resnet网络时所使用的源码 我们去使用了官方实现的image folder去读取我们的图像数据 然…...
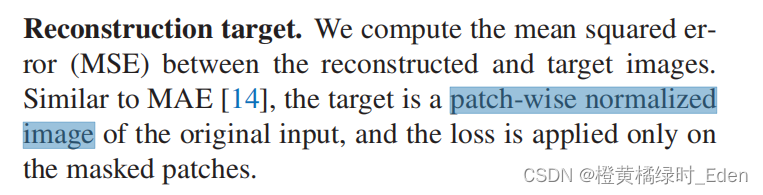
ConvNeXt V2: Co-designing and Scaling ConvNets with Masked Autoencoders
1.关于稀疏卷积的解释:https://zhuanlan.zhihu.com/p/382365889 2. 答案: 在深度学习领域,尤其是计算机视觉任务中,遮蔽图像建模(Masked Image Modeling, MIM)是一种自监督学习策略,其基本思想…...
Java后端的登录、注册接口是怎么实现的
目录 Java后端的登录、注册接口是怎么实现的 Java后端的登录接口是怎么实现的 Java后端的注册接口怎么实现? 如何防止SQL注入攻击? Java后端的登录、注册接口是怎么实现的 Java后端的登录接口是怎么实现的 Java后端的登录接口的实现方式有很多种&a…...
TCP Keepalive 和 HTTP Keep-Aliv
HTTP的Keep-Alive 在http1.0的版本中,它是基于请求-应答模型和TCP协议的,也就是在建立TCP连接后,客户端发送一次请求并且接收到响应后,就会立马断开TCP连接,称为HTTP短连接,这种方式比较耗费时间以及浪费资…...
操作系统 复习笔记
操作系统的目标和作用 操作系统的目标 1.方便性 2.有效性 3.可扩展性 4.开放性 操作系统的作用 1.OS作为用户与计算机硬件系统之间的接口 2.OS作为计算机系统资源的管理者 3.OS实现了对计算机系统资源的抽象 推动操作系统发展的主要动力 1.不断提高计算机系统资源的…...
Java中实现单例模式的方式
1. 使用静态内部类实现单例模式 在Java中,使用静态内部类实现单例模式是一种常见而又有效的方式。这种方式被称为“静态内部类单例模式”或者“Holder模式”。这种实现方式有以下优点: 懒加载(Lazy Initialization):静…...
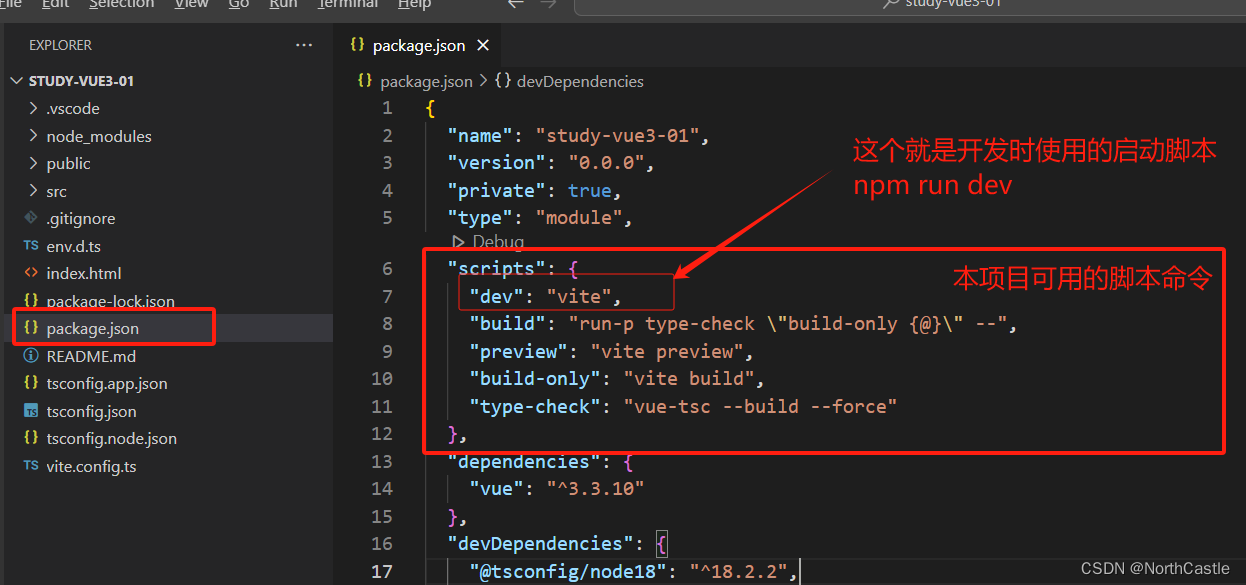
Vue3-01-创建项目
环境准备 1.需要用到 16.0 以及更高版本的 node.js 2.使用vscode编辑器进行项目开发可以在命令行中查看node的版本号: node -v创建项目 1.准备一个目录 例如,我创建项目的时候是在该目录下进行的;D:\projectsTest\vue3project2.执行创建命令(*&#x…...
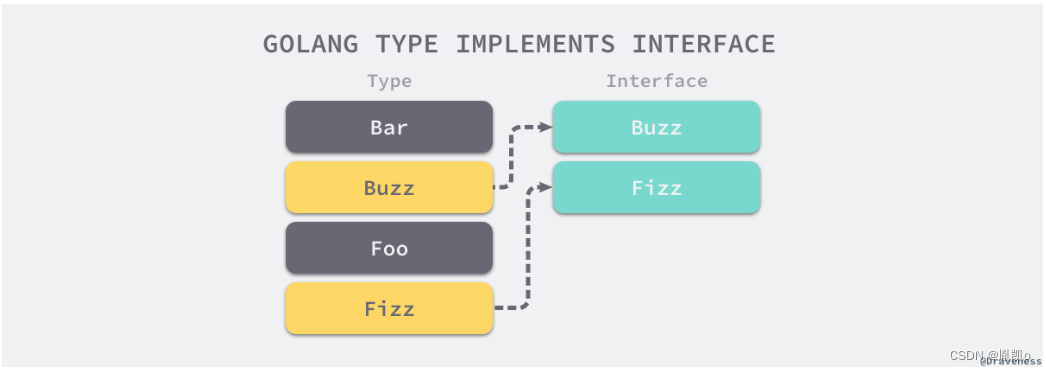
Go 语言中的反射机制
欢迎大家到我的博客浏览,更好的阅读体验请点击 反射 | YinKais Blog 反射在大多数的应用和服务中并不常见,但是很多框架都依赖 Go 语言的反射机制简化代码。<!--more-->因为 Go 语言的语法元素很少、设计简单,所以它没有特别强的表达能…...
[leetcode 前缀和]
525. 连续数组 M :::details 给定一个二进制数组 nums , 找到含有相同数量的 0 和 1 的最长连续子数组,并返回该子数组的长度。 示例 1: 输入: nums [0,1] 输出: 2 说明: [0, 1] 是具有相同数量 0 和 1 的最长连续子数组。示例 2: 输入: nums [0,1,0] 输出: …...
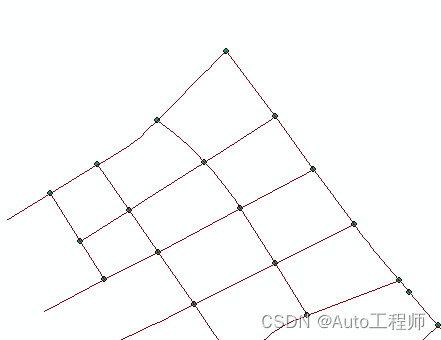
Python与ArcGIS系列(十五)根据距离抓取字段
目录 0 简述1 实例需求2 arcpy开发脚本0 简述 在处理gis数据的时候,会遇到这种需求:将一个图层与另一个图层中相近的要素进行字段赋值。本篇将介绍如何利用arcpy及arcgis的工具箱实现这个功能。 1 实例需求 为了介绍这个功能的实现,我们需要有一个特定的功能需求。在这里选…...
YOLOv8分割训练及分割半自动标注
YOLOv8是基于目标检测算法YOLOv5的改进版,它在YOLOv5的基础上进行了优化和改进,加入了一些新的特性和技术,如切片注意力机制、骨干网络的选择等。 本文以yolov8-seg为基准,主要整理分割训练流程及使用v8分割模型进行半自动标注的过程。 一、v8-seg训练 1.1 环境配置 github…...
jsp页面通过class或者id获取a标签上的属性的值
要通过class和id两种方式获取a标签上的某个属性的值,或者给其赋值,可以使用JavaScript。以下是两种方法的示例: <!DOCTYPE html> <html lang"en"> <head><meta charset"UTF-8"><meta name&q…...
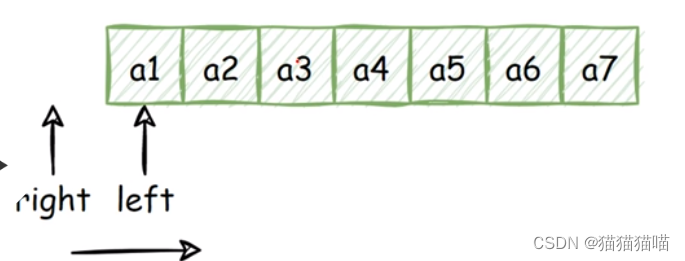
题目:美丽的区间(蓝桥OJ 1372)
题目描述: 解题思路: 采用双指针的快慢指针。 图解 可以采用前缀和,但会相较麻烦。 题解: #include<bits/stdc.h> using namespace std;const int N 1e5 9; int a[N];// 因为是连续区间(连续区间࿱…...
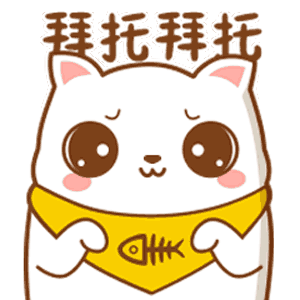
解决:During handling of the above exception, another exception occurred
解决:During handling of the above exception, another exception occurred 文章目录 解决:During handling of the above exception, another exception occurred背景报错问题报错翻译报错位置代码报错原因解决方法参考内容:今天的分享就到…...

计算机基础知识65
cookie和session的使用 # 概念:cookie 是客户端浏览器上的键值对 # 目的:为了做会话保持 # 来源:服务端写入的,服务端再返回的响应头中写入,浏览器会自动取出来 存起来是以key value 形式,有过期时间、path…...
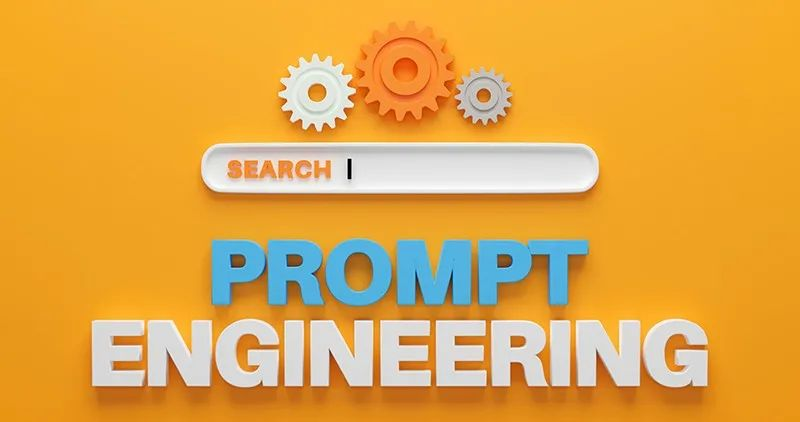
深入剖析AI大模型:大模型时代的 Prompt 工程全解析
今天聊的内容,我认为是AI开发里面非常重要的内容。它在AI开发里无处不在,当你对 AI 助手说 "用李白的风格写一首关于人工智能的诗",或者让翻译模型 "将这段合同翻译成商务日语" 时,输入的这句话就是 Prompt。…...
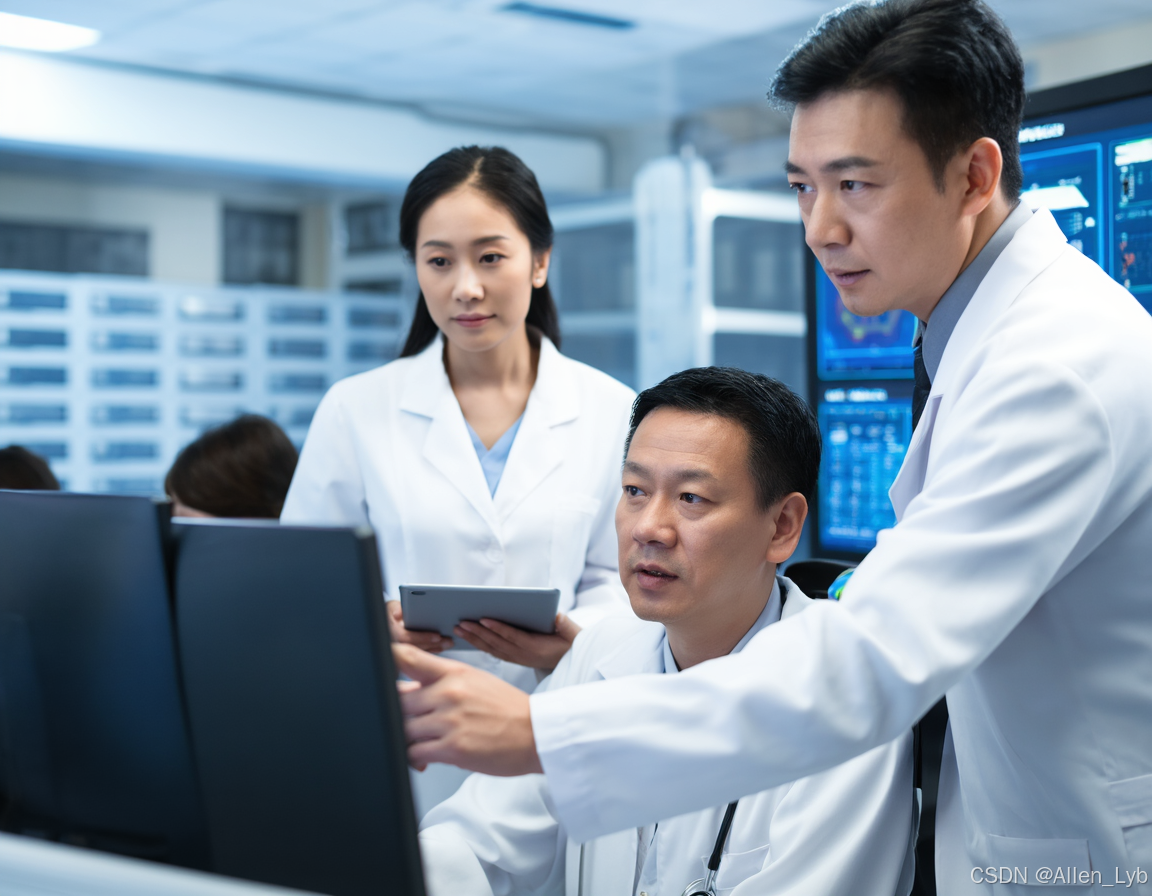
智慧医疗能源事业线深度画像分析(上)
引言 医疗行业作为现代社会的关键基础设施,其能源消耗与环境影响正日益受到关注。随着全球"双碳"目标的推进和可持续发展理念的深入,智慧医疗能源事业线应运而生,致力于通过创新技术与管理方案,重构医疗领域的能源使用模式。这一事业线融合了能源管理、可持续发…...
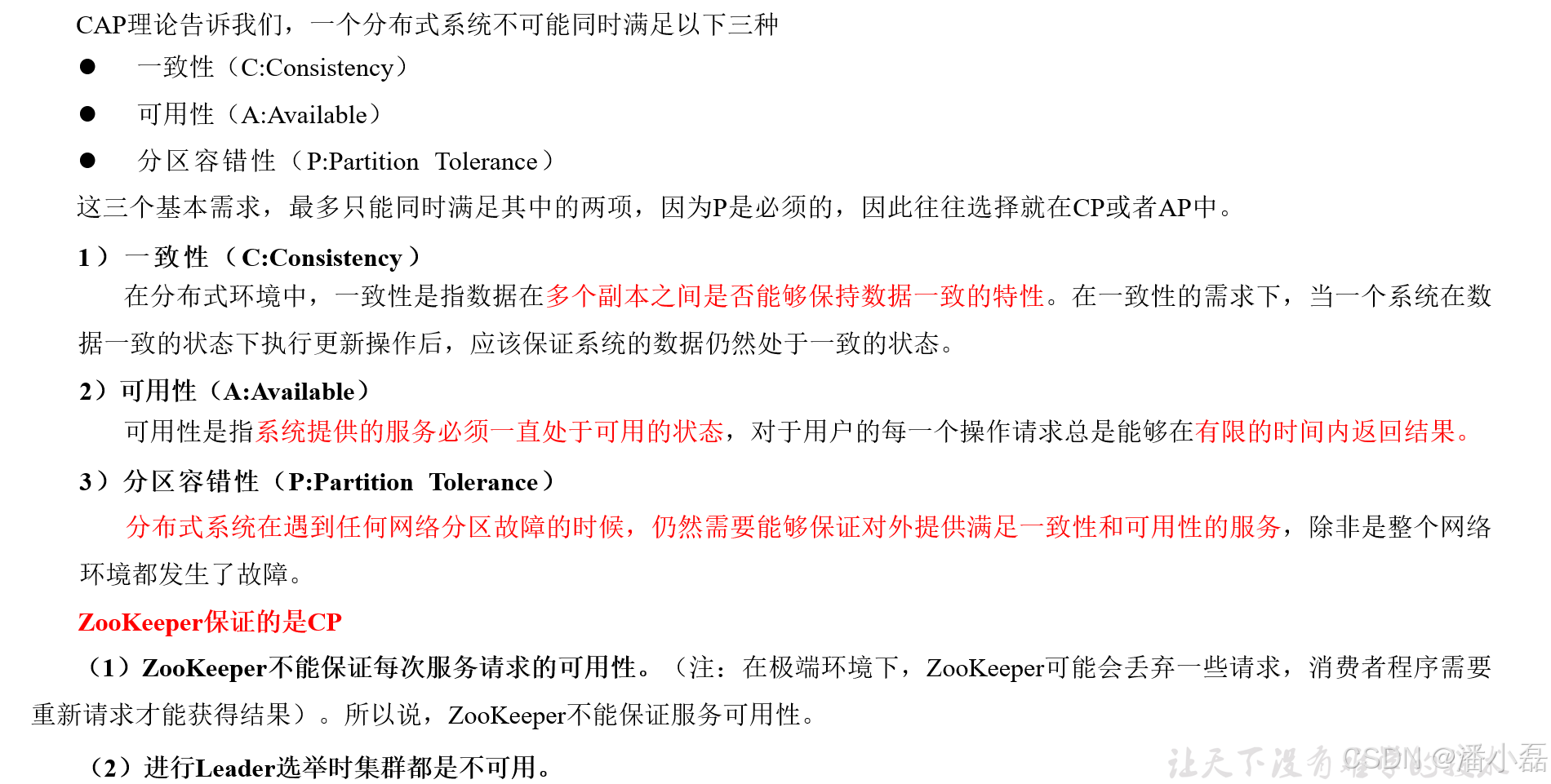
高频面试之3Zookeeper
高频面试之3Zookeeper 文章目录 高频面试之3Zookeeper3.1 常用命令3.2 选举机制3.3 Zookeeper符合法则中哪两个?3.4 Zookeeper脑裂3.5 Zookeeper用来干嘛了 3.1 常用命令 ls、get、create、delete、deleteall3.2 选举机制 半数机制(过半机制࿰…...
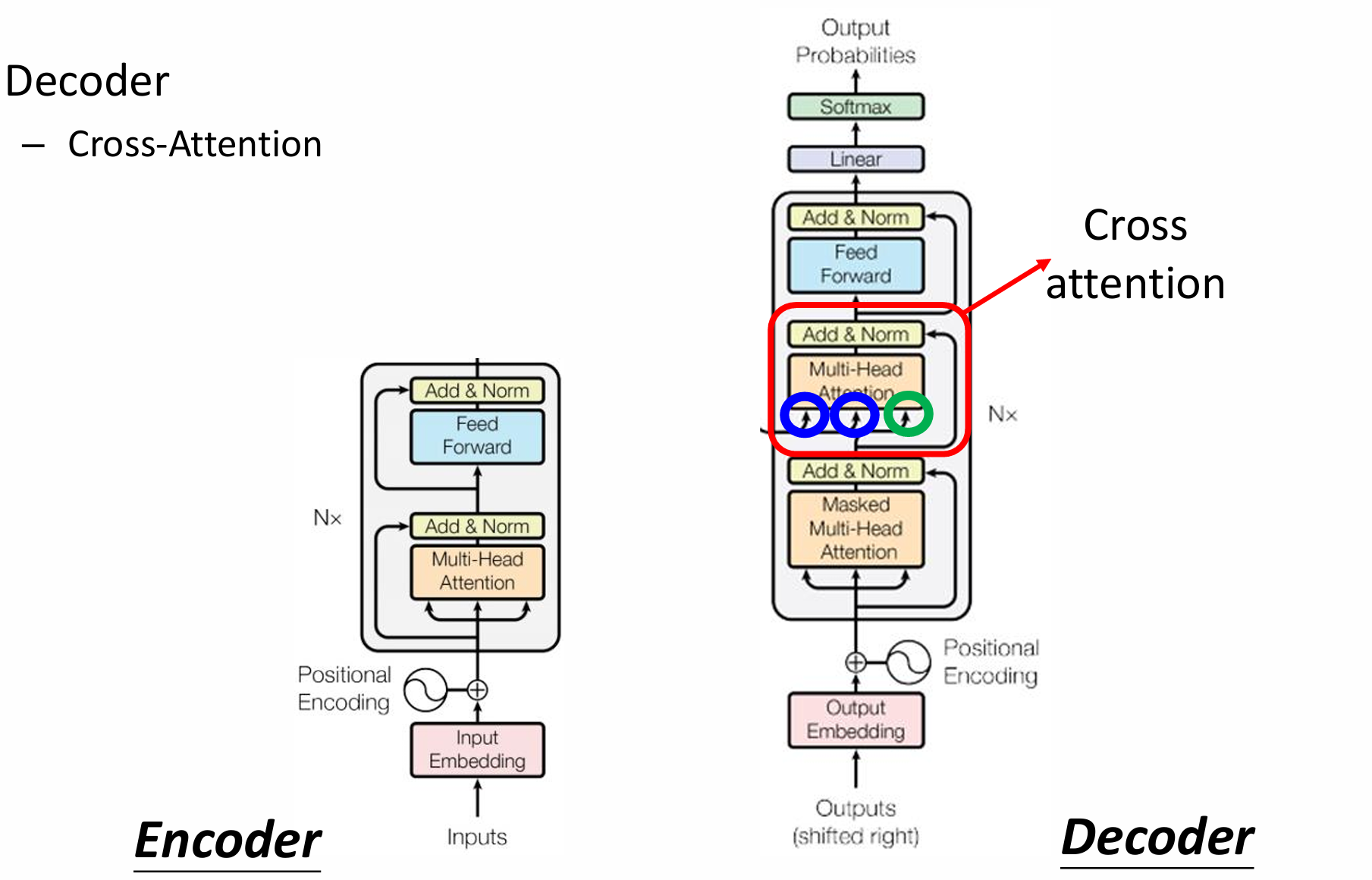
自然语言处理——Transformer
自然语言处理——Transformer 自注意力机制多头注意力机制Transformer 虽然循环神经网络可以对具有序列特性的数据非常有效,它能挖掘数据中的时序信息以及语义信息,但是它有一个很大的缺陷——很难并行化。 我们可以考虑用CNN来替代RNN,但是…...
汇编常见指令
汇编常见指令 一、数据传送指令 指令功能示例说明MOV数据传送MOV EAX, 10将立即数 10 送入 EAXMOV [EBX], EAX将 EAX 值存入 EBX 指向的内存LEA加载有效地址LEA EAX, [EBX4]将 EBX4 的地址存入 EAX(不访问内存)XCHG交换数据XCHG EAX, EBX交换 EAX 和 EB…...
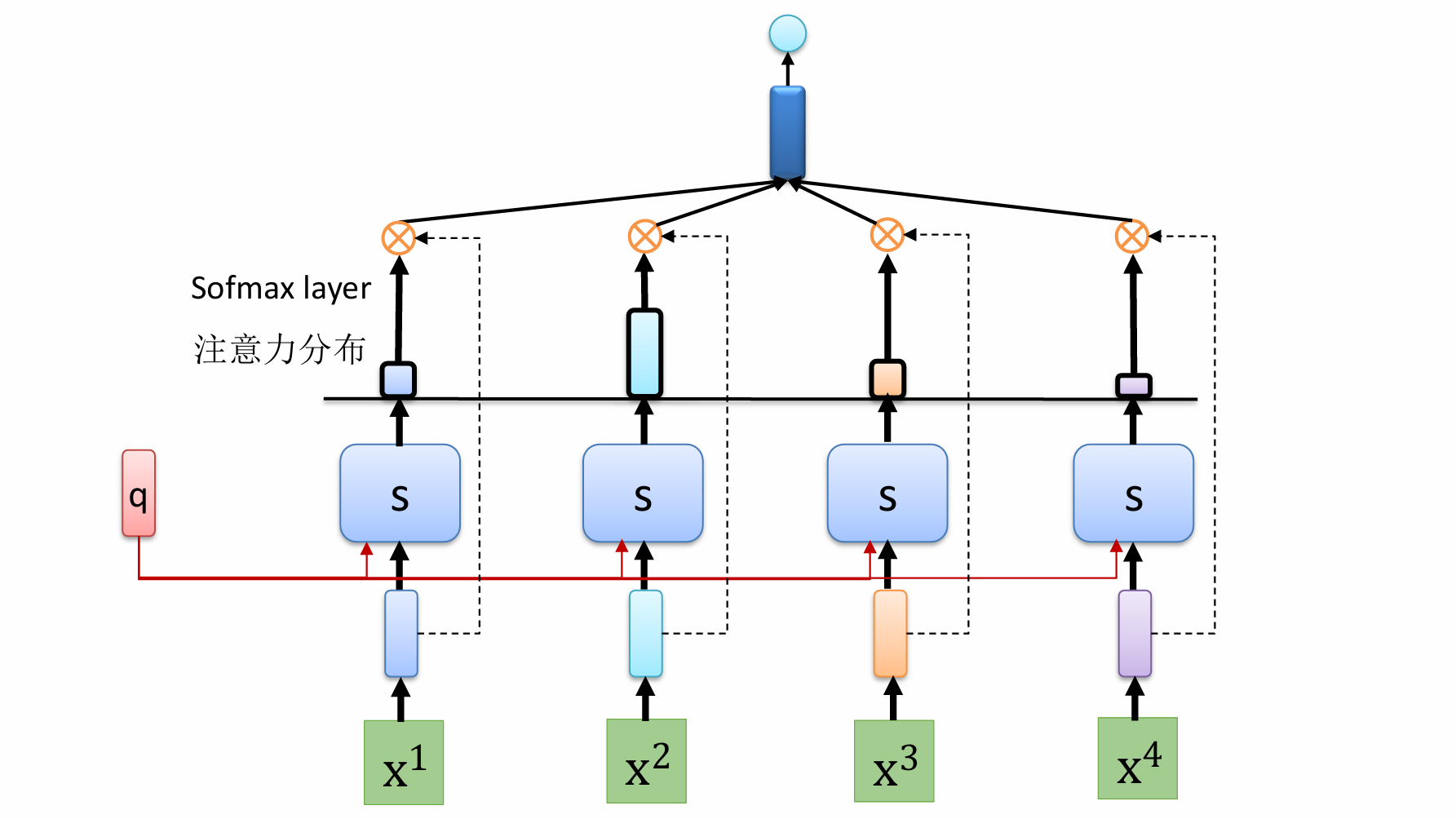
自然语言处理——循环神经网络
自然语言处理——循环神经网络 循环神经网络应用到基于机器学习的自然语言处理任务序列到类别同步的序列到序列模式异步的序列到序列模式 参数学习和长程依赖问题基于门控的循环神经网络门控循环单元(GRU)长短期记忆神经网络(LSTM)…...
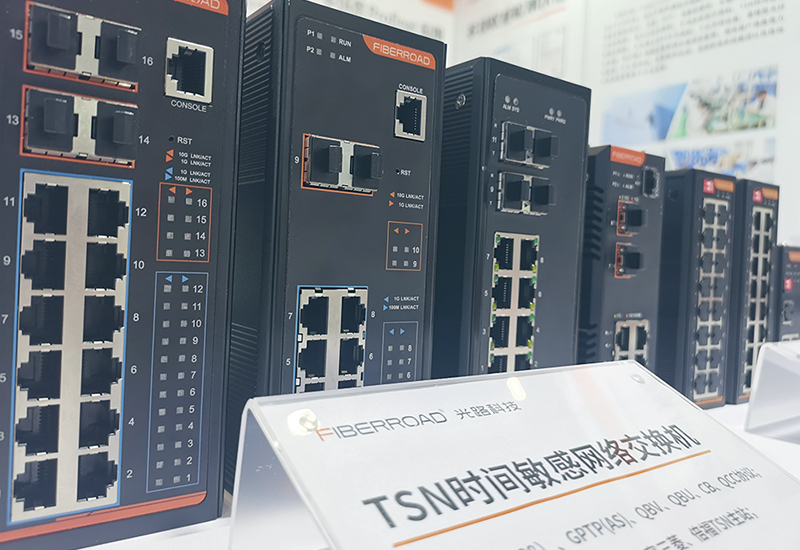
TSN交换机正在重构工业网络,PROFINET和EtherCAT会被取代吗?
在工业自动化持续演进的今天,通信网络的角色正变得愈发关键。 2025年6月6日,为期三天的华南国际工业博览会在深圳国际会展中心(宝安)圆满落幕。作为国内工业通信领域的技术型企业,光路科技(Fiberroad&…...
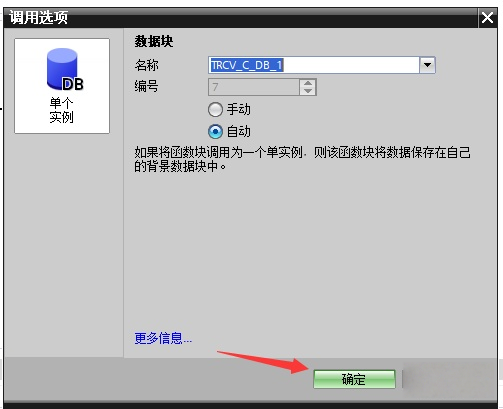
协议转换利器,profinet转ethercat网关的两大派系,各有千秋
随着工业以太网的发展,其高效、便捷、协议开放、易于冗余等诸多优点,被越来越多的工业现场所采用。西门子SIMATIC S7-1200/1500系列PLC集成有Profinet接口,具有实时性、开放性,使用TCP/IP和IT标准,符合基于工业以太网的…...
MFE(微前端) Module Federation:Webpack.config.js文件中每个属性的含义解释
以Module Federation 插件详为例,Webpack.config.js它可能的配置和含义如下: 前言 Module Federation 的Webpack.config.js核心配置包括: name filename(定义应用标识) remotes(引用远程模块࿰…...
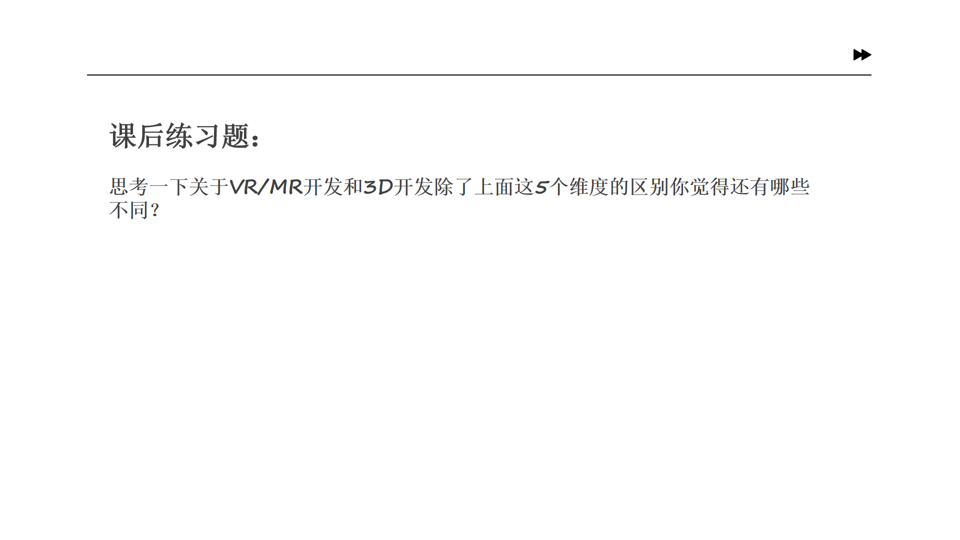
Unity VR/MR开发-VR开发与传统3D开发的差异
视频讲解链接:【XR马斯维】VR/MR开发与传统3D开发的差异【UnityVR/MR开发教程--入门】_哔哩哔哩_bilibili...