Yolov5封装detect.py面向对象
主要目标是适应摄像头rtsp流的检测
如果是普通文件夹或者图片,run中的while True去掉即可。
web_client是根据需求创建的客户端,将检测到的数据打包发送给服务器
# YOLOv5 🚀 by Ultralytics, GPL-3.0 license
"""
Run inference on images, videos, directories, streams, etc.Usage:$ python path/to/detect.py --source path/to/img.jpg --weights yolov5s.pt --img 640
"""import argparse
import json
import os
import sys
import time
import moment
from pathlib import Pathimport cv2
import numpy as np
import torch
import torch.backends.cudnn as cudnnFILE = Path(__file__).resolve()
ROOT = FILE.parents[0] # YOLOv5 root directory
if str(ROOT) not in sys.path:sys.path.append(str(ROOT)) # add ROOT to PATH
ROOT = Path(os.path.relpath(ROOT, Path.cwd())) # relativefrom models.experimental import attempt_load
from utils.datasets import LoadImages, LoadStreams
from utils.general import apply_classifier, check_img_size, check_imshow, check_requirements, check_suffix, colorstr, \increment_path, non_max_suppression, print_args, save_one_box, scale_coords, set_logging, \strip_optimizer, xyxy2xywh
from utils.plots import Annotator, colors
from utils.torch_utils import load_classifier, select_device, time_syncfrom mytools import read_yaml_all, base64_encode_img
from message_base import MessageBase
from websocket_client import WebClientclass Detect:def __init__(self, config: dict, client: WebClient):self.config = configself.weights = self.config.get("weights") # weights pathself.source = self.config.get("source") # source self.imgsz = self.config.get("imgsz") # imgszself.conf_thres = self.config.get("conf_thres")self.iou_thres = self.config.get("iou_thres")self.max_det = self.config.get("max_det")self.device = self.config.get("device") # "cpu" or "0,1,2,3"self.view_img = self.config.get("view_img") # show resultsself.save_txt = self.config.get("save_txt") # save results to *.txtself.save_conf = self.config.get("save_conf") # save confidences in --save-txt labelsself.save_crop = self.config.get("save_crop") # save cropped prediction boxesself.nosave = self.config.get("nosave") # do not save images/videosself.classes = self.config.get("classes") # filter by class: --class 0, or --class 0 2 3self.agnostic_nms = self.config.get("agnostic_nms") # class-agnostic NMSself.augment = self.config.get("augment") # augmented inferenceself.visualize = self.config.get("visualize") # visualize featuresself.update = self.config.get("update") # update all modelsself.save_path = self.config.get("save_path") # save results to project/nameself.line_thickness = self.config.get("line_thickness") # bounding box thickness (pixels)self.hide_labels = self.config.get("hide_labels") # hide labelsself.hide_conf = self.config.get("hide_conf") # hide confidencesself.half = self.config.get("half") # use FP16 half-precision inferenceself.dnn = self.config.get("dnn") # use OpenCV DNN for ONNX inferenceself.func_device = self.config.get("func_device") # 对应功能的设备名字self.save_img = not self.nosave and not self.source.endswith('.txt') # save inference imagesself.webcam = self.source.isnumeric() or self.source.endswith('.txt') or self.source.lower().startswith(('rtsp://', 'rtmp://', 'http://', 'https://'))set_logging()self.device = select_device(self.device)self.half = self.device.type != 'cpu' # half precision only supported on CUDAself.model = attempt_load(self.weights, map_location=self.device)self.imgsz = check_img_size(self.imgsz, s=int(self.model.stride.max()))self.stride = int(self.model.stride.max())self.names = self.model.module.names if hasattr(self.model, 'module') else self.model.names# 获取数据if self.webcam:self.view_img = check_imshow()cudnn.benchmark = True # set True to speed up constant image size inferenceself.dataset = LoadStreams(self.source, img_size=self.imgsz, stride=self.stride, auto=True)self.bs = len(self.dataset) # batch_sizeelse:self.dataset = LoadImages(self.source, img_size=self.imgsz, stride=self.stride, auto=True)self.bs = 1 # batch_sizeself.client = client # 客户端self.last_time = moment.now()self.check_time_step = 5 # 每隔多少时间检测一次os.mkdir(self.save_path) if not os.path.exists(self.save_path) else Nonedef inference(self, img):img = torch.from_numpy(img).to(self.device)img = img.half() if self.half else img.float() # uint8 to fp16/32img /= 255.0 # 0 - 255 to 0.0 - 1.0if img.ndimension() == 3:img = img.unsqueeze(0)pred = self.model(img, augment=self.augment)[0]# NMSpred = non_max_suppression(pred, self.conf_thres, self.iou_thres,self.classes, self.agnostic_nms, max_det=self.max_det)return preddef process(self, im0s, img, pred, path):for i, det in enumerate(pred): # per imageif self.webcam: # batch_size >= 1p, s, im0, frame = path[i], f'{i}: ', im0s[i].copy(), self.dataset.countelse:p, s, im0, frame = path, '', im0s.copy(), getattr(self.dataset, 'frame', 0)p = Path(p) # to Pathtxt_path = str(self.save_path + "/" + 'labels' + "/" + p.stem) + ('' if self.dataset.mode == 'image' else f'_{frame}') # img.txts += '%gx%g ' % img.shape[2:] # print stringgn = torch.tensor(im0.shape)[[1, 0, 1, 0]] # normalization gain whwhimc = im0.copy() if self.save_crop else im0 # for save_cropannotator = Annotator(im0, line_width=self.line_thickness, example=str(self.names))if len(det):# Rescale boxes from img_size to im0 sizedet[:, :4] = scale_coords(img.shape[2:], det[:, :4], im0.shape).round()# Print resultsfor c in det[:, -1].unique():n = (det[:, -1] == c).sum() # detections per classs += f"{n} {self.names[int(c)]}{'s' * (n > 1)}, " # add to string# Write resultsfor *xyxy, conf, cls in reversed(det):c = int(cls)label = self.names[c]# if label == "person":if label: # 根据对应标签做处理# annotator.box_label(xyxy, label, color=colors(c, True)) # 画框t = int(time.time())img_path = f"{self.save_path}/{self.func_device}_{label}_{t}.jpg"crop = save_one_box(xyxy, imc, img_path, BGR=True)x1, y1, x2, y2 = int(xyxy[0]), int(xyxy[1]), int(xyxy[2]), int(xyxy[3])data = {"device": self.func_device,"value": {"label": label,"time": t,"locate": (x1, y1, x2, y2),"crop": base64_encode_img(crop)}}data = json.dumps(data) # 打包数据try:self.client.send(data) # 客户端发送数据passexcept Exception as err:print("发送失败:", err)self.client.connect()self.client.send(data)print("重连成功!")print(data)# if self.save_txt: # Write to file# xywh = (xyxy2xywh(torch.tensor(xyxy).view(1, 4)) / gn).view(# -1).tolist() # normalized xywh# line = (cls, *xywh, conf) if self.save_conf else (cls, *xywh) # label format# with open(txt_path + '.txt', 'a') as f:# f.write(('%g ' * len(line)).rstrip() % line + '\n')# 画框# if self.save_img or self.save_crop or self.view_img: # Add bbox to image# c = int(cls) # integer class# label = None if self.hide_labels else (self.names[c] if self.hide_conf else# f'{self.names[c]} {conf:.2f}')# annotator.box_label(xyxy, label, color=colors(c, True))def run(self):self.client.connect()while True:for path, img, im0s, vid_cap in self.dataset:if self.last_time.__lt__(moment.now()):self.last_time = moment.now().add(seconds=self.check_time_step)try:pred = self.inference(img)self.process(im0s, img, pred, path) except Exception as err:print(err)if self.save_txt or self.save_img:s = f"\n{len(list(self.save_path.glob('labels/*.txt')))} labels saved to {self.save_path / 'labels'}" if self.save_txt else ''print(f"Results saved to {colorstr('bold', self.save_path)}{s}")if self.update:strip_optimizer(self.weights) # update model (to fix SourceChangeWarning)if __name__ == "__main__":message_base = MessageBase()wc = WebClient("192.168.6.28", 8000)configs = read_yaml_all("yolo_configs.yaml")config = read_yaml_all("configs.yaml")device_name = config.get("DEVICE_LIST")[0]device_source = config.get("RTSP_URLS").get(device_name)configs["source"] = device_sourceconfigs["func_device"] = device_nameprint(configs)detect = Detect(configs, wc)detect.run()
相关文章:
Yolov5封装detect.py面向对象
主要目标是适应摄像头rtsp流的检测 如果是普通文件夹或者图片,run中的while True去掉即可。 web_client是根据需求创建的客户端,将检测到的数据打包发送给服务器 # YOLOv5 🚀 by Ultralytics, GPL-3.0 license """ Run inf…...
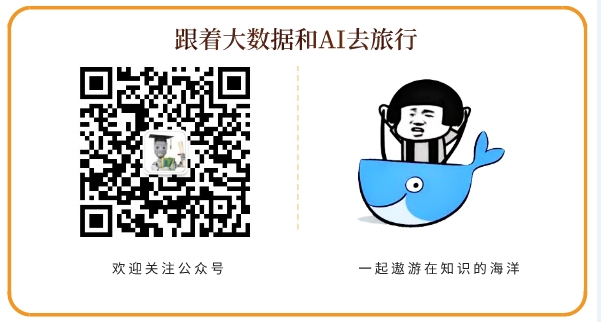
入门级深度学习主机组装过程
一 配置 先附上电脑配置图,如下: 利用公司的办公电脑对配置进行升级改造完成。除了显卡和电源,其他硬件都是公司电脑原装。 二 显卡 有钱直接上 RTX4090,也不能复用公司的电脑,其他配置跟不上。 进行深度学习&…...
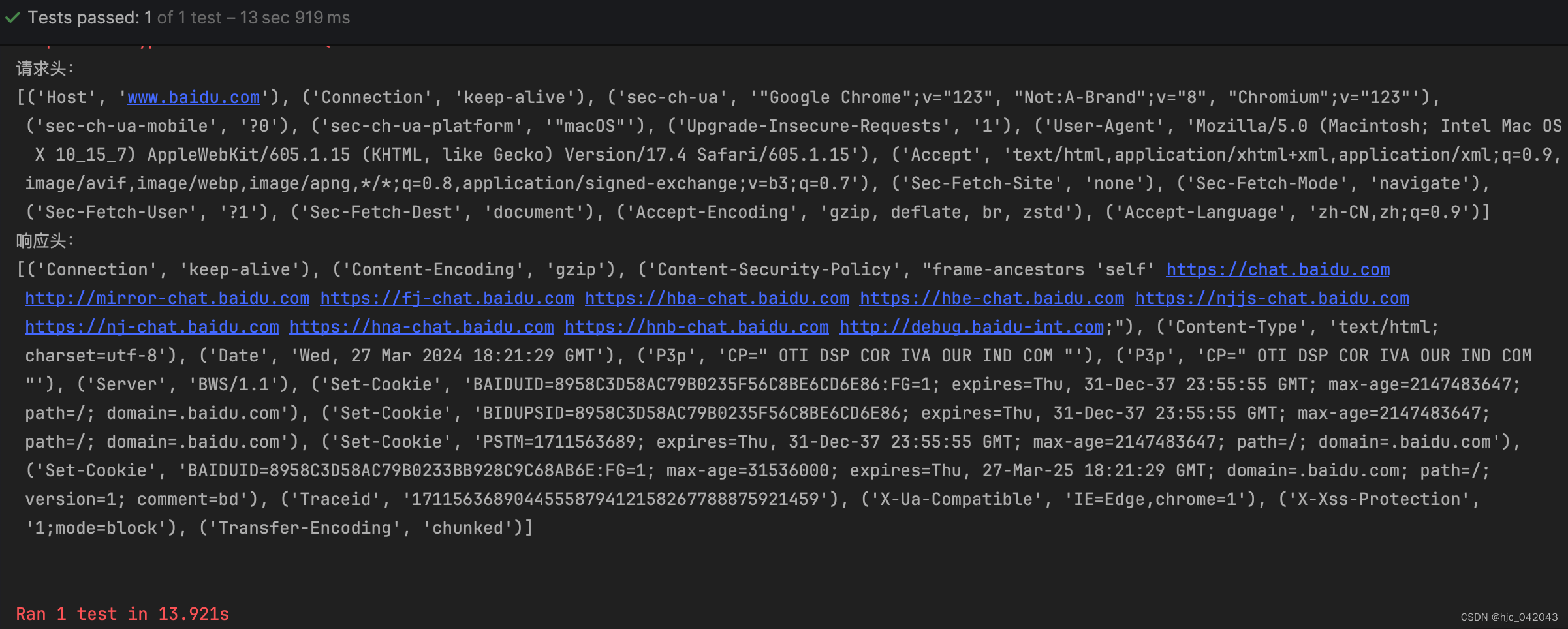
python爬虫之selenium4使用(万字讲解)
文章目录 一、前言二、selenium的介绍1、优点:2、缺点: 三、selenium环境搭建1、安装python模块2、selenium4新特性3、安装驱动WebDriver驱动选择驱动安装和测试 基础操作1、属性和方法2、单个元素定位通过id定位通过class_name定位一个元素通过xpath定位…...
【ARM 嵌入式 C 头文件系列 22 -- 头文件 stdint.h 介绍】
请阅读【嵌入式开发学习必备专栏 】 文章目录 C 头文件 stdint.h定长整数类型最小宽度整数类型最快最小宽度整数类型整数指针类型最大整数类型 C 头文件 stdint.h 在 C 语言中,头文件 <stdint.h> 是 C99 标准的一部分,旨在提供一组明确的整数类型…...
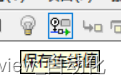
LabVIEW专栏三、探针和断点
探针和断点是LabVIEW调试的常用手段,该节以上一节的"测试耗时"为例 探针可以打在有线条的任何地方,打上后,经过这条线的所有最后一次的数值都会显示在探针窗口。断点可以打在程序框图的所有G代码对象,包括结构…...
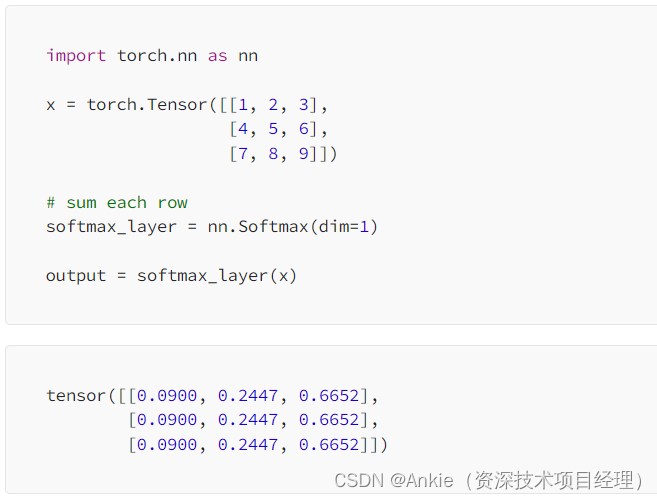
Transformer模型-softmax的简明介绍
今天介绍transformer模型的softmax softmax的定义和目的: softmax:常用于神经网络的输出层,以将原始的输出值转化为概率分布,从而使得每个类别的概率值在0到1之间,并且所有类别的概率之和为1。这使得Softmax函数特别适…...
记录一下做工厂的打印pdf程序
功能:在网页点击按钮调起本地的打印程序 本人想到的就是直接调起方式,网上大佬们说用注册表的形式来进行。 后面想到一种,在电脑开机时就开启,并在后台运行,等到有人去网页里面进行触发,这时候就有个问题&a…...
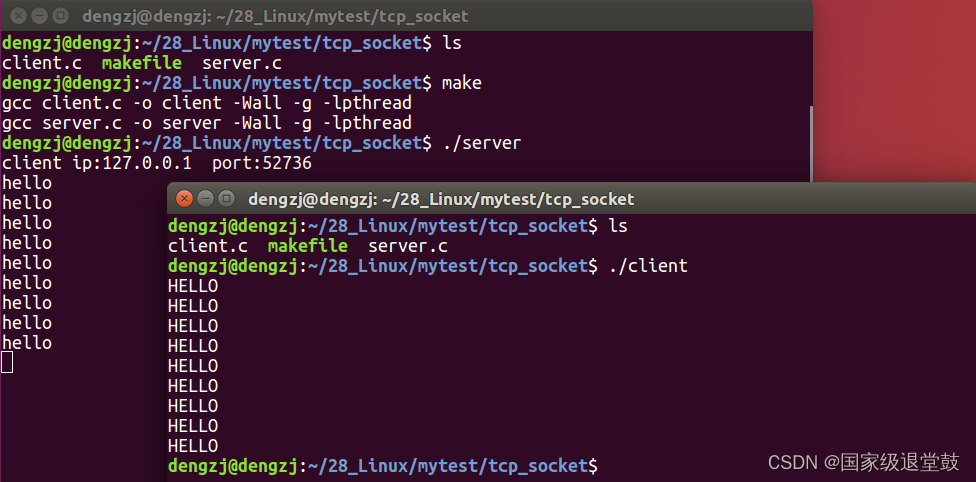
Linux网络编程一(协议、TCP协议、UDP、socket编程、TCP服务器端及客户端)
文章目录 协议1、分层模型结构2、网络应用程序设计模式3、ARP协议4、IP协议5、UDP协议6、TCP协议 Socket编程1、网络套接字(socket)2、网络字节序3、IP地址转换4、一系列函数5、TCP通信流程分析 第二次更新,自己再重新梳理一遍… 协议 协议:指一组规则&…...
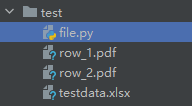
Python读取Excel根据每行信息生成一个PDF——并自定义添加文本,可用于制作准考证
文章目录 有点小bug的:最终代码(无换行):有换行最终代码无bug根据Excel自动生成PDF,目录结构如上 有点小bug的: # coding=utf-8 import pandas as pd from reportlab.pdfgen import canvas from reportlab.lib.pagesizes import letter from reportlab.pdfbase import pdf…...
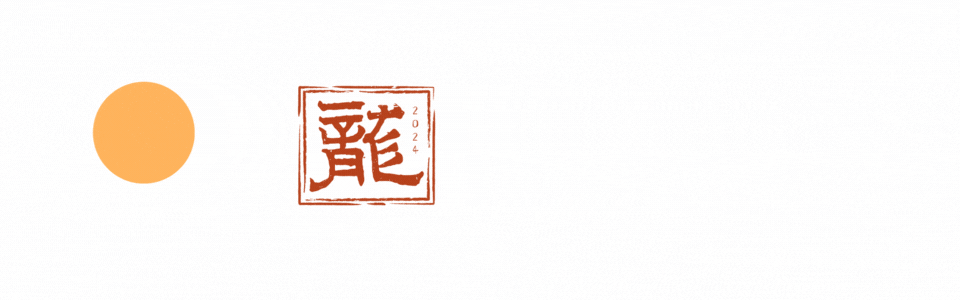
http: server gave HTTP response to HTTPS client 分析一下这个问题如何解决中文告诉我详细的解决方案
这个错误信息表明 Docker 客户端在尝试通过 HTTPS 协议连接到 Docker 仓库时,但是服务器却返回了一个 HTTP 响应。这通常意味着 Docker 仓库没有正确配置为使用 HTTPS,或者客户端没有正确配置以信任仓库的 SSL 证书。以下是几种可能的解决方案࿱…...
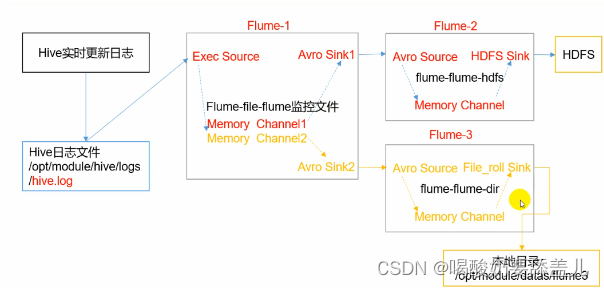
Flume学习笔记
视频地址:https://www.bilibili.com/video/BV1wf4y1G7EQ/ 定义 Flume是一个高可用的、高可靠的、分布式的海量日志采集、聚合和传输的系统。 Flume高最要的作用就是实时读取服务器本地磁盘的数据,将数据写入HDFS。 官网:https://flume.apache.org/releases/content/1.9.0/…...
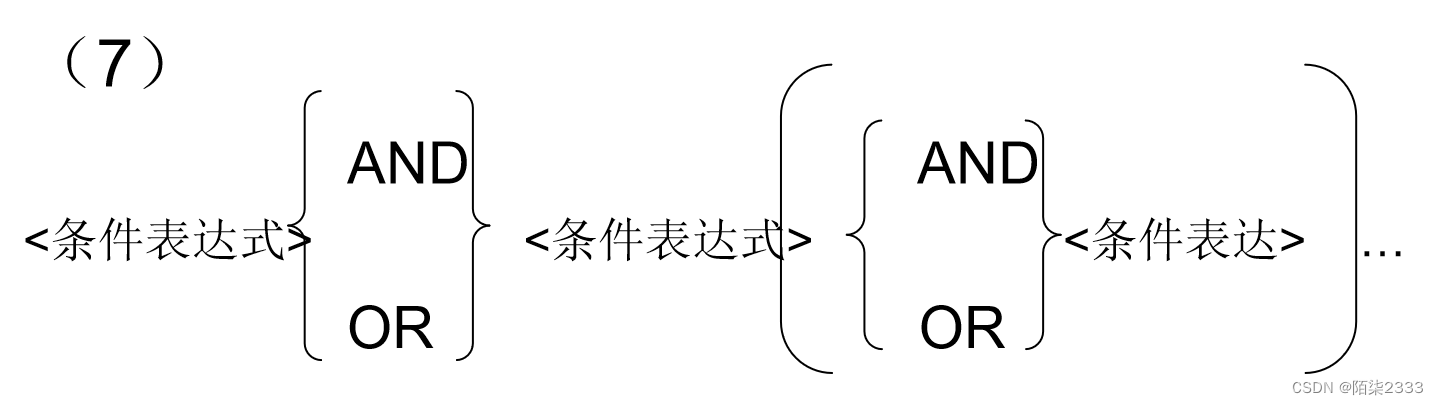
数据库系统概论(超详解!!!) 第三节 关系数据库标准语言SQL(Ⅳ)
1.集合查询 集合操作的种类 并操作UNION 交操作INTERSECT 差操作EXCEPT 参加集合操作的各查询结果的列数必须相同;对应项的数据类型也必须相同 查询计算机科学系的学生及年龄不大于19岁的学生。SELECT *FROM StudentWHERE Sdept CSUNIONSELECT *FROM StudentWHERE Sage&l…...
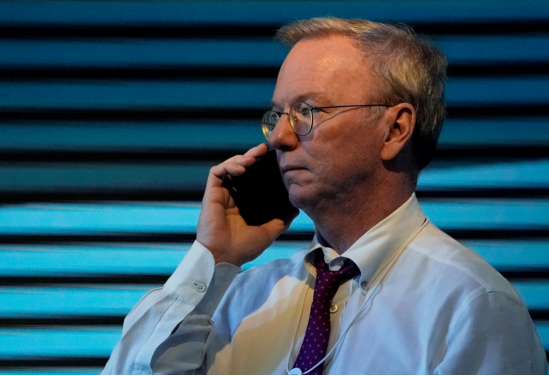
与谷歌“分家”两年后,SandboxAQ推出统一加密管理平台
3月27日,SandboxAQ宣布其AQtive Guard平台现已全面可用(GA),适用于所有行业,以防范人工智能驱动和量子攻击的威胁。前者是在两年前3月从谷歌母公司Alphabet分拆出来的初创公司,并在当时获得了“九位数”的融…...
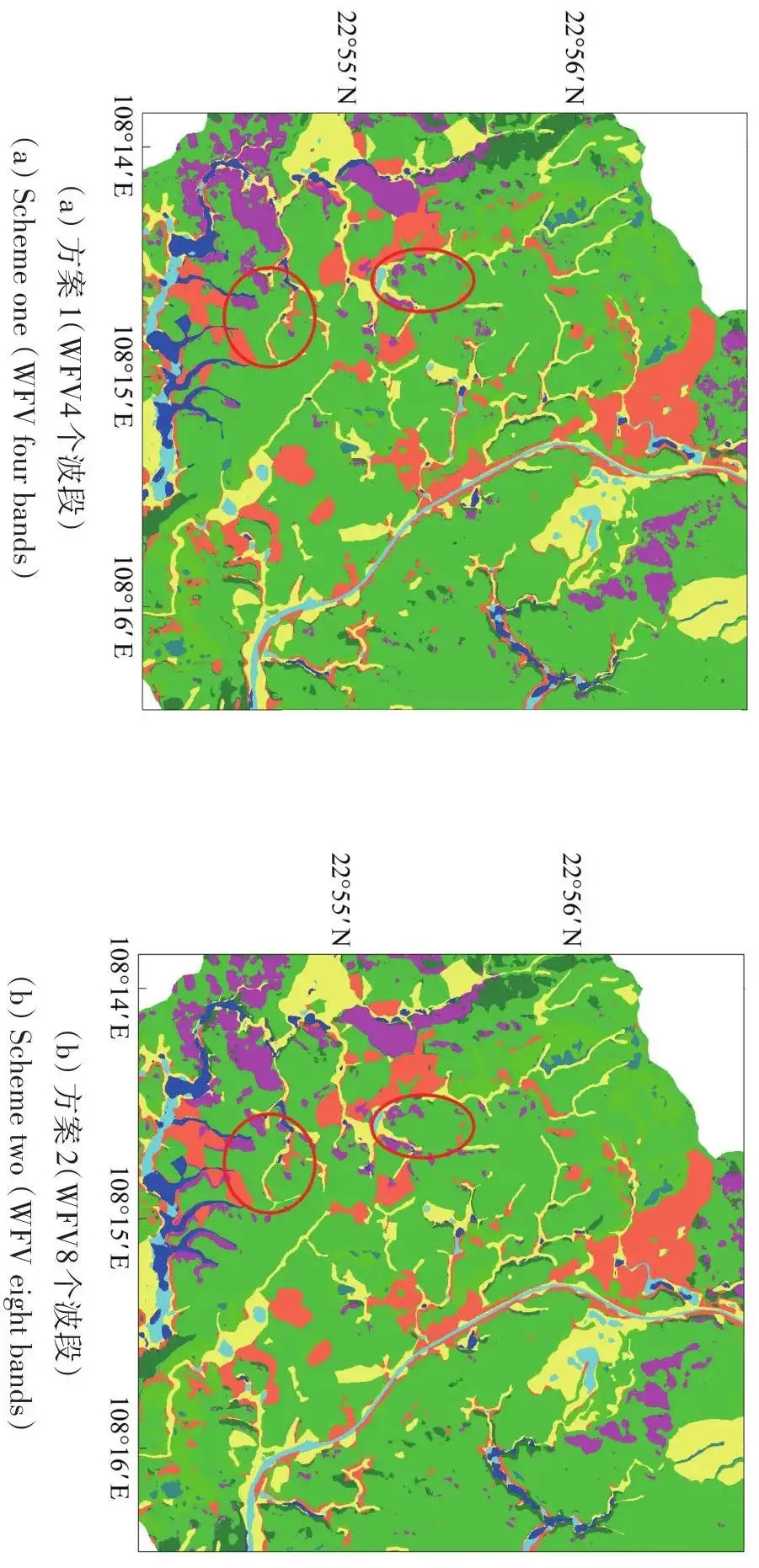
【卫星家族】 | 高分六号卫星影像及获取
1. 卫星简介 高分六号卫星(GF-6)于2018年6月2日在酒泉卫星发射中心成功发射,是高分专项中的一颗低轨光学遥感卫星,也是我国首颗精准农业观测的高分卫星,具有高分辨率、宽覆盖、高质量成像、高效能成像、国产化率高等特…...
XML与Xpath
XML与Xpath XML是一种具有某种层次结构的文件,Xpath则是解析这种文件的工具 接下来将会解释XML文件的结构和Xpath的基本使用,并且用Java语言进行操作展示。 XML结构 XML(可扩展标记语言)文件具有一种层次结构,由标签…...
【c++20】CPP-20-STL-Cookbook 学习笔记
Cpp20-STL-Cookbook-src简单的阅读笔记。c++20更好用了,比如STL 包含了一些这样的辅助函数,比如 make_pair() 和make_tuple() 等。 这些代码现在已经过时了,但是为了与旧代码兼容,会保留这些代码。比如 可以声明是一个std的string:Sum s1 {1u, 2.0, 3, 4.0f }?...

Python 之 Flask 框架学习
毕业那会使用过这个轻量级的框架,最近再来回看一下,依赖相关的就不多说了,直接从例子开始。下面示例中的 html 模板,千万记得要放到 templates 目录下。 Flask基础示例 hello world from flask import Flask, jsonify, url_fora…...
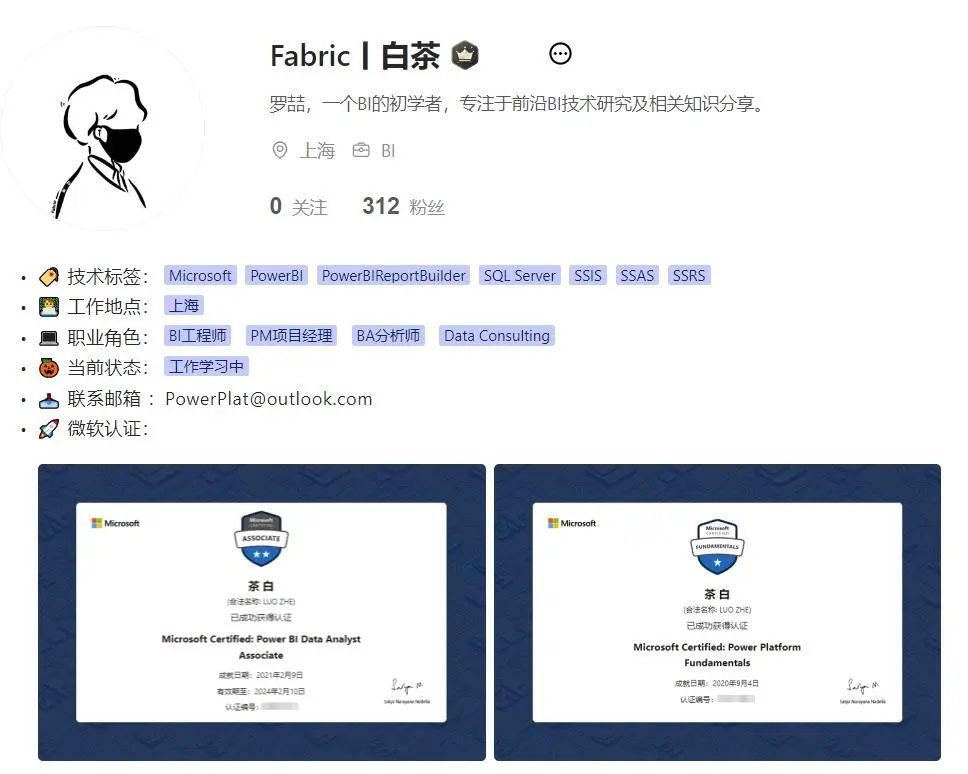
精品丨PowerBI负载测试和容量规划
当选择Power BI作为业务报表平台时,如何判断许可证的选择是否符合业务需求,价格占了主导因素。 Power BI的定价是基于SKU和服务器内核决定的,但是很多IT的负责人都不确定自己公司业务具体需要多少。 不幸的是,Power BI的容量和预期…...
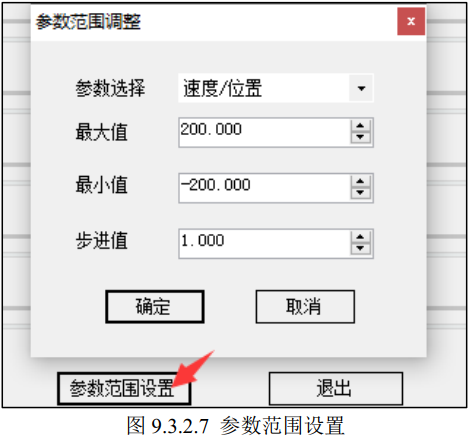
【算法-PID】
算法-PID ■ PID■ 闭环原理■ PID 控制流程■ PID 比例环节(Proportion)■ PID 积分环节(Integral)■ PID 微分环节(Differential) ■ 位置式PID,增量式PID介绍■ 位置式 PID 公式■ 增量式 PI…...
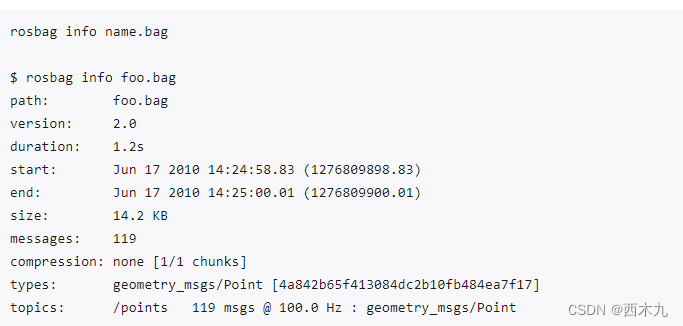
ros rosbag使用记录
rosbag: 1. rosbag record -a 记录当前所有消息(较少用)2. rosbag record -O bag_name.bag /topic 记录指定消息3. rosbag info 查阅bag文件信息4. rosbag play 播放bag文件内容5. python script 查看bag文件内容参考: 1. rosbag record -a 记…...
变量 varablie 声明- Rust 变量 let mut 声明与 C/C++ 变量声明对比分析
一、变量声明设计:let 与 mut 的哲学解析 Rust 采用 let 声明变量并通过 mut 显式标记可变性,这种设计体现了语言的核心哲学。以下是深度解析: 1.1 设计理念剖析 安全优先原则:默认不可变强制开发者明确声明意图 let x 5; …...
web vue 项目 Docker化部署
Web 项目 Docker 化部署详细教程 目录 Web 项目 Docker 化部署概述Dockerfile 详解 构建阶段生产阶段 构建和运行 Docker 镜像 1. Web 项目 Docker 化部署概述 Docker 化部署的主要步骤分为以下几个阶段: 构建阶段(Build Stage):…...
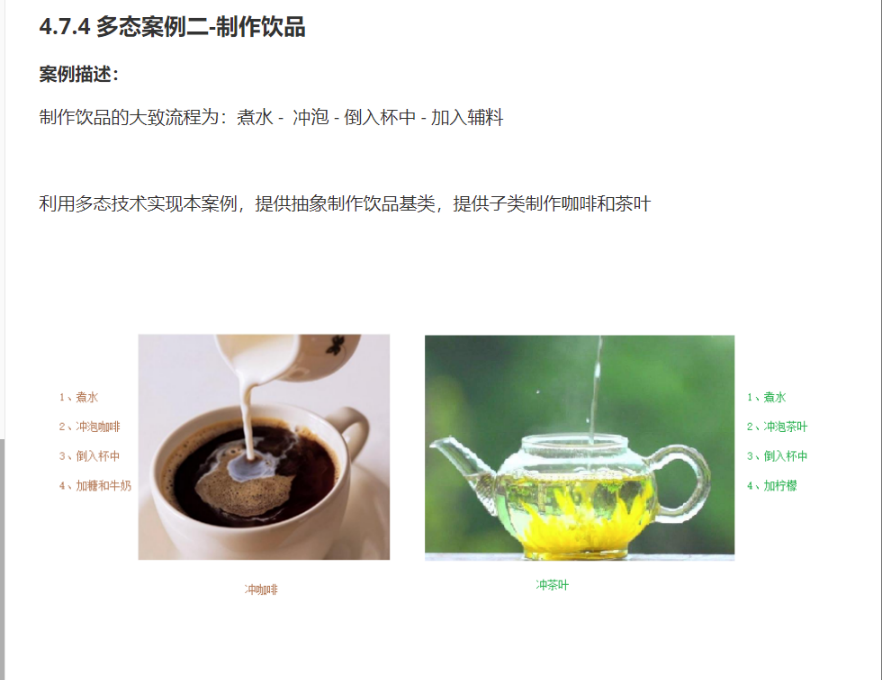
C++_核心编程_多态案例二-制作饮品
#include <iostream> #include <string> using namespace std;/*制作饮品的大致流程为:煮水 - 冲泡 - 倒入杯中 - 加入辅料 利用多态技术实现本案例,提供抽象制作饮品基类,提供子类制作咖啡和茶叶*//*基类*/ class AbstractDr…...
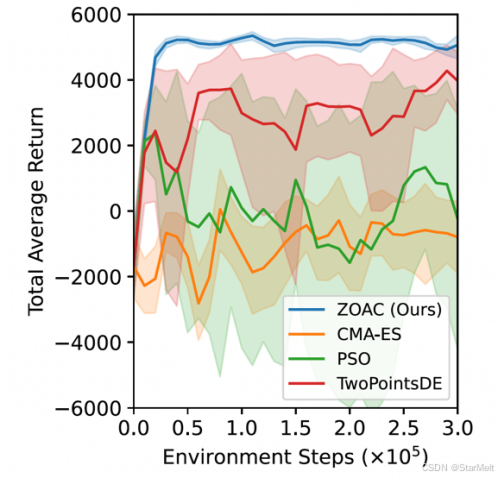
突破不可导策略的训练难题:零阶优化与强化学习的深度嵌合
强化学习(Reinforcement Learning, RL)是工业领域智能控制的重要方法。它的基本原理是将最优控制问题建模为马尔可夫决策过程,然后使用强化学习的Actor-Critic机制(中文译作“知行互动”机制),逐步迭代求解…...
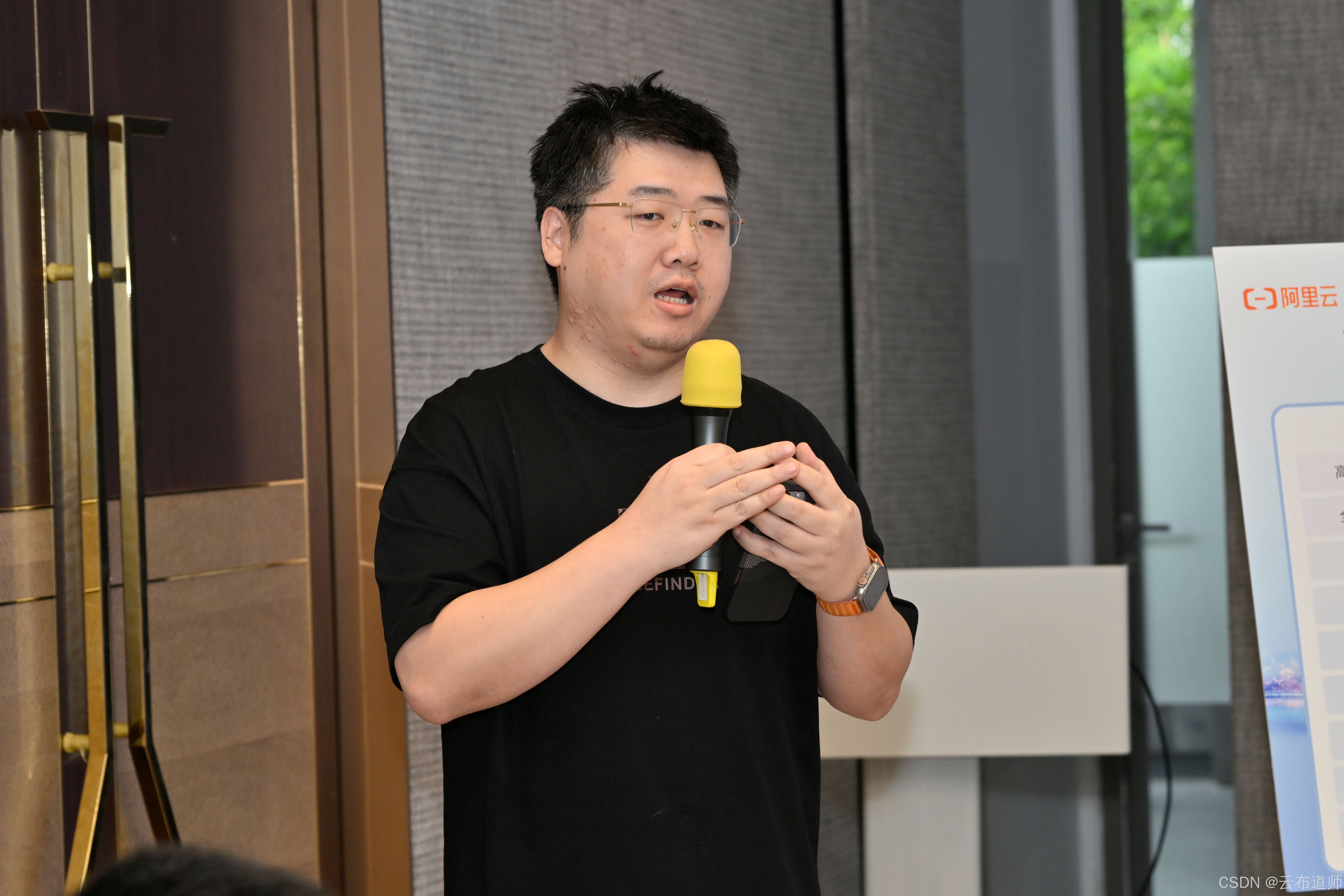
云启出海,智联未来|阿里云网络「企业出海」系列客户沙龙上海站圆满落地
借阿里云中企出海大会的东风,以**「云启出海,智联未来|打造安全可靠的出海云网络引擎」为主题的阿里云企业出海客户沙龙云网络&安全专场于5.28日下午在上海顺利举办,现场吸引了来自携程、小红书、米哈游、哔哩哔哩、波克城市、…...
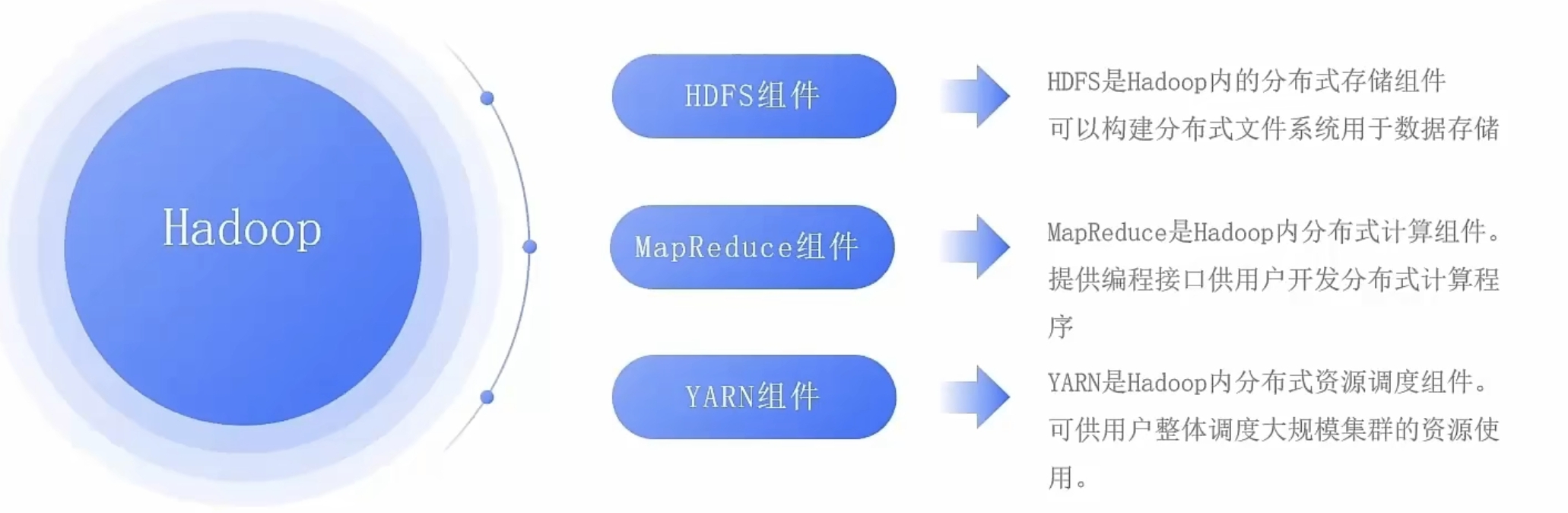
大数据零基础学习day1之环境准备和大数据初步理解
学习大数据会使用到多台Linux服务器。 一、环境准备 1、VMware 基于VMware构建Linux虚拟机 是大数据从业者或者IT从业者的必备技能之一也是成本低廉的方案 所以VMware虚拟机方案是必须要学习的。 (1)设置网关 打开VMware虚拟机,点击编辑…...
【磁盘】每天掌握一个Linux命令 - iostat
目录 【磁盘】每天掌握一个Linux命令 - iostat工具概述安装方式核心功能基础用法进阶操作实战案例面试题场景生产场景 注意事项 【磁盘】每天掌握一个Linux命令 - iostat 工具概述 iostat(I/O Statistics)是Linux系统下用于监视系统输入输出设备和CPU使…...
JDK 17 新特性
#JDK 17 新特性 /**************** 文本块 *****************/ python/scala中早就支持,不稀奇 String json “”" { “name”: “Java”, “version”: 17 } “”"; /**************** Switch 语句 -> 表达式 *****************/ 挺好的ÿ…...
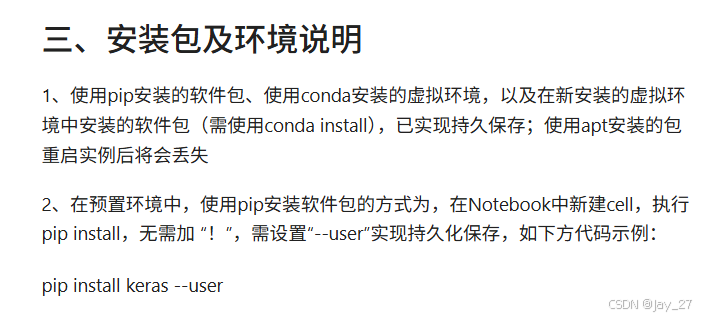
九天毕昇深度学习平台 | 如何安装库?
pip install 库名 -i https://pypi.tuna.tsinghua.edu.cn/simple --user 举个例子: 报错 ModuleNotFoundError: No module named torch 那么我需要安装 torch pip install torch -i https://pypi.tuna.tsinghua.edu.cn/simple --user pip install 库名&#x…...
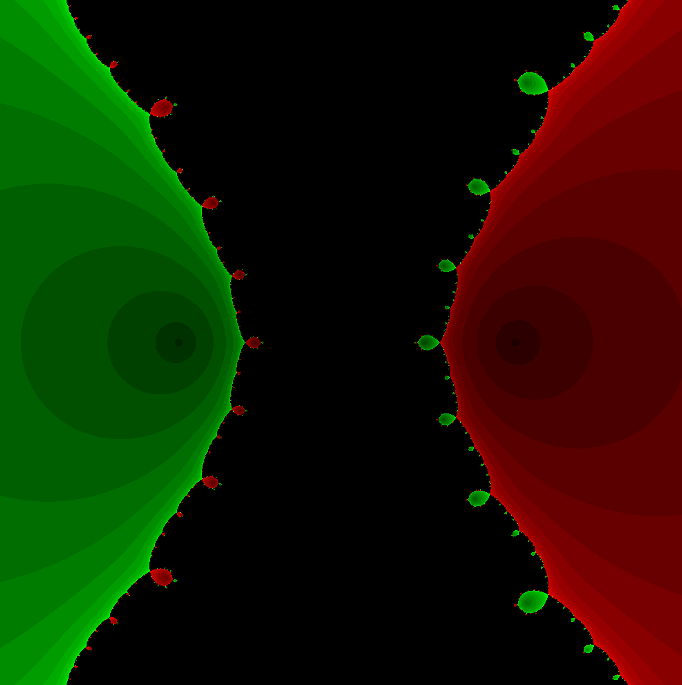
在Mathematica中实现Newton-Raphson迭代的收敛时间算法(一般三次多项式)
考察一般的三次多项式,以r为参数: p[z_, r_] : z^3 (r - 1) z - r; roots[r_] : z /. Solve[p[z, r] 0, z]; 此多项式的根为: 尽管看起来这个多项式是特殊的,其实一般的三次多项式都是可以通过线性变换化为这个形式…...