用Flask搭建简单的web模型部署服务
目录结构如下:
分类模型web部署
classification.py
import os
import cv2
import numpy as np
import onnxruntime
from flask import Flask, render_template, request, jsonifyapp = Flask(__name__)onnx_session = onnxruntime.InferenceSession("mobilenet_v2.onnx", providers=['CPUExecutionProvider'])input_name = []
for node in onnx_session.get_inputs():input_name.append(node.name)output_name = []
for node in onnx_session.get_outputs():output_name.append(node.name)def allowed_file(filename):return '.' in filename and filename.rsplit('.', 1)[1] in set(['bmp', 'jpg', 'JPG', 'png', 'PNG'])def preprocess(image):if image.shape[0] < image.shape[1]: #h<wimage = cv2.resize(image, (int(256*image.shape[1]/image.shape[0]), 256))else:image = cv2.resize(image, (256, int(256*image.shape[0]/image.shape[1])))crop_size = min(image.shape[0], image.shape[1])left = int((image.shape[1]-crop_size)/2)top = int((image.shape[0]-crop_size)/2)image_crop = image[top:top+crop_size, left:left+crop_size]image_crop = cv2.resize(image_crop, (224,224))image_crop = image_crop[:,:,::-1].transpose(2,0,1).astype(np.float32) #BGR2RGB和HWC2CHWimage_crop[0,:] = (image_crop[0,:] - 123.675) / 58.395 image_crop[1,:] = (image_crop[1,:] - 116.28) / 57.12image_crop[2,:] = (image_crop[2,:] - 103.53) / 57.375return np.expand_dims(image_crop, axis=0) @app.route('/classification', methods=['POST', 'GET']) # 添加路由
def classification():if request.method == 'POST':f = request.files['file']if not (f and allowed_file(f.filename)):return jsonify({"error": 1001, "msg": "only support image formats: .bmp .png .PNG .jpg .JPG"})basepath = os.path.dirname(__file__) # 当前文件所在路径upload_path = os.path.join(basepath, 'static/images/temp.jpg') # 注意:没有的文件夹一定要先创建,不然会提示没有该路径f.save(upload_path)image = cv2.imread(upload_path) tensor = preprocess(image)inputs = {}for name in input_name:inputs[name] = tensor outputs = onnx_session.run(None, inputs)[0]label = np.argmax(outputs)score = np.exp(outputs[0][label]) / np.sum(np.exp(outputs), axis=1)return render_template('classification.html', label=label, score=score[0])return render_template('upload.html')if __name__ == '__main__':app.run(host='0.0.0.0', port=8000, debug=True)
classification.html
<!DOCTYPE html>
<html lang="en">
<head><meta charset="UTF-8">
</head>
<body><h1>请上传本地图片</h1><form action="" enctype='multipart/form-data' method='POST'><input type="file" name="file" style="margin-top:20px;"/><input type="submit" value="上传" class="button-new" style="margin-top:15px;"/></form><h2>图片类别为:{{label}} 置信度为:{{score}} </h2><img src="{{ url_for('static', filename= './images/temp.jpg') }}" alt="你的图片被外星人劫持了~~"/>
</body>
</html>
运行程序,在浏览器输入http://127.0.0.1:8000/classification,效果展示:
检测模型web部署
detection.py
import os
import cv2
import numpy as np
import onnxruntime
from flask import Flask, render_template, request, jsonifyapp = Flask(__name__)class_names = ['person', 'bicycle', 'car', 'motorcycle', 'airplane', 'bus', 'train', 'truck', 'boat', 'traffic light','fire hydrant', 'stop sign', 'parking meter', 'bench', 'bird', 'cat', 'dog', 'horse', 'sheep', 'cow','elephant', 'bear', 'zebra', 'giraffe', 'backpack', 'umbrella', 'handbag', 'tie', 'suitcase', 'frisbee','skis', 'snowboard', 'sports ball', 'kite', 'baseball bat', 'baseball glove', 'skateboard', 'surfboard','tennis racket', 'bottle', 'wine glass', 'cup', 'fork', 'knife', 'spoon', 'bowl', 'banana', 'apple','sandwich', 'orange', 'broccoli', 'carrot', 'hot dog', 'pizza', 'donut', 'cake', 'chair', 'couch','potted plant', 'bed', 'dining table', 'toilet', 'tv', 'laptop', 'mouse', 'remote', 'keyboard', 'cell phone','microwave', 'oven', 'toaster', 'sink', 'refrigerator', 'book', 'clock', 'vase', 'scissors', 'teddy bear','hair drier', 'toothbrush'] #coco80类别
input_shape = (640, 640)
score_threshold = 0.2
nms_threshold = 0.5
confidence_threshold = 0.2 onnx_session = onnxruntime.InferenceSession("yolov5n.onnx", providers=['CPUExecutionProvider'])input_name = []
for node in onnx_session.get_inputs():input_name.append(node.name)output_name = []
for node in onnx_session.get_outputs():output_name.append(node.name)def allowed_file(filename):return '.' in filename and filename.rsplit('.', 1)[1] in set(['bmp', 'jpg', 'JPG', 'png', 'PNG'])def nms(boxes, scores, score_threshold, nms_threshold):x1 = boxes[:, 0]y1 = boxes[:, 1]x2 = boxes[:, 2]y2 = boxes[:, 3]areas = (y2 - y1 + 1) * (x2 - x1 + 1)keep = []index = scores.argsort()[::-1] while index.size > 0:i = index[0]keep.append(i)x11 = np.maximum(x1[i], x1[index[1:]]) y11 = np.maximum(y1[i], y1[index[1:]])x22 = np.minimum(x2[i], x2[index[1:]])y22 = np.minimum(y2[i], y2[index[1:]])w = np.maximum(0, x22 - x11 + 1) h = np.maximum(0, y22 - y11 + 1) overlaps = w * hious = overlaps / (areas[i] + areas[index[1:]] - overlaps)idx = np.where(ious <= nms_threshold)[0]index = index[idx + 1]return keepdef xywh2xyxy(x):y = np.copy(x)y[:, 0] = x[:, 0] - x[:, 2] / 2y[:, 1] = x[:, 1] - x[:, 3] / 2y[:, 2] = x[:, 0] + x[:, 2] / 2y[:, 3] = x[:, 1] + x[:, 3] / 2return ydef filter_box(outputs): #过滤掉无用的框 outputs = np.squeeze(outputs)outputs = outputs[outputs[..., 4] > confidence_threshold]classes_scores = outputs[..., 5:]boxes = []scores = []class_ids = []for i in range(len(classes_scores)):class_id = np.argmax(classes_scores[i])outputs[i][4] *= classes_scores[i][class_id]outputs[i][5] = class_idif outputs[i][4] > score_threshold:boxes.append(outputs[i][:6])scores.append(outputs[i][4])class_ids.append(outputs[i][5])if len(boxes) == 0 :return boxes = np.array(boxes)boxes = xywh2xyxy(boxes)scores = np.array(scores)indices = nms(boxes, scores, score_threshold, nms_threshold) output = boxes[indices]return outputdef letterbox(im, new_shape=(416, 416), color=(114, 114, 114)):# Resize and pad image while meeting stride-multiple constraintsshape = im.shape[:2] # current shape [height, width]# Scale ratio (new / old)r = min(new_shape[0] / shape[0], new_shape[1] / shape[1])# Compute paddingnew_unpad = int(round(shape[1] * r)), int(round(shape[0] * r)) dw, dh = (new_shape[1] - new_unpad[0])/2, (new_shape[0] - new_unpad[1])/2 # wh padding top, bottom = int(round(dh - 0.1)), int(round(dh + 0.1))left, right = int(round(dw - 0.1)), int(round(dw + 0.1))if shape[::-1] != new_unpad: # resizeim = cv2.resize(im, new_unpad, interpolation=cv2.INTER_LINEAR)im = cv2.copyMakeBorder(im, top, bottom, left, right, cv2.BORDER_CONSTANT, value=color) # add borderreturn imdef scale_boxes(boxes, shape): # Rescale boxes (xyxy) from input_shape to shapegain = min(input_shape[0] / shape[0], input_shape[1] / shape[1]) # gain = old / newpad = (input_shape[1] - shape[1] * gain) / 2, (input_shape[0] - shape[0] * gain) / 2 # wh paddingboxes[..., [0, 2]] -= pad[0] # x paddingboxes[..., [1, 3]] -= pad[1] # y paddingboxes[..., :4] /= gainboxes[..., [0, 2]] = boxes[..., [0, 2]].clip(0, shape[1]) # x1, x2boxes[..., [1, 3]] = boxes[..., [1, 3]].clip(0, shape[0]) # y1, y2return boxesdef draw(image, box_data):box_data = scale_boxes(box_data, image.shape)boxes = box_data[...,:4].astype(np.int32) scores = box_data[...,4]classes = box_data[...,5].astype(np.int32)for box, score, cl in zip(boxes, scores, classes):top, left, right, bottom = boxcv2.rectangle(image, (top, left), (right, bottom), (255, 0, 0), 1)cv2.putText(image, '{0} {1:.2f}'.format(class_names[cl], score), (top, left), cv2.FONT_HERSHEY_SIMPLEX, 0.6, (0, 0, 255), 1)def preprocess(img):input = letterbox(img, input_shape)input = input[:, :, ::-1].transpose(2, 0, 1).astype(dtype=np.float32)input = input / 255.0input = np.expand_dims(input, axis=0)return input@app.route('/detection', methods=['POST', 'GET']) # 添加路由
def detection():if request.method == 'POST':f = request.files['file']if not (f and allowed_file(f.filename)):return jsonify({"error": 1001, "msg": "only support image formats: .bmp .png .PNG .jpg .JPG"})basepath = os.path.dirname(__file__) # 当前文件所在路径upload_path = os.path.join(basepath, 'static/images/temp.jpg') # 注意:没有的文件夹一定要先创建,不然会提示没有该路径f.save(upload_path)image = cv2.imread(upload_path) tensor = preprocess(image)inputs = {}for name in input_name:inputs[name] = tensor outputs = onnx_session.run(None, inputs)[0]boxes = filter_box(outputs)if boxes is not None:draw(image, boxes)cv2.imwrite(os.path.join(basepath, 'static/images/temp.jpg'), image)return render_template('detection.html')return render_template('upload.html')if __name__ == '__main__':app.run(host='0.0.0.0', port=8000, debug=True)
detection.html
<!DOCTYPE html>
<html lang="en">
<head><meta charset="UTF-8">
</head>
<body><h1>请上传本地图片</h1><form action="" enctype='multipart/form-data' method='POST'><input type="file" name="file" style="margin-top:20px;"/><input type="submit" value="上传" class="button-new" style="margin-top:15px;"/></form><img src="{{ url_for('static', filename= './images/temp.jpg') }}" alt="你的图片被外星人劫持了~~"/>
</body>
</html>
运行程序,在浏览器输入http://127.0.0.1:8000/detection,效果展示:
相关文章:
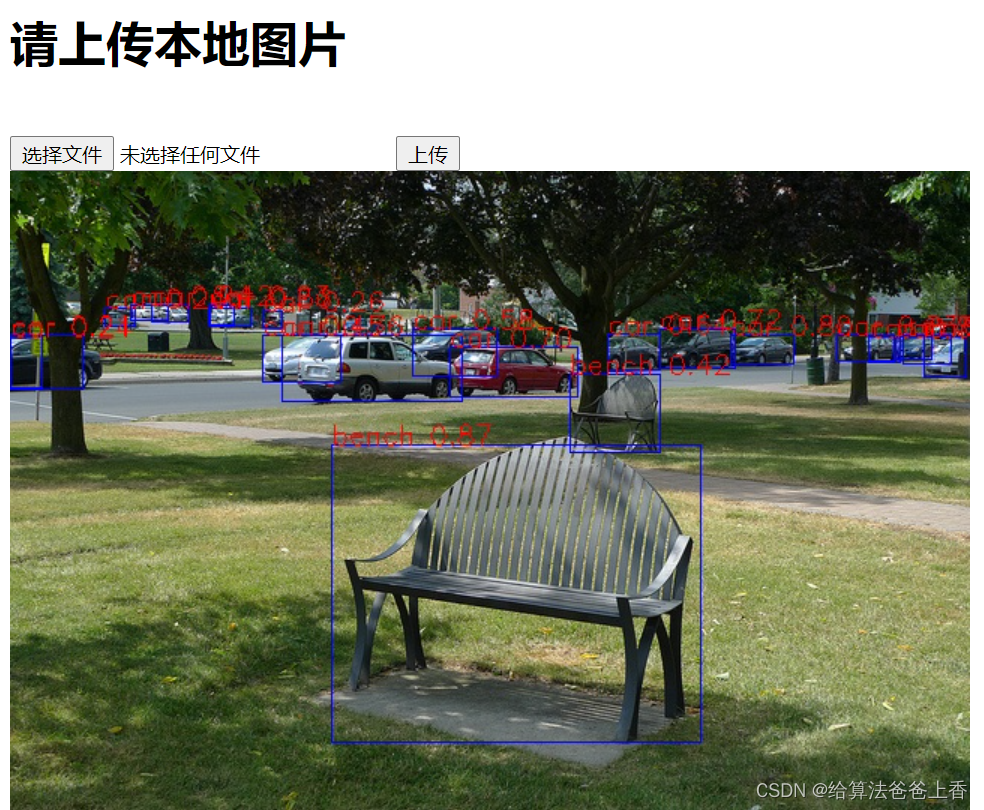
用Flask搭建简单的web模型部署服务
目录结构如下: 分类模型web部署 classification.py import os import cv2 import numpy as np import onnxruntime from flask import Flask, render_template, request, jsonifyapp Flask(__name__)onnx_session onnxruntime.InferenceSession("mobilen…...

PCL 点云匹配 3 之 (非线性迭代点云匹配)lM-ICP
一、IM迭代法 PCL IterativeClosestPointNonLinear 非线性L-M迭代法-CSDN博客 Matlab 非线性迭代法(3)阻尼牛顿法 L-M-CSDN博客 MATLAB实现最小二乘法_matlab最小二乘法-CSDN博客...

【C语言】SCU安全项目2-BufBomb
目录 关键代码解读: getxs() getbuf() test() 核心思路 具体操作1 具体操作2 前段时间忙于强网杯、英语4级和一些其他支线,有点摸不清头绪了,特别是qwb只有一个输出,太过坐牢,决定这个安全项目做完后就继续投身…...
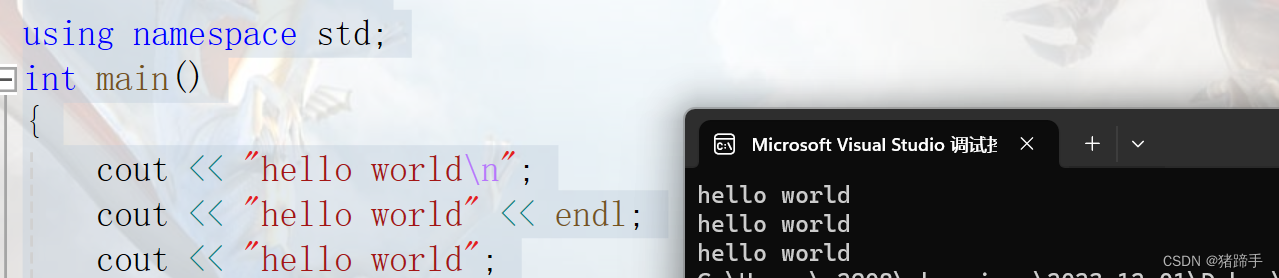
C++第一讲之初入C++
注:本文是对于学完C语言再学C同学的讲解,主要补充C与C语言不同之处,如果你没学过C语言,不建议观看本文。 一.C简介 我们都知道C语言是过程性语言(强调的是实现过程),即对计算机语言要处理的两…...
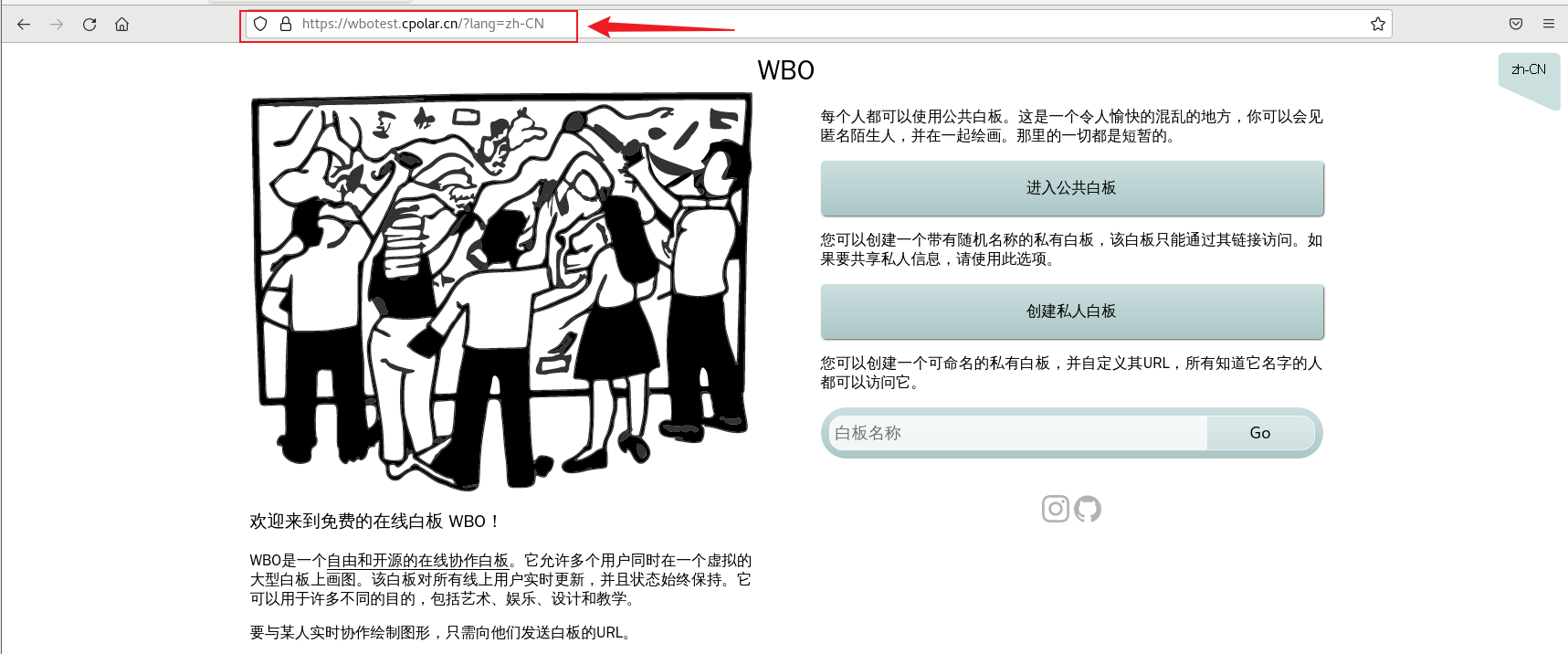
如何实现公网访问本地内网搭建的WBO白板远程协作办公【内网穿透】
最近,我发现了一个超级强大的人工智能学习网站。它以通俗易懂的方式呈现复杂的概念,而且内容风趣幽默。我觉得它对大家可能会有所帮助,所以我在此分享。点击这里跳转到网站。 文章目录 前言1. 部署WBO白板2. 本地访问WBO白板3. Linux 安装cp…...
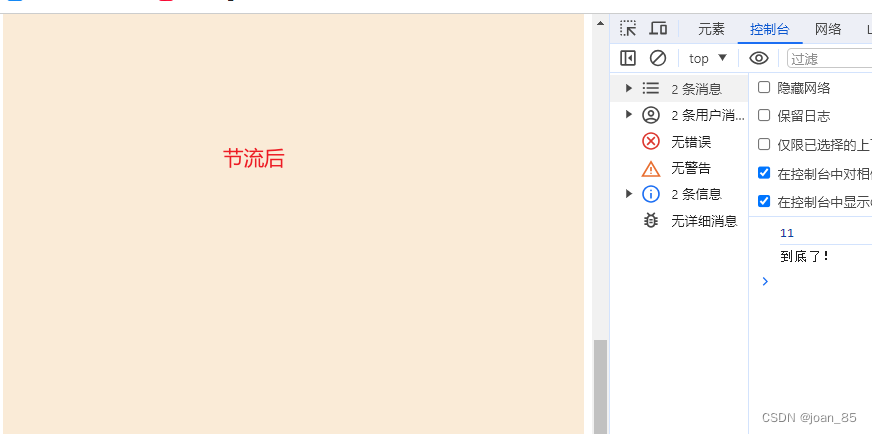
js知识点1:防抖节流
js知识点1:防抖节流 防抖节流 防抖节流,本质上是优化高频率执行代码的一种手段 定义: 防抖: n 秒后再执行该事件,若在 n 秒内被重复触发,则重新计时 节流: n 秒内只运行一次,若在 n 秒内重复触发࿰…...
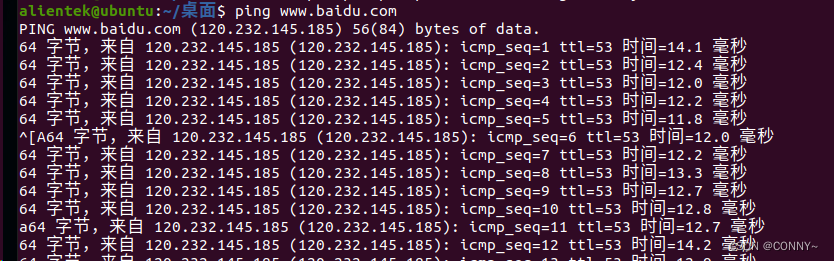
虚拟机下Ubuntu上网设置
文章目录 一、虚拟机上网的两种方式1.1 NAT模式(Network Address Translation)1.2 桥接模式(Bridge Mode)1.3 简介 二、实际配置2.1 NAT模式配置2.2 桥接模式配置 之前跟着博客配了好几个也没用,后来自己慢慢模式实践测…...
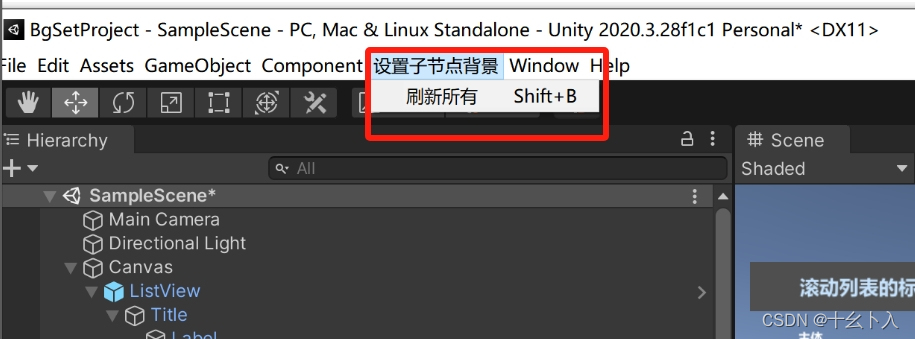
Unity3d C#利用Editor编辑器拓展实现配置UI背景样式一键设置UI背景样式功能(含源码)
前言 在开发UI滚动列表的时候,经常会有每项的背景图不统一的情况,会间隔重复的情况居多。这种情况下,手动去设置间隔一行的背景图或者颜色是比较麻烦的。在此背景下,笔者尝试写个小工具,在搭建UI时配置一下循环背景的…...

【微服务】服务间调用
当我们的应用从一个大单体拆分成多个微服务之后,服务间调用有多少种方式?服务间调用如果出现超时,如果避免雪崩,即如何做限流熔断机制,原理是什么? 服务间调用方式 OpenFeign 使用Feign进行服务间调用,我们会在启动类加@EnableFeignClients启用。 package org.sprin…...

一句话分清C/C++声明和定义
定义告诉编译器在在哪个位置存储变量,声明没有 声明:告诉编译器,变量类型和名字 定义:告诉编译器变量存储的位置。 举例子 int i; // 这是声明定义。声明:告诉编译器变量类型int,变量名字i, // 定义&…...

《哥德尔证明》阅读笔记——初等命题逻辑的一致性证明过程
前言 前两节主要阐述了公理系统的发展历史,一致性问题的提出,以及希尔伯特的洞见,本节将给出哥德尔证明所需的最后一次具体背景阐述,包含两个问题:一是罗素所著的《数学原理》是为何而写?二是从数学原理中…...
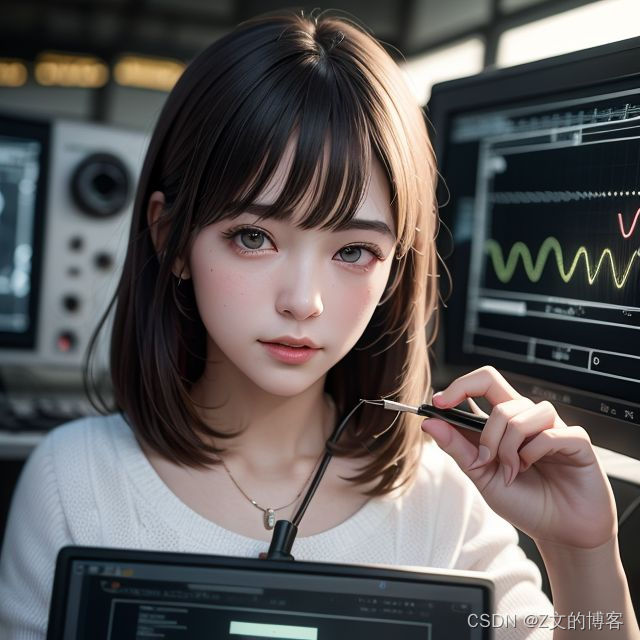
迪文屏开发保姆级教程—弹出键盘录入ASCII字符
本篇文章主要介绍了在DGBUS平台上使用文本录入键盘录入ASCII字符,数字和字母的教程。 文本录入键盘支持录入ASCII字符,数字和字母。 官方开发指南PDF:(不方便下载的私聊我发给你) https://download.csdn.net/download…...

Java,处理字符串的案例()
场景 为了得到一个都是英雄名字的字符数组,需要对如下字符串进行处理 疾风剑豪,影流之主,封魔剑魂,暗裔剑魔,无极剑圣,无双剑姬,武器大师,德邦总管,蛮族之王࿰…...

微信小程序更新机制
1/同步更新 1、定期检查更新时; 2、长期未使用,首次进入会同步更新,但在弱网或者下载新版本失败的情况下,还会使用旧版本。 2/异步更新: 启动时异步更新 3/开发者手动触发更新 在启动时异步更新的情况下,…...
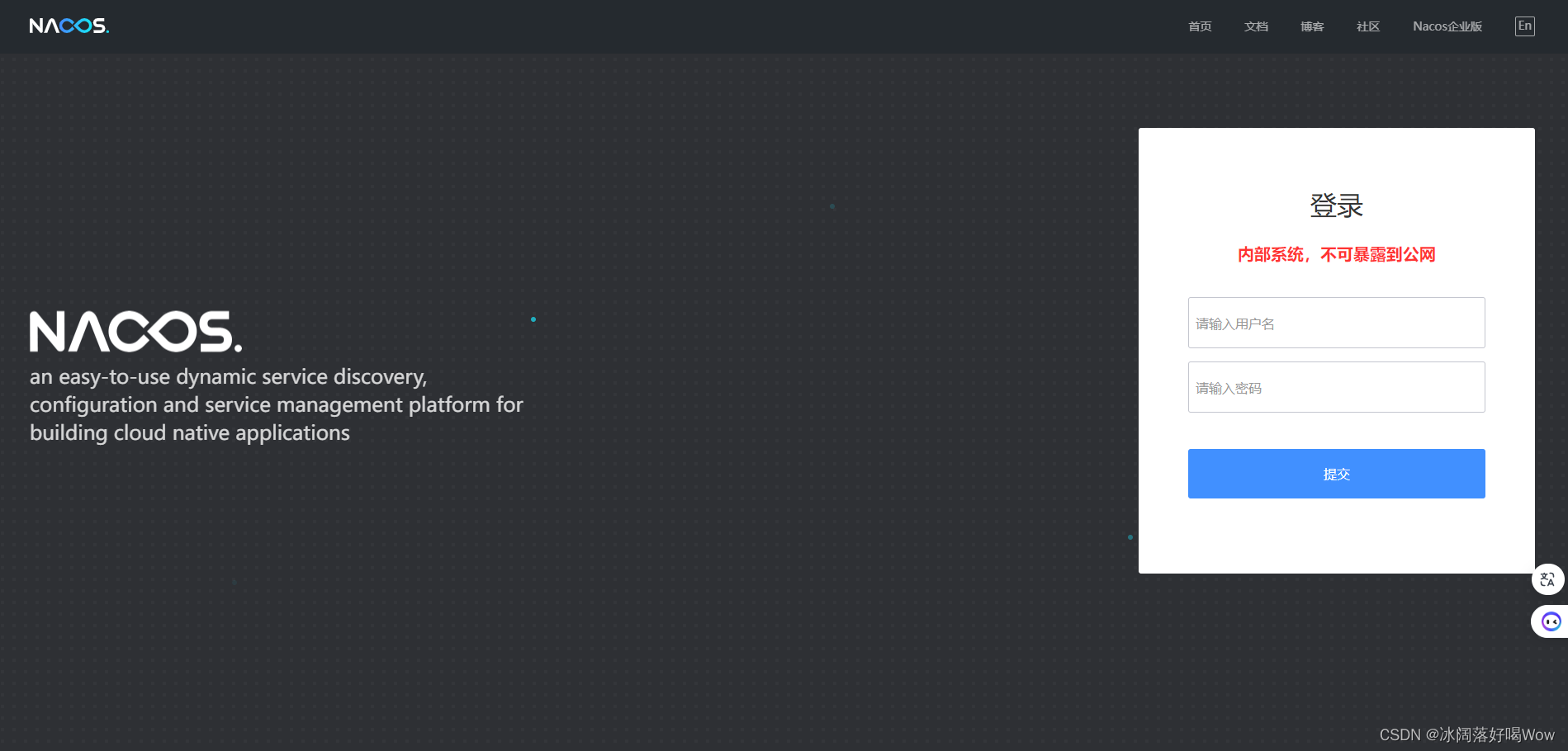
CentOS 7 部署 Nacos-2.3.0 (单机版)
CentOS 7 部署 Nacos-2.3.0 (单机版) 1. 下载 Nacos 安装包 历史版本:https://github.com/alibaba/nacos/releases/ 我选的是 2.3.0 版本,https://github.com/alibaba/nacos/releases/download/2.3.0/nacos-server-2.3.0.tar.g…...
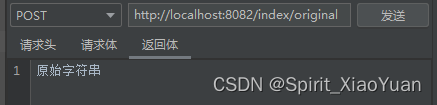
Springboot优雅实现对接口返回统一封装
前端在调用后端接口时往往不同的接口返回的数据是不一样的,但是通常我们会与前端约定一个固定的返回格式,通过固定的格式告诉他们什么时候接口是返回成功,什么时候返回失败,返回成功后他们如何拿到接口返回的数据去渲染前端页面。…...
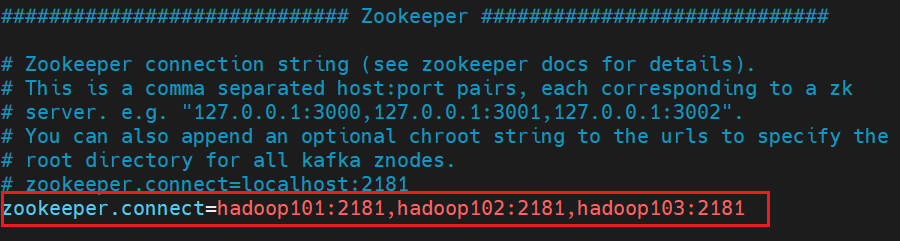
Kafka 安装与部署
目录 Kafka 下载 (1)将 kafka_2.11-2.4.1.tgz 上传至 /opt/software/ (2)解压安装包至 /opt/module/ [huweihadoop101 ~]$ cd /opt/software/ [huweihadoop101 software]$ tar -zxvf kafka_2.11-2.4.1.tgz -C ../module/&#…...

计算 N*4*4 位姿形状的逆变换,在N*3*4位姿后补充 [0,0,0,1]
针对 [N,4,4] shape 的 poses,函数 ComputeInversePoses 返回 相同 shape,但是每个 pose 都是前面的 逆 pose。 针对 [N,3,4] shape 的 poses,函数 AddIdentityToPoses 返回 在每个 [3,4] pose下加上 [0,0,0,1] 后的pose,返回的…...
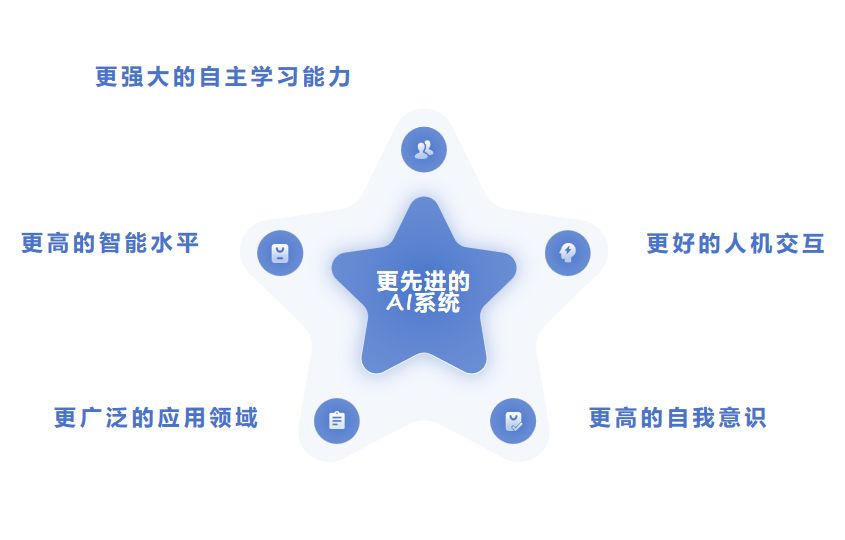
人工智能可以战胜人类智慧大脑么?
引言 在当今快速发展的科技时代,人工智能的进步日新月异,引发了一场深刻的讨论:能否有一天,人工智能能够超越甚至战胜人类智慧?这个问题涉及到人类认知的广泛领域,牵涉到人类思维的深层次特质以及AI技术在…...

【数据结构和算法】 K 和数对的最大数目
其他系列文章导航 Java基础合集数据结构与算法合集 设计模式合集 多线程合集 分布式合集 ES合集 文章目录 其他系列文章导航 文章目录 前言 一、题目描述 二、题解 2.1 方法一:双指针排序 三、代码 3.1 方法一:双指针排序 3.2 方法二࿱…...
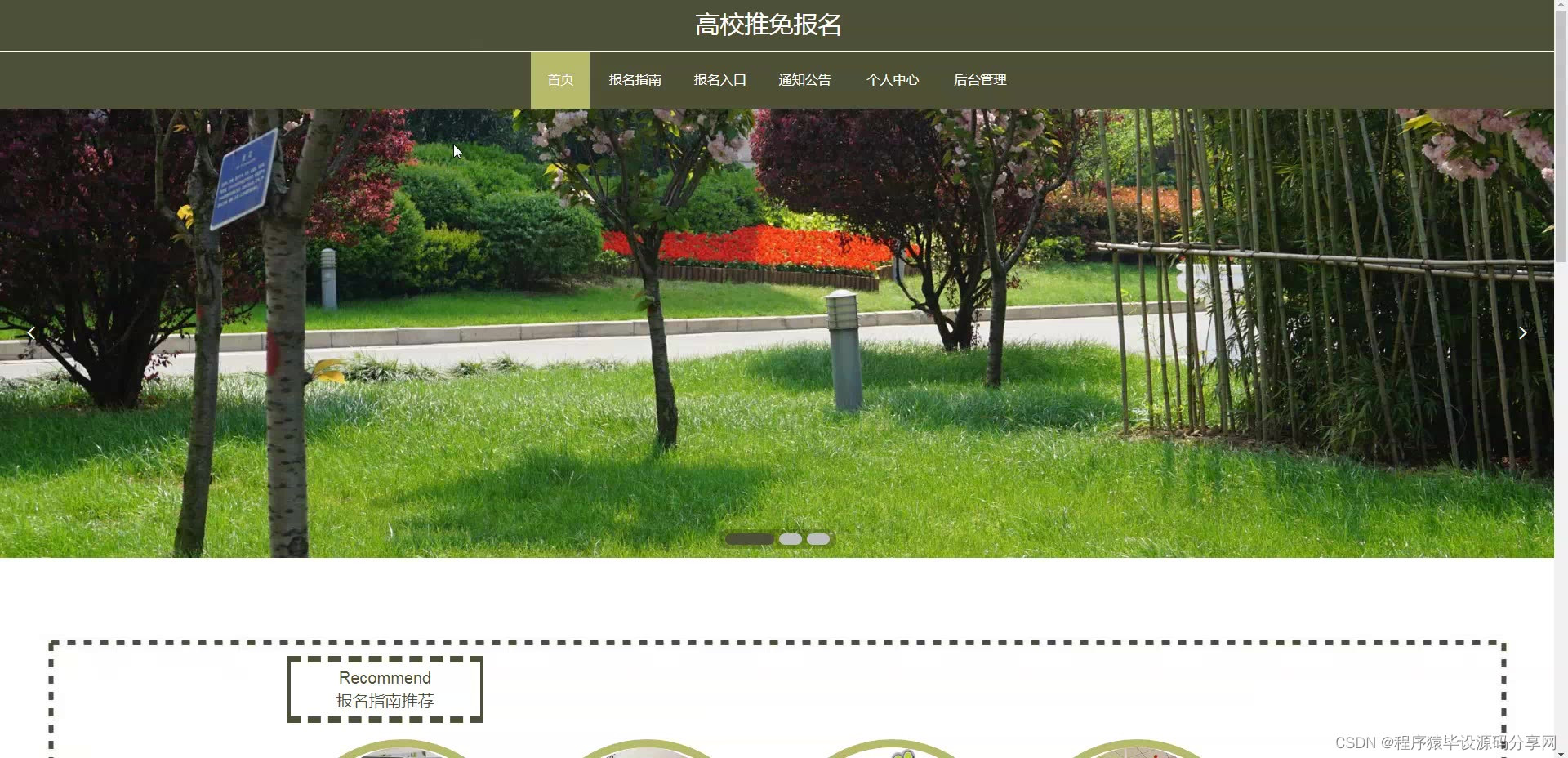
基于ssm高校推免报名系统源码和论文
网络的广泛应用给生活带来了十分的便利。所以把高校推免报名管理与现在网络相结合,利用java技术建设高校推免报名管理系统,实现高校推免报名的信息化。则对于进一步提高高校推免报名管理发展,丰富高校推免报名管理经验能起到不少的促进作用。…...
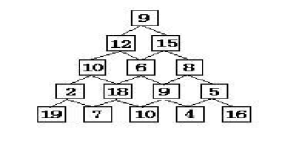
算法设计与分析2023秋-头歌实验-实验七 动态规划
文章目录 第1关:数塔问题任务描述相关知识编程要求解题思路测试说明参考答案 第2关:最长公共子序列任务描述相关知识编程要求解题思路:测试说明参考答案 第3关:求序列-2 11 -4 13 -5 -2的最大子段和任务描述相关知识编程要求解题思…...

复杂 SQL 实现分组分情况分页查询
其他系列文章导航 Java基础合集数据结构与算法合集 设计模式合集 多线程合集 分布式合集 ES合集 文章目录 其他系列文章导航 文章目录 前言 一、根据 camp_status 字段分为 6 种情况 1.1 SQL语句 1.2 SQL解释 二、分页 SQL 实现 2.1 SQL语句 2.2 根据 camp_type 区分返…...

JavaScript---如何完美的判断返回对象是否有值
如何判断一个对象为空是我们在开发中经常会遇到的问题,今天我们来聊聊几种经常使用的方法,以及在不同的场景下我们如何去使用。 1. JSON.stringify JSON.stringify 方法可以使对象序列化,转为相应的 JSON 格式。 js 复制代码 const obj {…...
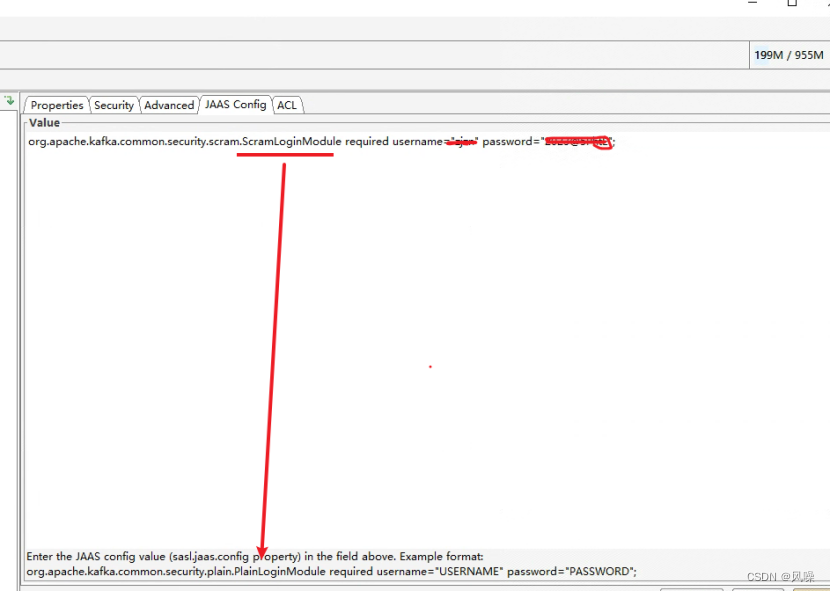
kafka offset sasl加密连接
kafka-tool(offset) 进行SCRAM连接,直接上图 填写jaas的认证(账密 引用包)...
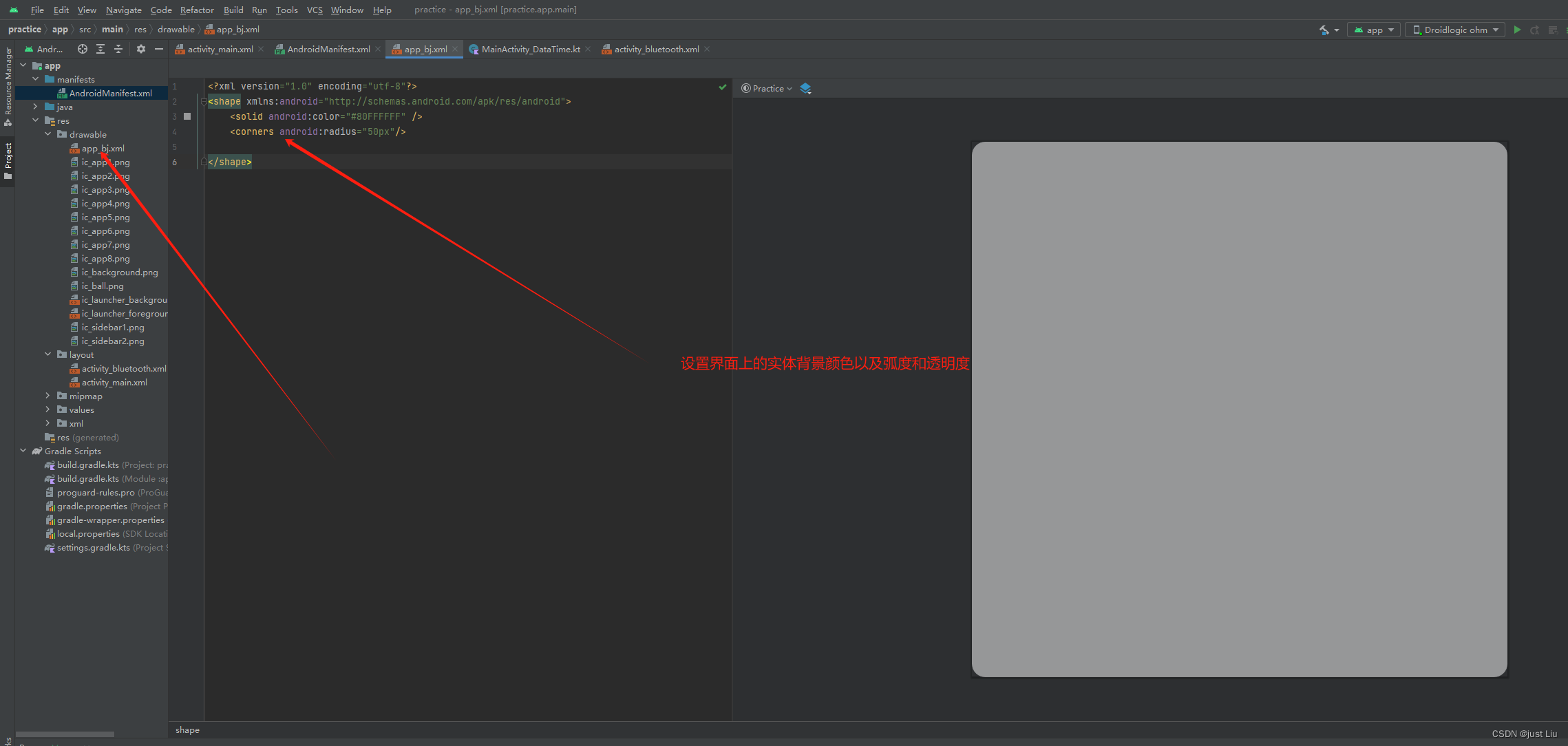
Android studio矩形背景颜色以及弧度的设置
在这里插入图片描述 Android的shape中主要设置的属性 corners:用于设置形状的圆角,可以设置圆角的半径、颜色等属性。 stroke:用于设置形状的边框,可以设置边框的宽度、颜色等属性。 padding:用于设置形状的内边距&…...
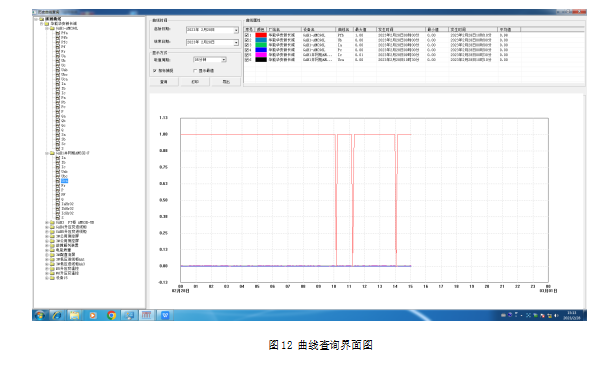
Acrel-1000DP分布式光伏系统在某重工企业18MW分布式光伏中应用——安科瑞 顾烊宇
摘 要:分布式光伏发电特指在用户场地附近建设,运行方式以用户侧自发自用、余电上网,且在配电系统平衡调节为特征的光伏发电设施,是一种新型的、具有广阔发展前景的发电和能源综合利用方式,它倡导就近发电,就…...
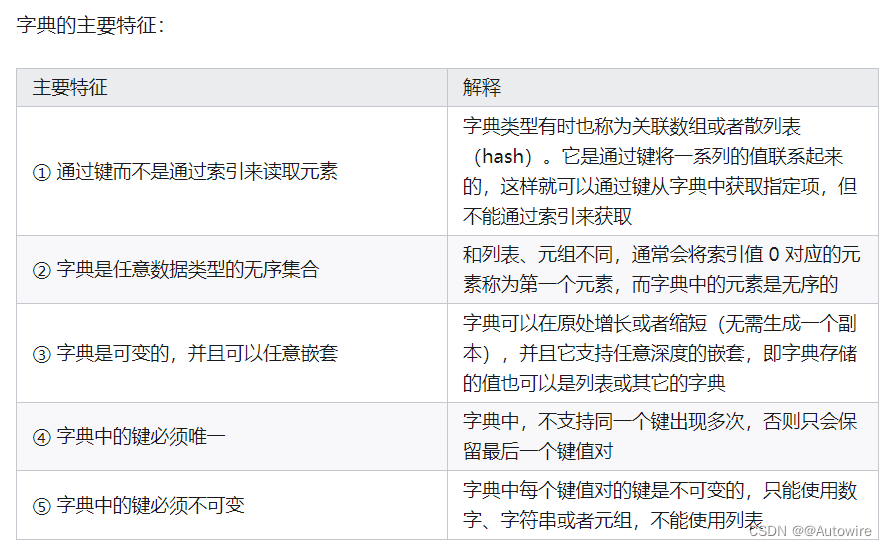
3 python基本语法 - Dict 字典
Python 中字典(dict)是一种无序的、可变的序列,它的元素以“键值对(key-value)”的形式存储。相对地,列表(list)和元组(tuple)都是有序的序列,它们…...
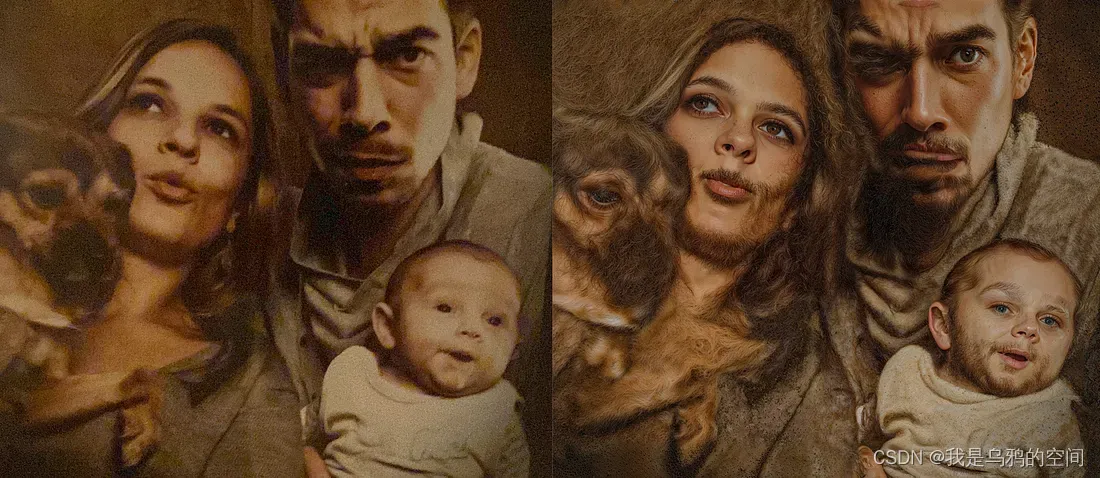
Magnific AI:彻底改变 AI 生成图像的升级
在我最近与 Magnific AI 的讨论中,我不仅感到惊讶,而且对该工具提供的质量和可能性着迷。我发现 Magnific AI 能够转换人工智能生成的图像(这些图像通常只能以低分辨率提供),尤其令人印象深刻,不仅在可打印…...
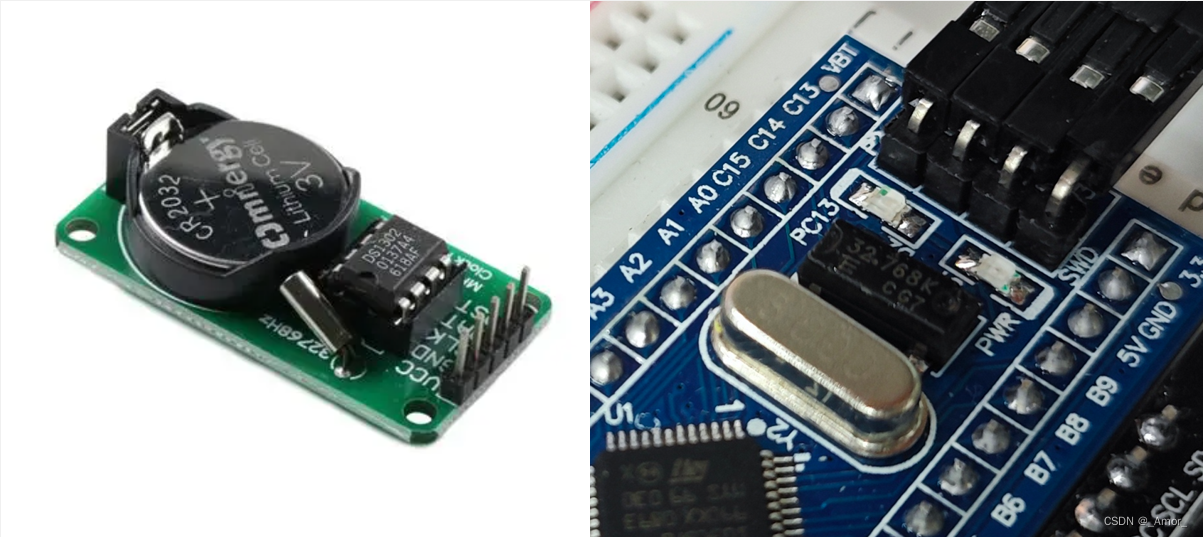
BKP 备份寄存器 RTC 实时时钟-stm32入门
这一章节我们要讲的主要内容是 RTC 实时时钟,对应手册,是第 16 章的位置。 实时时钟这个东西,本质上是一个定时器,但是这个定时器,是专门用来产生年月日时分秒,这种日期和时间信息的。所以学会了 STM32 的…...