Fastgpt配合chatglm+m3e或ollama+m3e搭建个人知识库
概述:
人工智能大语言模型是近年来人工智能领域的一项重要技术,它的出现标志着自然语言处理领域的重大突破。这些模型利用深度学习和大规模数据训练,能够理解和生成人类语言,为各种应用场景提供了强大的文本处理能力。AI大语言模型的技术原理主要基于深度学习和自然语言处理技术,通过自监督学习和大规模文本数据的预训练来学习语言的表示。训练完成后,可以通过微调等方法,将模型应用于特定的任务和应用场景。
未来,AI大语言模型有望在更多领域发挥作用,包括自然语言理解、文本生成、对话系统、语言翻译等。它们可以用于自动摘要、文档生成、智能客服、智能问答等多种应用场景,为用户提供了更加智能和个性化的服务。
本文为学习大语言模型及FastGPT部署的学习笔记。通过直接部署**ChatGML3大语言模型**
或**OLLAMA模型管理工具**
配合FastGPT私有化搭建知识库。其中**one-api**
、**fastgpt**
是两种方法都需要部署的,其他的更建议使用ollama直接进行部署,切换模型方便快捷,易于管理。
硬件要求
以下配置仅作参考
**chatglm3-6b+m3e:**3060 12 ↑
**qwen:4b+m3e:**3060 12 ↑
**qwen:2b+m3e:**1660 6g↑
总结:模型量级越大所需显卡性能越高,过小的量级的大模型在低端cpu亦可运行,只是推理的精准度很差,更不能配合m3e向量模型进行推理,速度会非常慢。
本文档涉及到的资源
conda
https://www.anaconda.com/
Conda 是一个运行在 Windows、MacOS 和 Linux 上的开源包管理系统和环境管理系统。Conda 可以:
- 快速安装、运行和更新包及其依赖项
- 轻松地在本地计算机上创建、保存、加载和切换环境
它是为 Python 程序创建的,但它可以为任何语言打包和分发软件。
简单总结一下就是 Conda 很好、很强大,使用 Conda 会让你很省心。(人生苦短,我选 “Conda”!)
one-api
https://github.com/songquanpeng/one-api
All in one 的 OpenAI 接口 整合各种 API 访问方式 一键部署,开箱即用
chatglm3
https://github.com/THUDM/ChatGLM3
ChatGLM3 是智谱 AI 和清华大学 KEG 实验室联合发布的对话预训练模型。
m3e
https://modelscope.cn/models/Jerry0/m3e-base/summary
M3E 是 Moka Massive Mixed Embedding 的缩写
- Moka,此模型由 MokaAI 训练,开源和评测,训练脚本使用 uniem ,评测 BenchMark 使用 MTEB-zh
- Massive,此模型通过千万级 (2200w+) 的中文句对数据集进行训练
- Mixed,此模型支持中英双语的同质文本相似度计算,异质文本检索等功能,未来还会支持代码检索
- Embedding,此模型是文本嵌入模型,可以将自然语言转换成稠密的向量
ollama
https://ollama.com/
大语言模型管理工具
fastgpt
https://github.com/labring/FastGPT
FastGPT 是一个基于 LLM 大语言模型的知识库问答系统,提供开箱即用的数据处理、模型调用等能力。同时可以通过 Flow 可视化进行工作流编排,从而实现复杂的问答场景!
魔搭社区
https://modelscope.cn/home
ModelScope旨在打造下一代开源的模型即服务共享平台,为泛AI开发者提供灵活、易用、低成本的一站式模型服务产品,让模型应用更简单!
CONDA的使用
官网:https://www.anaconda.com/
- 安装好后更新工具
conda update -n base -c defaults conda
- 更新各种库
conda update --all
- 创建虚拟环境
conda create --name windows_chatglm3-6b python=3.11 -y
- 激活并进入虚拟环境
conda activate windows_chatglm3-6b
- 安装pytorch
cmd:nvidia-smi
查看最高支持的 CUDA Version,我的是12.2
- 安装pytorch
PyTorch是一个开源的Python机器学习库,基于Torch,用于自然语言处理等应用程序。PyTorch既可以看作加入了GPU支持的numpy,同时也可以看成一个拥有自动求导功能的强大的深度神经网络 。
- 在 https://pytorch.org/get-started/locally/ 查询自己电脑需要执行的命令
- 在conda虚拟环境内执行以下命令安装pytorch
conda install pytorch torchvision torchaudio pytorch-cuda=12.1 -c pytorch -c nvidia
chatglm3环境搭建(非ollama模式)
模型及DEMO下载
一共需要下载两个模型chatglm3 及m3e
chatglm3下载
模型地址:https://modelscope.cn/models/ZhipuAI/chatglm3-6b/summary
下载方法:git
下载时间较久,耐心等待
git lfs install
git clone https://www.modelscope.cn/ZhipuAI/chatglm3-6b.git
m3e-base下载
git clone https://www.modelscope.cn/Jerry0/m3e-base.git
官方ChatGLM3 DEMO下载
地址:https://github.com/THUDM/ChatGLM3
下载方法:git
git clone https://github.com/THUDM/ChatGLM3
配置及运行
-
进入刚刚clone的 ChatGLM3/openai_api_demo文件夹
-
打开
api_server.py
的python文件 -
代码拉倒最下方
-
覆盖if name == “main”:方法内的代码 如下:
其中一些地方需要修改,`tokenizer`及`model`的地址对应的是[chatglm3](#eB5m3)的下载地址,`embedding_model`的地址对应的是[m3e](#FHYRP)的下载地址,`port`可根据个人需要自行配置
tokenizer = AutoTokenizer.from_pretrained("E:\Work\HaoQue\FastGPT\models\chatglm3-6B-32k-int4", trust_remote_code=True)model = AutoModel.from_pretrained("E:\Work\HaoQue\FastGPT\models\chatglm3-6B-32k-int4", trust_remote_code=True, device_map="auto").eval()
# load Embeddingembedding_model = SentenceTransformer("E:\Work\HaoQue\FastGPT\models\m3e-base", trust_remote_code=True, device="cuda")uvicorn.run(app, host='0.0.0.0', port=8000, workers=1)
- 回到ChatGLM3根目录 进入刚刚创建的
windows_chatglm3-6b
conda 虚拟环境 - cmd运行
pip install -r requirements.txt
安装依赖 - 耐心等待安装完成
- 完成后运行通过python运行
python openai_api_demo/api_server.py
- 查看运行结果,以下为运行成功。
ollama环境搭建
ollama程序下载及模型安装安装
- 下载:https://ollama.com/download
- 安装直接下一步
- 安装完成后进入cmd 输入
ollama -v
验证是否成功
通过ollama进行模型下载
- 模型列表:https://ollama.com/library
- 这里以qwen:1.8b为例
- cmd运行
ollama run qwen:1.8b
- 耐心等待下载即可
- 下载完成模型会自动启动,无需其他操作
m3e环境搭建(ollama模式)
使用docker进行部署,docker安装在此不做介绍。
docker run -d --name m3e -p 6008:6008 --gpus all -e sk-key=123321 registry.cn-hangzhou.aliyuncs.com/fastgpt_docker/m3e-large-api
one-api环境部署及配置
- 使用docker进行部署,docker安装在此不做介绍。
docker run --name one-api -d --restart always -p 3000:3000 -e TZ=Asia/Shanghai -v /home/ubuntu/data/one-api:/data justsong/one-api
- 然后访问
[http://localhost:3000/](http://localhost:3001/)
端口为docker run 时候-p的端口,
- 登陆 初始账号用户名为 root,密码为 123456。
- 登陆后来到渠道页面添加渠道,此步骤添加的是大语言模型
- 如果你是通过ollama运行的大模型,则需要再次添加新渠道,本次添加m3e渠道
- 新建令牌
- 新建令牌后,复制令牌sdk备用
fastgpt环境搭建
参考文档:https://doc.fastai.site/docs/development/docker/
- 非 Linux 环境或无法访问外网环境,可手动创建一个目录,并下载下面2个链接的文件: docker-compose.yml,config.json
注意: docker-compose.yml 配置文件中 Mongo 为 5.x,部分服务器不支持,需手动更改其镜像版本为 4.4.24(需要自己在docker hub下载,阿里云镜像没做备份)
- config.json配置文件修改
- 打开下载的config.json
- 复制并替换
**llmModels**
数组中的第一组数据,修改model和name属性为你部署的模型属性,其他可以不做修改
{"model": "gemma:2b","name": "gemma:2b","maxContext": 16000,"avatar": "/imgs/model/openai.svg","maxResponse": 4000,"quoteMaxToken": 13000,"maxTemperature": 1.2,"charsPointsPrice": 0,"censor": false,"vision": false,"datasetProcess": true,"usedInClassify": true,"usedInExtractFields": true,"usedInToolCall": true,"usedInQueryExtension": true,"toolChoice": true,"functionCall": true,"customCQPrompt": "","customExtractPrompt": "","defaultSystemChatPrompt": "","defaultConfig": {}},
- 如果你是ollama部署的大模型
- 打开下载的config.json
- 在
**vectorModels**
数组中添加以下数据
{"model": "m3e","name": "M3E","inputPrice": 0,"outputPrice": 0,"defaultToken": 700,"maxToken": 1800,"weight": 100}
- 打开docker-compose.yml
- 注释掉mysql 及 oneapi相关配置
- **启动容器 **
在 docker-compose.yml 同级目录下执行。请确保docker-compose版本最好在2.17以上,否则可能无法执行自动化命令。
# 启动容器
docker-compose up -d
# 等待10s,OneAPI第一次总是要重启几次才能连上Mysql
sleep 10
# 重启一次oneapi(由于OneAPI的默认Key有点问题,不重启的话会提示找不到渠道,临时手动重启一次解决,等待作者修复)
docker restart oneapi
- 访问 FastGPT
目前可以通过 ip:3000 直接访问(注意防火墙)。登录用户名为 root,密码为docker-compose.yml环境变量里设置的 DEFAULT_ROOT_PSW。
如果需要域名访问,请自行安装并配置 Nginx。
首次运行,会自动初始化 root 用户,密码为 1234
- 新建知识库
- 上传知识库文件
- 新建AI应用
- 开始使用
运行报错
报huggingface-hub的错
pip install huggingface-hub==0.20.3
显存不足尝试设置环境变量
set PYTORCH_CUDA_ALLOC_COFF=expandable_segments:True
再次运行python api_server.py(经测试无用)
torch.cuda.OutOfMemoryError: CUDA out of memory. Tried to allocate 108.00 MiB. GPU 0 has a total capacity of 6.00 GiB of which 0 bytes is free. Of the allocated memory 12.31 GiB is allocated by PyTorch, and 1.27 MiB is reserved by PyTorch but unallocated. If reserved but unallocated memory is large try setting PYTORCH_CUDA_ALLOC_CONF=expandable_segments:True to avoid fragmentation. See documentation for Memory Management (https://pytorch.org/docs/stable/notes/cuda.html#environment-variables))
api_server.py整体
"""
This script implements an API for the ChatGLM3-6B model,
formatted similarly to OpenAI's API (https://platform.openai.com/docs/api-reference/chat).
It's designed to be run as a web server using FastAPI and uvicorn,
making the ChatGLM3-6B model accessible through OpenAI Client.Key Components and Features:
- Model and Tokenizer Setup: Configures the model and tokenizer paths and loads them.
- FastAPI Configuration: Sets up a FastAPI application with CORS middleware for handling cross-origin requests.
- API Endpoints:- "/v1/models": Lists the available models, specifically ChatGLM3-6B.- "/v1/chat/completions": Processes chat completion requests with options for streaming and regular responses.- "/v1/embeddings": Processes Embedding request of a list of text inputs.
- Token Limit Caution: In the OpenAI API, 'max_tokens' is equivalent to HuggingFace's 'max_new_tokens', not 'max_length'.
For instance, setting 'max_tokens' to 8192 for a 6b model would result in an error due to the model's inability to output
that many tokens after accounting for the history and prompt tokens.
- Stream Handling and Custom Functions: Manages streaming responses and custom function calls within chat responses.
- Pydantic Models: Defines structured models for requests and responses, enhancing API documentation and type safety.
- Main Execution: Initializes the model and tokenizer, and starts the FastAPI app on the designated host and port.Note:This script doesn't include the setup for special tokens or multi-GPU support by default.Users need to configure their special tokens and can enable multi-GPU support as per the provided instructions.Embedding Models only support in One GPU.Running this script requires 14-15GB of GPU memory. 2 GB for the embedding model and 12-13 GB for the FP16 ChatGLM3 LLM."""import os
import time
import tiktoken
import torch
import uvicornfrom fastapi import FastAPI, HTTPException, Response
from fastapi.middleware.cors import CORSMiddlewarefrom contextlib import asynccontextmanager
from typing import List, Literal, Optional, Union
from loguru import logger
from pydantic import BaseModel, Field
from transformers import AutoTokenizer, AutoModel
from utils import process_response, generate_chatglm3, generate_stream_chatglm3
from sentence_transformers import SentenceTransformerfrom sse_starlette.sse import EventSourceResponse# Set up limit request time
EventSourceResponse.DEFAULT_PING_INTERVAL = 1000000# set LLM path
MODEL_PATH = os.environ.get('MODEL_PATH', 'D:\WangMing\FastGPT\models\chatglm3-6b-copy')
TOKENIZER_PATH = os.environ.get("TOKENIZER_PATH", 'D:\WangMing\FastGPT\models\chatglm3-6b-copy')# set Embedding Model path
EMBEDDING_PATH = os.environ.get('EMBEDDING_PATH', 'D:\WangMing\FastGPT\models\m3e-base')@asynccontextmanager
async def lifespan(app: FastAPI):yieldif torch.cuda.is_available():torch.cuda.empty_cache()torch.cuda.ipc_collect()app = FastAPI(lifespan=lifespan)app.add_middleware(CORSMiddleware,allow_origins=["*"],allow_credentials=True,allow_methods=["*"],allow_headers=["*"],
)class ModelCard(BaseModel):id: strobject: str = "model"created: int = Field(default_factory=lambda: int(time.time()))owned_by: str = "owner"root: Optional[str] = Noneparent: Optional[str] = Nonepermission: Optional[list] = Noneclass ModelList(BaseModel):object: str = "list"data: List[ModelCard] = []class FunctionCallResponse(BaseModel):name: Optional[str] = Nonearguments: Optional[str] = Noneclass ChatMessage(BaseModel):role: Literal["user", "assistant", "system", "function"]content: str = Nonename: Optional[str] = Nonefunction_call: Optional[FunctionCallResponse] = Noneclass DeltaMessage(BaseModel):role: Optional[Literal["user", "assistant", "system"]] = Nonecontent: Optional[str] = Nonefunction_call: Optional[FunctionCallResponse] = None## for Embedding
class EmbeddingRequest(BaseModel):input: List[str]model: strclass CompletionUsage(BaseModel):prompt_tokens: intcompletion_tokens: inttotal_tokens: intclass EmbeddingResponse(BaseModel):data: listmodel: strobject: strusage: CompletionUsage# for ChatCompletionRequestclass UsageInfo(BaseModel):prompt_tokens: int = 0total_tokens: int = 0completion_tokens: Optional[int] = 0class ChatCompletionRequest(BaseModel):model: strmessages: List[ChatMessage]temperature: Optional[float] = 0.8top_p: Optional[float] = 0.8max_tokens: Optional[int] = Nonestream: Optional[bool] = Falsetools: Optional[Union[dict, List[dict]]] = Nonerepetition_penalty: Optional[float] = 1.1class ChatCompletionResponseChoice(BaseModel):index: intmessage: ChatMessagefinish_reason: Literal["stop", "length", "function_call"]class ChatCompletionResponseStreamChoice(BaseModel):delta: DeltaMessagefinish_reason: Optional[Literal["stop", "length", "function_call"]]index: intclass ChatCompletionResponse(BaseModel):model: strid: strobject: Literal["chat.completion", "chat.completion.chunk"]choices: List[Union[ChatCompletionResponseChoice, ChatCompletionResponseStreamChoice]]created: Optional[int] = Field(default_factory=lambda: int(time.time()))usage: Optional[UsageInfo] = None@app.get("/health")
async def health() -> Response:"""Health check."""return Response(status_code=200)@app.post("/v1/embeddings", response_model=EmbeddingResponse)
async def get_embeddings(request: EmbeddingRequest):embeddings = [embedding_model.encode(text) for text in request.input]embeddings = [embedding.tolist() for embedding in embeddings]def num_tokens_from_string(string: str) -> int:"""Returns the number of tokens in a text string.use cl100k_base tokenizer"""encoding = tiktoken.get_encoding('cl100k_base')num_tokens = len(encoding.encode(string))return num_tokensresponse = {"data": [{"object": "embedding","embedding": embedding,"index": index}for index, embedding in enumerate(embeddings)],"model": request.model,"object": "list","usage": CompletionUsage(prompt_tokens=sum(len(text.split()) for text in request.input),completion_tokens=0,total_tokens=sum(num_tokens_from_string(text) for text in request.input),)}return response@app.get("/v1/models", response_model=ModelList)
async def list_models():model_card = ModelCard(id="chatglm3-6b")return ModelList(data=[model_card])@app.post("/v1/chat/completions", response_model=ChatCompletionResponse)
async def create_chat_completion(request: ChatCompletionRequest):global model, tokenizerif len(request.messages) < 1 or request.messages[-1].role == "assistant":raise HTTPException(status_code=400, detail="Invalid request")gen_params = dict(messages=request.messages,temperature=request.temperature,top_p=request.top_p,max_tokens=request.max_tokens or 1024,echo=False,stream=request.stream,repetition_penalty=request.repetition_penalty,tools=request.tools,)logger.debug(f"==== request ====\n{gen_params}")if request.stream:# Use the stream mode to read the first few characters, if it is not a function call, direct stram outputpredict_stream_generator = predict_stream(request.model, gen_params)output = next(predict_stream_generator)if not contains_custom_function(output):return EventSourceResponse(predict_stream_generator, media_type="text/event-stream")# Obtain the result directly at one time and determine whether tools needs to be called.logger.debug(f"First result output:\n{output}")function_call = Noneif output and request.tools:try:function_call = process_response(output, use_tool=True)except:logger.warning("Failed to parse tool call")# CallFunctionif isinstance(function_call, dict):function_call = FunctionCallResponse(**function_call)"""In this demo, we did not register any tools.You can use the tools that have been implemented in our `tools_using_demo` and implement your own streaming tool implementation here.Similar to the following method:function_args = json.loads(function_call.arguments)tool_response = dispatch_tool(tool_name: str, tool_params: dict)"""tool_response = ""if not gen_params.get("messages"):gen_params["messages"] = []gen_params["messages"].append(ChatMessage(role="assistant",content=output,))gen_params["messages"].append(ChatMessage(role="function",name=function_call.name,content=tool_response,))# Streaming output of results after function callsgenerate = predict(request.model, gen_params)return EventSourceResponse(generate, media_type="text/event-stream")else:# Handled to avoid exceptions in the above parsing function process.generate = parse_output_text(request.model, output)return EventSourceResponse(generate, media_type="text/event-stream")# Here is the handling of stream = Falseresponse = generate_chatglm3(model, tokenizer, gen_params)# Remove the first newline characterif response["text"].startswith("\n"):response["text"] = response["text"][1:]response["text"] = response["text"].strip()usage = UsageInfo()function_call, finish_reason = None, "stop"if request.tools:try:function_call = process_response(response["text"], use_tool=True)except:logger.warning("Failed to parse tool call, maybe the response is not a tool call or have been answered.")if isinstance(function_call, dict):finish_reason = "function_call"function_call = FunctionCallResponse(**function_call)message = ChatMessage(role="assistant",content=response["text"],function_call=function_call if isinstance(function_call, FunctionCallResponse) else None,)logger.debug(f"==== message ====\n{message}")choice_data = ChatCompletionResponseChoice(index=0,message=message,finish_reason=finish_reason,)task_usage = UsageInfo.model_validate(response["usage"])for usage_key, usage_value in task_usage.model_dump().items():setattr(usage, usage_key, getattr(usage, usage_key) + usage_value)return ChatCompletionResponse(model=request.model,id="", # for open_source model, id is emptychoices=[choice_data],object="chat.completion",usage=usage)async def predict(model_id: str, params: dict):global model, tokenizerchoice_data = ChatCompletionResponseStreamChoice(index=0,delta=DeltaMessage(role="assistant"),finish_reason=None)chunk = ChatCompletionResponse(model=model_id, id="", choices=[choice_data], object="chat.completion.chunk")yield "{}".format(chunk.model_dump_json(exclude_unset=True))previous_text = ""for new_response in generate_stream_chatglm3(model, tokenizer, params):decoded_unicode = new_response["text"]delta_text = decoded_unicode[len(previous_text):]previous_text = decoded_unicodefinish_reason = new_response["finish_reason"]if len(delta_text) == 0 and finish_reason != "function_call":continuefunction_call = Noneif finish_reason == "function_call":try:function_call = process_response(decoded_unicode, use_tool=True)except:logger.warning("Failed to parse tool call, maybe the response is not a tool call or have been answered.")if isinstance(function_call, dict):function_call = FunctionCallResponse(**function_call)delta = DeltaMessage(content=delta_text,role="assistant",function_call=function_call if isinstance(function_call, FunctionCallResponse) else None,)choice_data = ChatCompletionResponseStreamChoice(index=0,delta=delta,finish_reason=finish_reason)chunk = ChatCompletionResponse(model=model_id,id="",choices=[choice_data],object="chat.completion.chunk")yield "{}".format(chunk.model_dump_json(exclude_unset=True))choice_data = ChatCompletionResponseStreamChoice(index=0,delta=DeltaMessage(),finish_reason="stop")chunk = ChatCompletionResponse(model=model_id,id="",choices=[choice_data],object="chat.completion.chunk")yield "{}".format(chunk.model_dump_json(exclude_unset=True))yield '[DONE]'def predict_stream(model_id, gen_params):"""The function call is compatible with stream mode output.The first seven characters are determined.If not a function call, the stream output is directly generated.Otherwise, the complete character content of the function call is returned.:param model_id::param gen_params::return:"""output = ""is_function_call = Falsehas_send_first_chunk = Falsefor new_response in generate_stream_chatglm3(model, tokenizer, gen_params):decoded_unicode = new_response["text"]delta_text = decoded_unicode[len(output):]output = decoded_unicode# When it is not a function call and the character length is> 7,# try to judge whether it is a function call according to the special function prefixif not is_function_call and len(output) > 7:# Determine whether a function is calledis_function_call = contains_custom_function(output)if is_function_call:continue# Non-function call, direct stream outputfinish_reason = new_response["finish_reason"]# Send an empty string first to avoid truncation by subsequent next() operations.if not has_send_first_chunk:message = DeltaMessage(content="",role="assistant",function_call=None,)choice_data = ChatCompletionResponseStreamChoice(index=0,delta=message,finish_reason=finish_reason)chunk = ChatCompletionResponse(model=model_id,id="",choices=[choice_data],created=int(time.time()),object="chat.completion.chunk")yield "{}".format(chunk.model_dump_json(exclude_unset=True))send_msg = delta_text if has_send_first_chunk else outputhas_send_first_chunk = Truemessage = DeltaMessage(content=send_msg,role="assistant",function_call=None,)choice_data = ChatCompletionResponseStreamChoice(index=0,delta=message,finish_reason=finish_reason)chunk = ChatCompletionResponse(model=model_id,id="",choices=[choice_data],created=int(time.time()),object="chat.completion.chunk")yield "{}".format(chunk.model_dump_json(exclude_unset=True))if is_function_call:yield outputelse:yield '[DONE]'async def parse_output_text(model_id: str, value: str):"""Directly output the text content of value:param model_id::param value::return:"""choice_data = ChatCompletionResponseStreamChoice(index=0,delta=DeltaMessage(role="assistant", content=value),finish_reason=None)chunk = ChatCompletionResponse(model=model_id, id="", choices=[choice_data], object="chat.completion.chunk")yield "{}".format(chunk.model_dump_json(exclude_unset=True))choice_data = ChatCompletionResponseStreamChoice(index=0,delta=DeltaMessage(),finish_reason="stop")chunk = ChatCompletionResponse(model=model_id, id="", choices=[choice_data], object="chat.completion.chunk")yield "{}".format(chunk.model_dump_json(exclude_unset=True))yield '[DONE]'def contains_custom_function(value: str) -> bool:"""Determine whether 'function_call' according to a special function prefix.For example, the functions defined in "tools_using_demo/tool_register.py" are all "get_xxx" and start with "get_"[Note] This is not a rigorous judgment method, only for reference.:param value::return:"""return value and 'get_' in valueif __name__ == "__main__":# Load LLMtokenizer = AutoTokenizer.from_pretrained("D:\WangMing\FastGPT\models\chatglm3-6b-copy", trust_remote_code=True)model = AutoModel.from_pretrained("D:\WangMing\FastGPT\models\chatglm3-6b-copy", trust_remote_code=True, device_map="auto").quantize(4).eval()# load Embeddingembedding_model = SentenceTransformer("D:\WangMing\FastGPT\models\chatglm3-6b-copy", trust_remote_code=True, device="cuda")uvicorn.run(app, host='0.0.0.0', port=8000, workers=1)
相关文章:
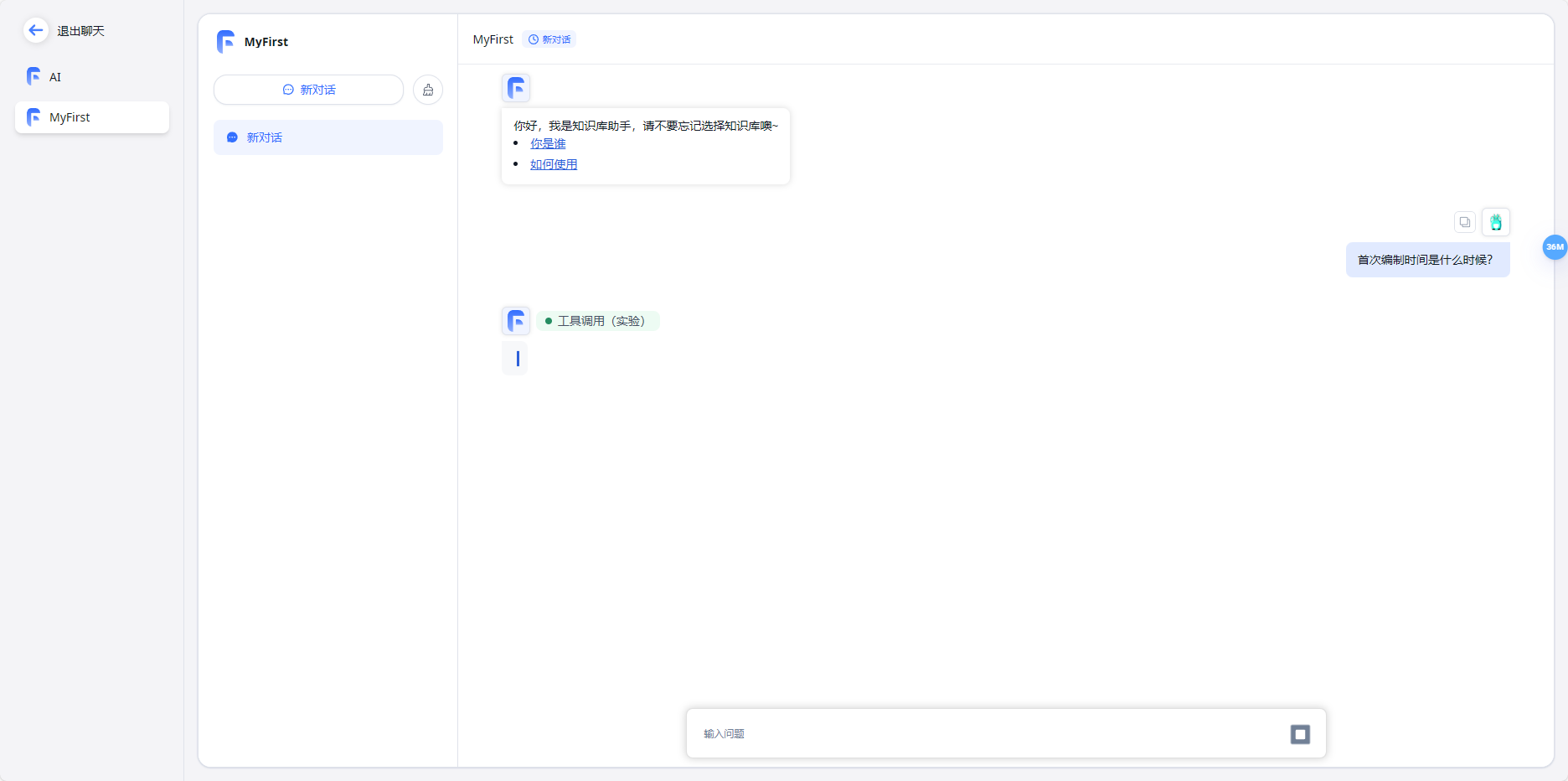
Fastgpt配合chatglm+m3e或ollama+m3e搭建个人知识库
概述: 人工智能大语言模型是近年来人工智能领域的一项重要技术,它的出现标志着自然语言处理领域的重大突破。这些模型利用深度学习和大规模数据训练,能够理解和生成人类语言,为各种应用场景提供了强大的文本处理能力。AI大语言模…...

如何使用选择器精确地控制网页中每一个元素的样式?
1. 基础知识 什么是 CSS 元素选择器 CSS 元素选择器是一种在网页中通过元素类型来应用样式的方法。 简单来说,它就像是一个指挥棒,告诉浏览器哪些 HTML 元素需要应用我们定义的 CSS 样式规则。 为何要使用 CSS 元素选择器 使用元素选择器可以让我们…...

各个微前端框架的优劣浅谈
各个微前端框架都有其独特的优势和劣势,下面我将针对几个主流的微前端框架进行简要的优劣分析: single-spa 优势: 轻量级:single-spa是一个非常轻量级的微前端框架,它主要提供了一个加载和管理微应用的机制,…...

自动化运维(二十二)Ansible实战 之Jenkins模块
Ansible提供了一些模块,可以用来与Jenkins进行交互,执行各种操作,如创建任务、触发构建、获取构建结果等。通过使用这些模块,我们可以将Jenkins的配置和管理集成到Ansible的自动化流程中。 以下是一些常用的Ansible Jenkins模块: 1、jenkins_job模块 jenkins_job模块用于创建…...

Python数据分析与应用 |第4章 使用pandas进行数据预处理 (实训)
表1-1healthcare-dataset-stroke.xlsx 部分中风患者的基础信息和体检数据 编号性别高血压是否结婚工作类型居住类型体重指数吸烟史中风9046男否是私人城市36.6以前吸烟是51676女否是私营企业农村N/A从不吸烟是31112男否是私人农村32.5从不吸烟...

基于双向长短期神经网络BILSTM的线损率预测,基于gru的线损率预测
目录 背影 摘要 LSTM的基本定义 LSTM实现的步骤 BILSTM神经网络 基于双向长短期神经网络BILSTM的线损率预测,基于gru的线损率预测 完整代码:基于双向长短期神经网络BILSTM的线损率预测,基于gru的线损率预测(代码完整,数据齐全)资源-CSDN文库 https://download.csdn.net/d…...
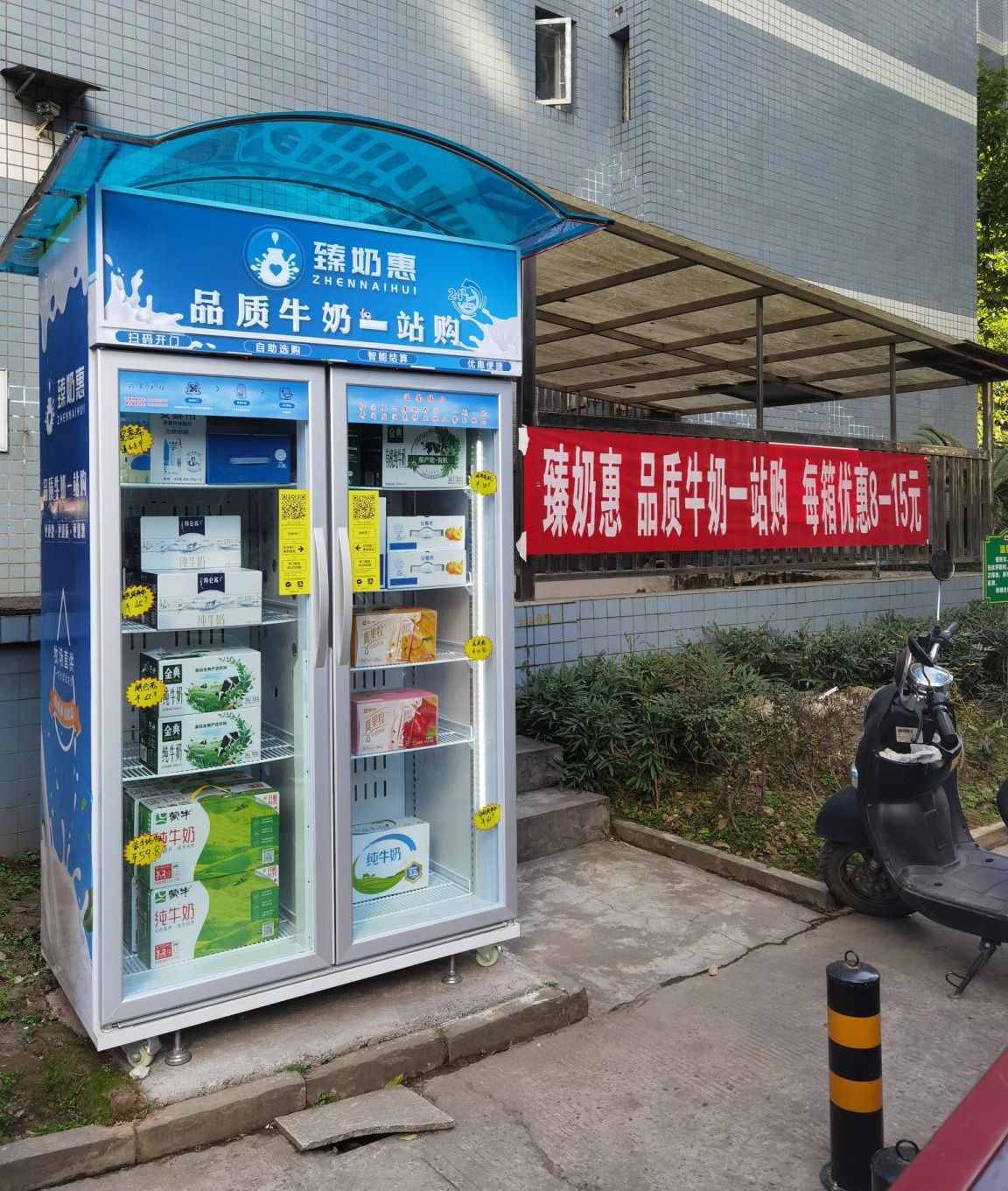
智能售货机:引领便捷生活
智能售货机:引领便捷生活 在这个科技迅速进步的时代,便捷已成为生活的必需。智能售货机作为技术与便利完美结合的产物,正逐渐改变我们的购物方式,为都市生活增添新的活力。 智能售货机的主要优势是它的极致便利性。不论是在地铁…...

正向代理和反向代理
正向代理和反向代理是网络中常见的两种代理方式,它们在网络通信中扮演着不同的角色。 正向代理: 正向代理是代理服务器位于客户端和目标服务器之间的一种代理方式。 客户端向代理服务器发送请求,然后代理服务器将请求转发给目标服务器&…...
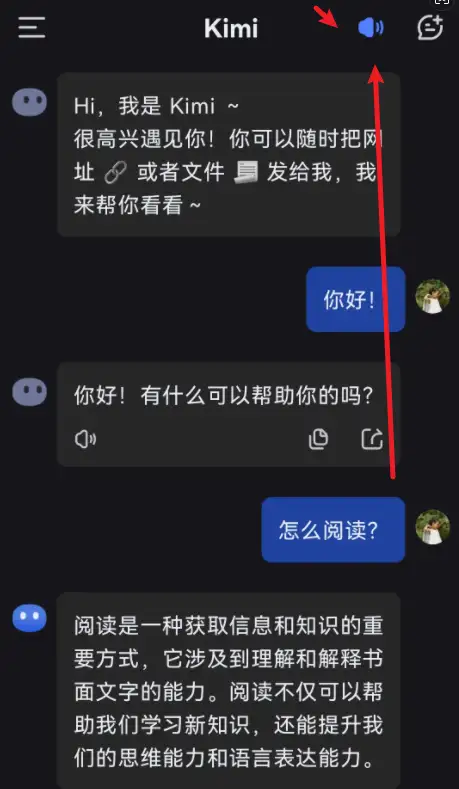
kimichat使用技巧:用语音对话聊天
kimichat之前是只能用文字聊天的,不过最近推出了语音新功能,也可以用语音畅快的对话聊天了。 这个功能目前支持手机app版本,所以首先要在手机上下载安装kimi智能助手。已经安装的,要点击检查更新,更新到最新的版本。 …...
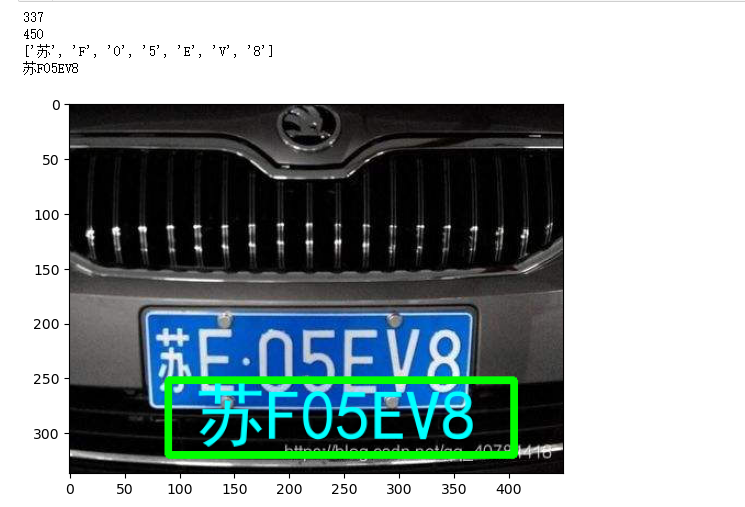
机器学习-09-图像处理02-PIL+numpy+OpenCV实践
总结 本系列是机器学习课程的系列课程,主要介绍机器学习中图像处理技术。 参考 【人工智能】PythonOpenCV图像处理(一篇全) 一文讲解方向梯度直方图(hog) 【杂谈】计算机视觉在人脸图像领域的十几个大的应用方向&…...
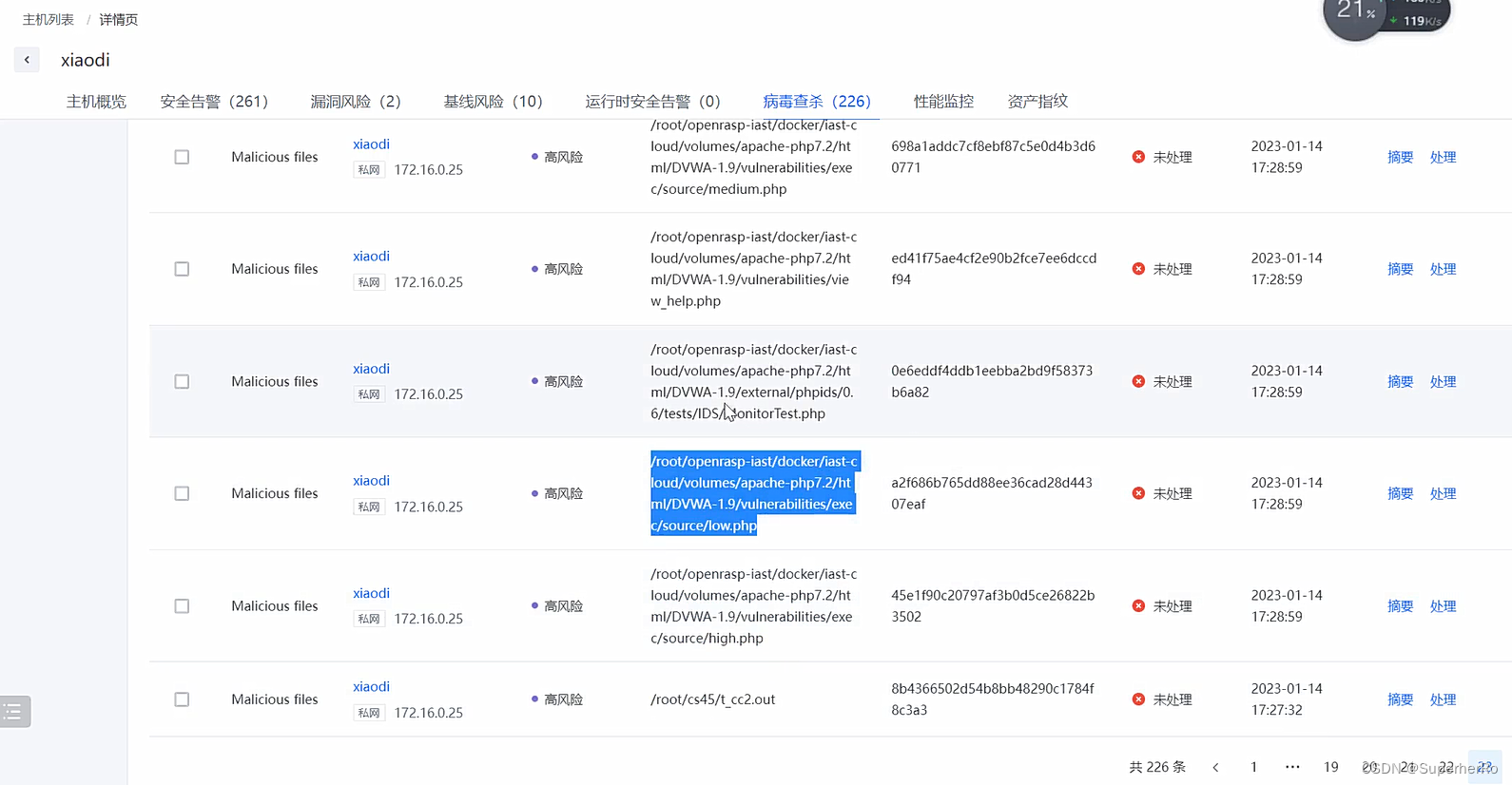
应急响应-战前反制主机HIDSElkeid蜜罐系统HFish
知识点 战前-反制-平台部署其他更多项目: https://github.com/birdhan/SecurityProduct HIDS:主机入侵检测系统,通常会有一个服务器承担服务端角色,其他主机就是客户端角色,客户端加入到服务端的检测范围里ÿ…...

C#:24小时制和12小时制之间的转换
任务描述 本关任务:编写一个程序,利用求余运算完成24小时制和12小时制之间的转换。 注意:要求输入的数字是0到24之间的整数。 测试说明 平台会对你编写的代码进行测试: 测试输入:4 预期输出: 现在是上午4…...
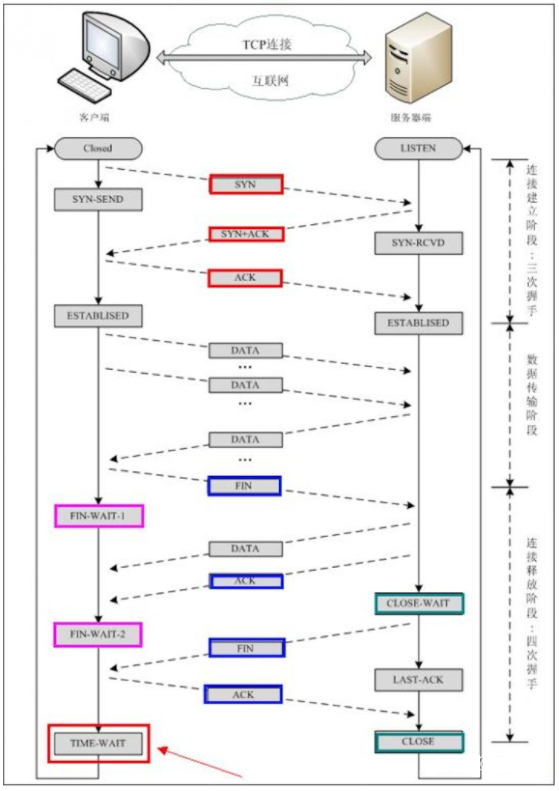
说说TCP为什么需要三次握手和四次挥手?
文章目录 一、三次握手为什么不是两次握手? 二、四次挥手四次挥手原因 三、总结参考文献 一、三次握手 三次握手(Three-way Handshake)其实就是指建立一个TCP连接时,需要客户端和服务器总共发送3个包 主要作用就是为了确认双方的接收能力和…...
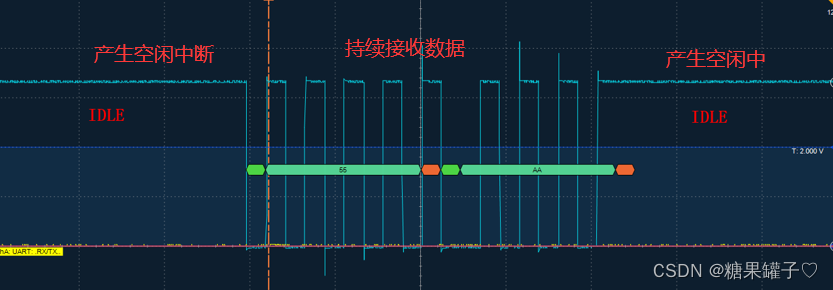
STM32 串口接收定长,不定长数据
本文为大家介绍如何使用 串口 接收定长 和 不定长 的数据。 文章目录 前言一、串口接收定长数据1. 函数介绍2.代码实现 二、串口接收不定长数据1.函数介绍2. 代码实现 三,两者回调函数的区别比较四,空闲中断的介绍总结 前言 一、串口接收定长数据 1. 函…...
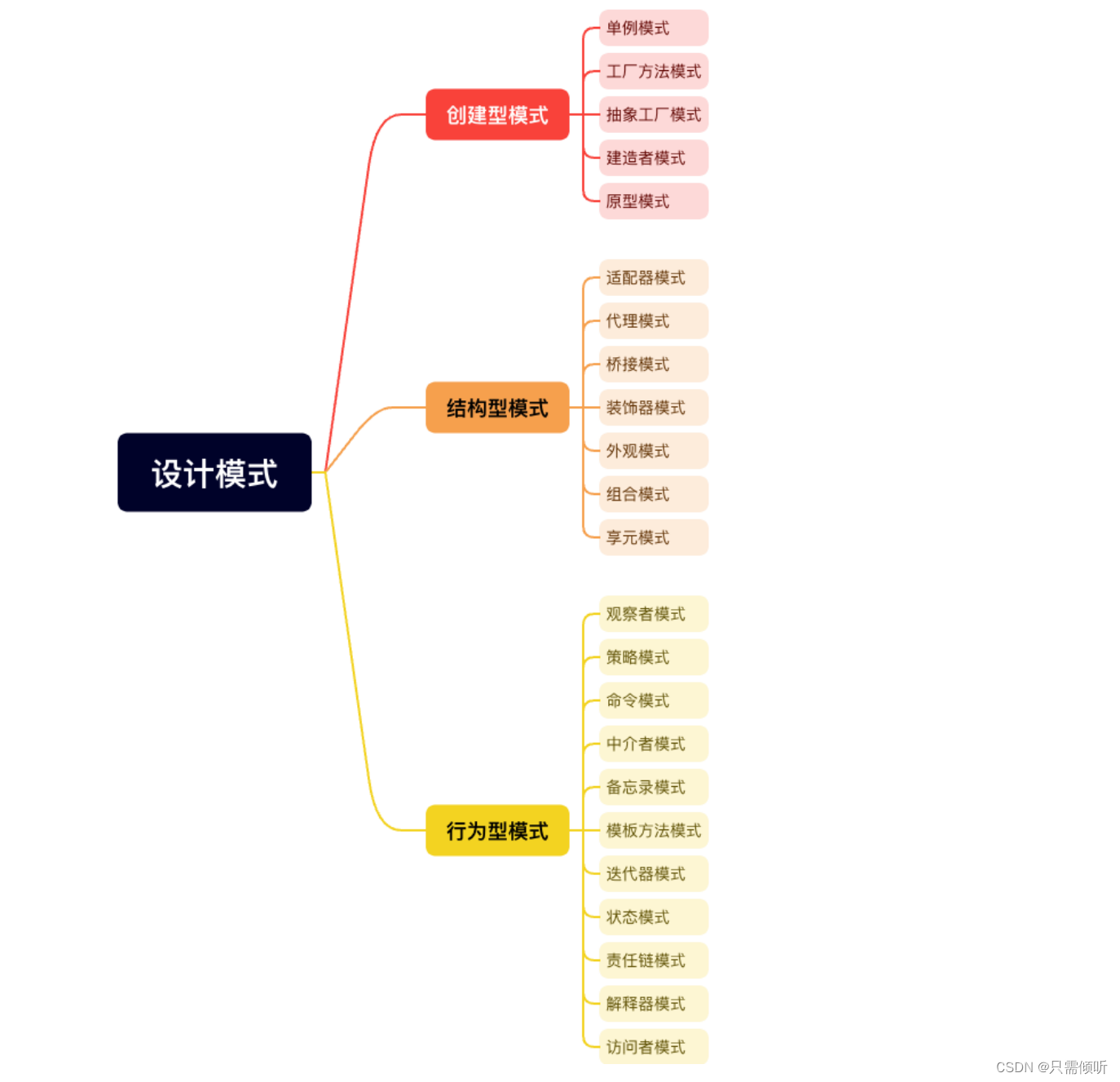
C++设计模式|0.前言
1.什么是设计模式? 简答来说,设计模式就是一套好用的代码经验总结,也就是怎么写好代码的方法论。使用设计模式是为了可重用代码、让代码更容易被他人理解、提高代码的可靠性。 2.设计模式的分类 设计模式可以分为三类:创建型、…...
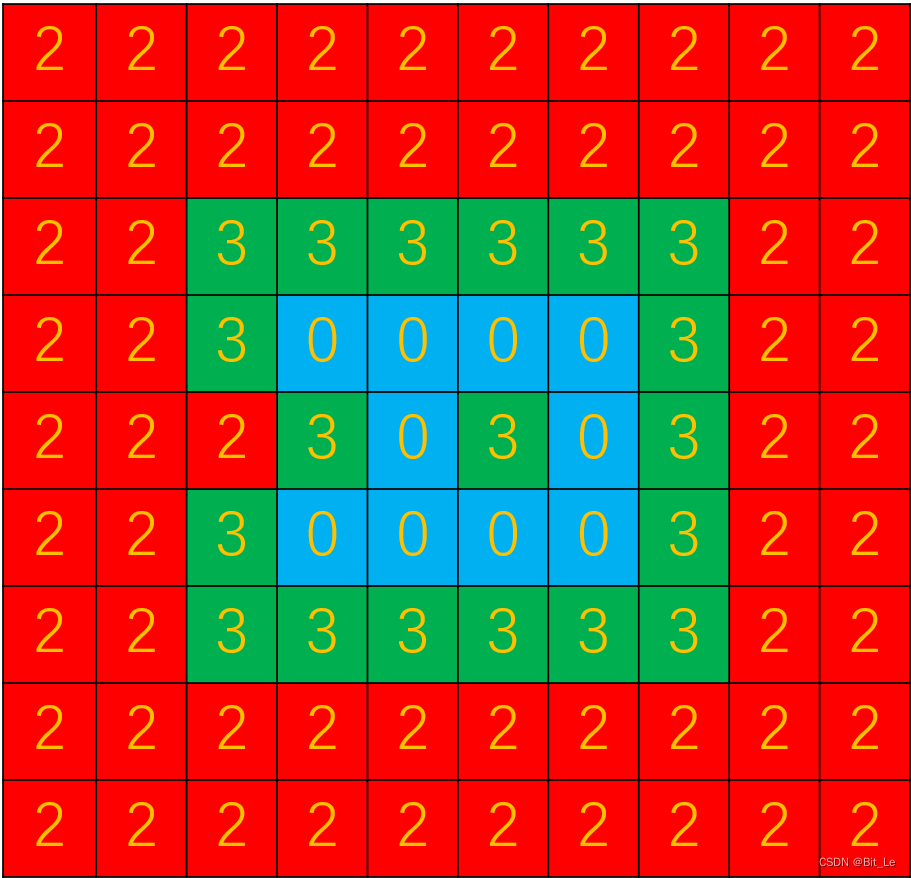
[蓝桥杯] 岛屿个数(C语言)
提示: 橙色字体为需要注意部分,红色字体为难点部分,会在文章“重难点解答”部分精讲。 题目链接 蓝桥杯2023年第十四届省赛真题-岛屿个数 - C语言网 题目理解 这道题让我们求岛屿个数,那么我们就应该先弄懂,对于一…...

Apache Storm的详细配置
Apache Storm的详细配置主要涉及以下几个方面: Zookeeper配置:Apache Storm使用Zookeeper来进行协调和配置管理。你需要配置Zookeeper集群的连接信息,包括Zookeeper服务器的主机和端口。 Storm Nimbus配置:Nimbus是Storm的主节点,负责分配任务给各个工作节点。你需要配置N…...

kylin v10 php源码安装后配置nginx
银河麒麟V10 源码编译安装php7.4 下载地址 https://www.php.net/distributions/php-7.4.33.tar.xz 安装依赖包,准备编译 dnf install libxml2-devel sqlite-devel bzip2-devel libcurl-devel libjpeg-turbo-devel freetype-devel openldap-devel libtool-devel p…...

【01背包】滚动数组优化实现一维01背包DP(对比朴素写法)
01背包 代码 背包问题的滚动数组优化版本建议在完全弄懂了普通的二维01背包问题后再进行食用,不然会出现消化不良的症状… 我们可以将背包问题中DP数组的下标看作成两个集合 下面对比两种不同实现方法的区别: 朴素二维DP版本 使用dp[不超过i的物品集合]…...
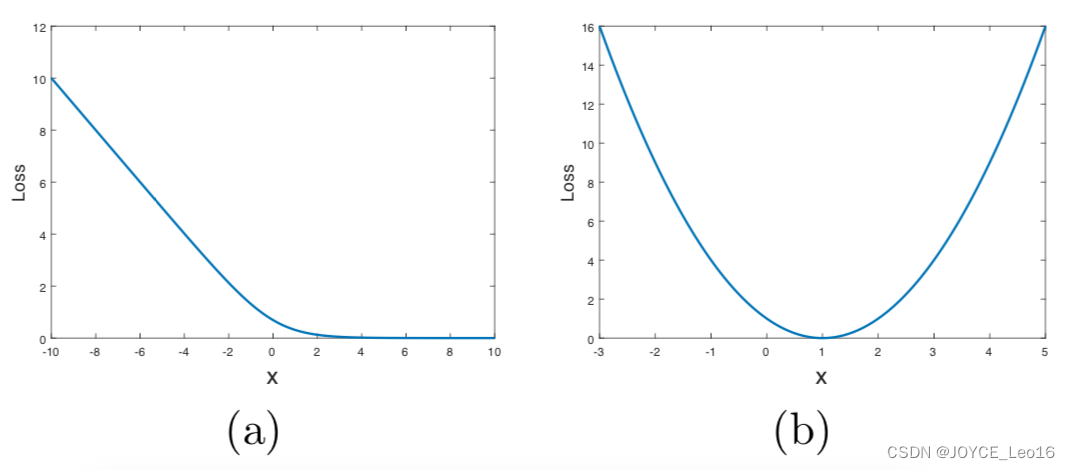
深度学习500问——Chapter07:生成对抗网络(GAN)(2)
文章目录 7.2 GAN的生成能力评价 7.2.1 如何客观评价GAN的生成能力 7.2.2 Inception Score 7.2.3 Mode Score 7.2.5 Wasserstein distance 7.2.6 Frchet Inception Distance (FID) 7.2.7 1-Nearest Neighbor classifier 7.2.8 其他评价方法 7.3 其他常见的生成式模型有哪些 7.…...

A13 STM32_HAL库函数 之 HAL-ETH通用驱动 -- B -- 所有函数的介绍及使用
A13 STM32_HAL库函数 之 HAL-ETH通用驱动 -- B -- 所有函数的介绍及使用 1 通用定时器(TIM)预览1.11 HAL_ETH_TxCpltCallback1.12 HAL_ETH_RxCpltCallback1.13 HAL_ETH_ErrorCallback1.14 HAL_ETH_ReadPHYRegister1.15 HAL_ETH_WritePHYRegister1.16 HAL…...
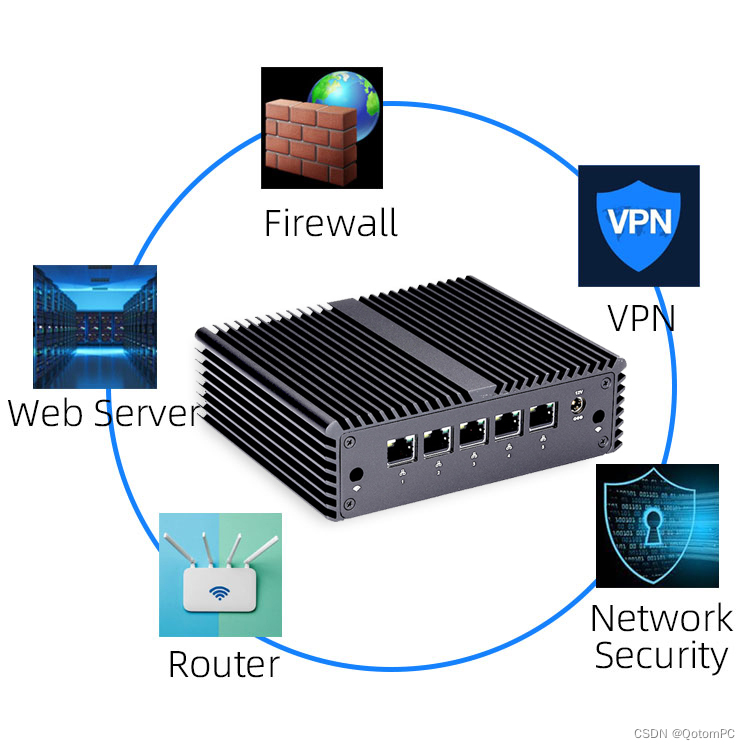
Qotom Q720G5英特尔赛扬处理器N4000高性价比无风扇迷你电脑5网口软路由防火墙
在数字时代,迷你电脑已经成为高效、灵活的解决方案,无论是个人用户还是企业用户,都能从中受益。Qotom Q720G5 无风扇迷你电脑就是这样一款强大的选择,它不仅可以作为软路由、防火墙和路由器,还有着更多的潜力等待发掘。…...

如何了解数字化和信息化的区别?
在数字化浪潮席卷全球的今天,企业如何乘风破浪、实现转型升级? 数字化和信息化,这两个看似相似却各有千秋的概念,一直被人们拿来对比。 下面就来讲一讲我的理解: 从简单了说: “信息化”可以理解为传统数…...
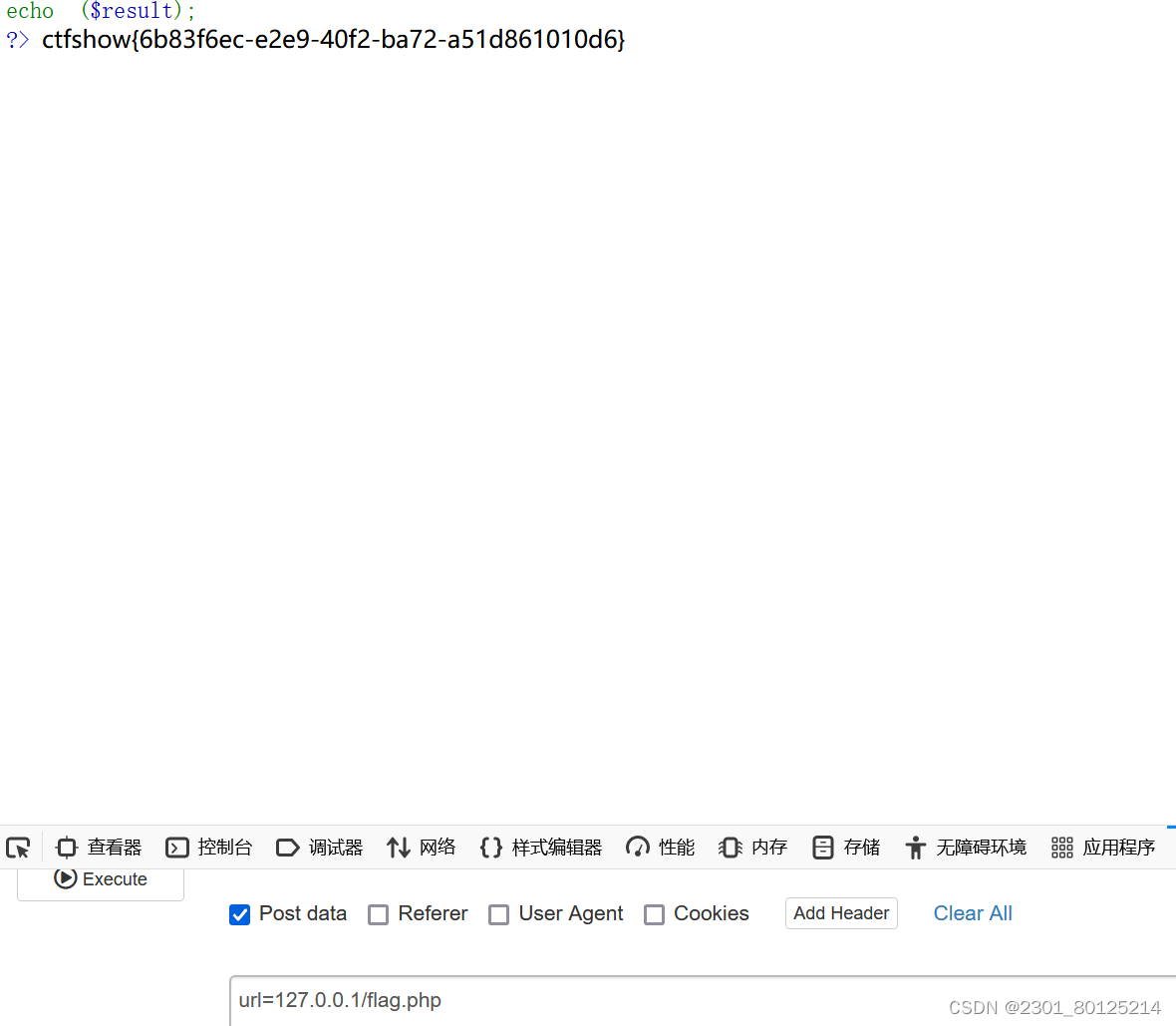
CTF-SHOW SSRF
web351 存在一个flag.php页面,访问会返回不是本地用户的消息,那肯定是要让我们以本地用户去访问127.0.0.1/flag.php web352 代码中先判断是否为HTTP或https协议,之后判断我们传入的url中是否含有localhost和127.0.0,如果没有则…...
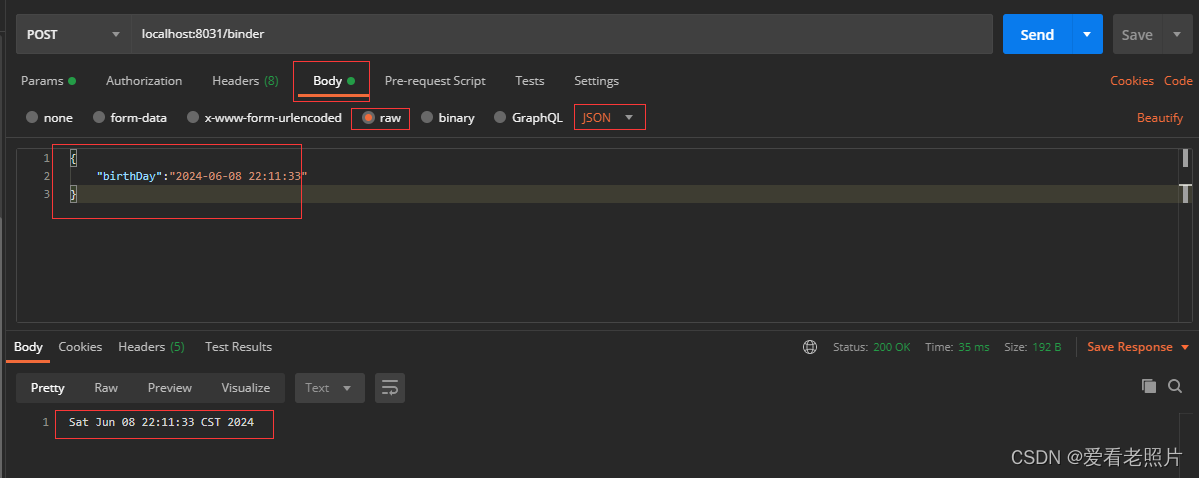
客户端传日期格式字段(String),服务端接口使用java.util.Date类型接收报错问题
客户端传日期格式字段(string),服务端接口使用java.util.Date类型接收报错问题 问题演示第1种:客户端以URL拼接的方式传值第2种:客户端以body中的form-data方式提交第3种 客户端以Body中的json方式提交 问题解决(全局解…...

【Python面试题收录】什么是堆?什么是栈?栈和堆的区别是什么?
一、堆和栈的定义 (1)堆(Heap) 数据结构:堆是一种特殊的完全二叉树,满足父节点的值总是大于或等于(大根堆)其子节点的值。也可以是总是小于或等于(小根堆)其…...

5-云原生监控体系-Grafana-使用配置文件实现自动化导入Dashboard
文章目录 1. 介绍(此文档使用的版本 grafana-enterprise-10.0.3-1)2. 清空之前的实验数据3. 使用配置文件方式配置 Datasource3.1. 创建配置文件3.2. 重启服务并检查是否生效4. 使用配置文件方式配置 Dashboard4.1. 创建配置文件5. 配置 Dashboard JSON 文件5.1. 下载 JSON 文…...
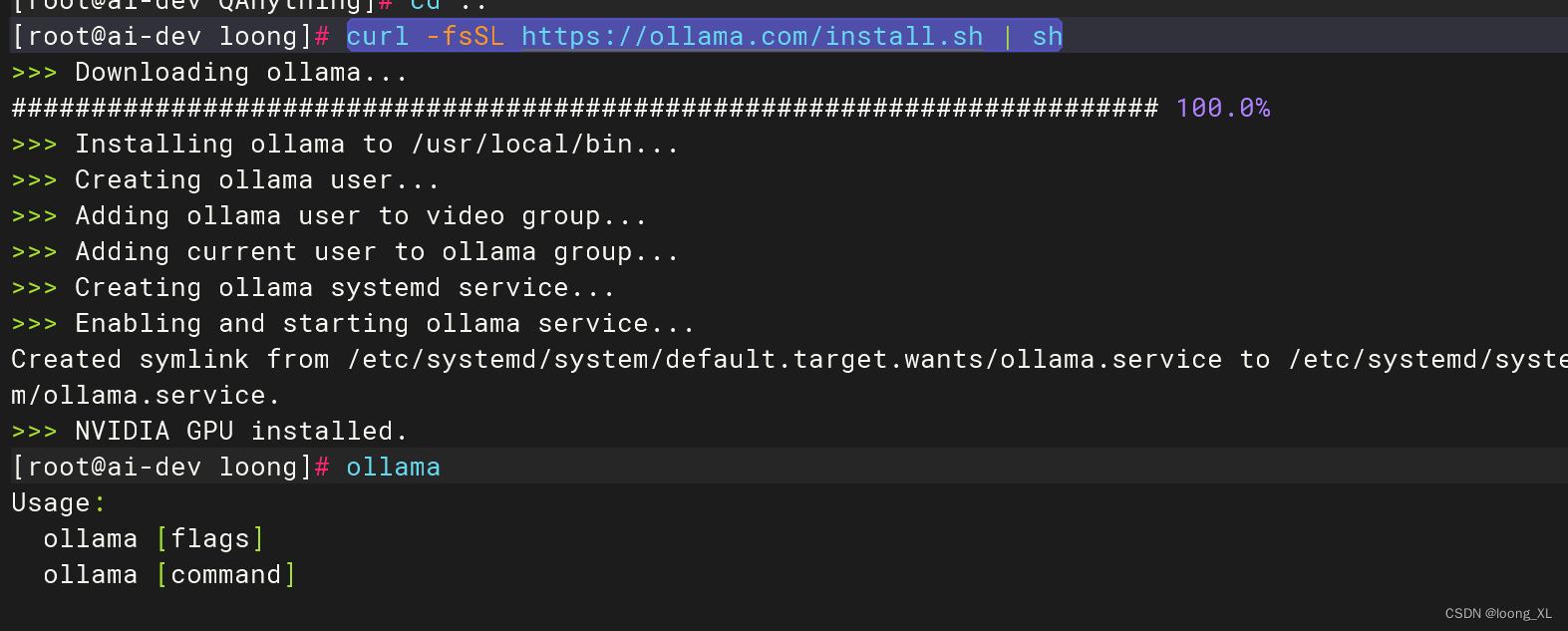
Ollama、FastGPT大模型RAG结合使用案例
参考: https://ollama.com/download/linux https://doc.fastai.site/docs/intro/ https://blog.csdn.net/m0_71142057/article/details/136738997 https://doc.fastgpt.run/docs/development/custom-models/m3e/ Ollama作为后端大模型加载运行 FastGPT作为前端页面聊天集成RA…...

夯实智慧新能源数据底座,TiDB Serverless 在 Sandisolar+ 的应用实践
本文介绍了 SandiSolar通过 TiDB Serverless 构建智慧新能源数据底座的思路与实践。作为一家致力于为全球提供清洁电力解决方案的新能源企业,SandiSolar面临着处理大量实时数据的挑战。为了应对这一问题,SandiSolar选择了 TiDB Serverless 作为他们的数据…...
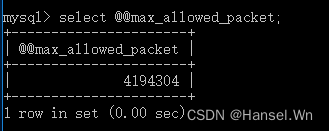
MySQL数据库max_allowed_packet参数
如上图所示的报错,我在提交接口的时候出现了这个错误: MySqlConnector.MySqlException:Error submitting 4MB packet;ensure max_allowed_packet is greater than 4MB.在MySQL数据库中,有一个参数叫max_allowed_packet,这个参数会…...
盐城网站开发厂商/公司网页网站建设
Datawhale干货 作者:[美]霍布森莱恩,科尔霍华德在学习神经网络之前,我们需要对神经网络底层先做一个基本的了解。我们将在本节介绍感知机、反向传播算法以及多种梯度下降法以给大家一个全面的认识。一、感知机数字感知机的本质是从数据集中…...

北京市做网站/企业宣传方式有哪些
高效使用IPython(一种交互式python开发环境) 作者 easyfly 1 利用tab进行代码自动补全 1.1 代码实例 In[1]: ab5 In [2]: a %alias %automagic add2 any %alias_magic a all apply %autocall ab an_apple as %autoindent abs and assert In [2]: a 2. 利…...

做网站都要用到框架吗/微信管理软件
本文系投稿,作者:主题曲。今年2月份,全国“扫黄打非”办公室曾召集百度、阿里巴巴、腾讯、新浪网、微博、今日头条、金山、奇虎、YY直播、映客直播、快手等16家互联网公司有关负责人,强调各互联网企业要健全内容审核、有害信息拦截…...
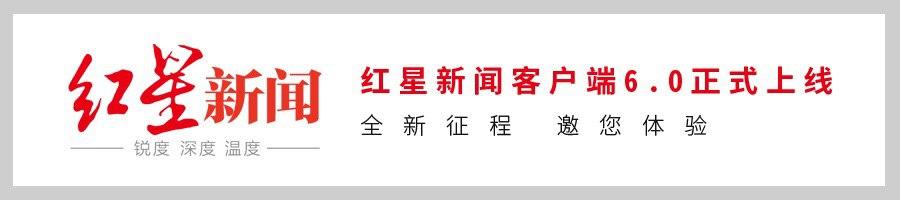
网站空间租用费用/广告投放公司
6月5日下午,中国国际广播电台(以下简称“国广”)俄东中心和成都电视台经济资讯频道联合摄制的纪录片《针传》在成都举行首映。这是一部讲述中国传统文化的纪录片,该片介绍了中国针灸悠久的历史、发展和传承。成都针灸医师邹群和她的徒弟们也来到首映仪式…...

彩页设计网站/抖音关键词排名优化软件
redis的事务是以命令multi开始,然后执行若干redis读写命令,最后以exec命令结束执行。整个过程中,在还没有执行 exec 命令前的所有 redis 的业务读写命令都没有真正的执行,只是放在了一个队列中,等 exec 命令执行时&…...

网站制作的文章/国内永久免费的云服务器
原标题:TVS管特性曲线、参数说明及应用TVS管的英文名是TRANSIENT VOLTAGE SUPPRESSOR,中文名叫瞬变抑制。它在承受瞬间高能量脉冲时,能在极短的时间内由原来的高阻抗状态变为低阻抗,并把电压箝制到特定的水平,从而有效…...