李宏毅2023机器学习作业1--homework1
一、前期准备
下载训练数据和测试数据
# dropbox link
!wget -O covid_train.csv https://www.dropbox.com/s/lmy1riadzoy0ahw/covid.train.csv?dl=0
!wget -O covid_test.csv https://www.dropbox.com/s/zalbw42lu4nmhr2/covid.test.csv?dl=0
导入包
# Numerical Operations
import math
import numpy as np # numpy操作数据,增加删除查找修改# Reading/Writing Data
import pandas as pd # pandas读取csv文件
import os # 进行文件夹操作
import csv# For Progress Bar
from tqdm import tqdm # 可视化# Pytorch
import torch # pytorch
import torch.nn as nn
from torch.utils.data import Dataset, DataLoader, random_split# For plotting learning curve
from torch.utils.tensorboard import SummaryWriter
定义一些功能函数
def same_seed(seed):'''Fixes random number generator seeds for reproducibility.'''torch.backends.cudnn.deterministic = Truetorch.backends.cudnn.benchmark = Falsenp.random.seed(seed)torch.manual_seed(seed)if torch.cuda.is_available():torch.cuda.manual_seed_all(seed)# 划分训练数据集和验证数据集
def train_valid_split(data_set, valid_ratio, seed):'''Split provided training data into training set and validation set'''valid_set_size = int(valid_ratio * len(data_set))train_set_size = len(data_set) - valid_set_sizetrain_set, valid_set = random_split(data_set, [train_set_size, valid_set_size], generator=torch.Generator().manual_seed(seed))return np.array(train_set), np.array(valid_set)
配置项
device = 'cuda' if torch.cuda.is_available() else 'cpu'
config = {'seed': 5201314, # Your seed number, you can pick your lucky number. :)'select_all': False, # Whether to use all features.'valid_ratio': 0.2, # validation_size = train_size * valid_ratio'n_epochs': 5000, # Number of epochs.'batch_size': 256,'learning_rate': 1e-5,'early_stop': 600, # If model has not improved for this many consecutive epochs, stop training.'save_path': './models/model.ckpt' # Your model will be saved here.
}
二、创建数据
创建Dataset
class COVID19Dataset(Dataset):'''x: Features.y: Targets, if none, do prediction.'''def __init__(self, x, y=None):if y is None:self.y = yelse:self.y = torch.FloatTensor(y)self.x = torch.FloatTensor(x)def __getitem__(self, idx):if self.y is None:return self.x[idx]else:return self.x[idx], self.y[idx]def __len__(self):return len(self.x)
特征选择
删除了belife和mental 的特征,belife和mental都是心理上精神上的特征,感觉可能和阳性率的偏差较大,就删去了这两类的特征
def select_feat(train_data, valid_data, test_data, select_all=True):'''Selects useful features to perform regression'''# [:,-1]第一个维度选择所有,选取所有行,第二个维度选择-1,-1是倒数第一个元素,也就是标签labely_train, y_valid = train_data[:,-1], valid_data[:,-1] # 选择标签元素# [:,:-1]第一个维度选择所有,所有行,第二个维度从开始元素到倒数第一个元素(不包含倒数第一个元素)raw_x_train, raw_x_valid, raw_x_test = train_data[:,:-1], valid_data[:,:-1], test_dataif select_all:feat_idx = list(range(raw_x_train.shape[1]))else:# feat_idx = list(range(35, raw_x_train.shape[1])) # TODO: Select suitable feature columns."""删除了belife和mental 的特征[0, 38, 39, 46, 51, 56, 57, 64, 69, 74, 75, 82, 87]是belife和mental所在列"""del_col = [0, 38, 39, 46, 51, 56, 57, 64, 69, 74, 75, 82, 87] raw_x_train = np.delete(raw_x_train, del_col, axis=1) # numpy数组增删查改方法raw_x_valid = np.delete(raw_x_valid, del_col, axis=1)raw_x_test = np.delete(raw_x_test, del_col, axis=1)return raw_x_train, raw_x_valid, raw_x_test, y_train, y_validreturn raw_x_train[:,feat_idx], raw_x_valid[:,feat_idx], raw_x_test[:,feat_idx], y_train, y_valid
创建 Dataloader
读取文件,设置训练,验证和测试数据集
# Set seed for reproducibility
same_seed(config['seed'])# train_data size: 3009 x 89 (35 states + 18 features x 3 days)
# train_data共3009条数据,每条数据89个维度
# test_data size: 997 x 88 (without last day's positive rate)
# test_data共997条数据,每条数据88个维度,没有最后一天的最后一列数据positive rate# pands读取csv数据
train_data, test_data = pd.read_csv('./covid_train.csv').values, pd.read_csv('./covid_test.csv').values # train_valid_split切分训练集和验证集
train_data, valid_data = train_valid_split(train_data, config['valid_ratio'], config['seed'])# Print out the data size.打印数据尺寸
print(f"""train_data size: {train_data.shape}
valid_data size: {valid_data.shape}
test_data size: {test_data.shape}""")# Select features 选择特征
x_train, x_valid, x_test, y_train, y_valid = select_feat(train_data, valid_data, test_data, config['select_all'])# Print out the number of features. 打印特征数
print(f'number of features: {x_train.shape[1]}')# 生成dataset
train_dataset, valid_dataset, test_dataset = COVID19Dataset(x_train, y_train), \COVID19Dataset(x_valid, y_valid), \COVID19Dataset(x_test)# Pytorch data loader loads pytorch dataset into batches.
# pytorch的dataloder加载dataset
train_loader = DataLoader(train_dataset, batch_size=config['batch_size'], shuffle=True, pin_memory=True)
valid_loader = DataLoader(valid_dataset, batch_size=config['batch_size'], shuffle=True, pin_memory=True)
test_loader = DataLoader(test_dataset, batch_size=config['batch_size'], shuffle=False, pin_memory=True)
三、创建神经网络模型
class My_Model(nn.Module): def __init__(self, input_dim):super(My_Model, self).__init__()# TODO: modify model's structure, be aware of dimensions.self.layers = nn.Sequential(nn.Linear(input_dim, 16),nn.ReLU(),nn.Linear(16, 8),nn.ReLU(),nn.Linear(8, 1))def forward(self, x):x = self.layers(x)x = x.squeeze(1) # (B, 1) -> (B)return x
四、模型训练和模型测试
模型训练
def trainer(train_loader, valid_loader, model, config, device):criterion = nn.MSELoss(reduction='mean') # Define your loss function, do not modify this.# Define your optimization algorithm.# TODO: Please check https://pytorch.org/docs/stable/optim.html to get more available algorithms.# TODO: L2 regularization (optimizer(weight decay...) or implement by your self).optimizer = torch.optim.SGD(model.parameters(), lr=config['learning_rate'], momentum=0.9)writer = SummaryWriter() # Writer of tensoboard.# 如果没有models文件夹,创建名称为models的文件夹,保存模型if not os.path.isdir('./models'): os.mkdir('./models') # Create directory of saving models.# math.inf为无限大n_epochs, best_loss, step, early_stop_count = config['n_epochs'], math.inf, 0, 0for epoch in range(n_epochs):model.train() # Set your model to train mode.loss_record = [] # 记录损失# tqdm is a package to visualize your training progress.train_pbar = tqdm(train_loader, position=0, leave=True)for x, y in train_pbar:optimizer.zero_grad() # Set gradient to zero.x, y = x.to(device), y.to(device) # Move your data to device.pred = model(x) # 数据传入模型model,生成预测值predloss = criterion(pred, y) # 预测值pred和真实值y计算损失loss loss.backward() # Compute gradient(backpropagation).optimizer.step() # Update parameters.step += 1loss_record.append(loss.detach().item()) # 当前步骤的loss加到loss_record[]# Display current epoch number and loss on tqdm progress bar.train_pbar.set_description(f'Epoch [{epoch+1}/{n_epochs}]')train_pbar.set_postfix({'loss': loss.detach().item()})mean_train_loss = sum(loss_record)/len(loss_record) # 计算训练集上平均损失writer.add_scalar('Loss/train', mean_train_loss, step) model.eval() # Set your model to evaluation mode.loss_record = []for x, y in valid_loader:x, y = x.to(device), y.to(device)with torch.no_grad():pred = model(x)loss = criterion(pred, y)loss_record.append(loss.item())mean_valid_loss = sum(loss_record)/len(loss_record) # 计算验证集上平均损失 print(f'Epoch [{epoch+1}/{n_epochs}]: Train loss: {mean_train_loss:.4f}, Valid loss: {mean_valid_loss:.4f}')writer.add_scalar('Loss/valid', mean_valid_loss, step)# 保存验证集上平均损失最小的模型if mean_valid_loss < best_loss: best_loss = mean_valid_losstorch.save(model.state_dict(), config['save_path']) # Save your best modelprint('Saving model with loss {:.3f}...'.format(best_loss))early_stop_count = 0else:early_stop_count += 1# 设置早停early_stop_count# 如果early_stop_count次数,验证集上的平均损失没有变化,模型性能没有提升,停止训练if early_stop_count >= config['early_stop']: print('\nModel is not improving, so we halt the training session.')return
模型测试
# 测试数据集的预测
def predict(test_loader, model, device):model.eval() # Set your model to evaluation mode.preds = []for x in tqdm(test_loader):x = x.to(device)with torch.no_grad(): # 关闭梯度pred = model(x)preds.append(pred.detach().cpu())preds = torch.cat(preds, dim=0).numpy()return preds
五、训练模型
model = My_Model(input_dim=x_train.shape[1]).to(device) # put your model and data on the same computation device.trainer(train_loader, valid_loader, model, config, device)
六、测试模型,生成预测值
def save_pred(preds, file):''' Save predictions to specified file '''with open(file, 'w') as fp:writer = csv.writer(fp)writer.writerow(['id', 'tested_positive'])for i, p in enumerate(preds):writer.writerow([i, p])model = My_Model(input_dim=x_train.shape[1]).to(device)
model.load_state_dict(torch.load(config['save_path'])) # 加载模型
preds = predict(test_loader, model, device) # 生成预测结果preds
save_pred(preds, 'pred.csv') # 保存preds到pred.csv
tensorboard可视化训练和验证损失图像
%reload_ext tensorboard
%tensorboard --logdir=./runs/
参考:
李宏毅_机器学习_作业1(详解)_COVID-19 Cases Prediction (Regression)-物联沃-IOTWORD物联网
【深度学习】2023李宏毅homework1作业一代码详解_李宏毅作业1-CSDN博客
np.delete详解-CSDN博客
相关文章:
李宏毅2023机器学习作业1--homework1
一、前期准备 下载训练数据和测试数据 # dropbox link !wget -O covid_train.csv https://www.dropbox.com/s/lmy1riadzoy0ahw/covid.train.csv?dl0 !wget -O covid_test.csv https://www.dropbox.com/s/zalbw42lu4nmhr2/covid.test.csv?dl0 导入包 # Numerical Operation…...
Mysql的SQL调优-面试
面试SQL优化的具体操作: 1、在表中建立索引,优先考虑where、group by使用到的字段。 2、尽量避免使用select *,返回无用的字段会降低查询效率。错误如下: SELECT * FROM table 优化方式:使用具体的字段代替 *…...
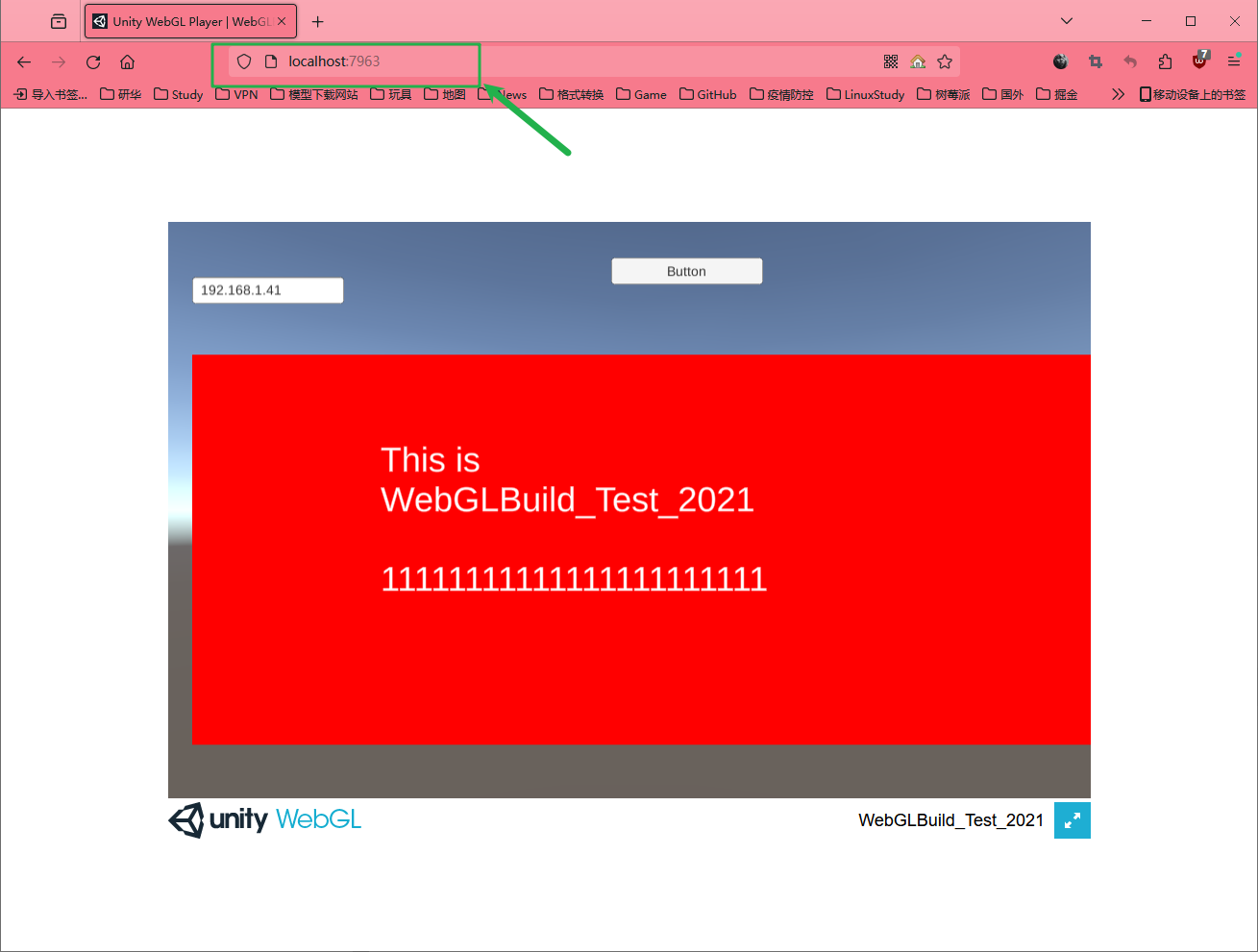
Unity 2021.3发布WebGL设置以及nginx的配置
使用unity2021.3发布webgl 使用Unity制作好项目之后建议进行代码清理,这样会即将不用的命名空间去除,不然一会在发布的时候有些命名空间webgl会报错。 平台转换 将平台设置为webgl 设置色彩空间压缩方式 Compression Format 设置为DisabledDecompre…...
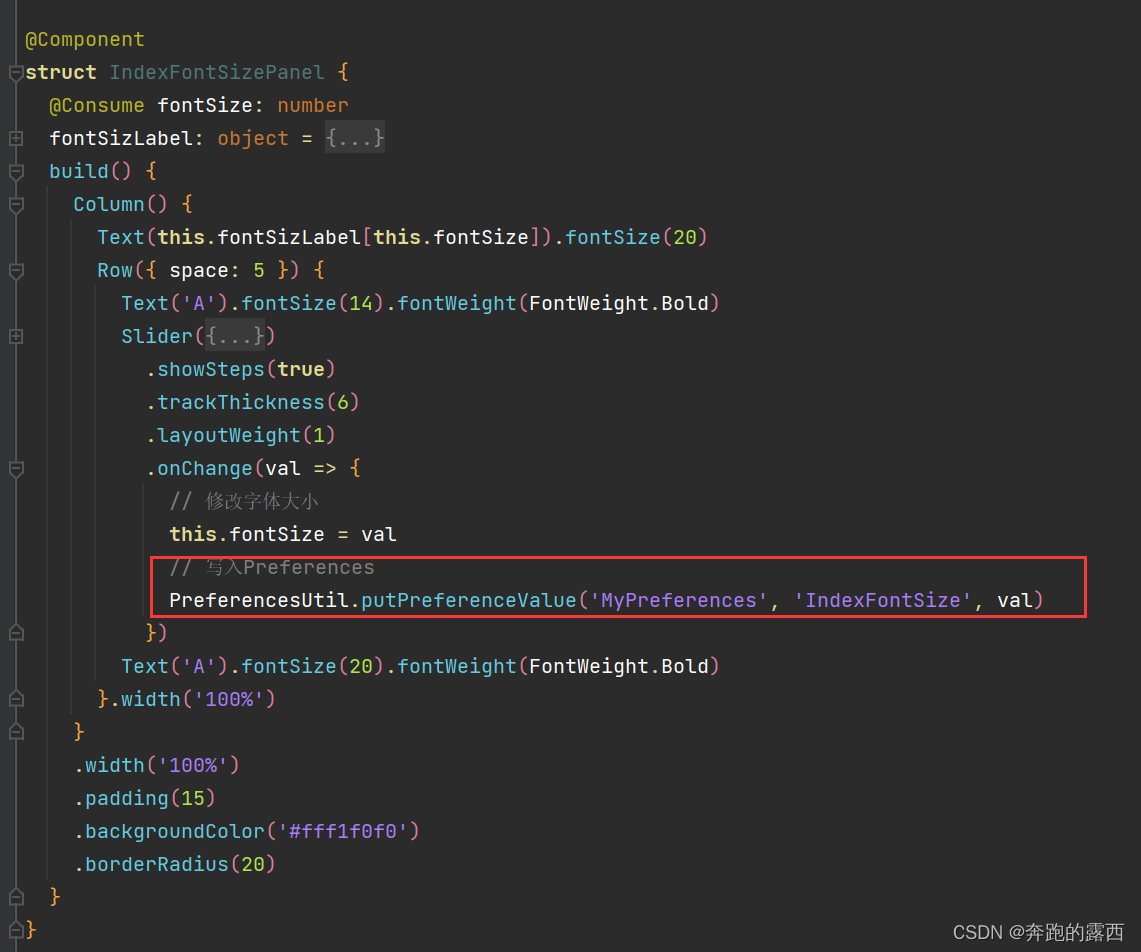
【鸿蒙 HarmonyOS 4.0】数据持久化
一、数据持久化介绍 数据持久化是将内存数据(内存是临时的存储空间),通过文件或数据库的形式保存在设备中。 HarmonyOS提供两种数据持久化方案: 1.1、用户首选项(Preferences): 通常用于保存应用的配置信息。数据通…...
mysql mgr集群多主部署
一、前言 mgr多主集群是将集群中的所有节点都设为可写,减轻了单主节点的写压力,从而提高了mysql的写入性能 二、部署 基础部署与mgr集群单主部署一致,只是在创建mgr集群时有所不同 基础部署参考:mysql mgr集群部署-CSDN博客 设置…...
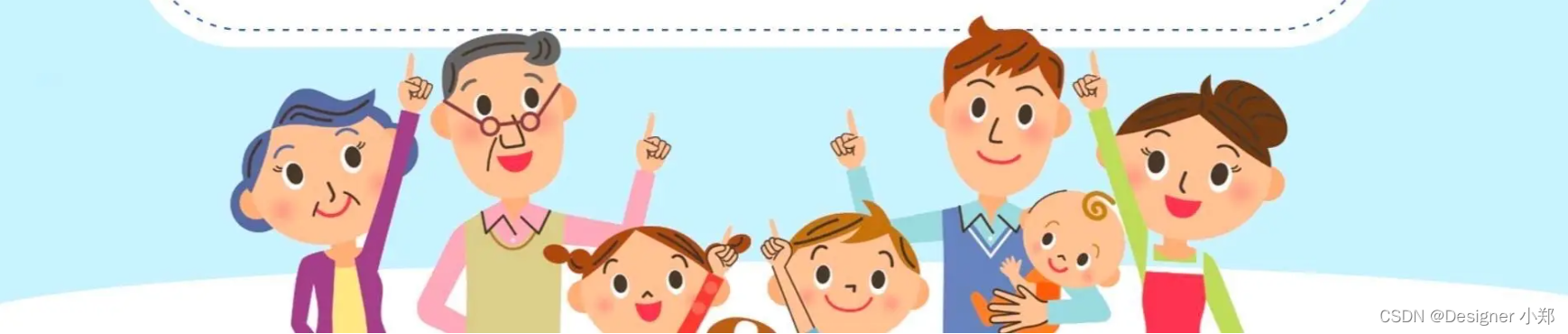
【开源】JAVA+Vue.js实现医院门诊预约挂号系统
目录 一、摘要1.1 项目介绍1.2 项目录屏 二、功能模块2.1 功能性需求2.1.1 数据中心模块2.1.2 科室医生档案模块2.1.3 预约挂号模块2.1.4 医院时政模块 2.2 可行性分析2.2.1 可靠性2.2.2 易用性2.2.3 维护性 三、数据库设计3.1 用户表3.2 科室档案表3.3 医生档案表3.4 医生放号…...
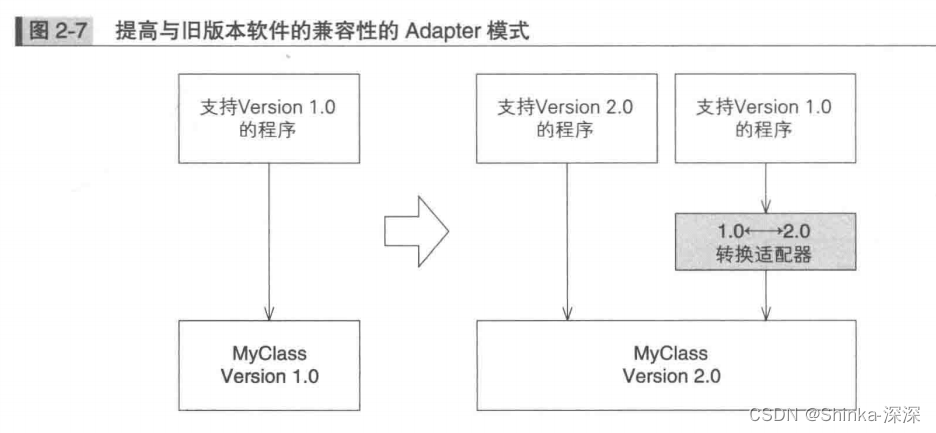
《图解设计模式》笔记(一)适应设计模式
图灵社区 - 图解设计模式 - 随书下载 评论区 雨帆 2017-01-11 16:14:04 对于设计模式,我个人认为,其实代码和设计原则才是最好的老师。理解了 SOLID,如何 SOLID,自然而然地就用起来设计模式了。Github 上有一个 tdd-training&…...
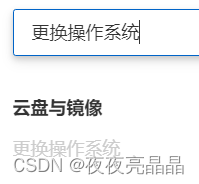
图文说明Linux云服务器如何更改实例镜像
一、应用场景举例 在学习Linux的vim时,我们难免要对vim进行一些配置,这里我们提供一个vim插件的安装包: curl -sLf https://gitee.com/HGtz2222/VimForCpp/raw/master/install.sh -o./install.sh && bash ./install.sh 但是此安装包…...
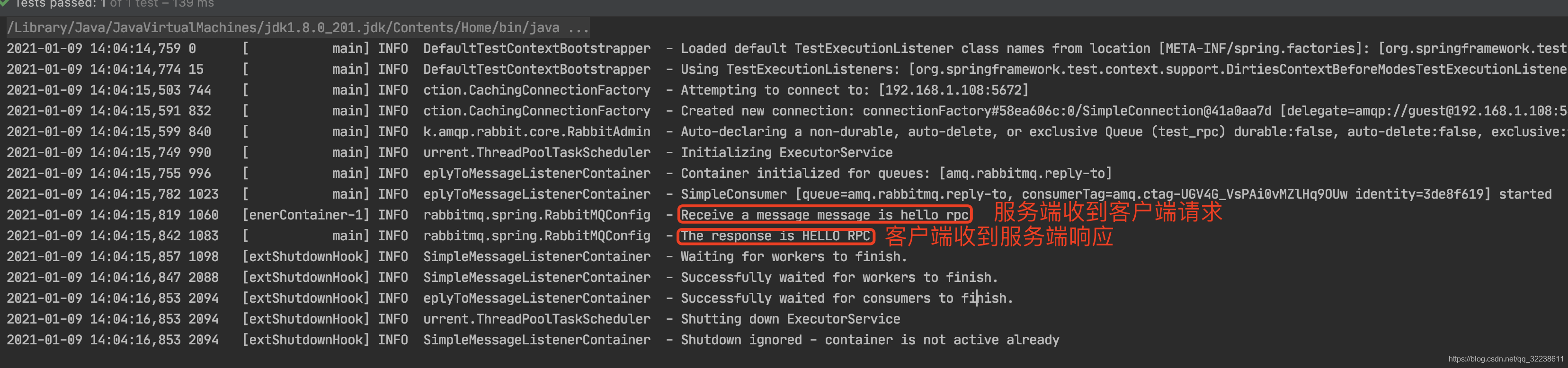
RabbitMQ学习整理————基于RabbitMQ实现RPC
基于RabbitMQ实现RPC 前言什么是RPCRabbitMQ如何实现RPCRPC简单示例通过Spring AMQP实现RPC 前言 这边参考了RabbitMQ的官网,想整理一篇关于RabbitMQ实现RPC调用的博客,打算把两种实现RPC调用的都整理一下,一个是使用官方提供的一个Java cli…...
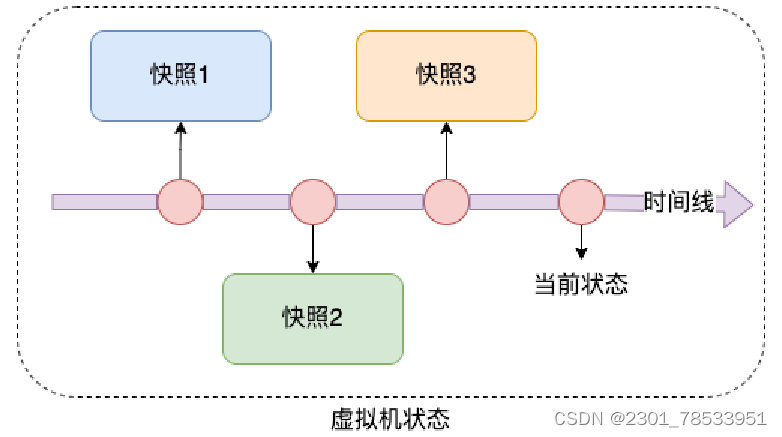
Linux-基础知识(黑马学习笔记)
硬件和软件 我们所熟知的计算机是由:硬件和软件组成。 硬件:计算机系统中电子,机械和光电元件等组成的各种物理装置的总称。 软件:是用户和计算机硬件之间的接口和桥梁,用户通过软件与计算机进行交流。 而操作系统…...
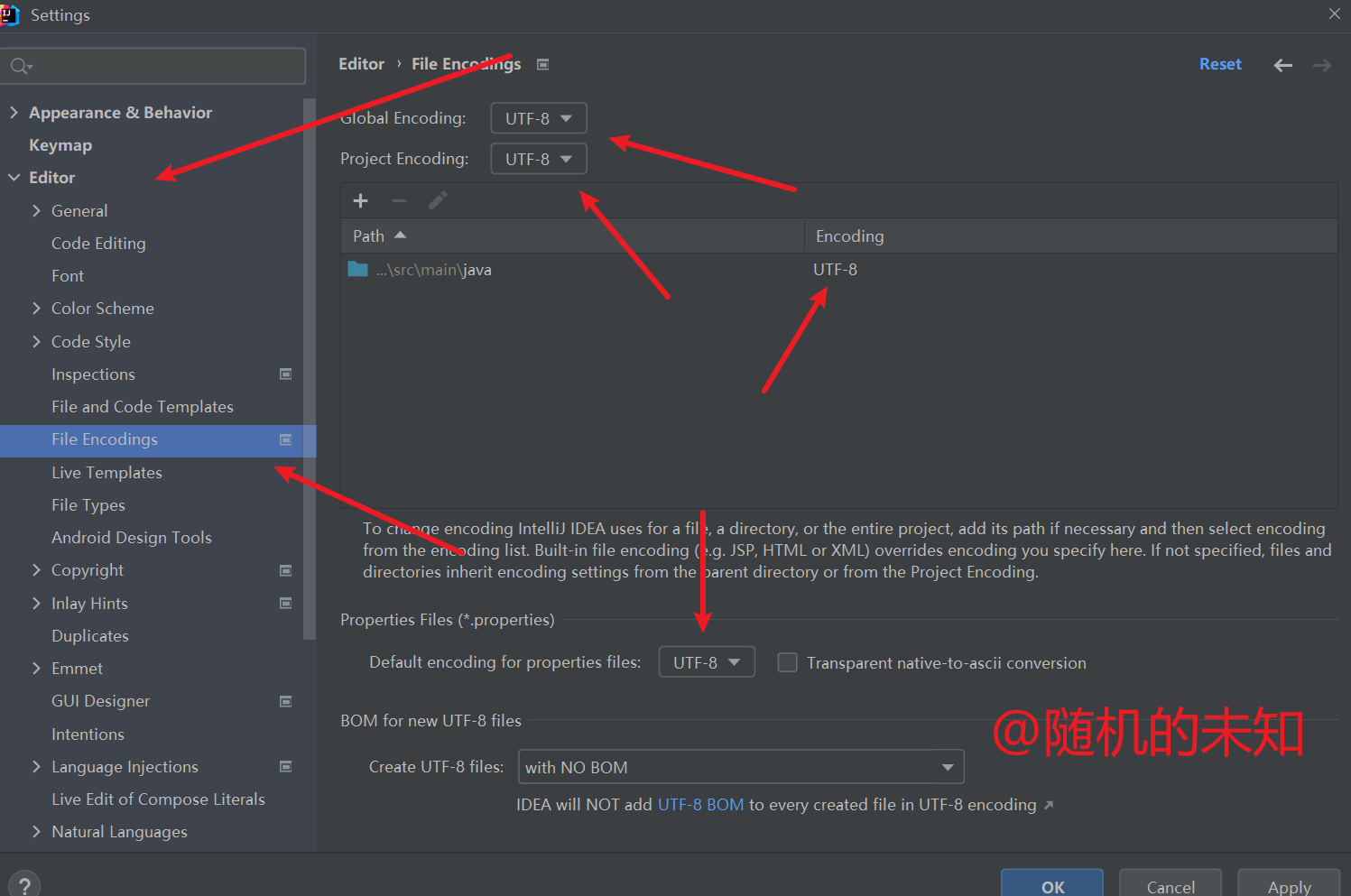
SpringBoot项目启动报java.nio.charset.MalformedInputException Input length = 1解决方案
报错详情 SpringBoot启动报错java.nio.charset.MalformedInputException: Input length 1 报错原因 出现这个的原因,就是解析yml文件时,中文字符集不是utf-8的原因,这是maven在项目编译时,默认字符集编码是GBK。 解决方式 检…...

【Unity2019.4.35f1】配置JDK、NDK、SDK、Gradle
目录 JDK NDK SDK 环境变量 Gradle JDK JDK:jdk-1.8版本Java Downloads | Oracle 下载要登录,搜索JDK下载公用账号:Oracle官网 JDK下载 注册登录公共账号和密码_oracle下载账号-CSDN博客 路径:C:\Program Files\Java\jd…...
MySQL中的高级查询
通过条件查询可以查询到符合条件的数据,但如同要实现对字段的值进行计算、根据一个或多个字段对查询结果进行分组等操作时,就需要使用更高级的查询,MySQL提供了聚合函数、分组查询、排序查询、限量查询、内置函数以实现更复杂的查询需求。接下…...
leetcode383赎金信
用字符数组ch来记录magazine每个字母出现频率,用ransomNote的字母减去字符数组ch对应的字符出现频率,如果该字符对应的频率小于0,则不够,无法组成ransomNote! class Solution { public:bool canConstruct(string rans…...
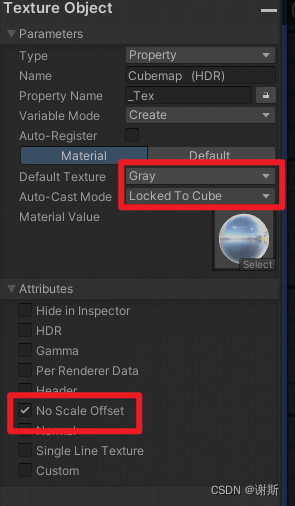
【Unity3D】ASE制作天空盒
找到官方shader并分析 下载对应资源包找到\DefaultResourcesExtra\Skybox-Cubed.shader找到\CGIncludes\UnityCG.cginc观察变量, 观察tag, 观察代码 需要注意的内容 ASE要处理的内容 核心修改 添加一个Custom Expression节点 code内容为: return DecodeHDR(In0, In1);outp…...
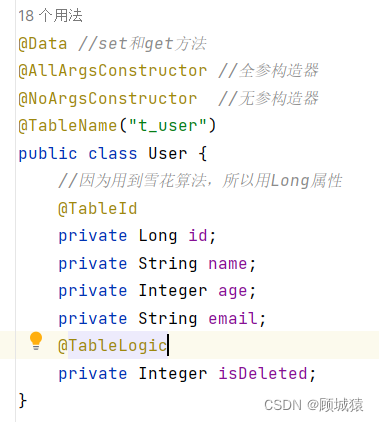
MyBatisPlus常用注解
目录 一、TableName 二、TableId 三、TableField 四、TableLogic 一、TableName 在使用MyBatis-Plus实现基本的CRUD时,我们并没有指定要操作的表,只是在Mapper接口继承BaseMapper时,设置了泛型User,而操作的表为user表 由此得出…...
Putty中运行matlab文件
首先使用命令 cd /home/ya/CodeTest/Matlab进入路径:到Matlab文件夹下 然后键入matlab,进入matlab环境,如果main.m文件在Matlab文件夹下,直接键入main即可运行该文件。细节代码如下: Unable to use key file "y…...
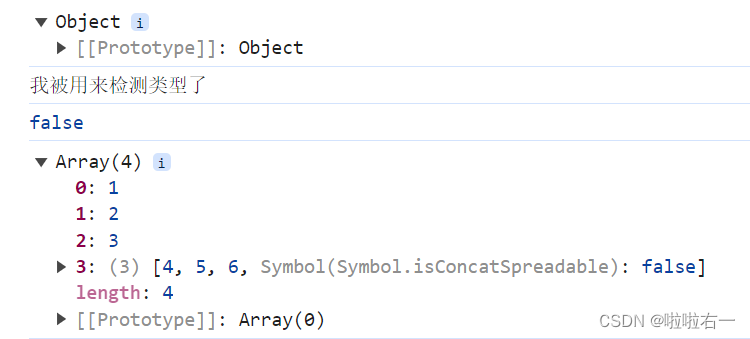
ES6 | (一)ES6 新特性(上) | 尚硅谷Web前端ES6教程
文章目录 📚ES6新特性📚let关键字📚const关键字📚变量的解构赋值📚模板字符串📚简化对象写法📚箭头函数📚函数参数默认值设定📚rest参数📚spread扩展运算符&a…...

生产环境下,应用模式部署flink任务,通过hdfs提交
前言 通过通过yarn.provided.lib.dirs配置选项指定位置,将flink的依赖上传到hdfs文件管理系统 1. 实践 (1)生产集群为cdh集群,从cm上下载配置文件,设置环境 export HADOOP_CONF_DIR/home/conf/auth export HADOOP_CL…...
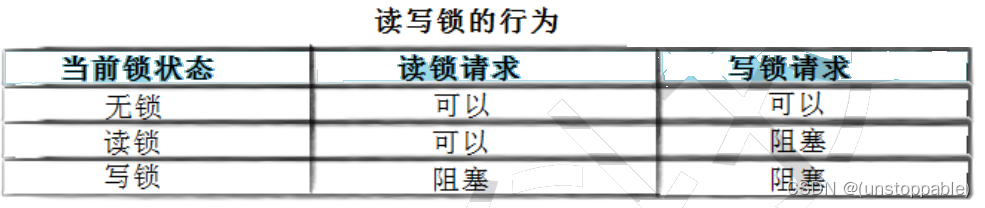
【lesson59】线程池问题解答和读者写者问题
文章目录 线程池问题解答什么是单例模式什么是设计模式单例模式的特点饿汉和懒汉模式的理解STL中的容器是否是线程安全的?智能指针是否是线程安全的?其他常见的各种锁 读者写者问题 线程池问题解答 什么是单例模式 单例模式是一种 “经典的, 常用的, 常考的” 设…...
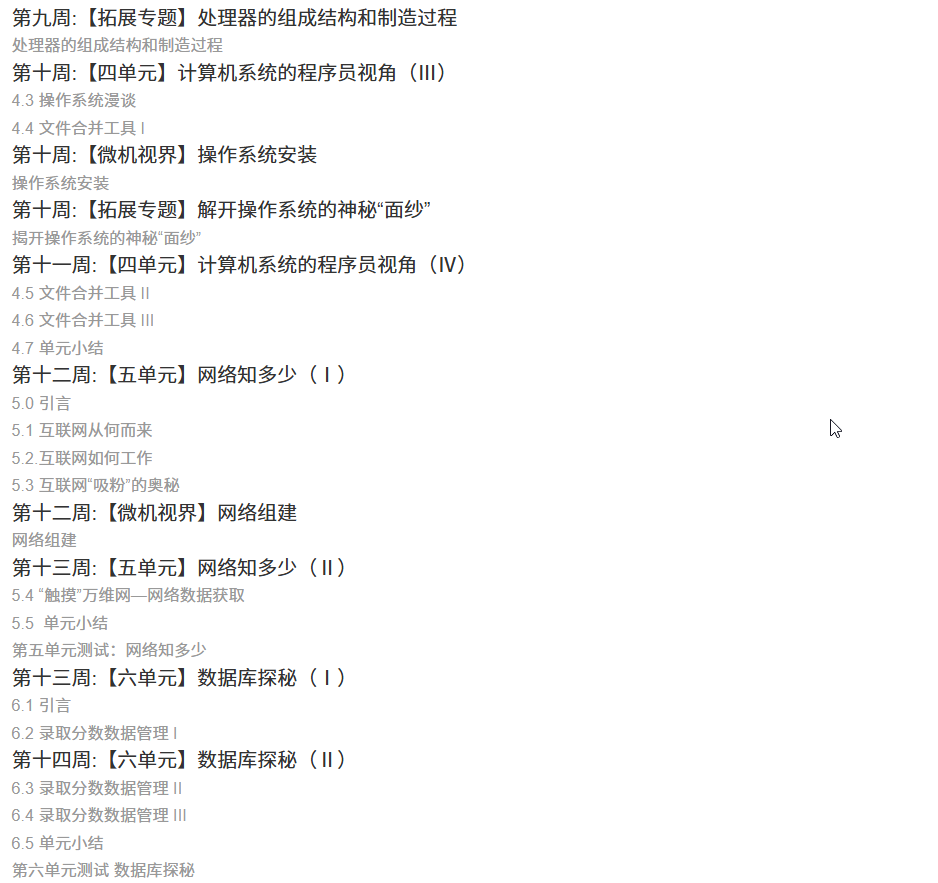
国防科技大学计算机基础课程笔记02信息编码
1.机内码和国标码 国标码就是我们非常熟悉的这个GB2312,但是因为都是16进制,因此这个了16进制的数据既可以翻译成为这个机器码,也可以翻译成为这个国标码,所以这个时候很容易会出现这个歧义的情况; 因此,我们的这个国…...
Admin.Net中的消息通信SignalR解释
定义集线器接口 IOnlineUserHub public interface IOnlineUserHub {/// 在线用户列表Task OnlineUserList(OnlineUserList context);/// 强制下线Task ForceOffline(object context);/// 发布站内消息Task PublicNotice(SysNotice context);/// 接收消息Task ReceiveMessage(…...
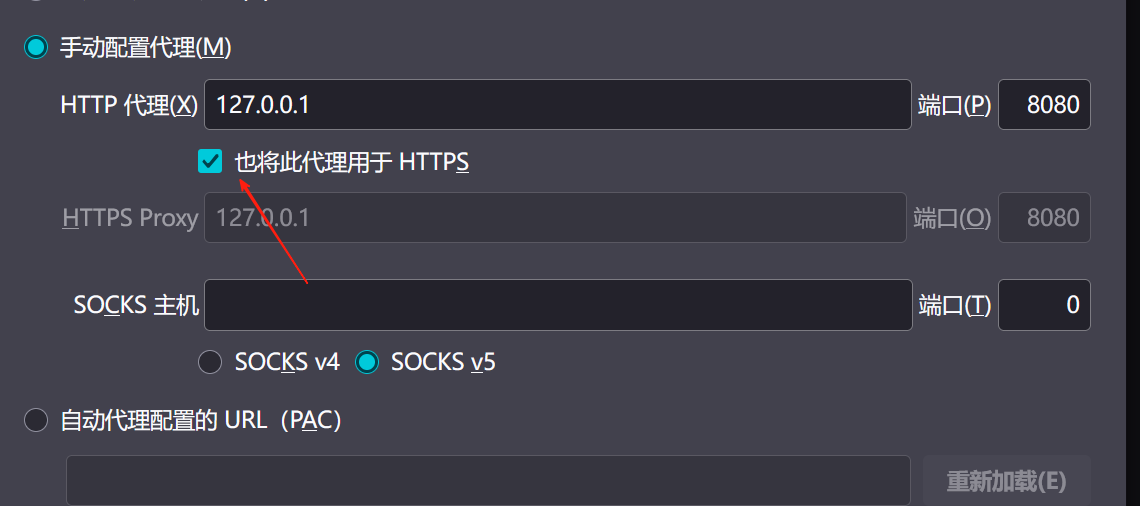
【2025年】解决Burpsuite抓不到https包的问题
环境:windows11 burpsuite:2025.5 在抓取https网站时,burpsuite抓取不到https数据包,只显示: 解决该问题只需如下三个步骤: 1、浏览器中访问 http://burp 2、下载 CA certificate 证书 3、在设置--隐私与安全--…...
什么?连接服务器也能可视化显示界面?:基于X11 Forwarding + CentOS + MobaXterm实战指南
文章目录 什么是X11?环境准备实战步骤1️⃣ 服务器端配置(CentOS)2️⃣ 客户端配置(MobaXterm)3️⃣ 验证X11 Forwarding4️⃣ 运行自定义GUI程序(Python示例)5️⃣ 成功效果
Java面试专项一-准备篇
一、企业简历筛选规则 一般企业的简历筛选流程:首先由HR先筛选一部分简历后,在将简历给到对应的项目负责人后再进行下一步的操作。 HR如何筛选简历 例如:Boss直聘(招聘方平台) 直接按照条件进行筛选 例如:…...
全面解析各类VPN技术:GRE、IPsec、L2TP、SSL与MPLS VPN对比
目录 引言 VPN技术概述 GRE VPN 3.1 GRE封装结构 3.2 GRE的应用场景 GRE over IPsec 4.1 GRE over IPsec封装结构 4.2 为什么使用GRE over IPsec? IPsec VPN 5.1 IPsec传输模式(Transport Mode) 5.2 IPsec隧道模式(Tunne…...
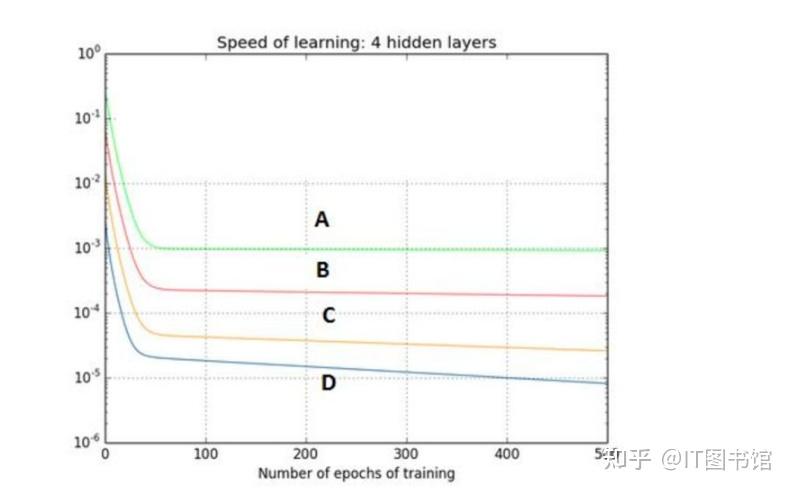
深度学习习题2
1.如果增加神经网络的宽度,精确度会增加到一个特定阈值后,便开始降低。造成这一现象的可能原因是什么? A、即使增加卷积核的数量,只有少部分的核会被用作预测 B、当卷积核数量增加时,神经网络的预测能力会降低 C、当卷…...
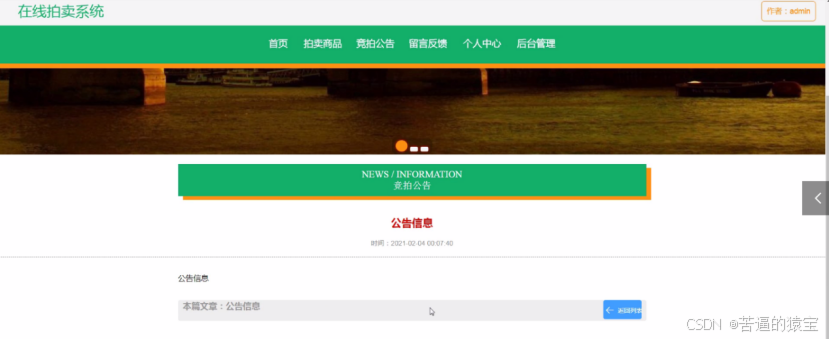
基于SpringBoot在线拍卖系统的设计和实现
摘 要 随着社会的发展,社会的各行各业都在利用信息化时代的优势。计算机的优势和普及使得各种信息系统的开发成为必需。 在线拍卖系统,主要的模块包括管理员;首页、个人中心、用户管理、商品类型管理、拍卖商品管理、历史竞拍管理、竞拍订单…...
4. TypeScript 类型推断与类型组合
一、类型推断 (一) 什么是类型推断 TypeScript 的类型推断会根据变量、函数返回值、对象和数组的赋值和使用方式,自动确定它们的类型。 这一特性减少了显式类型注解的需要,在保持类型安全的同时简化了代码。通过分析上下文和初始值,TypeSc…...
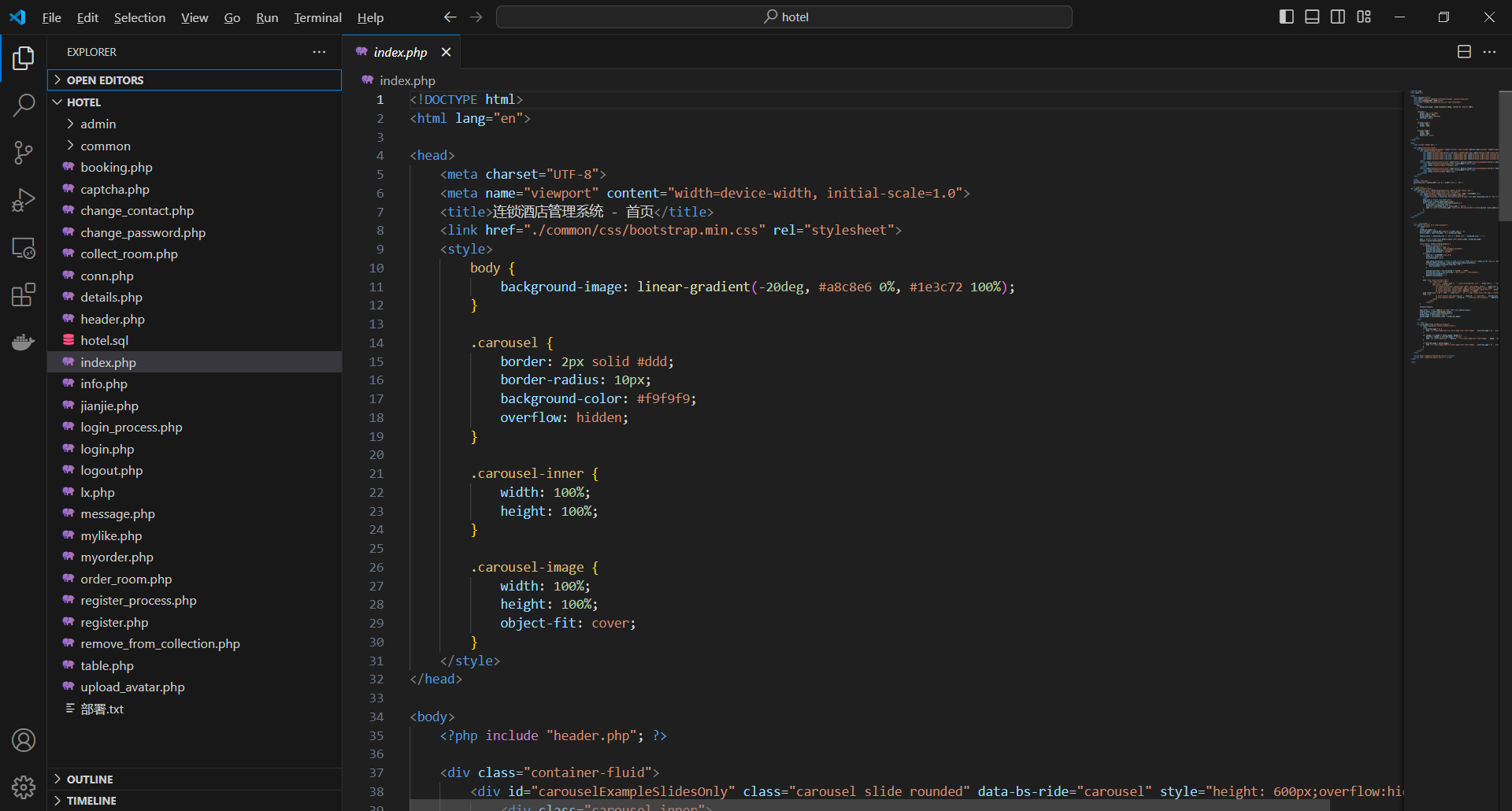
基于PHP的连锁酒店管理系统
有需要请加文章底部Q哦 可远程调试 基于PHP的连锁酒店管理系统 一 介绍 连锁酒店管理系统基于原生PHP开发,数据库mysql,前端bootstrap。系统角色分为用户和管理员。 技术栈 phpmysqlbootstrapphpstudyvscode 二 功能 用户 1 注册/登录/注销 2 个人中…...