【PyTorch】教程:对抗学习实例生成
ADVERSARIAL EXAMPLE GENERATION
研究推动 ML
模型变得更快、更准、更高效。设计和模型的安全性和鲁棒性经常被忽视,尤其是面对那些想愚弄模型故意对抗时。
本教程将提供您对 ML
模型的安全漏洞的认识,并将深入了解对抗性机器学习这一热门话题。在图像中添加难以察觉的扰动会导致模型性能的显著不同,鉴于这是一个教程,我们将通过图像分类器的示例来探讨这个主题。具体来说,我们将使用第一种也是最流行的攻击方法之一,快速梯度符号攻击( FGSM
)来欺骗 MNIST
分类器。
Threat Model (攻击模型)
在论文中,有许多类型的对抗攻击,每种攻击都有不同的目标和攻击者的知识假设。然而,总的来说,首要目标是向输入数据添加最小数量的扰动,以导致期望的错误分类。攻击者的知识有几种假设,其中两种是: white-box
(白盒)和 black-box
(黑盒);白盒攻击假定攻击者具有对模型的完整知识和访问权限,包括体系结构、输入、输出和权重。黑盒攻击假设攻击者只能访问模型的输入和输出,并且对底层架构或权重一无所知。还有几种类型的目标,包括 misclassification
(错误分类)和 source/target misclassification
源/目标错误分类。错误分类的目标意味着对手只希望输出分类错误,而不在乎新的分类是什么。源/目标错误分类意味着对手希望更改最初属于特定源类别的图像,从而将其分类为特定目标类别。
Fast Gradient Sign Attack
FGSM 攻击是白盒攻击,目标是错误分类。
迄今为止最早也是最流行的的对抗攻击是 Fast Gradient Sign Attack, FGSM
(Explaining and Harnessing Adversarial Examples),这种攻击非常强大, 也很直观。它旨在利用神经网络的学习方式,即梯度来攻击神经网络。这个想法很简单,而不是通过基于反向传播梯度调整权重来最小化损失,而是基于相同的反向传播梯度来调整输入数据以最大化损失。换句话说,攻击使用输入数据的损失梯度,然后调整输入数据以最大化损失。
从图中可以看出, xxx 是被正确分类为 panda 的原始图像,yyy 是 xxx 的正确标签,θ\thetaθ 代表的是模型参数,$ J(\theta, x, y)$ 是训练网络的 loss
。攻击反向传播梯度到输入数据计算 ∇xJ(θ,x,y)\nabla_x J(\theta, x, y)∇xJ(θ,x,y) , 然后利用很小的步长 ( ϵ\epsilonϵ 或 0.007 ) 在某个方向上最大化损失(例如: sign(∇xJ(θ,x,y))sign(\nabla_x J(\theta, x, y))sign(∇xJ(θ,x,y)) ),最后的扰动图像 x′x'x′ 最后被错误分类为 gibbon
, 实际上图像还是 panda
。
import torch
import torch.nn as nn
import torch.nn.functional as F
import torch.optim as optim
from torchvision import datasets, transforms
import numpy as np
import matplotlib.pyplot as plt from six.moves import urllib
opener = urllib.request.build_opener()
opener.addheaders = [('User-agent', 'Mozilla/5.0')]
urllib.request.install_opener(opener)
Implementation
本节中,我们将讨论教程的输入参数,定义攻击下的模型,以及相关的测试
Inputs
三个输入:
- epsilons: epsilon 列表值,保持 0 在列表中非常重要,代表着原始模型的性能。 epsilon 越大代表着攻击越大。
- pretrained_model: 预训练模型,训练模型的代码在 这里. 也可以直接下载 预训练模型. 因为 google drive 无法下载,所以还可以在 CSDN资源 下载
- use_cuda: 使用 GPU;
Model Under Attack
定义了模型和 DataLoader,初始化模型和加载权重。
class Net(nn.Module):def __init__(self):super(Net, self).__init__()self.conv1 = nn.Conv2d(1, 32, 3, 1)self.conv2 = nn.Conv2d(32, 64, 3, 1)self.dropout1 = nn.Dropout(0.25)self.dropout2 = nn.Dropout(0.5)self.fc1 = nn.Linear(9216, 128)self.fc2 = nn.Linear(128, 10)def forward(self, x):x = self.conv1(x)x = F.relu(x)x = self.conv2(x)x = F.relu(x)x = F.max_pool2d(x, 2)x = self.dropout1(x)x = torch.flatten(x, 1)x = self.fc1(x)x = F.relu(x)x = self.dropout2(x)x = self.fc2(x)output = F.log_softmax(x, dim=1)return outputepsilons = [0, .05, .1, .15, .2, .25, .3]
pretrained_model = "lenet_mnist_model.pt"
use_cuda = True# MNIST Test dataset and dataloader declaration
test_loader = torch.utils.data.DataLoader(datasets.MNIST('../../../datasets', train=False, download=True, transform=transforms.Compose([transforms.ToTensor(),])),batch_size=1, shuffle=True)print("CUDA Available: ", torch.cuda.is_available())
device = torch.device('cuda' if (use_cuda and torch.cuda.is_available()) else 'cpu')# init network
model = Net().to(device)# load the pretrained model
model.load_state_dict(torch.load(pretrained_model, map_location='cpu'))# set the model in evaluation mode. In this case this is for the Dropout layers
model.eval()
CUDA Available: True
Net((conv1): Conv2d(1, 32, kernel_size=(3, 3), stride=(1, 1))(conv2): Conv2d(32, 64, kernel_size=(3, 3), stride=(1, 1))(dropout1): Dropout(p=0.25, inplace=False)(dropout2): Dropout(p=0.5, inplace=False)(fc1): Linear(in_features=9216, out_features=128, bias=True)(fc2): Linear(in_features=128, out_features=10, bias=True)
)
FGSM Attack (FGSM 攻击)
我们现在定义一个函数创建一个对抗实例,通过对原始输入进行干扰。 fgsm_attack 函数有3个输入,原始输入图像 xxx,像素方向扰动量 ϵ\epsilonϵ ,梯度损失,(例如 ∇xJ(θ,x,y)\nabla_x J(\mathbf{\theta}, \mathbf{x}, y)∇xJ(θ,x,y))
创建干扰图像
perturbedimage=image+epsilon∗sign(datagrad)=x+ϵ∗sign(∇xJ(θ,x,y))perturbed_image=image+epsilon∗sign(data_grad)=x+ϵ∗sign(∇x J(θ,x,y)) perturbedimage=image+epsilon∗sign(datagrad)=x+ϵ∗sign(∇xJ(θ,x,y))
最后,为了保持原始图像的数据范围,干扰图像被缩放到 [0, 1]
# FGSM attack code
def fgsm_attack(image, epsilon, data_grad):# collect the element-wise sign of the data gradientsign_data_grad = data_grad.sign()# create the perturbed image by adjusting each pixel of the input image perturbed_image = image + epsilon * sign_data_grad # adding clipping to maintain [0, 1] range perturbed_image = torch.clamp(perturbed_image, 0, 1)# return the perturbed image return perturbed_image
Testing Function (测试函数)
def test(model, device, test_loader, epsilon):# accuracy countercorrect = 0adv_examples = []# loop over all examples in test set for data, target in test_loader:data, target = data.to(device), target.to(device)# Set requires_grad attribute of tensor. Important for Attackdata.requires_grad = True# output = model(data)init_pred = output.max(1, keepdim=True)[1]# if the initial prediction is wrong, don't botter attacking, just move onif init_pred.item() != target.item():continue # calculate the lossloss = F.nll_loss(output, target)# zero all existing gradmodel.zero_grad()# calculate gradients of model in backward loss loss.backward()# collect datagraddata_grad = data.grad.data # call FGSM attackperturbed_data = fgsm_attack(data, epsilon, data_grad)# reclassify the perturbed image output = model(perturbed_data)# check for success final_pred = output.max(1, keepdim=True)[1]# if final_pred.item() == target.item():correct += 1# special case for saving 0 epsilon examplesif (epsilon == 0) and (len(adv_examples) < 5):adv_ex = perturbed_data.squeeze().detach().cpu().numpy()adv_examples.append((init_pred.item(), final_pred.item(), adv_ex))else:# Save some adv examples for visualization laterif len(adv_examples) < 5:adv_ex = perturbed_data.squeeze().detach().cpu().numpy()adv_examples.append( (init_pred.item(), final_pred.item(), adv_ex) )# Calculate final accuracy for this epsilonfinal_acc = correct/float(len(test_loader))print("Epsilon: {}\tTest Accuracy = {} / {} = {}".format(epsilon, correct, len(test_loader), final_acc))# Return the accuracy and an adversarial examplereturn final_acc, adv_examples
Run Attack (执行攻击)
实现的最后一步是执行攻击,我们针对每个 epsilon 执行全部的 test step,并且保存最终的准确率和一些成功的对抗实例。 ϵ=0\epsilon=0ϵ=0 不执行攻击
accuracies = []
examples = []# Run test for each epsilon
for eps in epsilons:acc, ex = test(model, device, test_loader, eps)accuracies.append(acc)examples.append(ex)
Epsilon: 0 Test Accuracy = 9906 / 10000 = 0.9906
Epsilon: 0.05 Test Accuracy = 9517 / 10000 = 0.9517
Epsilon: 0.1 Test Accuracy = 8070 / 10000 = 0.807
Epsilon: 0.15 Test Accuracy = 4242 / 10000 = 0.4242
Epsilon: 0.2 Test Accuracy = 1780 / 10000 = 0.178
Epsilon: 0.25 Test Accuracy = 1292 / 10000 = 0.1292
Epsilon: 0.3 Test Accuracy = 1180 / 10000 = 0.118
Accuracy vs Epsilon (正确率 VS epsilon)
ϵ\epsilonϵ 增大时,我们期望正确率下降,因为大的 ϵ\epsilonϵ 我们在方向上有大的变换可以最大化 loss. 他们的变换不是线性的,一开始下降的慢,中间下降的快,最后下降的慢。
plt.figure(figsize=(5, 5))
plt.plot(epsilons, accuracies, "*-")
plt.yticks(np.arange(0, 1.1, step=0.1))
plt.xticks(np.arange(0, .35, step=0.05))
plt.title("Accuracy vs Epsilon")
plt.xlabel("Epsilon")
plt.ylabel("Accuracy")
plt.show()
Sample Adversarial Examples (对抗实例)
# Plot several examples of adversarial samples at each epsilon
cnt = 0
plt.figure(figsize=(8,10))
for i in range(len(epsilons)):for j in range(len(examples[i])):cnt += 1plt.subplot(len(epsilons),len(examples[0]),cnt)plt.xticks([], [])plt.yticks([], [])if j == 0:plt.ylabel("Eps: {}".format(epsilons[i]), fontsize=14)orig,adv,ex = examples[i][j]plt.title("{} -> {}".format(orig, adv))plt.imshow(ex, cmap="gray")
plt.tight_layout()
plt.show()
完整代码
import torch
import torch.nn as nn
import torch.nn.functional as F
import torch.optim as optim
from torchvision import datasets, transforms
import numpy as np
import matplotlib.pyplot as plt from six.moves import urllib
opener = urllib.request.build_opener()
opener.addheaders = [('User-agent', 'Mozilla/5.0')]
urllib.request.install_opener(opener) class Net(nn.Module):def __init__(self):super(Net, self).__init__()self.conv1 = nn.Conv2d(1, 32, 3, 1)self.conv2 = nn.Conv2d(32, 64, 3, 1)self.dropout1 = nn.Dropout(0.25)self.dropout2 = nn.Dropout(0.5)self.fc1 = nn.Linear(9216, 128)self.fc2 = nn.Linear(128, 10)def forward(self, x):x = self.conv1(x)x = F.relu(x)x = self.conv2(x)x = F.relu(x)x = F.max_pool2d(x, 2)x = self.dropout1(x)x = torch.flatten(x, 1)x = self.fc1(x)x = F.relu(x)x = self.dropout2(x)x = self.fc2(x)output = F.log_softmax(x, dim=1)return outputepsilons = [0, .05, .1, .15, .2, .25, .3]
pretrained_model = "lenet_mnist_model.pt"
use_cuda = True# MNIST Test dataset and dataloader declaration
test_loader = torch.utils.data.DataLoader(datasets.MNIST('../../../datasets', train=False, download=True, transform=transforms.Compose([transforms.ToTensor(),])),batch_size=1, shuffle=True)print("CUDA Available: ", torch.cuda.is_available())
device = torch.device('cuda' if (use_cuda and torch.cuda.is_available()) else 'cpu')# init network
model = Net().to(device)# load the pretrained model
model.load_state_dict(torch.load(pretrained_model, map_location='cpu'))# set the model in evaluation mode. In this case this is for the Dropout layers
model.eval()# FGSM attack code
def fgsm_attack(image, epsilon, data_grad):# collect the element-wise sign of the data gradientsign_data_grad = data_grad.sign()# create the perturbed image by adjusting each pixel of the input image perturbed_image = image + epsilon * sign_data_grad # adding clipping to maintain [0, 1] range perturbed_image = torch.clamp(perturbed_image, 0, 1)# return the perturbed image return perturbed_imagedef test(model, device, test_loader, epsilon):# accuracy countercorrect = 0adv_examples = []# loop over all examples in test setfor data, target in test_loader:data, target = data.to(device), target.to(device)# Set requires_grad attribute of tensor. Important for Attackdata.requires_grad = True#output = model(data)init_pred = output.max(1, keepdim=True)[1]# if the initial prediction is wrong, don't botter attacking, just move onif init_pred.item() != target.item():continue# calculate the lossloss = F.nll_loss(output, target)# zero all existing gradmodel.zero_grad()# calculate gradients of model in backward lossloss.backward()# collect datagraddata_grad = data.grad.data# call FGSM attackperturbed_data = fgsm_attack(data, epsilon, data_grad)# reclassify the perturbed imageoutput = model(perturbed_data)# check for successfinal_pred = output.max(1, keepdim=True)[1]#if final_pred.item() == target.item():correct += 1# special case for saving 0 epsilon examplesif (epsilon == 0) and (len(adv_examples) < 5):adv_ex = perturbed_data.squeeze().detach().cpu().numpy()adv_examples.append((init_pred.item(), final_pred.item(), adv_ex))else:# Save some adv examples for visualization laterif len(adv_examples) < 5:adv_ex = perturbed_data.squeeze().detach().cpu().numpy()adv_examples.append((init_pred.item(), final_pred.item(), adv_ex))# Calculate final accuracy for this epsilonfinal_acc = correct/float(len(test_loader))print("Epsilon: {}\tTest Accuracy = {} / {} = {}".format(epsilon, correct,len(test_loader), final_acc))# Return the accuracy and an adversarial examplereturn final_acc, adv_examplesaccuracies = []
examples = []# Run test for each epsilon
for eps in epsilons:acc, ex = test(model, device, test_loader, eps)accuracies.append(acc)examples.append(ex)plt.figure(figsize=(5, 5))
plt.plot(epsilons, accuracies, "*-")
plt.yticks(np.arange(0, 1.1, step=0.1))
plt.xticks(np.arange(0, .35, step=0.05))
plt.title("Accuracy vs Epsilon")
plt.xlabel("Epsilon")
plt.ylabel("Accuracy")
plt.show()# Plot several examples of adversarial samples at each epsilon
cnt = 0
plt.figure(figsize=(8, 10))
for i in range(len(epsilons)):for j in range(len(examples[i])):cnt += 1plt.subplot(len(epsilons), len(examples[0]), cnt)plt.xticks([], [])plt.yticks([], [])if j == 0:plt.ylabel("Eps: {}".format(epsilons[i]), fontsize=14)orig, adv, ex = examples[i][j]plt.title("{} -> {}".format(orig, adv))plt.imshow(ex, cmap="gray")
plt.tight_layout()
plt.show()
【参考】
ADVERSARIAL EXAMPLE GENERATION
相关文章:
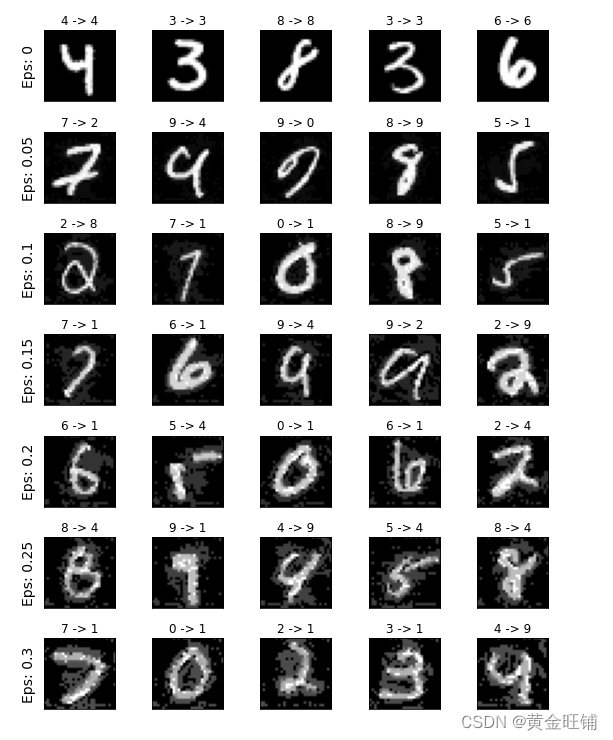
【PyTorch】教程:对抗学习实例生成
ADVERSARIAL EXAMPLE GENERATION 研究推动 ML 模型变得更快、更准、更高效。设计和模型的安全性和鲁棒性经常被忽视,尤其是面对那些想愚弄模型故意对抗时。 本教程将提供您对 ML 模型的安全漏洞的认识,并将深入了解对抗性机器学习这一热门话题。在图像…...
中国区使用Open AI账号试用Chat GPT指南
最近推出强大的ChatGPT功能,各大程序员使用后发出感叹:程序员要失业了 不过在国内并不支持OpenAI账号注册,多数会提示: OpenAI’s services are not available in your country. 经过一番搜索后,发现如下方案可以完…...
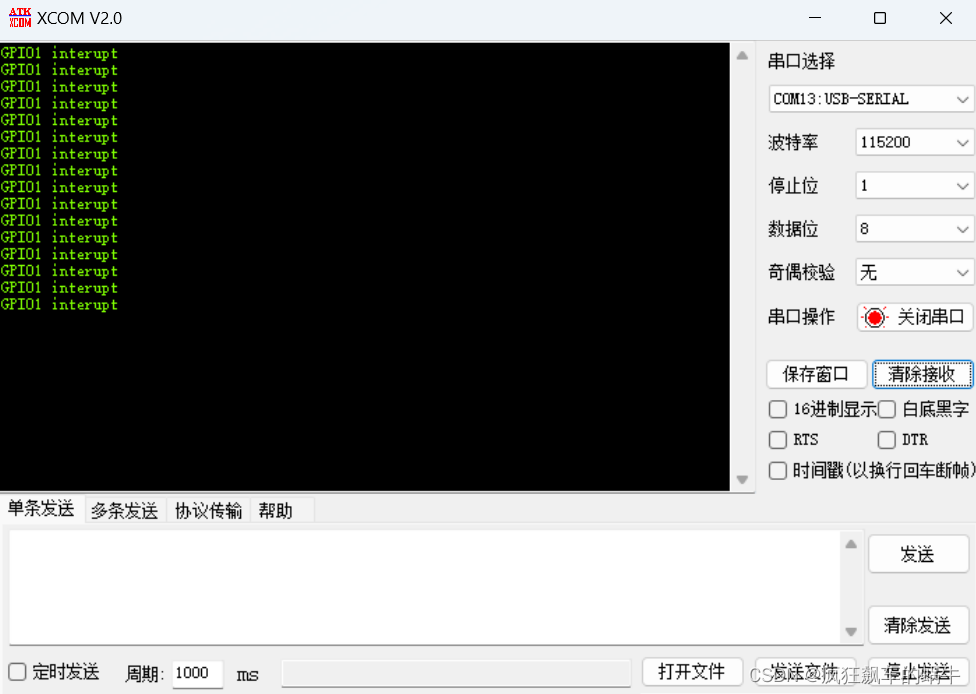
STM32开发(9)----CubeMX配置外部中断
CubeMX配置外部中断前言一、什么是中断1.STM32中断架构体系2.外部中断/事件控制器(EXTI)3.嵌套向量中断控制器(NIVC)二、实验过程1.CubeMX配置2.代码实现3.硬件连接4.实验结果总结前言 本章介绍使用STM32CubeMX对引脚的外部中断进…...
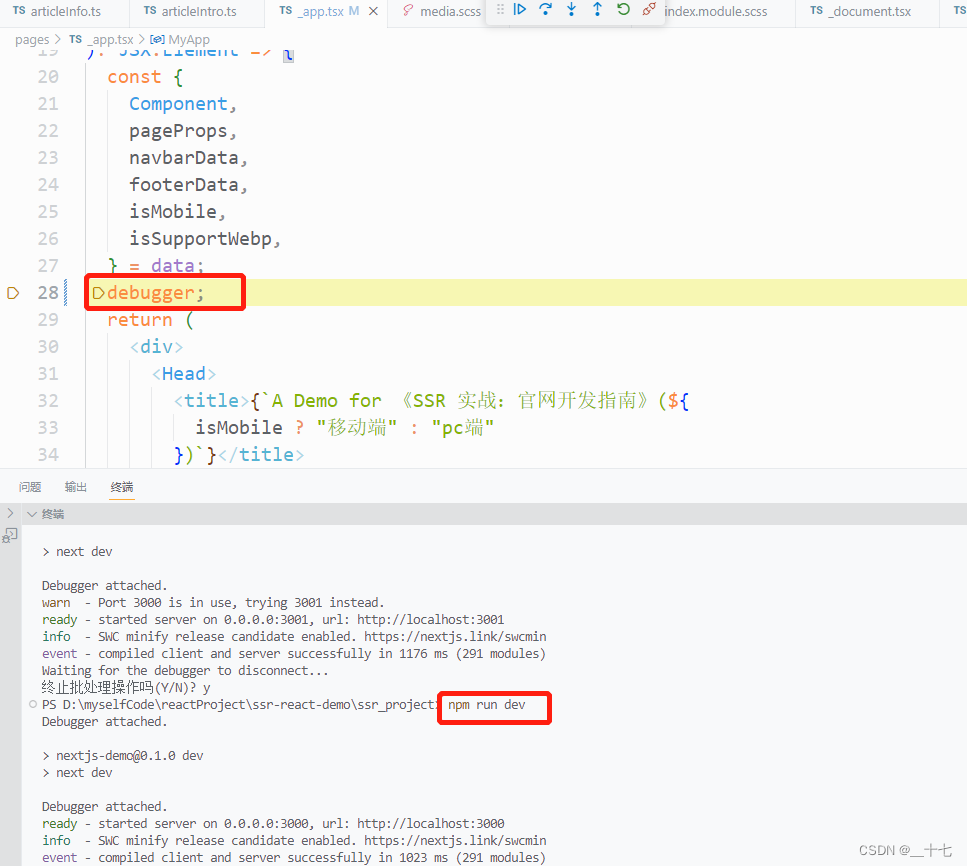
Nextjs了解内容
目录Next.jsnext.js的实现1,nextjs初始化2, 项目结构3, 数据注入getInitialPropsgetServerSidePropsgetStaticProps客户端注入3,CSS Modules4,layout组件5,文件式路由6,BFF层的文件式路由7&…...
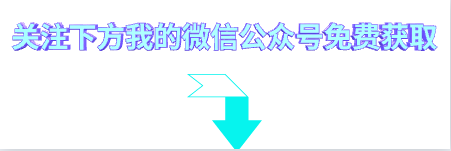
从事功能测试1年,裸辞1个月,找不到工作的“我”怎么办?
做功能测试一年多了裸辞职一个月了,大部分公司都要求有自动化测试经验,可是哪来的自动化测试呢? 我要是简历上写了吧又有欺诈性,不写他们给的招聘又要自动化优先,将项目带向自动化不是一个容易的事情,很多…...
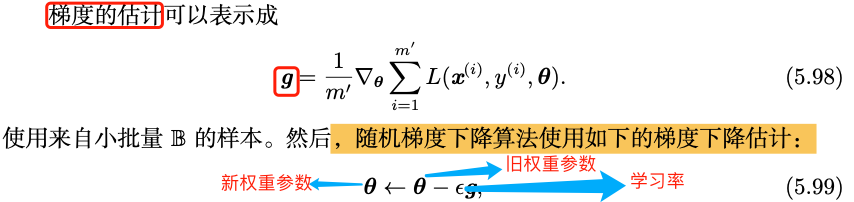
机器学习基本原理总结
本文大部分内容参考《深度学习》书籍,从中抽取重要的知识点,并对部分概念和原理加以自己的总结,适合当作原书的补充资料阅读,也可当作快速阅览机器学习原理基础知识的参考资料。 前言 深度学习是机器学习的一个特定分支。我们要想…...
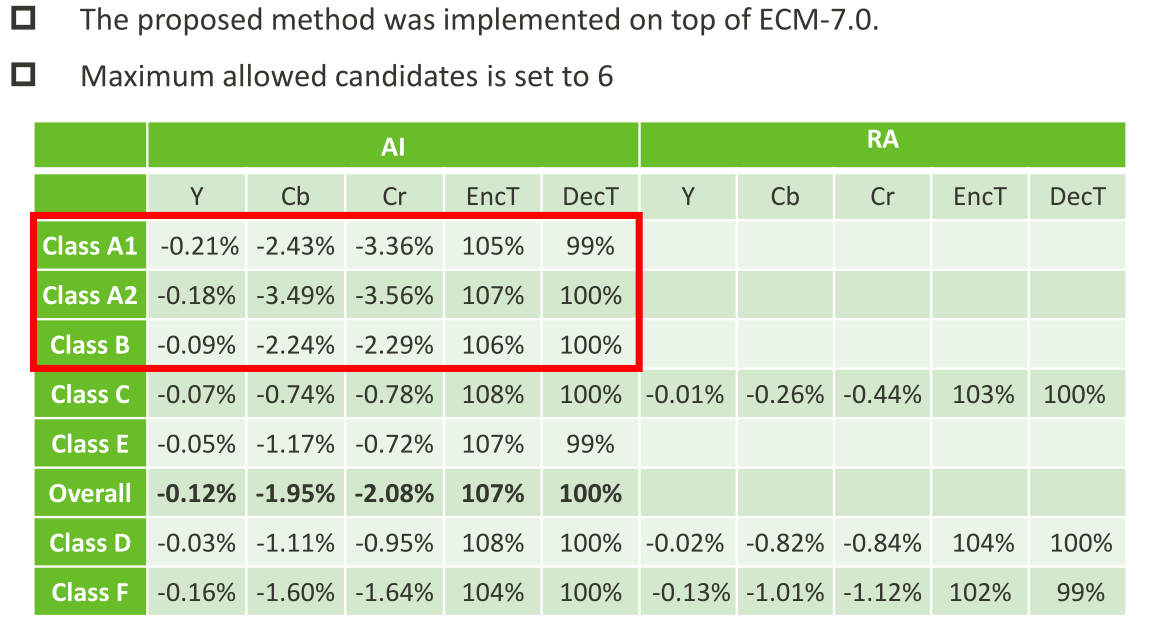
JVET-AC0315:用于色度帧内预测的跨分量Merge模式
ECM采用了许多跨分量的预测(Cross-componentprediction,CCP)模式,包括跨分量包括跨分量线性模型(CCLM)、卷积跨分量模型(CCCM)和梯度线性模型(GLM)࿰…...
Session与Cookie的区别(二)
脸盲症的困扰 小明身为杂货店的店长兼唯一的店员,所有大小事都是他一个人在处理。传统杂货店跟便利商店最大的差别在哪里?在于人情味。 就像是你去菜市场买菜的时候会被说帅哥或美女,或者是去买早餐的时候老板会问你:「一样&#…...
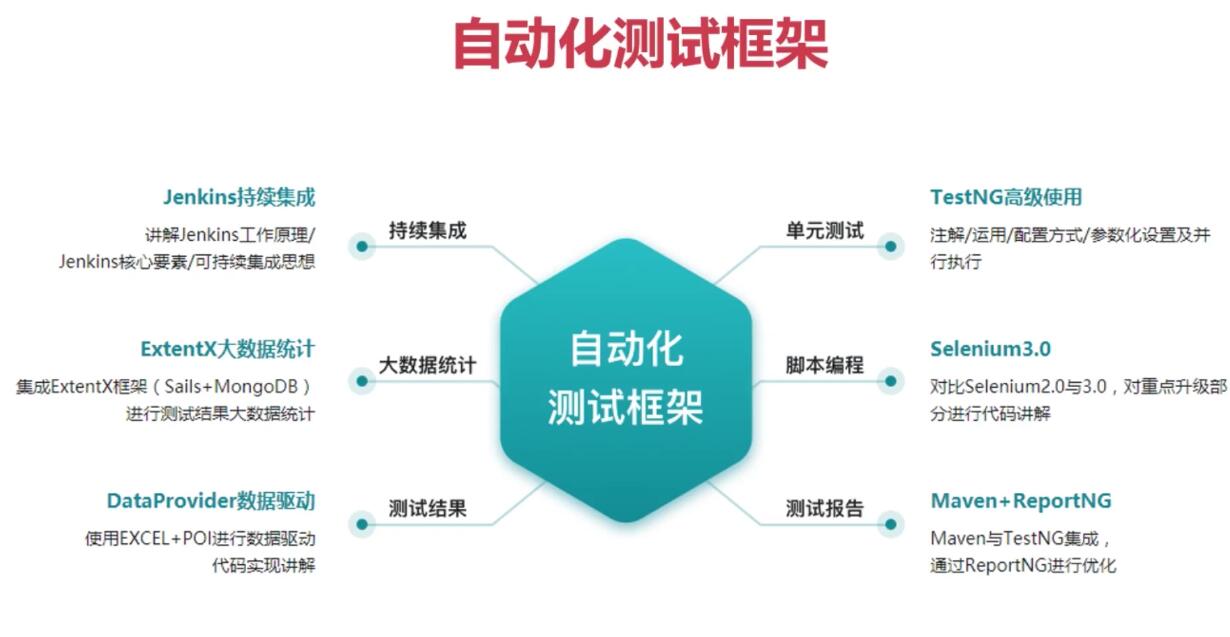
疫情开发,软件测试行情趋势是怎么样的?
如果说,2022年对于全世界来说,都是一场极大的挑战的话;那么,2023年绝对是机遇多多的一年。众所周知,随着疫情在全球范围内逐步得到控制,无论是国际还是国内的环境,都会呈现逐步回升的趋势&#…...
Java中间件描述与使用,面试可以用
myCat 用于切分mysql数据库(为什么要切分:当数据量过大时,mysql查询效率变低) ActiveMQ 订阅,消息推送 swagger 前后端分离,后台接口调式 dubbo 阿里的面向服务RPC框架,为什么要面向服务&#x…...
[OpenMMLab]AI实战营第七节课
语义分割代码实战教学 HRNet 高分辨率神经网络 安装配置 # 选择分支 git branch -a git switch 3.x # 配置环境 conda create -n mmsegmentation python3.8 conda activate mmsegmentation pip install torch1.11.0cu113 torchvision0.12.0cu113 torchaudio0.11.0 --extra-i…...
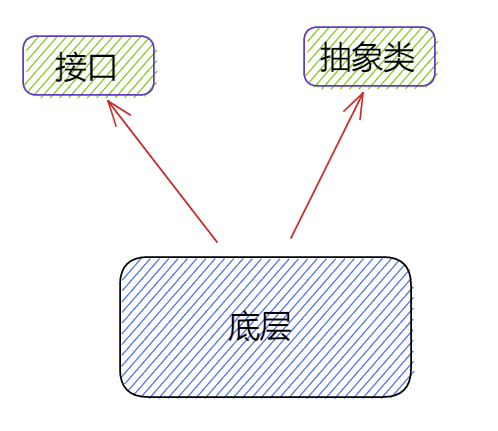
面向对象的设计模式
"万丈高楼平地起,7种模式打地基",模式是一种规范,我们应该站在巨人的肩膀上越看越远,接下来,让我们去仔细了解了解面向对象的7种设计模式7种设计模式设计原则的核心思想:找出应用中可能需要变化之…...
里氏替换原则|SOLID as a rock
文章目录 意图动机:违反里氏替换原则解决方案:C++中里氏替换原则的例子里氏替换原则的优点1、可兼容性2、类型安全3、可维护性在C++中用好LSP的标准费几句话本文是关于 SOLID as Rock 设计原则系列的五部分中的第三部分。 SOLID 设计原则侧重于开发 易于维护、可重用和可扩展…...
【C++】右左法则,指针、函数与数组
右左法则——判断复杂的声明对于一个复杂的声明,可以用右左法则判断它是个什么东西:1.先找到变量名称2.从变量名往右看一个部分,再看变量名左边的一个部分3.有小括号先看小括号里面的,一层一层往外看4.先看到的东西优先级大&#…...
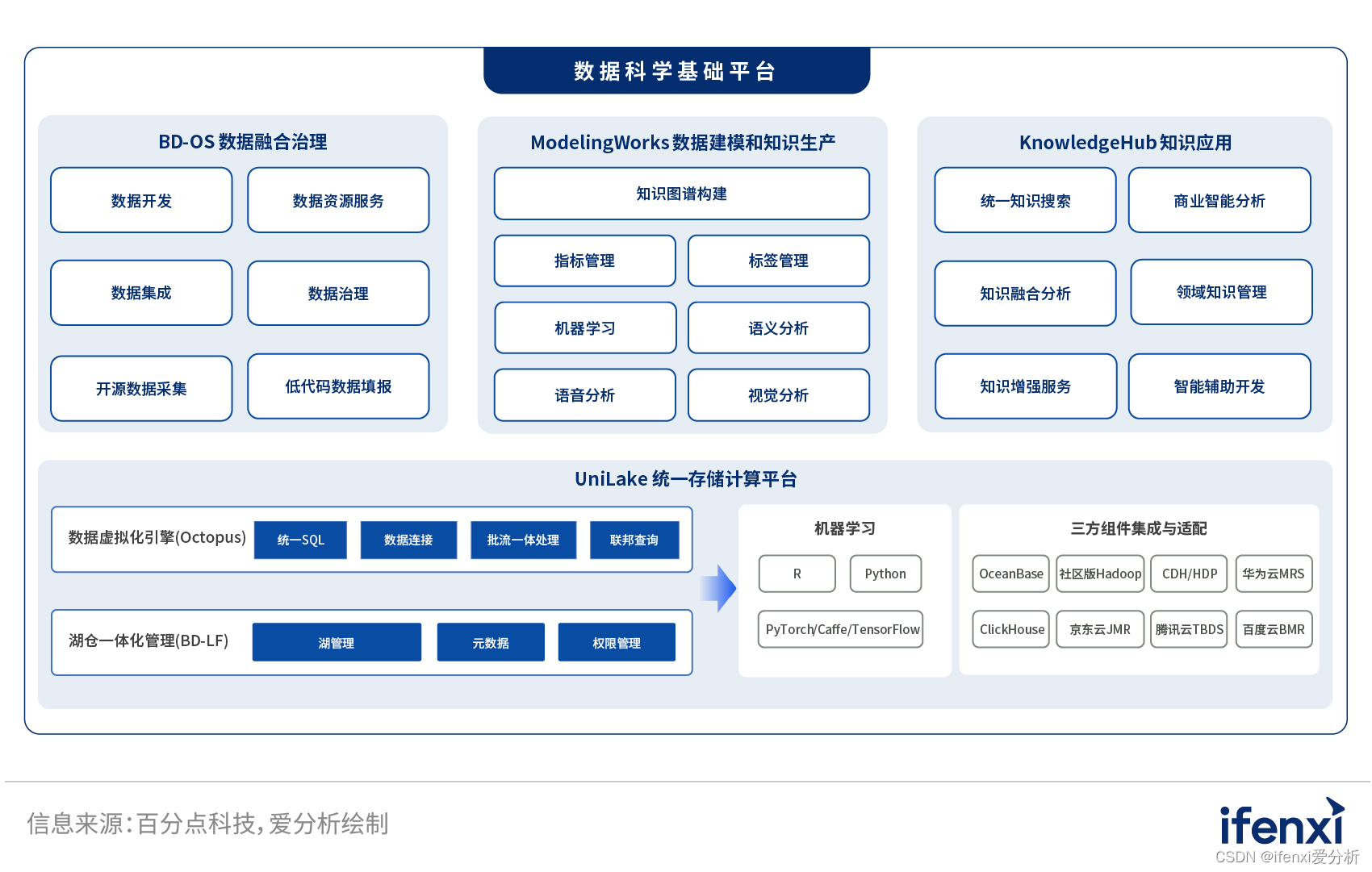
打通数据价值链,百分点数据科学基础平台实现数据到决策的价值转换 | 爱分析调研
随着企业数据规模的大幅增长,如何利用数据、充分挖掘数据价值,服务于企业经营管理成为当下企业数字化转型的关键。 如何挖掘数据价值?企业需要一步步完成数据价值链条的多个环节,如数据集成、数据治理、数据建模、数据分析、数据…...
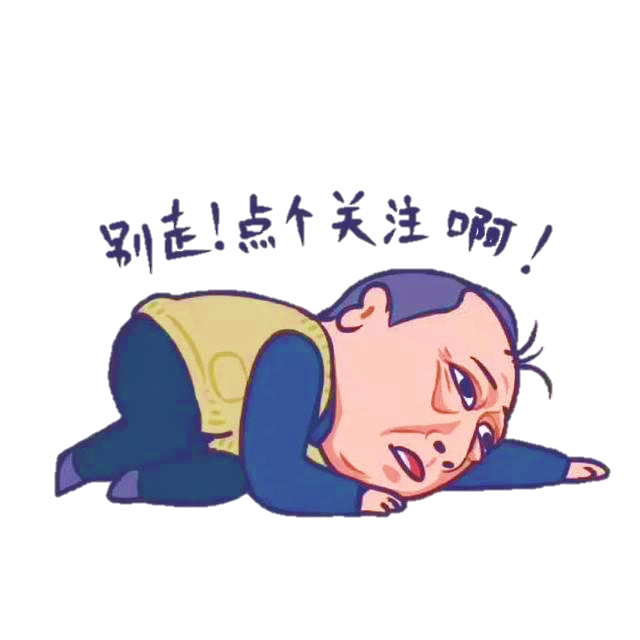
C++之多态【详细总结】
前言 想必大家都知道面向对象的三大特征:封装,继承,多态。封装的本质是:对外暴露必要的接口,但内部的具体实现细节和部分的核心接口对外是不可见的,仅对外开放必要功能性接口。继承的本质是为了复用&#x…...
ThingsBoard-RPC
1、使用 RPC 功能 ThingsBoard 允许您将远程过程调用 (RPC) 从服务器端应用程序发送到设备,反之亦然。基本上,此功能允许您向/从设备发送命令并接收命令执行的结果。本指南涵盖 ThingsBoard RPC 功能。阅读本指南后,您将熟悉以下主题: RPC 类型;基本 RPC 用例;RPC 客户端…...
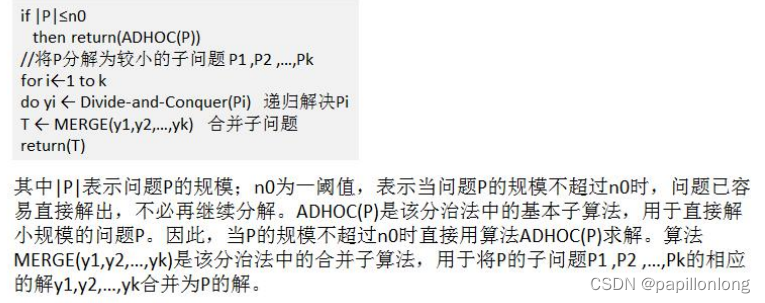
java分治算法
分治算法介绍 分治法是一种很重要的算法。字面上的解释是“分而治之”,就是把一个复杂的问题分成两个或更多的相同或 相似的子问题,再把子问题分成更小的子问题……直到最后子问题可以简单的直接求解,原问题的解即子问题 的解的合并。这个技…...
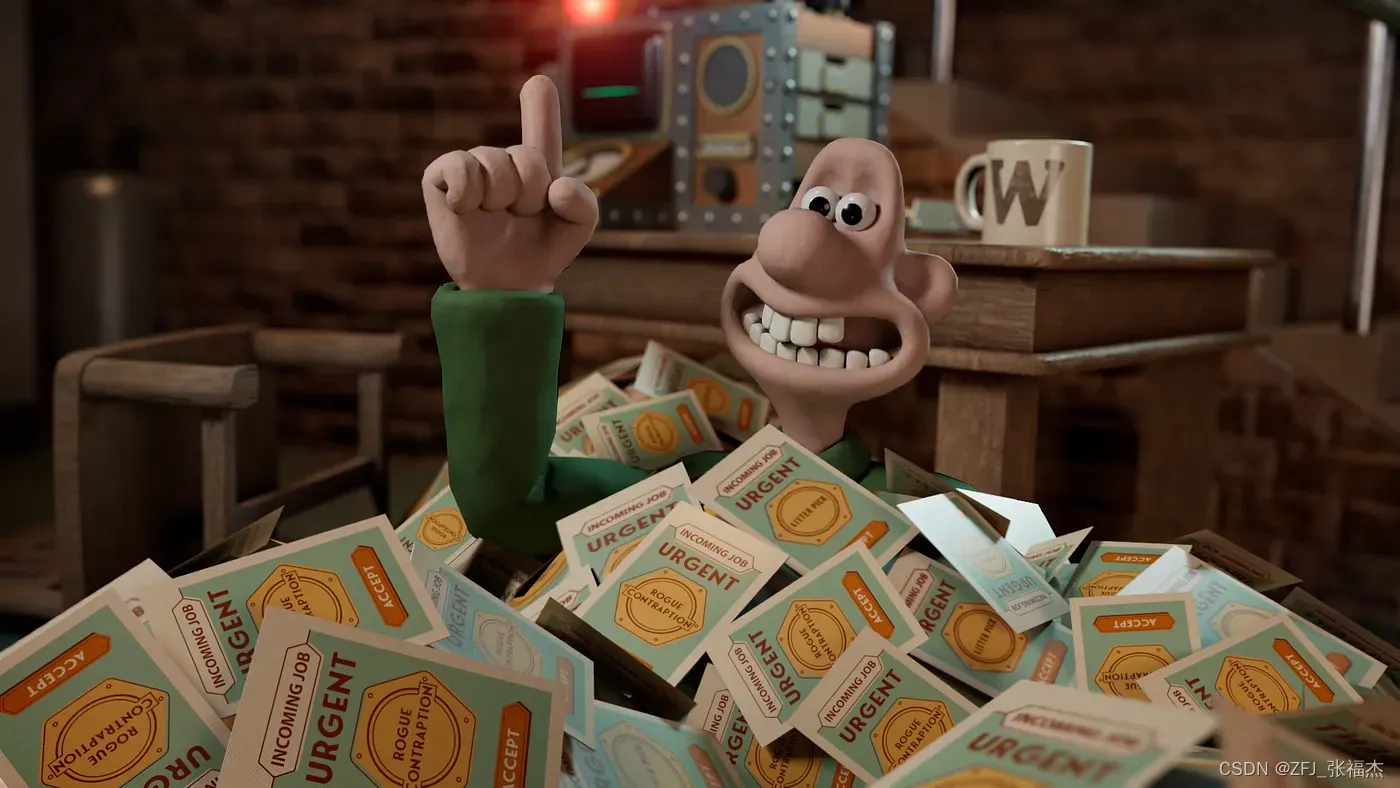
【Flutter】【Unity】使用 Flutter + Unity 构建(AR 体验工具包)
使用 Flutter Unity 构建(AR 体验工具包)【翻译】 原文:https://medium.com/potato/building-with-flutter-unity-ar-experience-toolkit-6aaf17dbb725 由于屡获殊荣的独立动画工作室 Aardman 与讲故事的风险投资公司 Fictioneers&#x…...
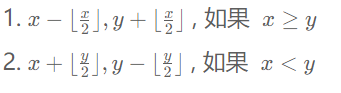
MC0108白给-MC0109新河妇荡杯
MC0108白给 小码哥和小码妹在玩一个游戏,初始小码哥拥有 x的金钱,小码妹拥有 y的金钱。 虽然他们不在同一个队伍中,但他们仍然可以通过游戏的货币系统进行交易,通过互相帮助以达到共赢的目的。具体来说,在每一回合&a…...
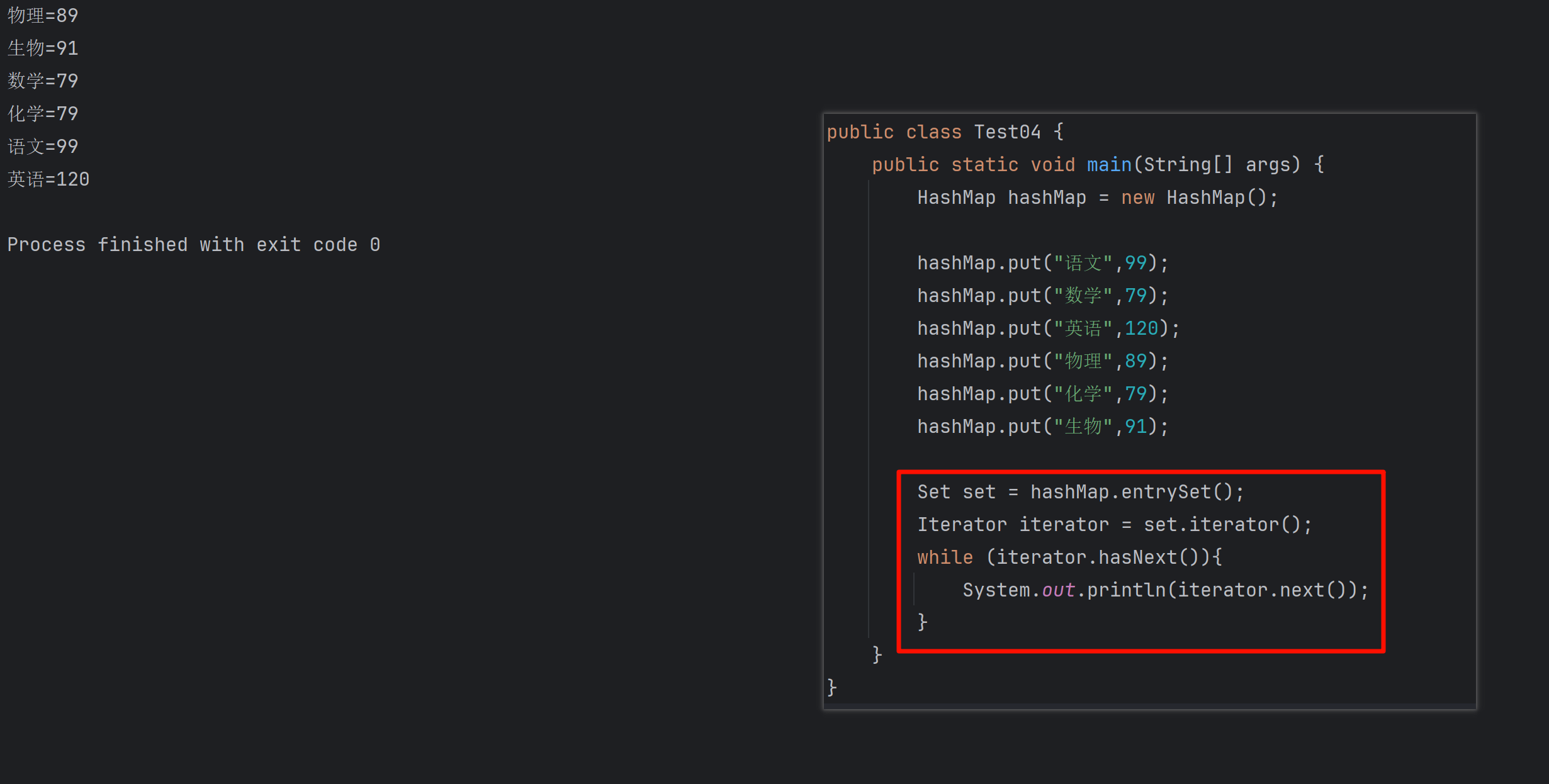
遍历 Map 类型集合的方法汇总
1 方法一 先用方法 keySet() 获取集合中的所有键。再通过 gey(key) 方法用对应键获取值 import java.util.HashMap; import java.util.Set;public class Test {public static void main(String[] args) {HashMap hashMap new HashMap();hashMap.put("语文",99);has…...
macOS多出来了:Google云端硬盘、YouTube、表格、幻灯片、Gmail、Google文档等应用
文章目录 问题现象问题原因解决办法 问题现象 macOS启动台(Launchpad)多出来了:Google云端硬盘、YouTube、表格、幻灯片、Gmail、Google文档等应用。 问题原因 很明显,都是Google家的办公全家桶。这些应用并不是通过独立安装的…...
MySQL用户和授权
开放MySQL白名单 可以通过iptables-save命令确认对应客户端ip是否可以访问MySQL服务: test: # iptables-save | grep 3306 -A mp_srv_whitelist -s 172.16.14.102/32 -p tcp -m tcp --dport 3306 -j ACCEPT -A mp_srv_whitelist -s 172.16.4.16/32 -p tcp -m tcp -…...
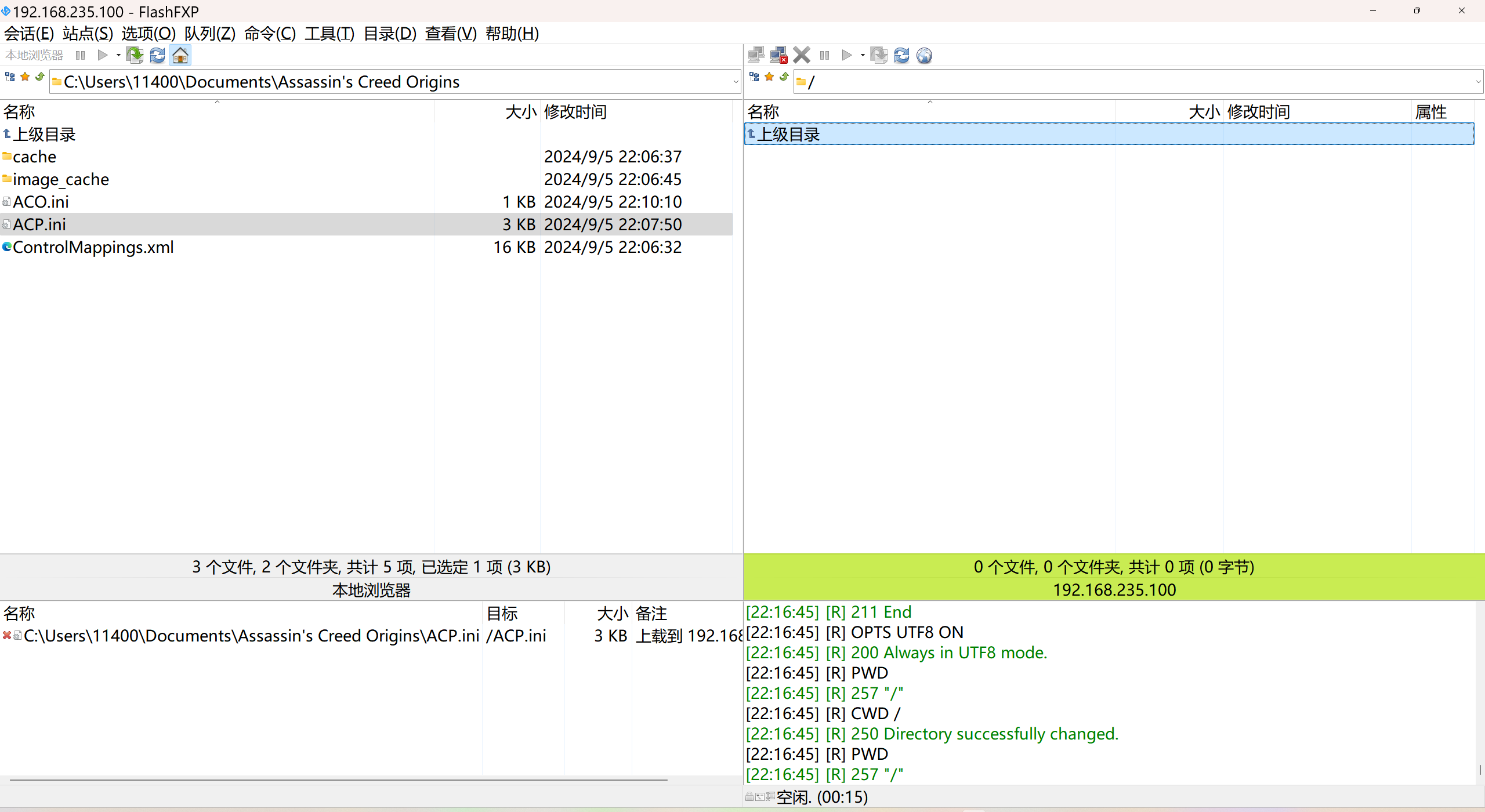
LINUX 69 FTP 客服管理系统 man 5 /etc/vsftpd/vsftpd.conf
FTP 客服管理系统 实现kefu123登录,不允许匿名访问,kefu只能访问/data/kefu目录,不能查看其他目录 创建账号密码 useradd kefu echo 123|passwd -stdin kefu [rootcode caozx26420]# echo 123|passwd --stdin kefu 更改用户 kefu 的密码…...
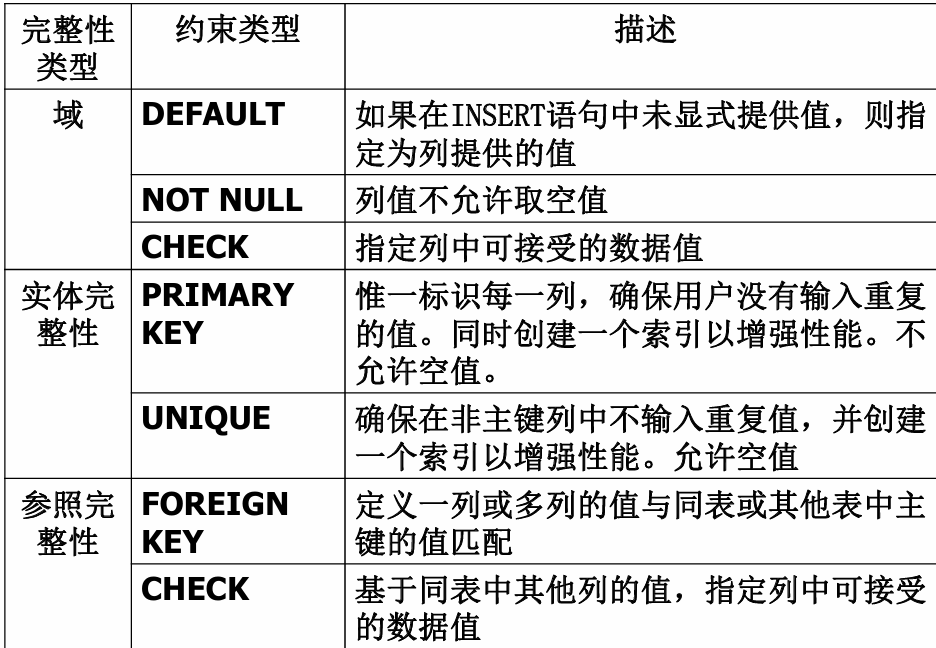
七、数据库的完整性
七、数据库的完整性 主要内容 7.1 数据库的完整性概述 7.2 实体完整性 7.3 参照完整性 7.4 用户定义的完整性 7.5 触发器 7.6 SQL Server中数据库完整性的实现 7.7 小结 7.1 数据库的完整性概述 数据库完整性的含义 正确性 指数据的合法性 有效性 指数据是否属于所定…...
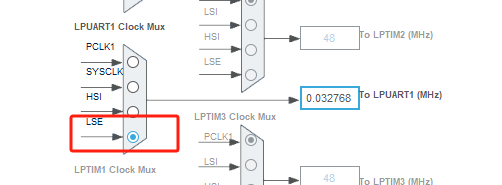
stm32wle5 lpuart DMA数据不接收
配置波特率9600时,需要使用外部低速晶振...
书籍“之“字形打印矩阵(8)0609
题目 给定一个矩阵matrix,按照"之"字形的方式打印这个矩阵,例如: 1 2 3 4 5 6 7 8 9 10 11 12 ”之“字形打印的结果为:1,…...
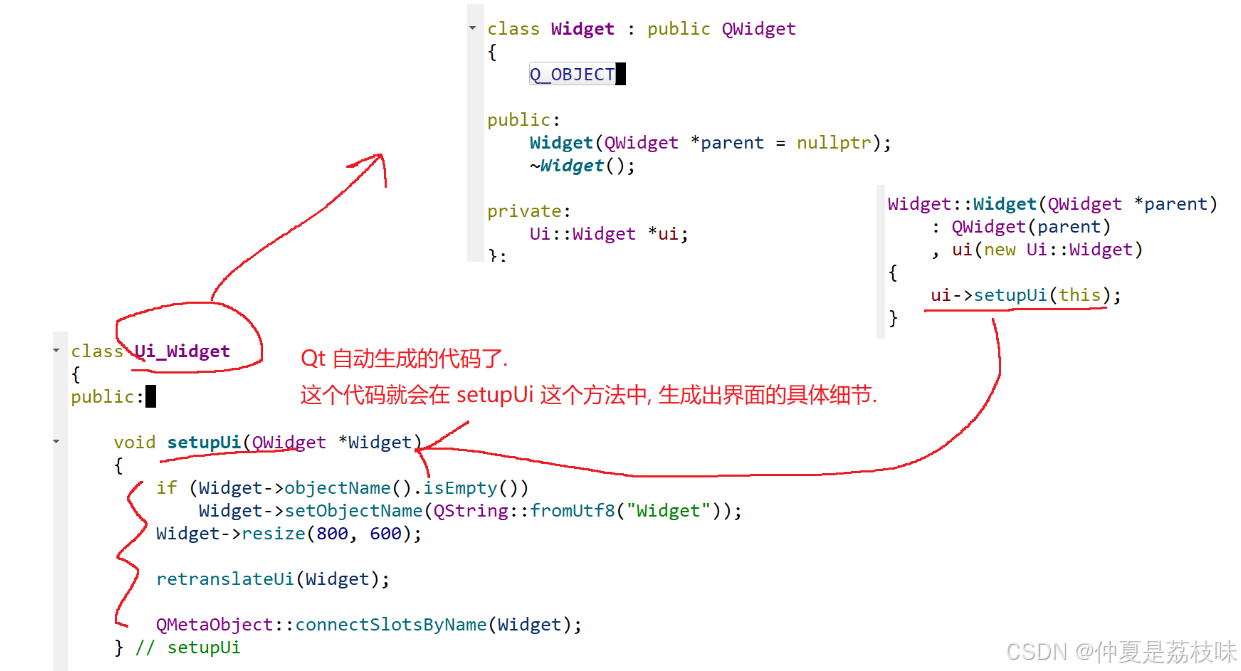
Qt的学习(一)
1.什么是Qt Qt特指用来进行桌面应用开发(电脑上写的程序)涉及到的一套技术Qt无法开发网页前端,也不能开发移动应用。 客户端开发的重要任务:编写和用户交互的界面。一般来说和用户交互的界面,有两种典型风格&…...
鸿蒙HarmonyOS 5军旗小游戏实现指南
1. 项目概述 本军旗小游戏基于鸿蒙HarmonyOS 5开发,采用DevEco Studio实现,包含完整的游戏逻辑和UI界面。 2. 项目结构 /src/main/java/com/example/militarychess/├── MainAbilitySlice.java // 主界面├── GameView.java // 游戏核…...
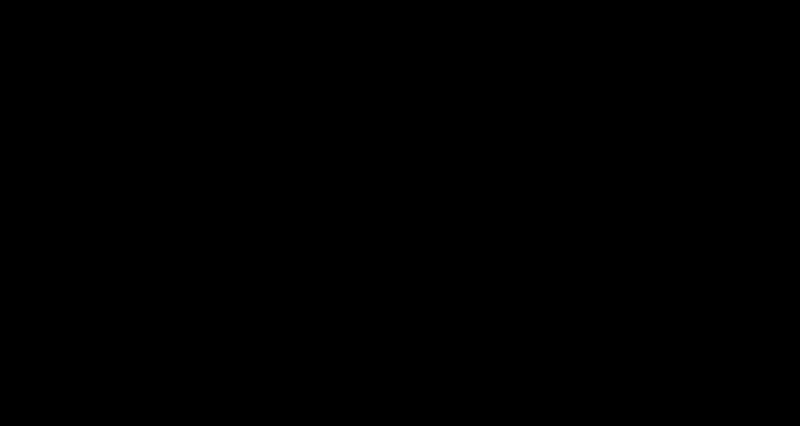
aardio 自动识别验证码输入
技术尝试 上周在发学习日志时有网友提议“在网页上识别验证码”,于是尝试整合图像识别与网页自动化技术,完成了这套模拟登录流程。核心思路是:截图验证码→OCR识别→自动填充表单→提交并验证结果。 代码在这里 import soImage; import we…...