【大模型】更强的开源可商用的中英文大语言模型baichuan2来了,从零开始搭建
【大模型】更强的开源可商用的中英文大语言模型baichuan2来了,从零开始搭建
- Baichuan 2 介绍
- 技术报告
- github 地址
- 模型下载
- 开放协议
- 协议
- 测试评估
- 通用领域测试
- 7B 模型结果
- 13B 模型结果
- 法律、医疗
- 7B 模型结果
- 13B 模型结果
- 数学、代码
- 7B 模型结果
- 13B 模型结果
- 多语言翻译
- 7B 模型结果
- 13B 模型结果
- 推理和部署
- 安装依赖
- Python 代码方式
- Chat 模型推理方法示范
- Base 模型推理方法示范
- 命令行工具方式
- 网页 demo 方式
- 量化部署
- 量化方法
- 在线量化
- 离线量化
- 量化效果
- CPU 部署
- 对 Baichuan 1 的推理优化迁移到 Baichuan 2
- 模型微调
- 依赖安装
- 单机训练
- 多机训练
- 轻量化微调
- 参考
baichuan-7B 可以查看这篇文章:
【AI实战】开源可商用的中英文大语言模型baichuan-7B,从零开始搭建
Baichuan 2 介绍
Baichuan 2 是百川智能推出的第二代开源大语言模型,采用 2.6 万亿 Tokens 的高质量语料训练。
Baichuan 2 在多个权威的中文、英文和多语言的通用、领域 benchmark 上取得同尺寸最佳的效果。
本次发布包含有 7B、13B 的 Base 和 Chat 版本,并提供了 Chat 版本的 4bits 量化。
技术报告
Baichuan 2: Open Large-scale Language Models
github 地址
https://github.com/baichuan-inc/Baichuan2
模型下载
- huggingface
本次发布版本和下载链接见下表:
基座模型 | 对齐模型 | 对齐模型 4bits 量化 | |
---|---|---|---|
7B | 🤗 Baichuan2-7B-Base | 🤗 Baichuan2-7B-Chat | 🤗 Baichuan2-7B-Chat-4bits |
13B | 🤗 Baichuan2-13B-Base | 🤗 Baichuan2-13B-Chat | 🤗 Baichuan2-13B-Chat-4bits |
- 国内的modelscope
百川2-7B-预训练模型
开放协议
所有版本对学术研究完全开放。同时,开发者通过邮件申请并获得官方商用许可后,即可免费商用。
协议
对本仓库源码的使用遵循开源许可协议 Apache 2.0。对 Baichuan 2 模型的社区使用需遵循《Baichuan 2 模型社区许可协议》。Baichuan 2 支持商用。如果将 Baichuan 2 模型或其衍生品用作商业用途,请您通过邮箱 opensource@baichuan-inc.com 联系许可方,申请书面授权。
测试评估
通用领域测试
7B 模型结果
C-Eval | MMLU | CMMLU | Gaokao | AGIEval | BBH | |
---|---|---|---|---|---|---|
5-shot | 5-shot | 5-shot | 5-shot | 5-shot | 3-shot | |
GPT-4 | 68.40 | 83.93 | 70.33 | 66.15 | 63.27 | 75.12 |
GPT-3.5 Turbo | 51.10 | 68.54 | 54.06 | 47.07 | 46.13 | 61.59 |
LLaMA-7B | 27.10 | 35.10 | 26.75 | 27.81 | 28.17 | 32.38 |
LLaMA2-7B | 28.90 | 45.73 | 31.38 | 25.97 | 26.53 | 39.16 |
MPT-7B | 27.15 | 27.93 | 26.00 | 26.54 | 24.83 | 35.20 |
Falcon-7B | 24.23 | 26.03 | 25.66 | 24.24 | 24.10 | 28.77 |
ChatGLM2-6B | 50.20 | 45.90 | 49.00 | 49.44 | 45.28 | 31.65 |
Baichuan-7B | 42.80 | 42.30 | 44.02 | 36.34 | 34.44 | 32.48 |
Baichuan2-7B-Base | 54.00 | 54.16 | 57.07 | 47.47 | 42.73 | 41.56 |
13B 模型结果
C-Eval | MMLU | CMMLU | Gaokao | AGIEval | BBH | |
---|---|---|---|---|---|---|
5-shot | 5-shot | 5-shot | 5-shot | 5-shot | 3-shot | |
GPT-4 | 68.40 | 83.93 | 70.33 | 66.15 | 63.27 | 75.12 |
GPT-3.5 Turbo | 51.10 | 68.54 | 54.06 | 47.07 | 46.13 | 61.59 |
LLaMA-13B | 28.50 | 46.30 | 31.15 | 28.23 | 28.22 | 37.89 |
LLaMA2-13B | 35.80 | 55.09 | 37.99 | 30.83 | 32.29 | 46.98 |
Vicuna-13B | 32.80 | 52.00 | 36.28 | 30.11 | 31.55 | 43.04 |
Chinese-Alpaca-Plus-13B | 38.80 | 43.90 | 33.43 | 34.78 | 35.46 | 28.94 |
XVERSE-13B | 53.70 | 55.21 | 58.44 | 44.69 | 42.54 | 38.06 |
Baichuan-13B-Base | 52.40 | 51.60 | 55.30 | 49.69 | 43.20 | 43.01 |
Baichuan2-13B-Base | 58.10 | 59.17 | 61.97 | 54.33 | 48.17 | 48.78 |
法律、医疗
7B 模型结果
JEC-QA | CEval-MMLU-CMMLU | MedQA-USMLE | MedQA-MCMLE | MedMCQA | |
---|---|---|---|---|---|
5-shot | 5-shot | 5-shot | 5-shot | 5-shot | |
GPT-4 | 59.32 | 77.16 | 80.28 | 74.58 | 72.51 |
GPT-3.5 Turbo | 42.31 | 61.17 | 53.81 | 52.92 | 56.25 |
LLaMA-7B | 27.45 | 33.34 | 24.12 | 21.72 | 27.45 |
LLaMA2-7B | 29.20 | 36.75 | 27.49 | 24.78 | 37.93 |
MPT-7B | 27.45 | 26.67 | 16.97 | 19.79 | 31.96 |
Falcon-7B | 23.66 | 25.33 | 21.29 | 18.07 | 33.88 |
ChatGLM2-6B | 40.76 | 44.54 | 26.24 | 45.53 | 30.22 |
Baichuan-7B | 34.64 | 42.37 | 27.42 | 39.46 | 31.39 |
Baichuan2-7B-Base | 44.46 | 56.39 | 32.68 | 54.93 | 41.73 |
13B 模型结果
JEC-QA | CEval-MMLU-CMMLU | MedQA-USMLE | MedQA-MCMLE | MedMCQA | |
---|---|---|---|---|---|
5-shot | 5-shot | 5-shot | 5-shot | 5-shot | |
GPT-4 | 59.32 | 77.16 | 80.28 | 74.58 | 72.51 |
GPT-3.5 Turbo | 42.31 | 61.17 | 53.81 | 52.92 | 56.25 |
LLaMA-13B | 27.54 | 35.14 | 28.83 | 23.38 | 39.52 |
LLaMA2-13B | 34.08 | 47.42 | 35.04 | 29.74 | 42.12 |
Vicuna-13B | 28.38 | 40.99 | 34.80 | 27.67 | 40.66 |
Chinese-Alpaca-Plus-13B | 35.32 | 46.31 | 27.49 | 32.66 | 35.87 |
XVERSE-13B | 46.42 | 58.08 | 32.99 | 58.76 | 41.34 |
Baichuan-13B-Base | 41.34 | 51.77 | 29.07 | 43.67 | 39.60 |
Baichuan2-13B-Base | 47.40 | 59.33 | 40.38 | 61.62 | 42.86 |
数学、代码
7B 模型结果
GSM8K | MATH | HumanEval | MBPP | |
---|---|---|---|---|
4-shot | 4-shot | 0-shot | 3-shot | |
GPT-4 | 89.99 | 40.20 | 69.51 | 63.60 |
GPT-3.5 Turbo | 57.77 | 13.96 | 52.44 | 61.40 |
LLaMA-7B | 9.78 | 3.02 | 11.59 | 14.00 |
LLaMA2-7B | 16.22 | 3.24 | 12.80 | 14.80 |
MPT-7B | 8.64 | 2.90 | 14.02 | 23.40 |
Falcon-7B | 5.46 | 1.68 | - | 10.20 |
ChatGLM2-6B | 28.89 | 6.40 | 9.15 | 9.00 |
Baichuan-7B | 9.17 | 2.54 | 9.20 | 6.60 |
Baichuan2-7B-Base | 24.49 | 5.58 | 18.29 | 24.20 |
13B 模型结果
GSM8K | MATH | HumanEval | MBPP | |
---|---|---|---|---|
4-shot | 4-shot | 0-shot | 3-shot | |
GPT-4 | 89.99 | 40.20 | 69.51 | 63.60 |
GPT-3.5 Turbo | 57.77 | 13.96 | 52.44 | 61.40 |
LLaMA-13B | 20.55 | 3.68 | 15.24 | 21.40 |
LLaMA2-13B | 28.89 | 4.96 | 15.24 | 27.00 |
Vicuna-13B | 28.13 | 4.36 | 16.46 | 15.00 |
Chinese-Alpaca-Plus-13B | 11.98 | 2.50 | 16.46 | 20.00 |
XVERSE-13B | 18.20 | 2.18 | 15.85 | 16.80 |
Baichuan-13B-Base | 26.76 | 4.84 | 11.59 | 22.80 |
Baichuan2-13B-Base | 52.77 | 10.08 | 17.07 | 30.20 |
多语言翻译
7B 模型结果
CN-EN | CN-FR | CN-ES | CN-AR | CN-RU | CN-JP | CN-DE | Average | |
---|---|---|---|---|---|---|---|---|
GPT-4 | 29.94 | 29.56 | 20.01 | 10.76 | 18.62 | 13.26 | 20.83 | 20.43 |
GPT-3.5 Turbo | 27.67 | 26.15 | 19.58 | 10.73 | 17.45 | 1.82 | 19.70 | 17.59 |
LLaMA-7B | 17.27 | 12.02 | 9.54 | 0.00 | 4.47 | 1.41 | 8.73 | 7.63 |
LLaMA2-7B | 25.76 | 15.14 | 11.92 | 0.79 | 4.99 | 2.20 | 10.15 | 10.14 |
MPT-7B | 20.77 | 9.53 | 8.96 | 0.10 | 3.54 | 2.91 | 6.54 | 7.48 |
Falcon-7B | 22.13 | 15.67 | 9.28 | 0.11 | 1.35 | 0.41 | 6.41 | 7.91 |
ChatGLM2-6B | 22.28 | 9.42 | 7.77 | 0.64 | 1.78 | 0.26 | 4.61 | 6.68 |
Baichuan-7B | 25.07 | 16.51 | 12.72 | 0.41 | 6.66 | 2.24 | 9.86 | 10.50 |
Baichuan2-7B-Base | 27.27 | 20.87 | 16.17 | 1.39 | 11.21 | 3.11 | 12.76 | 13.25 |
13B 模型结果
CN-EN | CN-FR | CN-ES | CN-AR | CN-RU | CN-JP | CN-DE | Average | |
---|---|---|---|---|---|---|---|---|
GPT-4 | 29.94 | 29.56 | 20.01 | 10.76 | 18.62 | 13.26 | 20.83 | 20.43 |
GPT-3.5 Turbo | 27.67 | 26.15 | 19.58 | 10.73 | 17.45 | 1.82 | 19.70 | 17.59 |
LLaMA-13B | 21.75 | 16.16 | 13.29 | 0.58 | 7.61 | 0.41 | 10.66 | 10.07 |
LLaMA2-13B | 25.44 | 19.25 | 17.49 | 1.38 | 10.34 | 0.13 | 11.13 | 12.17 |
Vicuna-13B | 22.63 | 18.04 | 14.67 | 0.70 | 9.27 | 3.59 | 10.25 | 11.31 |
Chinese-Alpaca-Plus-13B | 22.53 | 13.82 | 11.29 | 0.28 | 1.52 | 0.31 | 8.13 | 8.27 |
XVERSE-13B | 29.26 | 24.03 | 16.67 | 2.78 | 11.61 | 3.08 | 14.26 | 14.53 |
Baichuan-13B-Base | 30.24 | 20.90 | 15.92 | 0.98 | 9.65 | 2.64 | 12.00 | 13.19 |
Baichuan2-13B-Base | 30.61 | 22.11 | 17.27 | 2.39 | 14.17 | 11.58 | 14.53 | 16.09 |
推理和部署
推理所需的模型权重、源码、配置已发布在 Hugging Face,下载链接见本文档最开始的表格。我们在此示范多种推理方式。程序会自动从 Hugging Face 下载所需资源。
安装依赖
pip install -r requirements.txt
Python 代码方式
Chat 模型推理方法示范
>>> import torch
>>> from transformers import AutoModelForCausalLM, AutoTokenizer
>>> from transformers.generation.utils import GenerationConfig
>>> tokenizer = AutoTokenizer.from_pretrained("baichuan-inc/Baichuan2-13B-Chat", use_fast=False, trust_remote_code=True)
>>> model = AutoModelForCausalLM.from_pretrained("baichuan-inc/Baichuan2-13B-Chat", device_map="auto", torch_dtype=torch.bfloat16, trust_remote_code=True)
>>> model.generation_config = GenerationConfig.from_pretrained("baichuan-inc/Baichuan2-13B-Chat")
>>> messages = []
>>> messages.append({"role": "user", "content": "解释一下“温故而知新”"})
>>> response = model.chat(tokenizer, messages)
>>> print(response)
"温故而知新"是一句中国古代的成语,出自《论语·为政》篇。这句话的意思是:通过回顾过去,我们可以发现新的知识和理解。换句话说,学习历史和经验可以让我们更好地理解现在和未来。这句话鼓励我们在学习和生活中不断地回顾和反思过去的经验,从而获得新的启示和成长。通过重温旧的知识和经历,我们可以发现新的观点和理解,从而更好地应对不断变化的世界和挑战。
Base 模型推理方法示范
>>> from transformers import AutoModelForCausalLM, AutoTokenizer
>>> tokenizer = AutoTokenizer.from_pretrained("baichuan-inc/Baichuan2-13B-Base", trust_remote_code=True)
>>> model = AutoModelForCausalLM.from_pretrained("baichuan-inc/Baichuan2-13B-Base", device_map="auto", trust_remote_code=True)
>>> inputs = tokenizer('登鹳雀楼->王之涣\n夜雨寄北->', return_tensors='pt')
>>> inputs = inputs.to('cuda:0')
>>> pred = model.generate(**inputs, max_new_tokens=64, repetition_penalty=1.1)
>>> print(tokenizer.decode(pred.cpu()[0], skip_special_tokens=True))
登鹳雀楼->王之涣
夜雨寄北->李商隐
在上述两段代码中,模型加载指定
device_map='auto'
,会使用所有可用显卡。如需指定使用的设备,可以使用类似export CUDA_VISIBLE_DEVICES=0,1
(使用了0、1号显卡)的方式控制。
命令行工具方式
python cli_demo.py
本命令行工具是为 Chat 场景设计,因此我们不支持使用该工具调用 Base 模型。
网页 demo 方式
依靠 streamlit 运行以下命令,会在本地启动一个 web 服务,把控制台给出的地址放入浏览器即可访问。本网页 demo 工具是为 Chat 场景设计,因此我们不支持使用该工具调用 Base 模型。
streamlit run web_demo.py
量化部署
为了让不同的用户以及不同的平台都能运行 Baichuan 2 模型,我们针对 Baichuan 2 模型做了相应地量化工作(包括 Baichuan2-7B-Chat 和 Baichuan2-13B-Chat),方便用户快速高效地在自己的平台部署 Baichuan 2 模型。
量化方法
Baichuan 2 的采用社区主流的量化方法:BitsAndBytes。该方法可以保证量化后的效果基本不掉点,目前已经集成到 transformers 库里,并在社区得到了广泛应用。BitsAndBytes 支持 8bits 和 4bits 两种量化,其中 4bits 支持 FP4 和 NF4 两种格式,Baichuan 2 选用 NF4 作为 4bits 量化的数据类型。
基于该量化方法,Baichuan 2 支持在线量化和离线量化两种模式。
在线量化
对于在线量化,我们支持 8bits 和 4bits 量化,使用方式和 Baichuan-13B 项目中的方式类似,只需要先加载模型到 CPU 的内存里,再调用quantize()
接口量化,最后调用 cuda()
函数,将量化后的权重拷贝到 GPU 显存中。实现整个模型加载的代码非常简单,我们以 Baichuan2-7B-Chat 为例:
8bits 在线量化:
model = AutoModelForCausalLM.from_pretrained("baichuan-inc/Baichuan2-7B-Chat", torch_dtype=torch.float16, trust_remote_code=True)
model = model.quantize(8).cuda()
4bits 在线量化:
model = AutoModelForCausalLM.from_pretrained("baichuan-inc/Baichuan2-7B-Chat", torch_dtype=torch.float16, trust_remote_code=True)
model = model.quantize(4).cuda()
需要注意的是,在用 from_pretrained
接口的时候,用户一般会加上 device_map="auto"
,在使用在线量化时,需要去掉这个参数,否则会报错。
离线量化
为了方便用户的使用,我们提供了离线量化好的 4bits 的版本 Baichuan2-7B-Chat-4bits,供用户下载。
用户加载 Baichuan2-7B-Chat-4bits 模型很简单,只需要执行:
model = AutoModelForCausalLM.from_pretrained("baichuan-inc/Baichuan2-7B-Chat-4bits", device_map="auto", trust_remote_code=True)
对于 8bits 离线量化,我们没有提供相应的版本,因为 Hugging Face transformers 库提供了相应的 API 接口,可以很方便的实现 8bits 量化模型的保存和加载。用户可以自行按照如下方式实现 8bits 的模型保存和加载:
# Model saving: model_id is the original model directory, and quant8_saved_dir is the directory where the 8bits quantized model is saved.
model = AutoModelForCausalLM.from_pretrained(model_id, load_in_8bit=True, device_map="auto", trust_remote_code=True)
model.save_pretrained(quant8_saved_dir)
model = AutoModelForCausalLM.from_pretrained(quant8_saved_dir, device_map="auto", trust_remote_code=True)
量化效果
量化前后显存占用对比 (GPU Mem in GB):
Precision | Baichuan2-7B | Baichuan2-13B |
---|---|---|
bf16 / fp16 | 15.3 | 27.5 |
8bits | 8.0 | 16.1 |
4bits | 5.1 | 8.6 |
量化后在各个 benchmark 上的结果和原始版本对比如下:
Model 5-shot | C-Eval | MMLU | CMMLU |
---|---|---|---|
Baichuan2-13B-Chat | 56.74 | 57.32 | 59.68 |
Baichuan2-13B-Chat-4bits | 56.05 | 56.24 | 58.82 |
Baichuan2-7B-Chat | 54.35 | 52.93 | 54.99 |
Baichuan2-7B-Chat-4bits | 53.04 | 51.72 | 52.84 |
C-Eval 是在其 val set 上进行的评测
可以看到,4bits 相对 bfloat16 精度损失在 1 - 2 个百分点左右。
CPU 部署
Baichuan 2 模型支持 CPU 推理,但需要强调的是,CPU 的推理速度相对较慢。需按如下方式修改模型加载的方式:
# Taking Baichuan2-7B-Chat as an example
model = AutoModelForCausalLM.from_pretrained("baichuan-inc/Baichuan2-7B-Chat", torch_dtype=torch.float32, trust_remote_code=True)
对 Baichuan 1 的推理优化迁移到 Baichuan 2
由于很多用户在 Baichuan 1 (Baichuan-7B, Baichuan-13B)上做了很多优化的工作,例如编译优化、量化等,为了将这些工作零成本地应用于 Baichuan 2,用户可以对 Baichuan 2 模型做一个离线转换,转换后就可以当做 Baichuan 1 模型来使用。具体来说,用户只需要利用以下脚本离线对 Baichuan 2 模型的最后一层 lm_head 做归一化,并替换掉lm_head.weight
即可。替换完后,就可以像对 Baichuan 1 模型一样对转换后的模型做编译优化等工作了。
import torch
import os
ori_model_dir = 'your Baichuan 2 model directory'
# To avoid overwriting the original model, it's best to save the converted model to another directory before replacing it
new_model_dir = 'your normalized lm_head weight Baichuan 2 model directory'
model = torch.load(os.path.join(ori_model_dir, 'pytorch_model.bin'))
lm_head_w = model['lm_head.weight']
lm_head_w = torch.nn.functional.normalize(lm_head_w)
model['lm_head.weight'] = lm_head_w
torch.save(model, os.path.join(new_model_dir, 'pytorch_model.bin'))
模型微调
依赖安装
git clone https://github.com/baichuan-inc/Baichuan2.git
cd Baichuan2/fine-tune
pip install -r requirements.txt
- 如需使用 LoRA 等轻量级微调方法需额外安装 peft
- 如需使用 xFormers 进行训练加速需额外安装 xFormers
单机训练
下面我们给一个微调 Baichuan2-7B-Base 的单机训练例子。
训练数据:data/belle_chat_ramdon_10k.json
,该样例数据是从 multiturn_chat_0.8M 采样出 1 万条,并且做了格式转换。主要是展示多轮数据怎么训练,不保证效果。
hostfile=""
deepspeed --hostfile=$hostfile fine-tune.py \--report_to "none" \--data_path "data/belle_chat_ramdon_10k.json" \--model_name_or_path "baichuan-inc/Baichuan2-7B-Base" \--output_dir "output" \--model_max_length 512 \--num_train_epochs 4 \--per_device_train_batch_size 16 \--gradient_accumulation_steps 1 \--save_strategy epoch \--learning_rate 2e-5 \--lr_scheduler_type constant \--adam_beta1 0.9 \--adam_beta2 0.98 \--adam_epsilon 1e-8 \--max_grad_norm 1.0 \--weight_decay 1e-4 \--warmup_ratio 0.0 \--logging_steps 1 \--gradient_checkpointing True \--deepspeed ds_config.json \--bf16 True \--tf32 True
多机训练
多机训练只需要给一下 hostfile ,内容类似如下:
ip1 slots=8
ip2 slots=8
ip3 slots=8
ip4 slots=8
....
同时在训练脚本里面指定 hosftfile 的路径:
hostfile="/path/to/hostfile"
deepspeed --hostfile=$hostfile fine-tune.py \--report_to "none" \--data_path "data/belle_chat_ramdon_10k.json" \--model_name_or_path "baichuan-inc/Baichuan2-7B-Base" \--output_dir "output" \--model_max_length 512 \--num_train_epochs 4 \--per_device_train_batch_size 16 \--gradient_accumulation_steps 1 \--save_strategy epoch \--learning_rate 2e-5 \--lr_scheduler_type constant \--adam_beta1 0.9 \--adam_beta2 0.98 \--adam_epsilon 1e-8 \--max_grad_norm 1.0 \--weight_decay 1e-4 \--warmup_ratio 0.0 \--logging_steps 1 \--gradient_checkpointing True \--deepspeed ds_config.json \--bf16 True \--tf32 True
轻量化微调
代码已经支持轻量化微调如 LoRA,如需使用仅需在上面的脚本中加入以下参数:
--use_lora True
LoRA 具体的配置可见 fine-tune.py
脚本。
使用 LoRA 微调后可以使用下面的命令加载模型:
from peft import AutoPeftModelForCausalLM
model = AutoPeftModelForCausalLM.from_pretrained("output", trust_remote_code=True)
参考
1.https://github.com/baichuan-inc/Baichuan2
2.https://modelscope.cn/models/baichuan-inc/Baichuan2-7B-Base/summary
3.【AI实战】开源可商用的中英文大语言模型baichuan-7B,从零开始搭建
4.https://huggingface.co/baichuan-inc/Baichuan2-7B-Base
相关文章:

【大模型】更强的开源可商用的中英文大语言模型baichuan2来了,从零开始搭建
【大模型】更强的开源可商用的中英文大语言模型baichuan2来了,从零开始搭建 Baichuan 2 介绍技术报告github 地址 模型下载开放协议协议 测试评估通用领域测试7B 模型结果13B 模型结果 法律、医疗7B 模型结果13B 模型结果 数学、代码7B 模型结果13B 模型结果 多语言…...
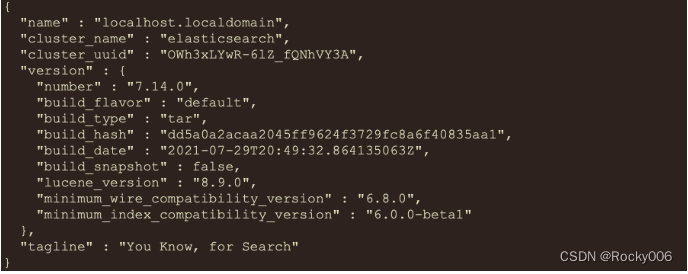
ElasticSearch系列-简介与安装详解
全文检索 讲ElasticSearch之前, 需要先提一下全文检索.全文检索是计算机程序通过扫描文章中的每一个词,对每一个词建立一个索引,指明该词在文章中出现的次数和位置。当用户查询时根据建立的索引查找,类似于通过字典的检索字表查字的过程。 …...

Layui + Flask | 表单组件(组件篇)(07)
http://layui.dev/docs/2.8/form 表单组件 form 是包含输入框、选择框、复选框、开关、单选框等表单项组件的集合,主要用于对表单域进行各类动态化渲染和相关的交互操作。form是 Layui 最常用的组件之一。 表单布局 form 组件自身的普通布局。其要点为: 通过 class="lay…...
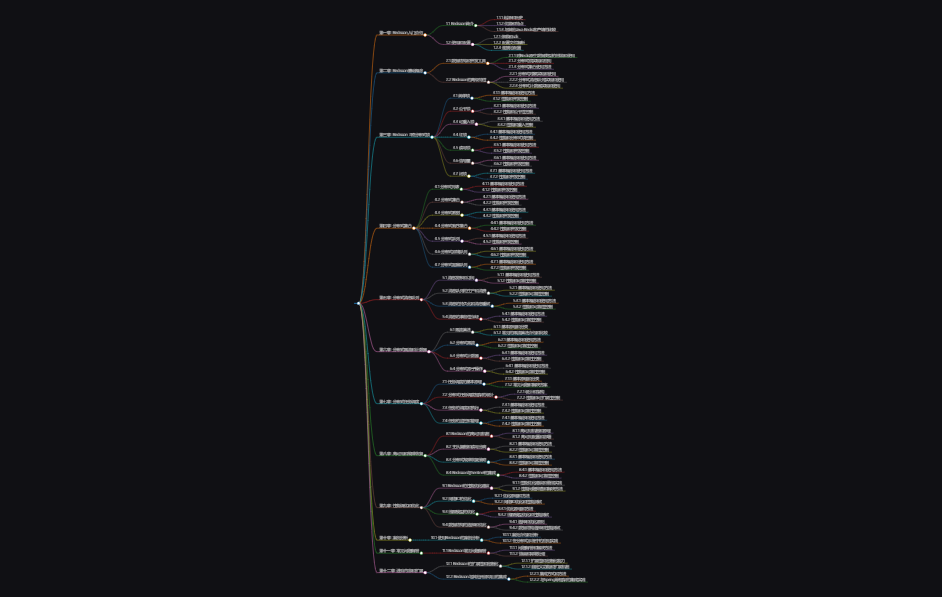
【实践篇】Redis最强Java客户端Redisson
文章目录 1. 前言2. Redisson基础概念2.1 数据结构和并发工具2.1.1 对Redis原生数据类型的封装和使用2.1.2 分布式锁实现和应用2.1.3 分布式集合使用方法 2.2 Redisson的高级特性2.2.1 分布式对象实现和使用2.2.2 分布式消息队列实现和使用2.2.3 分布式计数器实现和使用 3. 参考…...
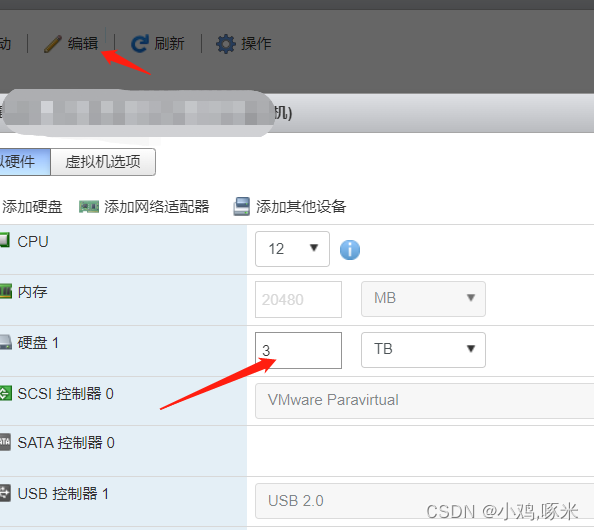
esxi扩容磁盘
esxi扩容磁盘 fdisk -l没用扩容 登录Esxi管理界面扩容磁盘 进入服务器查看 没用变化 (有些可能进去磁盘就是更新,直接就是扩容的,但是没扩容就需要执行下面的命令) [root234-ces /]# fdisk -l Disk /dev/sda: 85.9 GB, 858993…...
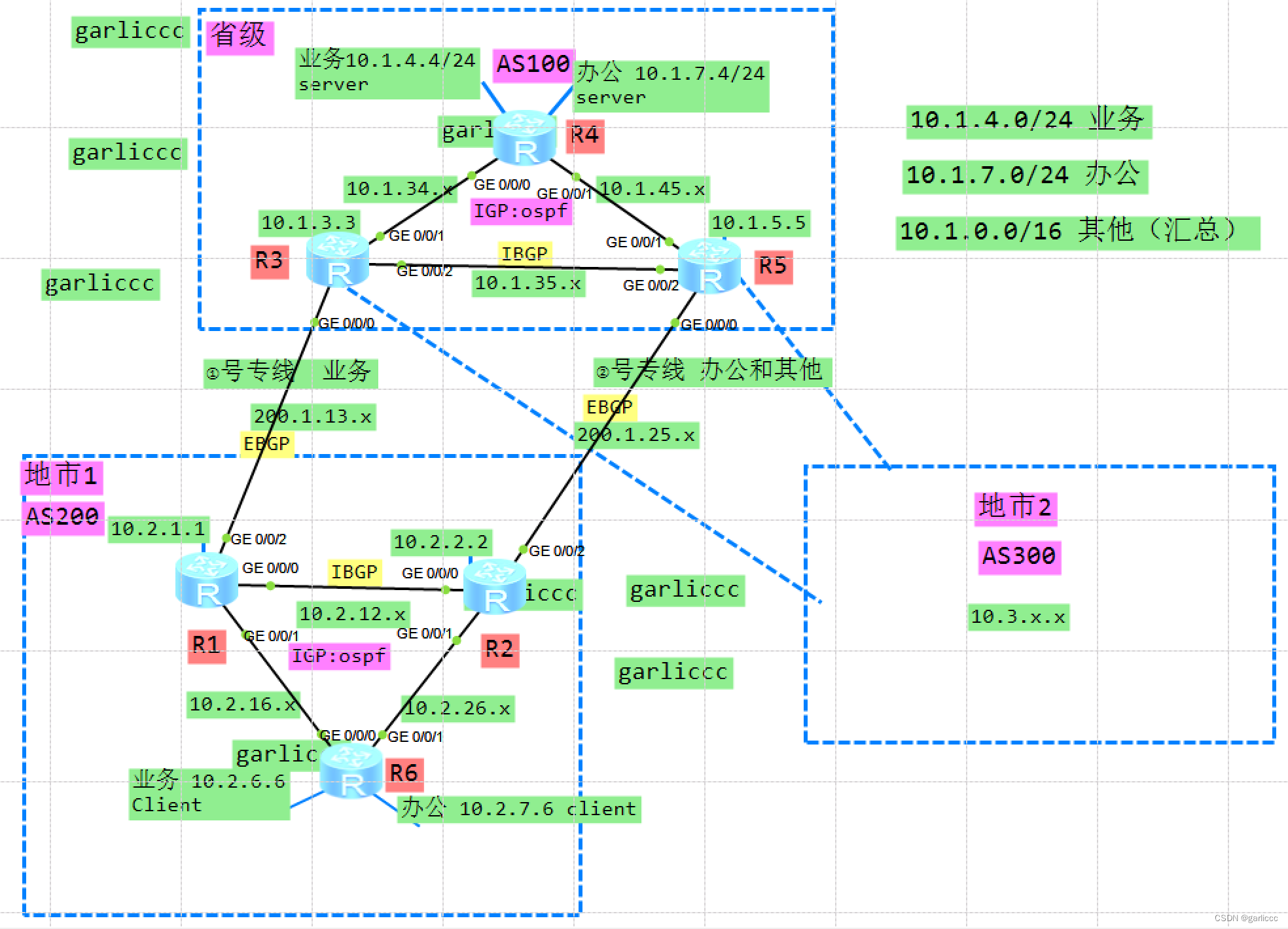
核心实验21_BGP高级(了解)(配置略)_ENSP
项目场景: 核心实验21_BGP基础_ENSP 通过bgp实现省市互通。 实搭拓扑图: 具体操作: 其他基础配置略(接口地址,ospf) 1.BGP邻居建立: R1: [R1]bgp 200 [R1-bgp]peer 10.2.2.2 as-number 200 …...

宝塔安装python和openssl
宝塔安装python和openssl OpenSSL Centos7 openssl 升级 1.1.1k.tar.gz centos7系统安装Vicuna(小羊驼)聊天机器人 CentOS中输入yum报错:sudo: unable to execute /bin/yum: No such file or directory opensslrpm安装指南-让你的网站更加…...

TDengine 3.1.1.0 来啦!更新如下
自 3.0 版本发布以来,在研发人员和社区用户的不断努力下,TDengine 做了大量更新,产品稳定性和易用性也在不断提升。近日,TDengine 3.1.1.0 成功发布,本文将向大家简单介绍一下该版本涉及的重大更新。 写在前面 伴随 …...
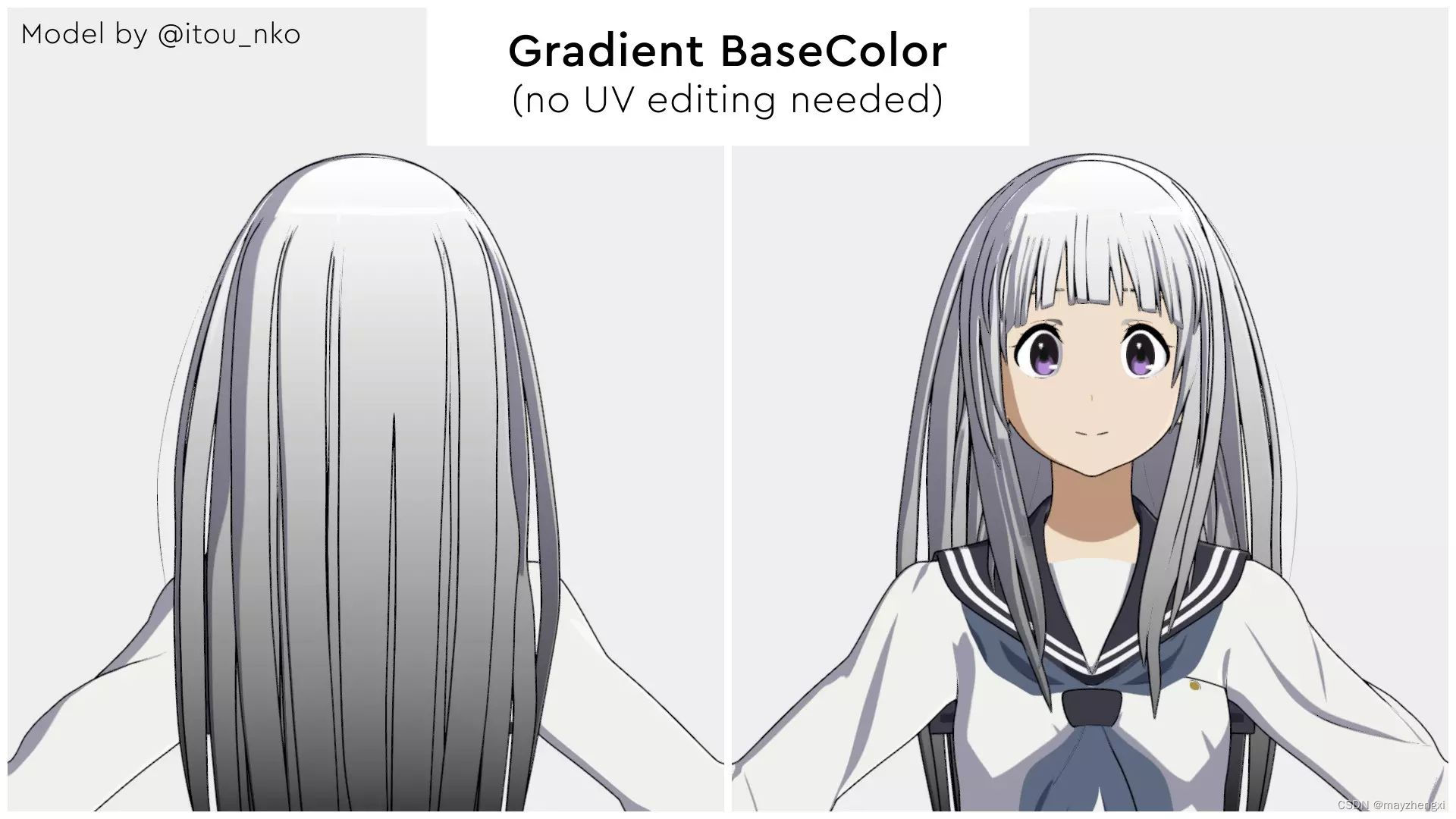
YSA Toon (Anime/Toon Shader)
这是一个Toon着色器/Cel阴影着色器,用于Unity URP 此着色器的目的是使角色或物体阴影实时看起来尽可能接近真实的动画或卡通效果 可以用于游戏,渲染,插图等 着色器特性,如:面的法线平滑、轮廓修复、先进的边缘照明、镜面照明、完全平滑控制 这个文档包括所有的功能https:/…...
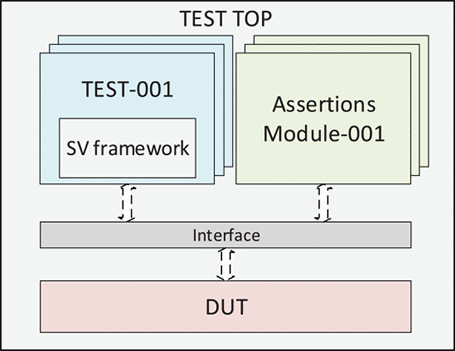
LabVIEW通过IEC61508标准验证ITER联锁系统
LabVIEW通过IEC61508标准验证ITER联锁系统 保护环境要求系统能够保护机器免受工厂系统故障或机器危险操作造成的严重损坏。负责此功能的ITER系统是联锁控制系统(ICS)。该系统通过中央联锁系统(CIS)监督和控制不同的工厂联锁系统&…...

如何处理日期和时间?
处理日期和时间是计算机编程中的常见任务,无论是在C语言还是其他编程语言中。C语言提供了一些库函数来处理日期和时间,主要是通过<time.h>头文件中的函数来完成的。在本文中,我将详细解释如何在C语言中处理日期和时间,包括日…...
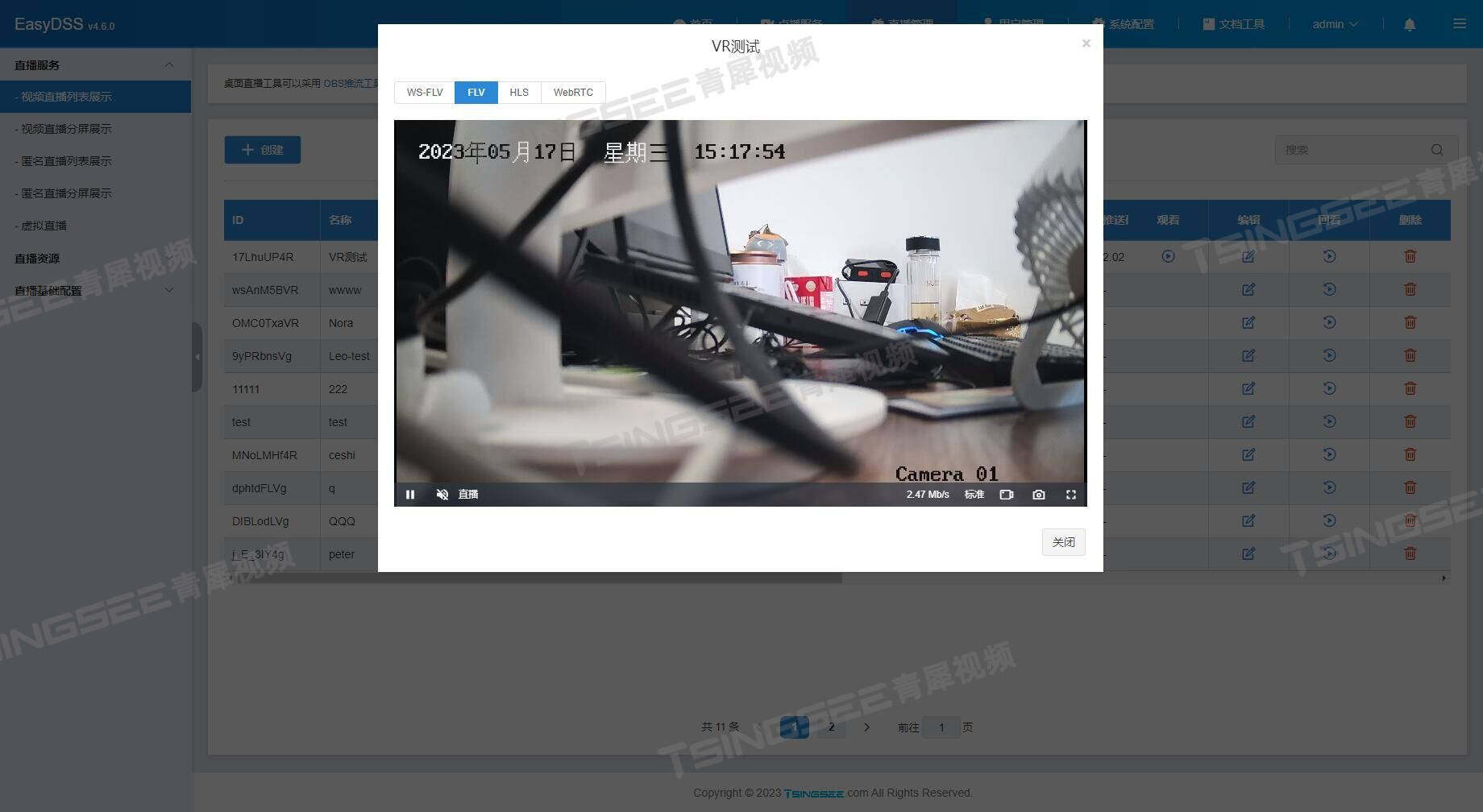
【开发】视频集中存储/直播点播平台EasyDSS点播文件分类功能优化
视频推拉流EasyDSS视频直播点播平台,集视频直播、点播、转码、管理、录像、检索、时移回看等功能于一体,可提供音视频采集、视频推拉流、播放H.265编码视频、存储、分发等视频能力服务。 TSINGSEE青犀视频的EasyDSS平台具有点播文件分类展示方法…...
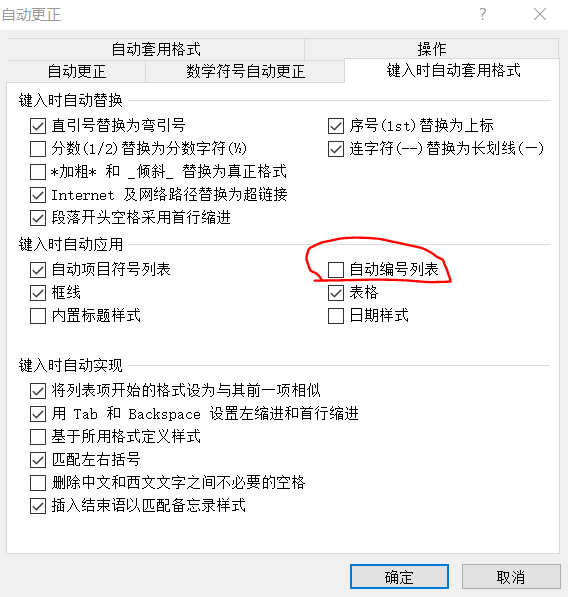
论文多级编号-word2010
多级列表-定义新的多级列表 注意1.1中的两个1必须是灰色(如果不是灰色,解决方法放在文本文末了) 如果定义过程中发现1.1中的1不是灰色,如下图,那么需要操作下述步骤 点击文件-选项 取消勾选自动编号列表。确定后关闭文…...

Jetpack Compose基础组件之 — Text
Text的源码参数预览 Composable fun Text(text: String,modifier: Modifier Modifier,color: Color Color.Unspecified,fontSize: TextUnit TextUnit.Unspecified,fontStyle: FontStyle? null,fontWeight: FontWeight? null,fontFamily: FontFamily? null,letterSpac…...
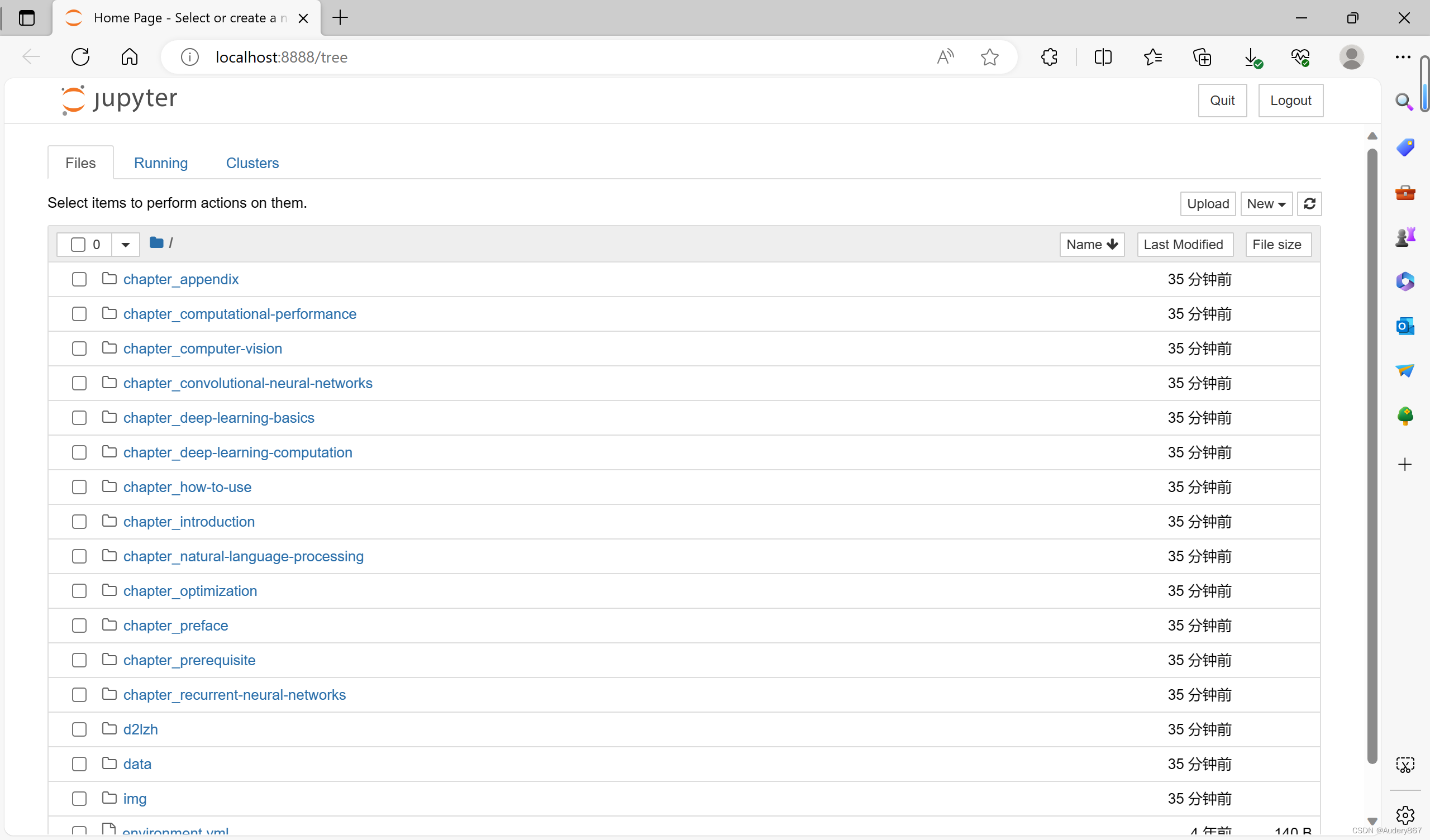
动手学深度学习——Windows下的环境安装流程(一步一步安装,图文并配)
目录 环境安装官网步骤图文版安装Miniconda下载包含本书全部代码的压缩包使用conda创建虚拟(运行)环境使用conda创建虚拟环境并安装本书需要的软件激活之前创建的环境打开Jupyter记事本 环境安装 文章参考来源:http://t.csdn.cn/tu8V8 官网…...
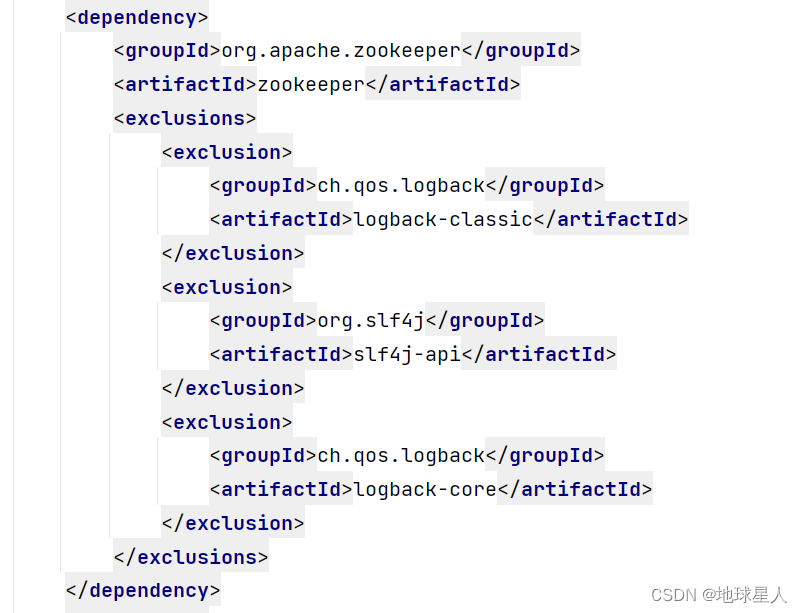
打印日志遇到的问题,logback与zookeeper冲突
在做项目时需要打印日志引入了logback打印日志,但是一直无法打印,于是一路查找原因。发现zookeeper中默认带的有个logback和我自己引入的logback版本冲突了,这样直接使用exclusions标签将zookeeper中自带的日志框架全部排除即可 按理说到这一…...

【Node.js操作SQLite指南】
Node.js操作SQLite指南 在本篇博客中,我们将学习如何在Node.js中操作SQLite数据库。我们将使用sqlite3模块来创建数据库、创建表以及进行数据的增删改查操作。 文章目录 Node.js操作SQLite指南安装sqlite3模块创建数据库创建表数据的增删改查插入数据查询数据更新…...
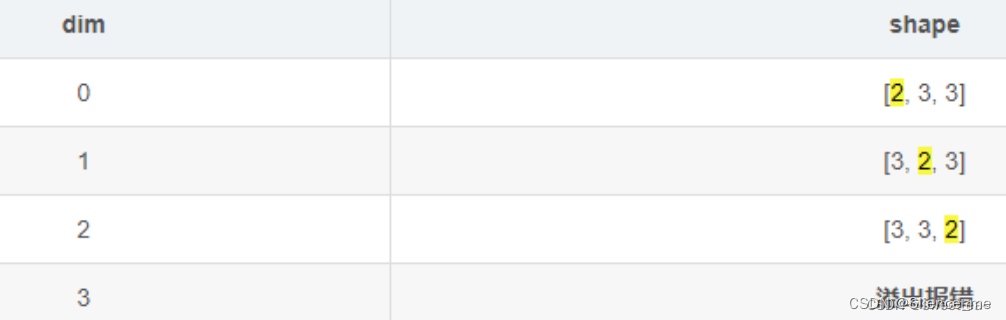
PyTorch之张量的相关操作大全 ->(个人学习记录笔记)
文章目录 Torch1. 张量的创建1.1 直接创建1.1.1 torch.tensor1.1.2 torch.from_numpy(ndarray) 1.2 依据数值创建1.2.1 torch.zeros1.2.2 torch.zeros_like1.2.3 torch.ones1.2.4 torch.ones_like1.2.5 torch.full1.2.6 torch.full_like1.2.7 torch.arange1.2.8 torch.linspace…...
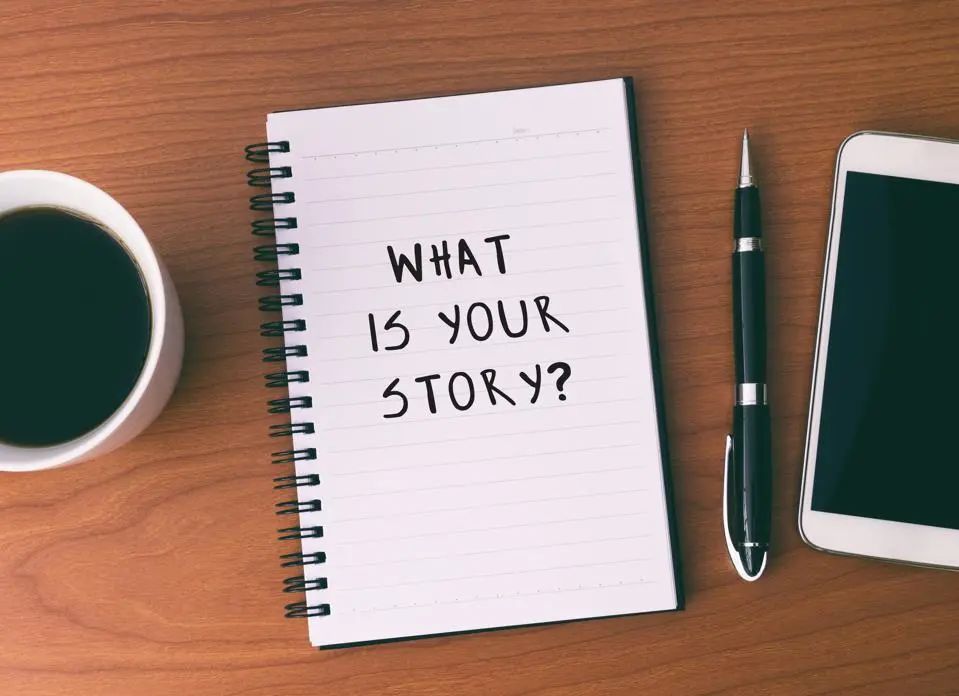
ChatGPT生成内容很难脱离标准化,不建议用来写留学文书
ChatGPT无疑是23年留学届的热门话题,也成为了不少留学生再也离不开的万能工具,从总结文献、润色论文、给教授写email似乎无所不能。 各大高校对于学生使用ChatGPT的态度也有所不同。例如,哈佛大学教育代理院长 Anne Harrington 在内部邮件中…...

sqlserver @@ROWCOUNT的使用
T-SQL是一种用于与关系型数据库(如Microsoft SQL Server)交互的SQL(Structured Query Language)方言。 在T-SQL中,ROWCOUNT是一个系统变量,它返回最后执行的语句影响的行数。你提供的代码检查ROWCOUNT的值…...

Hbase批量删除数据
一、TTL机制 HBase的TTL(Time To Live)是一种用于指定数据存活时间的机制。它允许用户为HBase中的数据设置一个固定的生存时间,在达到指定的时间后,HBase会自动删除这些数据。 具体操作如下: 三步走,先禁用…...
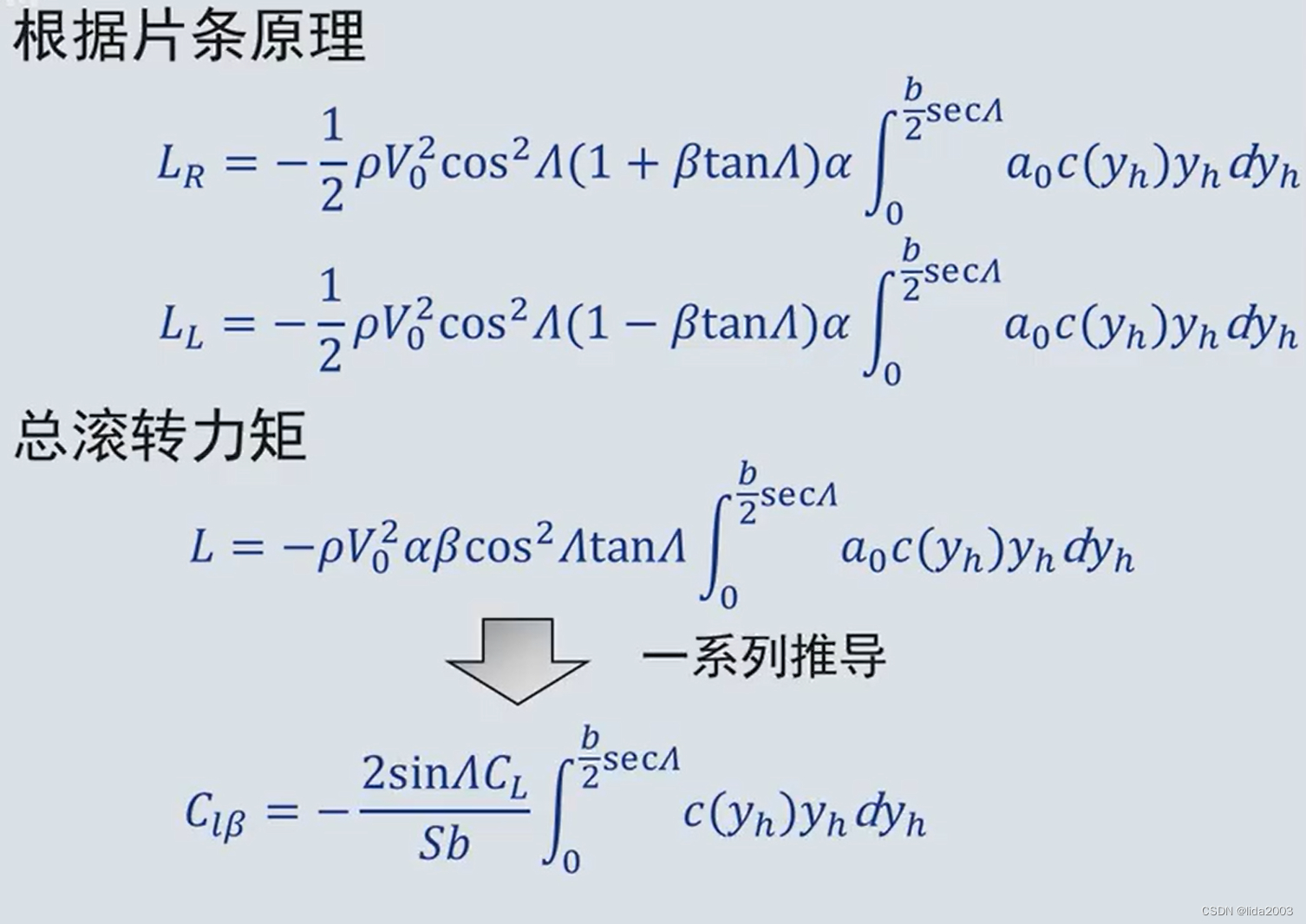
飞行动力学 - 第20节-part2-机翼上反及后掠对横向静稳定性的影响 之 基础点摘要
飞行动力学 - 第20节-part2-机翼上反及后掠对横向静稳定性的影响 之 基础点摘要 1. 上反角贡献2. 后掠角贡献3. 参考资料 1. 上反角贡献 对于无后掠、大展弦比带上反的矩形机翼,飞行状态为 α \alpha α, β \beta β及V。 上反角增加稳定性,…...
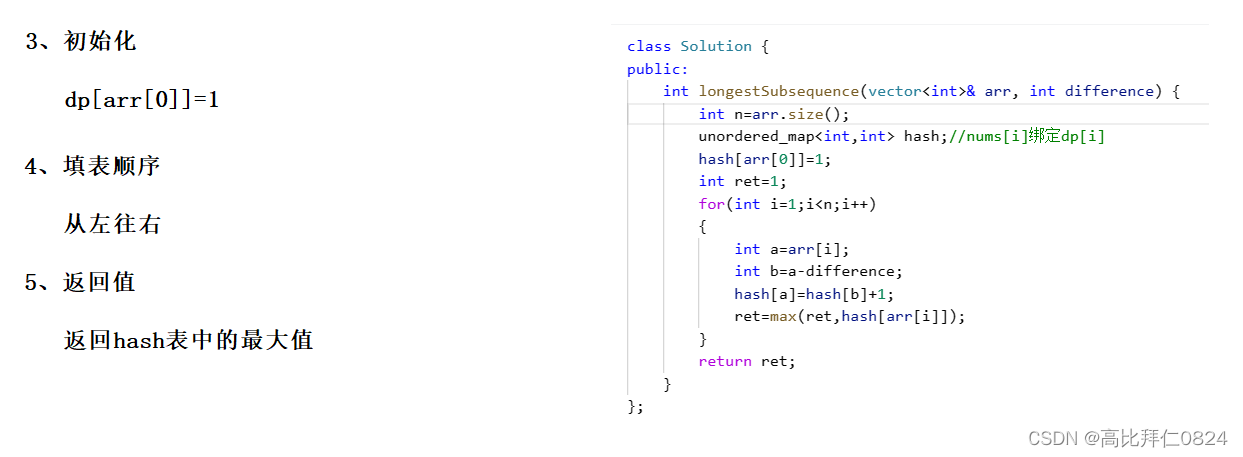
力扣 -- 1218. 最长定差子序列
参考代码: class Solution { public:int longestSubsequence(vector<int>& arr, int difference) {int narr.size();unordered_map<int,int> hash;//nums[i]绑定dp[i]hash[arr[0]]1;int ret1;for(int i1;i<n;i){int aarr[i];int ba-difference;…...

【程序员装机】在右键菜单中添加Notepad++选项
文章目录 前言在右键菜单中添加Notepad选项的批处理脚本上述批处理脚本的功能包括 总结 前言 本文将介绍如何通过批处理脚本来在Windows右键菜单中添加Notepad选项,使您能够轻松使用Notepad打开各种文件。 在右键菜单中添加Notepad选项的批处理脚本 以下是一个用于…...
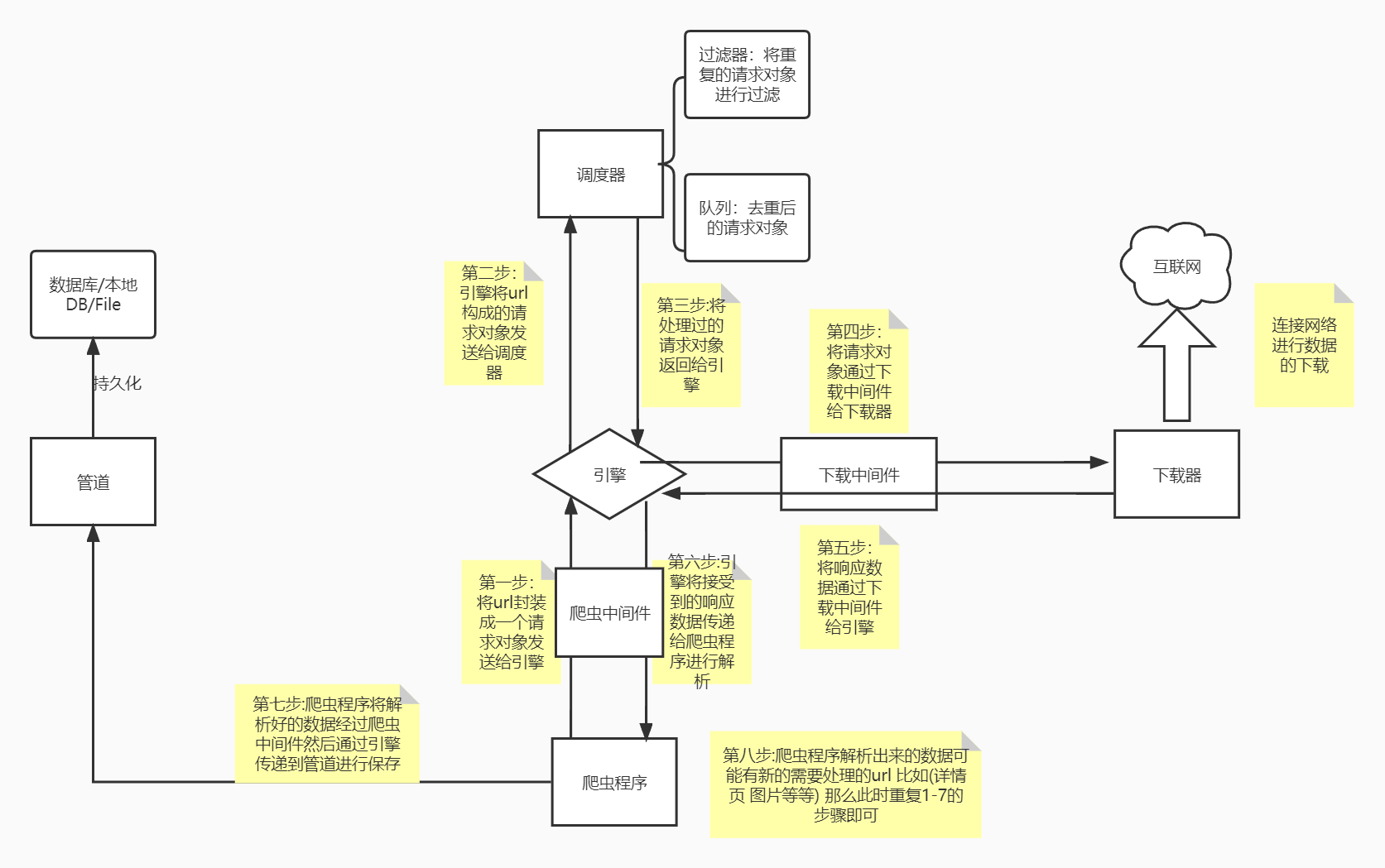
Scrapy的基本介绍、安装及工作流程
一.Scrapy介绍 Scrapy是什么? Scrapy 是用 Python 实现的一个为了爬取网站数据、提取结构性数据而编写的应用框架(异步爬虫框架) 通常我们可以很简单的通过 Scrapy 框架实现一个爬虫,抓取指定网站的内容或图片。 Scrapy使用了Twisted异步网络框架&…...
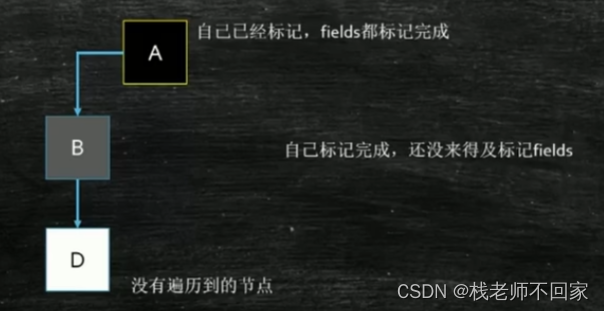
CMS 三色标记【JVM调优】
文章目录 1. 垃圾回收器2. CMS 原理3. 三色标记算法 1. 垃圾回收器 ① Serial:最原始的垃圾回收器,用于新生代,是单线程的,GC 时需要停止其它所有的工作,算法简单,但它只能在内存较小时勉强使用;…...
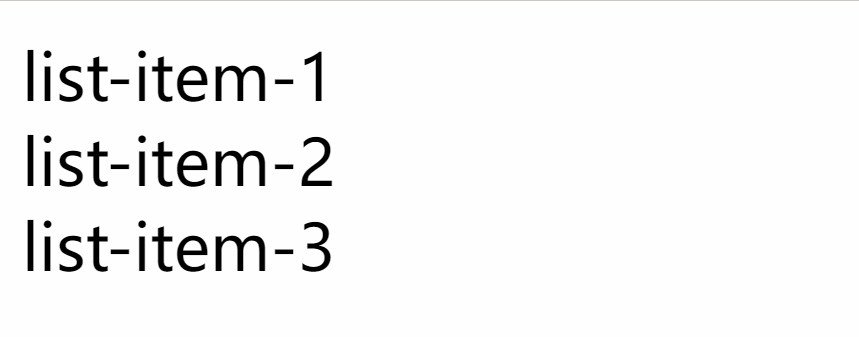
使用 CSS 伪类的attr() 展示 tooltip
效果图: 使用场景: 使用React渲染后台返回的数据, 遍历以列表的形式展示, 可能简要字段内容需要鼠标放上去才显示的 可以借助DOM的自定义属性和CSS伪类的attr来实现 所有代码: <!DOCTYPE html> <html lang"en"><head><meta charset"UTF-…...

在命令窗口便捷快速复制输出结果到剪贴板
在macOS上,将命令的输出结果复制到剪贴板 在日常的工作中, 经常使用命令的小伙伴可能会遇到一个场景, 就是把命令执行的结果复制出来另作它用. 每次都需要通过鼠标进行选择然后复制, 虽然 macOS 的命令行的复制快捷键和普通的复制是一样的, 非常友好, 但是还要选择…...
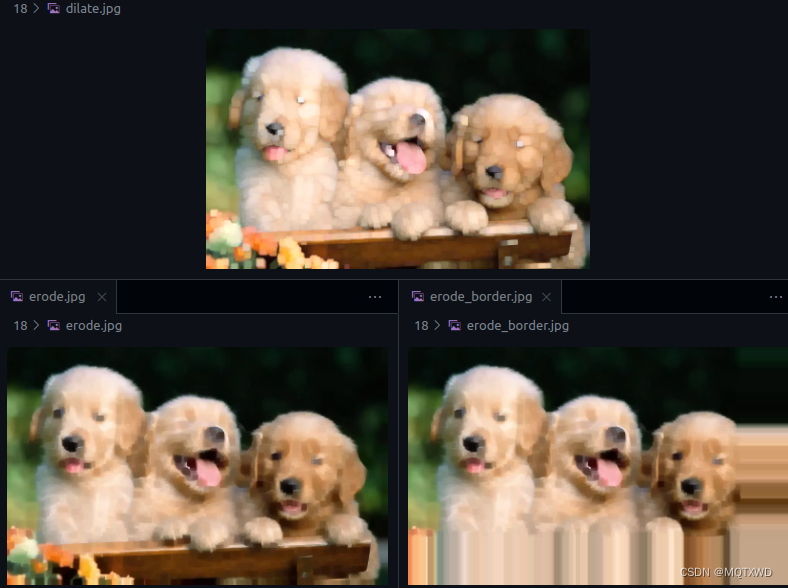
CUDA小白 - NPP(8) 图像处理 Morphological Operations
cuda小白 原始API链接 NPP GPU架构近些年也有不少的变化,具体的可以参考别的博主的介绍,都比较详细。还有一些cuda中的专有名词的含义,可以参考《详解CUDA的Context、Stream、Warp、SM、SP、Kernel、Block、Grid》 常见的NppStatus…...

java获取音频,文本准转语音时长
jar 以上传到资源中 <dependency><groupId>it.sauronsoftware</groupId><artifactId>jave</artifactId><version>1.0.2</version></dependency> mvn install:install-file -DfileD:\xxx\xxx\jave-1.0.2.jar -DgroupIdit.sauro…...