深度学习部署笔记(十五): CUDA_Run_Time_API_parallel_多流并行,以及多流之间互相同步等待的操作方式
// CUDA运行时头文件
#include <cuda_runtime.h>#include <chrono>
#include <stdio.h>
#include <string.h>using namespace std;#define checkRuntime(op) __check_cuda_runtime((op), #op, __FILE__, __LINE__)bool __check_cuda_runtime(cudaError_t code, const char* op, const char* file, int line){if(code != cudaSuccess){ const char* err_name = cudaGetErrorName(code); const char* err_message = cudaGetErrorString(code); printf("runtime error %s:%d %s failed. \n code = %s, message = %s\n", file, line, op, err_name, err_message); return false;}return true;
}__global__ void add_vector(const float* a, const float* b, float* c, int count){int index = blockDim.x * blockIdx.x + threadIdx.x;if(index >= count) return;c[index] = a[index] + b[index];
}__global__ void mul_vector(const float* a, const float* b, float* c, int count){int index = blockDim.x * blockIdx.x + threadIdx.x;if(index >= count) return;c[index] = a[index] * b[index];
}cudaStream_t stream1, stream2;
float *a, *b, *c1, *c2;
const int num_element = 100000;
const size_t bytes = sizeof(float) * num_element;
const int blocks = 512;
const int grids = (num_element + blocks - 1) / blocks;
const int ntry = 1000;// 多个流异步
void async(){cudaEvent_t event_start1, event_stop1;cudaEvent_t event_start2, event_stop2;checkRuntime(cudaEventCreate(&event_start1));checkRuntime(cudaEventCreate(&event_stop1));checkRuntime(cudaEventCreate(&event_start2));checkRuntime(cudaEventCreate(&event_stop2));auto tic = chrono::duration_cast<chrono::microseconds>(chrono::system_clock::now().time_since_epoch()).count() / 1000.0;checkRuntime(cudaEventRecord(event_start1, stream1));for(int i = 0; i < ntry; ++i)add_vector<<<grids, blocks, 0, stream1>>>(a, b, c1, num_element);checkRuntime(cudaEventRecord(event_stop1, stream1));checkRuntime(cudaEventRecord(event_start2, stream2));for(int i = 0; i < ntry; ++i)add_vector<<<grids, blocks, 0, stream2>>>(a, b, c2, num_element);checkRuntime(cudaEventRecord(event_stop2, stream2));checkRuntime(cudaStreamSynchronize(stream1));checkRuntime(cudaStreamSynchronize(stream2));auto toc = chrono::duration_cast<chrono::microseconds>(chrono::system_clock::now().time_since_epoch()).count() / 1000.0;float time1, time2;checkRuntime(cudaEventElapsedTime(&time1, event_start1, event_stop1));checkRuntime(cudaEventElapsedTime(&time2, event_start2, event_stop2));printf("async: time1 = %.2f ms, time2 = %.2f ms, count = %.2f ms\n", time1, time2, toc - tic);
}// 单个流串行
void sync(){cudaEvent_t event_start1, event_stop1;checkRuntime(cudaEventCreate(&event_start1));checkRuntime(cudaEventCreate(&event_stop1));auto tic = chrono::duration_cast<chrono::microseconds>(chrono::system_clock::now().time_since_epoch()).count() / 1000.0;checkRuntime(cudaEventRecord(event_start1, stream1));for(int i = 0; i < ntry; ++i)add_vector<<<grids, blocks, 0, stream1>>>(a, b, c1, num_element);for(int i = 0; i < ntry; ++i)add_vector<<<grids, blocks, 0, stream1>>>(a, b, c2, num_element);checkRuntime(cudaEventRecord(event_stop1, stream1));checkRuntime(cudaStreamSynchronize(stream1));auto toc = chrono::duration_cast<chrono::microseconds>(chrono::system_clock::now().time_since_epoch()).count() / 1000.0;float time1;checkRuntime(cudaEventElapsedTime(&time1, event_start1, event_stop1));printf("sync: time1 = %.2f ms, count = %.2f ms\n", time1, toc - tic);
}// 多个流之间并行
void multi_stream_async(){// 这个案例主要实现多个流之间互相等待,使用event控制实现// 存在step1 -> step2 \ // -> step3 -> step4// stepa / //// 这个案例中,存在流程1:step1 -> step2的流程// 存在流程2:stepa// 存在流程3:step3 -> step4,step3要求step2与stepa作为输入// 此时,可以让流程1使用stream1,流程2使用stream2,而流程3继续使用stream1,仅仅在stream1中加入等待(event的等待)// step1 = add_vector// step2 = mul_vector// step3 = add_vector// step4 = mul_vector// stepa = add_vector#define step1 add_vector#define step2 mul_vector#define step3 add_vector#define step4 mul_vector#define stepa add_vectorcudaEvent_t event_async;checkRuntime(cudaEventCreate(&event_async));// stream1的执行流程step1<<<grids, blocks, 0, stream1>>>(a, b, c1, num_element);step2<<<grids, blocks, 0, stream1>>>(a, b, c1, num_element);// 等待event_async有事件checkRuntime(cudaStreamWaitEvent(stream1, event_async));step3<<<grids, blocks, 0, stream1>>>(a, b, c2, num_element);step4<<<grids, blocks, 0, stream1>>>(a, b, c2, num_element);// stream2的执行流程stepa<<<grids, blocks, 0, stream2>>>(a, b, c2, num_element);// 为event_async触发事件,通知cudaStreamWaitEvent函数可以继续了checkRuntime(cudaEventRecord(event_async, stream2));checkRuntime(cudaStreamSynchronize(stream1));printf("multi_stream_async done.\n");
}int main(){// 本程序实现两个核函数的并行,通过多个流实现checkRuntime(cudaStreamCreate(&stream1));checkRuntime(cudaStreamCreate(&stream2));checkRuntime(cudaMalloc(&a, bytes));checkRuntime(cudaMalloc(&b, bytes));checkRuntime(cudaMalloc(&c1, bytes));checkRuntime(cudaMalloc(&c2, bytes));// 演示多流之间的异步执行async();// 演示单个流内的同步执行sync();// 演示多个流之间互相等待的操作multi_stream_async();return 0;
}
2. 单个流串行
void sync(){cudaEvent_t event_start1, event_stop1;checkRuntime(cudaEventCreate(&event_start1));checkRuntime(cudaEventCreate(&event_stop1));auto tic = chrono::duration_cast<chrono::microseconds>(chrono::system_clock::now().time_since_epoch()).count() / 1000.0;checkRuntime(cudaEventRecord(event_start1, stream1));for(int i = 0; i < ntry; ++i)add_vector<<<grids, blocks, 0, stream1>>>(a, b, c1, num_element);for(int i = 0; i < ntry; ++i)add_vector<<<grids, blocks, 0, stream1>>>(a, b, c2, num_element);checkRuntime(cudaEventRecord(event_stop1, stream1));checkRuntime(cudaStreamSynchronize(stream1));auto toc = chrono::duration_cast<chrono::microseconds>(chrono::system_clock::now().time_since_epoch()).count() / 1000.0;float time1;checkRuntime(cudaEventElapsedTime(&time1, event_start1, event_stop1));printf("sync: time1 = %.2f ms, count = %.2f ms\n", time1, toc - tic);
}
cuda count time: 12.26, cpp count time: 12.28
这个函数演示了单个流中的同步执行,具体解释如下:
cudaEvent_t 是 CUDA Runtime API 中的一个结构体,定义在 cuda_runtime_api.h 中。它用于表示一个 CUDA 事件对象,用于记录 GPU 上某个时间点的状态。
CUDA 事件可以用于两种目的:
记录一个时间点(如开始时间点或结束时间点)。
记录一个时间间隔(即时间差)。
通常情况下,CUDA 事件被用于在主机和设备之间进行同步,或在设备内部进行同步。例如,可以在主机代码中调用 cudaEventRecord() 来记录一个事件,然后在设备代码中使用 cudaStreamWaitEvent() 等待该事件,以确保某些设备操作发生在之前记录的事件之后。又或者,可以在设备代码中记录两个事件,然后在主机代码中使用 cudaEventElapsedTime() 计算它们之间的时间差。
首先创建两个事件 event_start1 和 event_stop1,用于记录同步执行的时间;
使用 cudaEventRecord 将 event_start1 记录在 stream1 中,表示从这个时间点开始,将会执行在 stream1 中的操作;
使用 for 循环调用 add_vector 核函数,在 stream1 中执行 ntry 次,计算向量 a 和 b 的加和,存储在向量 c1 和 c2 中;
使用 cudaEventRecord 将 event_stop1 记录在 stream1 中,表示到达这个时间点,stream1 中的操作都已经完成;
使用 cudaStreamSynchronize 等待 stream1 中的所有操作执行完毕;
计算同步执行的时间 time1,并输出时间和整个操作的时间。
可以看到,这个函数中只使用了一个流,因此 add_vector 的计算是按照顺序执行的,不能充分发挥 GPU 的并行计算能力。因此,这个函数的计算时间会比异步执行的 async 函数要长
这段代码中使用了两种方法来计算代码执行的时间。
第一种方法是使用了C++标准库中的chrono库来计算代码执行的起始时间和终止时间,通过计算时间差得到代码执行的时间,这个方法在计算异步执行时比较方便,因为我们需要分别记录多个异步操作的起始时间和终止时间。
第二种方法是使用了CUDA提供的API cudaEventElapsedTime,这个API可以计算CUDA事件的时间差,用于计算CUDA事件执行的时间。在这个例子中,我们使用了这个API来计算在单个流上串行执行的时间。
3. 向量相加相乘的kernel function
__global__ void add_vector(const float* a, const float* b, float* c, int count){int index = blockDim.x * blockIdx.x + threadIdx.x;if(index >= count) return;c[index] = a[index] + b[index];
}__global__ void mul_vector(const float* a, const float* b, float* c, int count){int index = blockDim.x * blockIdx.x + threadIdx.x;if(index >= count) return;c[index] = a[index] * b[index];
}
count 是用来限制线程不要访问到超出数组的地址,因为数组的长度在我们开辟的时候就已经定义好了
checkRuntime(cudaMalloc(&a, bytes));
count是num_element, byte是num_element * sizeof(float), 超出地址会访问到虚拟地址
4. 多个流的异步
void async(){cudaEvent_t event_start1, event_stop1;cudaEvent_t event_start2, event_stop2;checkRuntime(cudaEventCreate(&event_start1));checkRuntime(cudaEventCreate(&event_stop1));checkRuntime(cudaEventCreate(&event_start2));checkRuntime(cudaEventCreate(&event_stop2));auto tic = chrono::duration_cast<chrono::microseconds>(chrono::system_clock::now().time_since_epoch()).count() / 1000.0;checkRuntime(cudaEventRecord(event_start1, stream1));for(int i = 0; i < ntry; ++i)add_vector<<<grids, blocks, 0, stream1>>>(a, b, c1, num_element);checkRuntime(cudaEventRecord(event_stop1, stream1));checkRuntime(cudaEventRecord(event_start2, stream2));for(int i = 0; i < ntry; ++i)add_vector<<<grids, blocks, 0, stream2>>>(a, b, c2, num_element);checkRuntime(cudaEventRecord(event_stop2, stream2));checkRuntime(cudaStreamSynchronize(stream1));checkRuntime(cudaStreamSynchronize(stream2));auto toc = chrono::duration_cast<chrono::microseconds>(chrono::system_clock::now().time_since_epoch()).count() / 1000.0;float time1, time2;checkRuntime(cudaEventElapsedTime(&time1, event_start1, event_stop1));checkRuntime(cudaEventElapsedTime(&time2, event_start2, event_stop2));printf("async: time1 = %.2f ms, time2 = %.2f ms, count = %.2f ms\n", time1, time2, toc - tic);
}
async: time1 = 6.97 ms, time2 = 6.94 ms, count = 9.32 ms
输出的内容中包含了在两个流上异步执行的两个内核函数的时间,分别为time1和time2,它们的值应该是相当接近的。同时,输出中还包含了整个函数执行的总时间count,可以看出相比于同步执行的情况,异步执行使得程序的总执行时间更短,效率更高。
5. 多个流之间互相等待的操作
// 这个案例主要实现多个流之间互相等待,使用event控制实现// 存在step1 -> step2 \ // -> step3 -> step4// stepa / //// 这个案例中,存在流程1:step1 -> step2的流程// 存在流程2:stepa// 存在流程3:step3 -> step4,step3要求step2与stepa作为输入// 此时,可以让流程1使用stream1,流程2使用stream2,而流程3继续使用stream1,仅仅在stream1中加入等待(event的等待)// step1 = add_vector// step2 = mul_vector// step3 = add_vector// step4 = mul_vector// stepa = add_vector#define step1 add_vector#define step2 mul_vector#define step3 add_vector#define step4 mul_vector#define stepa add_vectorcudaEvent_t event_async;checkRuntime(cudaEventCreate(&event_async));// stream1的执行流程step1<<<grids, blocks, 0, stream1>>>(a, b, c1, num_element);step2<<<grids, blocks, 0, stream1>>>(a, b, c1, num_element);// 等待event_async有事件checkRuntime(cudaStreamWaitEvent(stream1, event_async));step3<<<grids, blocks, 0, stream1>>>(a, b, c2, num_element);step4<<<grids, blocks, 0, stream1>>>(a, b, c2, num_element);// stream2的执行流程stepa<<<grids, blocks, 0, stream2>>>(a, b, c2, num_element);// 为event_async触发事件,通知cudaStreamWaitEvent函数可以继续了checkRuntime(cudaEventRecord(event_async, stream2));checkRuntime(cudaStreamSynchronize(stream1));printf("multi_stream_async done.\n");
具体流程如下:
在stream1中先执行step1,然后执行step2,这两个步骤是串行执行的;
在stream1中调用cudaStreamWaitEvent函数等待event_async事件,此时流程3(step3和step4)还不能开始执行;
在stream2中执行stepa,此时stepa和之前的步骤是并行执行的;
在stream2中调用cudaEventRecord函数触发event_async事件,通知stream1可以开始执行流程3;
在stream1中执行step3和step4,这两个步骤是串行执行的;
在stream1中调用cudaStreamSynchronize函数等待所有在该流中的操作执行完毕,程序结束。
总结起来,这个多流程的示例展示了如何使用事件来控制不同流之间的顺序和同步,从而实现流程之间的依赖关系和并行执行。
相关文章:
深度学习部署笔记(十五): CUDA_Run_Time_API_parallel_多流并行,以及多流之间互相同步等待的操作方式
// CUDA运行时头文件 #include <cuda_runtime.h>#include <chrono> #include <stdio.h> #include <string.h>using namespace std;#define checkRuntime(op) __check_cuda_runtime((op), #op, __FILE__, __LINE__)bool __check_cuda_runtime(cudaErro…...
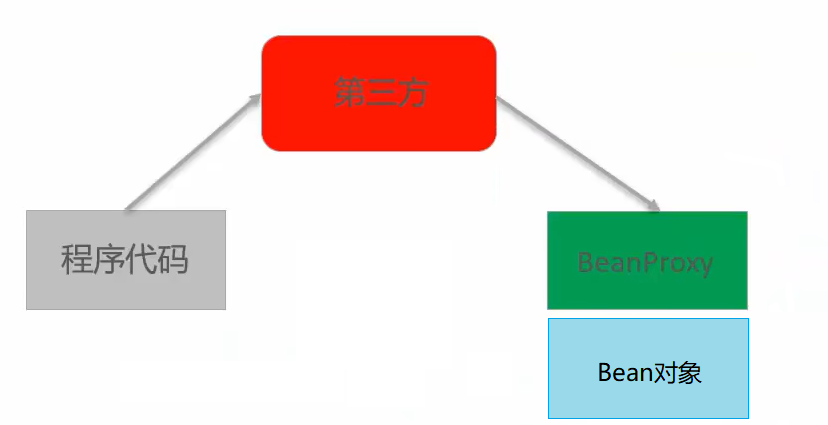
【Spring】spring框架简介
一、框架 1.框架的基本特点: 框架(Framework),是基于基础技术之上,从众多业务中抽取出的通用解决方案;框架是一个半成品,使用框架规定的语法开发可以提高开发效率,可以用简单的代码就能完成复杂的基础业务;框架内部使用大量的设…...
WuThreat身份安全云-TVD每日漏洞情报-2023-03-17
漏洞名称:TP-LINK Archer AX21 命令注入漏洞 漏洞级别:严重 漏洞编号:CVE-2023-1389,CNNVD-202303-1280 相关涉及:TP-LINK Archer AX21 1.1.4 Build 20230219之前的固件版本 漏洞状态:POC 参考链接:https://tvd.wuthreat.com/#/listDetail?TVD_IDTVD-2023-06347 漏洞名称:D-L…...

postman 调用webservice
有个外部接口需要提供古老的webservice 格式接口。1 设置格式按照xml 格式设置。2 消息体xml 封装不加envelope:<soap:Envelope xmlns:soap"" target"_blank">http://schemas.xmlsoap.org/soap/envelope/"><soap:Body><soap:Fault&…...
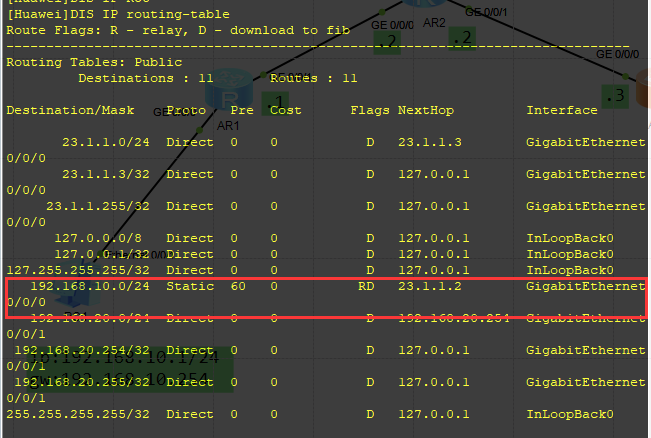
基于华为模拟器(ensp)的静态路由配置实验
一 实验需求静态路由实验,建立拓扑pc1>>R1>>R2>>R3>>pc2,使pc1与pc2能相互通信。二 实验拓扑三 ip地址规划设备接口ip地址AR1G0/0/0192.168.10.254/24G0/0/112.1.1.1/24AR2G0/0/012.1.1.2/24G0/0/123.1.1.2/24 AR3G0/0/023.1.1.…...
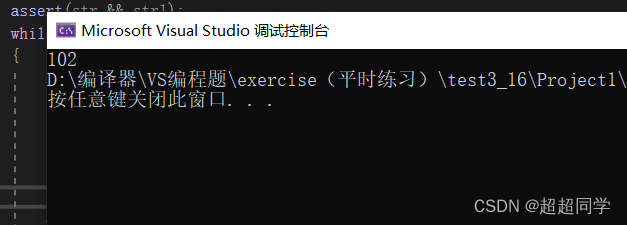
模拟实现字符串函数(长度受限制的详讲)
上次发布了长度不受限制的字符串函数的模拟实现方法,这次就给大家说说长度受限制的字符串函数。首先,长度受限制和不受限制有什么区别呢?其实从某种意义上来讲,长度受限制的字符串函数比长度不受限制的字符串安全,为什…...

分布式ID生成方案总结
什么是分布式 ID 分布式 ID 是指,在分布式环境下可用于对数据进行标识且易存储的全局唯一的 ID 标识。 为什么需要分布式 ID 对于单体系统来说,主键ID可能会常用主键自动的方式进行设置,这种ID生成方法在单体项目是可行的。 对于分布式系统…...
极智AI | 百度推出文心一言,对标ChatGPT功力几成
欢迎关注我,获取我的更多经验分享,极智传送《极智AI | 百度推出文心一言,对标 ChatGPT 功力几成》 大家好,我是极智视界,本文介绍一下 百度今日推出文心一言,对标ChatGPT功力几成。 邀您加入我的知识星球「极智视界」,星球内有超多好玩的项目实战源码下载,链接:https…...
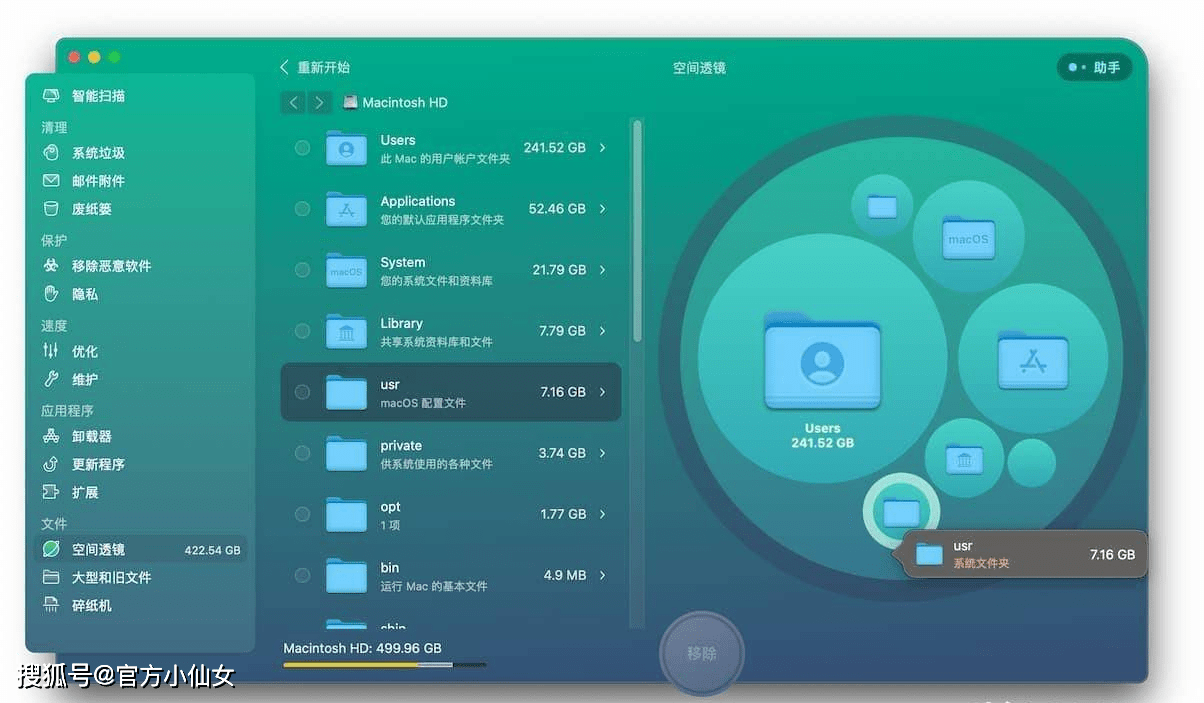
CleanMyMac X最新版本4.12.6
系统要求macOS 10.12及更高,M1机型及最新macOS 13 CleanMyMac可以为Mac腾出空间,软件已经更新到CleanMyMac X支持最新版Mac系统。CleanMyMac具有一系列巧妙的新功能,可让您安全,智能地扫描和清理整个系统,删除大量未使…...
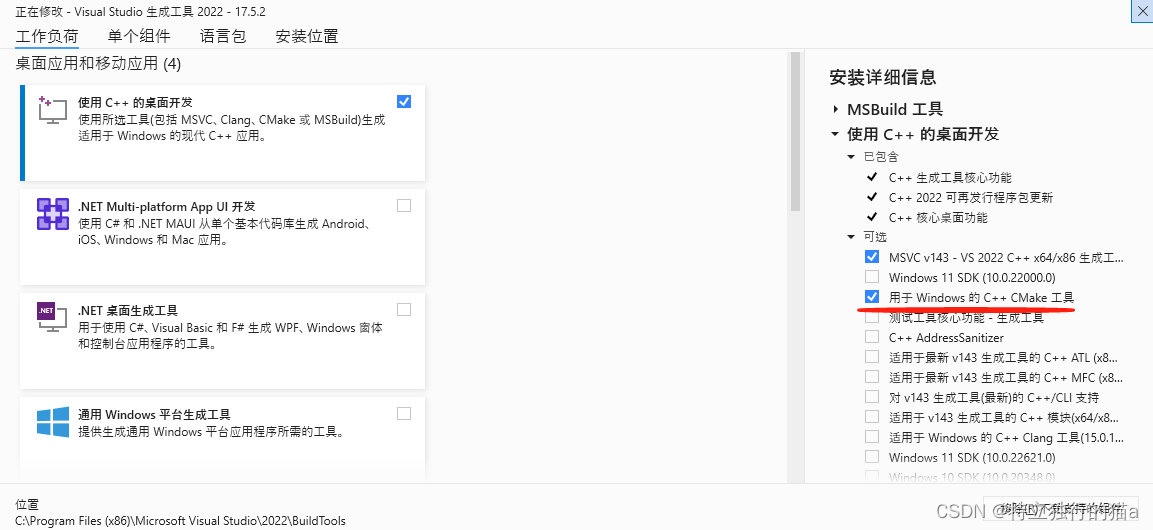
替代notepad++,notepad--介绍及插件cmake编译
Notepad 是一个文本编辑器小软件,用来替代windows自带的记事本。然而Notepad软件的作者是台湾省人,其具有明显的gd/jd/td倾向,如果你不赞同他的观点,Notepad将会在你的源码里面插入随机字符。推荐一款国产的开源跨平台软件NDD(not…...
机器学习笔记之集成学习(四)Gradient Boosting
机器学习笔记之集成学习——Gradient Boosting引言回顾:Boosting\text{Boosting}Boosting算法思想与AdaBoost\text{AdaBoost}AdaBoostGradient Boosting\text{Gradient Boosting}Gradient Boosting算法介绍场景构建算法过程迭代过程与梯度下降法之间的关联关系引言 …...
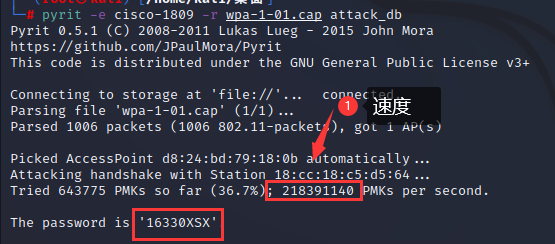
WPA渗透-pyrit:batch-table加速attack_db模块加速_“attack_db”模块加速
WPA渗透-pyrit:batch-table加速attack_db模块加速_“attack_db”模块加速 1.渗透WIFI 1.导入密码字典 pyrit -i 字典文件 import_passwords -i:输入的文件名 import_passwords:从类文件源导入密码。pyrit -i pwd.txt import_passwords2.导…...
kotlin第二部分复习纪要
扩展函数。 例如: fun Context.toast(msg: String, length: Int Toast.LENGTH_SHORT){Toast.makeText(this, msg, length).show() } 使用 val activity: Context? getActivity() activity?.toast("Hello world!") activity?.toast("Hello worl…...
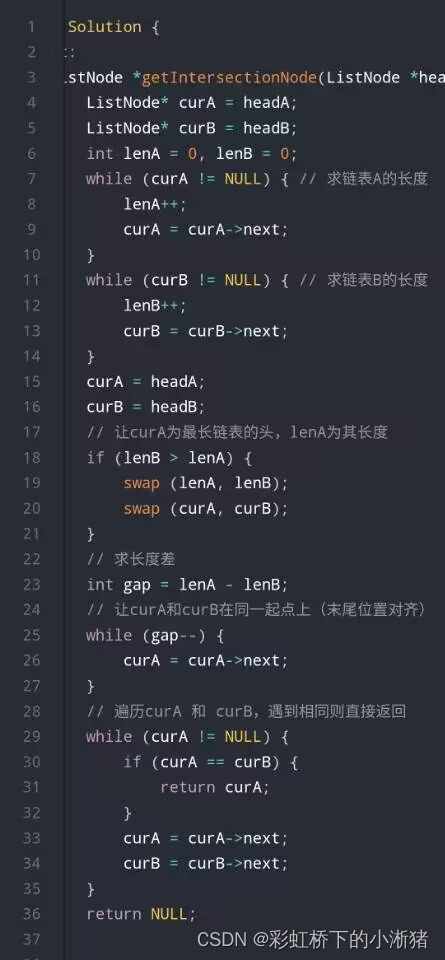
代码随想录--链表--删除链表第n个节点题型、链表相交题型
删除链表第n个节点题型 链表遍历学清楚! | LeetCode:19.删除链表倒数第N个节点 (opens new window) 这道题我一开始想的是,倒数第n个节点,链表不方便往前找,那就从链表头结点开始找链表长度减n,这时候就是…...
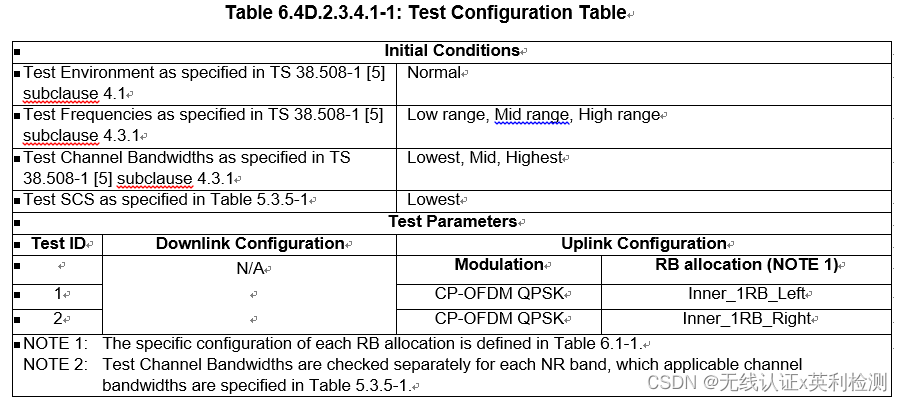
一起来学5G终端射频标准(In-band emissions-2)
上一篇我们列出了IBE的测试要求表格,今天我们详细说一下IBE如何测量计算,以及CA/NR-DC/SUL/UL-MIMO/V2X/Tx Diversity模式下的IBE情况。01—IBE如何测量和计算IBE的测试是对落入到未被分配的RB的干扰的测量,为12个子载波的平均发射功率&#…...
硬刚ChatGPT,中国版ChatGPT“狂飙”的机会在哪儿?
整体来讲,个人的态度是积极的。 ChatGPT、文心一言 都是在多重因素及大量 AI 模型/数据 长时间累积的成果,不是一蹴而就,立竿见影的功能产品。两者产生的基础和背景均不相同,各有优劣,不存在强行对比的概念。 以下是 …...
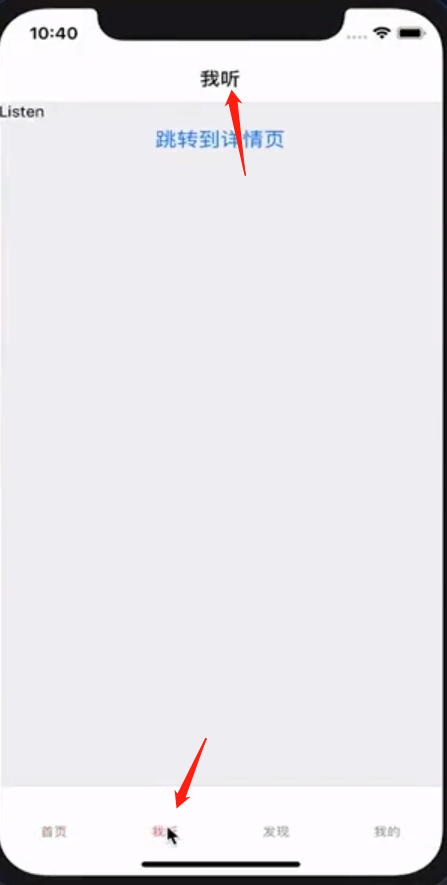
ReactNative——导航器createBottomTabNavigator(底部标签导航器篇)
上一篇有讲到堆栈式导航器的写法,点这里->堆栈式导航器标签导航器官网链接先安装依赖包yarn add react-navigation/bottom-tabs接着在src/navigator文件夹下新建BottomTabs.tsx文件,写法跟堆栈式导航器类似的~import React from react; import { NavigationConta…...
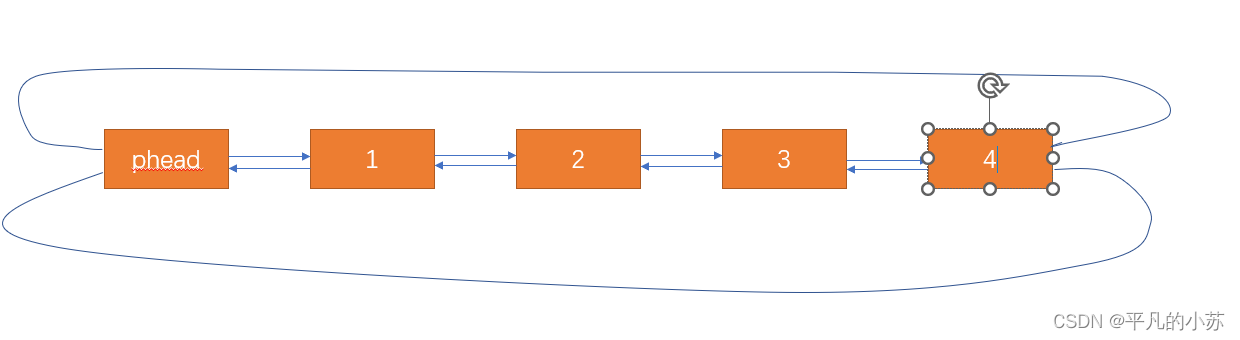
【数据结构】带头双向循环链表的实现
🌇个人主页:平凡的小苏 📚学习格言:别人可以拷贝我的模式,但不能拷贝我不断往前的激情 🛸C语言专栏:https://blog.csdn.net/vhhhbb/category_12174730.html 🚀数据结构专栏ÿ…...
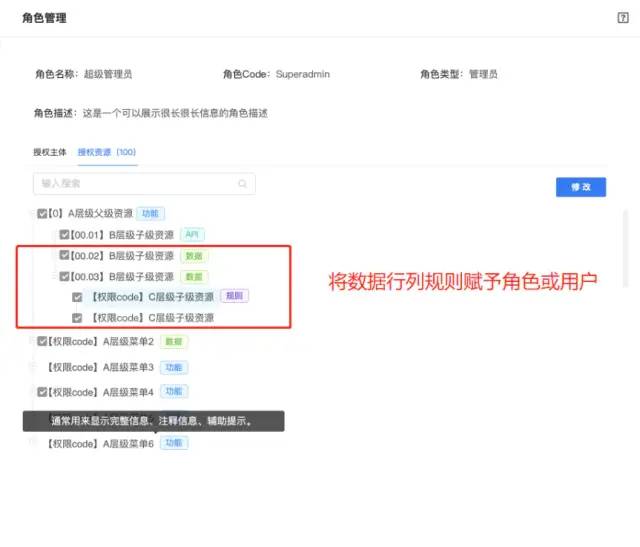
软件开发的权限系统功能模块设计,分享主流的九种常见权限模型
软件系统的权限控制几乎是非常常见且必备的,这篇文章整理下常见的九种模型,几乎基本够你用了,主流的权限模型主要有以下9种: 1、ACL模型 访问控制列表 2、DAC模型 自主访问控制 3、MAC模型 强制访问控制 4、ABAC模型 基于属性的访…...
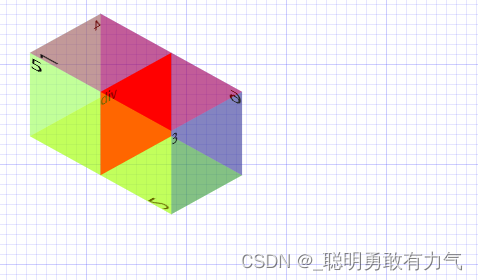
CSS3-数据可视化
2D动画 - transform CSS3 transform属性允许你旋转,缩放,倾斜或平移给定元素。 Transform是形变的意思(通常也叫变换),transformer就是变形金刚 常见的函数transform function有: 平移:transl…...

使用VSCode开发Django指南
使用VSCode开发Django指南 一、概述 Django 是一个高级 Python 框架,专为快速、安全和可扩展的 Web 开发而设计。Django 包含对 URL 路由、页面模板和数据处理的丰富支持。 本文将创建一个简单的 Django 应用,其中包含三个使用通用基本模板的页面。在此…...
解决本地部署 SmolVLM2 大语言模型运行 flash-attn 报错
出现的问题 安装 flash-attn 会一直卡在 build 那一步或者运行报错 解决办法 是因为你安装的 flash-attn 版本没有对应上,所以报错,到 https://github.com/Dao-AILab/flash-attention/releases 下载对应版本,cu、torch、cp 的版本一定要对…...
LLM基础1_语言模型如何处理文本
基于GitHub项目:https://github.com/datawhalechina/llms-from-scratch-cn 工具介绍 tiktoken:OpenAI开发的专业"分词器" torch:Facebook开发的强力计算引擎,相当于超级计算器 理解词嵌入:给词语画"…...
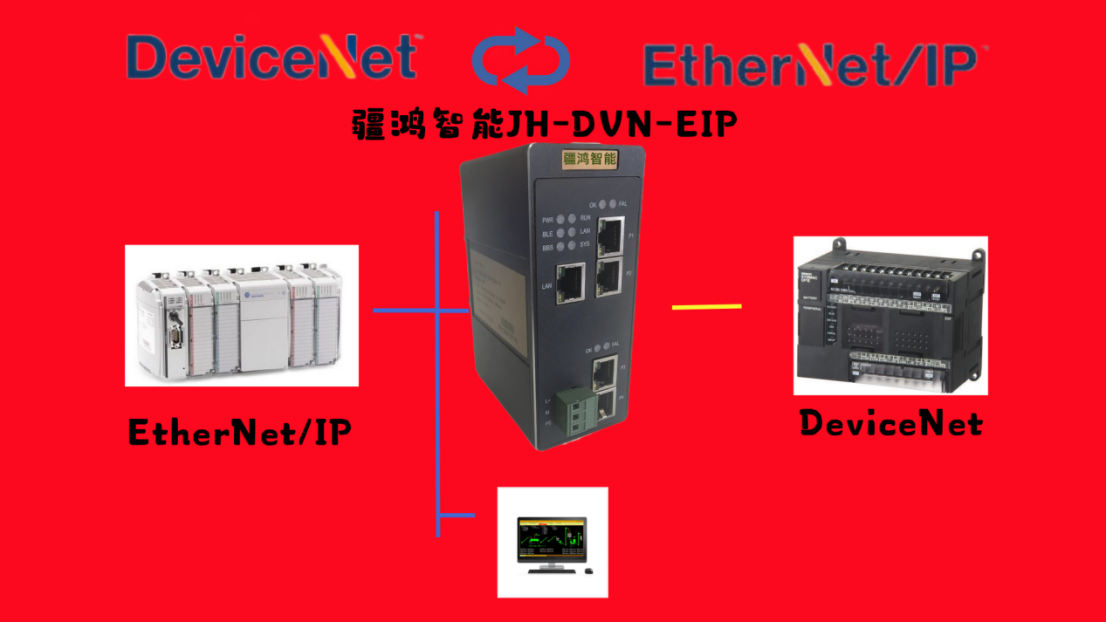
EtherNet/IP转DeviceNet协议网关详解
一,设备主要功能 疆鸿智能JH-DVN-EIP本产品是自主研发的一款EtherNet/IP从站功能的通讯网关。该产品主要功能是连接DeviceNet总线和EtherNet/IP网络,本网关连接到EtherNet/IP总线中做为从站使用,连接到DeviceNet总线中做为从站使用。 在自动…...
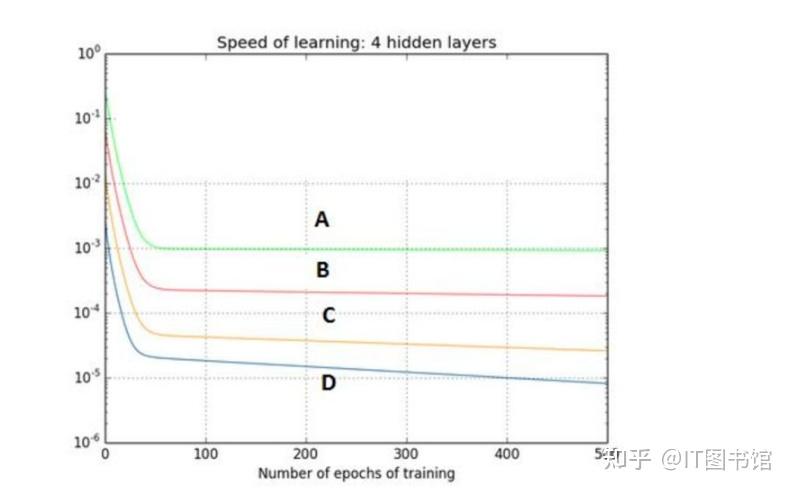
深度学习习题2
1.如果增加神经网络的宽度,精确度会增加到一个特定阈值后,便开始降低。造成这一现象的可能原因是什么? A、即使增加卷积核的数量,只有少部分的核会被用作预测 B、当卷积核数量增加时,神经网络的预测能力会降低 C、当卷…...
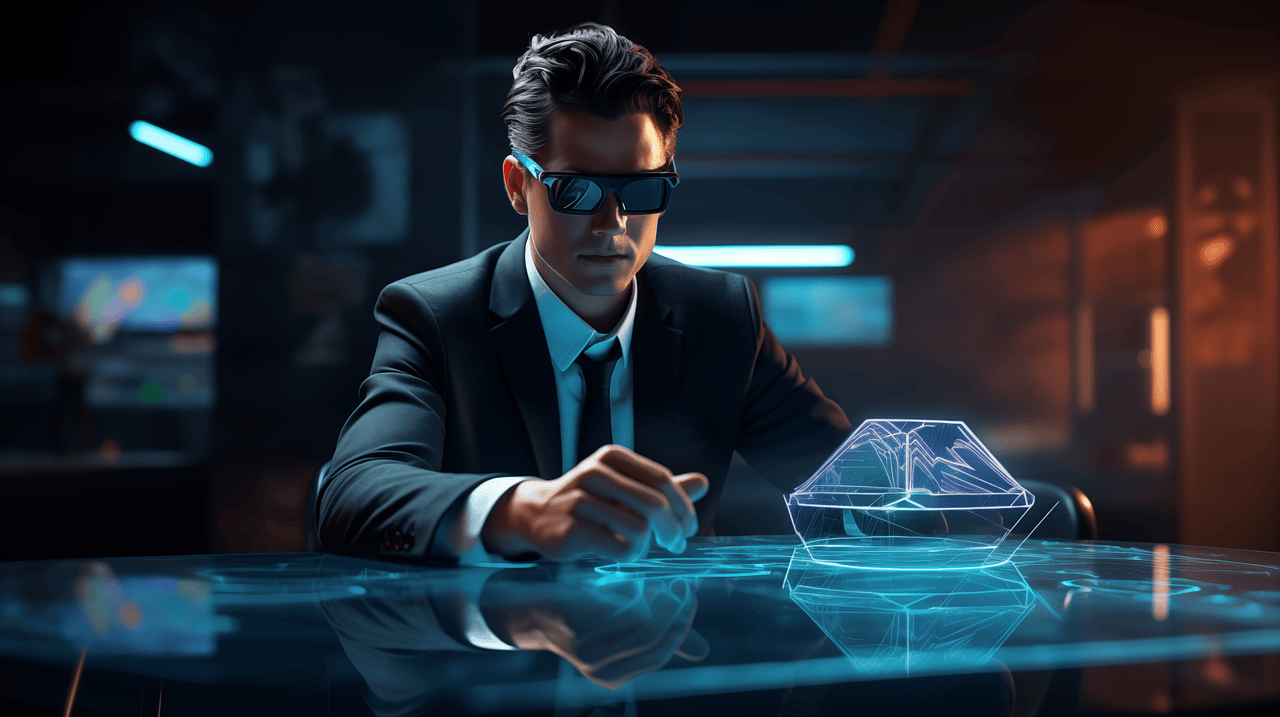
什么是VR全景技术
VR全景技术,全称为虚拟现实全景技术,是通过计算机图像模拟生成三维空间中的虚拟世界,使用户能够在该虚拟世界中进行全方位、无死角的观察和交互的技术。VR全景技术模拟人在真实空间中的视觉体验,结合图文、3D、音视频等多媒体元素…...
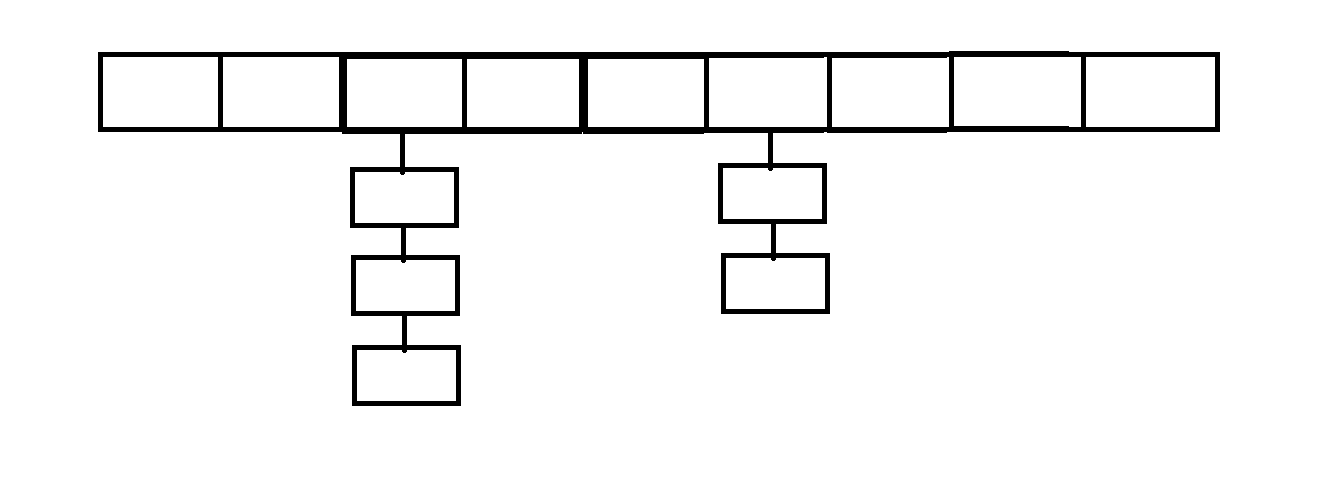
C++_哈希表
本篇文章是对C学习的哈希表部分的学习分享 相信一定会对你有所帮助~ 那咱们废话不多说,直接开始吧! 一、基础概念 1. 哈希核心思想: 哈希函数的作用:通过此函数建立一个Key与存储位置之间的映射关系。理想目标:实现…...
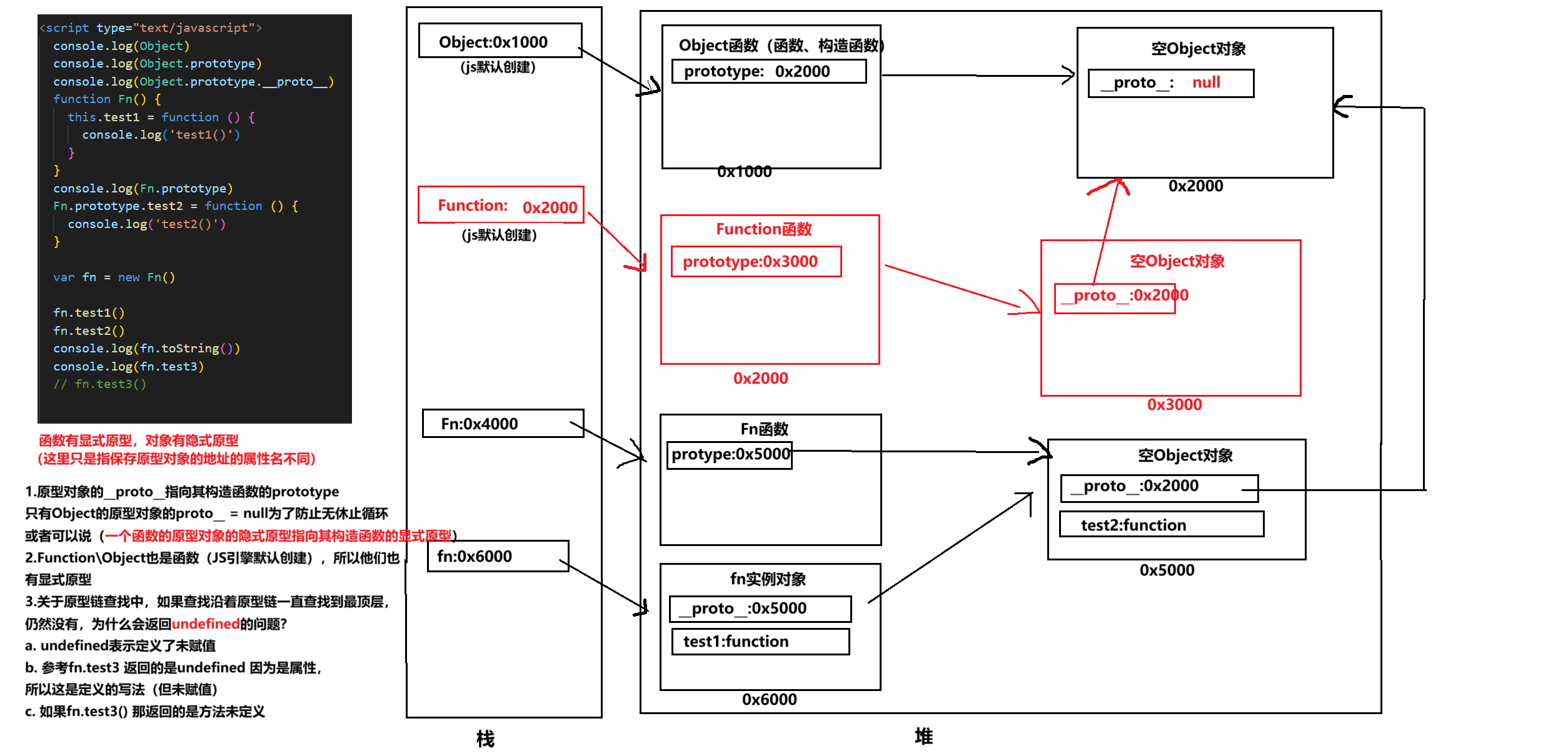
图解JavaScript原型:原型链及其分析 | JavaScript图解
忽略该图的细节(如内存地址值没有用二进制) 以下是对该图进一步的理解和总结 1. JS 对象概念的辨析 对象是什么:保存在堆中一块区域,同时在栈中有一块区域保存其在堆中的地址(也就是我们通常说的该变量指向谁&…...
数据库——redis
一、Redis 介绍 1. 概述 Redis(Remote Dictionary Server)是一个开源的、高性能的内存键值数据库系统,具有以下核心特点: 内存存储架构:数据主要存储在内存中,提供微秒级的读写响应 多数据结构支持&…...
Python的__call__ 方法
在 Python 中,__call__ 是一个特殊的魔术方法(magic method),它允许一个类的实例像函数一样被调用。当你在一个对象后面加上 () 并执行时(例如 obj()),Python 会自动调用该对象的 __call__ 方法…...