AIRNet模型使用与代码分析(All-In-One Image Restoration Network)
AIRNet提出了一种较为简易的pipeline,以单一网络结构应对多种任务需求(不同类型,不同程度)。但在效果上看,ALL-In-One是不如One-By-One的,且本文方法的亮点是batch内选择patch进行对比学习。在与sota对比上,仅是Denoise任务精度占优,在Derain与Dehaze任务上,效果不如One-By-One的MPRNet方法。本博客对AIRNet的关键结构实现,loss实现,data_patch实现进行深入分析,并对模型进行推理使用。
其论文的详细可以阅读:https://blog.csdn.net/a486259/article/details/139559389?spm=1001.2014.3001.5501
项目地址:https://blog.csdn.net/a486259/article/details/139559389?spm=1001.2014.3001.5501
项目依赖:torch、mmcv-full
安装mmcv-full时,需要注意torch所对应的cuda版本,要与系统中的cuda版本一致。
1、模型结构
AirNet的网络结构如下所示,输入图像x交由CBDE提取到嵌入空间z,z与x输入到DGRN模块的DGG block中逐步优化,最终输出预测结果。
模型代码在net\model.py
from torch import nnfrom net.encoder import CBDE
from net.DGRN import DGRNclass AirNet(nn.Module):def __init__(self, opt):super(AirNet, self).__init__()# Encoderself.E = CBDE(opt) #编码特征值# Restorerself.R = DGRN(opt) #特征解码def forward(self, x_query, x_key):if self.training:fea, logits, labels, inter = self.E(x_query, x_key)restored = self.R(x_query, inter)return restored, logits, labelselse:fea, inter = self.E(x_query, x_query)restored = self.R(x_query, inter)return restored
1.1 CBDE模块
CBDE模块的功能是在模块内进行对比学习,核心是MoCo. Moco论文地址:https://arxiv.org/pdf/1911.05722
class CBDE(nn.Module):def __init__(self, opt):super(CBDE, self).__init__()dim = 256# Encoderself.E = MoCo(base_encoder=ResEncoder, dim=dim, K=opt.batch_size * dim)def forward(self, x_query, x_key):if self.training:# degradation-aware represenetion learningfea, logits, labels, inter = self.E(x_query, x_key)return fea, logits, labels, interelse:# degradation-aware represenetion learningfea, inter = self.E(x_query, x_query)return fea, inter
ResEncoder所对应的网络结构如下所示
在AIRNet中的CBDE模块里的MoCo模块的关键代码如下,其在内部自行完成了正负样本的分配,最终输出logits, labels用于计算对比损失的loss。但其所优化的模块实际上是ResEncoder
。MoCo模块只是在训练阶段起作用,在推理阶段是不起作用的。
class MoCo(nn.Module):def forward(self, im_q, im_k):"""Input:im_q: a batch of query imagesim_k: a batch of key imagesOutput:logits, targets"""if self.training:# compute query featuresembedding, q, inter = self.encoder_q(im_q) # queries: NxCq = nn.functional.normalize(q, dim=1)# compute key featureswith torch.no_grad(): # no gradient to keysself._momentum_update_key_encoder() # update the key encoder_, k, _ = self.encoder_k(im_k) # keys: NxCk = nn.functional.normalize(k, dim=1)# compute logits# Einstein sum is more intuitive# positive logits: Nx1l_pos = torch.einsum('nc,nc->n', [q, k]).unsqueeze(-1)# negative logits: NxKl_neg = torch.einsum('nc,ck->nk', [q, self.queue.clone().detach()])# logits: Nx(1+K)logits = torch.cat([l_pos, l_neg], dim=1)# apply temperaturelogits /= self.T# labels: positive key indicatorslabels = torch.zeros(logits.shape[0], dtype=torch.long).cuda()# dequeue and enqueueself._dequeue_and_enqueue(k)return embedding, logits, labels, interelse:embedding, _, inter = self.encoder_q(im_q)return embedding, inter
1.2 DGRN模块
DGRN模块的实现代码如下所示,可以看到核心是DGG模块,其不断迭代优化输入图像。
class DGRN(nn.Module):def __init__(self, opt, conv=default_conv):super(DGRN, self).__init__()self.n_groups = 5n_blocks = 5n_feats = 64kernel_size = 3# head modulemodules_head = [conv(3, n_feats, kernel_size)]self.head = nn.Sequential(*modules_head)# bodymodules_body = [DGG(default_conv, n_feats, kernel_size, n_blocks) \for _ in range(self.n_groups)]modules_body.append(conv(n_feats, n_feats, kernel_size))self.body = nn.Sequential(*modules_body)# tailmodules_tail = [conv(n_feats, 3, kernel_size)]self.tail = nn.Sequential(*modules_tail)def forward(self, x, inter):# headx = self.head(x)# bodyres = xfor i in range(self.n_groups):res = self.body[i](res, inter)res = self.body[-1](res)res = res + x# tailx = self.tail(res)return x
DGG模块的结构示意如下所示
DGG代码实现如下所示,DGG模块内嵌DGB模块,DGB模块内嵌DGM模块,DGM模块内嵌SFT_layer模块与DCN_layer(可变性卷积)
2、loss实现
AIRNet中提到的loss如下所示,其中Lrec是L1 loss,Lcl是Moco模块实现的对比损失。
AIRNet的loss实现代码在train.py中,CE loss是针对CBDE(Moco模块)的输出进行计算,l1 loss是针对修复图像与清晰图片。
# Network Constructionnet = AirNet(opt).cuda()net.train()# Optimizer and Lossoptimizer = optim.Adam(net.parameters(), lr=opt.lr)CE = nn.CrossEntropyLoss().cuda()l1 = nn.L1Loss().cuda()# Start trainingprint('Start training...')for epoch in range(opt.epochs):for ([clean_name, de_id], degrad_patch_1, degrad_patch_2, clean_patch_1, clean_patch_2) in tqdm(trainloader):degrad_patch_1, degrad_patch_2 = degrad_patch_1.cuda(), degrad_patch_2.cuda()clean_patch_1, clean_patch_2 = clean_patch_1.cuda(), clean_patch_2.cuda()optimizer.zero_grad()if epoch < opt.epochs_encoder:_, output, target, _ = net.E(x_query=degrad_patch_1, x_key=degrad_patch_2)contrast_loss = CE(output, target)loss = contrast_losselse:restored, output, target = net(x_query=degrad_patch_1, x_key=degrad_patch_2)contrast_loss = CE(output, target)l1_loss = l1(restored, clean_patch_1)loss = l1_loss + 0.1 * contrast_loss# backwardloss.backward()optimizer.step()
这里可以看出来,AIRNet首先是训练CBDE模块,最后才训练CBDE模块+DGRN模块。
3、TrainDataset
TrainDataset的实现代码在utils\dataset_utils.py中,首先找到__getitem__函数进行分析。以下代码为关键部分,删除了大部分在逻辑上重复的部分。TrainDataset一共支持5种数据类型,‘denoise_15’: 0, ‘denoise_25’: 1, ‘denoise_50’: 2,是不需要图像对的(在代码里面自动对图像添加噪声);‘derain’: 3, ‘dehaze’: 4是需要图像对进行训练的。
class TrainDataset(Dataset):def __init__(self, args):super(TrainDataset, self).__init__()self.args = argsself.rs_ids = []self.hazy_ids = []self.D = Degradation(args)self.de_temp = 0self.de_type = self.args.de_typeself.de_dict = {'denoise_15': 0, 'denoise_25': 1, 'denoise_50': 2, 'derain': 3, 'dehaze': 4}self._init_ids()self.crop_transform = Compose([ToPILImage(),RandomCrop(args.patch_size),])self.toTensor = ToTensor()def __getitem__(self, _):de_id = self.de_dict[self.de_type[self.de_temp]]if de_id < 3:if de_id == 0:clean_id = self.s15_ids[self.s15_counter]self.s15_counter = (self.s15_counter + 1) % self.num_cleanif self.s15_counter == 0:random.shuffle(self.s15_ids)# clean_id = random.randint(0, len(self.clean_ids) - 1)clean_img = crop_img(np.array(Image.open(clean_id).convert('RGB')), base=16)clean_patch_1, clean_patch_2 = self.crop_transform(clean_img), self.crop_transform(clean_img)clean_patch_1, clean_patch_2 = np.array(clean_patch_1), np.array(clean_patch_2)# clean_name = self.clean_ids[clean_id].split("/")[-1].split('.')[0]clean_name = clean_id.split("/")[-1].split('.')[0]clean_patch_1, clean_patch_2 = random_augmentation(clean_patch_1, clean_patch_2)degrad_patch_1, degrad_patch_2 = self.D.degrade(clean_patch_1, clean_patch_2, de_id)clean_patch_1, clean_patch_2 = self.toTensor(clean_patch_1), self.toTensor(clean_patch_2)degrad_patch_1, degrad_patch_2 = self.toTensor(degrad_patch_1), self.toTensor(degrad_patch_2)self.de_temp = (self.de_temp + 1) % len(self.de_type)if self.de_temp == 0:random.shuffle(self.de_type)return [clean_name, de_id], degrad_patch_1, degrad_patch_2, clean_patch_1, clean_patch_2
可以看出TrainDataset返回的数据有:degrad_patch_1, degrad_patch_2, clean_patch_1, clean_patch_2。
3.1 clean_patch分析
通过以下代码可以看出 clean_patch_1, clean_patch_2是来自于同一个图片,然后基于crop_transform变化,变成了2个对象
clean_img = crop_img(np.array(Image.open(clean_id).convert('RGB')), base=16)clean_patch_1, clean_patch_2 = self.crop_transform(clean_img), self.crop_transform(clean_img)# clean_name = self.clean_ids[clean_id].split("/")[-1].split('.')[0]clean_name = clean_id.split("/")[-1].split('.')[0]clean_patch_1, clean_patch_2 = random_augmentation(clean_patch_1, clean_patch_2)
crop_transform的定义如下,可见是随机进行crop
crop_transform = Compose([ToPILImage(),RandomCrop(args.patch_size),])
random_augmentation的实现代码如下,可以看到只是随机对图像进行翻转或旋转,其目的是尽可能使随机crop得到clean_patch_1, clean_patch_2差异更大,避免裁剪出高度相似的patch。
def random_augmentation(*args):out = []flag_aug = random.randint(1, 7)for data in args:out.append(data_augmentation(data, flag_aug).copy())return out
def data_augmentation(image, mode):if mode == 0:# originalout = image.numpy()elif mode == 1:# flip up and downout = np.flipud(image)elif mode == 2:# rotate counterwise 90 degreeout = np.rot90(image)elif mode == 3:# rotate 90 degree and flip up and downout = np.rot90(image)out = np.flipud(out)elif mode == 4:# rotate 180 degreeout = np.rot90(image, k=2)elif mode == 5:# rotate 180 degree and flipout = np.rot90(image, k=2)out = np.flipud(out)elif mode == 6:# rotate 270 degreeout = np.rot90(image, k=3)elif mode == 7:# rotate 270 degree and flipout = np.rot90(image, k=3)out = np.flipud(out)else:raise Exception('Invalid choice of image transformation')return out
3.2 degrad_patch分析
degrad_patch来自于clean_patch,可以看到是通过D.degrade进行转换的。
degrad_patch_1, degrad_patch_2 = self.D.degrade(clean_patch_1, clean_patch_2, de_id)
D.degrade相关的代码如下,可以看到只是对图像添加噪声。难怪AIRNet在图像去噪上效果最好。
class Degradation(object):def __init__(self, args):super(Degradation, self).__init__()self.args = argsself.toTensor = ToTensor()self.crop_transform = Compose([ToPILImage(),RandomCrop(args.patch_size),])def _add_gaussian_noise(self, clean_patch, sigma):# noise = torch.randn(*(clean_patch.shape))# clean_patch = self.toTensor(clean_patch)noise = np.random.randn(*clean_patch.shape)noisy_patch = np.clip(clean_patch + noise * sigma, 0, 255).astype(np.uint8)# noisy_patch = torch.clamp(clean_patch + noise * sigma, 0, 255).type(torch.int32)return noisy_patch, clean_patchdef _degrade_by_type(self, clean_patch, degrade_type):if degrade_type == 0:# denoise sigma=15degraded_patch, clean_patch = self._add_gaussian_noise(clean_patch, sigma=15)elif degrade_type == 1:# denoise sigma=25degraded_patch, clean_patch = self._add_gaussian_noise(clean_patch, sigma=25)elif degrade_type == 2:# denoise sigma=50degraded_patch, clean_patch = self._add_gaussian_noise(clean_patch, sigma=50)return degraded_patch, clean_patchdef degrade(self, clean_patch_1, clean_patch_2, degrade_type=None):if degrade_type == None:degrade_type = random.randint(0, 3)else:degrade_type = degrade_typedegrad_patch_1, _ = self._degrade_by_type(clean_patch_1, degrade_type)degrad_patch_2, _ = self._degrade_by_type(clean_patch_2, degrade_type)return degrad_patch_1, degrad_patch_2
4、推理演示
项目中默认包含了All.pth,要单独任务的模型可以到预训练模型下载地址: Google Drive and Baidu Netdisk (password: cr7d). 下载模型放到 ckpt/ 目录下
打开demo.py,将 subprocess.check_output(['mkdir', '-p', opt.output_path])
替换为os.makedirs(opt.output_path,exist_ok=True)
,避免在window上报错,具体修改如下所示
demo.py默认从test\demo目录下读取图片进行测试,可见原始图像如下
代码运行后的输出结果默认保存在 output\demo目录下,可见对于去雨,去雾,去噪声效果都比较好。
模型推理时间如下所示,可以看到对一张320, 480的图片,要0.54s
相关文章:
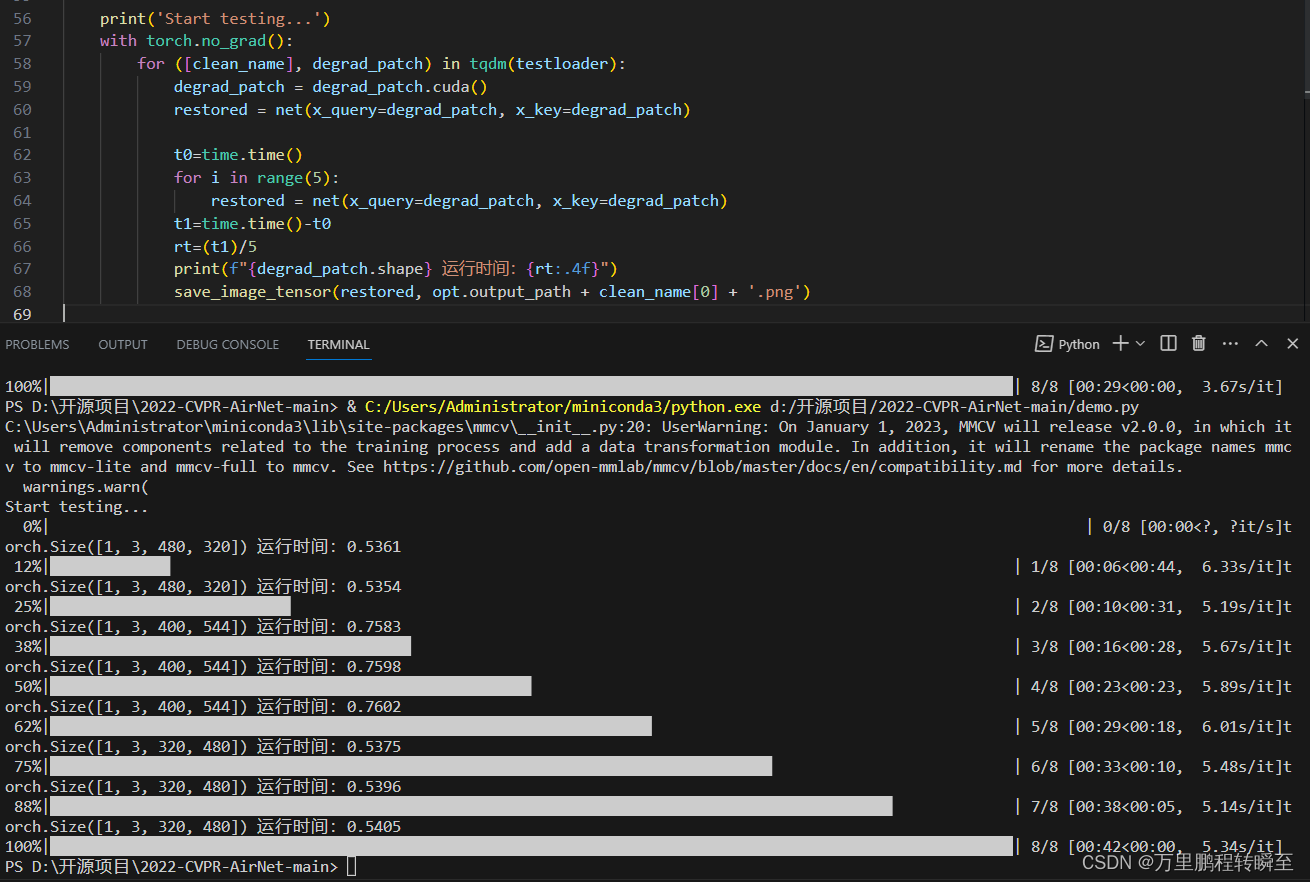
AIRNet模型使用与代码分析(All-In-One Image Restoration Network)
AIRNet提出了一种较为简易的pipeline,以单一网络结构应对多种任务需求(不同类型,不同程度)。但在效果上看,ALL-In-One是不如One-By-One的,且本文方法的亮点是batch内选择patch进行对比学习。在与sota对比上…...
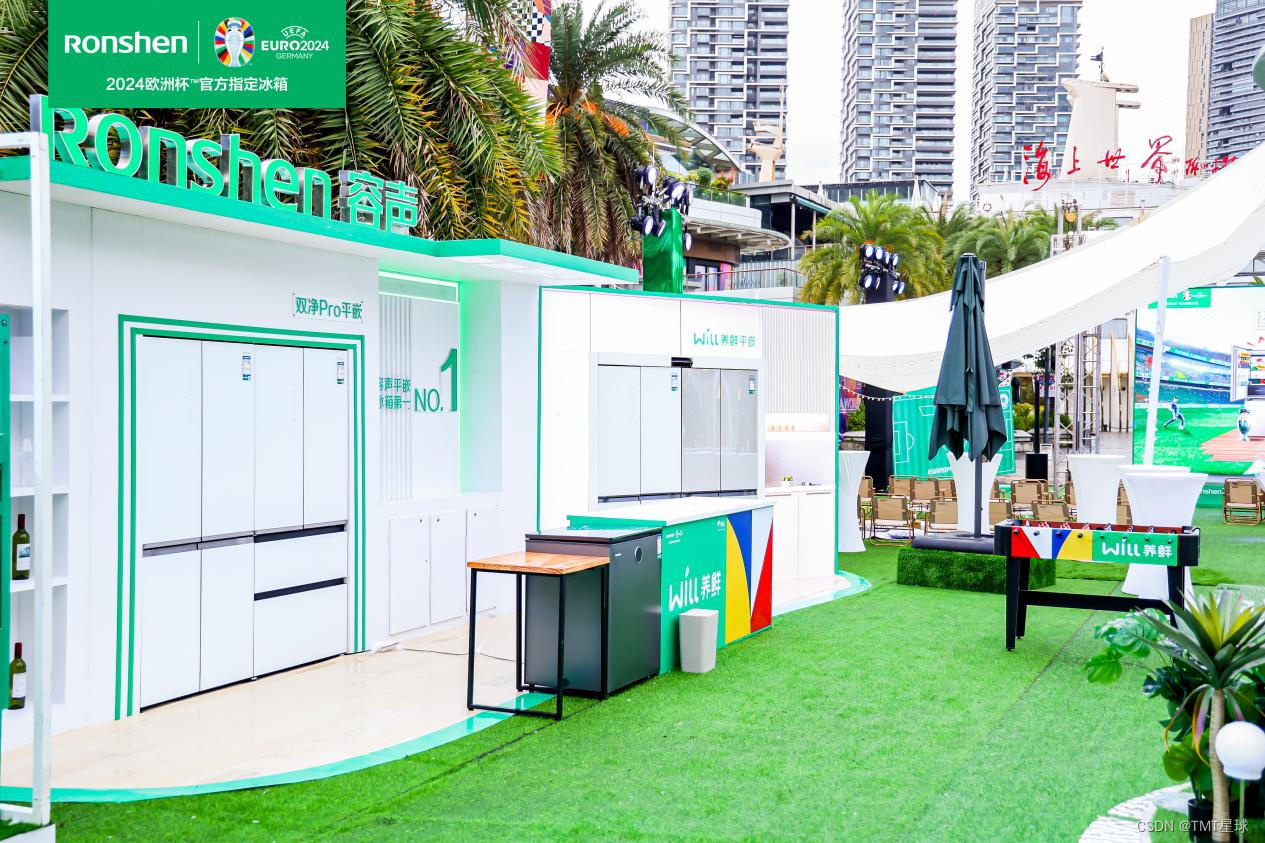
欧洲杯“球迷狂欢趴”开启,容声带来“健康养鲜”新理念
6月15日,容声冰箱在深圳举行了异彩纷呈的“欧洲杯养鲜补给站 球迷狂欢趴”系列活动。 容声国内营销总经理韩栋现场发布“以品质领先 为健康养鲜”的主题内容,强调容声将以健康养鲜技术产品的升级迭代,满足用户品质生活需求。 作为有着41年发…...
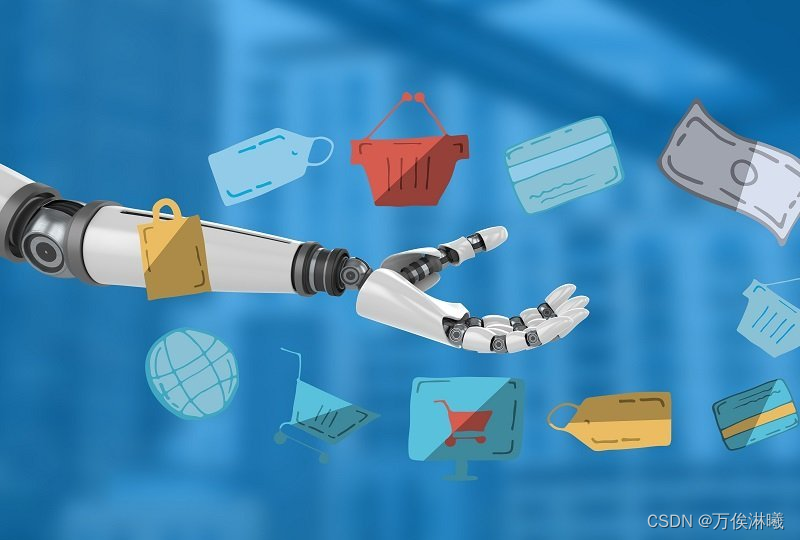
人工智能对零售业的影响
机器人、人工智能相关领域 news/events (专栏目录) 本文目录 一、人工智能如何改变零售格局二、利用人工智能实现购物体验自动化三、利用人工智能改善库存管理四、通过人工智能解决方案增强客户服务五、利用人工智能分析消费者行为六、利用 AI 打造个性化…...
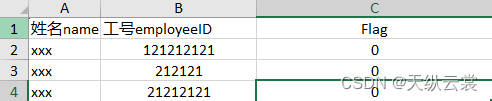
Spring Boot + EasyExcel + SqlServer 进行批量处理数据
前言 在日常开发和工作中,我们可能要根据用户上传的文件做一系列的处理,本篇文章就以Excel表格文件为例,模拟用户上传Excel文件,讲述后端如何高效的进行数据的处理。 一.引入 EasyExcel 依赖 <!-- https://mvnrepository.com/…...
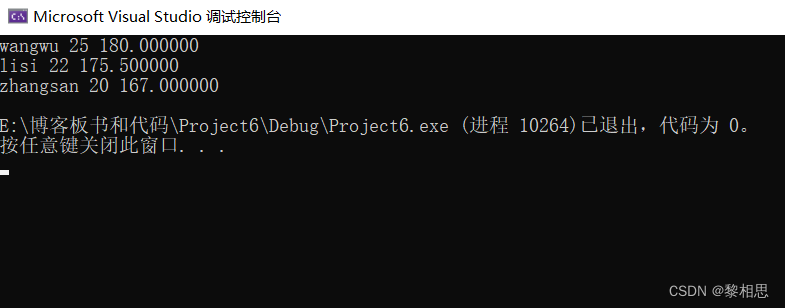
深入理解指针(四)
目录 1. 回调函数是什么? 2. qsort使用举例 2.1冒泡排序 2.2使用qsort函数排序整型数据 2.3 使用qsort排序结构数据(名字) 2.4 使用qsort排序结构数据(年龄) 3. qsort函数的模拟实现 1. 回调函数是什么? 回调函数就是⼀个通过函数指针调⽤的函数。 如果你把函数…...

k-means聚类模型的优缺点
一、k-means聚类模型的优点 1. 简单高效:k-means算法思想简单直观,易于实现。它通过迭代计算样本点与聚类中心之间的距离,并不断调整聚类中心的位置,直至满足终止条件。由于其计算过程相对直接,所以具有较高的执行效率…...
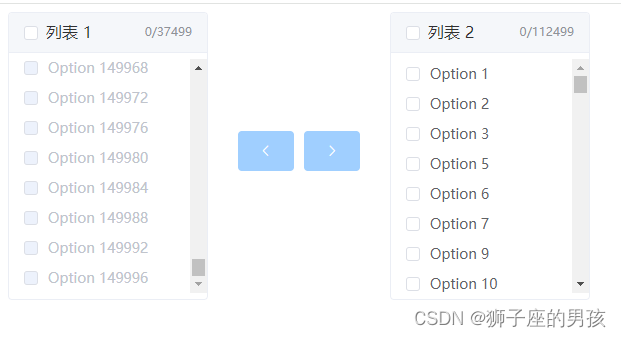
我的创作纪念日(1825天)
Ⅰ、机缘 1. 记得是大一、大二的时候就听学校的大牛说,可以通过写 CSDN 博客,来提升自己的代码和逻辑能力,虽然即将到了写作的第六个年头,但感觉这句话依旧受用; 2、今年一整年的创作都没有停止,本年度几乎是每周都来…...
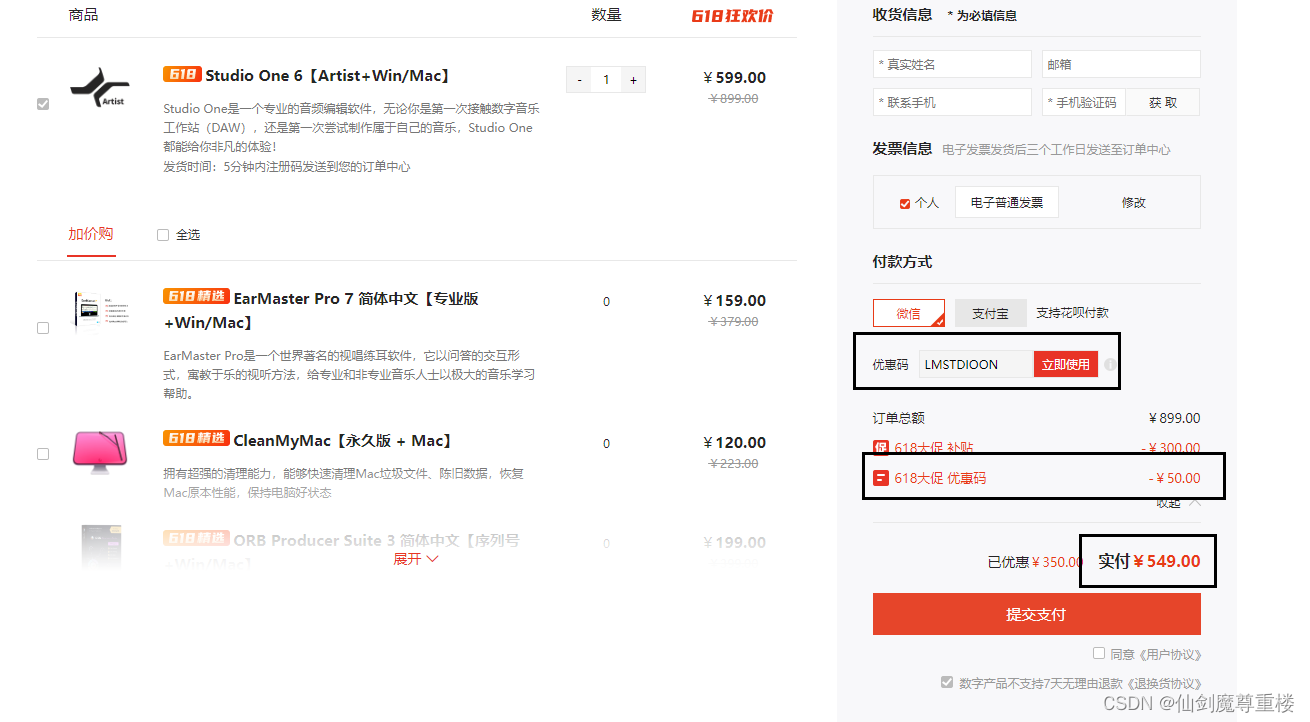
Studio One 6.6.2 for Mac怎么激活,有Studio One 6激活码吗?
如果您是一名音乐制作人,您是否曾经为了寻找一个合适的音频工作站而苦恼过?Studio One 6 for Mac是一款非常适合您的MacBook的音频工作站。它可以帮助您轻松地录制、编辑、混音和发布您的音乐作品。 Studio One 6.6.2 for Mac具有直观的界面和强大的功能…...
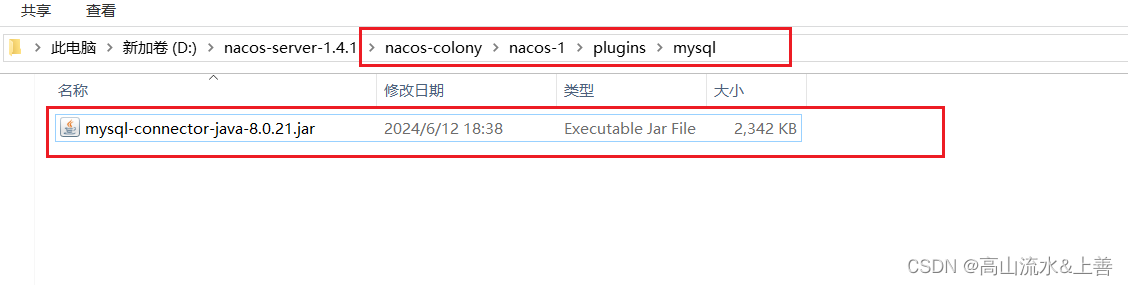
Windows搭建nacos集群
Nacos是阿里巴巴的产品,现在是SpringCloud中的一个组件。相比Eureka功能更加丰富,在国内受欢迎程度较高。 下载地址:Tags alibaba/nacos GitHub 链接:百度网盘 请输入提取码 提取码:8888 解压文件夹 目录说明&am…...

kotlin 中的字符
一、字符类型 1、kotlin中,字符用Char类型表示,值使用单引号 括起来。 fun main() {val a: Char 1println(a) // 1println("a类型为:${a.javaClass.simpleName}") // a类型为:char } 2、特殊字符的表示。 \t——制…...

yocto根文件系统如何配置静态IP地址
在Yocto根文件系统中配置静态IP地址,你可以参考以下步骤。请注意,这些步骤可能会因Yocto版本和具体硬件平台的不同而略有差异。 1. 获取网络配置信息 首先,你需要从网络运维方获取分配的IP地址、子网掩码、默认网关和DNS信息。 2. 确定配置文…...

【博客720】时序数据库基石:LSM Tree的辅助优化
时序数据库基石:LSM Tree的辅助优化 场景: LSM Tree其实本质是一种思想,而具体是否需要WAL,内存表用什么有序数据结构来组织,磁盘上的SSTable用什么结构来存放,是否需要布隆过滤器来加快不存在数据的判断等…...
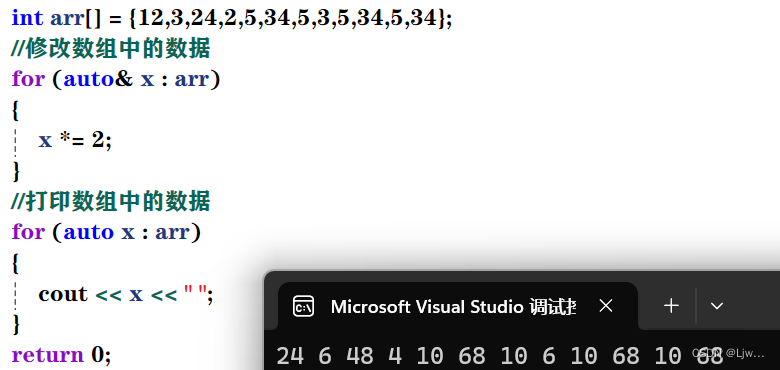
C++前期概念(重)
目录 命名空间 命名空间定义 1. 正常的命名空间定义 2. 命名空间可以嵌套 3.头文件中的合并 命名空间使用 命名空间的使用有三种方式: 1:加命名空间名称及作用域限定符(::) 2:用using将命名空间中某个成员引入 3:使用using namespa…...

Java字符串加密HMAC-SHA1密钥,转换成Base64编码
新建一个maven测试项目,直接把代码复制过去就行,把data和secretKey的值替换成想加密的值。 package test;import javax.crypto.Mac; import javax.crypto.spec.SecretKeySpec; import java.security.InvalidKeyException; import java.security.NoSuchA…...
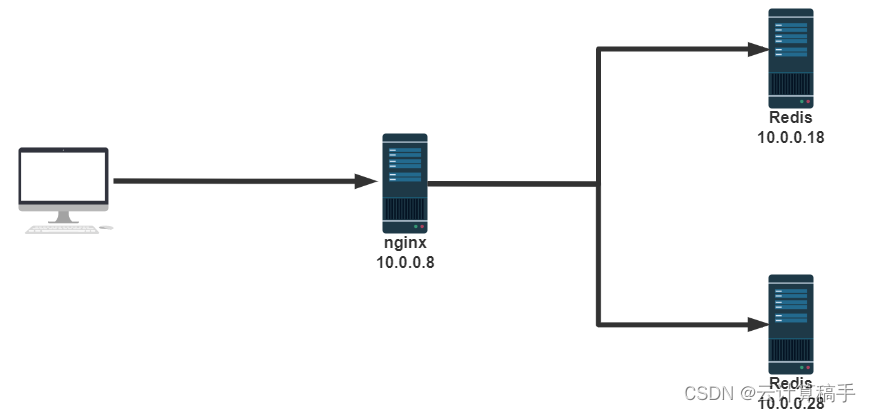
【网络架构】Nginx
目录 一、I/O模型 1.1 Linux 的 I/O 1.2 零拷贝技术 1.3 网络IO模型 1.3.1 阻塞型 I/O 模型(blocking IO)编辑 1.3.2非阻塞型 I/O 模型 (nonblocking IO)编辑 1.3.3 多路复用 I/O 型 ( I/O multiplexing )编辑 1.3.4 信号驱动式 I/O 模型 …...

C# OpenCvSharp 逻辑运算-bitwise_and、bitwise_or、bitwise_not、bitwise_xor
bitwise_and 函数 🤝 作用或原理: 将两幅图像进行与运算,通过逻辑与运算可以单独提取图像中的某些感兴趣区域。如果有掩码参数,则只计算掩码覆盖的图像区域。 示例: 在实际应用中,可以用 bitwise_and 来提取图像中的某些部分。例如,我们可以从图像中提取出一个特定的颜…...
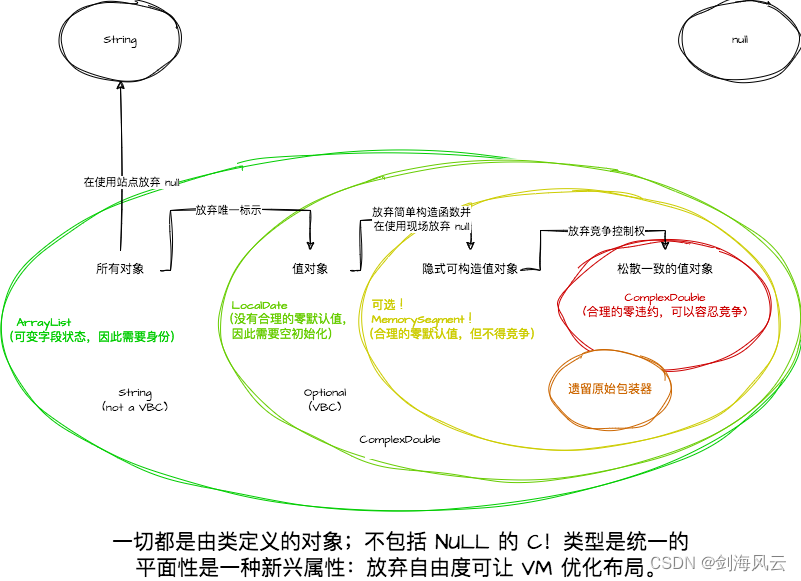
JVM常用概念之扁平化堆容器
扁平化堆容器是OpenJDK Valhalla 项目提出的,其主要目标为将值对象扁平化到其堆容器中,同时支持这些容器的所有指定行为,从而达到不影响原有功能的情况下,显著减少内存空间的占用(理想条件下可以减少24倍)。…...

python面试题5:浅拷贝和深拷贝之间有什么区别?(难度--中等)
文章目录 题目回答1.浅拷贝2.深拷贝 题目 浅拷贝和深拷贝之间有什么区别? 回答 1.浅拷贝 浅拷贝对于不可变数据,如字符串,整数,数组,往往是直接复制其的值。对于可变对象如列表,则是指向同一个地址。这…...

Jetson Linux 上安装ZMQ
1. 安装ZMQ 框架 apt-get install libzmq3-dev 2. 或者自己build ZMQ https://github.com/zeromq/libzmq.git 参考官网教程 3. 安装CPPZMQ CPPZMQ 是ZMQ 的友好的C封装,只需要一个zmq.hpp 头文件即可 git clone https://github.com/zeromq/cppzmq.git cd cppz…...
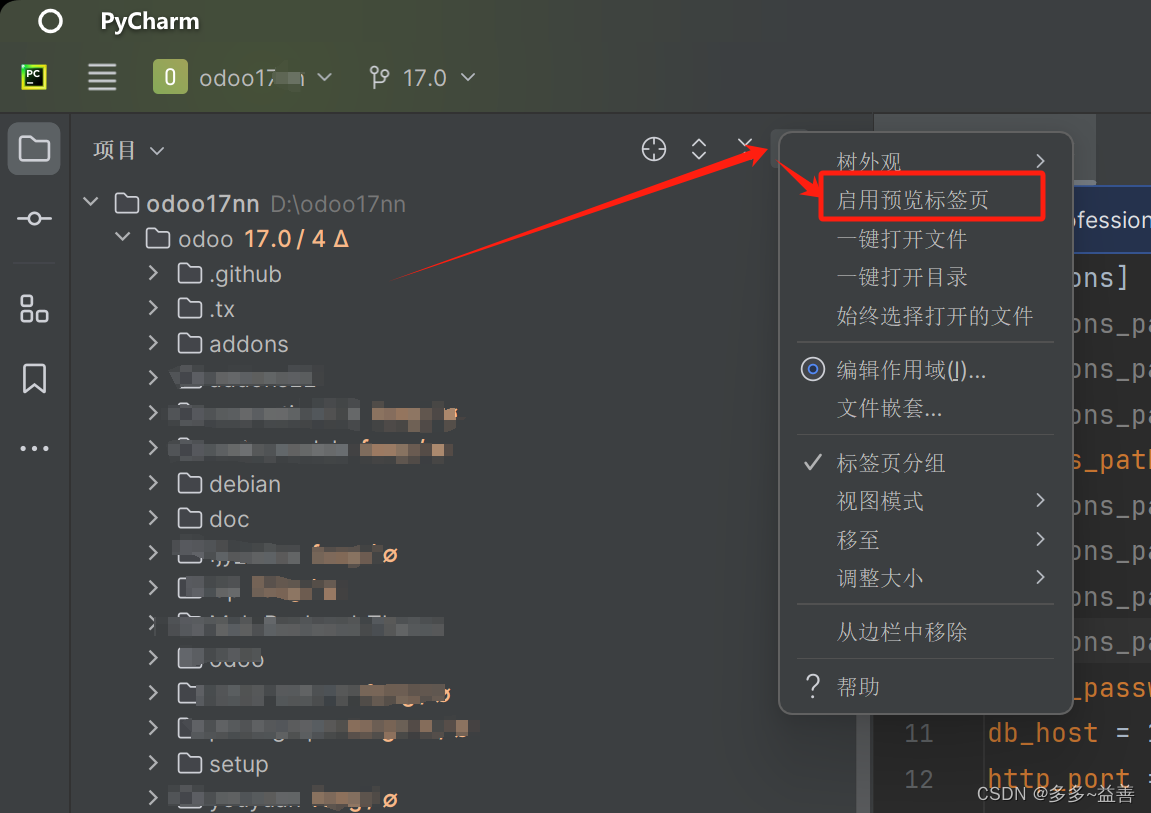
【Pycharm】设置双击打开文件
概要 习惯真可怕。很多小伙伴用习惯了VsCode开发,或者其他一些开发工具,然后某些开发工具是单击目录文件就能打开预览的,而换到pycharm后,发现目录是双击才能打开预览,那么这个用起来就特别不习惯。 解决办法 只需一…...

Web前端后端架构:构建高效、稳定与可扩展的互联网应用
Web前端后端架构:构建高效、稳定与可扩展的互联网应用 在构建互联网应用的过程中,Web前端与后端架构的设计与实施至关重要。一个优秀的架构能够确保应用的稳定性、高效性和可扩展性,为用户提供流畅、安全的体验。本文将从四个方面、五个方面…...
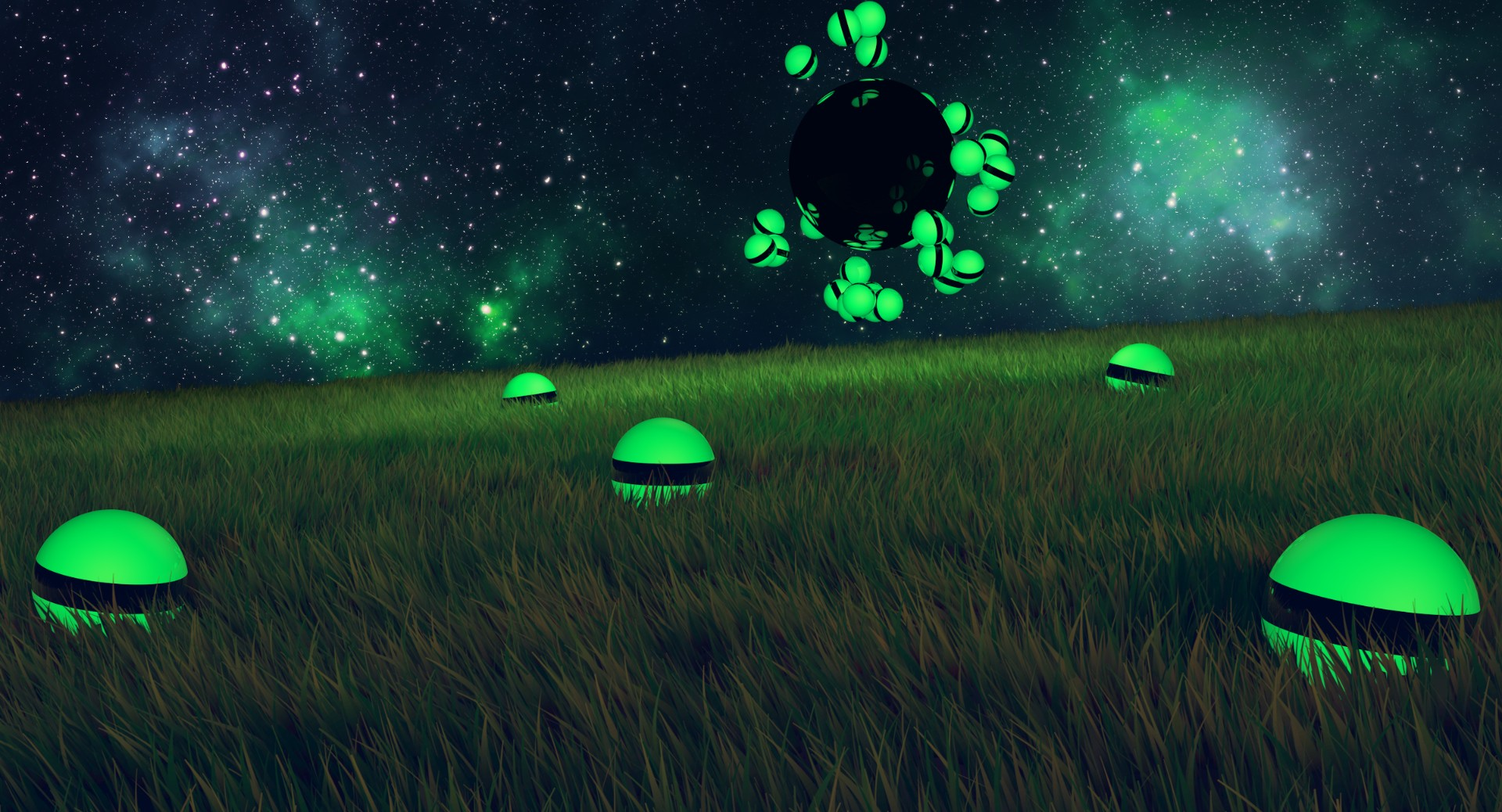
数据仓库核心:事实表深度解析与设计指南
文章目录 1. 引言1.1基本概念1.2 事实表定义 2. 设计原则2.1 原则一:全面覆盖业务相关事实2.2 原则二:精选与业务过程紧密相关的事实2.3 原则三:拆分不可加事实为可加度量2.4 原则四:明确声明事实表的粒度2.5 原则五:避…...

Reactor和epoll
Reactor模式和epoll都是与事件驱动的网络编程相关的术语,但它们属于不同的概念层面: Reactor模式 Reactor模式是一种事件驱动的编程模型,用于处理并发的I/O事件。这种模式使用一个或多个输入源(如套接字),…...
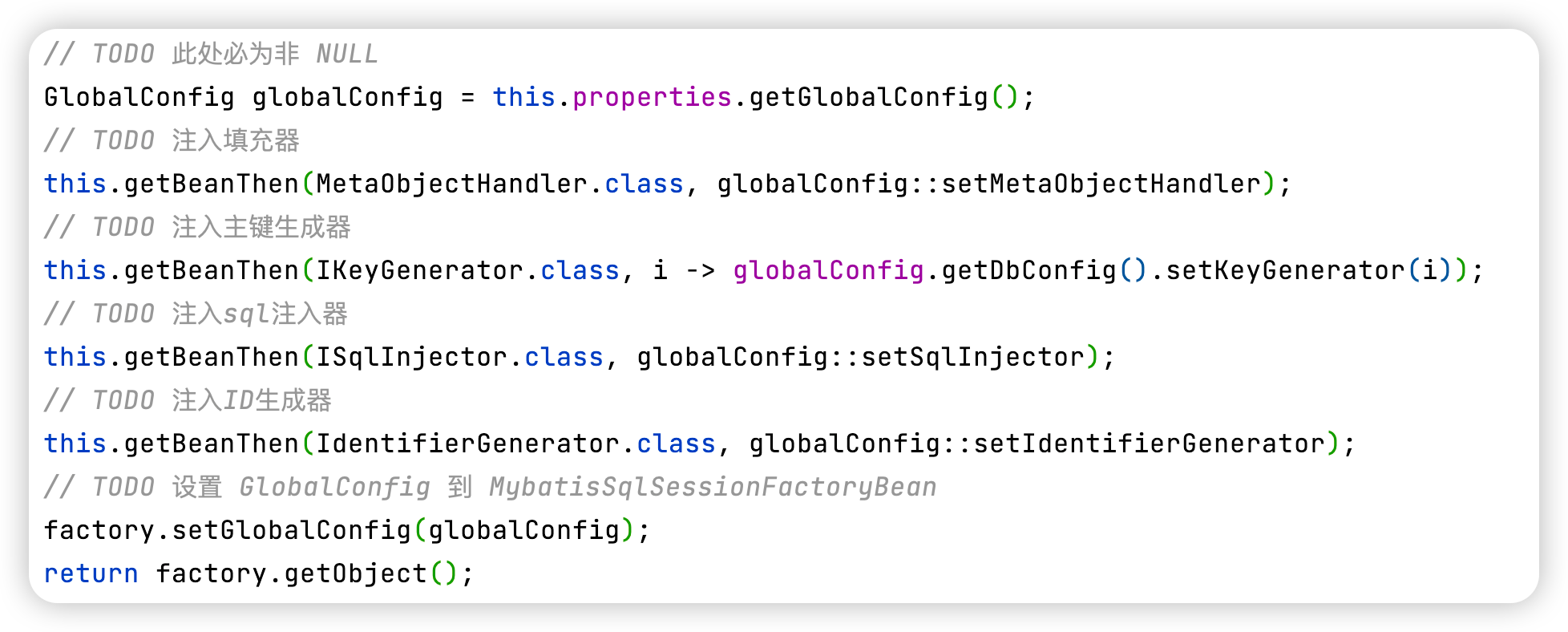
Mybatis-Plus多种批量插入方案对比
背景 六月某日上线了一个日报表任务,因是第一次上线,故需要为历史所有日期都初始化一次报表数据 在执行过程中发现新增特别的慢:插入十万条左右的数据,SQL执行耗费高达三分多钟 因很早就听闻过mybatis-plus的[伪]批量新增的问题&…...

数据库面试
1. 简单介绍一下Spring中的事务管理。 答:事务就是对一系列的数据库操作(比如将insert,delete,update,select多条sql语句)作为一个整体执行,进行统一的提交或回滚操作,如果这组sql语…...
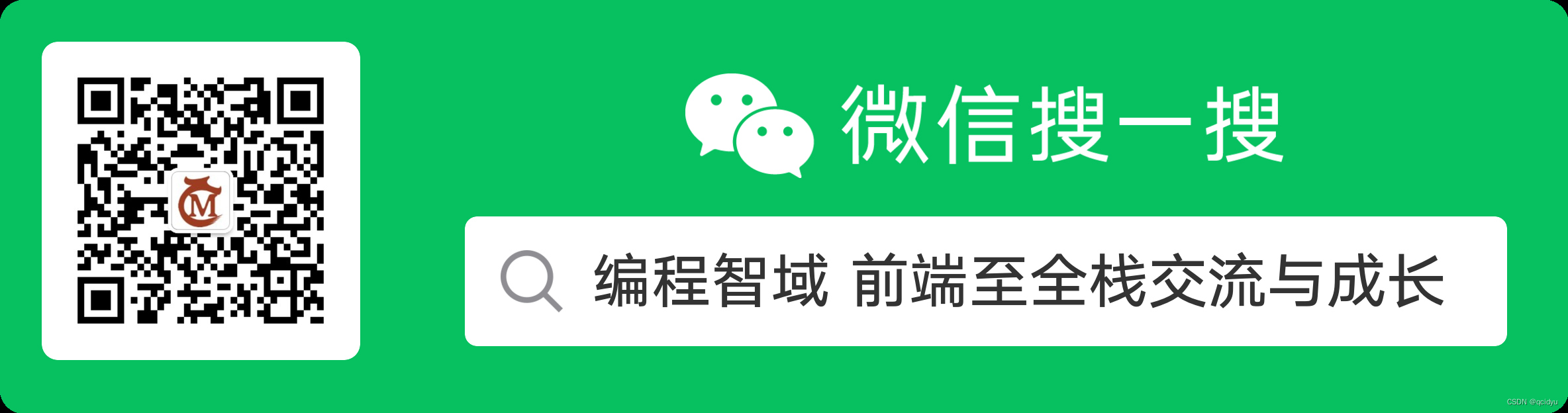
探索Web Components
title: 探索Web Components date: 2024/6/16 updated: 2024/6/16 author: cmdragon excerpt: 这篇文章介绍了Web Components技术,它允许开发者创建可复用、封装良好的自定义HTML元素,并直接在浏览器中运行,无需依赖外部库。通过组合HTML模…...
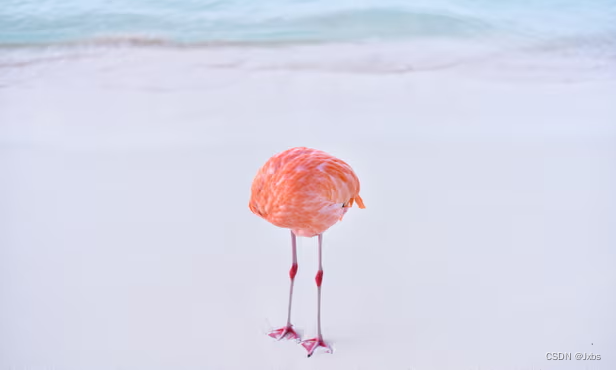
摄影师在人工智能竞赛中与机器较量并获胜
摄影师在人工智能竞赛中与机器较量并获胜 自从生成式人工智能出现以来,由来已久的人机大战显然呈现出一边倒的态势。但是有一位摄影师,一心想证明用人眼拍摄的照片是有道理的,他向算法驱动的竞争对手发起了挑战,并取得了胜利。 迈…...
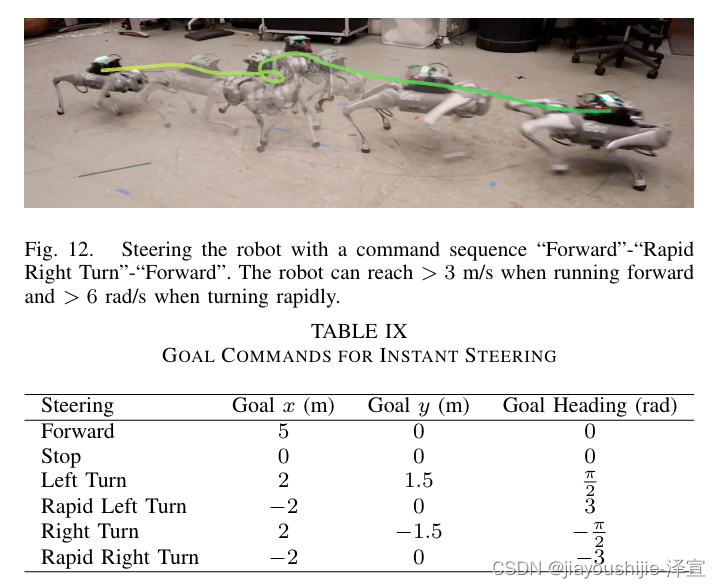
CMU最新论文:机器人智慧流畅的躲避障碍物论文详细讲解
CMU华人博士生Tairan He最新论文:Agile But Safe: Learning Collision-Free High-Speed Legged Locomotion 代码开源:Code: https://github.com/LeCAR-Lab/ABS B站实际效果展示视频地址:bilibili效果地址 我会详细解读论文的内容,让我们开始吧…...

Spring中自定义注解进行类方法增强
说明 说到对类方法增强,第一时间想到自定义注解,通过aop切面进行实现。这是一种常用做法,但是在某些场景下,如开发公共组件,定义aop切面可能不是最优方案。以后通过原生aop方式,自定义注解,对类…...

TS:元组
问: 解释下什么是元组 回答: 元组(Tuple)是一种数据结构,类似于数组,但与数组不同的是,元组中的元素类型可以各不相同,且元组的长度是固定的。元组在许多编程语言中都有实现,包括 TypeScript…...