【大模型】基于Hugging Face调用及微调大模型(1)
文章目录
- 一、前言
- 二、Transformer
- 三、Hugging Face
- 3.1 Hugging Face Dataset
- 3. 2 Hugging Face Tokenizer
- 3.3 Hugging Face Transformer
- 3.4 Hugging Face Accelerate
- 四、基于Hugging Face调用模型
- 4.1 调用示例
- 4.2 调用流程概述
- 4.2.1 Tokenizer
- 4.2.2 模型的加载
- 4.2.3 模型基本逻辑
- 4.2.4 加入输出头
- 参考资料
一、前言
ChatGPT的基本原理以及预训练大语言模型的发展史,我们知道ChatGPT和所有预训练大语言模型的核心是什么?其实就是 Transformer,Hugging Face 的火爆离不开他们开源的这个 Transformers 库。这个开源库里有数万个我们可以直接调用的模型。很多场景下,这个开源模型已经足够我们使用了。接下来我们就从Transformer的架构和具体的案例来介绍Hugging Face Transformer。
二、Transformer
Transformer 是一种用于自然语言处理和其它序列到序列任务的神经网络模型,它是在2017年由Vaswani等人提出来的 ,Transformer的核心模块是通过自注意力机制(Self Attention) 捕捉序列之间的依赖关系。
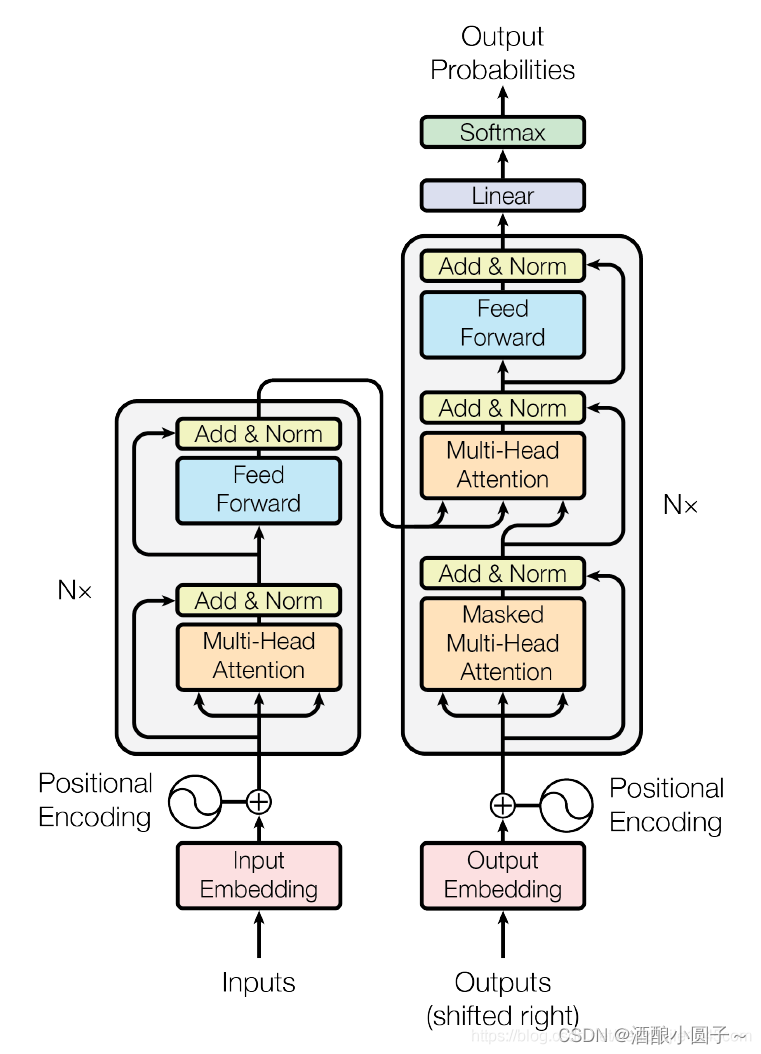
我们在之前的博客中介绍过Transformer,具体参考:Transformer 模型详解
三、Hugging Face
Hugging Face Transformers 是一家公司,在Hugging Face提供的API中,我们几乎可以下载到所有前面提到的预训练大模型的全部信息和各种参数。我们可以认为这些模型在Hugging Face基本就是开源的了,我们只需要拿过来微调或者重新训练这些模型。
用官方的话来说,Hugging Face Transformers 是一个用于自然语言处理的Python库,提供了预训练的语言模型和工具,使得研究者和工程师能够轻松的训练使用共享最先进的NLP模型,其中包括BERT、GPT、RoBERTa、XLNet、DistillBERT等等。
通过 Transformers 可以轻松的用这些预训练模型进行文本分类、命名实体识别、机器翻译、问答系统等NLP任务。这个库还提供了方便的API、示例代码和文档,让我们使用这些模型或者学习模型变得非常简单。
Hugging Face官网:https://huggingface.co/
Hugging Face的主要产品包括Hugging Face Dataset、Hugging Face Tokenizer、Hugging Face Transformer和Hugging Face Accelerate。
-
Hugging Face Dataset:是一个库,用于轻松访问和共享音频、计算机视觉和自然语言处理(NLP)任务的数据集。只需一行代码即可加载数据集,并使用强大的数据处理方法快速准备好数据集,以便在深度学习模型中进行训练。在Apache Arrow格式的支持下,以零拷贝读取处理大型数据集,没有任何内存限制,以实现最佳速度和效率。
-
Hugging Face Tokenizer:是一个用于将文本转换为数字表示形式的库。它支持多种编码器,包括BERT、GPT-2等,并提供了一些高级对齐方法,可以用于映射原始字符串(字符和单词)和标记空间之间的关系。
-
Hugging Face Transformer:是一个用于自然语言处理(NLP)任务的库。它提供了各种预训练模型,包括BERT、GPT-2等,并提供了一些高级功能,例如控制生成文本的长度、温度等。
-
Hugging Face Accelerate:是一个用于加速训练和推理的库。它支持各种硬件加速器,例如GPU、TPU等,并提供了一些高级功能,例如混合精度训练、梯度累积等。
3.1 Hugging Face Dataset
Hugging Face Dataset是一个公共数据集仓库,用于轻松访问和共享音频、计算机视觉和自然语言处理(NLP)任务的数据集。只需一行代码即可加载数据集,并使用强大的数据处理方法快速准备好数据集,以便在深度学习模型中进行训练。
在Apache Arrow格式的支持下,以零拷贝读取处理大型数据集,没有任何内存限制,以实现最佳速度和效率。Hugging Face Dataset还与拥抱面部中心深度集成,使您可以轻松加载数据集并与更广泛的机器学习社区共享数据集。
在花时间下载数据集之前,快速获取有关数据集的一些常规信息通常会很有帮助。数据集的信息存储在 DatasetInfo 中,可以包含数据集描述、要素和数据集大小等信息。
使用 load_dataset_builder() 函数加载数据集构建器并检查数据集的属性,而无需提交下载:
>>> from datasets import load_dataset_builder
>>> ds_builder = load_dataset_builder("rotten_tomatoes")# Inspect dataset description
>>> ds_builder.info.description
Movie Review Dataset. This is a dataset of containing 5,331 positive and 5,331 negative processed sentences from Rotten Tomatoes movie reviews. This data was first used in Bo Pang and Lillian Lee, ``Seeing stars: Exploiting class relationships for sentiment categorization with respect to rating scales.'', Proceedings of the ACL, 2005.# Inspect dataset features
>>> ds_builder.info.features
{'label': ClassLabel(num_classes=2, names=['neg', 'pos'], id=None),'text': Value(dtype='string', id=None)}
如果您对数据集感到满意,请使用 load_dataset() 加载它:
from datasets import load_datasetdataset = load_dataset("rotten_tomatoes", split="train")
3. 2 Hugging Face Tokenizer
Tokenizers 提供了当今最常用的分词器的实现,重点是性能和多功能性。这些分词器也用于Transformers。
Tokenizer 把文本序列输入到模型之前的预处理,相当于数据预处理的环节,因为模型是不可能直接读文字信息的,还是需要经过分词处理,把文本变成一个个token,每个模型比如BERT、GPT需要的Tokenizer都不一样,它们都有自己的字典,因为每一个模型它的训练语料库是不一样的,所以它的token和它的字典大小、token的格式都会各有不同。整体来讲,就是给各种各样的词进行分词,然后编码,以123456来代表词的状态,这个就是Tokenizer的作用。
所以,Tokenizer的任务就是把输入的文本转换成一个一个的标记,它还可以负责对文本序列的清洗、截断、填充进行处理。简而言之,就是为了满足具体模型所要求的格式。
主要特点:
- 使用当今最常用的分词器训练新的词汇表并进行标记化。
- 由于Rust实现,因此非常快速(训练和标记化),在服务器CPU上对1GB文本进行标记化不到20秒。
- 易于使用,但也非常多功能。
- 旨在用于研究和生产。
- 完全对齐跟踪。即使进行破坏性规范化,也始终可以获得与任何令牌对应的原始句子部分。
- 执行所有预处理:截断、填充、添加模型所需的特殊令牌。
这里演示如何使用 BPE 模型实例化一个:classTokenizer
from tokenizers import Tokenizer
from tokenizers.models import BPE
tokenizer = Tokenizer(BPE(unk_token="[UNK]"))
3.3 Hugging Face Transformer
Transformers提供API和工具,可轻松下载和训练最先进的预训练模型。使用预训练模型可以降低计算成本、碳足迹,并节省训练模型所需的时间和资源。这些模型支持不同模态中的常见任务,例如:
- 自然语言处理:文本分类、命名实体识别、问答、语言建模、摘要、翻译、多项选择和文本生成。
- 计算机视觉:图像分类、目标检测和分割。
- 音频:自动语音识别和音频分类。
- 多模式:表格问答、光学字符识别、从扫描文档中提取信息、视频分类和视觉问答。
Transformers支持PyTorch、TensorFlow和JAX之间的框架互操作性。这提供了在模型的每个阶段使用不同框架的灵活性;在一个框架中用三行代码训练一个模型,在另一个框架中加载它进行推理。模型还可以导出到ONNX和TorchScript等格式,以在生产环境中部署。
# 导入必要的库
from transformers import AutoModelForSequenceClassification# 初始化分词器和模型
model_name = "bert-base-cased"
model = AutoModelForSequenceClassification.from_pretrained(model_name, num_labels=2)# 将文本编码为模型期望的张量格式
inputs = tokenizer(dataset["train"]["text"][:10], padding=True, truncation=True, return_tensors="pt")# 将编码后的张量输入模型进行预测
outputs = model(**inputs)# 获取预测结果和标签
predictions = outputs.logits.argmax(dim=-1)
3.4 Hugging Face Accelerate
Accelerate 是一个库,只需添加四行代码,即可在任何分布式配置中运行相同的 PyTorch 代码!简而言之,大规模的训练和推理变得简单、高效和适应性强。
from accelerate import Acceleratoraccelerator = Accelerator()model, optimizer, training_dataloader, scheduler = accelerator.prepare(model, optimizer, training_dataloader, scheduler
)
四、基于Hugging Face调用模型
首先需要安装Hugging Face必要的库:
pip install transformers
4.1 调用示例
首先安装 transformers
依赖包:
pip install transformers
from transformers import pipeline#用人家设计好的流程完成一些简单的任务
classifier = pipeline("sentiment-analysis")
classifier(["I've been waiting for a HuggingFace course my whole life.","I hate this so much!",]
)
这里重点讲讲pipeline,它是hugging face的基本工具,可以理解为一个端到端(end-to-end)的一键调用Transformer模型的工具。它具备了数据预处理、模型处理、模型输出后处理等步骤,可以直接输入原始数据,然后给出预测结果,十分方便,在第三部分调用流程中再详细说明。通过pipeline,可以很方便地调用预训练模型!
- 符合预期的正常结果,输出情感分类的结果:
[{'label': 'POSITIVE', 'score': 0.9598049521446228},{'label': 'NEGATIVE', 'score': 0.9994558691978455}]
- 不符合预期的异常结果,输出报错信息:
OSError: We couldn't connect to 'https://huggingface.co' to load this file, couldn't find it in the cached files and it looks like google/mt5-small is not the path to a directory containing a file named config.json. Checkout your internet connection or see how to run the library in offline mode at 'https://huggingface.co/docs/transformers/installation#offline-mode'.
【报错原因】:Hugging Face模型在国外,国内服务器无法访问到国外的模型,需要将模型下载到本地来加载。
【解决步骤】:
在HuggingFace官方找到对应的model:
可以看到有非常多 sentiment-analysis
相关的模型,这里我们下载 avichr/heBERT_sentiment_analysis
这个model的相关文件:
将下载的文件放到本地"./models/sentiment_analysis" 目录下,并将代码修改为:
from transformers import pipeline
model_path = "./models/sentiment_analysis"
classifier = pipeline("sentiment-analysis", model=model_path) # 通过本地路径加载模型
classifier(["I've been waiting for a HuggingFace course my whole life.","I hate this so much!",]
)
4.2 调用流程概述
首先原始文本用Tokenizer进行分词处理得到输入的文本,然后通过模型进行学习,学习之后进行处理、预测分析。huggingface有个好处,分词器、数据集、模型都封装好了!很方便。
4.2.1 Tokenizer
Tokenizer会做3件事:
- 分词,分字以及特殊字符(起始,终止,间隔,分类等特殊字符可以自己设计的)
- 对每一个token映射得到一个ID(每个词都会对应一个唯一的ID)
- 还有一些辅助信息也可以得到,比如当前词属于哪个句子(还有一些MASK,表示是否是原来的词还是特殊字符等)
Hugging Face中自带AutoTokenizer
工具,可以自动根据模型来判断采用哪个分词器:
from transformers import AutoTokenizer#自动判断checkpoint = "distilbert-base-uncased-finetuned-sst-2-english"#根据这个模型所对应的来加载
tokenizer = AutoTokenizer.from_pretrained(checkpoint)
输入文本:
raw_inputs = ["I've been waiting for a this course my whole life.","I hate this so much!",
]
inputs = tokenizer(raw_inputs, padding=True, truncation=True, return_tensors="pt")
print(inputs)
打印结果(得到两个字典映射,‘input_ids’,一个tensor集合,每个词所对应的ID集合;attention_mask,一个tensor集合,表示是否是原来的词还是特殊字符等):
{'input_ids': tensor([[ 101, 1045, 1005, 2310, 2042, 3403, 2005, 1037, 2023, 2607, 2026, 2878,2166, 1012, 102],[ 101, 1045, 5223, 2023, 2061, 2172, 999, 102, 0, 0, 0, 0,0, 0, 0]]), 'attention_mask': tensor([[1, 1, 1, 1, 1, 1, 1, 1, 1, 1, 1, 1, 1, 1, 1],[1, 1, 1, 1, 1, 1, 1, 1, 0, 0, 0, 0, 0, 0, 0]])}
如果想根据id重新获得原始句子,如下操作:
tokenizer.decode([ 101, 1045, 1005, 2310, 2042, 3403, 2005, 1037, 2023, 2607, 2026, 2878,2166, 1012, 102])
生成的文本会存在特殊字符,这些特殊字符是因为人家模型训练的时候就加入了这个东西,所以这里默认也加入了(google系的处理)
"[CLS] i've been waiting for a this course my whole life. [SEP]"
4.2.2 模型的加载
模型的加载直接指定好名字即可(先不加输出层),这里checkpoint相当于一个文本,只是方便引用,checkpoint在hugging face中也是专门用来保留原来模型,然后再来训练的。
另外AutoModel类也做下说明,AutoModel类及其相关模型类覆盖了非常多模型。它能够根据checkpoint名称分析得到合适的模型架构,并且使用该架构实例化model,方便后续调用。
from transformers import AutoModelcheckpoint = "distilbert-base-uncased-finetuned-sst-2-english"
model = AutoModel.from_pretrained(checkpoint)
model
打印出来模型架构,就是DistilBertModel(蒸馏后的bert模型,模型参数大约只有原来的60%,训练更快,但准确率下降不多)的架构了,能看到embeddings层、transformer层,看得还比较清晰:
DistilBertModel((embeddings): Embeddings((word_embeddings): Embedding(30522, 768, padding_idx=0)(position_embeddings): Embedding(512, 768)(LayerNorm): LayerNorm((768,), eps=1e-12, elementwise_affine=True)(dropout): Dropout(p=0.1, inplace=False))(transformer): Transformer((layer): ModuleList((0): TransformerBlock((attention): MultiHeadSelfAttention((dropout): Dropout(p=0.1, inplace=False)(q_lin): Linear(in_features=768, out_features=768, bias=True)(k_lin): Linear(in_features=768, out_features=768, bias=True)(v_lin): Linear(in_features=768, out_features=768, bias=True)(out_lin): Linear(in_features=768, out_features=768, bias=True))(sa_layer_norm): LayerNorm((768,), eps=1e-12, elementwise_affine=True)(ffn): FFN((dropout): Dropout(p=0.1, inplace=False)(lin1): Linear(in_features=768, out_features=3072, bias=True)(lin2): Linear(in_features=3072, out_features=768, bias=True))(output_layer_norm): LayerNorm((768,), eps=1e-12, elementwise_affine=True))(1): TransformerBlock((attention): MultiHeadSelfAttention((dropout): Dropout(p=0.1, inplace=False)(q_lin): Linear(in_features=768, out_features=768, bias=True)(k_lin): Linear(in_features=768, out_features=768, bias=True)(v_lin): Linear(in_features=768, out_features=768, bias=True)(out_lin): Linear(in_features=768, out_features=768, bias=True))(sa_layer_norm): LayerNorm((768,), eps=1e-12, elementwise_affine=True)(ffn): FFN((dropout): Dropout(p=0.1, inplace=False)(lin1): Linear(in_features=768, out_features=3072, bias=True)(lin2): Linear(in_features=3072, out_features=768, bias=True))(output_layer_norm): LayerNorm((768,), eps=1e-12, elementwise_affine=True))(2): TransformerBlock((attention): MultiHeadSelfAttention((dropout): Dropout(p=0.1, inplace=False)(q_lin): Linear(in_features=768, out_features=768, bias=True)(k_lin): Linear(in_features=768, out_features=768, bias=True)(v_lin): Linear(in_features=768, out_features=768, bias=True)(out_lin): Linear(in_features=768, out_features=768, bias=True))(sa_layer_norm): LayerNorm((768,), eps=1e-12, elementwise_affine=True)(ffn): FFN((dropout): Dropout(p=0.1, inplace=False)(lin1): Linear(in_features=768, out_features=3072, bias=True)(lin2): Linear(in_features=3072, out_features=768, bias=True))(output_layer_norm): LayerNorm((768,), eps=1e-12, elementwise_affine=True))(3): TransformerBlock((attention): MultiHeadSelfAttention((dropout): Dropout(p=0.1, inplace=False)(q_lin): Linear(in_features=768, out_features=768, bias=True)(k_lin): Linear(in_features=768, out_features=768, bias=True)(v_lin): Linear(in_features=768, out_features=768, bias=True)(out_lin): Linear(in_features=768, out_features=768, bias=True))(sa_layer_norm): LayerNorm((768,), eps=1e-12, elementwise_affine=True)(ffn): FFN((dropout): Dropout(p=0.1, inplace=False)(lin1): Linear(in_features=768, out_features=3072, bias=True)(lin2): Linear(in_features=3072, out_features=768, bias=True))(output_layer_norm): LayerNorm((768,), eps=1e-12, elementwise_affine=True))(4): TransformerBlock((attention): MultiHeadSelfAttention((dropout): Dropout(p=0.1, inplace=False)(q_lin): Linear(in_features=768, out_features=768, bias=True)(k_lin): Linear(in_features=768, out_features=768, bias=True)(v_lin): Linear(in_features=768, out_features=768, bias=True)(out_lin): Linear(in_features=768, out_features=768, bias=True))(sa_layer_norm): LayerNorm((768,), eps=1e-12, elementwise_affine=True)(ffn): FFN((dropout): Dropout(p=0.1, inplace=False)(lin1): Linear(in_features=768, out_features=3072, bias=True)(lin2): Linear(in_features=3072, out_features=768, bias=True))(output_layer_norm): LayerNorm((768,), eps=1e-12, elementwise_affine=True))(5): TransformerBlock((attention): MultiHeadSelfAttention((dropout): Dropout(p=0.1, inplace=False)(q_lin): Linear(in_features=768, out_features=768, bias=True)(k_lin): Linear(in_features=768, out_features=768, bias=True)(v_lin): Linear(in_features=768, out_features=768, bias=True)(out_lin): Linear(in_features=768, out_features=768, bias=True))(sa_layer_norm): LayerNorm((768,), eps=1e-12, elementwise_affine=True)(ffn): FFN((dropout): Dropout(p=0.1, inplace=False)(lin1): Linear(in_features=768, out_features=3072, bias=True)(lin2): Linear(in_features=3072, out_features=768, bias=True))(output_layer_norm): LayerNorm((768,), eps=1e-12, elementwise_affine=True))))
)
从里面取一个TransformerBlock进行分析,如下所示,可以看出由 注意力层+标准化层+前馈神经网络(全连接)层+标准化层 组成,可以看到每一层的逻辑,然后由多个TransformerBlock堆叠。哈哈,有这个东东要想改某一层只需要动动手调一调就行了!
TransformerBlock((attention): MultiHeadSelfAttention((dropout): Dropout(p=0.1, inplace=False)(q_lin): Linear(in_features=768, out_features=768, bias=True)(k_lin): Linear(in_features=768, out_features=768, bias=True)(v_lin): Linear(in_features=768, out_features=768, bias=True)(out_lin): Linear(in_features=768, out_features=768, bias=True))(sa_layer_norm): LayerNorm((768,), eps=1e-12, elementwise_affine=True)(ffn): FFN((dropout): Dropout(p=0.1, inplace=False)(lin1): Linear(in_features=768, out_features=3072, bias=True)(lin2): Linear(in_features=3072, out_features=768, bias=True))(output_layer_norm): LayerNorm((768,), eps=1e-12, elementwise_affine=True))
看下输出层的结构,这里**表示分配字典,按照参数顺序依次赋值:
outputs = model(**inputs)
print(outputs.last_hidden_state.shape)
输出:
torch.Size([2, 15, 768])
4.2.3 模型基本逻辑
根据上面代码总结模型的逻辑:input—>词嵌入—>Transformer—>隐藏层—>Head层。
4.2.4 加入输出头
from transformers import AutoModelForSequenceClassificationcheckpoint = "distilbert-base-uncased-finetuned-sst-2-english"
model = AutoModelForSequenceClassification.from_pretrained(checkpoint)
outputs = model(**inputs)
print(outputs.logits.shape)
这里就得到分类后的结果:
torch.Size([2, 2])
再来看看模型的结构:
model
输出:
DistilBertForSequenceClassification((distilbert): DistilBertModel((embeddings): Embeddings((word_embeddings): Embedding(30522, 768, padding_idx=0)(position_embeddings): Embedding(512, 768)(LayerNorm): LayerNorm((768,), eps=1e-12, elementwise_affine=True)(dropout): Dropout(p=0.1, inplace=False))(transformer): Transformer((layer): ModuleList((0): TransformerBlock((attention): MultiHeadSelfAttention((dropout): Dropout(p=0.1, inplace=False)(q_lin): Linear(in_features=768, out_features=768, bias=True)(k_lin): Linear(in_features=768, out_features=768, bias=True)(v_lin): Linear(in_features=768, out_features=768, bias=True)(out_lin): Linear(in_features=768, out_features=768, bias=True))(sa_layer_norm): LayerNorm((768,), eps=1e-12, elementwise_affine=True)(ffn): FFN((dropout): Dropout(p=0.1, inplace=False)(lin1): Linear(in_features=768, out_features=3072, bias=True)(lin2): Linear(in_features=3072, out_features=768, bias=True))(output_layer_norm): LayerNorm((768,), eps=1e-12, elementwise_affine=True))(1): TransformerBlock((attention): MultiHeadSelfAttention((dropout): Dropout(p=0.1, inplace=False)(q_lin): Linear(in_features=768, out_features=768, bias=True)(k_lin): Linear(in_features=768, out_features=768, bias=True)(v_lin): Linear(in_features=768, out_features=768, bias=True)(out_lin): Linear(in_features=768, out_features=768, bias=True))(sa_layer_norm): LayerNorm((768,), eps=1e-12, elementwise_affine=True)(ffn): FFN((dropout): Dropout(p=0.1, inplace=False)(lin1): Linear(in_features=768, out_features=3072, bias=True)(lin2): Linear(in_features=3072, out_features=768, bias=True))(output_layer_norm): LayerNorm((768,), eps=1e-12, elementwise_affine=True))(2): TransformerBlock((attention): MultiHeadSelfAttention((dropout): Dropout(p=0.1, inplace=False)(q_lin): Linear(in_features=768, out_features=768, bias=True)(k_lin): Linear(in_features=768, out_features=768, bias=True)(v_lin): Linear(in_features=768, out_features=768, bias=True)(out_lin): Linear(in_features=768, out_features=768, bias=True))(sa_layer_norm): LayerNorm((768,), eps=1e-12, elementwise_affine=True)(ffn): FFN((dropout): Dropout(p=0.1, inplace=False)(lin1): Linear(in_features=768, out_features=3072, bias=True)(lin2): Linear(in_features=3072, out_features=768, bias=True))(output_layer_norm): LayerNorm((768,), eps=1e-12, elementwise_affine=True))(3): TransformerBlock((attention): MultiHeadSelfAttention((dropout): Dropout(p=0.1, inplace=False)(q_lin): Linear(in_features=768, out_features=768, bias=True)(k_lin): Linear(in_features=768, out_features=768, bias=True)(v_lin): Linear(in_features=768, out_features=768, bias=True)(out_lin): Linear(in_features=768, out_features=768, bias=True))(sa_layer_norm): LayerNorm((768,), eps=1e-12, elementwise_affine=True)(ffn): FFN((dropout): Dropout(p=0.1, inplace=False)(lin1): Linear(in_features=768, out_features=3072, bias=True)(lin2): Linear(in_features=3072, out_features=768, bias=True))(output_layer_norm): LayerNorm((768,), eps=1e-12, elementwise_affine=True))(4): TransformerBlock((attention): MultiHeadSelfAttention((dropout): Dropout(p=0.1, inplace=False)(q_lin): Linear(in_features=768, out_features=768, bias=True)(k_lin): Linear(in_features=768, out_features=768, bias=True)(v_lin): Linear(in_features=768, out_features=768, bias=True)(out_lin): Linear(in_features=768, out_features=768, bias=True))(sa_layer_norm): LayerNorm((768,), eps=1e-12, elementwise_affine=True)(ffn): FFN((dropout): Dropout(p=0.1, inplace=False)(lin1): Linear(in_features=768, out_features=3072, bias=True)(lin2): Linear(in_features=3072, out_features=768, bias=True))(output_layer_norm): LayerNorm((768,), eps=1e-12, elementwise_affine=True))(5): TransformerBlock((attention): MultiHeadSelfAttention((dropout): Dropout(p=0.1, inplace=False)(q_lin): Linear(in_features=768, out_features=768, bias=True)(k_lin): Linear(in_features=768, out_features=768, bias=True)(v_lin): Linear(in_features=768, out_features=768, bias=True)(out_lin): Linear(in_features=768, out_features=768, bias=True))(sa_layer_norm): LayerNorm((768,), eps=1e-12, elementwise_affine=True)(ffn): FFN((dropout): Dropout(p=0.1, inplace=False)(lin1): Linear(in_features=768, out_features=3072, bias=True)(lin2): Linear(in_features=3072, out_features=768, bias=True))(output_layer_norm): LayerNorm((768,), eps=1e-12, elementwise_affine=True)))))(pre_classifier): Linear(in_features=768, out_features=768, bias=True)(classifier): Linear(in_features=768, out_features=2, bias=True)(dropout): Dropout(p=0.2, inplace=False)
)
之后采用softmax进行预测:
import torchpredictions = torch.nn.functional.softmax(outputs.logits, dim=-1)
print(predictions)
输出:
tensor([[1.5446e-02, 9.8455e-01],[9.9946e-01, 5.4418e-04]], grad_fn=<SoftmaxBackward0>)
id2label这个我们后续可以自己设计,标签名字对应都可以自己指定:
model.config.id2label
输出:
{0: 'NEGATIVE', 1: 'POSITIVE'}
参考资料
- Hugging Face Transformer:从原理到实战的全面指南
- Huggingface中Transformer模型使用
相关文章:
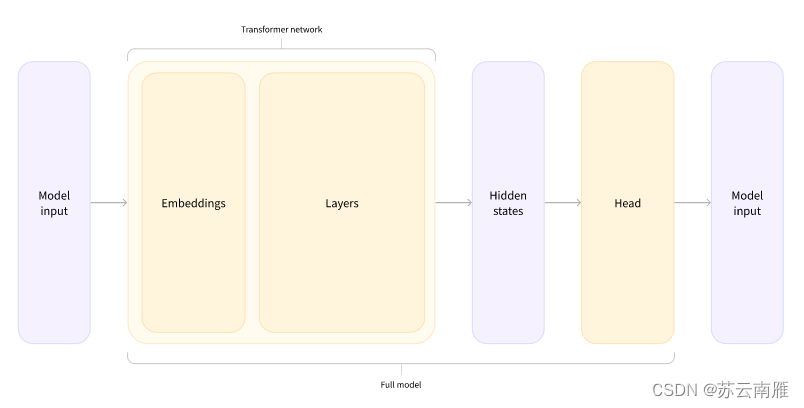
【大模型】基于Hugging Face调用及微调大模型(1)
文章目录 一、前言二、Transformer三、Hugging Face3.1 Hugging Face Dataset3. 2 Hugging Face Tokenizer3.3 Hugging Face Transformer3.4 Hugging Face Accelerate 四、基于Hugging Face调用模型4.1 调用示例4.2 调用流程概述4.2.1 Tokenizer4.2.2 模型的加载4.2.3 模型基本…...
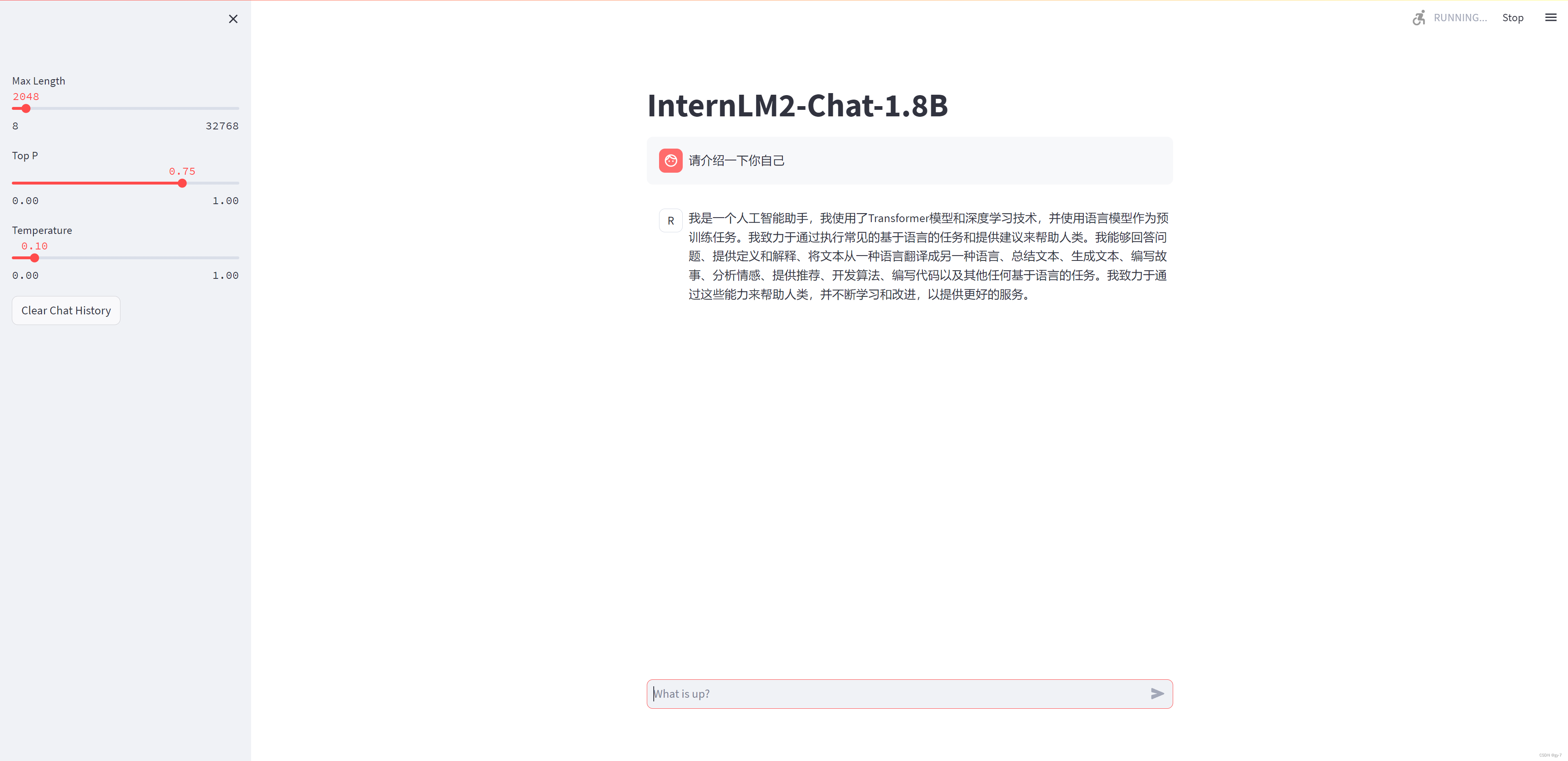
书生·浦语大模型全链路开源体系-笔记作业4
XTuner 微调 LLM:1.8B、多模态、Agent 引自:Tutorial/xtuner/personal_assistant_document.md at camp2 InternLM/Tutorial GitHub 1. XTuner介绍 引自:欢迎来到 XTuner 的中文文档 — XTuner 0.1.18.dev0 文档 1.1. 什么是 XTuner ? X…...
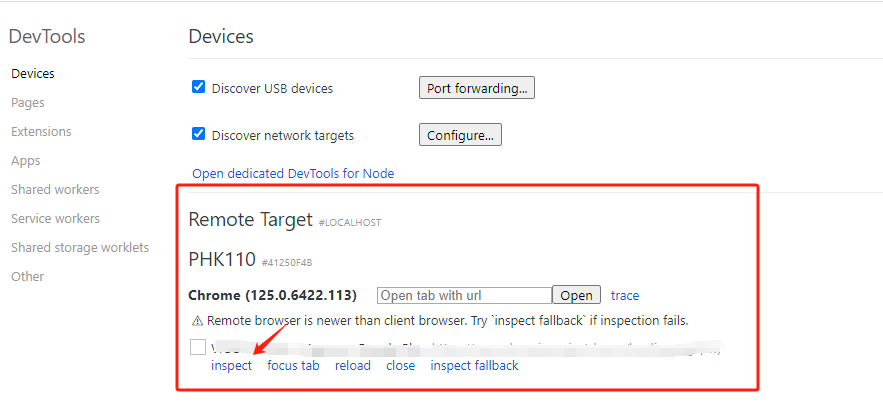
chrome调试手机网页
前期准备 1、 PC端安装好chrmoe浏览器 2、 安卓手机安装好chrmoe浏览器 3、 数据线 原文地址:https://lengmo714.top/343880cb.html 手机打开调试模式 进入手机设置,找到开发者模式,然后启用USB调试 打开PC端chrome调试功能 1、点击chr…...
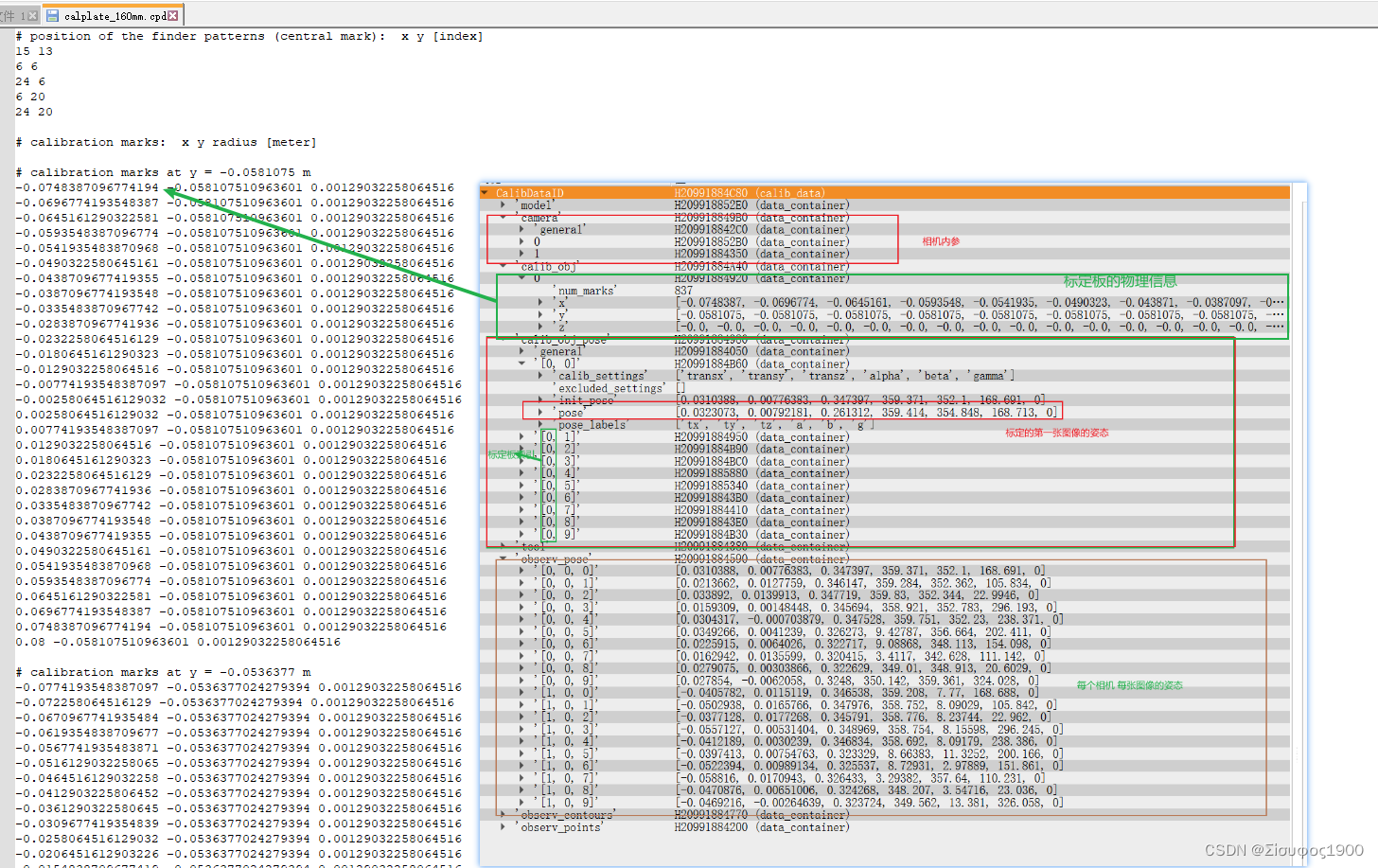
Halcon 双相机标定与拼图(一)
一、概述 最近有一个多相机标定的项目,大概是4个相机来标定,同一坐标系,然后拼接图,之前双相机标定的时候也大概看看,所以今天就找了那个halcon 案例多学一下,后面我打算做一个对位贴合的东西,…...
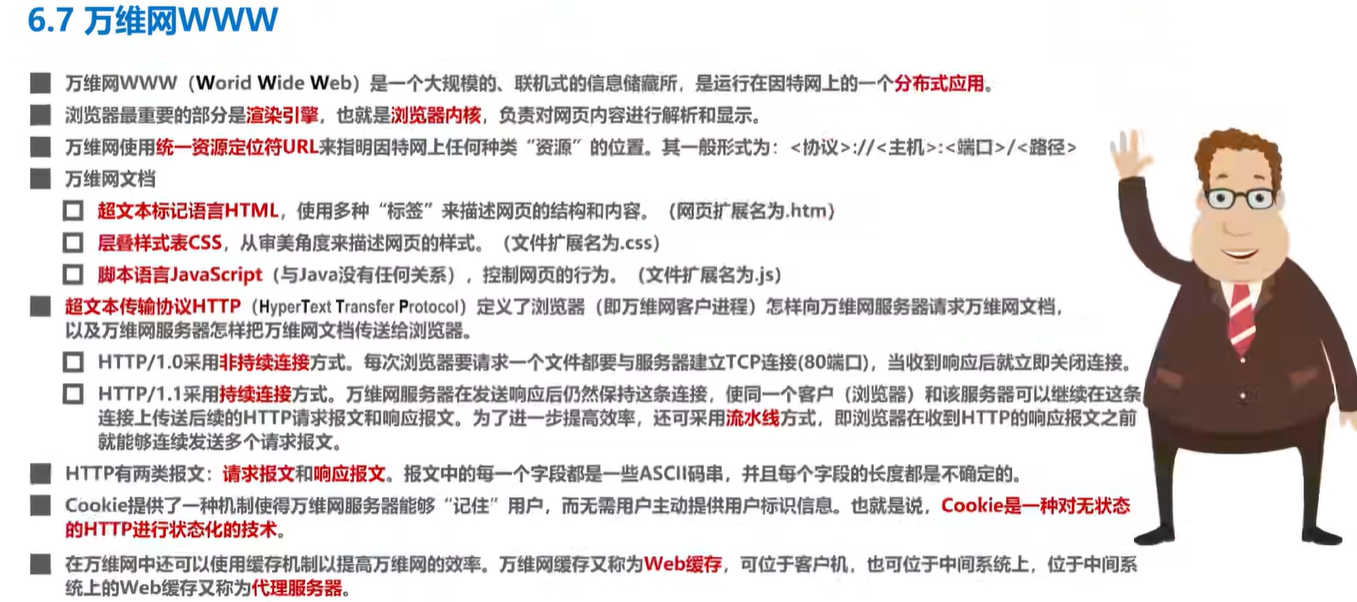
计算机网络学习记录 应用层 Day6
你好,我是Qiuner. 为记录自己编程学习过程和帮助别人少走弯路而写博客 这是我的 github https://github.com/Qiuner ⭐️ gitee https://gitee.com/Qiuner 🌹 如果本篇文章帮到了你 不妨点个赞吧~ 我会很高兴的 😄 (^ ~ ^) 想看更多 那就点个关注吧 我…...
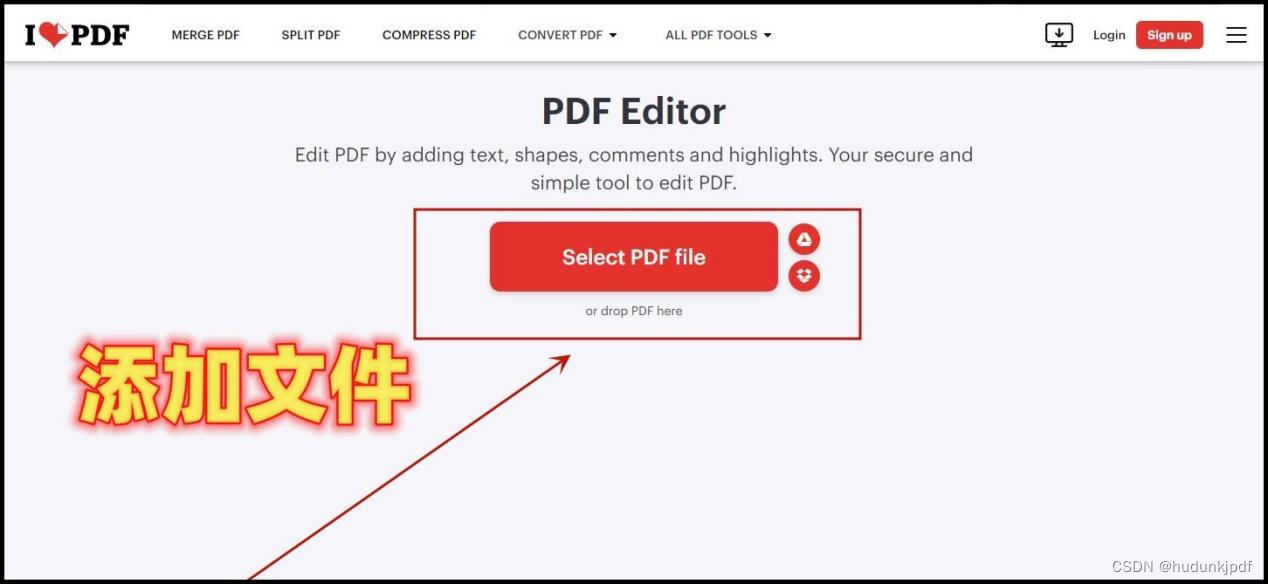
如何编辑pdf文件内容?3种PDF编辑方法分享
如何编辑pdf文件内容?在当今数字化时代,PDF文件因其跨平台兼容性和保持原样不变的特点,在办公、学习、生活等多个领域得到了广泛应用。然而,PDF文件的不可编辑性也让许多用户感到困扰。你是否曾经遇到过需要修改PDF文件内容&#…...

汇总!7种大模型的部署方法!
我们如何在本地部署运行私有的开源大型语言模型(LLMs)呢?本文将向您梳理七种实用的方法及如何选择。 Hugging Face的Transformers 这是一个强大的Python库,专为简化本地运行LLM而设计。其优势在于自动模型下载、提供丰富的代码片段…...

什么是函数?在C语言中如何定义一个函数
函数是编程中用于执行特定任务的一组指令的集合。它有一个名称(即函数名),可以通过该名称在程序中多次调用该函数以执行相同的任务。这有助于提高代码的可重用性和可维护性。 在C语言中,函数的定义通常包括以下几个部分ÿ…...
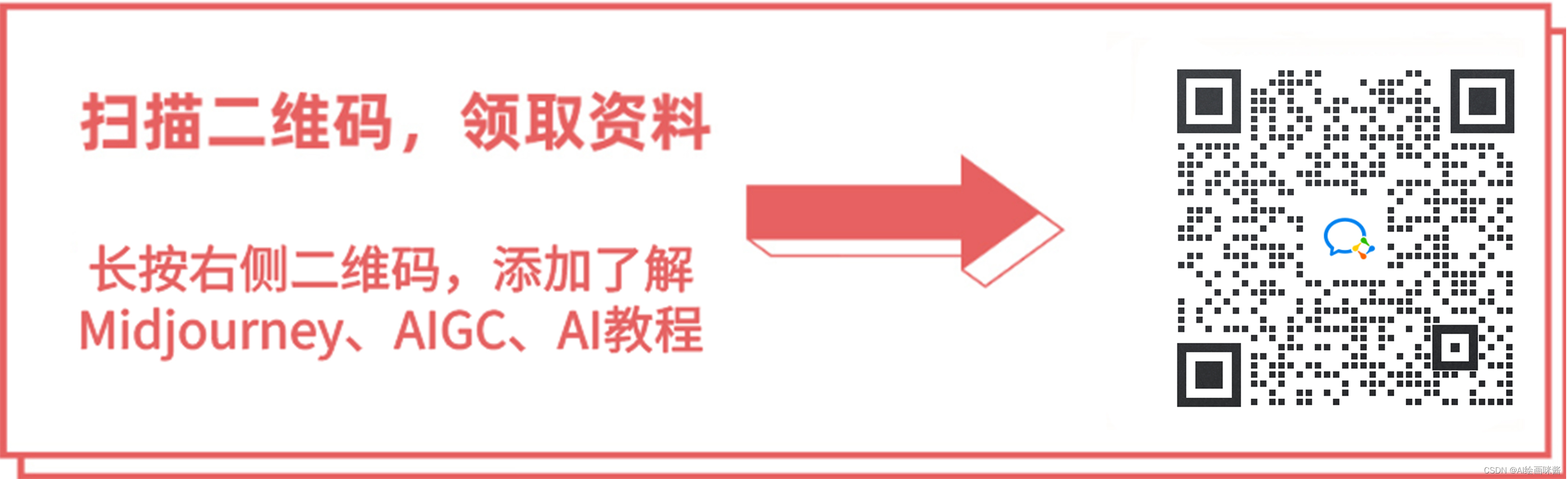
Stable Diffusion——四种模型 LoRA(包括LyCORIS)、Embeddings、Dreambooth、Hypernetwork
目前 Stable diffusion 中用到主要有四种模型,分别是 Textual Inversion (TI)以 Embeddings 为训练结果的模型、Hypernetwork 超网络模型、LoRA(包括 LoRA 的变体 LyCORIS)模型、Dreambooth 模型。 视频博主 koiboi 用…...
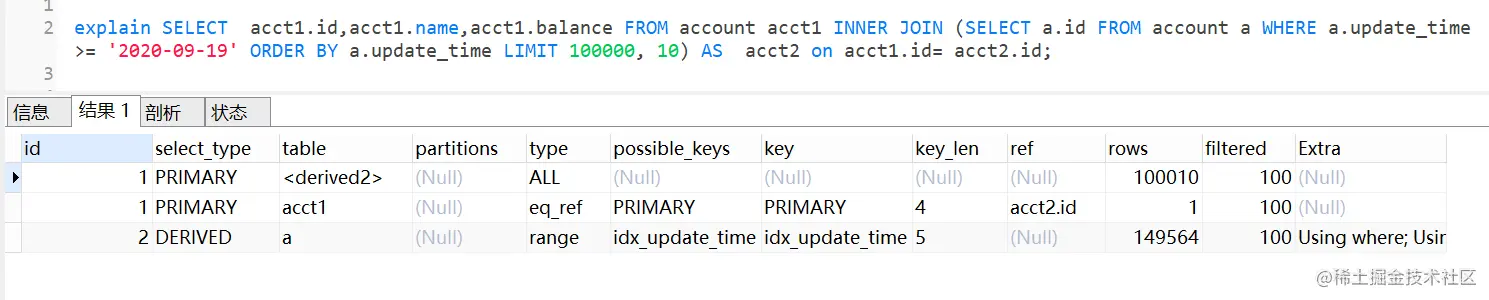
MySQL深分页,limit 100000,10 优化
文章目录 一、limit深分页为什么会变慢二、优化方案2.1 通过子查询优化(覆盖索引)回顾B树结构覆盖索引把条件转移到主键索引树 2.2 INNER JOIN 延迟关联2.3 标签记录法(要求id是有序的)2.4 使用between...and... 我们日常做分页需…...
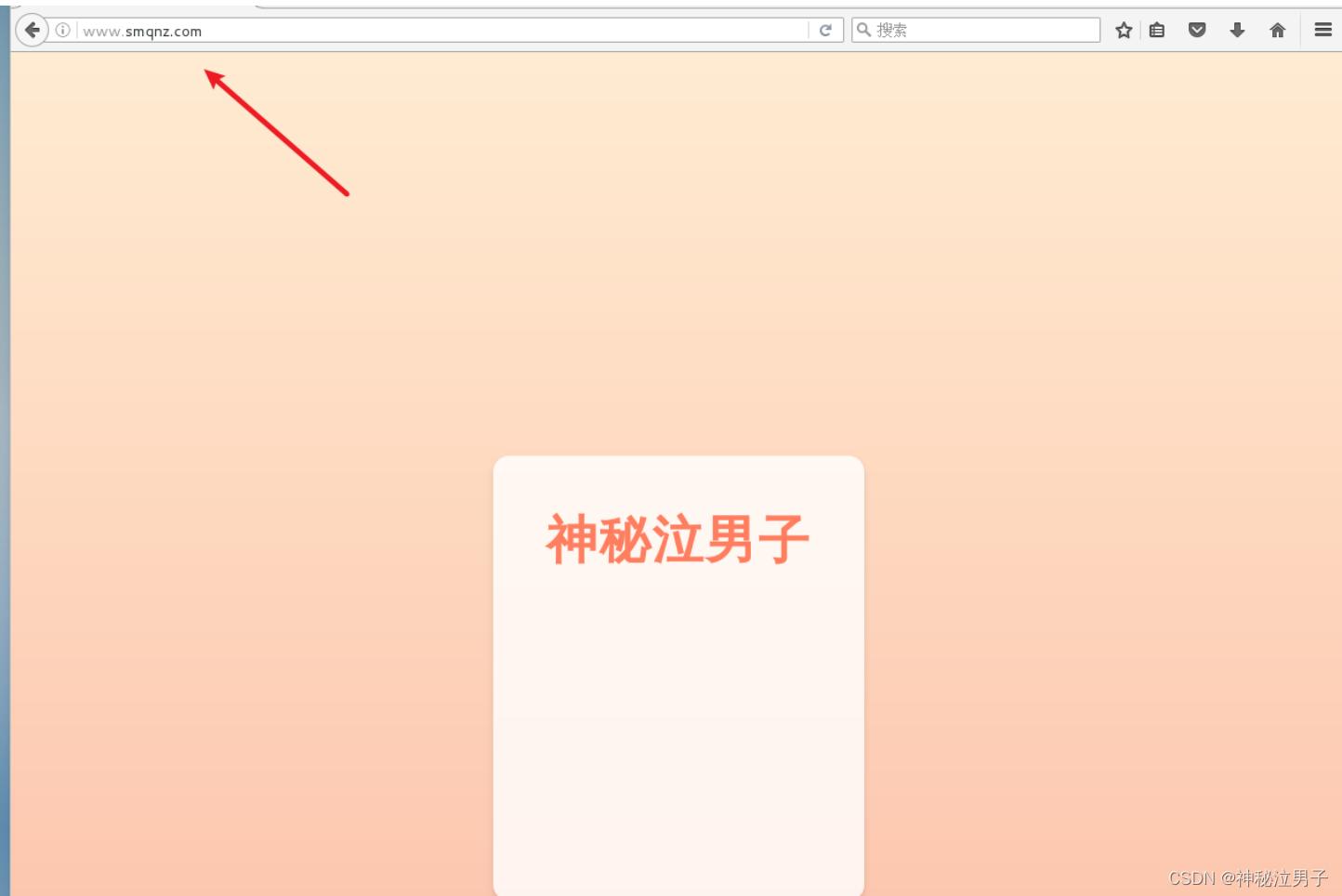
Linux[高级管理]——使用源码包编译安装Apache网站
🏡作者主页:点击! 👨💻Linux高级管理专栏:点击! ⏰️创作时间:2024年5月31日14点20分 🀄️文章质量:96分 在Linux系统上编译和安装Apache HTTP Server是…...
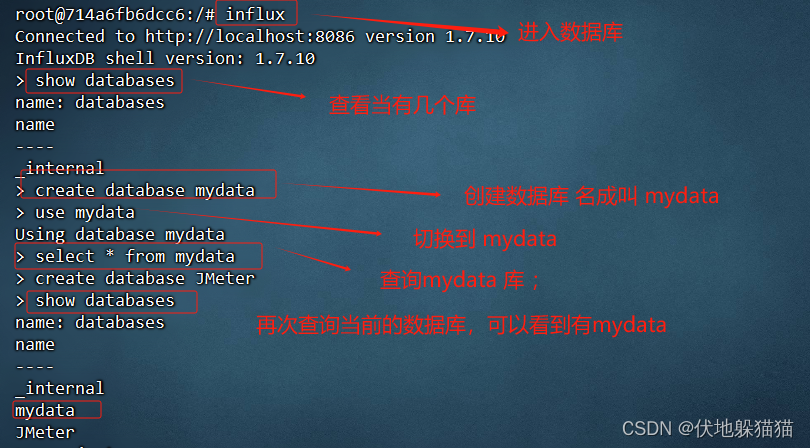
Docker+JMeter+InfluxDB+Grafana 搭建性 能监控平台
JMeter原生报告的缺点: 无法实时共享 报告信息的展示不美观 需求方案 为了解决上述问题,可以通过 InfluxDB Grafana解决 : InfluxDB :是一个开源分布式指标数据库,使用 Go 语言编写,无需外部依赖 应用&am…...
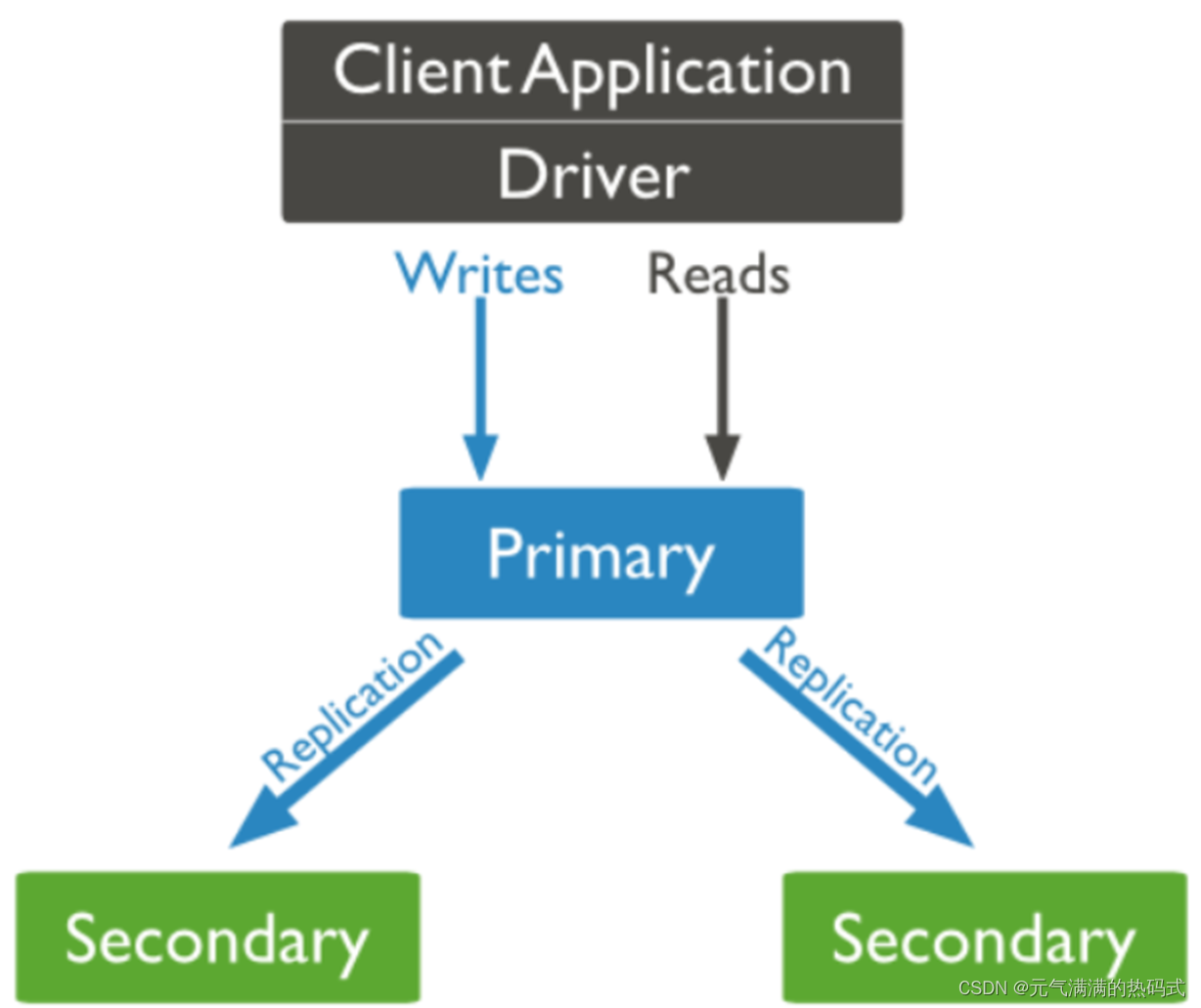
NoSQL实战(MongoDB搭建主从复制)
什么是复制集? MongoDB复制是将数据同步到多个服务器的过程; 复制集提供了数据的冗余备份并提高了数据的可用性,通常可以保证数据的安全性; 复制集还允许您从硬件故障和服务中断中恢复数据。 保障数据的安全性 数据高可用性 (2…...

【讯为Linux驱动开发】3.内核空间和用户空间
【问】内存空间的组成部分?? 内存空间分为内核空间和用户空间 1.内核空间控制硬件资源,提供系统调用接口,保护系统自身安全稳定 2.用户空间实现业务逻辑 【问】如何进入内核空间使用硬件资源? 1.系统调用 2.软中断 3.…...
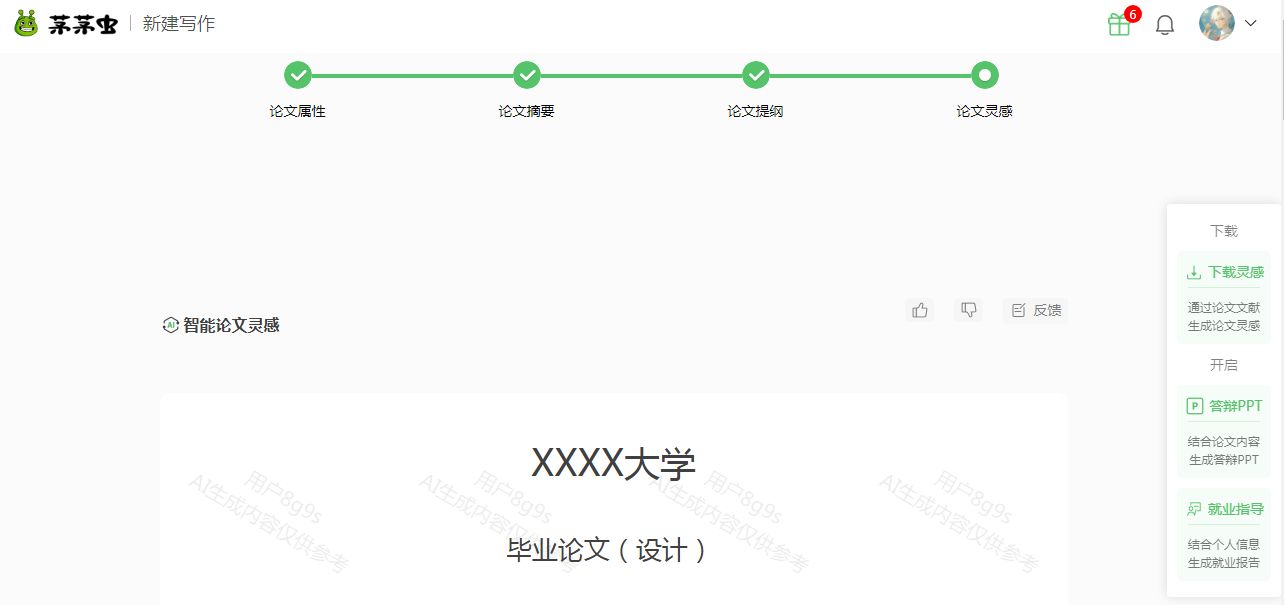
AI论文:一键生成论文的高效工具
说到这个问题,那真的得看你对“靠谱”的定义是怎样的啦? 众所周知,写论文是一项极其耗时间的事情,从开始的选题到文献资料搜索查阅,大纲整理等等一大堆的繁杂工作是极艰辛的。用AI写论文就不一样了,自动化…...

申请医疗设备注册变更时,需要补充考虑网络安全的情况有哪些?
在申请医疗器械设备注册变更时,需要补充网络安全的情况主要包括以下几点: 网络安全功能更新:如果医疗器械的自研软件发生网络安全功能更新,或者合并网络安全补丁更新的情形,需要单独提交一份自研软件网络安全功能更新…...

打对钩的方式做人机验证(vue+javascript)
要实现一个通过打对钩方式的人机验证,并且让它不容易被破解,可以考虑以下几点: 动态生成选项和题目:每次生成的验证选项和题目都不一样,防止简单的脚本通过固定的答案绕过验证。使用图像和文字混合验证:增…...

可视化脚本用于使用MMDetection库进行图像的目标检测
# Copyright (c) OpenMMLab. All rights reserved. import asyncio from argparse import ArgumentParserfrom mmdet.apis import (async_inference_detector, inference_detector,init_detector, show_result_pyplot) import denseclip# 解析命令行参数 def parse_args():pars…...
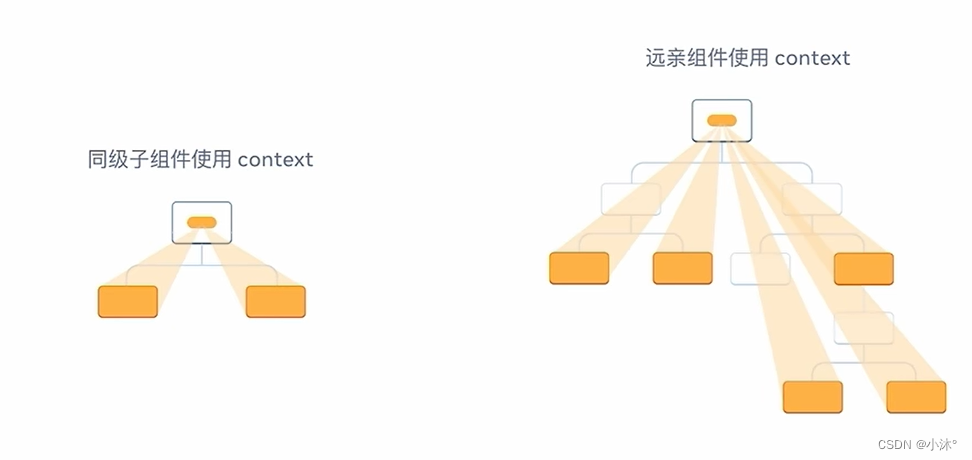
React-组件通信
组件通信 概念:组件通信就是组件之间的数据传递,根据组件嵌套关系的不同,有不同的通信方法 父传子 基础实现 实现步骤: 1.父组件传递数据-在子组件标签上绑定属性 2.子组件接收数据-子组件通过props参数接收数据 props说明 1.…...
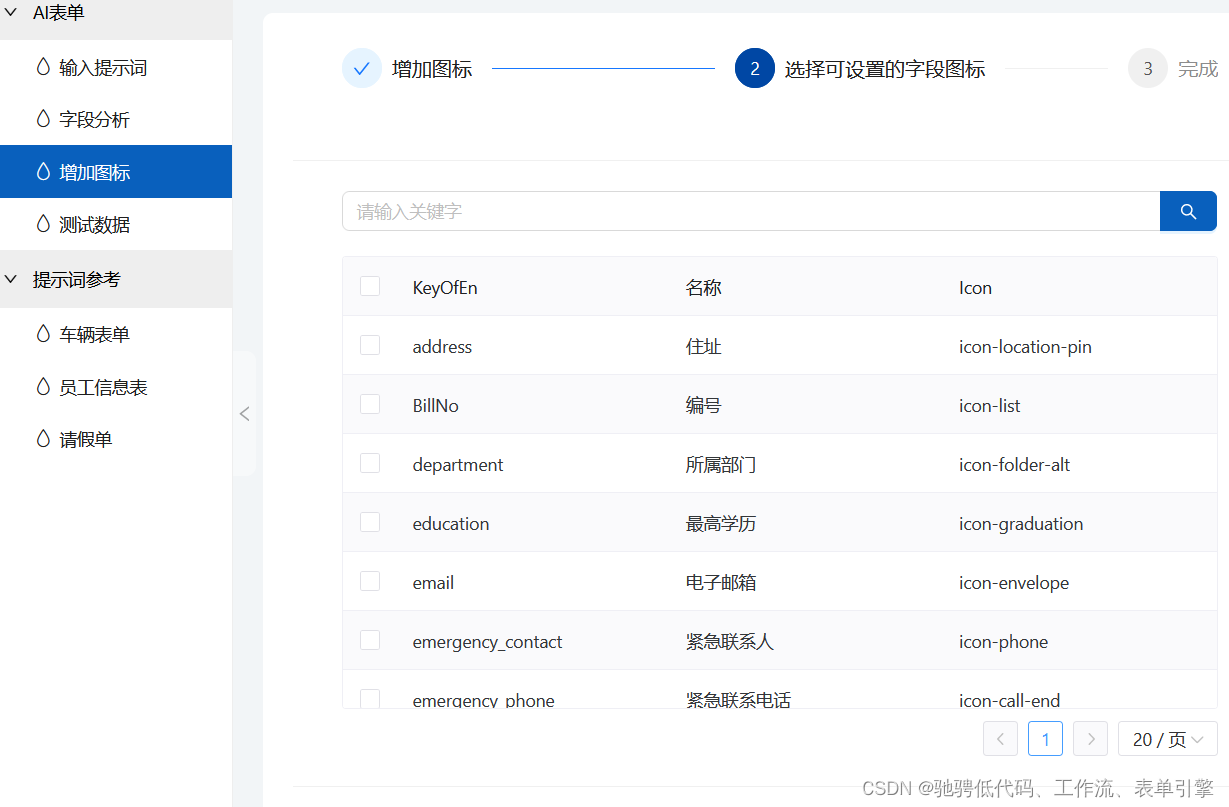
低代码选型要注意什么问题?
低代码选型时,确实需要从多个角度综合考虑,以下是根据您给出的角度进行的分析和建议: 公司的人才资源: 评估团队中是否有具备编程能力的开发人员,以确保能够充分利用低代码平台的高级功能和进行必要的定制开发。考察实…...
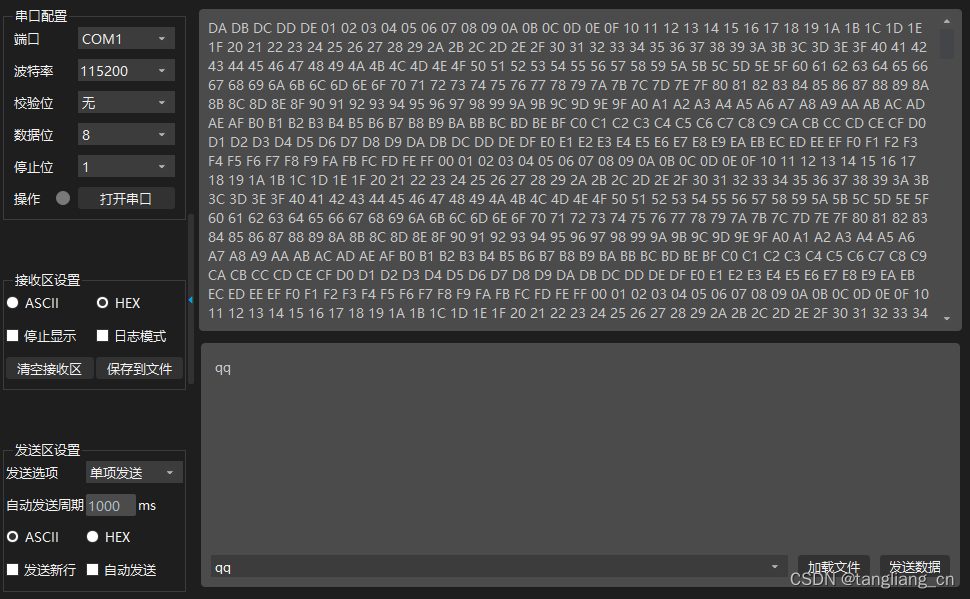
fpga入门 串口定时1秒发送1字节
一、 程序说明 FPGA通过串口定时发送数据,每秒发送1字节,数据不断自增 参考小梅哥教程 二、 uart_tx.v timescale 1ns / 1psmodule uart_tx(input wire sclk,input wire rst_n,output reg uart_tx);parameter …...

总结一下自己,最近三年,我做了哪些工作
简单总结下吧,我算是业务架构师,确实对得起这个名字,经常冲在一线,业务和架构相关的东西都有做,系统比较复杂,不过逐步了解谁都会熟悉的 下面简单列一列我这三年的工作情况吧,也算是给自己一个交…...

SpringCloud Gateway基础入门与使用实践总结
官网文档:点击查看官网文档 Cloud全家桶中有个很重要的组件就是网关,在1.x版本中都是采用的Zuul网关。但在2.x版本中,zuul的升级一直跳票,SpringCloud最后自己研发了一个网关替代Zuul,那就是SpringCloud Gateway一句话…...
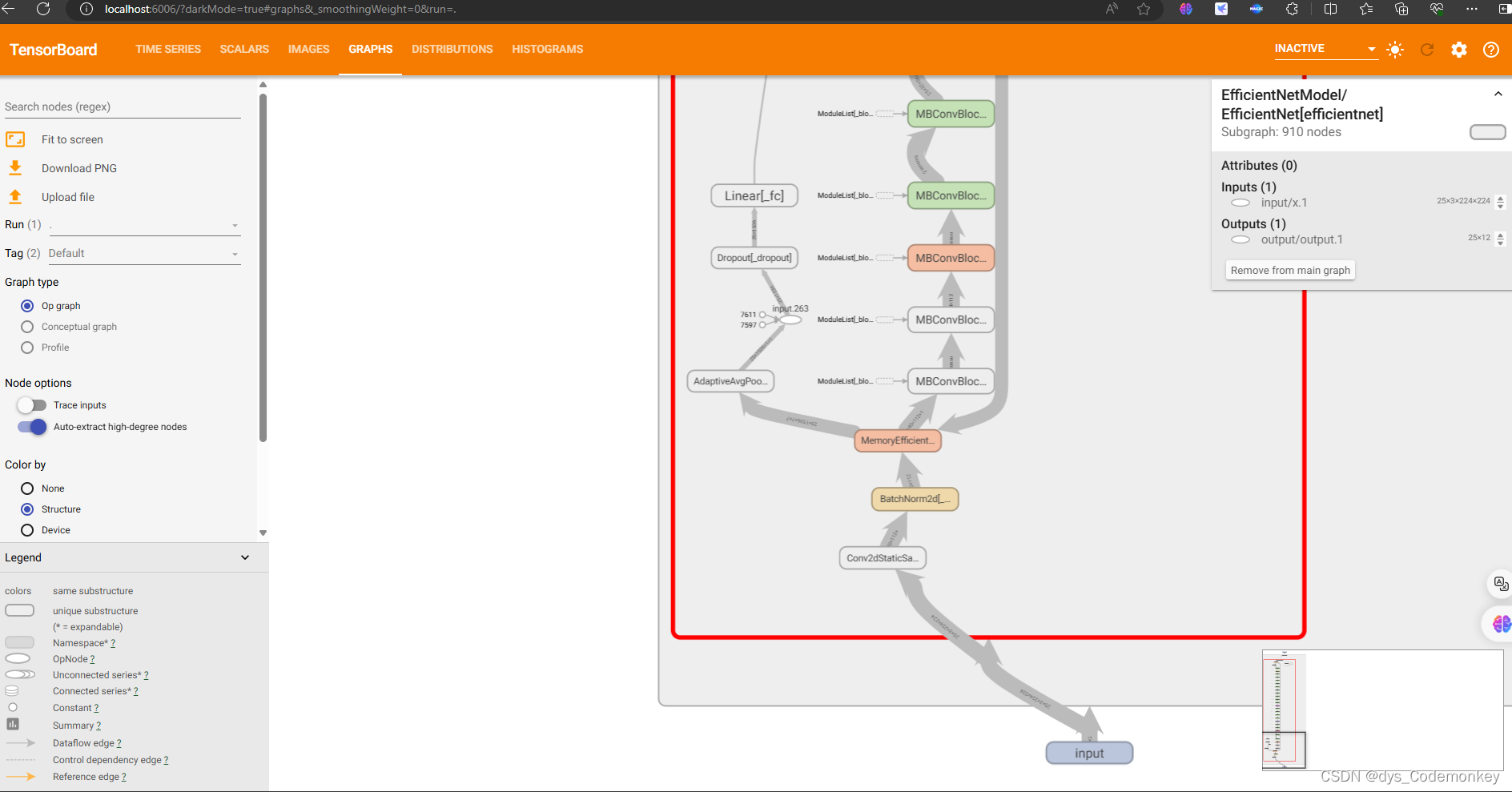
TensorBoard在pytorch训练过程中如何使用,及数据读取问题解决方法
TensorBoard 模块导入日志记录文件的创建训练中如何写入数据如何提取保存的数据调用TensorBoard面板可能会遇到的问题 模块导入 首先从torch中导入tensorboard的SummaryWriter日志记录模块 from torch.utils.tensorboard import SummaryWriter然后导入要用到的os库࿰…...
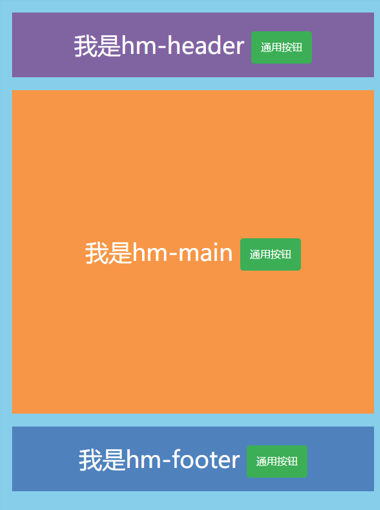
【Vue】普通组件的注册使用-全局注册
文章目录 一、使用步骤二、练习 一、使用步骤 步骤 创建.vue组件(三个组成部分)main.js中进行全局注册 使用方式 当成HTML标签直接使用 <组件名></组件名> 注意 组件名规范 —> 大驼峰命名法, 如 HmHeader 技巧…...
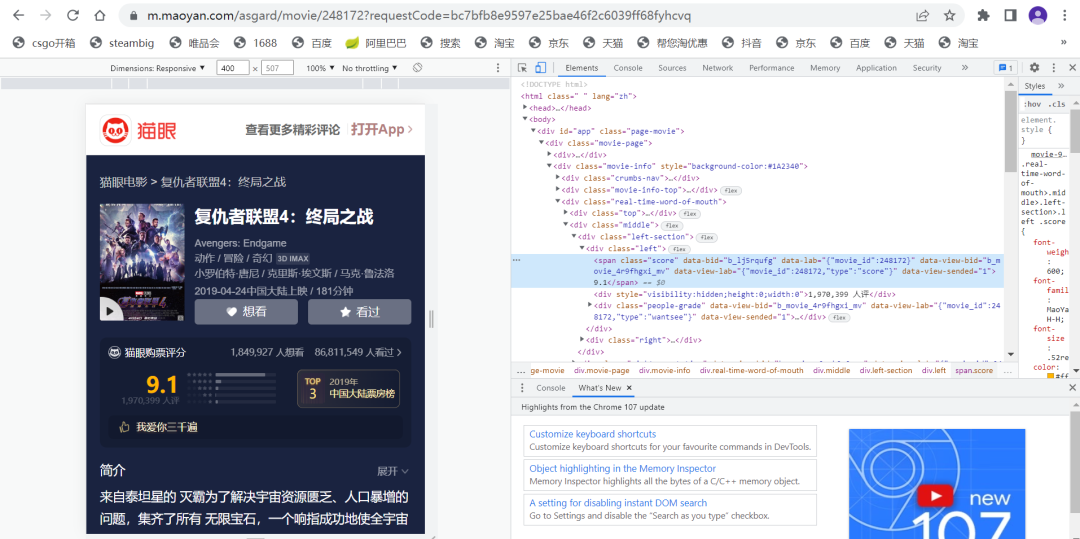
爬虫之反爬思路与解决手段
阅读时间建议:4分钟 本篇概念比较多,嗯。。 0x01 反爬思路与解决手段 1、服务器反爬虫的原因 因为爬虫的访问次数高,浪费资源,公司资源被批量抓走,丧失竞争力,同时也是法律的灰色地带。 2、服务器反什么…...
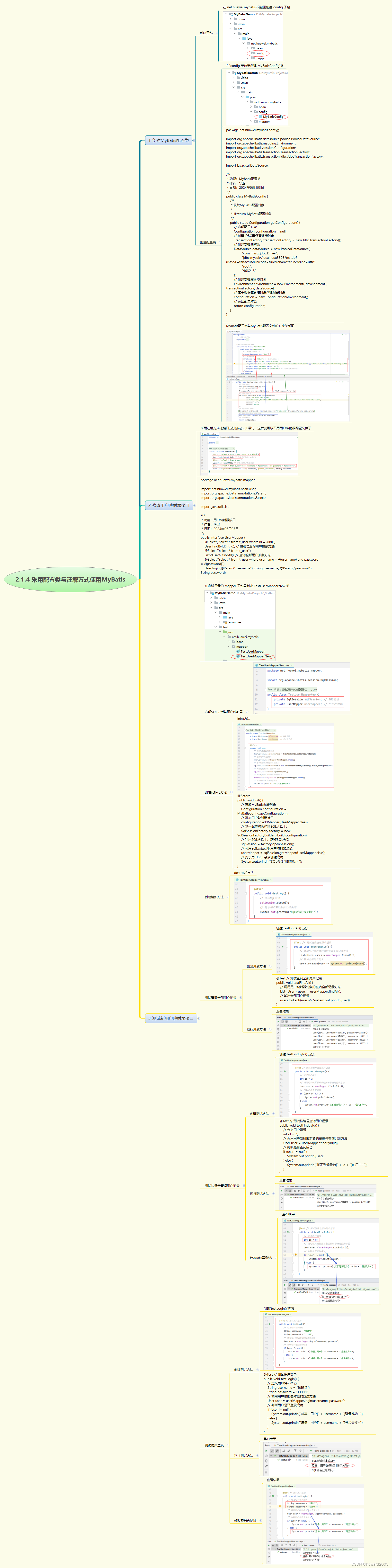
2.1.4 采用配置类与注解方式使用MyBatis
实战概述:采用配置类与注解方式使用MyBatis 创建MyBatis配置类 在net.huawei.mybatis.config包中创建MyBatisConfig类,用于配置MyBatis核心组件,包括数据源、事务工厂和环境设置。 配置数据源和事务 使用PooledDataSource配置MySQL数据库连接…...
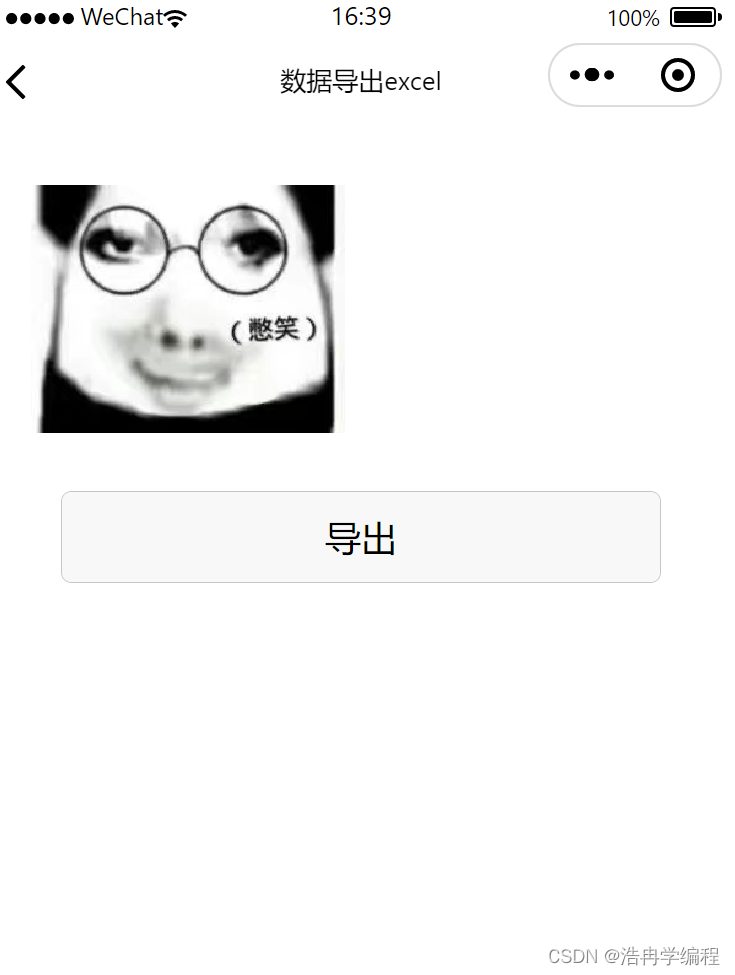
微信小程序云开发实现利用云函数将数据库表的数据导出到excel中
实现目标 将所有订单信息导出到excel表格中 思路 1、在页面中bindtap绑定一个导出点击事件daochu() 2、先获取所有订单信息,并将数据添加到List数组中 3、传入以List数组作为参数,调用get_excel云函数 4、get_excel云函数利用node-xlsx第三方库&#…...