特征检测之HOG特征算法详解及Opencv接口使用
1. HOG特征简介
特征描述符是图像或图像补丁的表示形式,它通过提取有用信息并丢弃无关信息来简化图像。
- 通常,特征描述符将大小
W x H x 3
(通道)的图像转换为长度为n
的特征向量/数组。对于 HOG 特征描述符,输入图像的大小为64 x 128 x 3
,输出特征向量的长度为3780
。 - 在HOG特征描述符中,梯度方向的分布(直方图)被用作特征。图像的渐变(x和y导数)很有用,因为边缘和角落(强度突然变化的区域)周围的梯度大小很大,我们知道边缘和角落比平面区域包含更多关于物体形状的信息。
- HOG(Histogram of Oriented Gridients的简写)特征检测算法,最早是由法国研究员Dalal等在CVPR-2005上提出来的,一种解决人体目标检测的图像描述子,是一种用于表征图像局部梯度方向和梯度强度分布特性的描述符。其主要思想是:在边缘具体位置未知的情况下,边缘方向的分布也可以很好的表示行人目标的外形轮廓。
- HOG特征检测算法的几个步骤:
图像预处理—>梯度计算—>梯度方向直方图—>重叠块直方图归一化—>HOG特征
。下面分别对其进行介绍。
2. HOG算法实现
2.1 图像预处理
-
图像缩放
用于行人检测的 HOG 特征描述符是在图像的 64×128 个尺寸上计算的。当然,图像可以是任何大小。通常,在许多图像位置分析多个尺度的斑块。唯一的限制是正在分析的修补程序具有固定的纵横比。在我们的例子中,补丁需要具有 1:2 的纵横比。例如,它们可以是 100×200、128×256 或 1000×2000,但不能是 101×205。
-
灰度化
对于彩色图像,可以将将RGB分量转化成灰度图像,其转化公式为:
-
伽马校正
在图像照度不均匀的情况下,可以通过Gamma校正,将图像整体亮度提高或降低。在实际中可以采用两种不同的方式进行Gamma标准化,平方根、对数法。这里我们采用平方根的办法,公式如下(其中γ=0.5
):
2.2 计算梯度图像
- 注:下边步骤省略灰度化、伽马变化过程,以行人的彩色图像为例计算HOG特征
要计算 HOG 描述符,我们需要首先计算水平和垂直梯度;毕竟,我们要计算梯度的直方图。这可以通过使用以下内核过滤图像轻松实现。
我们也可以通过在内核大小为 1 的 OpenCV 中使用 Sobel 运算符来实现相同的结果:
// C++ gradient calculation.
// Read image
Mat img = imread("bolt.png");
img.convertTo(img, CV_32F, 1/255.0);// Calculate gradients gx, gy
Mat gx, gy;
Sobel(img, gx, CV_32F, 1, 0, 1);
Sobel(img, gy, CV_32F, 0, 1, 1);
# Python gradient calculation # Read image
im = cv2.imread('bolt.png')
im = np.float32(im) / 255.0# Calculate gradient
gx = cv2.Sobel(img, cv2.CV_32F, 1, 0, ksize=1)
gy = cv2.Sobel(img, cv2.CV_32F, 0, 1, ksize=1)
接下来,我们可以使用以下公式找到梯度的大小和方向:
如果您使用的是OpenCV,则可以使用函数cartToPolar完成计算,如下所示:
// C++ Calculate gradient magnitude and direction (in degrees)
Mat mag, angle;
cartToPolar(gx, gy, mag, angle, 1);
Python代码如下:
# Python Calculate gradient magnitude and direction ( in degrees )
mag, angle = cv2.cartToPolar(gx, gy, angleInDegrees=True)
下图显示了渐变:
在每个像素处,梯度都有一个大小和一个方向。对于彩色图像,将评估三个通道的梯度(如上图所示)。一个像素处的梯度大小是三个通道梯度大小的最大值,角度是最大梯度对应的角度。
2.3 计算8×8个单元格中的梯度直方图
- 为什么是8×8补丁?为什么不是 32×32 ?这是一个由我们正在寻找的功能规模决定的设计选择。HOG最初用于行人检测。在一张比例为8×8的行人照片中,64×128个单元格足够大,可以捕捉有趣的特征(例如面部,头顶等)。
- 下一步是在这 8×8 个单元格中创建梯度直方图。直方图包含 9 个箱,对应于角度 0、20、40 …160. 下图说明了该过程。我们正在研究与上图相同的 8×8 补丁的梯度的大小和方向。
- 根据方向选择箱,并根据大小选择投票(进入箱的值)。让我们首先关注用蓝色包围的像素。它的角度(方向)为80度,星等为2。因此,它将 2 添加到第 5 个箱中。使用红色包围的像素处的梯度角度为 10 度,星等为 4。由于 10 度介于 0 和 20 之间,因此像素的投票将均匀地分成两个箱。
- 还有一个细节需要注意。如果角度大于 160 度,则介于 160 和 180 之间,我们知道角度环绕使 0 和 180 等效。因此,在下面的示例中,角度为 165 度的像素按比例贡献 0 度箱和 160 度箱。
- 将 8×8 个单元格中所有像素的贡献相加以创建 9 箱直方图。对于上面的尺寸,它看起来像这样:
在我们的表示中,y 轴为 0 度。您可以看到直方图在 0 度和 180 度附近有很多权重,这只是另一种说法,在色块中梯度指向向上或向下。
2.4 16×16 块规范化
-
理想情况下,我们希望描述符独立于光照变化。换句话说,我们希望对直方图进行“归一化”,以便它们不受光照变化的影响。
-
如上图所示,一个 16×16 块有 4 个直方图,可以连接起来形成一个 36 x 1 元素向量,并且可以像 3×1 向量归一化一样进行归一化。然后窗口移动8个像素(参见动画),并在此窗口上计算归一化的36×1向量并重复该过程。其中,归一化实现为:分别对每个block进行标准化,一个block内有4个cell,每个cell含9维特征向量,故每个block就由4x9=36维特征向量来表征。
2.5 HOG特征
-
HOG特征即计算定向梯度特征向量的直方图
-
为了计算整个图像块的最终特征向量,将 36×1 个向量连接成一个巨大的向量。这个向量的大小是多少?让我们计算一下:
- 16×16 个区块有多少个仓位?有 7 个水平位置和 15 个垂直位置,总共 7 x 15 = 105 个位置。
- 每个 16×16 块由 36×1 向量表示。因此,当我们将它们全部连接成一个增益向量时,我们得到一个 36×105 = 3780 维向量。
-
计算结果可视化如下:
3. Opencv中HOG特征使用
- Opencv官方API参考:https://docs.opencv.org/4.x/d5/d33/structcv_1_1HOGDescriptor.html
- 附一个Opencv官方使用教程
#include "opencv2/imgproc.hpp"
#include "opencv2/highgui.hpp"
#include "opencv2/ml.hpp"
#include "opencv2/objdetect.hpp"
#include "opencv2/videoio.hpp"
#include <iostream>
#include <time.h>
using namespace cv;
using namespace cv::ml;
using namespace std;
vector< float > get_svm_detector( const Ptr< SVM >& svm );
void convert_to_ml( const std::vector< Mat > & train_samples, Mat& trainData );
void load_images( const String & dirname, vector< Mat > & img_lst, bool showImages );
void sample_neg( const vector< Mat > & full_neg_lst, vector< Mat > & neg_lst, const Size & size );
void computeHOGs( const Size wsize, const vector< Mat > & img_lst, vector< Mat > & gradient_lst, bool use_flip );
void test_trained_detector( String obj_det_filename, String test_dir, String videofilename );
vector< float > get_svm_detector( const Ptr< SVM >& svm )
{// get the support vectorsMat sv = svm->getSupportVectors();const int sv_total = sv.rows;// get the decision functionMat alpha, svidx;double rho = svm->getDecisionFunction( 0, alpha, svidx );CV_Assert( alpha.total() == 1 && svidx.total() == 1 && sv_total == 1 );CV_Assert( (alpha.type() == CV_64F && alpha.at<double>(0) == 1.) ||(alpha.type() == CV_32F && alpha.at<float>(0) == 1.f) );CV_Assert( sv.type() == CV_32F );vector< float > hog_detector( sv.cols + 1 );memcpy( &hog_detector[0], sv.ptr(), sv.cols*sizeof( hog_detector[0] ) );hog_detector[sv.cols] = (float)-rho;return hog_detector;
}
/*
* Convert training/testing set to be used by OpenCV Machine Learning algorithms.
* TrainData is a matrix of size (#samples x max(#cols,#rows) per samples), in 32FC1.
* Transposition of samples are made if needed.
*/
void convert_to_ml( const vector< Mat > & train_samples, Mat& trainData )
{//--Convert dataconst int rows = (int)train_samples.size();const int cols = (int)std::max( train_samples[0].cols, train_samples[0].rows );Mat tmp( 1, cols, CV_32FC1 ); //< used for transposition if neededtrainData = Mat( rows, cols, CV_32FC1 );for( size_t i = 0 ; i < train_samples.size(); ++i ){CV_Assert( train_samples[i].cols == 1 || train_samples[i].rows == 1 );if( train_samples[i].cols == 1 ){transpose( train_samples[i], tmp );tmp.copyTo( trainData.row( (int)i ) );}else if( train_samples[i].rows == 1 ){train_samples[i].copyTo( trainData.row( (int)i ) );}}
}
void load_images( const String & dirname, vector< Mat > & img_lst, bool showImages = false )
{vector< String > files;glob( dirname, files );for ( size_t i = 0; i < files.size(); ++i ){Mat img = imread( files[i] ); // load the imageif ( img.empty() ){cout << files[i] << " is invalid!" << endl; // invalid image, skip it.continue;}if ( showImages ){imshow( "image", img );waitKey( 1 );}img_lst.push_back( img );}
}
void sample_neg( const vector< Mat > & full_neg_lst, vector< Mat > & neg_lst, const Size & size )
{Rect box;box.width = size.width;box.height = size.height;srand( (unsigned int)time( NULL ) );for ( size_t i = 0; i < full_neg_lst.size(); i++ )if ( full_neg_lst[i].cols > box.width && full_neg_lst[i].rows > box.height ){box.x = rand() % ( full_neg_lst[i].cols - box.width );box.y = rand() % ( full_neg_lst[i].rows - box.height );Mat roi = full_neg_lst[i]( box );neg_lst.push_back( roi.clone() );}
}
void computeHOGs( const Size wsize, const vector< Mat > & img_lst, vector< Mat > & gradient_lst, bool use_flip )
{HOGDescriptor hog;hog.winSize = wsize;Mat gray;vector< float > descriptors;for( size_t i = 0 ; i < img_lst.size(); i++ ){if ( img_lst[i].cols >= wsize.width && img_lst[i].rows >= wsize.height ){Rect r = Rect(( img_lst[i].cols - wsize.width ) / 2,( img_lst[i].rows - wsize.height ) / 2,wsize.width,wsize.height);cvtColor( img_lst[i](r), gray, COLOR_BGR2GRAY );hog.compute( gray, descriptors, Size( 8, 8 ), Size( 0, 0 ) );gradient_lst.push_back( Mat( descriptors ).clone() );if ( use_flip ){flip( gray, gray, 1 );hog.compute( gray, descriptors, Size( 8, 8 ), Size( 0, 0 ) );gradient_lst.push_back( Mat( descriptors ).clone() );}}}
}
void test_trained_detector( String obj_det_filename, String test_dir, String videofilename )
{cout << "Testing trained detector..." << endl;HOGDescriptor hog;hog.load( obj_det_filename );vector< String > files;glob( test_dir, files );int delay = 0;VideoCapture cap;if ( videofilename != "" ){if ( videofilename.size() == 1 && isdigit( videofilename[0] ) )cap.open( videofilename[0] - '0' );elsecap.open( videofilename );}obj_det_filename = "testing " + obj_det_filename;namedWindow( obj_det_filename, WINDOW_NORMAL );for( size_t i=0;; i++ ){Mat img;if ( cap.isOpened() ){cap >> img;delay = 1;}else if( i < files.size() ){img = imread( files[i] );}if ( img.empty() ){return;}vector< Rect > detections;vector< double > foundWeights;hog.detectMultiScale( img, detections, foundWeights );for ( size_t j = 0; j < detections.size(); j++ ){Scalar color = Scalar( 0, foundWeights[j] * foundWeights[j] * 200, 0 );rectangle( img, detections[j], color, img.cols / 400 + 1 );}imshow( obj_det_filename, img );if( waitKey( delay ) == 27 ){return;}}
}
int main( int argc, char** argv )
{const char* keys ={"{help h| | show help message}""{pd | | path of directory contains positive images}""{nd | | path of directory contains negative images}""{td | | path of directory contains test images}""{tv | | test video file name}""{dw | | width of the detector}""{dh | | height of the detector}""{f |false| indicates if the program will generate and use mirrored samples or not}""{d |false| train twice}""{t |false| test a trained detector}""{v |false| visualize training steps}""{fn |my_detector.yml| file name of trained SVM}"};CommandLineParser parser( argc, argv, keys );if ( parser.has( "help" ) ){parser.printMessage();exit( 0 );}String pos_dir = parser.get< String >( "pd" );String neg_dir = parser.get< String >( "nd" );String test_dir = parser.get< String >( "td" );String obj_det_filename = parser.get< String >( "fn" );String videofilename = parser.get< String >( "tv" );int detector_width = parser.get< int >( "dw" );int detector_height = parser.get< int >( "dh" );bool test_detector = parser.get< bool >( "t" );bool train_twice = parser.get< bool >( "d" );bool visualization = parser.get< bool >( "v" );bool flip_samples = parser.get< bool >( "f" );if ( test_detector ){test_trained_detector( obj_det_filename, test_dir, videofilename );exit( 0 );}if( pos_dir.empty() || neg_dir.empty() ){parser.printMessage();cout << "Wrong number of parameters.\n\n"<< "Example command line:\n" << argv[0] << " -dw=64 -dh=128 -pd=/INRIAPerson/96X160H96/Train/pos -nd=/INRIAPerson/neg -td=/INRIAPerson/Test/pos -fn=HOGpedestrian64x128.xml -d\n"<< "\nExample command line for testing trained detector:\n" << argv[0] << " -t -fn=HOGpedestrian64x128.xml -td=/INRIAPerson/Test/pos";exit( 1 );}vector< Mat > pos_lst, full_neg_lst, neg_lst, gradient_lst;vector< int > labels;clog << "Positive images are being loaded..." ;load_images( pos_dir, pos_lst, visualization );if ( pos_lst.size() > 0 ){clog << "...[done] " << pos_lst.size() << " files." << endl;}else{clog << "no image in " << pos_dir <<endl;return 1;}Size pos_image_size = pos_lst[0].size();if ( detector_width && detector_height ){pos_image_size = Size( detector_width, detector_height );}else{for ( size_t i = 0; i < pos_lst.size(); ++i ){if( pos_lst[i].size() != pos_image_size ){cout << "All positive images should be same size!" << endl;exit( 1 );}}pos_image_size = pos_image_size / 8 * 8;}clog << "Negative images are being loaded...";load_images( neg_dir, full_neg_lst, visualization );clog << "...[done] " << full_neg_lst.size() << " files." << endl;clog << "Negative images are being processed...";sample_neg( full_neg_lst, neg_lst, pos_image_size );clog << "...[done] " << neg_lst.size() << " files." << endl;clog << "Histogram of Gradients are being calculated for positive images...";computeHOGs( pos_image_size, pos_lst, gradient_lst, flip_samples );size_t positive_count = gradient_lst.size();labels.assign( positive_count, +1 );clog << "...[done] ( positive images count : " << positive_count << " )" << endl;clog << "Histogram of Gradients are being calculated for negative images...";computeHOGs( pos_image_size, neg_lst, gradient_lst, flip_samples );size_t negative_count = gradient_lst.size() - positive_count;labels.insert( labels.end(), negative_count, -1 );CV_Assert( positive_count < labels.size() );clog << "...[done] ( negative images count : " << negative_count << " )" << endl;Mat train_data;convert_to_ml( gradient_lst, train_data );clog << "Training SVM...";Ptr< SVM > svm = SVM::create();/* Default values to train SVM */svm->setCoef0( 0.0 );svm->setDegree( 3 );svm->setTermCriteria( TermCriteria(TermCriteria::MAX_ITER + TermCriteria::EPS, 1000, 1e-3 ) );svm->setGamma( 0 );svm->setKernel( SVM::LINEAR );svm->setNu( 0.5 );svm->setP( 0.1 ); // for EPSILON_SVR, epsilon in loss function?svm->setC( 0.01 ); // From paper, soft classifiersvm->setType( SVM::EPS_SVR ); // C_SVC; // EPSILON_SVR; // may be also NU_SVR; // do regression tasksvm->train( train_data, ROW_SAMPLE, labels );clog << "...[done]" << endl;if ( train_twice ){clog << "Testing trained detector on negative images. This might take a few minutes...";HOGDescriptor my_hog;my_hog.winSize = pos_image_size;// Set the trained svm to my_hogmy_hog.setSVMDetector( get_svm_detector( svm ) );vector< Rect > detections;vector< double > foundWeights;for ( size_t i = 0; i < full_neg_lst.size(); i++ ){if ( full_neg_lst[i].cols >= pos_image_size.width && full_neg_lst[i].rows >= pos_image_size.height )my_hog.detectMultiScale( full_neg_lst[i], detections, foundWeights );elsedetections.clear();for ( size_t j = 0; j < detections.size(); j++ ){Mat detection = full_neg_lst[i]( detections[j] ).clone();resize( detection, detection, pos_image_size, 0, 0, INTER_LINEAR_EXACT);neg_lst.push_back( detection );}if ( visualization ){for ( size_t j = 0; j < detections.size(); j++ ){rectangle( full_neg_lst[i], detections[j], Scalar( 0, 255, 0 ), 2 );}imshow( "testing trained detector on negative images", full_neg_lst[i] );waitKey( 5 );}}clog << "...[done]" << endl;gradient_lst.clear();clog << "Histogram of Gradients are being calculated for positive images...";computeHOGs( pos_image_size, pos_lst, gradient_lst, flip_samples );positive_count = gradient_lst.size();clog << "...[done] ( positive count : " << positive_count << " )" << endl;clog << "Histogram of Gradients are being calculated for negative images...";computeHOGs( pos_image_size, neg_lst, gradient_lst, flip_samples );negative_count = gradient_lst.size() - positive_count;clog << "...[done] ( negative count : " << negative_count << " )" << endl;labels.clear();labels.assign(positive_count, +1);labels.insert(labels.end(), negative_count, -1);clog << "Training SVM again...";convert_to_ml( gradient_lst, train_data );svm->train( train_data, ROW_SAMPLE, labels );clog << "...[done]" << endl;}HOGDescriptor hog;hog.winSize = pos_image_size;hog.setSVMDetector( get_svm_detector( svm ) );hog.save( obj_det_filename );test_trained_detector( obj_det_filename, test_dir, videofilename );return 0;
}
参考
1.HOG:从理论到OpenCV实践
2.【特征检测】HOG特征算法
相关文章:
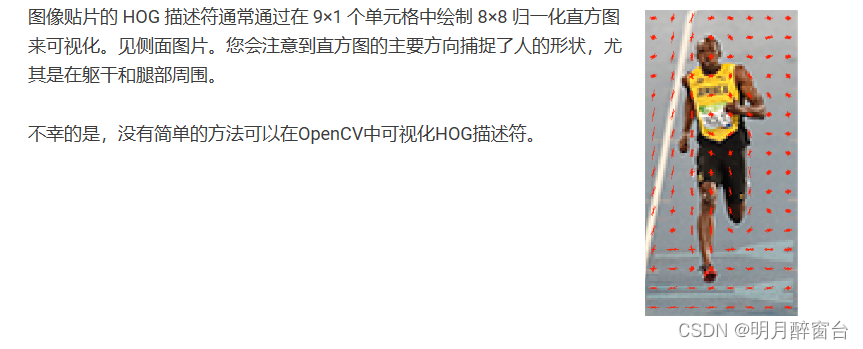
特征检测之HOG特征算法详解及Opencv接口使用
1. HOG特征简介 特征描述符是图像或图像补丁的表示形式,它通过提取有用信息并丢弃无关信息来简化图像。 通常,特征描述符将大小W x H x 3(通道)的图像转换为长度为n的特征向量/数组。对于 HOG 特征描述符,输入图像的…...
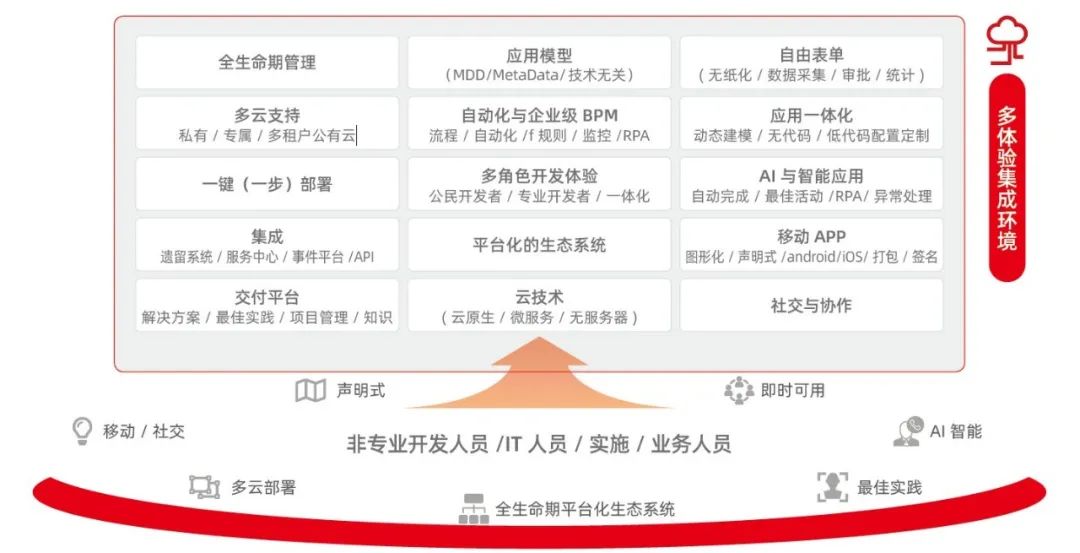
一款好的低代码开发平台应该是什么样?
一款好的低代码开发平台应该是什么样? 以企业级应用构建来讲,完成一个应用复杂度随着技术的进步、需求的细化、业务要求的变化并不是逐渐降低,而是逐渐提升。用户想要有更好的体验,复杂度更是成倍提升。 基于此,低代码…...
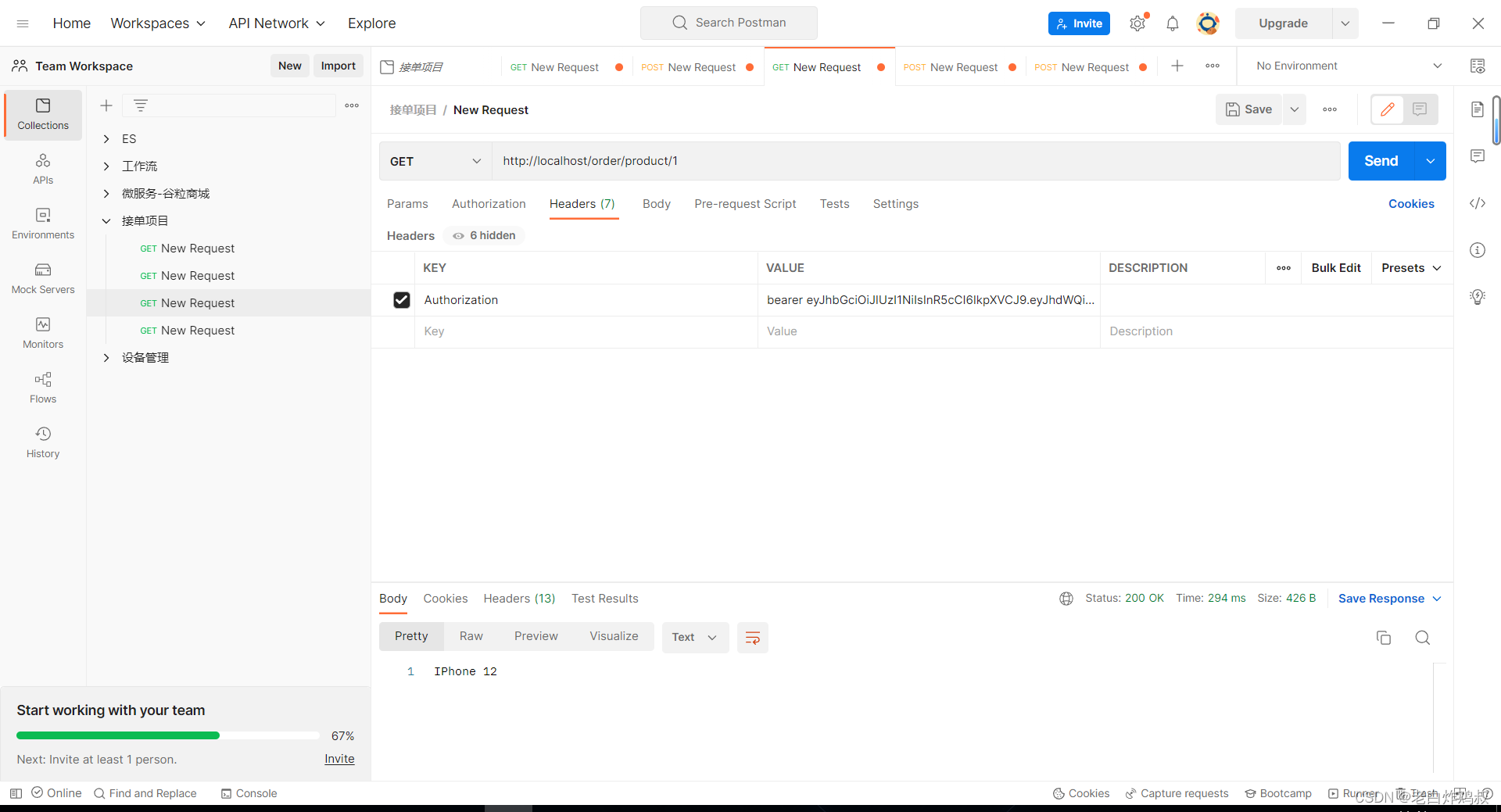
基于Spring cloud搭建oauth2
1,OAuth2.0简介 OAuth(开发授权)是一个开放标准,允许用户授权第三方应用访问他们存储在另外的服务提供者上的信息,而不需要将用户名和密码提供给第三方应用或分享他们数据的所有内容。 OAuth2.0是OAuth的延续…...
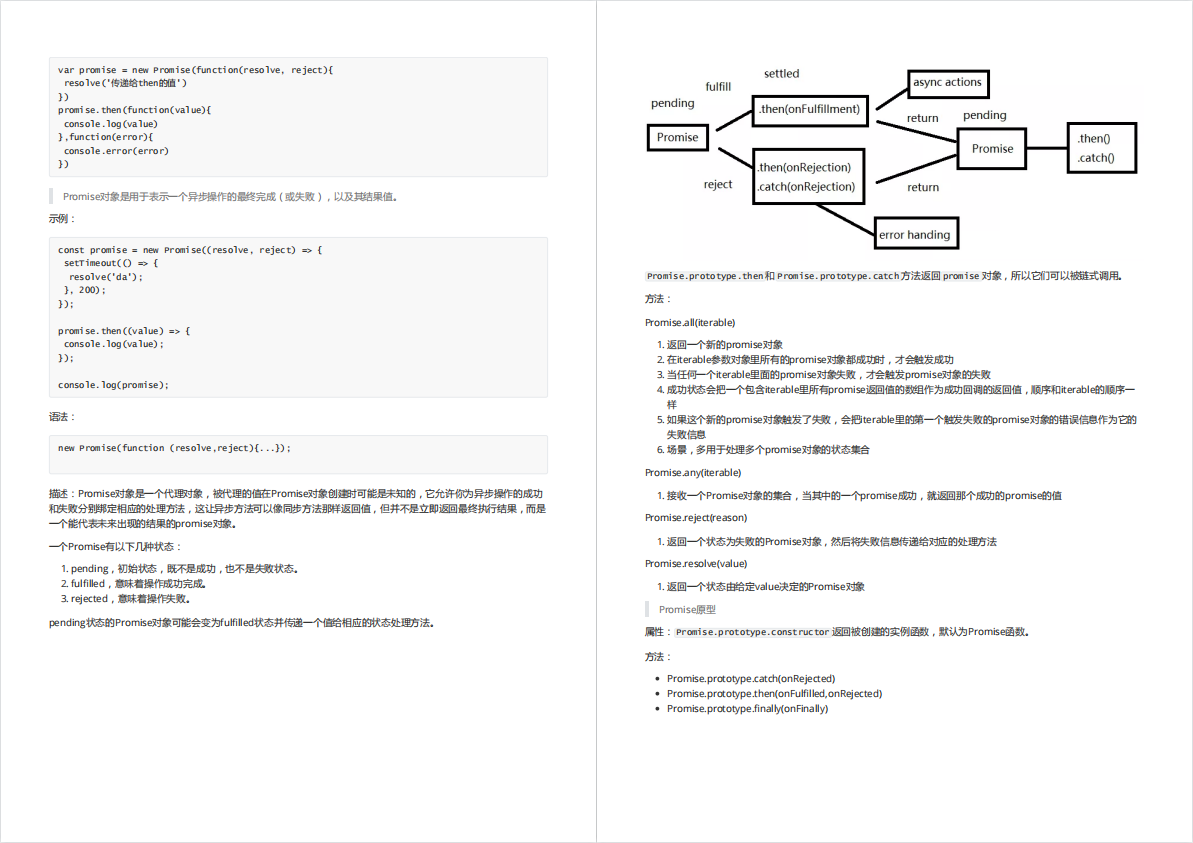
实现一个小程序分享图 wxml2canvas
我们经常会遇上动态生成海报的需求,而在小程序中,生成图片非Canvas莫属。但是在实际工作当中,为了追求效率,我们会不可避免地去使用一些JS插件,而 wxml-to-canvas 就是一款官方推荐且非常优秀的插件,它可以…...
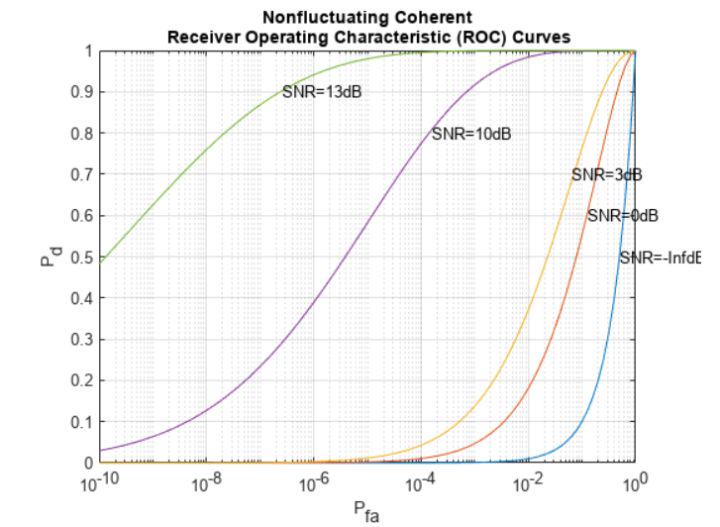
基于matlab设计x波段机载SAR系统
一、前言此示例说明如何设计在 X 波段工作的合成孔径雷达 (SAR) 传感器并计算传感器参数。SAR利用雷达天线在目标区域上的运动来提供目标区域的图像。当SAR平台在目标区域上空行进时,当脉冲从雷达天线发送和接收时,会产生合成孔径…...

WPF学习:Slider — 冒泡显示值
想做一个下图所示的Slider,以冒泡的方式显示其Value值,该怎么做呢? 功能要求,当鼠标放在滑块上的时候,冒“泡”显示值;当滑块移动的时候,“泡”跟随移动。 看似简单的功能,但要完美…...
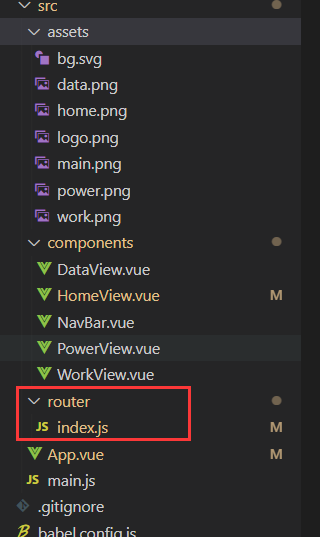
Vue实战第4章:主页设计之中部内容设计
前言 本篇在讲什么 接上篇文章,我们制作了一个自定义的网页导航栏,本篇文章我们简单制作一个内容页 仅介绍简单的应用,仅供参考 本篇适合什么 适合初学Vue的小白 适合想要自己搭建网站的新手 适合没有接触过vue-router的前端程序 本篇…...
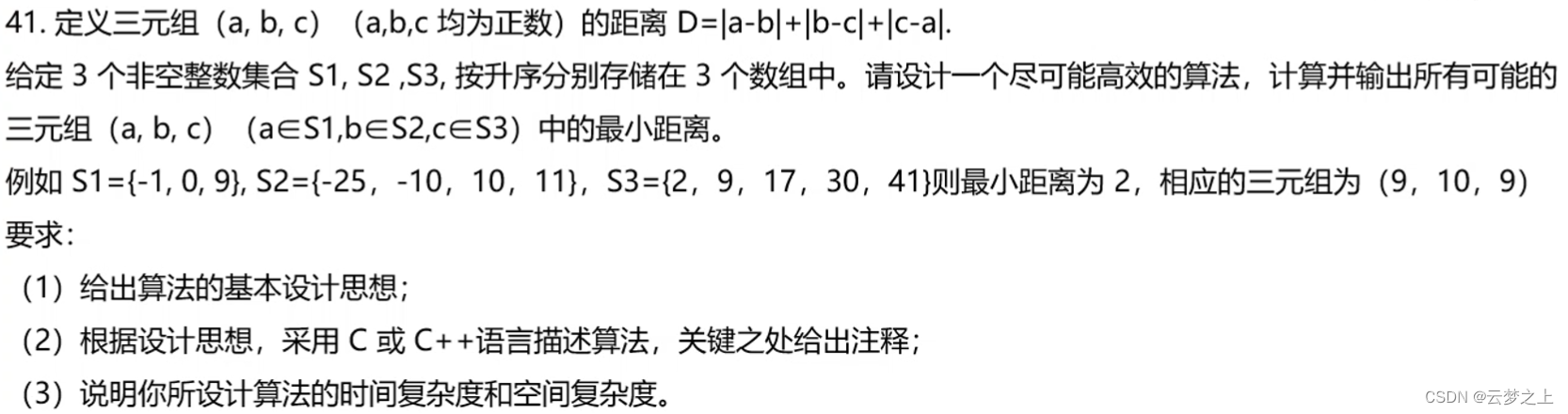
数据结构代码总结(C语言实现)
目录如何应对数据结构的代码题?采取的学习流程①首先对C语言的语法的熟悉②学习掌握基本代码的写法,做到熟练2.1插入排序2.2快速排序2.3二分查找2.4树的遍历③跟着网上视频开始熟悉对一些问题的解答④结合真题的代码,寻找其中的结题规律如何应…...
zookeeper 复习 ---- chapter04
zookeeper 复习 ---- chapter04zookeeper 的精髓是什么? 1:它有四个节点类型 持久无序的节点 临时无序的节点 持久有序的节点 临时有序的节点 临时的节点的特征:当客户端和服务器端断开连接,当前客户端创建的节点被服务器端自动删…...
thinkphp6.0连接MYSQL
目录8.连接多个数据库7.多级控制器不存在6.分页5.非法请求4.关于路由**3.初体验页面****2.加入fileheader添加注释****1.配置mysql0. 官方开发手册一些网址 http://127.0.0.1:8000/index 原桌面 http://127.0.0.1:8000/hello/fsh hello,fsh(index中hello方法&#x…...
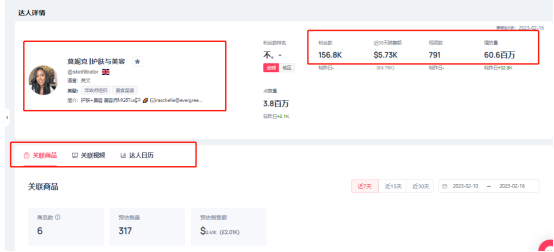
商家必读!超店有数分享,tiktok达人营销变现如何更快一步?
近几年来,“粉丝经济”发展越来越迅猛,“网红带货”已经成为了一种营销的方式。这种方式让商家能基于达人的影响下迅速抢占自己的私域流量池。消费者会基于对达人的信任,购买达人推荐的产品。达人效应可以助力品牌走出营销困境。如果商家想要…...
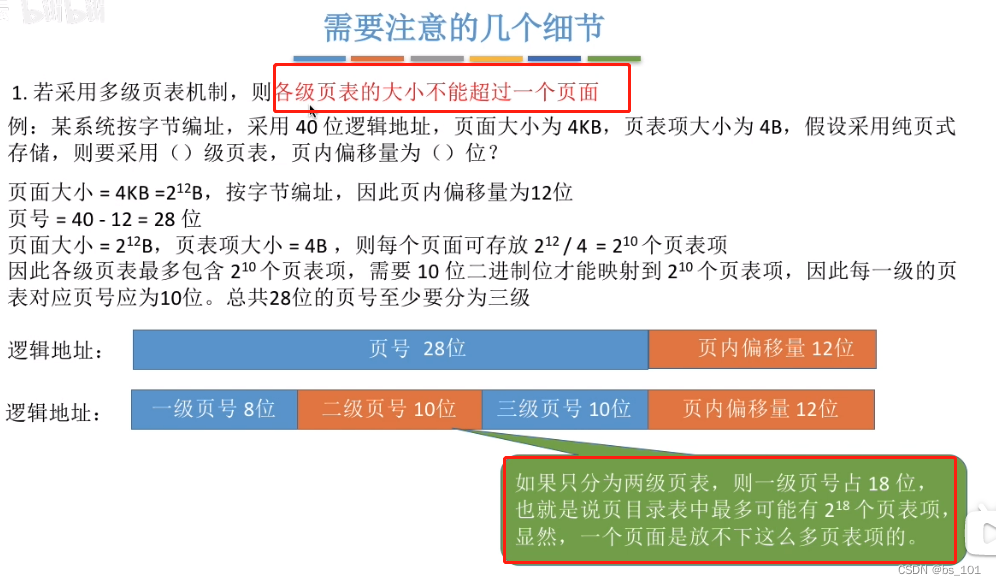
操作系统(day11)--快表,两级页表
具有快表的地址变换机构 时间局限性:会有大量连续的指令需要访问同一个内存块的数据的情况(程序中的循环) 空间局限性:一旦程序访问了某个存储单元,在不久之后,其附近的存储单元也很有可能被访问。…...
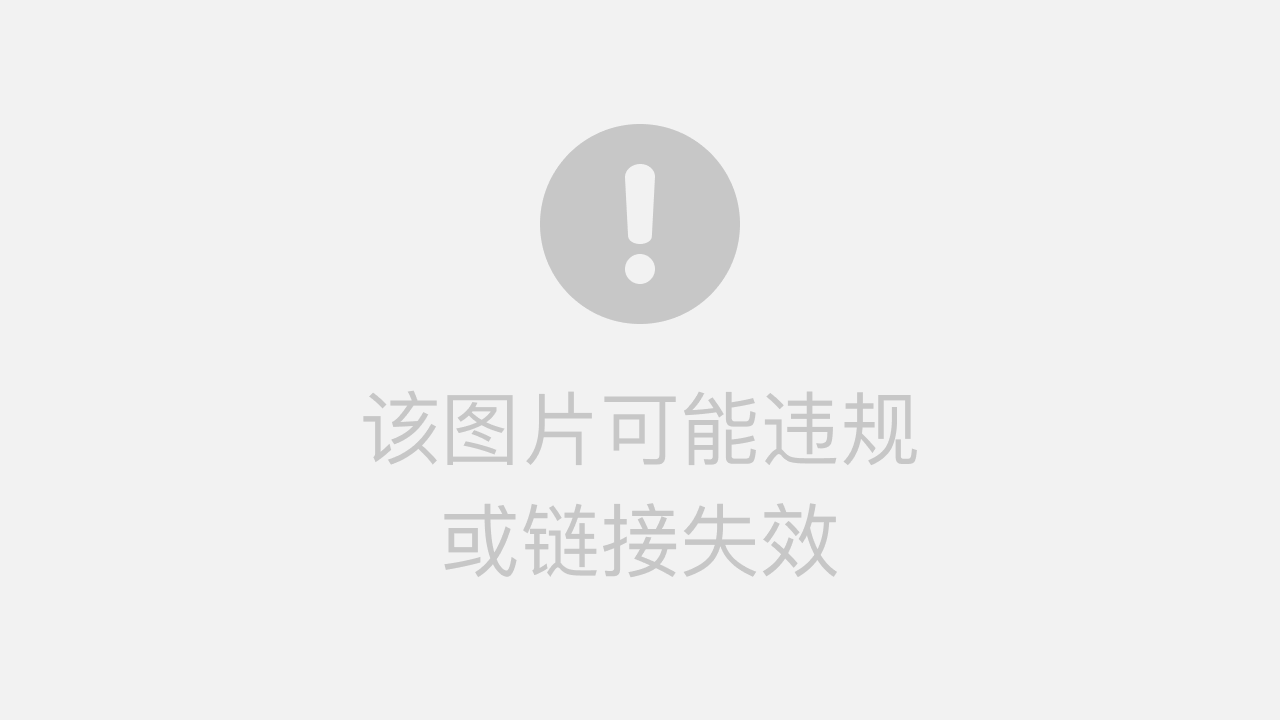
预告| 亮点抢先看!第四届OpenI/O启智开发者大会主论坛24日启幕!
2023年2月24日至25日,第四届OpenI/O启智开发者大会将在深圳隆重举行。“算网筑基、开源启智、AI赋能”作为今年大会的主题,吸引了全球业界关注的目光。大会集结中国算力网资源基座、开源社区治理及AI开源生态建设、国家级开放创新应用平台、NLP大模型等前…...
猪齿鱼(Choerodon UI )的通用提交的封装 —— 两种方案,A.使用dataSet的自身的submit,B.使用axios.post来提交
submit组件(otherSubmit/axiosSubmit) 一、背景与简介 1、首先我们申请表提交,分为【保存】提交与【其他】提交; 1.1【保存】提交,要求表单必须要有变更,DataToJSON默认为dirty(只转换变更的…...
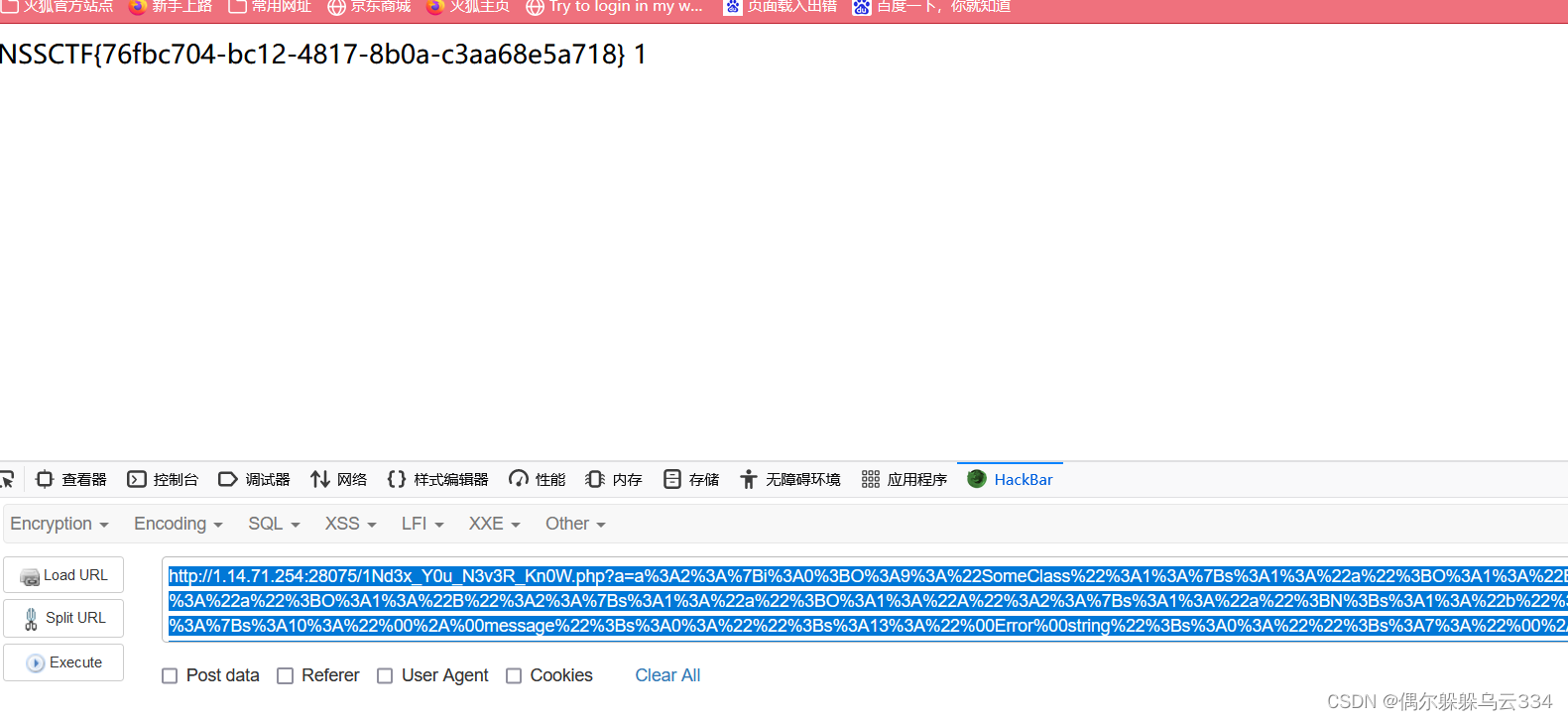
CISCN(Web Ezpentest)GC、序列化、case when
目录 REGEXP的一个点(正则) like(默认不区分大小写) 当禁用了空格 regexp,like的区分大小写的使用方法 [CISCN 2022 初赛]ezpentest 卡点 2022 HFCTF babysql 最近又学到了一道新知识,case when的错…...
OSG三维渲染引擎编程学习之五十七:“第六章:OSG场景工作机制” 之 “6.1 OSG访问器”
目录 第六章 OSG场景工作机制 6.1 OSG访问器 6.1.1 访问器模式 6.1.2 osg::NodeVisitor 6.1.3 访问器示例...
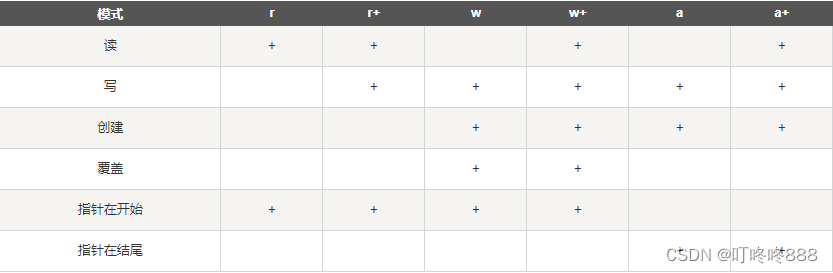
Python3 输入和输出实例及演示
在前面几个章节中,我们其实已经接触了 Python 的输入输出的功能。本章节我们将具体介绍 Python 的输入输出。 输出格式美化 Python两种输出值的方式: 表达式语句和 print() 函数。 第3种方式是使用文件对象的 write() 方法,标准输出文件可以用 sys.std…...
召回-回忆录(持续更新)
0.召回方法 词召回 swing、itemCF 缺点: 有冷启动问题不是全局召回,冷门活动难以得到召回结果容易召回过多的头部热门活动 向量召回 参考文献: 经典推荐算法学习(七)| Graph Embedding技术学习 | 从DeepWalk到No…...
1243. 糖果/状态压缩dp【AcWing】
1243. 糖果 糖果店的老板一共有 M种口味的糖果出售。 为了方便描述,我们将 M种口味编号 1∼M。 小明希望能品尝到所有口味的糖果。 遗憾的是老板并不单独出售糖果,而是 K颗一包整包出售。 幸好糖果包装上注明了其中 K颗糖果的口味,所以小…...
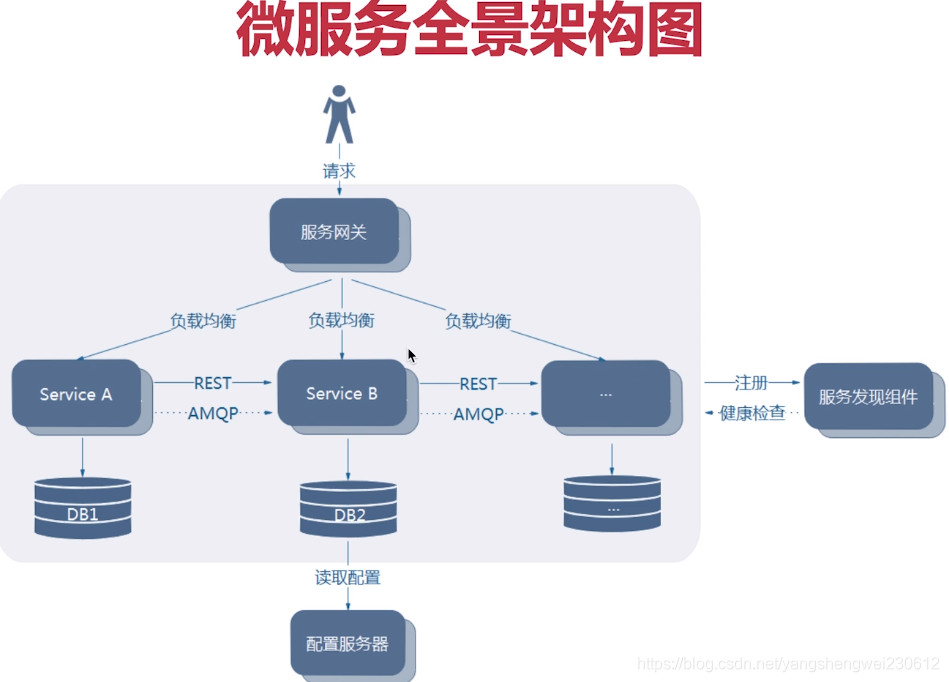
【Spring Cloud Alibaba】001-单体架构与微服务架构
【Spring Cloud Alibaba】001-单体架构与微服务 文章目录【Spring Cloud Alibaba】001-单体架构与微服务一、单体架构1、单体应用与单体架构2、单体应用架构图3、单体架构优缺点优点缺点二、微服务1、微服务的“定义”2、微服务的特性3、微服务架构图4、微服务的优缺点优点缺点…...
谷歌浏览器插件
项目中有时候会用到插件 sync-cookie-extension1.0.0:开发环境同步测试 cookie 至 localhost,便于本地请求服务携带 cookie 参考地址:https://juejin.cn/post/7139354571712757767 里面有源码下载下来,加在到扩展即可使用FeHelp…...
React Native 导航系统实战(React Navigation)
导航系统实战(React Navigation) React Navigation 是 React Native 应用中最常用的导航库之一,它提供了多种导航模式,如堆栈导航(Stack Navigator)、标签导航(Tab Navigator)和抽屉…...
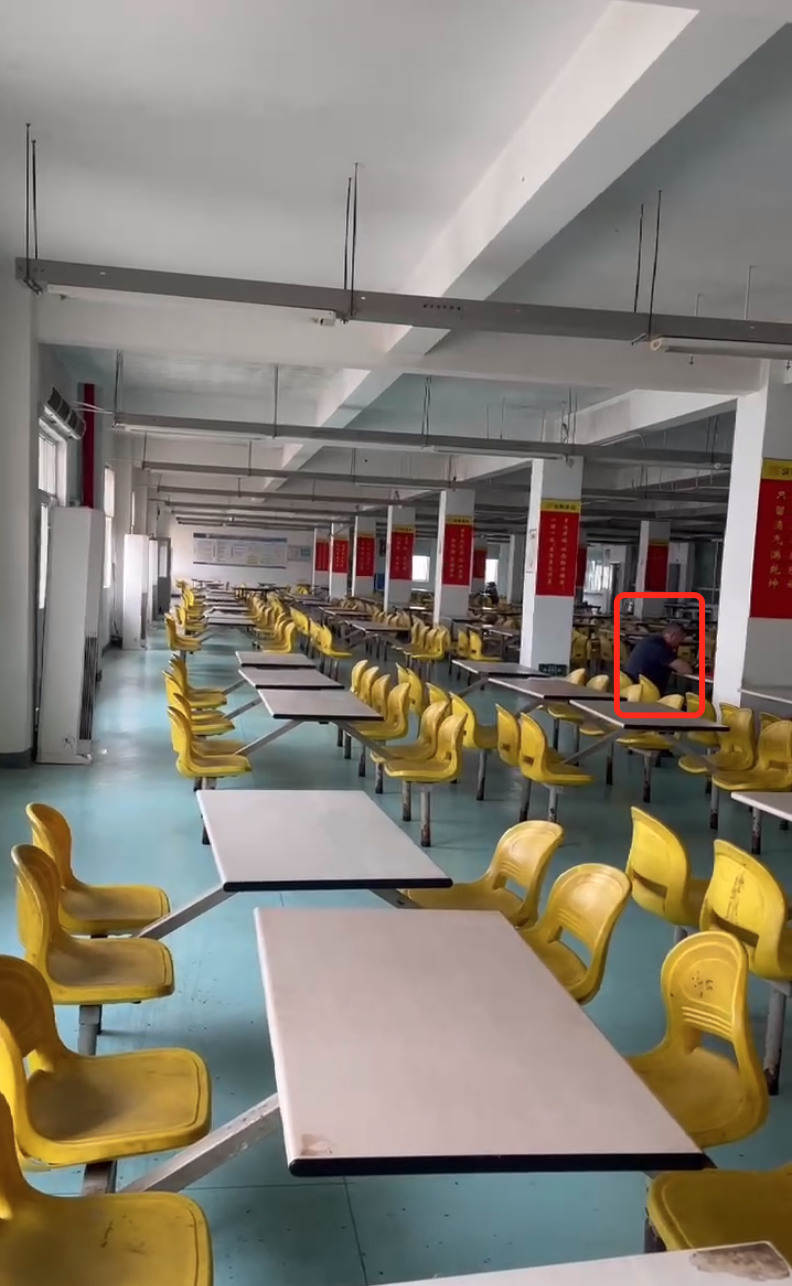
工业安全零事故的智能守护者:一体化AI智能安防平台
前言: 通过AI视觉技术,为船厂提供全面的安全监控解决方案,涵盖交通违规检测、起重机轨道安全、非法入侵检测、盗窃防范、安全规范执行监控等多个方面,能够实现对应负责人反馈机制,并最终实现数据的统计报表。提升船厂…...
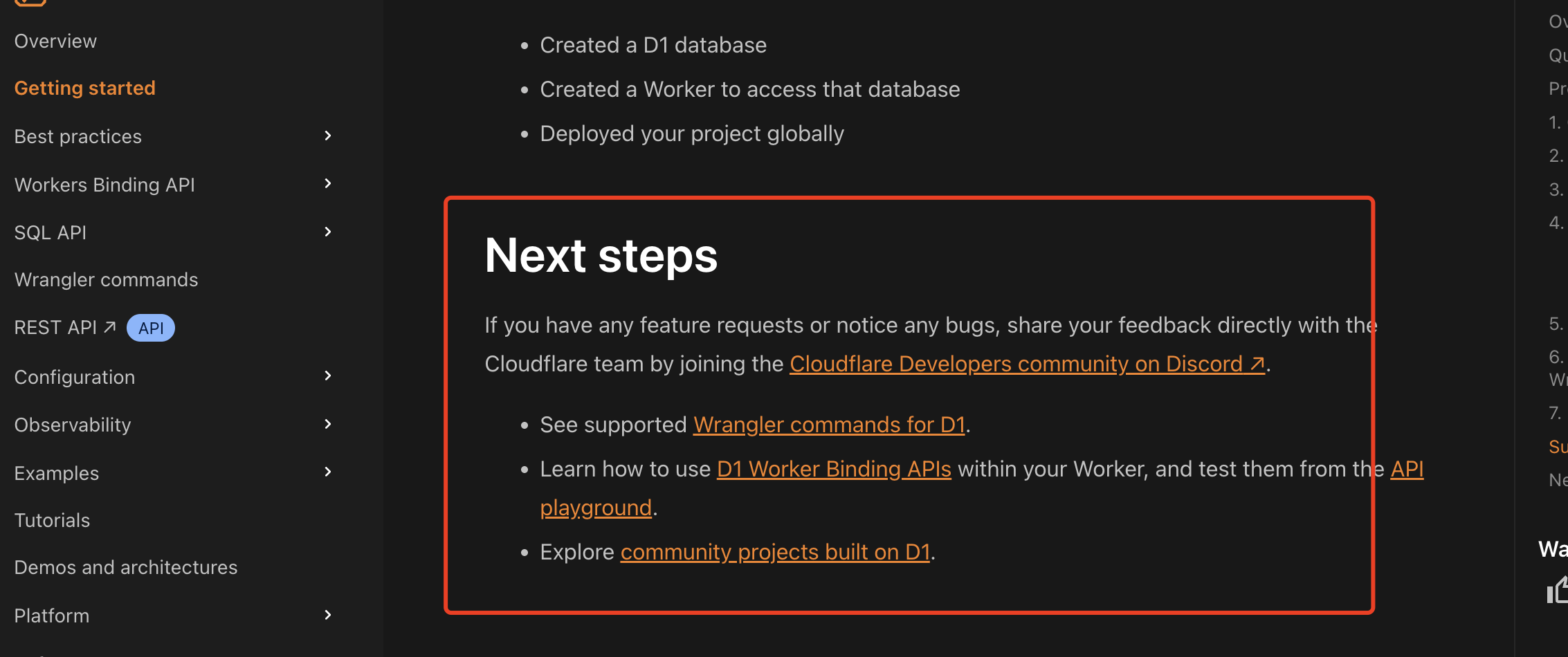
通过Wrangler CLI在worker中创建数据库和表
官方使用文档:Getting started Cloudflare D1 docs 创建数据库 在命令行中执行完成之后,会在本地和远程创建数据库: npx wranglerlatest d1 create prod-d1-tutorial 在cf中就可以看到数据库: 现在,您的Cloudfla…...
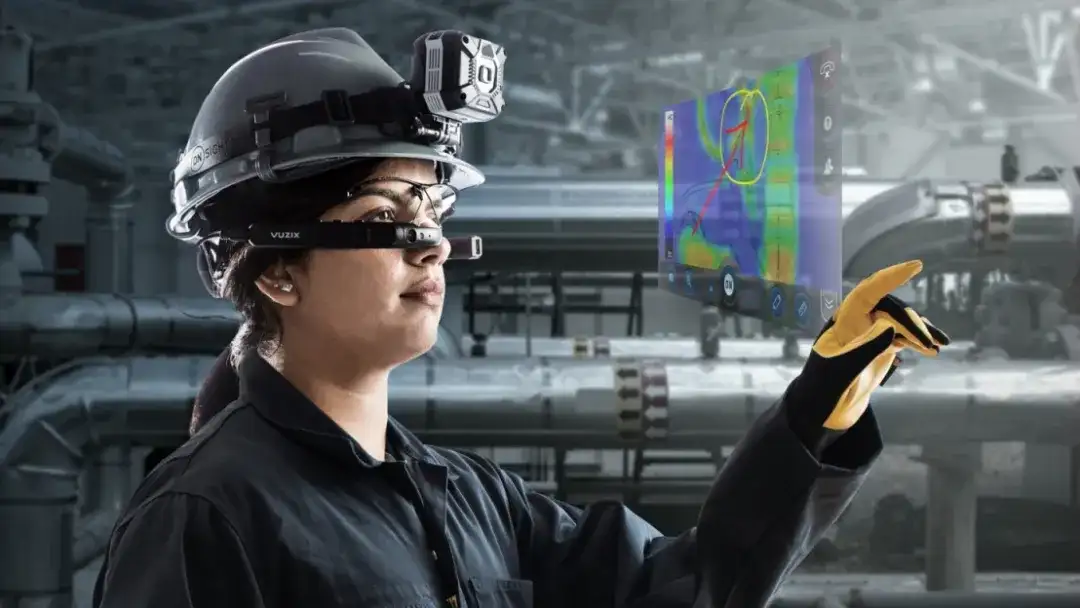
安宝特方案丨XRSOP人员作业标准化管理平台:AR智慧点检验收套件
在选煤厂、化工厂、钢铁厂等过程生产型企业,其生产设备的运行效率和非计划停机对工业制造效益有较大影响。 随着企业自动化和智能化建设的推进,需提前预防假检、错检、漏检,推动智慧生产运维系统数据的流动和现场赋能应用。同时,…...
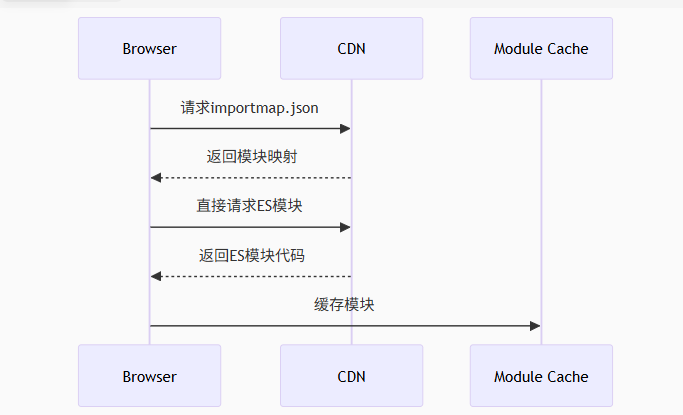
Module Federation 和 Native Federation 的比较
前言 Module Federation 是 Webpack 5 引入的微前端架构方案,允许不同独立构建的应用在运行时动态共享模块。 Native Federation 是 Angular 官方基于 Module Federation 理念实现的专为 Angular 优化的微前端方案。 概念解析 Module Federation (模块联邦) Modul…...
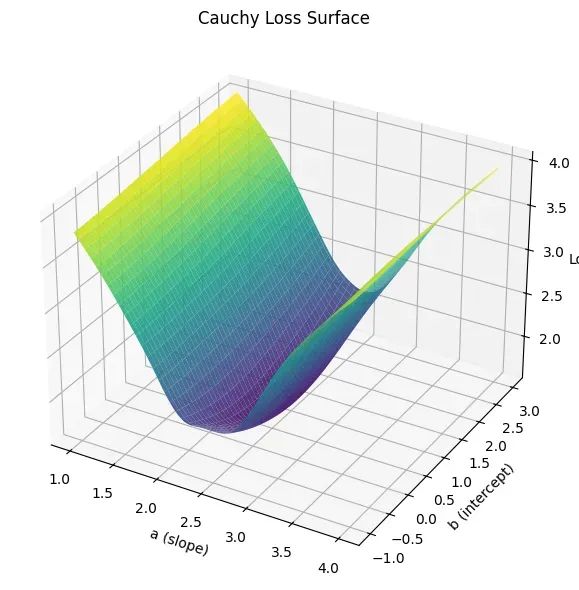
让回归模型不再被异常值“带跑偏“,MSE和Cauchy损失函数在噪声数据环境下的实战对比
在机器学习的回归分析中,损失函数的选择对模型性能具有决定性影响。均方误差(MSE)作为经典的损失函数,在处理干净数据时表现优异,但在面对包含异常值的噪声数据时,其对大误差的二次惩罚机制往往导致模型参数…...
SQL慢可能是触发了ring buffer
简介 最近在进行 postgresql 性能排查的时候,发现 PG 在某一个时间并行执行的 SQL 变得特别慢。最后通过监控监观察到并行发起得时间 buffers_alloc 就急速上升,且低水位伴随在整个慢 SQL,一直是 buferIO 的等待事件,此时也没有其他会话的争抢。SQL 虽然不是高效 SQL ,但…...
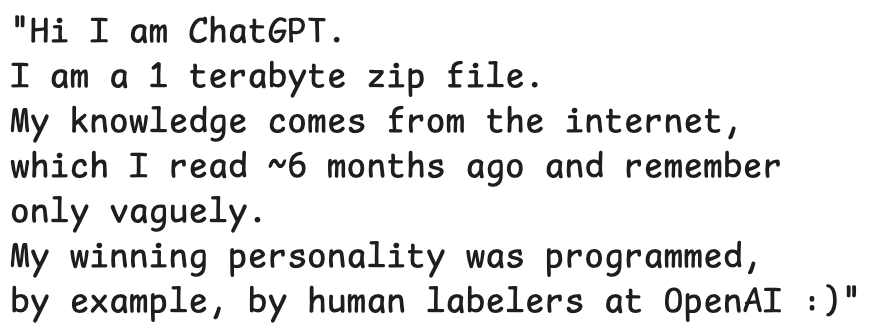
LLMs 系列实操科普(1)
写在前面: 本期内容我们继续 Andrej Karpathy 的《How I use LLMs》讲座内容,原视频时长 ~130 分钟,以实操演示主流的一些 LLMs 的使用,由于涉及到实操,实际上并不适合以文字整理,但还是决定尽量整理一份笔…...

C# 表达式和运算符(求值顺序)
求值顺序 表达式可以由许多嵌套的子表达式构成。子表达式的求值顺序可以使表达式的最终值发生 变化。 例如,已知表达式3*52,依照子表达式的求值顺序,有两种可能的结果,如图9-3所示。 如果乘法先执行,结果是17。如果5…...