Python酷库之旅-第三方库Pandas(051)
目录
一、用法精讲
186、pandas.Series.is_monotonic_increasing属性
186-1、语法
186-2、参数
186-3、功能
186-4、返回值
186-5、说明
186-6、用法
186-6-1、数据准备
186-6-2、代码示例
186-6-3、结果输出
187、pandas.Series.is_monotonic_decreasing属性
187-1、语法
187-2、参数
187-3、功能
187-4、返回值
187-5、说明
187-6、用法
187-6-1、数据准备
187-6-2、代码示例
187-6-3、结果输出
188、pandas.Series.value_counts方法
188-1、语法
188-2、参数
188-3、功能
188-4、返回值
188-5、说明
188-6、用法
188-6-1、数据准备
188-6-2、代码示例
188-6-3、结果输出
189、pandas.Series.align方法
189-1、语法
189-2、参数
189-3、功能
189-4、返回值
189-5、说明
189-6、用法
189-6-1、数据准备
189-6-2、代码示例
189-6-3、结果输出
190、pandas.Series.case_when方法
190-1、语法
190-2、参数
190-3、功能
190-4、返回值
190-5、说明
190-6、用法
190-6-1、数据准备
190-6-2、代码示例
190-6-3、结果输出
二、推荐阅读
1、Python筑基之旅
2、Python函数之旅
3、Python算法之旅
4、Python魔法之旅
5、博客个人主页
一、用法精讲
186、pandas.Series.is_monotonic_increasing属性
186-1、语法
# 186、pandas.Series.is_monotonic_increasing属性
property pandas.Series.is_monotonic_increasing
Return boolean if values in the object are monotonically increasing.Returns:
bool
186-2、参数
无
186-3、功能
用于判断系列中的值是否按非递减顺序排列。
186-4、返回值
返回一个布尔值,如果系列中的值是单调递增的(即每个值都大于或等于前一个值),返回True;如果系列中的值不是单调递增的,返回False。
186-5、说明
在数据分析过程中,该属性可以用来快速检查数据的趋势,例如时间序列数据是否按时间递增、价格数据是否持续上升等。
186-6、用法
186-6-1、数据准备
无
186-6-2、代码示例
# 186、pandas.Series.is_monotonic_increasing属性
import pandas as pd
# 创建一个单调递增的系列
data_increasing = pd.Series([1, 2, 3, 4, 5])
# 判断系列中的值是否单调递增
is_monotonic_increasing = data_increasing.is_monotonic_increasing
print(f"系列值是否单调递增: {is_monotonic_increasing}")
# 创建一个非单调递增的系列
data_not_increasing = pd.Series([1, 3, 2, 4, 5])
# 判断系列中的值是否单调递增
is_monotonic_increasing = data_not_increasing.is_monotonic_increasing
print(f"系列值是否单调递增: {is_monotonic_increasing}")
186-6-3、结果输出
# 186、pandas.Series.is_monotonic_increasing属性
# 系列值是否单调递增: True
# 系列值是否单调递增: False
187、pandas.Series.is_monotonic_decreasing属性
187-1、语法
# 187、pandas.Series.is_monotonic_decreasing属性
property pandas.Series.is_monotonic_decreasing
Return boolean if values in the object are monotonically decreasing.Returns:
bool
187-2、参数
无
187-3、功能
用于判断系列中的值是否按递减顺序排列。
187-4、返回值
返回一个布尔值,如果系列中的值是单调递减的(即每个值都小于或等于前一个值),返回True;
如果系列中的值不是单调递减的,返回False
。
187-5、说明
在数据分析过程中,该属性可以用来快速检查数据的趋势,例如库存数据是否持续减少、销售数据是否下降等。
187-6、用法
187-6-1、数据准备
无
187-6-2、代码示例
# 187、pandas.Series.is_monotonic_decreasing属性
import pandas as pd
# 创建一个单调递减的系列
data_decreasing = pd.Series([5, 4, 3, 2, 1])
# 判断系列中的值是否单调递减
is_monotonic_decreasing = data_decreasing.is_monotonic_decreasing
print(f"系列值是否单调递减: {is_monotonic_decreasing}")
# 创建一个非单调递减的系列
data_not_decreasing = pd.Series([5, 3, 4, 2, 1])
# 判断系列中的值是否单调递减
is_monotonic_decreasing = data_not_decreasing.is_monotonic_decreasing
print(f"系列值是否单调递减: {is_monotonic_decreasing}")
187-6-3、结果输出
# 187、pandas.Series.is_monotonic_decreasing属性
# 系列值是否单调递减: True
# 系列值是否单调递减: False
188、pandas.Series.value_counts方法
188-1、语法
# 188、pandas.Series.value_counts方法
pandas.Series.value_counts(normalize=False, sort=True, ascending=False, bins=None, dropna=True)
Return a Series containing counts of unique values.The resulting object will be in descending order so that the first element is the most frequently-occurring element. Excludes NA values by default.Parameters:
normalize
bool, default False
If True then the object returned will contain the relative frequencies of the unique values.sort
bool, default True
Sort by frequencies when True. Preserve the order of the data when False.ascending
bool, default False
Sort in ascending order.bins
int, optional
Rather than count values, group them into half-open bins, a convenience for pd.cut, only works with numeric data.dropna
bool, default True
Don’t include counts of NaN.Returns:
Series
188-2、参数
188-2-1、normalize(可选,默认值为False):如果设置为True
,返回每个唯一值的相对频率;否则返回绝对值的计数。
188-2-2、sort(可选,默认值为True):如果设置为True
,结果按计数值排序;如果设置为False
,则按唯一值本身排序。
188-2-3、ascending(可选,默认值为False):如果设置为True
,结果按升序排序;如果设置为False
(默认值),则按降序排序。
188-2-4、bins(可选,默认值为None):如果设置为整数,则会将数据分成相应数量的区间,并返回每个区间的计数。
188-2-5、dropna(可选,默认值为True):如果设置为True
(默认值),则不计算NaN
值;如果设置为False
,则包括NaN
值的计数。
188-3、功能
188-3-1、计数每个唯一值的出现次数:默认情况下,返回每个唯一值的绝对频率。
188-3-2、排序:默认按计数值降序排列,但可以通过参数设置为升序或按唯一值本身排序。
188-3-3、相对频率:通过设置normalize=True,可以返回每个唯一值的相对频率(比例)。
188-3-4、分箱:通过设置bins参数,可以将数据分成若干区间,并返回每个区间的计数。
188-3-5、处理缺失值:默认情况下,NaN值不包括在计数中,但可以通过设置dropna=False包括NaN值的计数。
188-4、返回值
返回一个pandas.Series对象,索引是原始Series中的唯一值(或区间),值是每个唯一值(或区间)的计数。
188-5、说明
使用场景:
188-5-1、探索性数据分析(EDA):在数据分析的初始阶段,使用value_counts()可以快速了解数据的分布情况。例如,分析客户年龄分布、产品销售量分布等。
188-5-2、数据清洗:在处理缺失值和重复值时,可以使用value_counts()找出异常值或频次较低的值,从而帮助清洗数据。
188-5-3、分类数据分析:对于分类变量(如性别、地区、职业等),可以使用value_counts()计算每个类别的频次,以便进一步的统计分析或可视化。
188-5-4、数据验证:在数据验证过程中,使用value_counts()可以检查数据是否符合预期分布,例如检查试验组和对照组的样本量是否均衡。
188-5-5、异常检测:通过value_counts()计算频次,识别出频次异常的值,有助于发现数据中的潜在问题或异常情况。
188-5-6、特征工程:在特征工程中,可以使用value_counts()生成新的特征,例如,计算某个特征的出现次数,并将其作为新的特征添加到数据集中。
188-5-7、文本数据分析:在自然语言处理任务中,可以使用value_counts()统计词频,分析文本数据的关键词或常用词。
188-5-8、时间序列分析:对于时间序列数据,可以使用value_counts()统计不同时间段内事件的发生频次,例如统计每月的销售订单数量。
188-5-9、用户行为分析:在用户行为分析中,使用value_counts()可以统计用户的操作频次,例如统计用户登录、点击、购买等行为的次数。
188-6、用法
188-6-1、数据准备
无
188-6-2、代码示例
# 188、pandas.Series.value_counts方法
# 188-1、基本用法
import pandas as pd
data = pd.Series([1, 2, 2, 3, 3, 3, 4, 4, 4, 4])
counts = data.value_counts()
print(counts, end='\n\n')# 188-2、返回相对频率
import pandas as pd
data = pd.Series([1, 2, 2, 3, 3, 3, 4, 4, 4, 4])
counts_normalized = data.value_counts(normalize=True)
print(counts_normalized, end='\n\n')# 188-3、按升序排列
import pandas as pd
data = pd.Series([1, 2, 2, 3, 3, 3, 4, 4, 4, 4])
counts_ascending = data.value_counts(ascending=True)
print(counts_ascending, end='\n\n')# 188-4、分箱计数
import pandas as pd
data = pd.Series([1, 2, 2, 3, 3, 3, 4, 4, 4, 4])
counts_bins = data.value_counts(bins=3)
print(counts_bins, end='\n\n')# 188-5、包括NaN值的计数
import pandas as pd
data_with_nan = pd.Series([1, 2, 2, 3, 3, 3, 4, 4, 4, 4, None])
counts_with_nan = data_with_nan.value_counts(dropna=False)
print(counts_with_nan)
188-6-3、结果输出
# 188、pandas.Series.value_counts方法
# 188-1、基本用法
# 4 4
# 3 3
# 2 2
# 1 1
# Name: count, dtype: int64# 188-2、返回相对频率
# 4 0.4
# 3 0.3
# 2 0.2
# 1 0.1
# Name: proportion, dtype: float64# 188-3、按升序排列
# 1 1
# 2 2
# 3 3
# 4 4
# Name: count, dtype: int64# 188-4、分箱计数
# (3.0, 4.0] 4
# (0.996, 2.0] 3
# (2.0, 3.0] 3
# Name: count, dtype: int64# 188-5、包括NaN值的计数
# 4.0 4
# 3.0 3
# 2.0 2
# 1.0 1
# NaN 1
# Name: count, dtype: int64
189、pandas.Series.align方法
189-1、语法
# 189、pandas.Series.align方法
pandas.Series.align(other, join='outer', axis=None, level=None, copy=None, fill_value=None, method=_NoDefault.no_default, limit=_NoDefault.no_default, fill_axis=_NoDefault.no_default, broadcast_axis=_NoDefault.no_default)
Align two objects on their axes with the specified join method.Join method is specified for each axis Index.Parameters:
otherDataFrame or Series
join{‘outer’, ‘inner’, ‘left’, ‘right’}, default ‘outer’
Type of alignment to be performed.left: use only keys from left frame, preserve key order.right: use only keys from right frame, preserve key order.outer: use union of keys from both frames, sort keys lexicographically.inner: use intersection of keys from both frames, preserve the order of the left keys.axisallowed axis of the other object, default None
Align on index (0), columns (1), or both (None).levelint or level name, default None
Broadcast across a level, matching Index values on the passed MultiIndex level.copybool, default True
Always returns new objects. If copy=False and no reindexing is required then original objects are returned.NoteThe copy keyword will change behavior in pandas 3.0. Copy-on-Write will be enabled by default, which means that all methods with a copy keyword will use a lazy copy mechanism to defer the copy and ignore the copy keyword. The copy keyword will be removed in a future version of pandas.You can already get the future behavior and improvements through enabling copy on write pd.options.mode.copy_on_write = Truefill_valuescalar, default np.nan
Value to use for missing values. Defaults to NaN, but can be any “compatible” value.method{‘backfill’, ‘bfill’, ‘pad’, ‘ffill’, None}, default None
Method to use for filling holes in reindexed Series:pad / ffill: propagate last valid observation forward to next valid.backfill / bfill: use NEXT valid observation to fill gap.Deprecated since version 2.1.limitint, default None
If method is specified, this is the maximum number of consecutive NaN values to forward/backward fill. In other words, if there is a gap with more than this number of consecutive NaNs, it will only be partially filled. If method is not specified, this is the maximum number of entries along the entire axis where NaNs will be filled. Must be greater than 0 if not None.Deprecated since version 2.1.fill_axis{0 or ‘index’} for Series, {0 or ‘index’, 1 or ‘columns’} for DataFrame, default 0
Filling axis, method and limit.Deprecated since version 2.1.broadcast_axis{0 or ‘index’} for Series, {0 or ‘index’, 1 or ‘columns’} for DataFrame, default None
Broadcast values along this axis, if aligning two objects of different dimensions.Deprecated since version 2.1.Returns:
tuple of (Series/DataFrame, type of other)
Aligned objects.
189-2、参数
189-2-1、other(必须):Series或DataFrame,表示需要与调用该方法的对象对齐的另一个对象。
189-2-2、join(可选,默认值为'outer'):{'outer', 'inner', 'left', 'right'}
,指定对齐方式。
189-2-2-1、'outer': 返回两个对象的并集,包含所有索引。
189-2-2-2、'inner': 返回两个对象的交集,仅包含共同的索引。
189-2-2-3、'left': 返回左侧对象的所有索引,缺失的右侧对象的索引用NaN填充。
189-2-2-4、'right': 返回右侧对象的所有索引,缺失的左侧对象的索引用NaN填充。
189-2-3、axis(可选,默认值为None):整数或字符串,仅在DataFrame
中有效,指明对齐的轴,0表示行,1表示列。
189-2-4、level(可选,默认值为None):整数或字符串,如果对象是多层索引,可以使用此参数指定对齐的层次。
189-2-5、copy(可选,默认值为None):如果为True
,则返回的对象会复制;如果为False
,则返回的对象可能共享数据;如果为None
,则根据数据的情况决定是否复制。
189-2-6、fill_value(可选,默认值为None):标量值,如果需要填充缺失的值,可以指定一个填充值。
189-2-7、method(可选):字符串,指定用于填充缺失数据的方法,如'pad'(前向填充)或者'backfill'(后向填充),如果不提供,默认不填充。
189-2-8、limit(可选):整数,用于限制填充的最大数量。
189-2-9、fill_axis(可选):整数或字符串,指定用于填充缺失值的轴,仅在DataFrame
中有效。
189-2-10、broadcast_axis(可选):整数或字符串,指定用于广播操作的轴。
189-3、功能
对齐两个对象,使它们的索引对齐,并允许用户选择如何处理缺失的值。
189-4、返回值
返回一个元组,包含对齐后的两个对象,形如(left, right)
:
left
是对齐后的左侧对象。right
是对齐后的右侧对象。
如果fill_value
被指定,而某些索引无法对齐,则缺失的部分将被填充为指定的值。
189-5、说明
使用场景:
189-5-1、数据合并与连接:在处理来自不同数据源的数据时,可能需要将它们合并成一个统一的格式,使用align
可以确保所有数据都以相同的索引对齐,从而方便后续处理。
189-5-2、时间序列分析:在时间序列数据中,不同的时间序列可能会有不同的时间索引,使用align
方法可以对齐它们,使得每个时间点的值能够方便地进行比较和计算。
189-5-3、数据补齐与填充:当某些数据缺失时,可以使用align
方法结合fill_value
进行补齐,确保后续分析时不会因为缺失值而出现错误。
189-5-4、计算与比较:在进行计算(如差异计算、比例计算等)时,需要对齐各个数据列的索引,使用align
方法可以保证参与计算的数据都是对齐的,从而得到合理的结果。
189-5-5、处理多层索引:对于具有多层索引的Series或DataFrame,可以利用level
参数对特定层次进行对齐,便于复杂数据结构的操作。
189-5-6、数据清理:在清理数据时,需要处理不一致的索引,在删减或重塑数据时,使用align
可以确保所有列都有相同的索引结构。
189-6、用法
189-6-1、数据准备
无
189-6-2、代码示例
# 189、pandas.Series.align方法
# 189-1、合并不同客户的销售数据
import pandas as pd
# 创建两个不同的销售数据 Series
sales_jan = pd.Series([200, 300], index=['Alice', 'Bob'])
sales_feb = pd.Series([150, 400, 250], index=['Alice', 'Charlie', 'Bob'])
# 使用align方法对齐两个Series
aligned_jan, aligned_feb = sales_jan.align(sales_feb, join='outer', fill_value=0)
print("对齐后的1月销售数据:")
print(aligned_jan)
print("\n对齐后的2月销售数据:")
print(aligned_feb)
# 计算每个客户在1月和2月的销售总额
total_sales = aligned_jan + aligned_feb
print("\n客户总销售额:")
print(total_sales, end='\n\n')# 189-2、时间序列对比
import pandas as pd
# 创建两个时间序列数据Series
temperature = pd.Series([22, 24, 23], index=pd.date_range('2023-01-01', periods=3))
humidity = pd.Series([30, 32], index=pd.date_range('2023-01-01', periods=2))
# 使用align方法对齐两个时间序列
aligned_temp, aligned_hum = temperature.align(humidity, join='inner')
print("对齐后的温度数据:")
print(aligned_temp)
print("\n对齐后的湿度数据:")
print(aligned_hum)
# 计算温度与湿度的关系
correlation = aligned_temp.corr(aligned_hum)
print(f"\n温度与湿度的相关性:{correlation}", end='\n\n')# 189-3、处理多层索引
import pandas as pd
# 创建多层索引的Series
index = pd.MultiIndex.from_tuples([('A', 1), ('A', 2), ('B', 1), ('B', 2)])
data1 = pd.Series([10, 20, 30, 40], index=index)
index2 = pd.MultiIndex.from_tuples([('A', 1), ('B', 1), ('B', 3)])
data2 = pd.Series([5, 25, 15], index=index2)
# 对齐多层索引的Series
aligned_data1, aligned_data2 = data1.align(data2, join='outer', fill_value=0)
print("对齐后的第一组数据:")
print(aligned_data1)
print("\n对齐后的第二组数据:")
print(aligned_data2)
# 计算对齐后数据的和
sum_data = aligned_data1 + aligned_data2
print("\n对齐后数据的和:")
print(sum_data, end='\n\n')# 189-4、数据清理与补齐
import pandas as pd
# 创建带有缺失值的Series
data1 = pd.Series([1, 2, None, 4], index=['A', 'B', 'C', 'D'])
data2 = pd.Series([None, 2, 3, None], index=['A', 'B', 'C', 'E'])
# 对齐两个Series,使用fill_value填充缺失值
aligned_data1, aligned_data2 = data1.align(data2, join='outer', fill_value=0)
print("对齐后的数据1:")
print(aligned_data1)
print("\n对齐后的数据2:")
print(aligned_data2)
# 计算补齐后的总和
total = aligned_data1 + aligned_data2
print("\n补齐后的数据总和:")
print(total, end='\n\n')# 189-5、数据比较
import pandas as pd
# 创建两个带有不同客户的销售数据Series
sales_last_month = pd.Series([300, 500, 200], index=['Alice', 'Bob', 'Charlie'])
sales_this_month = pd.Series([350, 450, 300, 100], index=['Alice', 'Bob', 'David', 'Charlie'])
# 对齐两个Series
aligned_last_month, aligned_this_month = sales_last_month.align(sales_this_month, join='outer', fill_value=0)
print("上个月的销售数据:")
print(aligned_last_month)
print("\n这个月的销售数据:")
print(aligned_this_month)
# 计算销售增长
sales_growth = aligned_this_month - aligned_last_month
print("\n销售增长(这个月 - 上个月):")
print(sales_growth, end='\n\n')# 189-6、索引重命名与对齐
import pandas as pd
# 创建两个带有不同索引的Series
data_a = pd.Series([5, 10, 15], index=['x', 'y', 'z'])
data_b = pd.Series([1, 2, 3], index=['y', 'z', 'w'])
# 重命名索引以便于理解
data_a.index = ['Item_A1', 'Item_A2', 'Item_A3']
data_b.index = ['Item_B1', 'Item_B2', 'Item_B3']
# 使用align方法对齐
aligned_a, aligned_b = data_a.align(data_b, join='outer', fill_value=0)
print("对齐后的数据A:")
print(aligned_a)
print("\n对齐后的数据B:")
print(aligned_b)
# 计算A和B的和
total_data = aligned_a + aligned_b
print("\n对齐后数据A和B的和:")
print(total_data, end='\n\n')# 189-7、填补缺失数据
import pandas as pd
# 创建一个带有缺失值的Series
series_a = pd.Series([1, 2, 3, None], index=['A', 'B', 'C', 'D'])
series_b = pd.Series([None, 2, None, 4, 5], index=['A', 'B', 'C', 'E', 'F'])
# 对齐两个Series,使用fill_value填充缺失值
aligned_a, aligned_b = series_a.align(series_b, join='outer', fill_value=0)
print("对齐后的数据A:")
print(aligned_a)
print("\n对齐后的数据B:")
print(aligned_b)
# 计算合并后的序列总和
filled_data = aligned_a + aligned_b
print("\n填补缺失值后的数据总和:")
print(filled_data, end='\n\n')# 189-8、在数据科学项目中的应用
import pandas as pd
# 创建Product价格数据和销量数据
prices = pd.Series([100, 150, 200], index=['Product_A', 'Product_B', 'Product_C'])
sales = pd.Series([10, 5, 8, 3], index=['Product_A', 'Product_B', 'Product_D', 'Product_C'])
# 对齐价格和销量数据
aligned_prices, aligned_sales = prices.align(sales, join='outer', fill_value=0)
print("对齐后的价格数据:")
print(aligned_prices)
print("\n对齐后的销量数据:")
print(aligned_sales)
# 计算每个产品的销售收入
revenue = aligned_prices * aligned_sales
print("\n每个产品的销售收入:")
print(revenue, end='\n\n')
189-6-3、结果输出
# 189、pandas.Series.align方法
# 189-1、合并不同客户的销售数据
# 对齐后的1月销售数据:
# Alice 200.0
# Bob 300.0
# Charlie 0.0
# dtype: float64
#
# 对齐后的2月销售数据:
# Alice 150
# Bob 250
# Charlie 400
# dtype: int64
#
# 客户总销售额:
# Alice 350.0
# Bob 550.0
# Charlie 400.0
# dtype: float64# 189-2、时间序列对比
# 对齐后的温度数据:
# 2023-01-01 22
# 2023-01-02 24
# Freq: D, dtype: int64
#
# 对齐后的湿度数据:
# 2023-01-01 30
# 2023-01-02 32
# Freq: D, dtype: int64
#
# 温度与湿度的相关性:0.9999999999999999# 189-3、处理多层索引
# 对齐后的第一组数据:
# A 1 10.0
# 2 20.0
# B 1 30.0
# 2 40.0
# 3 0.0
# dtype: float64
#
# 对齐后的第二组数据:
# A 1 5.0
# 2 0.0
# B 1 25.0
# 2 0.0
# 3 15.0
# dtype: float64
#
# 对齐后数据的和:
# A 1 15.0
# 2 20.0
# B 1 55.0
# 2 40.0
# 3 15.0
# dtype: float64# 189-4、数据清理与补齐
# 对齐后的数据1:
# A 1.0
# B 2.0
# C 0.0
# D 4.0
# E 0.0
# dtype: float64
#
# 对齐后的数据2:
# A 0.0
# B 2.0
# C 3.0
# D 0.0
# E 0.0
# dtype: float64
#
# 补齐后的数据总和:
# A 1.0
# B 4.0
# C 3.0
# D 4.0
# E 0.0
# dtype: float64# 189-5、数据比较
# 上个月的销售数据:
# Alice 300.0
# Bob 500.0
# Charlie 200.0
# David 0.0
# dtype: float64
#
# 这个月的销售数据:
# Alice 350
# Bob 450
# Charlie 100
# David 300
# dtype: int64
#
# 销售增长(这个月 - 上个月):
# Alice 50.0
# Bob -50.0
# Charlie -100.0
# David 300.0
# dtype: float64# 189-6、索引重命名与对齐
# 对齐后的数据A:
# Item_A1 5.0
# Item_A2 10.0
# Item_A3 15.0
# Item_B1 0.0
# Item_B2 0.0
# Item_B3 0.0
# dtype: float64
#
# 对齐后的数据B:
# Item_A1 0.0
# Item_A2 0.0
# Item_A3 0.0
# Item_B1 1.0
# Item_B2 2.0
# Item_B3 3.0
# dtype: float64
#
# 对齐后数据A和B的和:
# Item_A1 5.0
# Item_A2 10.0
# Item_A3 15.0
# Item_B1 1.0
# Item_B2 2.0
# Item_B3 3.0
# dtype: float64# 189-7、填补缺失数据
# 对齐后的数据A:
# A 1.0
# B 2.0
# C 3.0
# D 0.0
# E 0.0
# F 0.0
# dtype: float64
#
# 对齐后的数据B:
# A 0.0
# B 2.0
# C 0.0
# D 0.0
# E 4.0
# F 5.0
# dtype: float64
#
# 填补缺失值后的数据总和:
# A 1.0
# B 4.0
# C 3.0
# D 0.0
# E 4.0
# F 5.0
# dtype: float64# 189-8、在数据科学项目中的应用
# 对齐后的价格数据:
# Product_A 100.0
# Product_B 150.0
# Product_C 200.0
# Product_D 0.0
# dtype: float64
#
# 对齐后的销量数据:
# Product_A 10
# Product_B 5
# Product_C 3
# Product_D 8
# dtype: int64
#
# 每个产品的销售收入:
# Product_A 1000.0
# Product_B 750.0
# Product_C 600.0
# Product_D 0.0
# dtype: float64
190、pandas.Series.case_when方法
190-1、语法
# 190、pandas.Series.case_when方法
pandas.Series.case_when(caselist)
Replace values where the conditions are True.Parameters:
caselistA list of tuples of conditions and expected replacements
Takes the form: (condition0, replacement0), (condition1, replacement1), … . condition should be a 1-D boolean array-like object or a callable. If condition is a callable, it is computed on the Series and should return a boolean Series or array. The callable must not change the input Series (though pandas doesn`t check it). replacement should be a 1-D array-like object, a scalar or a callable. If replacement is a callable, it is computed on the Series and should return a scalar or Series. The callable must not change the input Series (though pandas doesn`t check it).New in version 2.2.0.Returns:
Series
190-2、参数
190-2-1、caselist(必须):一个包含条件和值的列表,每个元素都是一个元组(condition, value)
,其中,condition
是一个返回布尔值的表达式或函数,用于判断条件是否满足;value
是当条件满足时返回的值。
190-3、功能
根据一系列条件来设置Series
中每个元素的值,并返回一个新的Series
。
190-4、返回值
返回一个新的Series
对象。
190-5、说明
无
190-6、用法
190-6-1、数据准备
无
190-6-2、代码示例
# 190、pandas.Series.case_when方法
import pandas as pd
c = pd.Series([6, 7, 8, 9], name='c')
a = pd.Series([0, 0, 1, 2])
b = pd.Series([0, 3, 4, 5])
c.case_when(caselist=[(a.gt(0), a), (b.gt(0), b)])
print(c)
190-6-3、结果输出
# 190、pandas.Series.case_when方法
# 0 6
# 1 7
# 2 8
# 3 9
# Name: c, dtype: int64
二、推荐阅读
1、Python筑基之旅
2、Python函数之旅
3、Python算法之旅
4、Python魔法之旅
5、博客个人主页
相关文章:

Python酷库之旅-第三方库Pandas(051)
目录 一、用法精讲 186、pandas.Series.is_monotonic_increasing属性 186-1、语法 186-2、参数 186-3、功能 186-4、返回值 186-5、说明 186-6、用法 186-6-1、数据准备 186-6-2、代码示例 186-6-3、结果输出 187、pandas.Series.is_monotonic_decreasing属性 187…...

linux timestamp
驱动或应用中获取时间戳的接口。 #include <stdio.h> #include <stdlib.h> #include <string.h> #include <time.h> #include <sys/time.h> #if 0 #include <linux/ktime.h> /* 内核驱动中获取时间戳 */ static ktime_t get_kernel_time…...

Vue.js 搭建大屏可视化项目
引言 在数字化转型的时代背景下,大屏可视化项目因其直观的数据展示和实时的业务监控能力而变得日益重要。Vue.js,以其简洁的语法、高效的虚拟DOM和强大的组件化能力,成为了构建大屏可视化应用的首选框架之一。本文将从零开始,引导…...
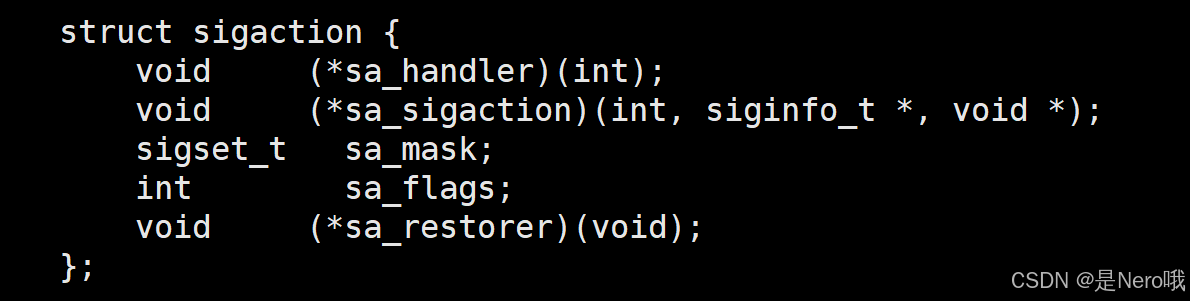
Linux:进程信号(二.信号的保存与处理、递达、volatile关键字、SIGCHLD信号)
上次介绍了:(Linux:进程信号(一.认识信号、信号的产生及深层理解、Term与Core))[https://blog.csdn.net/qq_74415153/article/details/140624810] 文章目录 1.信号保存1.1递达、未决、阻塞等概念1.2再次理解信号产生与保存1.3信号…...
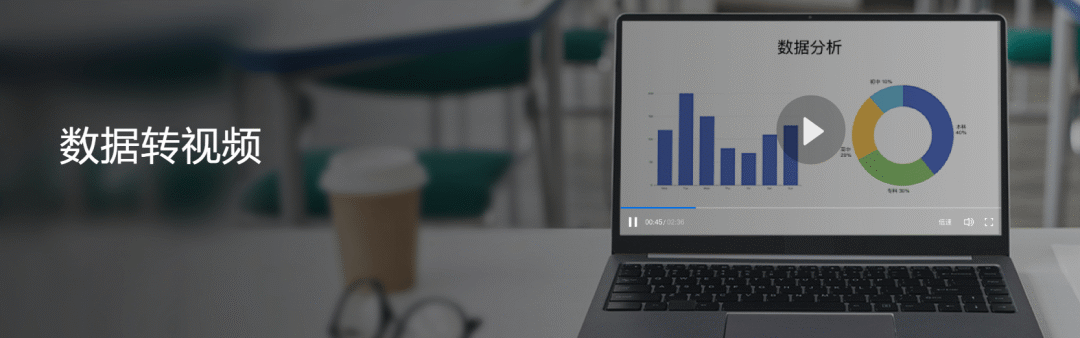
最值得推荐的5个AI大模型API
在这个以人工智能为主导的新时代,选择一个卓越的AI模型API接口,对于企业和个人在AI驱动的商业和技术革新中取得成功至关重要。 在人工智能的浪潮中,大型AI模型API接口正成为推动技术创新和业务发展的重要力量。随着2024年技术的持续进步和应用…...
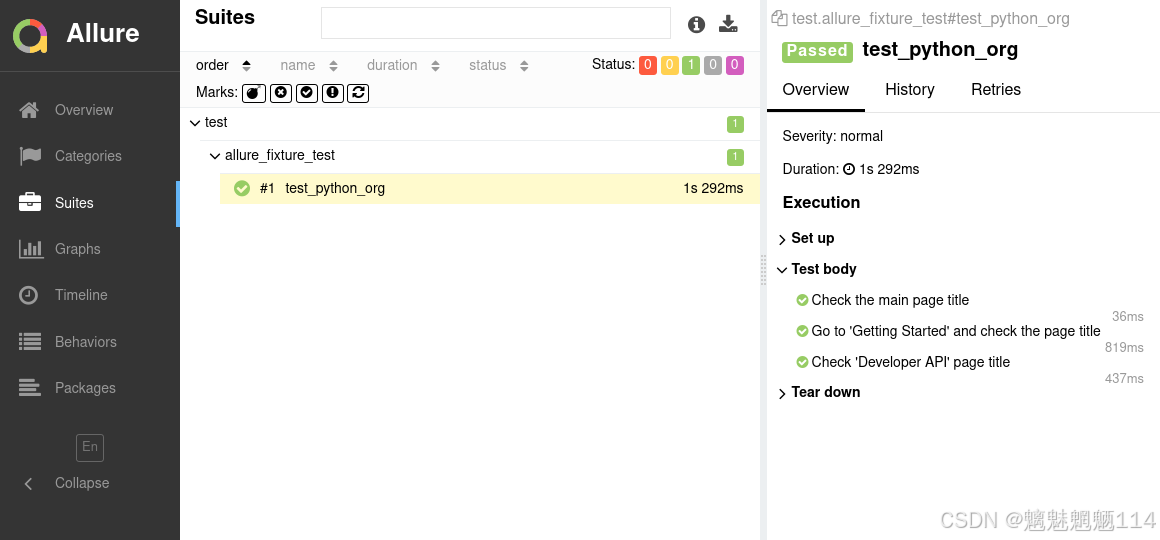
PyTest+Allure生成测试报告
一、官网文档(权威) 1. Allure Report 官网:Allure Report Docs — Introduction 2. Allure GitHub地址:GitHub - allure-framework/allure2: Allure Report is a flexible, lightweight multi-language test reporting tool. It …...

ROS2教程(10) - 编写接收程序、添加frame - Linux
注意 : 本篇文章接上节 (点击此处跳转到上节) 编写接收程序 cpp <the_work_ws>/src/learning_tf2_cpp/src/turtle_tf2_listener.cpp #include <chrono> #include <functional> #include <memory> #include <string>#include "geometry_…...

Arraylist与LinkedList的区别
Arraylist 概念 Arraylist非线程安全Arraylist 底层使用的是Object数组ArrayList 采用数组存储,插入和删除元素的时间复杂度受元素位置的影响ArrayList 支持快速随机访问,就是通过元素的序号快速获取元素对象ArrayList的空间浪费主要体现在列表的结尾会预留一定的容…...
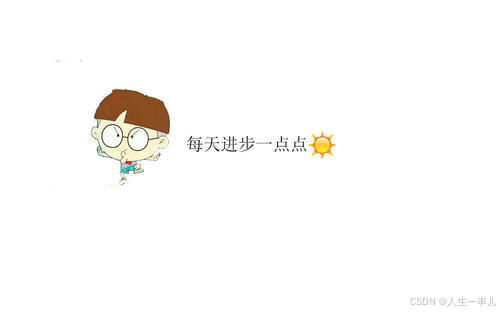
Nestjs使用Redis的最佳实践
前几天在项目中有用到Redis JWT实现服务端对token的主动删除(退出登录功能)。故此介绍下如何在Nestjs中使用Redis,并做下总结。 知识准备 了解Redis - 网上很多简介。了解Nestjs如何使用jwt生成token - 可移步看下我之前的文章 效果展示 一、mac安装与使用 示…...
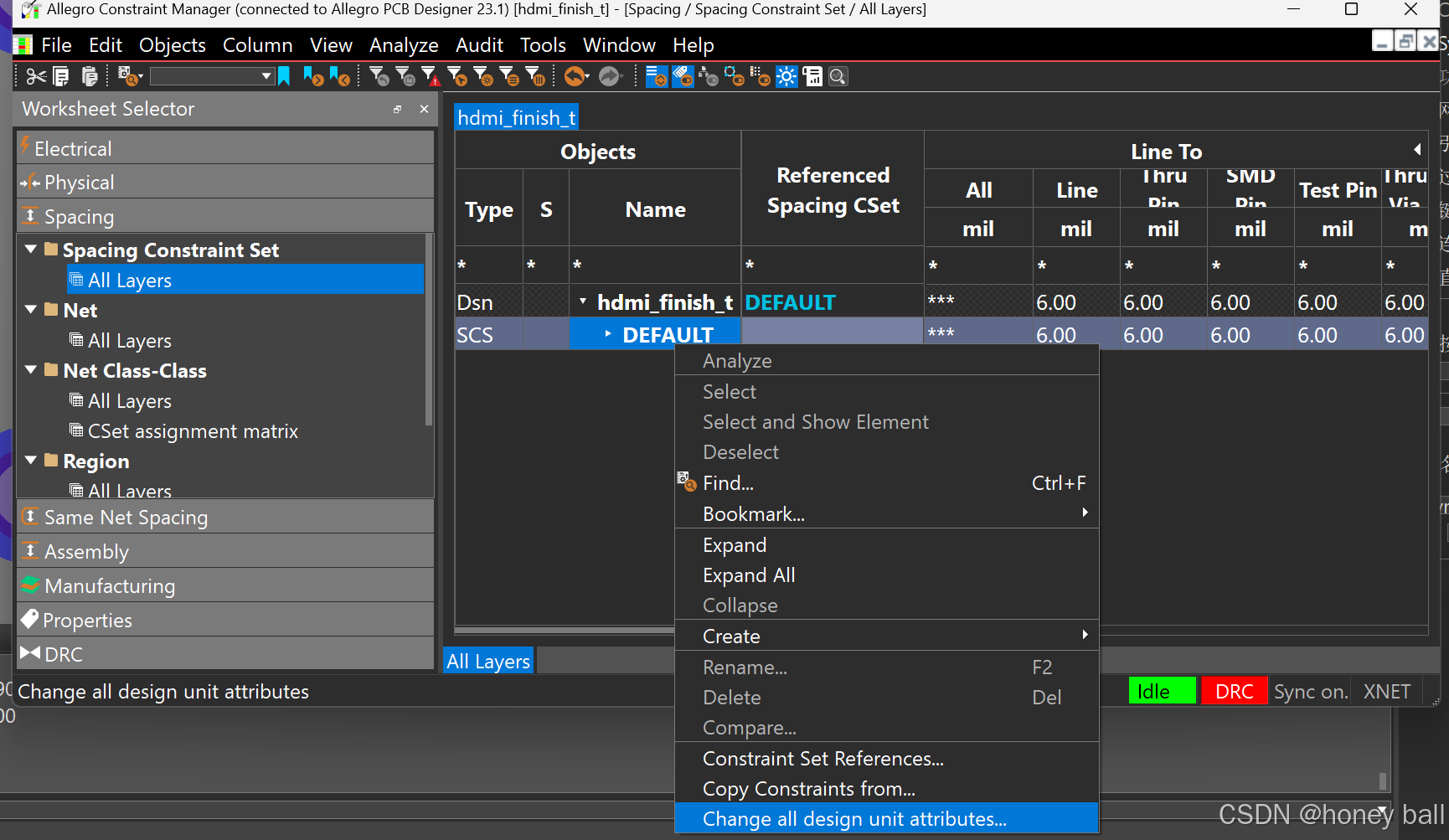
Cadence23学习笔记(十四)
ARC就是圆弧走线的意思: 仅打开网络的话可以只针对net进行修改走线的属性: 然后现在鼠标左键点那个走线,那个走线就会变为弧形: 添加差分对: 之后,分别点击两条线即可分配差分对: 选完差分对之后…...
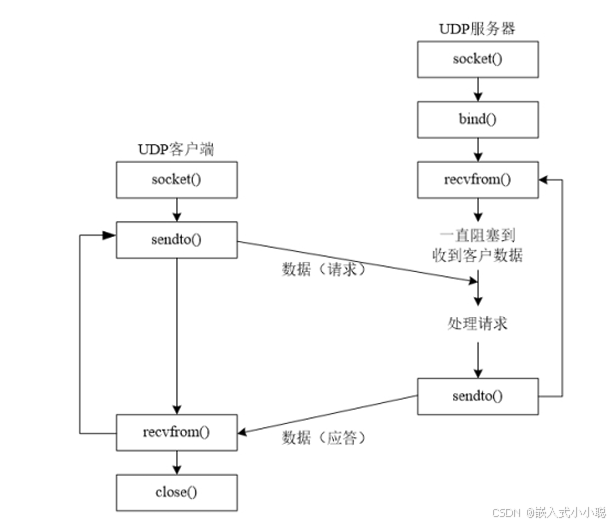
socket 编程
1. socket 套接字 Socket 是一个用于网络通信的技术。Socket 通信允许客户端——服务器之间进行双向通信。它可以使任何客户端机器连接到任何服务器,安装在客户端和服务器两侧的程序就可以实现双向的通信。Socket的作用就是把连接两个计算机的通信软件“中间接”起来…...

如何使用 HTTPie 进行高效的 HTTP 请求
如何使用 HTTPie 进行高效的 HTTP 请求 引言 HTTPie 是一个命令行 HTTP 客户端,它以其简洁的语法和人性化的输出格式赢得了广大开发者的喜爱。与 curl 相比,HTTPie 提供了更加直观和用户友好的接口,使得执行 HTTP 请求变得轻松愉快。本文将…...
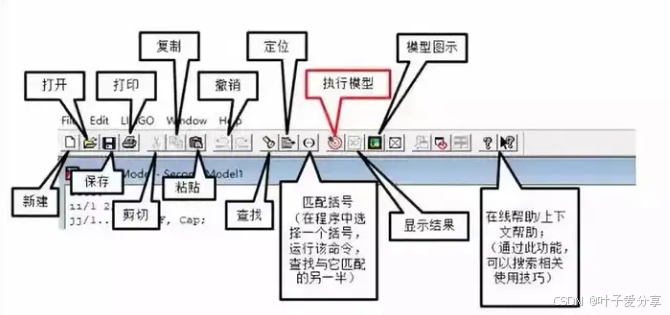
Lingo求解器百度云下载 ling 8.0/lingo 18安装包资源分享
如大家所熟悉的,Lingo是Linear Interaction and General Optimizer的缩写,中文名称为“交互式线性和通用优化求解器”,是一套专门用于求解最优化问题的软件包。 在大部分人认知里,Lingo可用于求解线性规划、二次规划、整数规划、…...
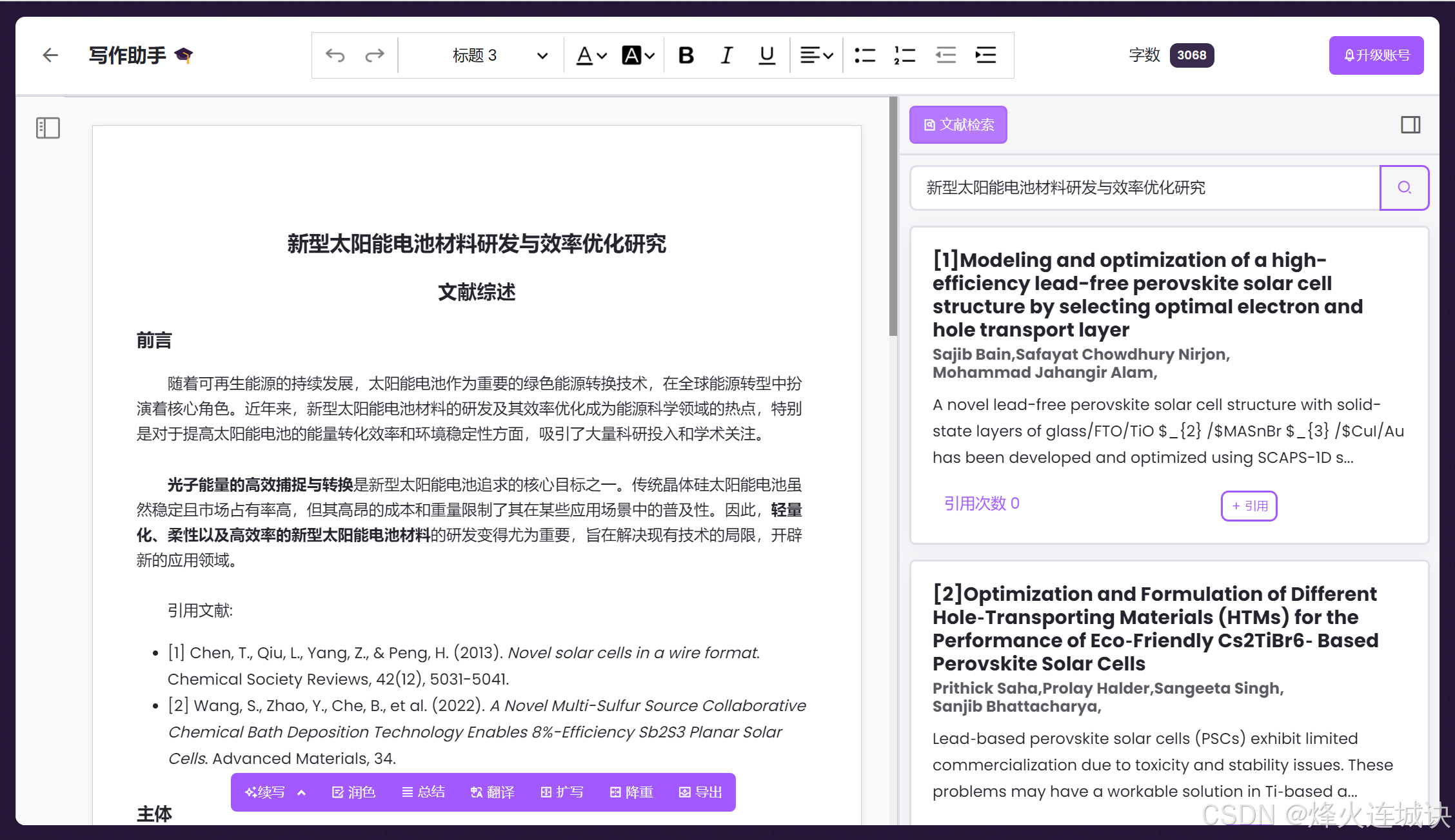
文献综述如何为研究的理论框架做出贡献
VersaBot一键生成文献综述 文献综述在几个关键方面对塑造和巩固研究的理论框架起着至关重要的作用; 1. 识别相关理论和概念: 通过对现有研究的探索,您将遇到与您的主题相关的突出理论和概念。这些可以作为您自己的理论框架的构建块。 2. 理…...
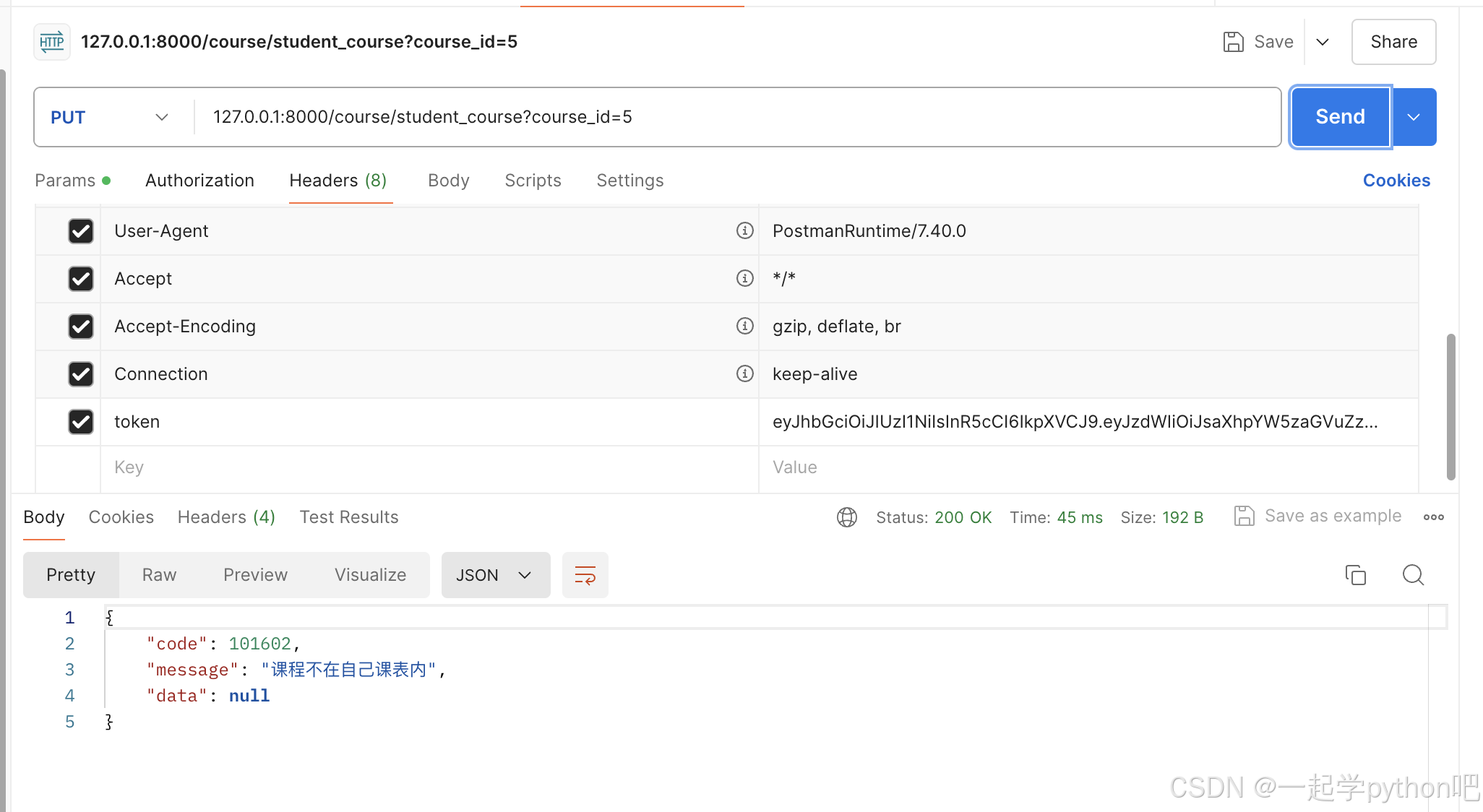
FastAPI(七十九)实战开发《在线课程学习系统》接口开发-- 加入课程和退出课程
源码见:"fastapi_study_road-learning_system_online_courses: fastapi框架实战之--在线课程学习系统" 加入课程 我们先看下加入课程 1.是否登录 2.课程是否存在 3.是否已经存在 4.添加 首先实现逻辑 def get_student_course(db: Session, course: int…...
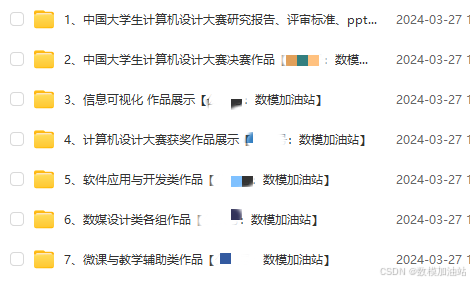
【赛事推荐】2024中国高校计算机大赛人工智能创意赛
“中国高校计算机大赛”(China Collegiate Computing Contest,简称C4)是面向全国高校各专业在校学生的科技类竞赛活动,于2016年由教育部高等学校计算机类专业教学指导委员会、教育部高等学校大学软件工程专业教学指导委员会、教育…...

C++沉思:预处理和编译
预处理和编译 条件编译源代码使用方式典型示例原理 使用static_assert执行编译时断言检查使用方式原理 在C中,编译是将源代码转换为机器代码并组织在目标文件中,然后将目标文件链接在一起生成可执行文件的过程。编译器实际上一次只处理一个文件ÿ…...
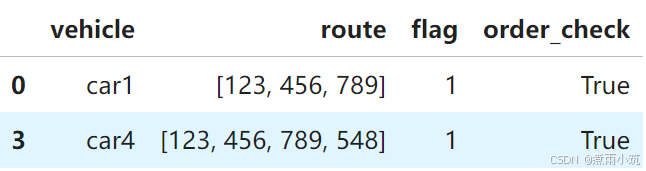
交通数据处理-计算途径某些路段的车辆数
根据车辆的运行轨迹,计算先经过某些路段,再经过某些路段的车辆数。 欢迎关注本人公众号--交通数据探索师 如下表, 其中:vehicle: 车辆编号;route: 车辆轨迹。 以第一行为例,车辆car1按顺序经过了路段123…...
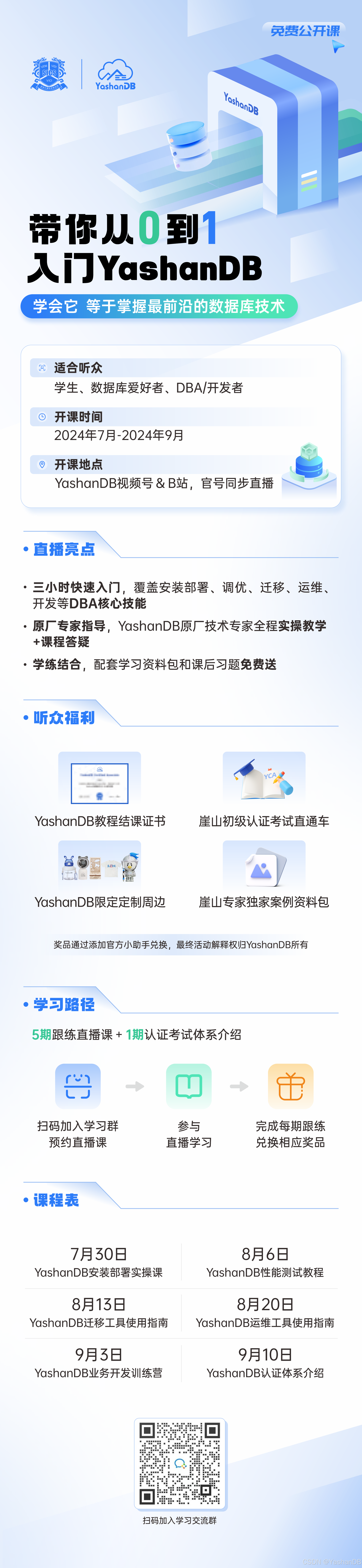
从0到1入门系列 | 崖山公开课再加码,三小时带你入门崖山数据库!
对不断更新的技术心生迷茫 不知如何正确的提升自己? 对新兴的国产数据库领域充满好奇 却不知从何入手? 崖山专家团队精心筹备 《从0到1入门》系列直播课 6节课 三小时 助力数据库小白变身技术高手 掌握最前沿的数据库技术 现在开始 开启职场“金…...

Powershell自定义带参数的别名
提示:文章写完后,目录可以自动生成,如何生成可参考右边的帮助文档 文章目录 前言一、函数二、使用步骤总结 前言 之前写了一篇文章定义别名让powershell尽可能接近Unix风格,增强两者的互操作性,今天给出方法可以定义带…...
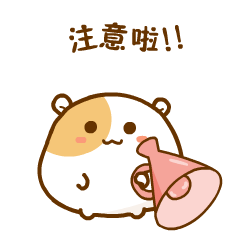
文件操作相关的精讲
目录: 思维导图 一. 文件定义 二. 文件的打开和关闭 三. 文件的顺序读写操作 四. 文件的随机读写操作 五. 文本文件和二进制文件 六. 文件读取结束的判断 七.文件缓冲区 思维导图: 一. 文件定义 1.文件定义 C语言中,文件是指一组相…...
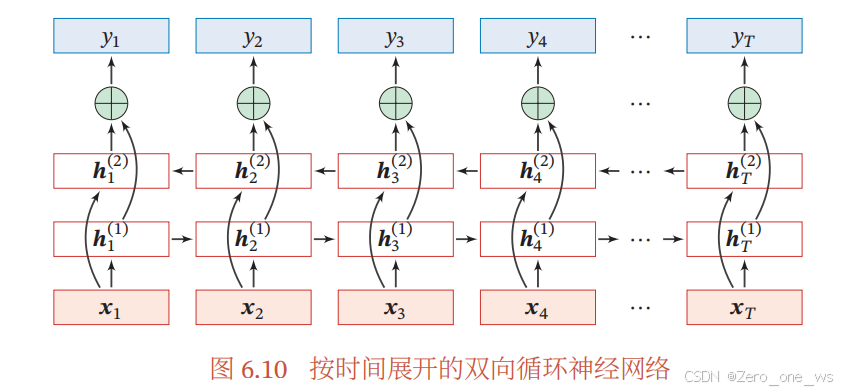
05 循环神经网络
目录 1. 基本概念 2. 简单循环网络 2.1 简单循环网络 2.2 长程依赖问题 3. 循环神经网络的模式与参数学习 3.1 循环神经网络的模式 3.2 参数学习 4. 基于门控的循环神经网络 4.1 长短期记忆网络 4.2 LSTM网络的变体网络 4.3 门控循环单元网络 5. 深层循环神经网络…...

C#初级——条件判断语句、循环语句和运算符
条件判断语句 简单的条件判断语句,if()里面进行条件判断,如果条件判断正确就执行语句块1,如果不符合就执行语句块2。 if (条件判断) { 语句块1 } else { 语句块2 } int age 18;if (age < 18){Console.WriteLine("未…...

Laravel路由模型绑定:简化依赖注入的艺术
Laravel路由模型绑定:简化依赖注入的艺术 引言 在现代Web应用开发中,Laravel框架以其优雅和简洁的代码而闻名。Laravel的路由模型绑定(Route Model Binding)是框架提供的一项强大功能,它允许开发者在路由处理中自动注…...
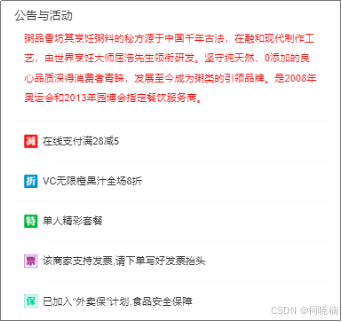
【vue前端项目实战案例】之Vue仿饿了么App
本文将介绍一款仿“饿了么”商家页面的App。该案例是基于 Vue2.0 Vue Router webpack ES6 等技术栈实现的一款外卖类App,适合初学者进行学习。 项目源码下载链接在文章末尾 1 项目概述 该项目是一款仿“饿了么”商家页面的外卖类App,主要有以下功能…...

冷热分离——Java全栈知识(36)
之前在面试的时候有老师问: 我看你使用了水平分表,但是如果有些 1%的数据占了访问量的 90%,而剩下 99%的数据只占了访问量的 10%。这种情况怎么处理。 1 、冷热分离 1.1、什么是冷热分离 冷热分离指的是在处理数据时将数据库分为冷库和热库…...
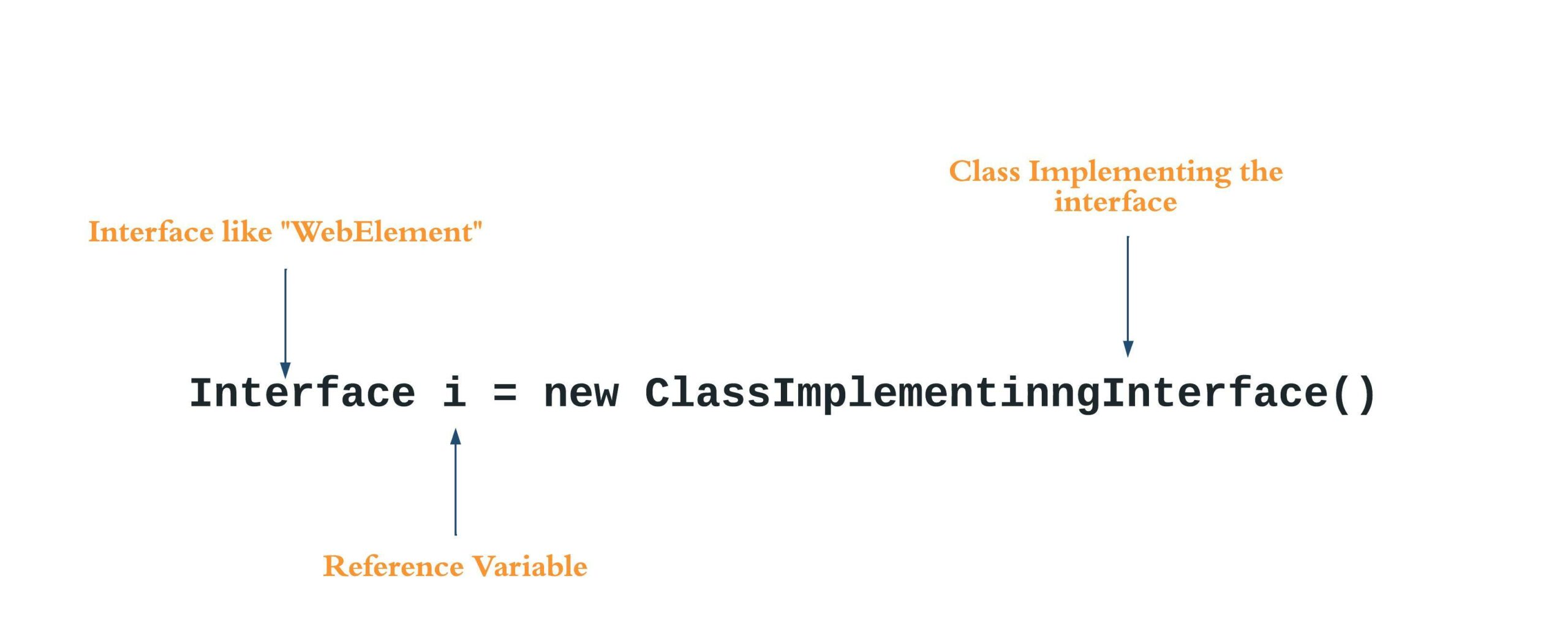
了解Selenium中的WebElement
Selenium中到处都使用WebElement来执行各种操作。什么是WebElement?这篇文章将详细讨论WebElement。 Selenium中的WebElement是一个表示网站HTML元素的Java接口。HTML元素包含一个开始标记和一个结束标记,内容位于这两个标记之间。 HTML元素的重命名 …...
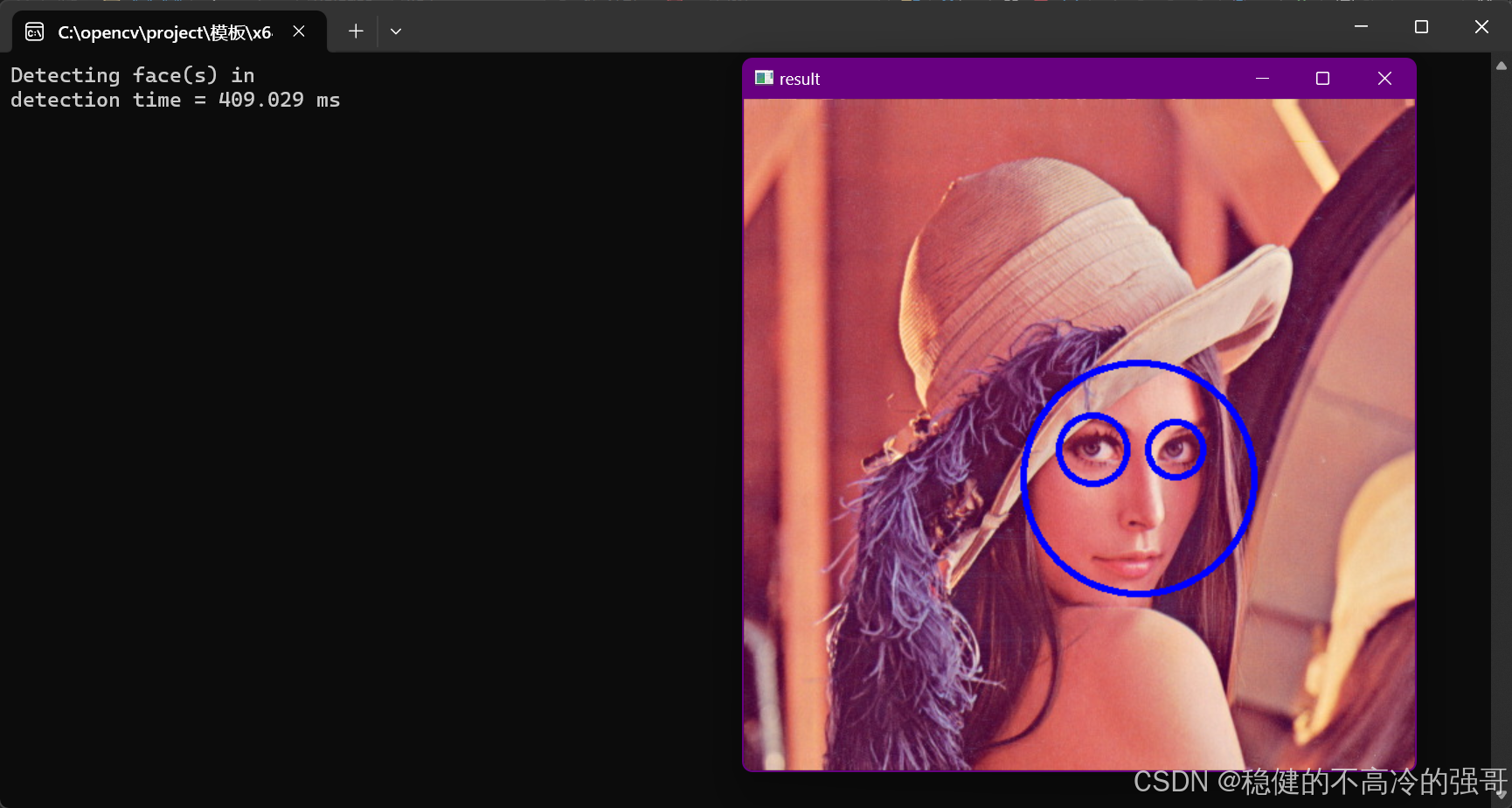
OpenCV facedetect 人脸检测官方示例项目配置
运行程序。该程序会自动打开摄像头,识别并定位摄像头前的人脸以及眼睛部位。 输入q或者Q,退出程序。 或进行文本中所包含的图片路径 或 单个图片进行检测,自行修改代码即可 配置环境项目,debug 解决error C4996: ‘fopen’: This…...

自定义Laravel Artisan风格:打造个性化命令行体验
自定义Laravel Artisan风格:打造个性化命令行体验 引言 Laravel的Artisan命令行工具是开发过程中不可或缺的一部分,它提供了一个强大的接口来执行各种开发、维护、测试等任务。除了执行命令,Artisan还允许开发者自定义命令行输出的风格&…...
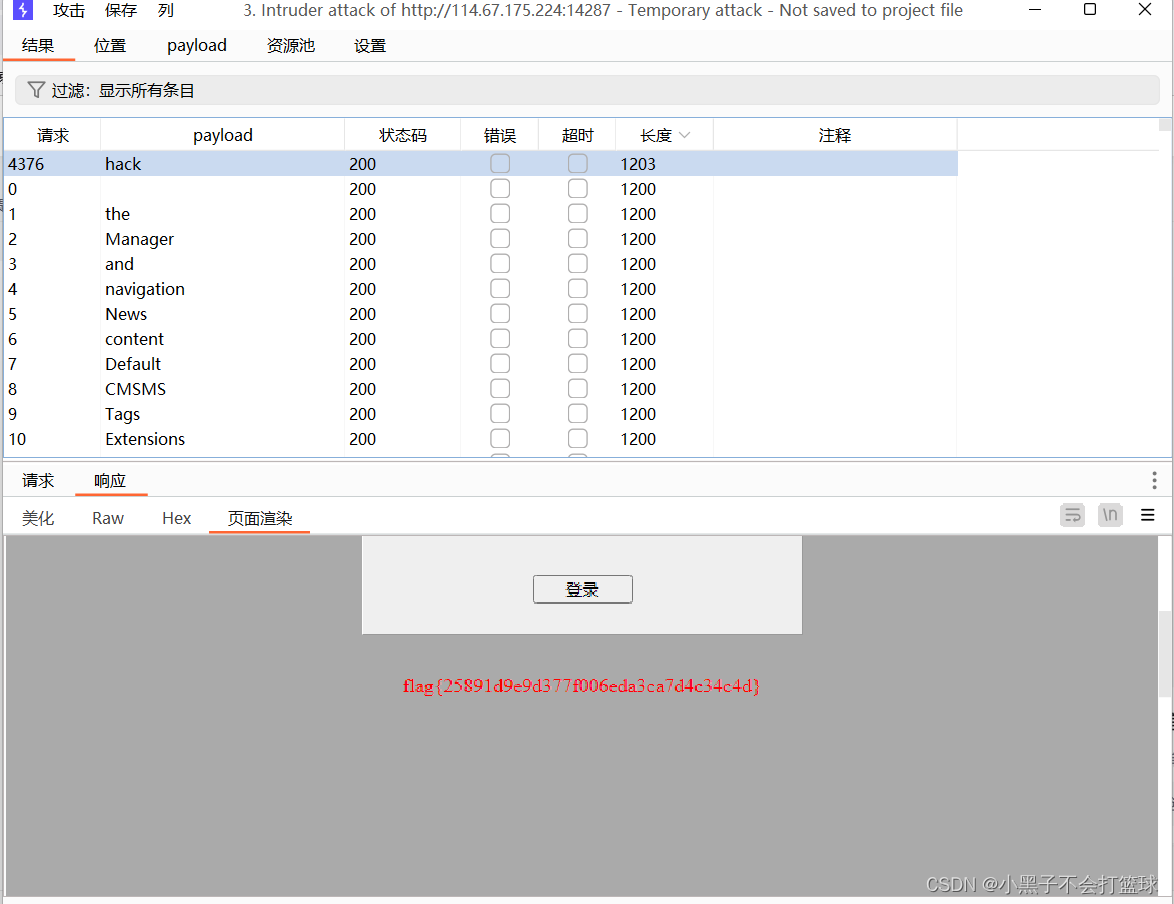
CTF之网站被黑
简单看一下网页和源码没发现什么明显漏洞 那就扫描一下目录 发现了/shell.php文件,访问一下,发现是一个后台管理登录页面 别无他法只能爆破喽,爆破后发现密码是hack flag{25891d9e9d377f006eda3ca7d4c34c4d}...
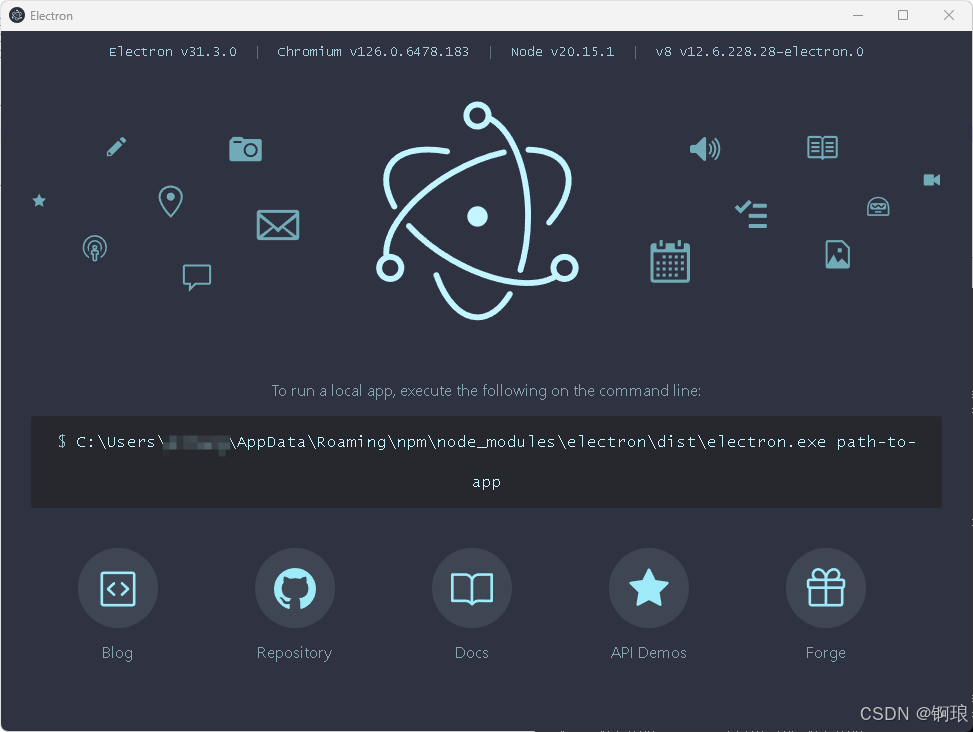
Electron学习笔记(一)基础环境
目录 前言 基础环境准备 安装 Node.js 配置项目文件 通过代理服务安装 通过国内仓库安装 一些常见问题: 前言 一个新手学习Electron的笔记,记录为主,仅供参考。 其他文章见专栏目录。 基础环境准备 开发之前先将基础环境搭建好。 …...
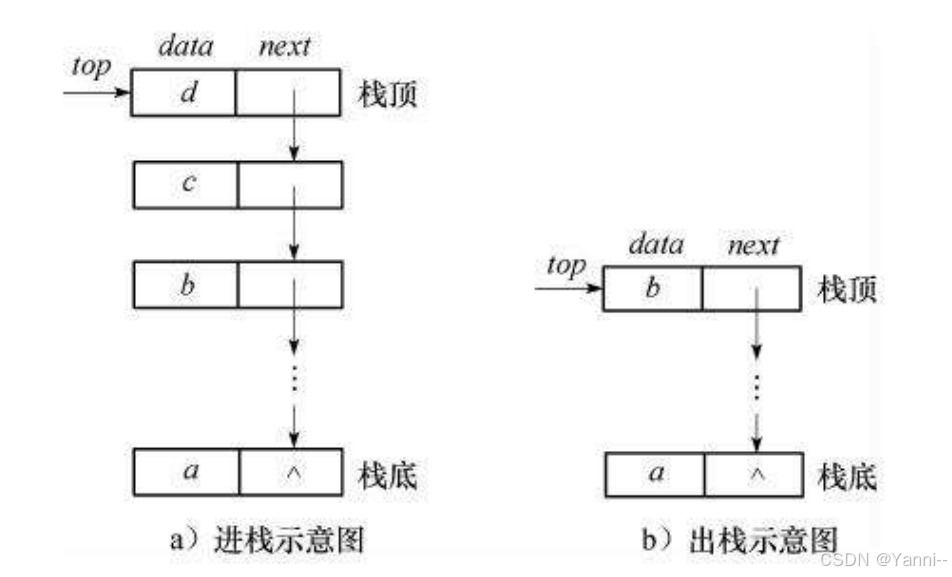
【C语言】栈的实现(数据结构)
前言: 还是举一个生活中的例子,大家都玩过积木,当我们把积木叠起来的时候,如果要拿到最底部的积木,我们必须从顶端一个一个打出,最后才能拿到底部的积木,也就是后进先出(先进后出&a…...
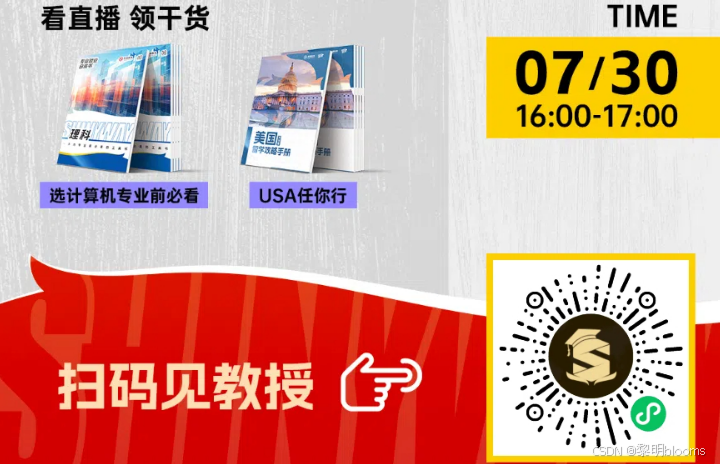
前端三大主流框架对比
在现代前端开发中,React、Vue和Angular是三大流行的框架/库。它们各自有独特的优缺点,适用于不同的开发需求和项目规模。下面是对这三者的详细比较: 一、 React 简介: 由Facebook开发和维护,是一个用于构建用户界面…...

AOP~面向切面编程介绍
AOP基础 概述 AOP:Aspect Oriented Programming(面向切面编程、面向方面编程),面向特定方法的编程。 动态代理是面向切面编程最主流的实现。 SpringAOP是Spring框架的高级技术,旨在管理bean对象的过程中,…...

Android SurfaceFlinger——GraphicBuffer的提交(三十三)
在 SurfaceFlinger 中,我们 dequeueBuffer 和 queueBuffer 是 Surface 控制接口中非常重要的两个函数,分别用于从 Surface 的 BufferQueue 中取出缓冲区和向 BufferQueue 提交(队列)缓冲区。这两个函数在生产者和消费者模型中扮演着核心角色,确保了图像数据的高效和有序传…...
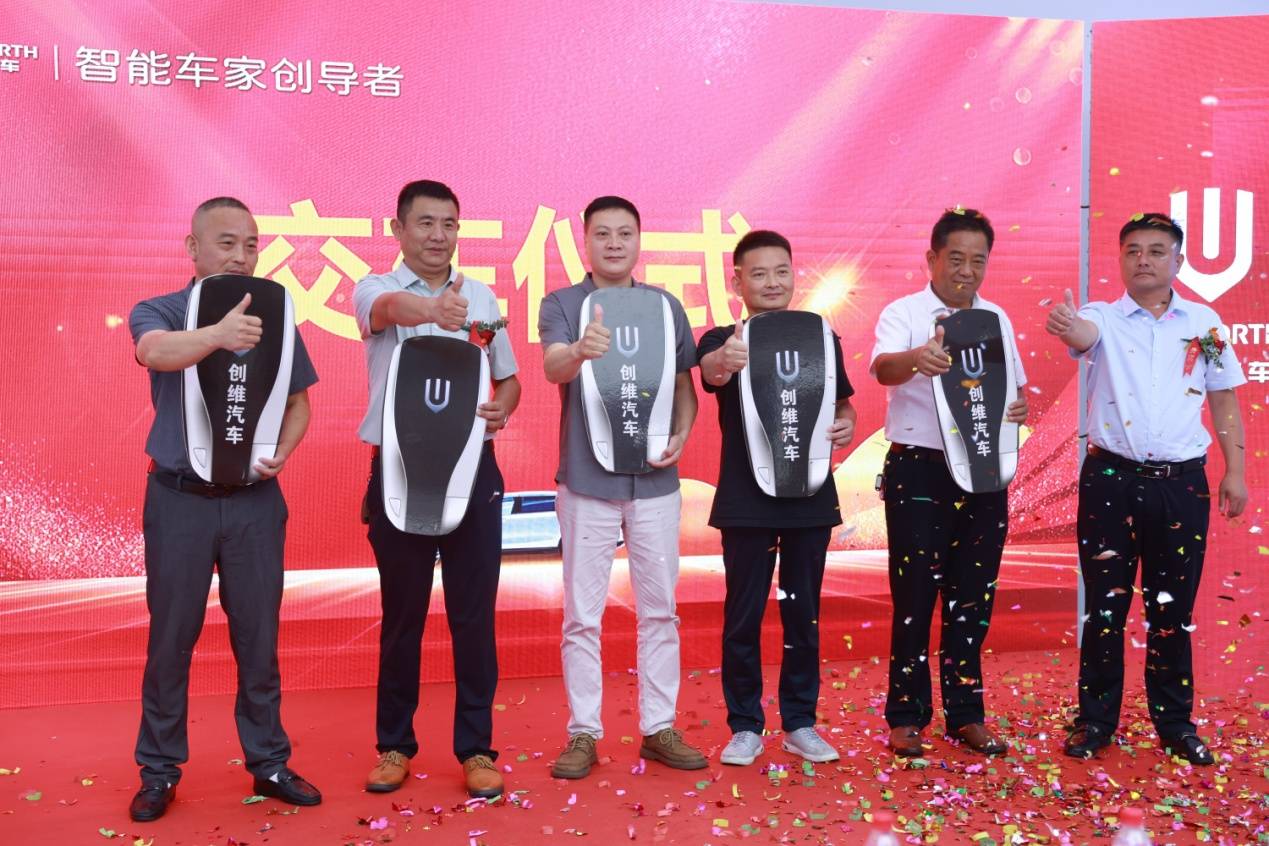
创维汽车滁州永通体验中心开业仪式暨超充车型区域上市会圆满成功
2024年7月20日,创维汽车滁州永通体验中心盛大开业,当日,创维汽车市场部经理周世鹏、安徽大区总监王大明等领导参加本次开业盛典,共同见证创维汽车滁州永通体验中心成功落地。 2021年,新能源乘用车高速发展,…...
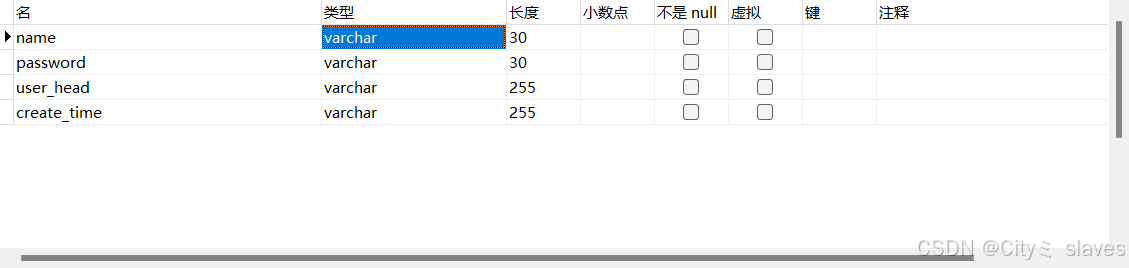
【PHP】系统的登录和注册
一、为什么要学习系统的登录和注册 系统的登录和注册可能存在多种漏洞,这些漏洞可能被恶意攻击者利用,从而对用户的安全和隐私构成威胁。通过学习系统的登录和注册理解整个登录和注册的逻辑方便后续更好站在开发的角度思考问题发现漏洞。以下是一些常见…...

2024.7.29 刷题总结
2024.7.29 **每日一题** 682.棒球比赛,这道题是一道简单的模拟题,用栈模拟题中的四个操作就可以了,操作一是将x加到列表末尾,操作二是将列表的后两项之和加到列表末尾,操作三是把列表最后一项的两倍加到列表末尾&#…...
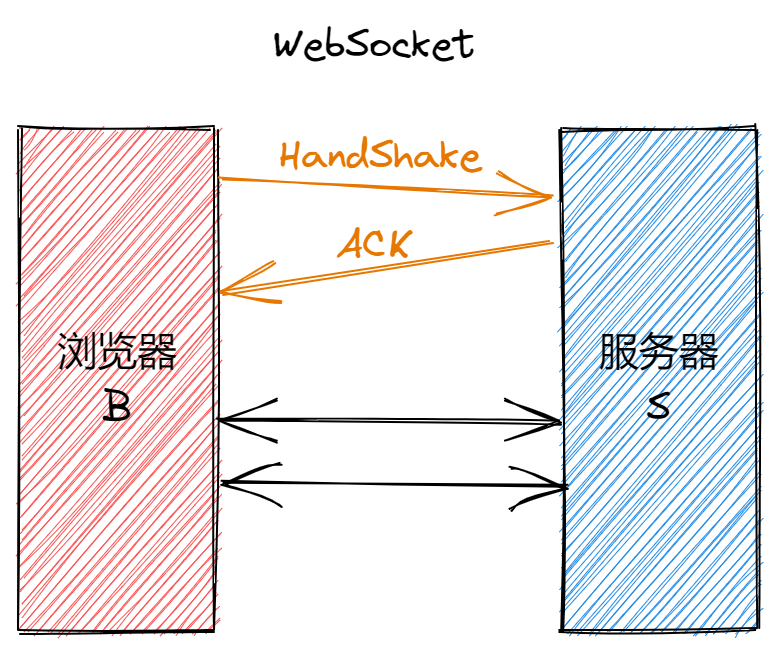
WebSocket程序设计
协议说明 WebSocket 是一种在单个TCP连接上进行全双工通信的协议。WebSocket 使得客户端和服务器之间的数据交换变得更加简单,允许服务端主动向客户端推送数据。Websocket主要用在B/S架构的应用程序中,在 WebSocket API 中,浏览器和服务器只…...
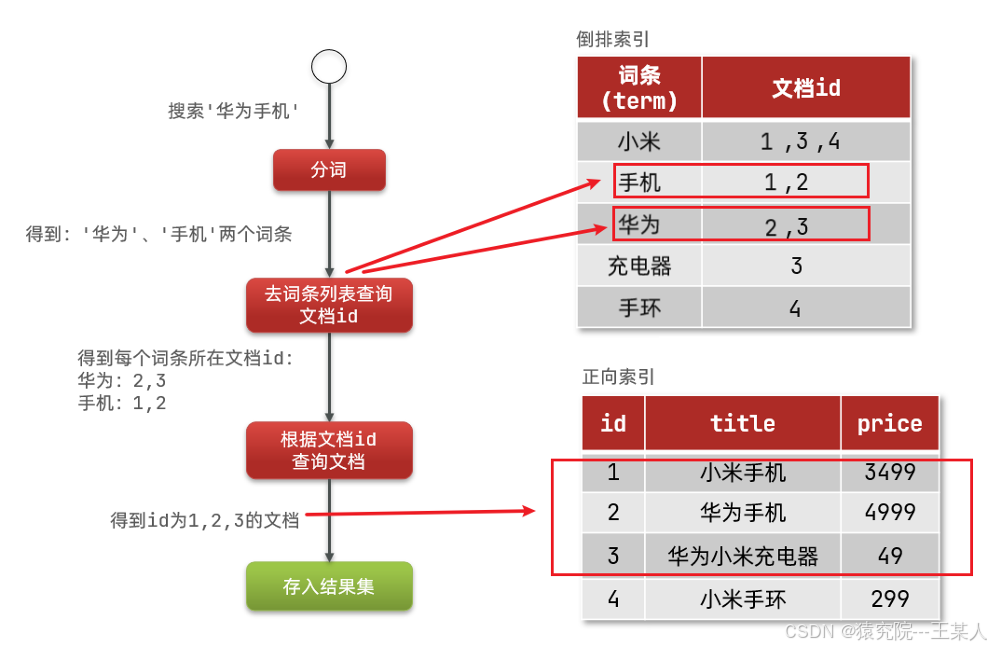
ES(ElasticSearch)倒排索引
目录 正排与倒排索引 1.正排索引 作用: 优点: 缺点: 2.倒排索引 原理: 倒排索引的构建流程: 倒排索引的搜索流程: 优点: 缺点: 3. 应用场景 倒排索引中有几个非常重要的概念…...
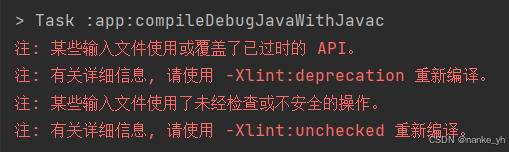
Android Studio Build窗口出现中文乱码问题
刚安装成功的android studio软件打开工程,编译时下方build窗口中中文是乱码。 解决: 可点击studio状态栏的Help—>Edit Custom VM Options ,在打开的studio64.exe.vmoptions文件后面添加:(要注意不能有空格,否则st…...

java生成随机数
代码 startValue 开始值 endValue 结束值 per生成的位数也就是精度 /*** 随机数的生成* param startValue* param endValue* return*/private BigDecimal randomBigDecimal(String startValue, String endValue,int per) {BigDecimal min new BigDecimal(startValue);BigDeci…...

动态定制深度学习:Mojo模型与自定义训练算法的无缝切换
动态定制深度学习:Mojo模型与自定义训练算法的无缝切换 引言 在机器学习领域,算法的选择对模型的性能有着决定性的影响。随着研究的深入和技术的发展,开发者可能需要根据不同的数据特性和业务需求,动态地切换或自定义训练算法。…...

昇思25天学习打卡营第19天|DCGAN生成漫画头像
DCGAN生成漫画头像总结 实验概述 本实验旨在利用深度卷积生成对抗网络(DCGAN)生成动漫头像,通过设置网络、优化器以及损失函数,使用MindSpore进行实现。 实验目的 学习和掌握DCGAN的基本原理和应用。熟悉使用MindSpore进行图像…...

排序题目:按照频率将数组升序排序
文章目录 题目标题和出处难度题目描述要求示例数据范围 解法思路和算法代码复杂度分析 题目 标题和出处 标题:按照频率将数组升序排序 出处:1636. 按照频率将数组升序排序 难度 3 级 题目描述 要求 给定一个整数数组 nums \texttt{nums} nums&a…...

实分析与测度论问题的分类
实分析主要研究实数、实数序列、实数极限以及实值函数的分析,而度量空间则是一个具有距离函数的集合,其分类可以从多个角度进行。 实分析 实分析主要关注实数、实数序列、实数极限以及实值函数的分析。它涉及到多个重要的概念和理论,包括但…...
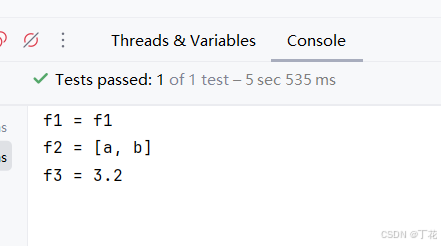
动态代理更改Java方法的返回参数(可用于优化feign调用后R对象的统一处理)
动态代理更改Java方法的返回参数(可用于优化feign调用后R对象的统一处理) 需求原始解决方案优化后方案1.首先创建AfterInterface.java2.创建InvocationHandler处理代理方法3. 调用 实际运行场景拓展 需求 某些场景,调用别人的方法࿰…...
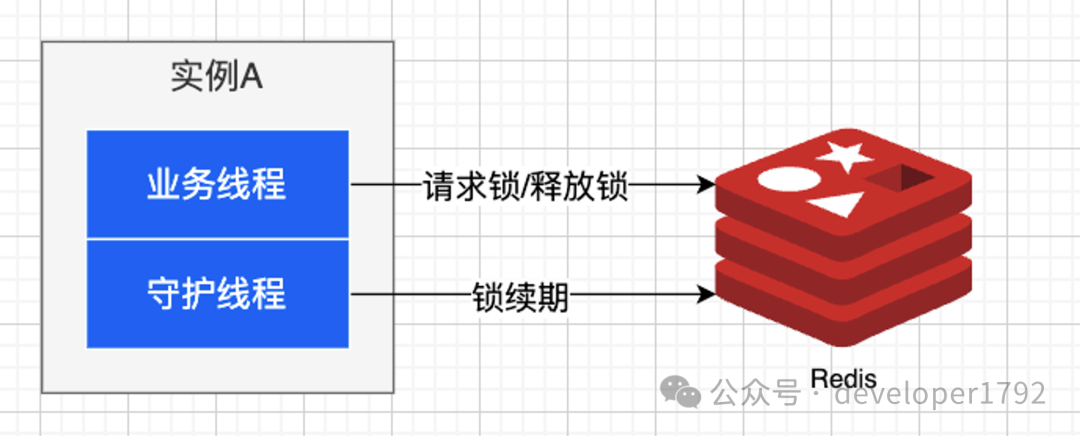
Redis缓存数据库进阶——Redis与分布式锁(6)
分布式锁简介 1. 什么是分布式锁 分布式锁是一种在分布式系统环境下,通过多个节点对共享资源进行访问控制的一种同步机制。它的主要目的是防止多个节点同时操作同一份数据,从而避免数据的不一致性。 线程锁: 也被称为互斥锁(Mu…...

网络芯片(又称为PHY网络芯片)
Realtek RTL8152B是一种常见的主板集成网络芯片(又称为PHY网络芯片)。PHY芯片是指将网络控制芯片的运算部分交由处理器或南桥芯片处理,以简化线路设计,从而降低成本。 https://www.realtek.com/Download/List?cate_id585 Realt…...
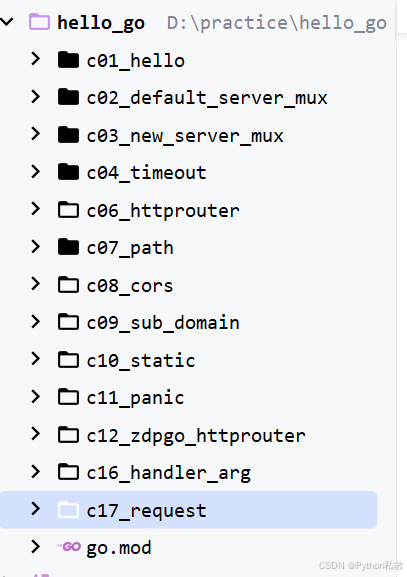
01 Go Web基础_20240728 课程笔记
概述 如果您没有Golang的基础,应该学习如下前置课程。 基础不好的同学每节课的代码最好配合视频进行阅读和学习,如果基础比较扎实,则阅读本教程巩固一下相关知识点即可,遇到不会的知识点再看视频。 视频课程 最近发现越来越多…...