67、yolov8目标检测和旋转目标检测算法部署Atlas 200I DK A2开发板上
基本思想:需求部署yolov8目标检测和旋转目标检测算法部署atlas 200dk 开发板上
一、转换模型 链接: https://pan.baidu.com/s/1hJPX2QvybI4AGgeJKO6QgQ?pwd=q2s5 提取码: q2s5
from ultralytics import YOLO# Load a model
model = YOLO("yolov8s.yaml") # build a new model from scratch
model = YOLO("yolov8s.pt") # load a pretrained model (recommended for training)# Use the modelresults = model(r"F:\zhy\Git\McQuic\bus.jpg") # predict on an image
path = model.export(format="onnx") # export the model to ONNX format
精简模型
(base) root@davinci-mini:~/sxj731533730# python3 -m onnxsim yolov8s.onnx yolov8s_sim.onnx
Simplifying...
Finish! Here is the difference:
┏━━━━━━━━━━━━┳━━━━━━━━━━━━━━━━┳━━━━━━━━━━━━━━━━━━┓
┃ ┃ Original Model ┃ Simplified Model ┃
┡━━━━━━━━━━━━╇━━━━━━━━━━━━━━━━╇━━━━━━━━━━━━━━━━━━┩
│ Add │ 9 │ 8 │
│ Concat │ 19 │ 19 │
│ Constant │ 22 │ 0 │
│ Conv │ 64 │ 64 │
│ Div │ 2 │ 1 │
│ Gather │ 1 │ 0 │
│ MaxPool │ 3 │ 3 │
│ Mul │ 60 │ 58 │
│ Reshape │ 5 │ 5 │
│ Resize │ 2 │ 2 │
│ Shape │ 1 │ 0 │
│ Sigmoid │ 58 │ 58 │
│ Slice │ 2 │ 2 │
│ Softmax │ 1 │ 1 │
│ Split │ 9 │ 9 │
│ Sub │ 2 │ 2 │
│ Transpose │ 1 │ 1 │
│ Model Size │ 42.8MiB │ 42.7MiB │
└────────────┴────────────────┴──────────────────┘
使用huawei板子进行转换模型
(base) root@davinci-mini:~/sxj731533730# atc --model=yolov8s.onnx --framework=5 --output=yolov8s --input_format=NCHW --i
nput_shape="images:1,3,640,640" --log=error --soc_version=Ascend310B1
配置pycharm professional
pycharm professional 设置
/usr/local/miniconda3/bin/python3
LD_LIBRARY_PATH=/usr/local/Ascend/ascend-toolkit/latest/lib64:/usr/local/Ascend/ascend-toolkit/latest/lib64/plugin/opskernel:/usr/local/Ascend/ascend-toolkit/latest/lib64/plugin/nnengine:$LD_LIBRARY_PATH;PYTHONPATH=/usr/local/Ascend/ascend-toolkit/latest/python/site-packages:/usr/local/Ascend/ascend-toolkit/latest/opp/op_impl/built-in/ai_core/tbe:$PYTHONPATH;PATH=/usr/local/Ascend/ascend-toolkit/latest/bin:/usr/local/Ascend/ascend-toolkit/latest/compiler/ccec_compiler/bin:$PATH;ASCEND_AICPU_PATH=/usr/local/Ascend/ascend-toolkit/latest;ASCEND_OPP_PATH=/usr/local/Ascend/ascend-toolkit/latest/opp;TOOLCHAIN_HOME=/usr/local/Ascend/ascend-toolkit/latest/toolkit;ASCEND_HOME_PATH=/usr/local/Ascend/ascend-toolkit/latest/usr/local/miniconda3/bin/python3.9 /home/sxj731533730/sgagtyeray.py
测试代码pyhon
import ctypes
import os
import shutil
import random
import sys
import threading
import time
import cv2
import numpy as np
import numpy as np
import cv2
from ais_bench.infer.interface import InferSession
class_names = ['person', 'bicycle', 'car', 'motorcycle', 'airplane', 'bus', 'train', 'truck', 'boat', 'traffic light','fire hydrant', 'stop sign', 'parking meter', 'bench', 'bird', 'cat', 'dog', 'horse', 'sheep', 'cow','elephant', 'bear', 'zebra', 'giraffe', 'backpack', 'umbrella', 'handbag', 'tie', 'suitcase', 'frisbee','skis', 'snowboard', 'sports ball', 'kite', 'baseball bat', 'baseball glove', 'skateboard', 'surfboard','tennis racket', 'bottle', 'wine glass', 'cup', 'fork', 'knife', 'spoon', 'bowl', 'banana', 'apple','sandwich', 'orange', 'broccoli', 'carrot', 'hot dog', 'pizza', 'donut', 'cake', 'chair', 'couch','potted plant', 'bed', 'dining table', 'toilet', 'tv', 'laptop', 'mouse', 'remote', 'keyboard','cell phone', 'microwave', 'oven', 'toaster', 'sink', 'refrigerator', 'book', 'clock', 'vase','scissors', 'teddy bear', 'hair drier', 'toothbrush']# Create a list of colors for each class where each color is a tuple of 3 integer values
rng = np.random.default_rng(3)
colors = rng.uniform(0, 255, size=(len(class_names), 3))model_path = 'yolov8s.om'
IMG_PATH = 'bus.jpg'conf_threshold = 0.5
iou_threshold = 0.4
input_w=640
input_h=640def get_img_path_batches(batch_size, img_dir):ret = []batch = []for root, dirs, files in os.walk(img_dir):for name in files:if len(batch) == batch_size:ret.append(batch)batch = []batch.append(os.path.join(root, name))if len(batch) > 0:ret.append(batch)return retdef plot_one_box(x, img, color=None, label=None, line_thickness=None):"""description: Plots one bounding box on image img,this function comes from YoLov8 project.param:x: a box likes [x1,y1,x2,y2]img: a opencv image objectcolor: color to draw rectangle, such as (0,255,0)label: strline_thickness: intreturn:no return"""tl = (line_thickness or round(0.002 * (img.shape[0] + img.shape[1]) / 2) + 1) # line/font thicknesscolor = color or [random.randint(0, 255) for _ in range(3)]c1, c2 = (int(x[0]), int(x[1])), (int(x[2]), int(x[3]))cv2.rectangle(img, c1, c2, color, thickness=tl, lineType=cv2.LINE_AA)if label:tf = max(tl - 1, 1) # font thicknesst_size = cv2.getTextSize(label, 0, fontScale=tl / 3, thickness=tf)[0]c2 = c1[0] + t_size[0], c1[1] - t_size[1] - 3cv2.rectangle(img, c1, c2, color, -1, cv2.LINE_AA) # filledcv2.putText(img,label,(c1[0], c1[1] - 2),0,tl / 3,[225, 255, 255],thickness=tf,lineType=cv2.LINE_AA,)def preprocess_image( raw_bgr_image):"""description: Convert BGR image to RGB,resize and pad it to target size, normalize to [0,1],transform to NCHW format.param:input_image_path: str, image pathreturn:image: the processed imageimage_raw: the original imageh: original heightw: original width"""image_raw = raw_bgr_imageh, w, c = image_raw.shapeimage = cv2.cvtColor(image_raw, cv2.COLOR_BGR2RGB)# Calculate widht and height and paddingsr_w = input_w / wr_h = input_h / hif r_h > r_w:tw = input_wth = int(r_w * h)tx1 = tx2 = 0ty1 = int(( input_h - th) / 2)ty2 = input_h - th - ty1else:tw = int(r_h * w)th = input_htx1 = int(( input_w - tw) / 2)tx2 = input_w - tw - tx1ty1 = ty2 = 0# Resize the image with long side while maintaining ratioimage = cv2.resize(image, (tw, th))# Pad the short side with (128,128,128)image = cv2.copyMakeBorder(image, ty1, ty2, tx1, tx2, cv2.BORDER_CONSTANT, None, (128, 128, 128))image = image.astype(np.float32)# Normalize to [0,1]image /= 255.0# HWC to CHW format:image = np.transpose(image, [2, 0, 1])# CHW to NCHW formatimage = np.expand_dims(image, axis=0)# Convert the image to row-major order, also known as "C order":image = np.ascontiguousarray(image)return image, image_raw, h, w,r_h,r_wdef xywh2xyxy( origin_h, origin_w, x):"""description: Convert nx4 boxes from [x, y, w, h] to [x1, y1, x2, y2] where xy1=top-left, xy2=bottom-rightparam:origin_h: height of original imageorigin_w: width of original imagex: A boxes numpy, each row is a box [center_x, center_y, w, h]return:y: A boxes numpy, each row is a box [x1, y1, x2, y2]"""y = np.zeros_like(x)r_w = input_w / origin_wr_h = input_h / origin_hif r_h > r_w:y[:, 0] = x[:, 0]y[:, 2] = x[:, 2]y[:, 1] = x[:, 1] - ( input_h - r_w * origin_h) / 2y[:, 3] = x[:, 3] - ( input_h - r_w * origin_h) / 2y /= r_welse:y[:, 0] = x[:, 0] - ( input_w - r_h * origin_w) / 2y[:, 2] = x[:, 2] - ( input_w - r_h * origin_w) / 2y[:, 1] = x[:, 1]y[:, 3] = x[:, 3]y /= r_hreturn y
def rescale_boxes( boxes,img_width, img_height, input_width, input_height):# Rescale boxes to original image dimensionsinput_shape = np.array([input_width, input_height,input_width, input_height])boxes = np.divide(boxes, input_shape, dtype=np.float32)boxes *= np.array([img_width, img_height, img_width, img_height])return boxes
def xywh2xyxy(x):# Convert bounding box (x, y, w, h) to bounding box (x1, y1, x2, y2)y = np.copy(x)y[..., 0] = x[..., 0] - x[..., 2] / 2y[..., 1] = x[..., 1] - x[..., 3] / 2y[..., 2] = x[..., 0] + x[..., 2] / 2y[..., 3] = x[..., 1] + x[..., 3] / 2return y
def compute_iou(box, boxes):# Compute xmin, ymin, xmax, ymax for both boxesxmin = np.maximum(box[0], boxes[:, 0])ymin = np.maximum(box[1], boxes[:, 1])xmax = np.minimum(box[2], boxes[:, 2])ymax = np.minimum(box[3], boxes[:, 3])# Compute intersection areaintersection_area = np.maximum(0, xmax - xmin) * np.maximum(0, ymax - ymin)# Compute union areabox_area = (box[2] - box[0]) * (box[3] - box[1])boxes_area = (boxes[:, 2] - boxes[:, 0]) * (boxes[:, 3] - boxes[:, 1])union_area = box_area + boxes_area - intersection_area# Compute IoUiou = intersection_area / union_areareturn iou
def nms(boxes, scores, iou_threshold):# Sort by scoresorted_indices = np.argsort(scores)[::-1]keep_boxes = []while sorted_indices.size > 0:# Pick the last boxbox_id = sorted_indices[0]keep_boxes.append(box_id)# Compute IoU of the picked box with the restious = compute_iou(boxes[box_id, :], boxes[sorted_indices[1:], :])# Remove boxes with IoU over the thresholdkeep_indices = np.where(ious < iou_threshold)[0]# print(keep_indices.shape, sorted_indices.shape)sorted_indices = sorted_indices[keep_indices + 1]return keep_boxesdef multiclass_nms(boxes, scores, class_ids, iou_threshold):unique_class_ids = np.unique(class_ids)keep_boxes = []for class_id in unique_class_ids:class_indices = np.where(class_ids == class_id)[0]class_boxes = boxes[class_indices,:]class_scores = scores[class_indices]class_keep_boxes = nms(class_boxes, class_scores, iou_threshold)keep_boxes.extend(class_indices[class_keep_boxes])return keep_boxes
def extract_boxes(predictions,img_width, img_height, input_width, input_height):# Extract boxes from predictionsboxes = predictions[:, :4]# Scale boxes to original image dimensionsboxes = rescale_boxes(boxes,img_width, img_height, input_width, input_height)# Convert boxes to xyxy formatboxes = xywh2xyxy(boxes)return boxes
def process_output(output,img_width, img_height, input_width, input_height):predictions = np.squeeze(output[0]).T# Filter out object confidence scores below thresholdscores = np.max(predictions[:, 4:], axis=1)predictions = predictions[scores > conf_threshold, :]scores = scores[scores > conf_threshold]if len(scores) == 0:return [], [], []# Get the class with the highest confidenceclass_ids = np.argmax(predictions[:, 4:], axis=1)# Get bounding boxes for each objectboxes = extract_boxes(predictions,img_width, img_height, input_width, input_height)# Apply non-maxima suppression to suppress weak, overlapping bounding boxes# indices = nms(boxes, scores, self.iou_threshold)indices = multiclass_nms(boxes, scores, class_ids, iou_threshold)return boxes[indices], scores[indices], class_ids[indices]def bbox_iou( box1, box2, x1y1x2y2=True):"""description: compute the IoU of two bounding boxesparam:box1: A box coordinate (can be (x1, y1, x2, y2) or (x, y, w, h))box2: A box coordinate (can be (x1, y1, x2, y2) or (x, y, w, h))x1y1x2y2: select the coordinate formatreturn:iou: computed iou"""if not x1y1x2y2:# Transform from center and width to exact coordinatesb1_x1, b1_x2 = box1[:, 0] - box1[:, 2] / 2, box1[:, 0] + box1[:, 2] / 2b1_y1, b1_y2 = box1[:, 1] - box1[:, 3] / 2, box1[:, 1] + box1[:, 3] / 2b2_x1, b2_x2 = box2[:, 0] - box2[:, 2] / 2, box2[:, 0] + box2[:, 2] / 2b2_y1, b2_y2 = box2[:, 1] - box2[:, 3] / 2, box2[:, 1] + box2[:, 3] / 2else:# Get the coordinates of bounding boxesb1_x1, b1_y1, b1_x2, b1_y2 = box1[:, 0], box1[:, 1], box1[:, 2], box1[:, 3]b2_x1, b2_y1, b2_x2, b2_y2 = box2[:, 0], box2[:, 1], box2[:, 2], box2[:, 3]# Get the coordinates of the intersection rectangleinter_rect_x1 = np.maximum(b1_x1, b2_x1)inter_rect_y1 = np.maximum(b1_y1, b2_y1)inter_rect_x2 = np.minimum(b1_x2, b2_x2)inter_rect_y2 = np.minimum(b1_y2, b2_y2)# Intersection areainter_area = np.clip(inter_rect_x2 - inter_rect_x1 + 1, 0, None) * \np.clip(inter_rect_y2 - inter_rect_y1 + 1, 0, None)# Union Areab1_area = (b1_x2 - b1_x1 + 1) * (b1_y2 - b1_y1 + 1)b2_area = (b2_x2 - b2_x1 + 1) * (b2_y2 - b2_y1 + 1)iou = inter_area / (b1_area + b2_area - inter_area + 1e-16)return ioudef non_max_suppression( prediction, origin_h, origin_w, conf_thres=0.5, nms_thres=0.4):"""description: Removes detections with lower object confidence score than 'conf_thres' and performsNon-Maximum Suppression to further filter detections.param:prediction: detections, (x1, y1, x2, y2, conf, cls_id)origin_h: original image heightorigin_w: original image widthconf_thres: a confidence threshold to filter detectionsnms_thres: a iou threshold to filter detectionsreturn:boxes: output after nms with the shape (x1, y1, x2, y2, conf, cls_id)"""# Get the boxes that score > CONF_THRESHboxes = prediction[prediction[:, 4] >= conf_thres]# Trandform bbox from [center_x, center_y, w, h] to [x1, y1, x2, y2]boxes[:, :4] = xywh2xyxy(origin_h, origin_w, boxes[:, :4])# clip the coordinatesboxes[:, 0] = np.clip(boxes[:, 0], 0, origin_w - 1)boxes[:, 2] = np.clip(boxes[:, 2], 0, origin_w - 1)boxes[:, 1] = np.clip(boxes[:, 1], 0, origin_h - 1)boxes[:, 3] = np.clip(boxes[:, 3], 0, origin_h - 1)# Object confidenceconfs = boxes[:, 4]# Sort by the confsboxes = boxes[np.argsort(-confs)]# Perform non-maximum suppressionkeep_boxes = []while boxes.shape[0]:large_overlap = bbox_iou(np.expand_dims(boxes[0, :4], 0), boxes[:, :4]) > nms_threslabel_match = boxes[0, -1] == boxes[:, -1]# Indices of boxes with lower confidence scores, large IOUs and matching labelsinvalid = large_overlap & label_matchkeep_boxes += [boxes[0]]boxes = boxes[~invalid]boxes = np.stack(keep_boxes, 0) if len(keep_boxes) else np.array([])return boxesdef draw_box( image: np.ndarray, box: np.ndarray, color: tuple[int, int, int] = (0, 0, 255),thickness: int = 2) -> np.ndarray:x1, y1, x2, y2 = box.astype(int)return cv2.rectangle(image, (x1, y1), (x2, y2), color, thickness)def draw_text(image: np.ndarray, text: str, box: np.ndarray, color: tuple[int, int, int] = (0, 0, 255),font_size: float = 0.001, text_thickness: int = 2) -> np.ndarray:x1, y1, x2, y2 = box.astype(int)(tw, th), _ = cv2.getTextSize(text=text, fontFace=cv2.FONT_HERSHEY_SIMPLEX,fontScale=font_size, thickness=text_thickness)th = int(th * 1.2)cv2.rectangle(image, (x1, y1),(x1 + tw, y1 - th), color, -1)return cv2.putText(image, text, (x1, y1), cv2.FONT_HERSHEY_SIMPLEX, font_size, (255, 255, 255), text_thickness, cv2.LINE_AA)def draw_detections(image, boxes, scores, class_ids, mask_alpha=0.3):det_img = image.copy()img_height, img_width = image.shape[:2]font_size = min([img_height, img_width]) * 0.0006text_thickness = int(min([img_height, img_width]) * 0.001)# Draw bounding boxes and labels of detectionsfor class_id, box, score in zip(class_ids, boxes, scores):color = colors[class_id]draw_box(det_img, box, color)label = class_names[class_id]caption = f'{label} {int(score * 100)}%'draw_text(det_img, caption, box, color, font_size, text_thickness)return det_imgif __name__ == "__main__":# load custom plugin and engine# loa# 初始化推理模型model = InferSession(0, model_path)image = cv2.imread(IMG_PATH)h,w,_=image.shape# img, ratio, (dw, dh) = letterbox(img, new_shape=(IMG_SIZE, IMG_SIZE))img = cv2.cvtColor(image, cv2.COLOR_BGR2RGB)img = cv2.resize(img, (input_w, input_h))img_ = img / 255.0 # 归一化到0~1img_ = img_.transpose(2, 0, 1)img_ = np.ascontiguousarray(img_, dtype=np.float32)outputs = model.infer([img_])import picklewith open('yolov8.pkl', 'wb') as f:pickle.dump(outputs, f)with open('yolov8.pkl', 'rb') as f:outputs = pickle.load(f)#print(outputs)boxes, scores, class_ids = process_output(outputs,w,h,input_w,input_h)print(boxes, scores, class_ids)original_image=draw_detections(image, boxes, scores, class_ids, mask_alpha=0.3)#print(boxes, scores, class_ids)cv2.imwrite("result.jpg",original_image)print("------------------")
测试结果
[INFO] acl init success
[INFO] open device 0 success
[INFO] load model yolov8s.om success
[INFO] create model description success
[[5.0941406e+01 4.0057031e+02 2.4648047e+02 9.0386719e+02][6.6951562e+02 3.9065625e+02 8.1000000e+02 8.8087500e+02][2.2377832e+02 4.0732031e+02 3.4512012e+02 8.5999219e+02][3.1640625e-01 5.5096875e+02 7.3762207e+01 8.7075000e+02][3.1640625e+00 2.2865625e+02 8.0873438e+02 7.4503125e+02]] [0.9140625 0.9121094 0.87597656 0.55566406 0.8984375 ] [0 0 0 0 5]
------------------
[INFO] unload model success, model Id is 1
[INFO] end to destroy context
[INFO] end to reset device is 0
[INFO] end to finalize aclProcess finished with exit code 0
二、c++版本
cmakelists.txt
cmake_minimum_required(VERSION 3.16)
project(untitled10)
set(CMAKE_CXX_FLAGS "-std=c++11")
set(CMAKE_CXX_STANDARD 11)
add_definitions(-DENABLE_DVPP_INTERFACE)include_directories(/usr/local/samples/cplusplus/common/acllite/include)
include_directories(/usr/local/Ascend/ascend-toolkit/latest/aarch64-linux/include)
find_package(OpenCV REQUIRED)
#message(STATUS ${OpenCV_INCLUDE_DIRS})
#添加头文件
include_directories(${OpenCV_INCLUDE_DIRS})
#链接Opencv库
add_library(libascendcl SHARED IMPORTED)
set_target_properties(libascendcl PROPERTIES IMPORTED_LOCATION /usr/local/Ascend/ascend-toolkit/latest/aarch64-linux/lib64/libascendcl.so)
add_library(libacllite SHARED IMPORTED)
set_target_properties(libacllite PROPERTIES IMPORTED_LOCATION /usr/local/samples/cplusplus/common/acllite/out/aarch64/libacllite.so)add_executable(untitled10 main.cpp)
target_link_libraries(untitled10 ${OpenCV_LIBS} libascendcl libacllite)
main.cpp
#include <opencv2/opencv.hpp>
#include "AclLiteUtils.h"
#include "AclLiteImageProc.h"
#include "AclLiteResource.h"
#include "AclLiteError.h"
#include "AclLiteModel.h"
#include <opencv2/dnn.hpp>
#include <opencv2/imgproc.hpp>
#include <opencv2/highgui.hpp>
#include <algorithm>using namespace std;
using namespace cv;typedef enum Result {SUCCESS = 0,FAILED = 1
} Result;
std::vector<std::string> CLASS_NAMES = {"person", "bicycle", "car", "motorcycle", "airplane", "bus", "train", "truck", "boat","traffic light","fire hydrant", "stop sign", "parking meter", "bench", "bird", "cat", "dog", "horse","sheep", "cow","elephant", "bear", "zebra", "giraffe", "backpack", "umbrella", "handbag", "tie","suitcase", "frisbee","skis", "snowboard", "sports ball", "kite", "baseball bat", "baseball glove","skateboard", "surfboard","tennis racket", "bottle", "wine glass", "cup", "fork", "knife", "spoon", "bowl","banana", "apple","sandwich", "orange", "broccoli", "carrot", "hot dog", "pizza", "donut", "cake","chair", "couch","potted plant", "bed", "dining table", "toilet", "tv", "laptop", "mouse", "remote","keyboard", "cell phone","microwave", "oven", "toaster", "sink", "refrigerator", "book", "clock", "vase","scissors", "teddy bear","hair drier", "toothbrush"};const std::vector<std::vector<float>> COLOR_LIST = {{1, 1, 1},{0.098, 0.325, 0.850},{0.125, 0.694, 0.929},{0.556, 0.184, 0.494},{0.188, 0.674, 0.466},{0.933, 0.745, 0.301},{0.184, 0.078, 0.635},{0.300, 0.300, 0.300},{0.600, 0.600, 0.600},{0.000, 0.000, 1.000},{0.000, 0.500, 1.000},{0.000, 0.749, 0.749},{0.000, 1.000, 0.000},{1.000, 0.000, 0.000},{1.000, 0.000, 0.667},{0.000, 0.333, 0.333},{0.000, 0.667, 0.333},{0.000, 1.000, 0.333},{0.000, 0.333, 0.667},{0.000, 0.667, 0.667},{0.000, 1.000, 0.667},{0.000, 0.333, 1.000},{0.000, 0.667, 1.000},{0.000, 1.000, 1.000},{0.500, 0.333, 0.000},{0.500, 0.667, 0.000},{0.500, 1.000, 0.000},{0.500, 0.000, 0.333},{0.500, 0.333, 0.333},{0.500, 0.667, 0.333},{0.500, 1.000, 0.333},{0.500, 0.000, 0.667},{0.500, 0.333, 0.667},{0.500, 0.667, 0.667},{0.500, 1.000, 0.667},{0.500, 0.000, 1.000},{0.500, 0.333, 1.000},{0.500, 0.667, 1.000},{0.500, 1.000, 1.000},{1.000, 0.333, 0.000},{1.000, 0.667, 0.000},{1.000, 1.000, 0.000},{1.000, 0.000, 0.333},{1.000, 0.333, 0.333},{1.000, 0.667, 0.333},{1.000, 1.000, 0.333},{1.000, 0.000, 0.667},{1.000, 0.333, 0.667},{1.000, 0.667, 0.667},{1.000, 1.000, 0.667},{1.000, 0.000, 1.000},{1.000, 0.333, 1.000},{1.000, 0.667, 1.000},{0.000, 0.000, 0.333},{0.000, 0.000, 0.500},{0.000, 0.000, 0.667},{0.000, 0.000, 0.833},{0.000, 0.000, 1.000},{0.000, 0.167, 0.000},{0.000, 0.333, 0.000},{0.000, 0.500, 0.000},{0.000, 0.667, 0.000},{0.000, 0.833, 0.000},{0.000, 1.000, 0.000},{0.167, 0.000, 0.000},{0.333, 0.000, 0.000},{0.500, 0.000, 0.000},{0.667, 0.000, 0.000},{0.833, 0.000, 0.000},{1.000, 0.000, 0.000},{0.000, 0.000, 0.000},{0.143, 0.143, 0.143},{0.286, 0.286, 0.286},{0.429, 0.429, 0.429},{0.571, 0.571, 0.571},{0.714, 0.714, 0.714},{0.857, 0.857, 0.857},{0.741, 0.447, 0.000},{0.741, 0.717, 0.314},{0.000, 0.500, 0.500}
};struct Object {// The object class.int label{};// The detection's confidence probability.float probability{};// The object bounding box rectangle.cv::Rect_<float> rect;};float clamp_T(float value, float low, float high) {if (value < low) return low;if (high < value) return high;return value;
}
void drawObjectLabels(cv::Mat& image, const std::vector<Object> &objects, unsigned int scale=1) {// If segmentation information is present, start with that// Bounding boxes and annotationsfor (auto & object : objects) {// Choose the colorint colorIndex = object.label % COLOR_LIST.size(); // We have only defined 80 unique colorscv::Scalar color = cv::Scalar(COLOR_LIST[colorIndex][0],COLOR_LIST[colorIndex][1],COLOR_LIST[colorIndex][2]);float meanColor = cv::mean(color)[0];cv::Scalar txtColor;if (meanColor > 0.5){txtColor = cv::Scalar(0, 0, 0);}else{txtColor = cv::Scalar(255, 255, 255);}const auto& rect = object.rect;// Draw rectangles and textchar text[256];sprintf(text, "%s %.1f%%", CLASS_NAMES[object.label].c_str(), object.probability * 100);int baseLine = 0;cv::Size labelSize = cv::getTextSize(text, cv::FONT_HERSHEY_SIMPLEX, 0.35 * scale, scale, &baseLine);cv::Scalar txt_bk_color = color * 0.7 * 255;int x = object.rect.x;int y = object.rect.y + 1;cv::rectangle(image, rect, color * 255, scale + 1);cv::rectangle(image, cv::Rect(cv::Point(x, y), cv::Size(labelSize.width, labelSize.height + baseLine)),txt_bk_color, -1);cv::putText(image, text, cv::Point(x, y + labelSize.height), cv::FONT_HERSHEY_SIMPLEX, 0.35 * scale, txtColor, scale);}
}
int main() {const char *modelPath = "../model/yolov8s.om";const char *fileName="../bus.jpg";auto image_process_mode="letter_box";const int label_num=80;const float nms_threshold = 0.6;const float conf_threshold = 0.25;int TOP_K=100;std::vector<int> iImgSize={640,640};float resizeScales=1;cv::Mat iImg = cv::imread(fileName);int m_imgWidth=iImg.cols;int m_imgHeight=iImg.rows;cv::Mat oImg = iImg.clone();cv::cvtColor(oImg, oImg, cv::COLOR_BGR2RGB);cv::Mat detect_image;cv::resize(oImg, detect_image, cv::Size(iImgSize.at(0), iImgSize.at(1)));float m_ratio_w = 1.f / (iImgSize.at(0) / static_cast<float>(iImg.cols));float m_ratio_h = 1.f / (iImgSize.at(1) / static_cast<float>(iImg.rows));float* imageBytes;AclLiteResource aclResource_;AclLiteImageProc imageProcess_;AclLiteModel model_;aclrtRunMode runMode_;ImageData resizedImage_;const char *modelPath_;int32_t modelWidth_;int32_t modelHeight_;AclLiteError ret = aclResource_.Init();if (ret == FAILED) {ACLLITE_LOG_ERROR("resource init failed, errorCode is %d", ret);return FAILED;}ret = aclrtGetRunMode(&runMode_);if (ret == FAILED) {ACLLITE_LOG_ERROR("get runMode failed, errorCode is %d", ret);return FAILED;}// init dvpp resourceret = imageProcess_.Init();if (ret == FAILED) {ACLLITE_LOG_ERROR("imageProcess init failed, errorCode is %d", ret);return FAILED;}// load model from fileret = model_.Init(modelPath);if (ret == FAILED) {ACLLITE_LOG_ERROR("model init failed, errorCode is %d", ret);return FAILED;}// data standardizationfloat meanRgb[3] = {0, 0, 0};float stdRgb[3] = {1/255.0f, 1/255.0f, 1/255.0f};int32_t channel = detect_image.channels();int32_t resizeHeight = detect_image.rows;int32_t resizeWeight = detect_image.cols;imageBytes = (float *) malloc(channel * (iImgSize.at(0)) * (iImgSize.at(1)) * sizeof(float));memset(imageBytes, 0, channel * (iImgSize.at(0)) * (iImgSize.at(1)) * sizeof(float));// image to bytes with shape HWC to CHW, and switch channel BGR to RGBfor (int c = 0; c < channel; ++c) {for (int h = 0; h < resizeHeight; ++h) {for (int w = 0; w < resizeWeight; ++w) {int dstIdx = c * resizeHeight * resizeWeight + h * resizeWeight + w;imageBytes[dstIdx] = static_cast<float>((detect_image.at<cv::Vec3b>(h, w)[c] -1.0f * meanRgb[c]) * 1.0f * stdRgb[c] );}}}std::vector <InferenceOutput> inferOutputs;ret = model_.CreateInput(static_cast<void *>(imageBytes),channel * iImgSize.at(0) * iImgSize.at(1) * sizeof(float));if (ret == FAILED) {ACLLITE_LOG_ERROR("CreateInput failed, errorCode is %d", ret);return FAILED;}// inferenceret = model_.Execute(inferOutputs);if (ret != ACL_SUCCESS) {ACLLITE_LOG_ERROR("execute model failed, errorCode is %d", ret);return FAILED;}float *rawData = static_cast<float *>(inferOutputs[0].data.get());std::vector<cv::Rect> bboxes;std::vector<float> scores;std::vector<int> labels;std::vector<int> indices;auto numChannels =84;auto numAnchors =8400;auto numClasses = CLASS_NAMES.size();cv::Mat output = cv::Mat(numChannels, numAnchors, CV_32F,rawData);output = output.t();// Get all the YOLO proposalsfor (int i = 0; i < numAnchors; i++) {auto rowPtr = output.row(i).ptr<float>();auto bboxesPtr = rowPtr;auto scoresPtr = rowPtr + 4;auto maxSPtr = std::max_element(scoresPtr, scoresPtr + numClasses);float score = *maxSPtr;if (score > conf_threshold) {float x = *bboxesPtr++;float y = *bboxesPtr++;float w = *bboxesPtr++;float h = *bboxesPtr;float x0 = clamp_T((x - 0.5f * w) * m_ratio_w, 0.f, m_imgWidth);float y0 =clamp_T((y - 0.5f * h) * m_ratio_h, 0.f, m_imgHeight);float x1 = clamp_T((x + 0.5f * w) * m_ratio_w, 0.f, m_imgWidth);float y1 = clamp_T((y + 0.5f * h) * m_ratio_h, 0.f, m_imgHeight);int label = maxSPtr - scoresPtr;cv::Rect_<float> bbox;bbox.x = x0;bbox.y = y0;bbox.width = x1 - x0;bbox.height = y1 - y0;bboxes.push_back(bbox);labels.push_back(label);scores.push_back(score);}}// Run NMScv::dnn::NMSBoxes(bboxes, scores, conf_threshold, nms_threshold, indices);std::vector<Object> objects;// Choose the top k detectionsint cnt = 0;for (auto& chosenIdx : indices) {if (cnt >= TOP_K) {break;}Object obj{};obj.probability = scores[chosenIdx];obj.label = labels[chosenIdx];obj.rect = bboxes[chosenIdx];objects.push_back(obj);cnt += 1;}drawObjectLabels( iImg, objects);cv::imwrite("../result_c++.jpg",iImg);model_.DestroyResource();imageProcess_.DestroyResource();aclResource_.Release();return SUCCESS;
}
测试结果
三、旋转目标
待需
相关文章:
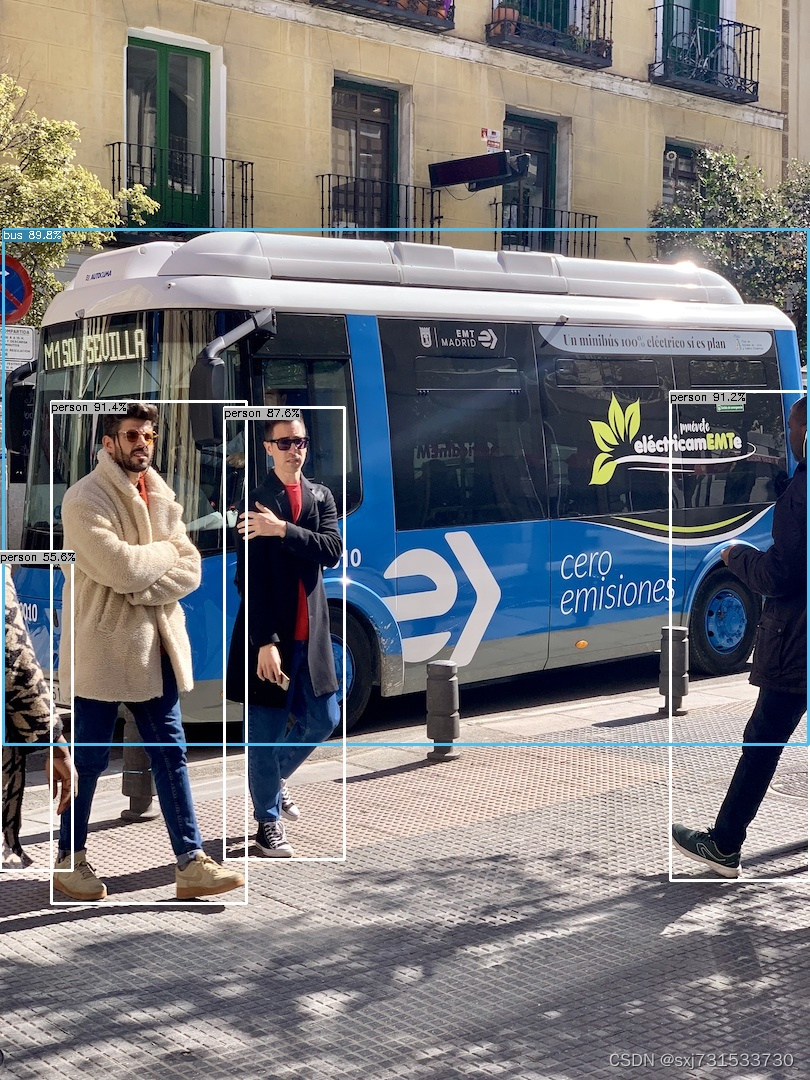
67、yolov8目标检测和旋转目标检测算法部署Atlas 200I DK A2开发板上
基本思想:需求部署yolov8目标检测和旋转目标检测算法部署atlas 200dk 开发板上 一、转换模型 链接: https://pan.baidu.com/s/1hJPX2QvybI4AGgeJKO6QgQ?pwdq2s5 提取码: q2s5 from ultralytics import YOLO# Load a model model YOLO("yolov8s.yaml")…...

A Little Is Enough: Circumventing Defenses For Distributed Learning
联邦学习的攻击方法:LIE 简单的总结,只是为了能快速想起来这个方法。 无目标攻击 例如总共50个客户端,有24个恶意客户端,那么这个时候,他需要拉拢2个良性客户端 计算 50 − 24 − 2 50 − 24 0.923 \frac{50-24-2}{…...
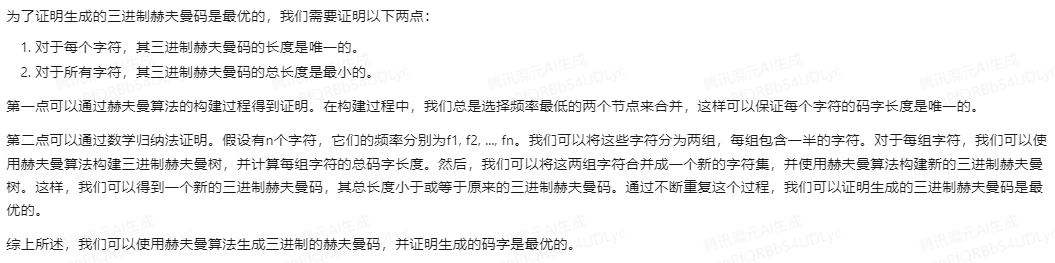
文心一言 VS 讯飞星火 VS chatgpt (225)-- 算法导论16.3 7题
七、推广赫夫曼算法,使之能生成三进制的码字(即码字由符号0、1、2组成),并证明你的算法能生成最优三进制码。如果要写代码,请用go语言。 文心一言: 推广赫夫曼算法以生成三进制码字需要对算法进行一定的修改,确保在每…...
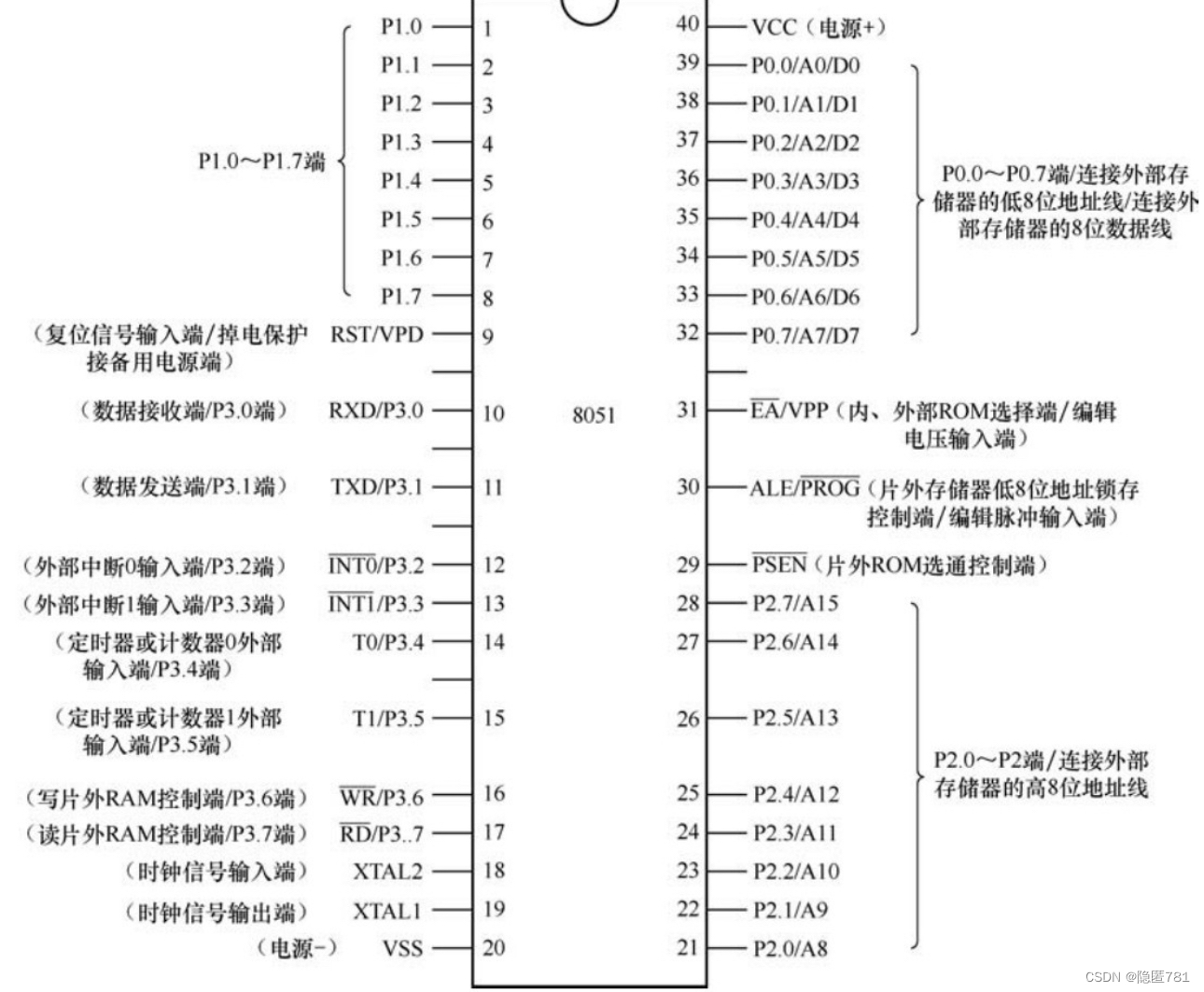
【计算机】——51单片机——持续更新
单片机是一种内部包含CPU、存储器和输入/输出接口等电路的集成电路(IC芯片) 单片机是单片微型计算机(Single Chip Microcomputer)的简称,用于控制领域,所以又称为微型控制器(Microcontroller U…...
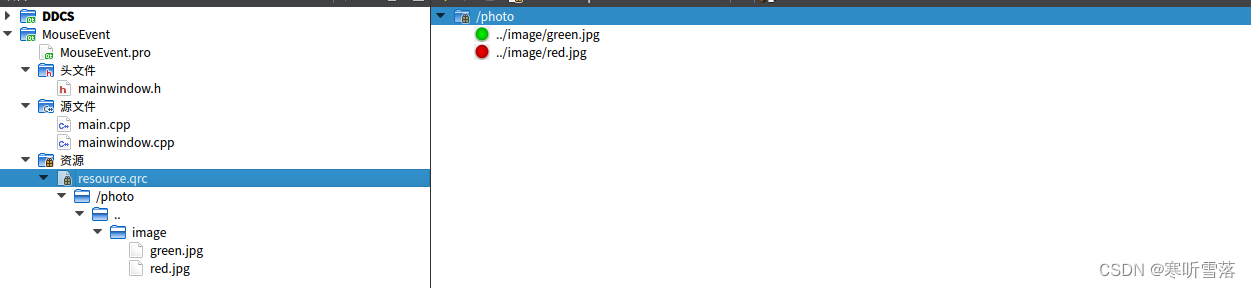
QT资源添加调用
添加资源文件,新建资源文件夹,命名resource,然后点下一步,点完成 资源,右键add Prefix 添加现有文件 展示的label图片切换 QLabel *led_show; #include "mainwindow.h" #include<QLabel> #include&l…...

LeetCode-49. 字母异位词分组【数组 哈希表 字符串 排序】
LeetCode-49. 字母异位词分组【数组 哈希表 字符串 排序】 题目描述:解题思路一:哈希表和排序,这里最关键的点是,乱序单词的排序结果必然是一样的(从而构成哈希表的key)。解题思路二:解题思路三…...

绘制特征曲线-ROC(Machine Learning 研习十七)
接收者操作特征曲线(ROC)是二元分类器的另一个常用工具。它与精确度/召回率曲线非常相似,但 ROC 曲线不是绘制精确度与召回率的关系曲线,而是绘制真阳性率(召回率的另一个名称)与假阳性率(FPR&a…...

.Net 知识杂记
记录平日中琐碎的.net 知识点。不定期更新 目标框架名称(TFM) 我们创建C#应用程序时,在项目的工程文件(*.csproj)中都有targetFramework标签,以表示项目使用的目标框架 各种版本的TFM .NET Framework .NET Standard .NET5 及更高版本 UMP等 参考文档&a…...
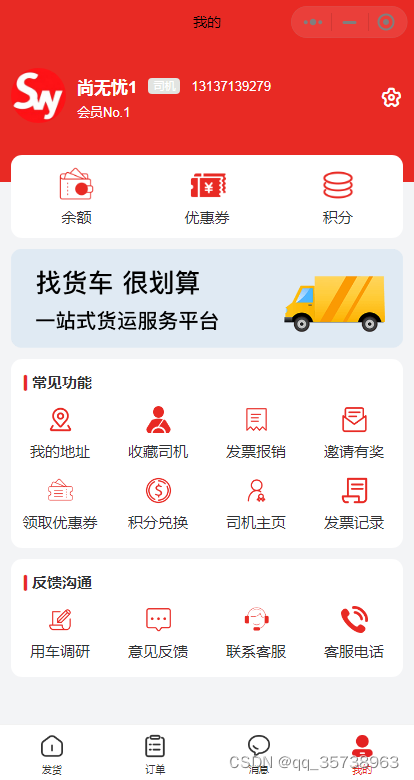
海豚【货运系统源码】货运小程序【用户端+司机端app】源码物流系统搬家系统源码师傅接单
技术栈:前端uniapp后端vuethinkphp 主要功能: 不通车型配置不通价格参数 多城市定位服务 支持发货地 途径地 目的地智能费用计算 支持日期时间 预约下单 支持添加跟单人数选择 支持下单优惠券抵扣 支持司机收藏订单评价 支持订单状态消息通知 支…...
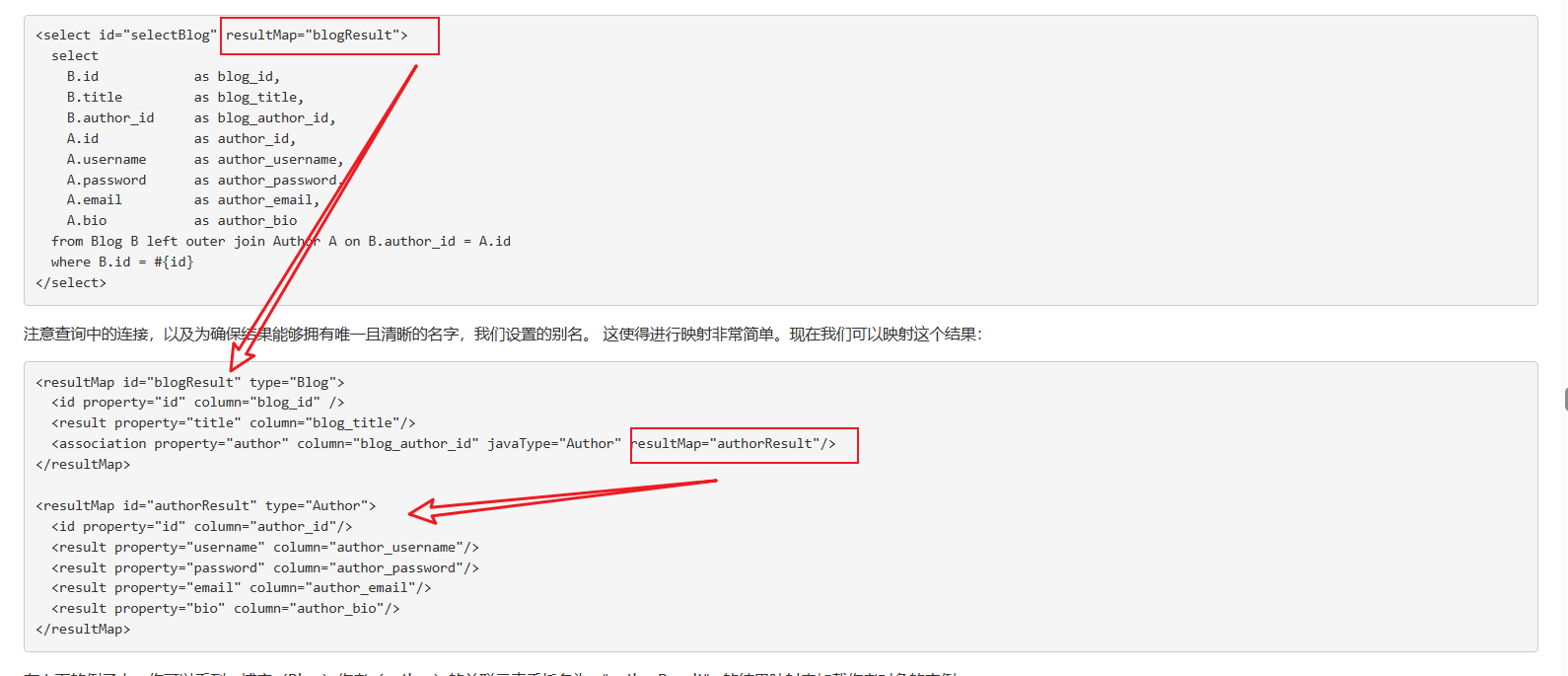
01---java面试八股文——mybatis-------10题
1、什么是MyBatis Mybatis是一个半ORM(对象关系映射)框架,它内部封装了JDBC,开发时只需要关注SQL语句本身,不需要花费精力去处理加载驱动、创建连接、创建statement等繁杂的过程。程序员直接编写原生态sql,…...
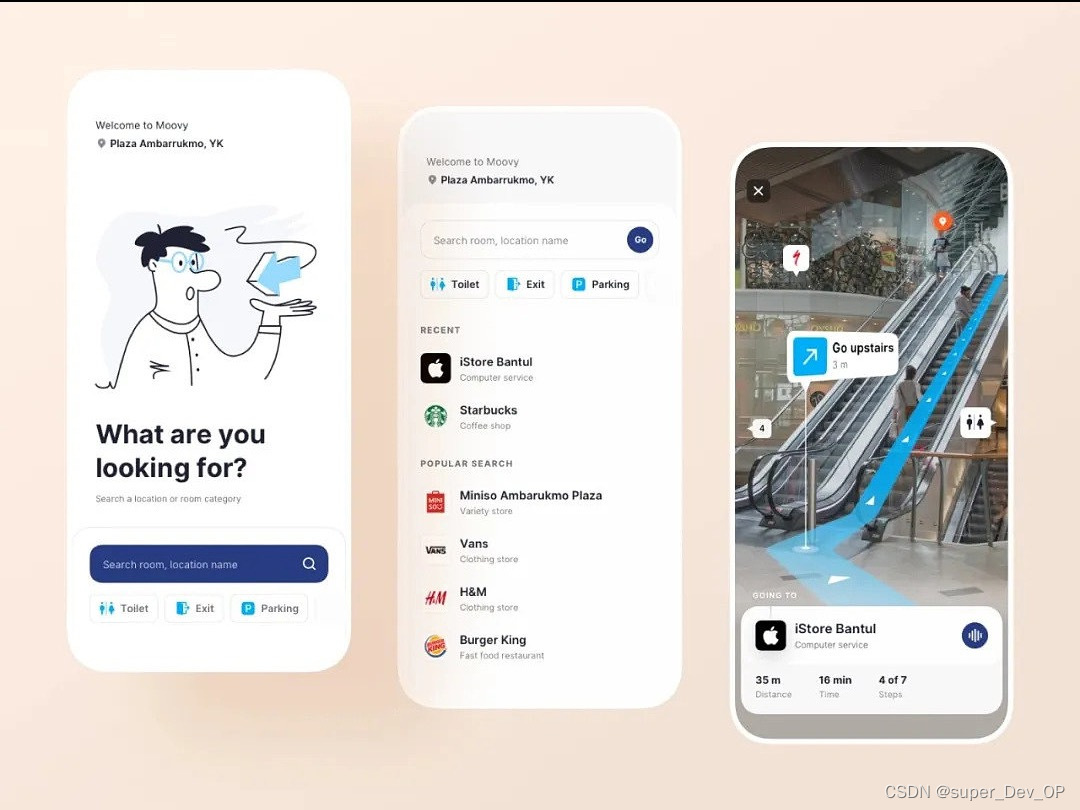
增强现实(AR)的开发工具
增强现实(AR)的开发工具涵盖了一系列的软件和平台,它们可以帮助开发者创造出能够将虚拟内容融入现实世界的应用程序。以下是一些在AR领域内广泛使用的开发工具。北京木奇移动技术有限公司,专业的软件外包开发公司,欢迎…...
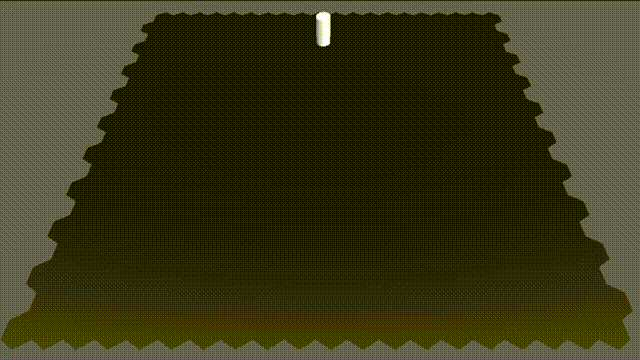
用Unity制作正六边形拼成的地面
目录 效果演示 1.在Unity中创建正六边形 2.创建一个用于管理正六边形的类 3.创建一个用于管理正六边形地面的类 4.创建一个空对象并将游戏控制脚本挂上 5.设置正六边形碰撞所需组件 6.创建正六边形行为触发脚本并挂上 7.创建圆柱体——田伯光 8.创建圆柱体移动脚本 运…...

Spark部署详细教程
Spark Local环境部署 下载地址 https://dlcdn.apache.org/spark/spark-3.4.2/spark-3.4.2-bin-hadoop3.tgz 条件 PYTHON 推荐3.8JDK 1.8 Anaconda On Linux 安装 本次课程的Python环境需要安装到Linux(虚拟机)和Windows(本机)上 参见最下方, 附: Anaconda On Linux 安装…...
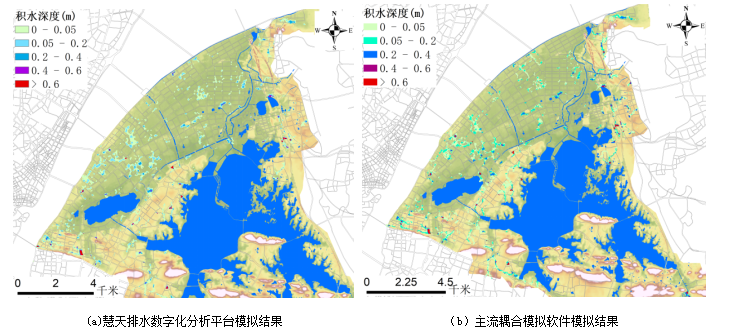
慧天[HTWATER]:创新城市水务科技,引领行业变革
【城市内涝水文水动力模型介绍】 慧天[HTWATER]软件:慧天排水数字化分析平台针对城市排水系统基础设施数据管理的需求,以及水文、水力及水质模拟对数据的需求,实现了以数据库方式对相应数据的存储。可以对分流制排水系统及合流制排水系统进行…...

vscode调试Unity
文章目录 vscode调试UnityC#环境需求开始调试 Lua添加Debugger环境配置联系.txt文件配置Java环境 添加调试代码断点不生效的问题 vscode调试Unity C# 现在使用vscode调试Unity的C#代码很简单,直接在vscode的EXTENSIONS里面搜索“Unity”,第一个就是&am…...

JavaScript是如何实现页面渲染的
JavaScript实现页面渲染主要涉及到对DOM的操作、样式的修改以及与后端数据的交互。以下是JavaScript实现页面渲染的主要步骤和方式: 一、DOM操作 创建和修改元素:JavaScript可以使用document.createElement()来创建新的DOM元素,使用appendC…...
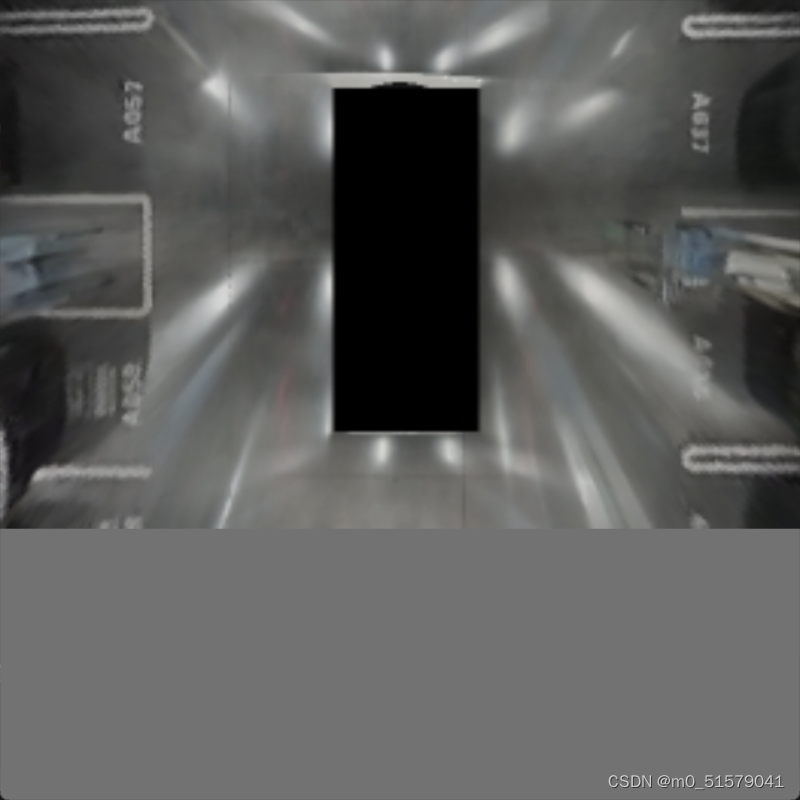
【YOLOv8 代码解读】数据增强代码梳理
1. LetterBox增强 当输入图片的尺寸和模型实际接收的尺寸可能不一致时,通常需要使用LetterBox增强技术。具体步骤是先将图片按比例缩放,将较长的边缩放到设定的尺寸以后,再将较短的边进行填充,最终短边的长度为stride的倍数即可。…...
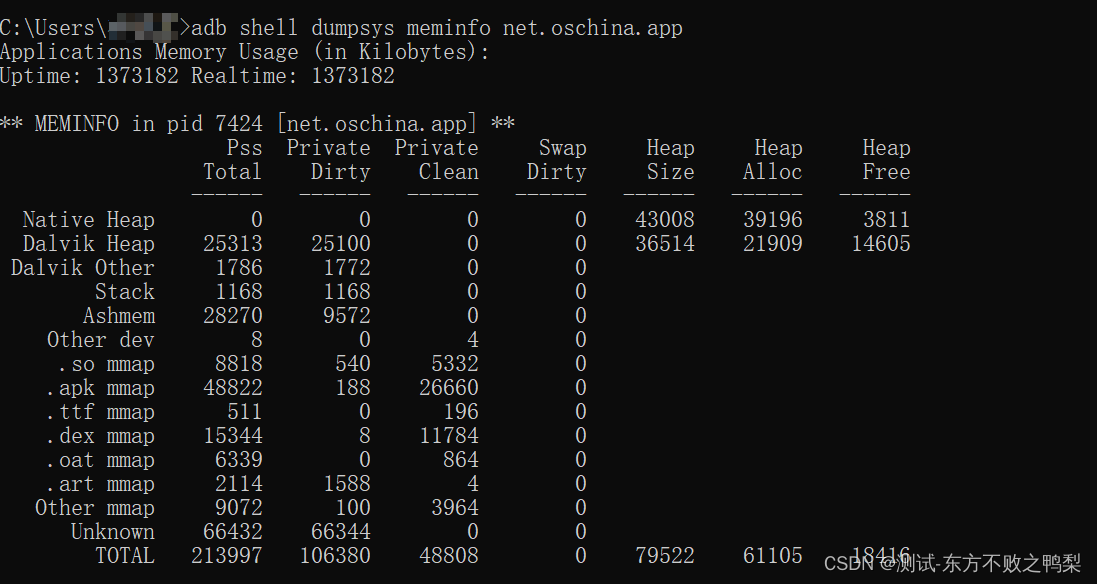
安卓调试桥ADB
Logcat 命令行工具 | Android Studio | Android Developers 什么是ADB ADB 全称为 Android Debug Bridge ,是 Android SDK (安卓的开发工具)中的一个工具,起到调试桥的作用,是一个 客户端 - 服务器端程序 。其中 …...
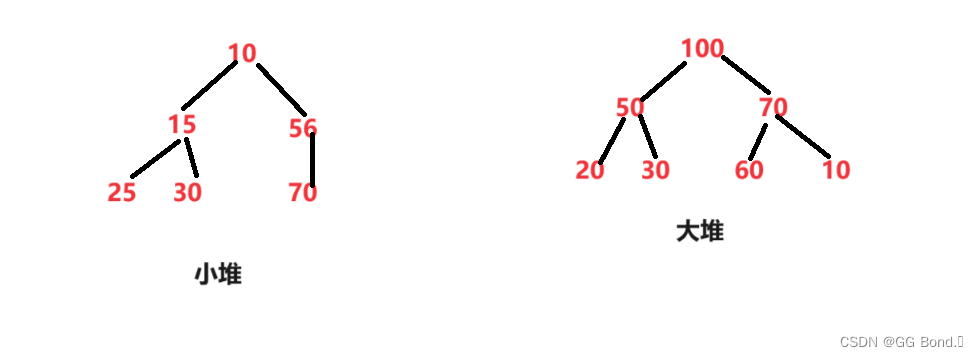
深入理解数据结构第一弹——二叉树(1)——堆
前言: 在前面我们已经学习了数据结构的基础操作:顺序表和链表及其相关内容,今天我们来学一点有些难度的知识——数据结构中的二叉树,今天我们先来学习二叉树中堆的知识,这部分内容还是非常有意思的,下面我们…...
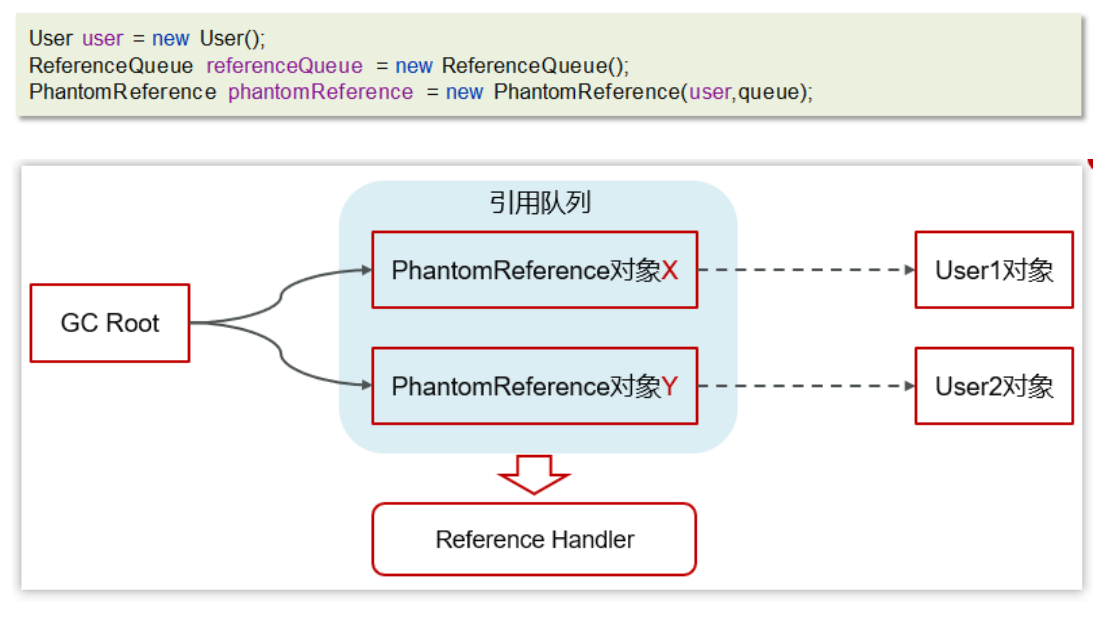
面试题:JVM的垃圾回收
一、GC概念 为了让程序员更专注于代码的实现,而不用过多的考虑内存释放的问题,所以,在Java语言中,有了自动的垃圾回收机制,也就是我们熟悉的GC(Garbage Collection)。 有了垃圾回收机制后,程序员只需要关…...
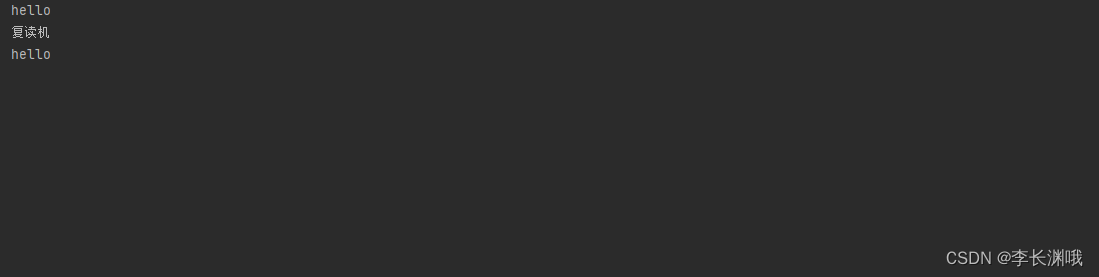
Java8之接口默认方法
Java8之接口默认方法 一、介绍二、代码1、接口2、实现类3、测试代码4、效果 一、介绍 在Java8中,允许为接口方法提供一个默认的实现。必须用default修饰符标记这样一个方法。默认方法也可以调用其他方法 二、代码 1、接口 public interface PersonService {void…...

发挥ChatGPT潜力:高效撰写学术论文技巧
ChatGPT无限次数:点击直达 发挥ChatGPT潜力:高效撰写学术论文技巧 在当今信息爆炸的时代,如何高效撰写学术论文成为许多研究者关注的焦点。而随着人工智能技术的不断发展,如何利用ChatGPT这一先进的技术工具来提升论文写作效率,成…...
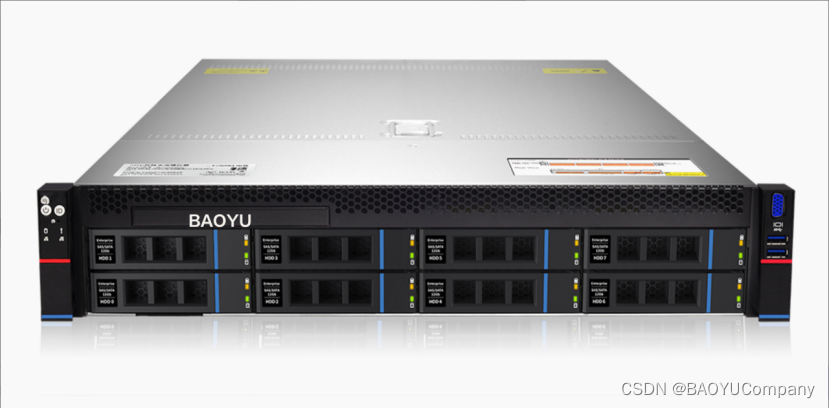
国产暴雨AI服务器X3418开启多元自主可控新篇章
在当前数字化转型的大潮中,算力作为新质生产力的重要动力引擎,对推动经济社会发展起着关键作用。尤其在人工智能领域,随着高性能、安全可控的AI算力需求持续攀升,国产化服务器的研发与应用显得尤为迫切。 作为国内专业的算力基础…...

webpack-dev-server 如何直接用IP打开
当你需要使用IP来访问服务器时,可能需要对 webpack-dev-server 进行相关设置; 当你使用PD虚拟机在Windows上调试时,可能会用到; 一、设置 host 通过webpack.config.js设置 devServer: {host: 0.0.0.0, }通过CLI设置 webpack-dev-s…...

Web框架开发-BBS项目预备知识
一、简介 博客系统(cnblog) https://www.cnblogs.com/ 1.django ORM (object relation mapping 对象关系映射) 表 = 类 对象 = 记录跨表查询 分组查询 annotate() 聚合查询 aggregate(*args, **kwargs) 2.bootstrap3.Ajax (jquery javascript) --- javascript 去写…...

力扣208---实现Trie(前缀树)
题目描述: Trie(发音类似 "try")或者说 前缀树 是一种树形数据结构,用于高效地存储和检索字符串数据集中的键。这一数据结构有相当多的应用情景,例如自动补完和拼写检查。 请你实现 Trie 类: …...
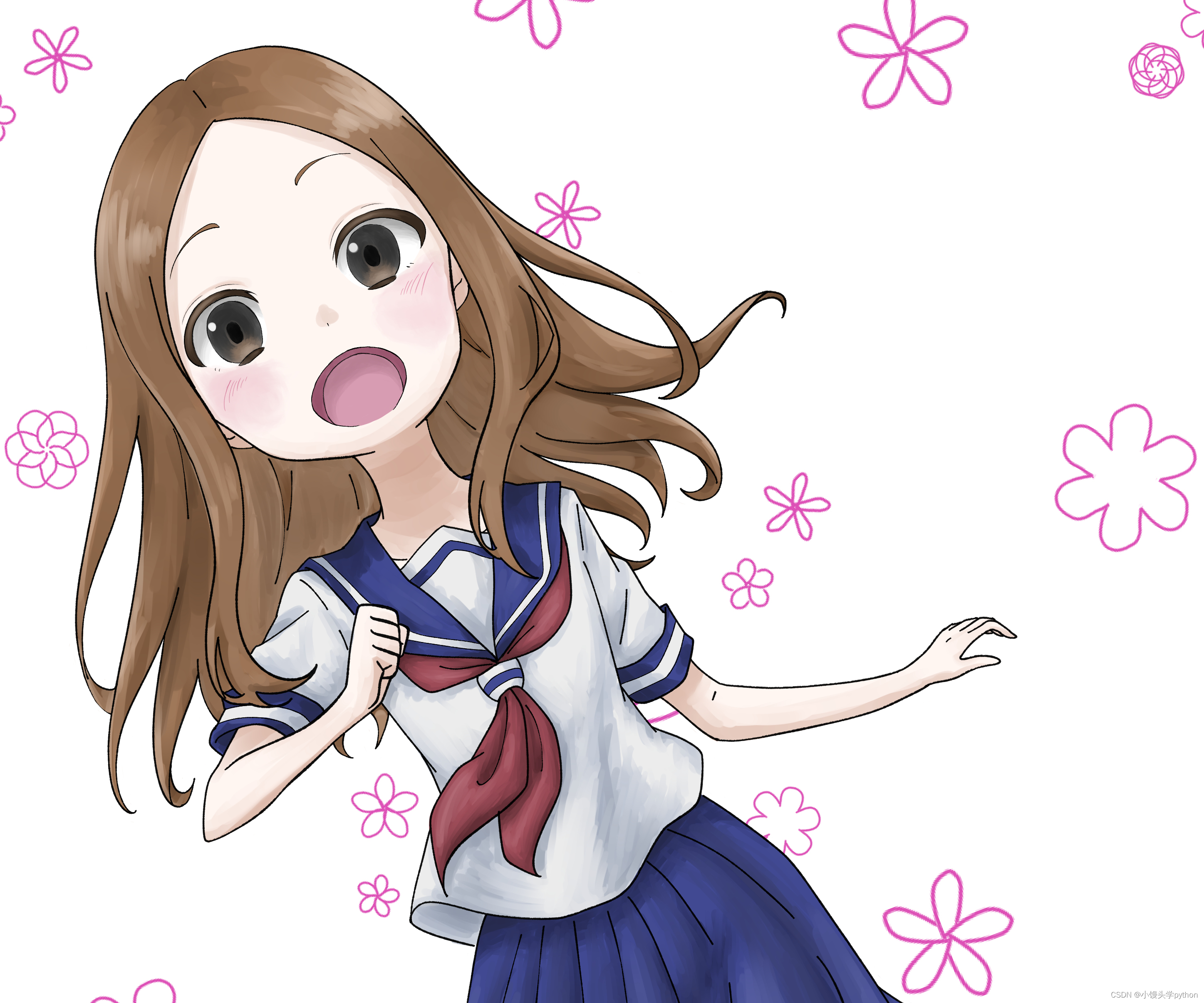
书生·浦语大模型开源体系(一)论文精读笔记
💗💗💗欢迎来到我的博客,你将找到有关如何使用技术解决问题的文章,也会找到某个技术的学习路线。无论你是何种职业,我都希望我的博客对你有所帮助。最后不要忘记订阅我的博客以获取最新文章,也欢…...
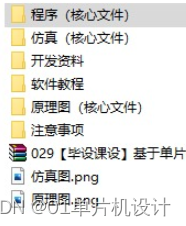
基于单片机模糊算法温度控制系统设计
**单片机设计介绍, 文章目录 一 概要二、功能设计设计思路 三、 软件设计原理图 五、 程序六、 文章目录 一 概要 基于单片机模糊算法温度控制系统设计是一个综合性的项目,结合了单片机技术、传感器技术、模糊控制算法等多个方面。以下是对该设计的概要…...

GESP Python编程四级认证真题 2024年3月
Python 四级 2024 年 03 月 1 单选题(每题 2 分,共 30 分) 第 1 题 小杨的父母最近刚刚给他买了一块华为手表,他说手表上跑的是鸿蒙,这个鸿蒙是?( ) A. 小程序 B. 计时器 C. 操作系统…...
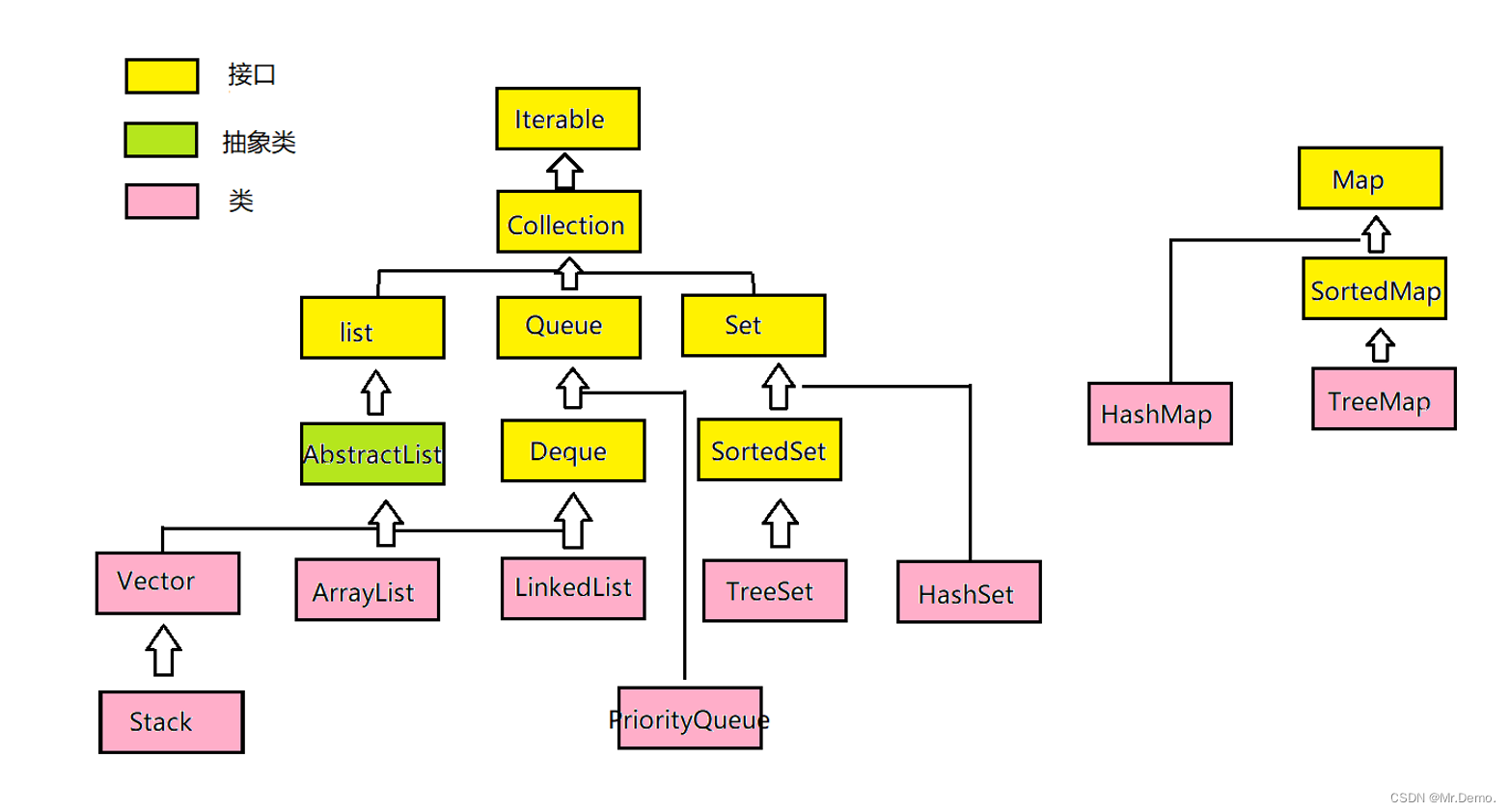
Collection与数据结构 顺序表与ArrayList
1. 线性表 线性表(linear list)是n个具有相同特性的数据元素的有限序列。 线性表是一种在实际中广泛使用的数据结构,常见的线性表:顺序表、链表、栈、队列… 线性表在逻辑上是线性结构,也就说是连续的一条直线。但是在…...