Halcon深度学习分类模型
1.Halcon20之后深度学习支持CPU训练模型,没有money买显卡的小伙伴有福了。但是缺点也很明显,就是训练速度超级慢,推理效果也没有GPU好,不过学习用足够。
2.分类模型是Halcon深度学习最简单的模型,可以用在物品分类,缺陷检测等项目。
3.图像预处理和训练代码
*分类网络
dev_update_off ()
dev_close_window ()
WindowWidth := 800
WindowHeight := 600
dev_open_window_fit_size (0, 0, WindowWidth, WindowHeight, -1, -1, WindowHandle)
set_display_font (WindowHandle, 16, ‘mono’, ‘true’, ‘false’)
*训练原图路径
RawImageBaseFolder :=‘D:/训练图/’+ [‘U’,‘SR’,‘MR’,‘BR’,‘C’,‘D’,‘NG’]
*预处理数据存储路径
ExampleDataDir := ‘D:/classify_pill_defects_data’
-
Dataset directory basename for any outputs written by preprocess_dl_dataset.
DataDirectoryBaseName := ExampleDataDir + ‘/dldataset_pill’
- ** Set parameters ***
- LabelSource for reading in the dataset.
LabelSource := ‘last_folder’ - Percentages for splitting the dataset.
TrainingPercent := 70
ValidationPercent := 15 - Image dimensions the images are rescaled to during preprocessing.
ImageWidth := 300
ImageHeight := 300
ImageNumChannels := 3 - Further parameters for image preprocessing.
NormalizationType := ‘none’
DomainHandling := ‘full_domain’ - In order to get a reproducible split we set a random seed.
- This means that re-running the script results in the same split of DLDataset.
SeedRand := 42
- ** Read the labeled data and split it into train, validation and test ***
- Set the random seed.
set_system (‘seed_rand’, SeedRand) - Read the dataset with the procedure read_dl_dataset_classification.
- Alternatively, you can read a DLDataset dictionary
- as created by e.g., the MVTec Deep Learning Tool using read_dict().
read_dl_dataset_classification (RawImageBaseFolder, LabelSource, DLDataset) - Generate the split.
split_dl_dataset (DLDataset, TrainingPercent, ValidationPercent, [])
- ** Preprocess the dataset ***
- Create the output directory if it does not exist yet.
file_exists (ExampleDataDir, FileExists)
if (not FileExists)
make_dir (ExampleDataDir)
endif - Create preprocess parameters.
create_dl_preprocess_param (‘classification’, ImageWidth, ImageHeight, ImageNumChannels, -127, 128, NormalizationType, DomainHandling, [], [], [], [], DLPreprocessParam) - Dataset directory for any outputs written by preprocess_dl_dataset.
DataDirectory := DataDirectoryBaseName + ‘_’ + ImageWidth + ‘x’ + ImageHeight - Preprocess the dataset. This might take a few seconds.
create_dict (GenParam)
set_dict_tuple (GenParam, ‘overwrite_files’, true)
preprocess_dl_dataset (DLDataset, DataDirectory, DLPreprocessParam, GenParam, DLDatasetFileName) - Store preprocess params separately in order to use it e.g. during inference.
PreprocessParamFileBaseName := DataDirectory + ‘/dl_preprocess_param.hdict’
write_dict (DLPreprocessParam, PreprocessParamFileBaseName, [], [])
- ** Preview the preprocessed dataset ***
- Before moving on to training, it is recommended to check the preprocessed dataset.
- Display the DLSamples for 10 randomly selected train images.
get_dict_tuple (DLDataset, ‘samples’, DatasetSamples)
find_dl_samples (DatasetSamples, ‘split’, ‘train’, ‘match’, SampleIndices)
tuple_shuffle (SampleIndices, ShuffledIndices)
read_dl_samples (DLDataset, ShuffledIndices[0:9], DLSampleBatchDisplay)
create_dict (WindowHandleDict)
for Index := 0 to |DLSampleBatchDisplay| - 1 by 1
* Loop over samples in DLSampleBatchDisplay.
dev_display_dl_data (DLSampleBatchDisplay[Index], [], DLDataset, ‘classification_ground_truth’, [], WindowHandleDict)
Text := ‘Press Run (F5) to continue’
dev_disp_text (Text, ‘window’, ‘bottom’, ‘right’, ‘black’, [], [])
stop ()
endfor
*
- Close windows that have been used for visualization.
dev_close_window_dict (WindowHandleDict)
*检测电脑是否有GPU,如果无GPU则使用CPU训练
query_available_dl_devices ([‘runtime’,‘runtime’], [‘gpu’,‘cpu’], DLDeviceHandles)
if (|DLDeviceHandles| == 0)
throw (‘No supported device found to continue this example.’)
endif
- Due to the filter used in query_available_dl_devices, the first device is a GPU, if available.
DLDevice := DLDeviceHandles[0]
get_dl_device_param (DLDevice, ‘type’, DLDeviceType)
if (DLDeviceType == ‘cpu’)- The number of used threads may have an impact
- on the training duration.
NumThreadsTraining := 4
set_system (‘thread_num’, NumThreadsTraining)
endif
- ** Set input and output paths ***
-
File path of the initialized model.
ModelFileName := ‘pretrained_dl_classifier_compact.hdl’ -
File path of the preprocessed DLDataset.
-
Note: Adapt DataDirectory after preprocessing with another image size.
DataDirectory := ExampleDataDir + ‘/dldataset_pill_300x300’
DLDatasetFileName := DataDirectory + ‘/dl_dataset.hdict’
DLPreprocessParamFileName := DataDirectory + ‘/dl_preprocess_param.hdict’ -
Output path of the best evaluated model.
BestModelBaseName := ExampleDataDir + ‘/best_dl_model_classification’ -
Output path for the final trained model.
FinalModelBaseName := ExampleDataDir + ‘/final_dl_model_classification’
- ** Set basic parameters ***
- The following parameters need to be adapted frequently.
- Model parameters.
- Batch size. In case this example is run on a GPU,
- you can set BatchSize to ‘maximum’ and it will be
- determined automatically.
BatchSize := 64 - Initial learning rate.
InitialLearningRate := 0.001 - Momentum should be high if batch size is small.
Momentum := 0.9 - Parameters used by train_dl_model.
- Number of epochs to train the model.
NumEpochs := 16 - Evaluation interval (in epochs) to calculate evaluation measures on the validation split.
EvaluationIntervalEpochs := 1 - Change the learning rate in the following epochs, e.g. [4, 8, 12].
- Set it to [] if the learning rate should not be changed.
ChangeLearningRateEpochs := [4,8,12] - Change the learning rate to the following values, e.g. InitialLearningRate * [0.1, 0.01, 0.001].
- The tuple has to be of the same length as ChangeLearningRateEpochs.
ChangeLearningRateValues := InitialLearningRate * [0.1,0.01,0.001]
- ** Set advanced parameters ***
- The following parameters might need to be changed in rare cases.
- Model parameter.
- Set the weight prior.
WeightPrior := 0.0005 - Parameters used by train_dl_model.
- Control whether training progress is displayed (true/false).
EnableDisplay := true - Set a random seed for training.
RandomSeed := 42
set_system (‘seed_rand’, RandomSeed) - In order to obtain nearly deterministic training results on the same GPU
- (system, driver, cuda-version) you could specify “cudnn_deterministic” as
- “true”. Note, that this could slow down training a bit.
- set_system (‘cudnn_deterministic’, ‘true’)
- Set generic parameters of create_dl_train_param.
- Please see the documentation of create_dl_train_param for an overview on all available parameters.
GenParamName := []
GenParamValue := [] - Augmentation parameters.
- If samples should be augmented during training, create the dict required by augment_dl_samples.
- Here, we set the augmentation percentage and method.
create_dict (AugmentationParam) - Percentage of samples to be augmented.
set_dict_tuple (AugmentationParam, ‘augmentation_percentage’, 50) - Mirror images along row and column.
set_dict_tuple (AugmentationParam, ‘mirror’, ‘rc’)
GenParamName := [GenParamName,‘augment’]
GenParamValue := [GenParamValue,AugmentationParam] - Change strategies.
- It is possible to change model parameters during training.
- Here, we change the learning rate if specified above.
if (|ChangeLearningRateEpochs| > 0)
create_dict (ChangeStrategy)- Specify the model parameter to be changed, here the learning rate.
set_dict_tuple (ChangeStrategy, ‘model_param’, ‘learning_rate’) - Start the parameter value at ‘initial_value’.
set_dict_tuple (ChangeStrategy, ‘initial_value’, InitialLearningRate) - Reduce the learning rate in the following epochs.
set_dict_tuple (ChangeStrategy, ‘epochs’, ChangeLearningRateEpochs) - Reduce the learning rate to the following values.
set_dict_tuple (ChangeStrategy, ‘values’, ChangeLearningRateValues) - Collect all change strategies as input.
GenParamName := [GenParamName,‘change’]
GenParamValue := [GenParamValue,ChangeStrategy]
endif
- Specify the model parameter to be changed, here the learning rate.
- Serialization strategies.
- There are several options for saving intermediate models to disk (see create_dl_train_param).
- Here, we save the best and the final model to the paths set above.
create_dict (SerializationStrategy)
set_dict_tuple (SerializationStrategy, ‘type’, ‘best’)
set_dict_tuple (SerializationStrategy, ‘basename’, BestModelBaseName)
GenParamName := [GenParamName,‘serialize’]
GenParamValue := [GenParamValue,SerializationStrategy]
create_dict (SerializationStrategy)
set_dict_tuple (SerializationStrategy, ‘type’, ‘final’)
set_dict_tuple (SerializationStrategy, ‘basename’, FinalModelBaseName)
GenParamName := [GenParamName,‘serialize’]
GenParamValue := [GenParamValue,SerializationStrategy] - Display parameters.
- In this example, 20% of the training split are selected to display the
- evaluation measure for the reduced training split during the training. A lower percentage
- helps to speed up the evaluation/training. If the evaluation measure for the training split
- shall not be displayed, set this value to 0 (default).
SelectedPercentageTrainSamples := 20 - Set the x-axis argument of the training plots.
XAxisLabel := ‘epochs’
create_dict (DisplayParam)
set_dict_tuple (DisplayParam, ‘selected_percentage_train_samples’, SelectedPercentageTrainSamples)
set_dict_tuple (DisplayParam, ‘x_axis_label’, XAxisLabel)
GenParamName := [GenParamName,‘display’]
GenParamValue := [GenParamValue,DisplayParam]
- ** Read initial model and dataset ***
- Check if all necessary files exist.
check_data_availability (ExampleDataDir, DLDatasetFileName, DLPreprocessParamFileName) - Read in the model that was initialized during preprocessing.
read_dl_model (ModelFileName, DLModelHandle) - Read in the preprocessed DLDataset file.
read_dict (DLDatasetFileName, [], [], DLDataset)
- ** Set model parameters ***
- Set model hyper-parameters as specified in the settings above.
set_dl_model_param (DLModelHandle, ‘learning_rate’, InitialLearningRate)
set_dl_model_param (DLModelHandle, ‘momentum’, Momentum) - Set the class names for the model.
get_dict_tuple (DLDataset, ‘class_names’, ClassNames)
set_dl_model_param (DLModelHandle, ‘class_names’, ClassNames) - Get image dimensions from preprocess parameters and set them for the model.
read_dict (DLPreprocessParamFileName, [], [], DLPreprocessParam)
get_dict_tuple (DLPreprocessParam, ‘image_width’, ImageWidth)
get_dict_tuple (DLPreprocessParam, ‘image_height’, ImageHeight)
get_dict_tuple (DLPreprocessParam, ‘image_num_channels’, ImageNumChannels)
set_dl_model_param (DLModelHandle, ‘image_dimensions’, [ImageWidth,ImageHeight,ImageNumChannels])
if (BatchSize == ‘maximum’ and DLDeviceType == ‘gpu’)
set_dl_model_param_max_gpu_batch_size (DLModelHandle, 100)
else
set_dl_model_param (DLModelHandle, ‘batch_size’, BatchSize)
endif - When the batch size is determined, set the device.
set_dl_model_param (DLModelHandle, ‘device’, DLDevice)
if (|WeightPrior| > 0)
set_dl_model_param (DLModelHandle, ‘weight_prior’, WeightPrior)
endif - Set class weights to counteract unbalanced training data. In this example
- we choose the default values, since the classes are evenly distributed in the dataset.
tuple_gen_const (|ClassNames|, 1.0, ClassWeights)
set_dl_model_param (DLModelHandle, ‘class_weights’, ClassWeights)
- ** Train the model ***
- Create training parameters.
create_dl_train_param (DLModelHandle, NumEpochs, EvaluationIntervalEpochs, EnableDisplay, RandomSeed, GenParamName, GenParamValue, TrainParam) - Start the training by calling the training operator
- train_dl_model_batch () within the following procedure.
train_dl_model (DLDataset, DLModelHandle, TrainParam, 0, TrainResults, TrainInfos, EvaluationInfos) - Stop after the training has finished, before closing the windows.
dev_disp_text (‘Press Run (F5) to continue’, ‘window’, ‘bottom’, ‘right’, ‘black’, [], [])
stop () - Close training windows.
dev_close_window ()
4.推理代码
dev_update_off ()
dev_close_window ()
WindowWidth := 800
WindowHeight := 600
dev_open_window_fit_size (0, 0, WindowWidth, WindowHeight, -1, -1, WindowHandle)
set_display_font (WindowHandle, 16, ‘mono’, ‘true’, ‘false’) - ** INFERENCE **
*检测电脑是否有GPU,如果无GPU则使用CPU推理
query_available_dl_devices ([‘runtime’,‘runtime’], [‘gpu’,‘cpu’], DLDeviceHandles)
if (|DLDeviceHandles| == 0)
throw (‘No supported device found to continue this example.’)
endif
- Due to the filter used in query_available_dl_devices, the first device is a GPU, if available.
DLDevice := DLDeviceHandles[0]
*总路径
ExampleDataDir := ‘D:/classify_pill_defects_data’
-
Dataset directory basename for any outputs written by preprocess_dl_dataset.
DataDirectoryBaseName := ExampleDataDir + ‘/dldataset_pill’ -
File name of the dict containing parameters used for preprocessing.
-
Note: Adapt DataDirectory after preprocessing with another image size.
DataDirectory := ExampleDataDir + ‘/dldataset_pill_300x300’
PreprocessParamFileName := DataDirectory + ‘/dl_preprocess_param.hdict’ -
File name of the finetuned object detection model.
RetrainedModelFileName := ExampleDataDir + ‘/best_dl_model_classification.hdl’ -
Batch Size used during inference.
BatchSizeInference := 1
- ** Inference ***
- Check if all necessary files exist.
check_data_availability (ExampleDataDir, PreprocessParamFileName, RetrainedModelFileName, false) - Read in the retrained model.
read_dl_model (RetrainedModelFileName, DLModelHandle) - Set the batch size.
set_dl_model_param (DLModelHandle, ‘batch_size’, BatchSizeInference) - Initialize the model for inference.
set_dl_model_param (DLModelHandle, ‘device’, DLDevice) - Get the class names and IDs from the model.
get_dl_model_param (DLModelHandle, ‘class_names’, ClassNames)
get_dl_model_param (DLModelHandle, ‘class_ids’, ClassIDs) - Get the parameters used for preprocessing.
read_dict (PreprocessParamFileName, [], [], DLPreprocessParam) - Create window dictionary for displaying results.
create_dict (WindowHandleDict) - Create dictionary with dataset parameters necessary for displaying.
create_dict (DLDataInfo)
set_dict_tuple (DLDataInfo, ‘class_names’, ClassNames)
set_dict_tuple (DLDataInfo, ‘class_ids’, ClassIDs) - Set generic parameters for visualization.
create_dict (GenParam)
set_dict_tuple (GenParam, ‘scale_windows’, 1.1)
list_files (‘E:/NG’, [‘files’,‘follow_links’], ImageFiles)
tuple_regexp_select (ImageFiles, [‘\.(tif|tiff|gif|bmp|jpg|jpeg|jp2|png|pcx|pgm|ppm|pbm|xwd|ima|hobj)$’,‘ignore_case’], ImageFiles)
for Index := 0 to |ImageFiles| - 1 by 1
read_image (ImageBatch, ImageFiles[Index])
gen_dl_samples_from_images (ImageBatch, DLSampleBatch)
preprocess_dl_samples (DLSampleBatch, DLPreprocessParam)
apply_dl_model (DLModelHandle, DLSampleBatch, [], DLResultBatch)
DLSample := DLSampleBatch[0]
DLResult := DLResultBatch[0]
*获取识别结果 参数:分类的结果,批处理中图像的索引,通用参数的名称,通用参数的值
get_dict_tuple (DLResult, ‘classification_class_ids’, ClassificationClassID)
get_dict_tuple (DLResult, ‘classification_class_names’, ClassificationClassName)
get_dict_tuple (DLResult, ‘classification_confidences’, ClassificationClassConfidence)
dev_display (ImageBatch)
Text := ‘预测类为: ’ + ClassificationClassName[0] + ’ 置信度:’+ClassificationClassConfidence[0]
dev_disp_text (Text, ‘window’, ‘top’, ‘left’, ‘red’, ‘box’, ‘false’)
stop ()
endfor
dev_close_window_dict (WindowHandleDict)
相关文章:
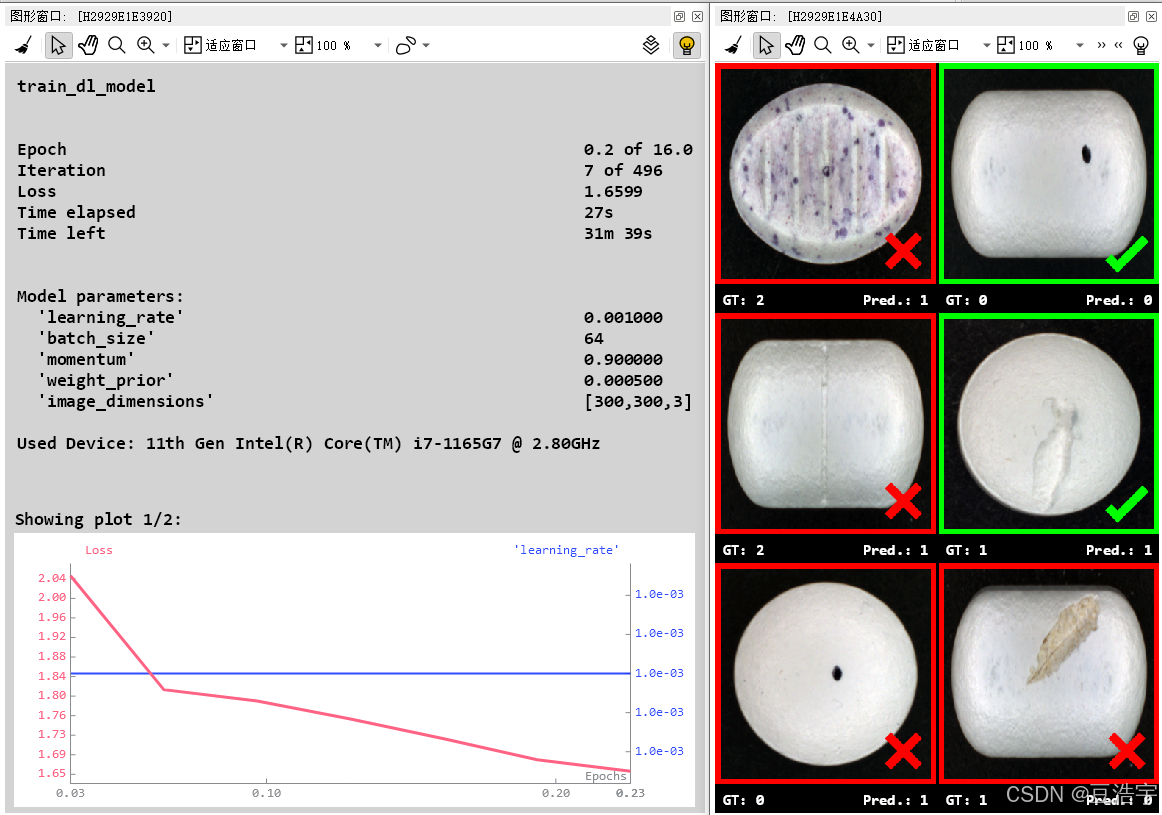
Halcon深度学习分类模型
1.Halcon20之后深度学习支持CPU训练模型,没有money买显卡的小伙伴有福了。但是缺点也很明显,就是训练速度超级慢,推理效果也没有GPU好,不过学习用足够。 2.分类模型是Halcon深度学习最简单的模型,可以用在物品分类&…...
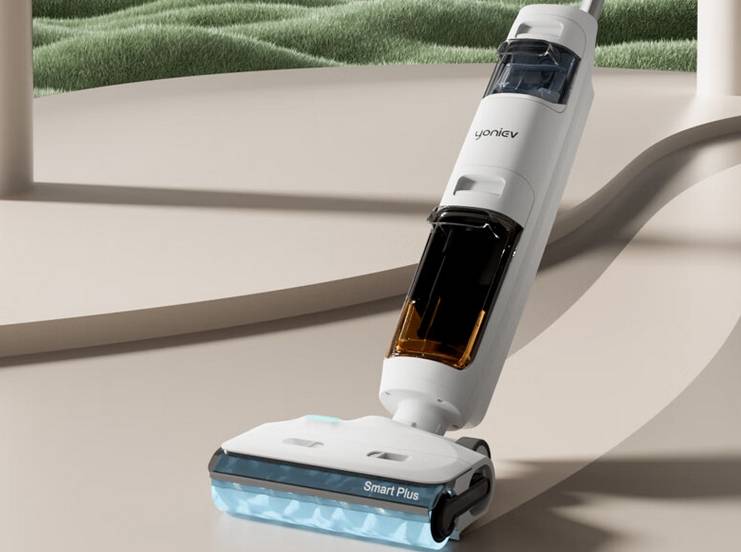
洗地机哪种牌子好?洗地机排行榜前十名公布
洗地机市场上品牌琳琅满目,每个品牌都有其独特的魅力和优势。消费者在选择时,往往会根据自己的实际需求、预算以及对产品性能的期望来做出决策。因此,无论是哪个品牌的洗地机,只要能够满足用户的清洁需求,提供便捷的操…...

C++中的虚函数与多态机制如何工作?
在C中,虚函数和多态机制是实现面向对象编程的重要概念。 虚函数是在基类中声明的函数,可以在派生类中进行重写。当基类的指针或引用指向派生类的对象时,通过调用虚函数可以实现动态绑定,即在运行时确定要调用的函数。 多态是指通…...
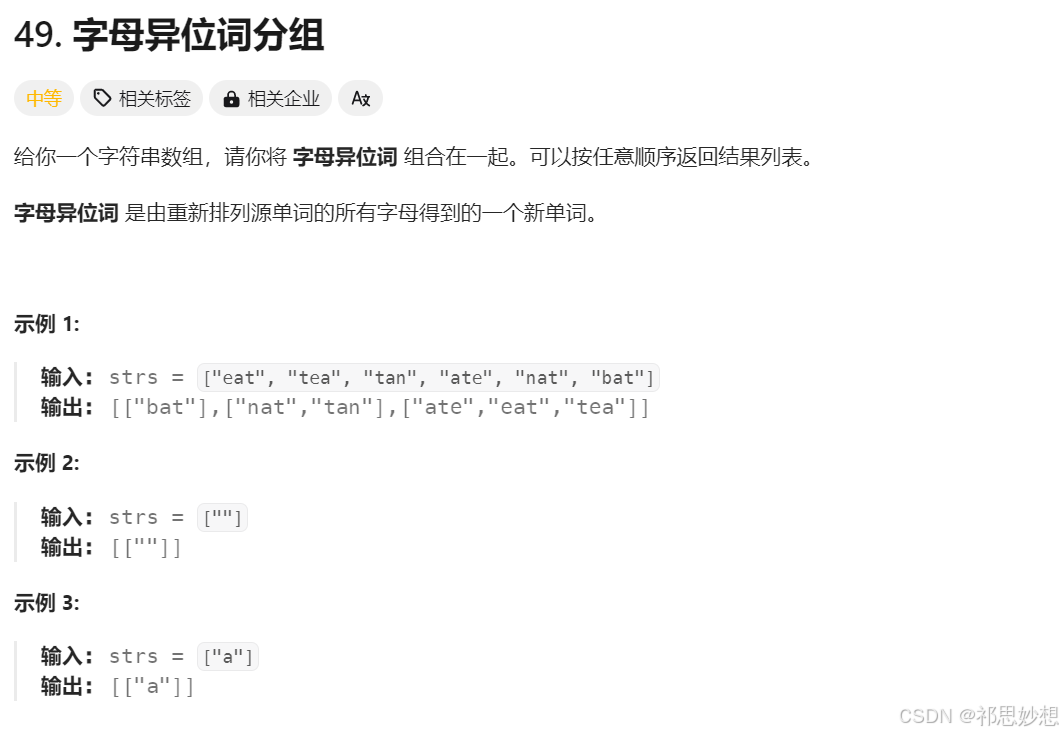
《LeetCode热题100》---<哈希三道>
本篇博客讲解 LeetCode热题100道中的哈希篇中的三道题。分别是 1.第一道:两数之和(简单) 2.第二道:字母异位词分组(中等) 3.第三道:最长连续序列(中等) 第一道࿱…...
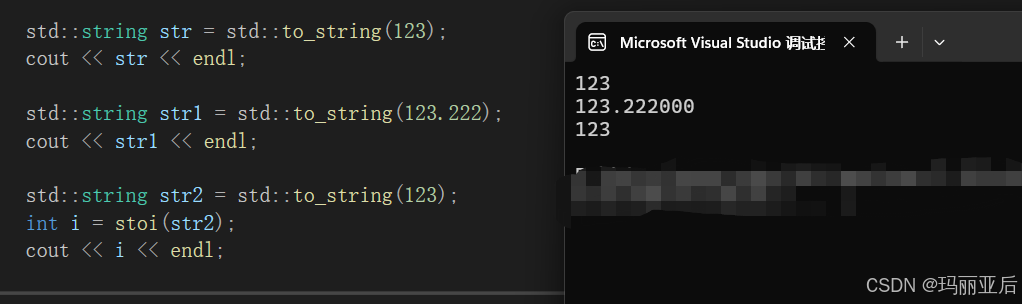
秒懂C++之string类(下)
目录 一.接口说明 1.1 erase 1.2 replace(最好别用) 1.3 find 1.4 substr 1.5 rfind 1.6 find_first_of 1.7 find_last_of 二.string类的模拟实现 2.1 构造 2.2 无参构造 2.3 析构 2.4.【】运算符 2.5 迭代器 2.6 打印 2.7 reserve扩容 …...
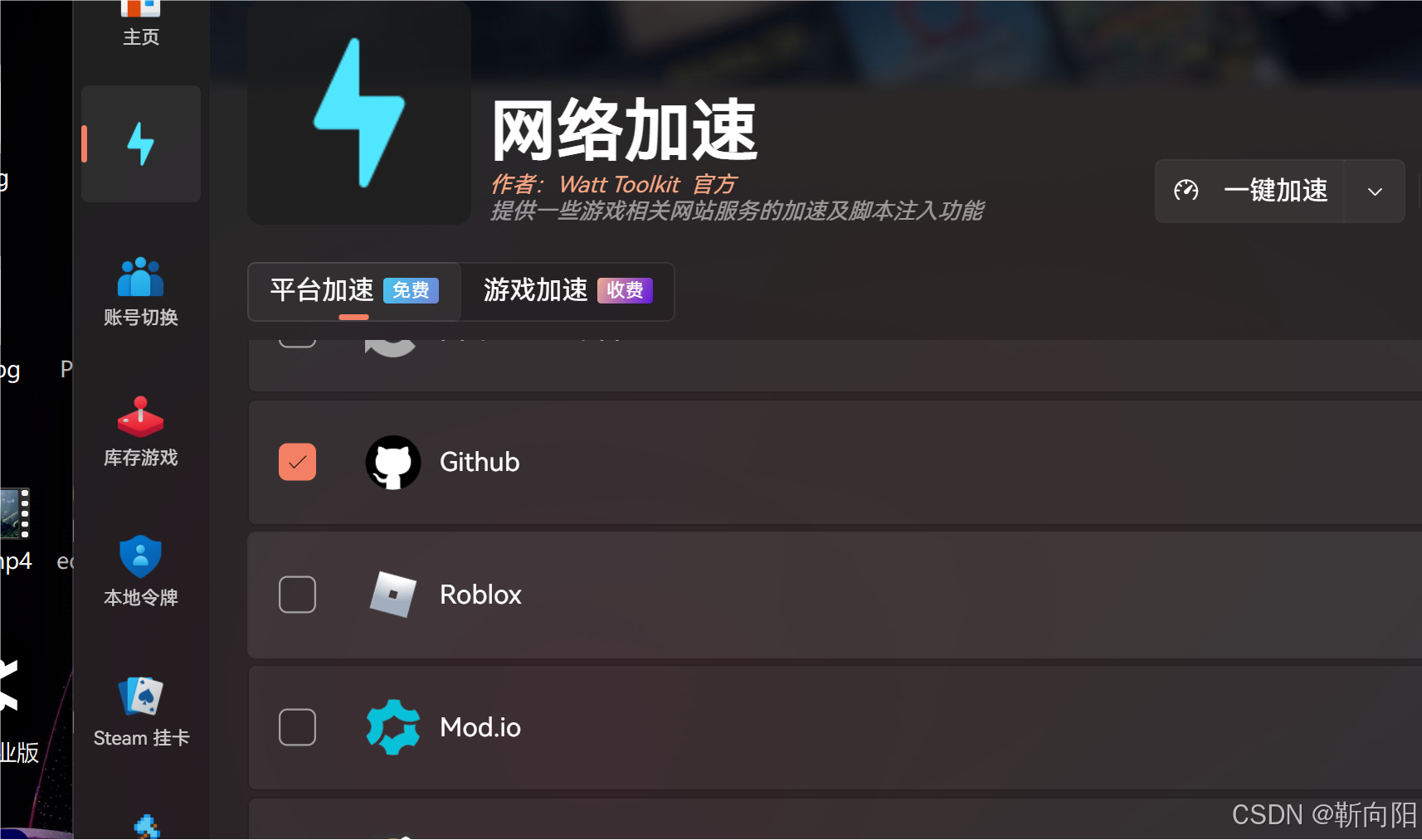
github简单地操作
1.调节字体大小 选择options 选择text 选择select 选择你需要的参数就可以了。 2.配置用户名和邮箱 桌面右键,选择git Bash Here git config --global user.name 用户名 git config --global user.email 邮箱名 3.用git实现代码管理的过程 下载别人的项目 git …...
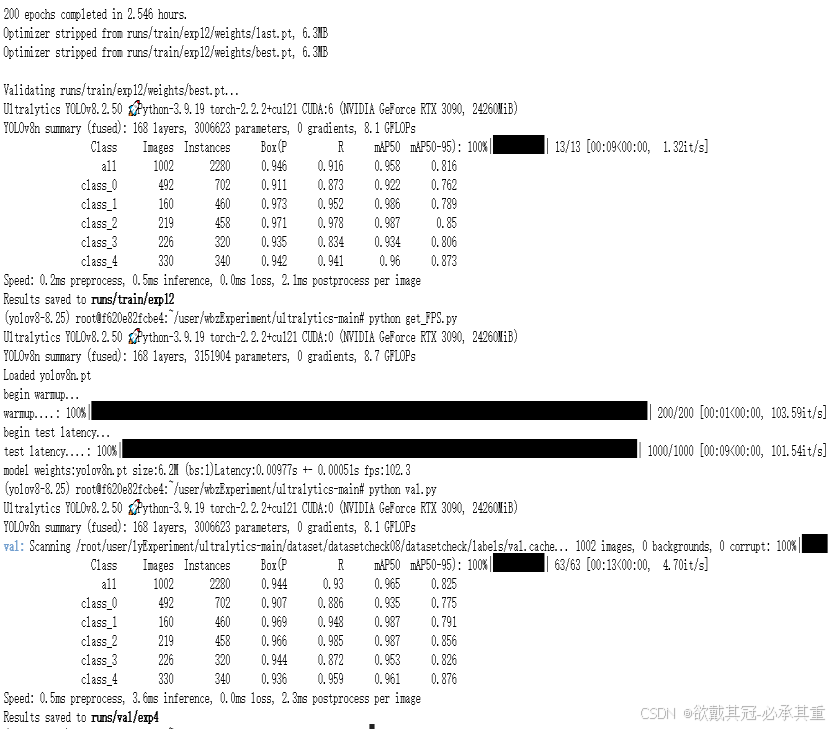
模型改进-损失函数合集
模版 第一步在哪些地方做出修改: 228行 self.use_wiseiouTrue 230行 self.wiou_loss WiseIouLoss(ltypeMPDIoU, monotonousFalse, inner_iouTrue, focaler_iouFalse) 238行 wiou self.wiou_loss(pred_bboxes[fg_mask], target_bboxes[fg_mask], ret_iouFalse…...
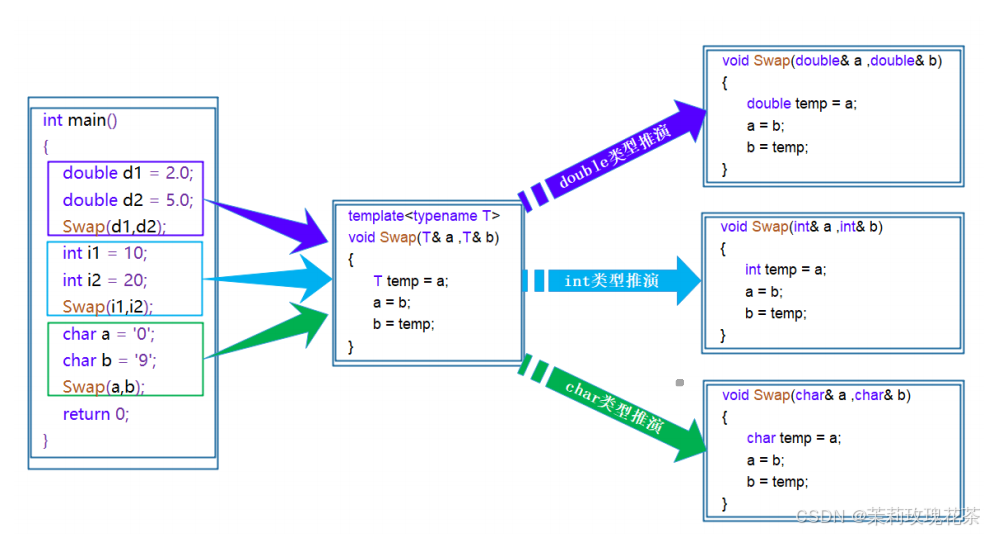
C++模板(初阶)
1.引入 在之前的笔记中有提到:函数重载(特别是交换函数(Swap)的实现) void Swap(int& left, int& right) {int temp left;left right;right temp; } void Swap(double& left, double& right) {do…...

下面关于Date类的描述错误的一项是?
下面关于Date类的描述错误的一项是? A. java.util.Date类下有三个子类:java.sql.Date、java.sql.Timestamp、java.sql.Time; B. 利用SimpleDateFormat类可以对java.util.Date类进行格式化显示; C. 直接输出Date类对象就可以取得日…...

【Python面试题收录】Python编程基础练习题①(数据类型+函数+文件操作)
本文所有代码打包在Gitee仓库中https://gitee.com/wx114/Python-Interview-Questions 一、数据类型 第一题(str) 请编写一个Python程序,完成以下任务: 去除字符串开头和结尾的空格。使用逗号(","&#…...
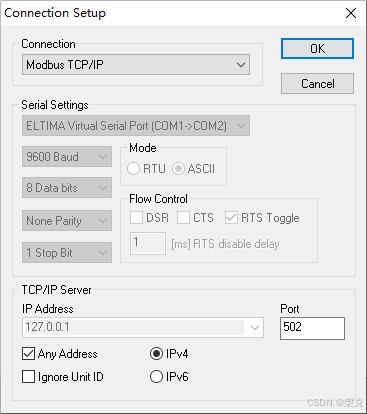
C# Nmodbus,EasyModbusTCP读写操作
Nmodbus读写 两个Button控件分别为 读取和写入 分别使用控件的点击方法 ①引用第三方《NModbus4》2.1.0版本 全局 public SerialPort port new SerialPort("COM2", 9600, Parity.None, 8, (StopBits)1); ModbusSerialMaster master; public Form1() port.Open();…...

spark常用参数调优
目录 1.set spark.grouping.sets.reference.hivetrue;2.set spark.locality.wait.rack0s3.set spark.locality.wait0s;4.set spark.executor.memoryOverhead 2G;5.set spark.sql.shuffle.partitions 1000;6.set spark.shuffle.file.buffer 256k7. set spark.reducer.maxSizeInF…...
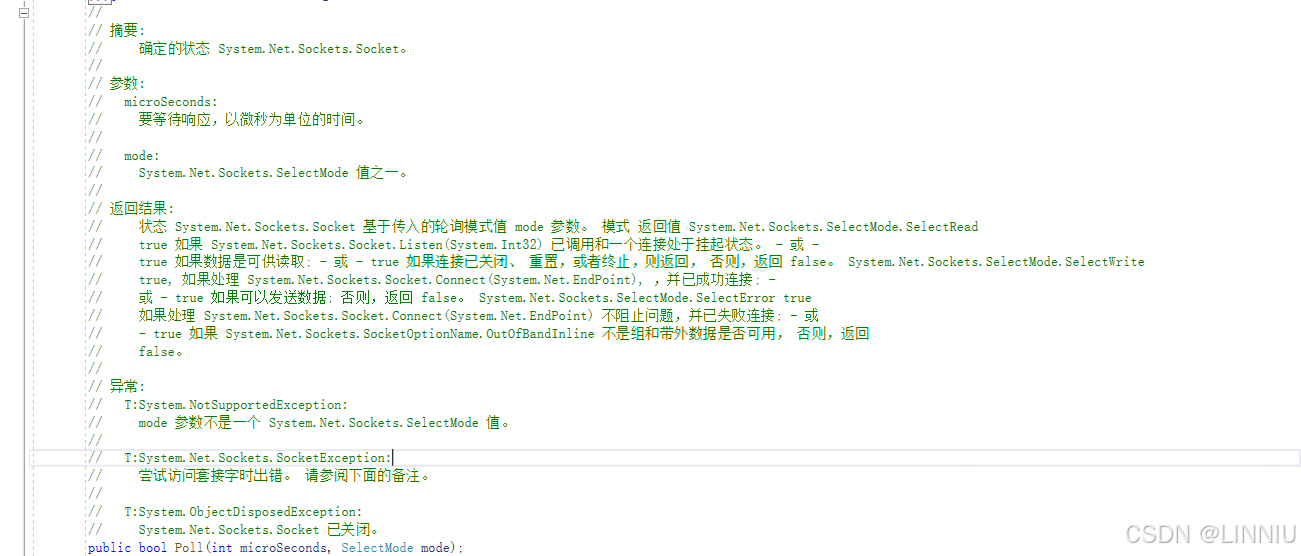
C#/WinFrom TCP通信+ 网线插拔检测+客服端异常掉线检测
Winfor Tcp通信(服务端) 今天给大家讲一下C# 关于Tcp 通信部分,这一块的教程网上一大堆,不过关于掉网,异常断开连接的这部分到是到是没有多少说明,有方法 不过基本上最多的两种方式(1.设置一个超时时间,2.…...
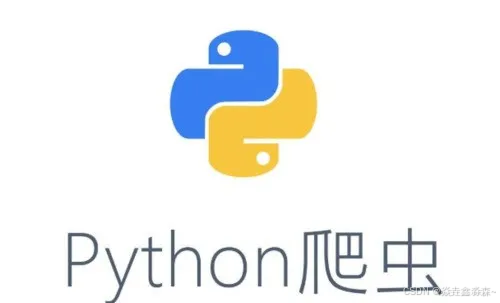
一篇文章掌握Python爬虫的80%
转载:一篇文章掌握Python爬虫的80% Python爬虫 Python 爬虫技术在数据采集和信息获取中有着广泛的应用。本文将带你掌握Python爬虫的核心知识,帮助你迅速成为一名爬虫高手。以下内容将涵盖爬虫的基本概念、常用库、核心技术和实战案例。 一、Python 爬虫…...

【用户会话信息在异步事件/线程池的传递】
用户会话信息在异步事件/线程池的传递 author:shengfq date:2024-07-29 version:1.0 背景: 同事写的一个代码功能,是在一个主线程中通过如下代码进行异步任务的执行,结果遇到了问题. 1.ThreadPool.execute(Runnable)启动一个子线程执行异步任务 2.applicationContext.publis…...

Java8: BigDecimal
Java8:BigDecimal 转两位小数的百分数-CSDN博客 BigDecimal 先做除法 然后取绝对值 在Java 8中,如果你想要对一个BigDecimal值进行除法操作,并随后取其绝对值,你可以通过组合divide方法和abs方法来实现这一目的。不过,需要注意的…...
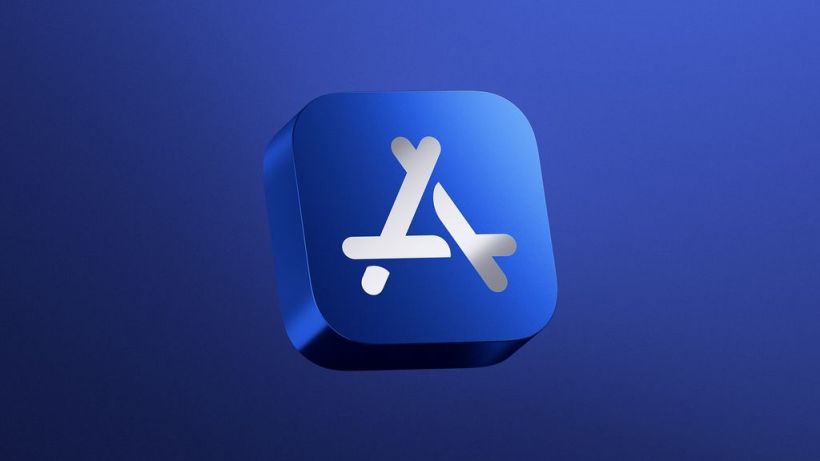
苹果推送iOS 18.1带来Apple Intelligence预览
🦉 AI新闻 🚀 苹果推送iOS 18.1带来Apple Intelligence预览 摘要:苹果向iPhone和iPad用户推送iOS 18.1和iPadOS 18.1开发者预览版Beta更新,带来“Apple Intelligence”预览。目前仅支持M1芯片或更高版本的设备。Apple Intellige…...
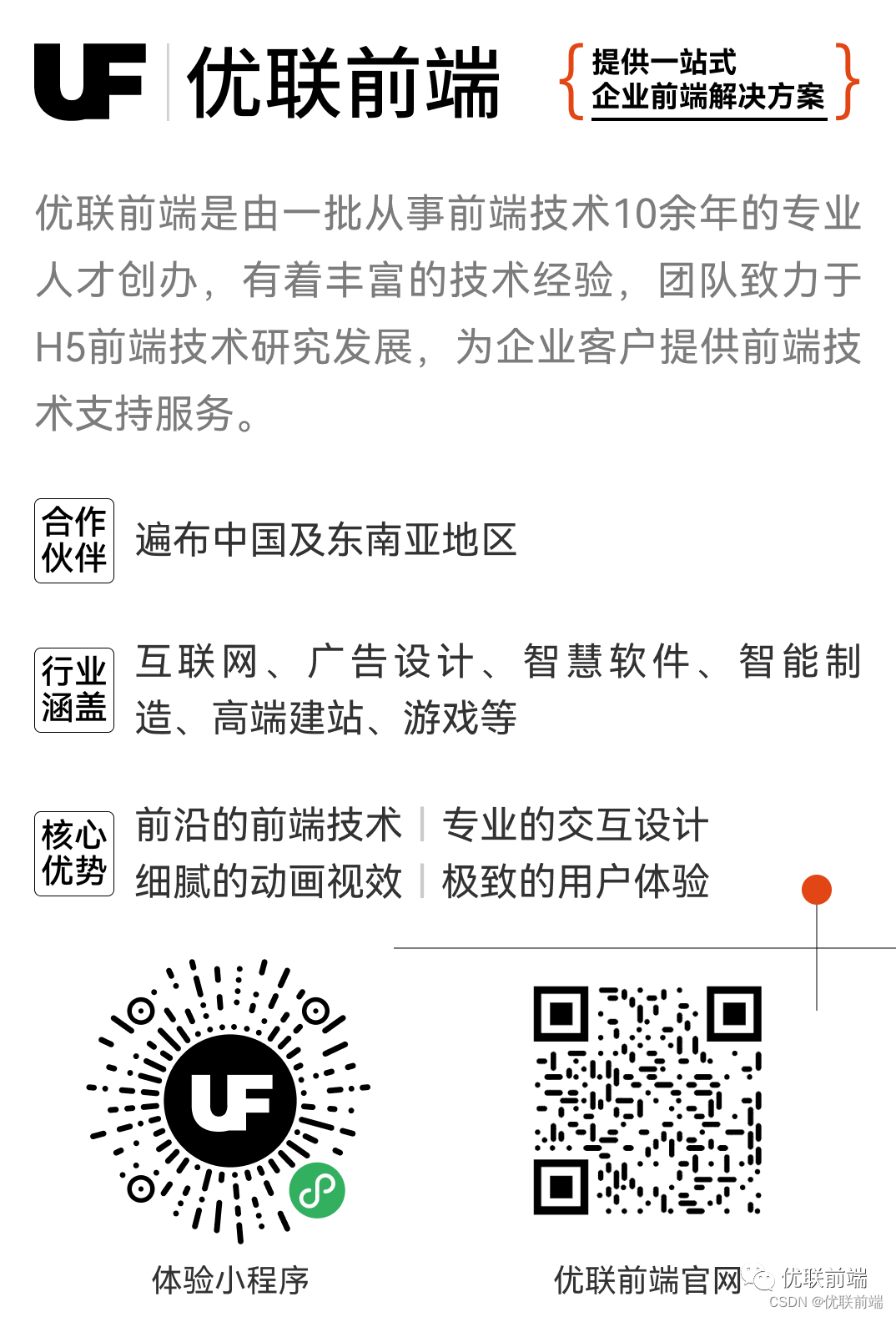
testRigor-基于人工智能驱动的无代码自动化测试平台
1、testRigor介绍 简单来说,testRigor是一款基于人工智能驱动的无代码自动化测试平台,它能够通过分析应用的行为模式,智能地生成测试用例,并自动执行这些测试,无需人工编写测试脚本。可以用于Web、移动、API和本机桌面…...
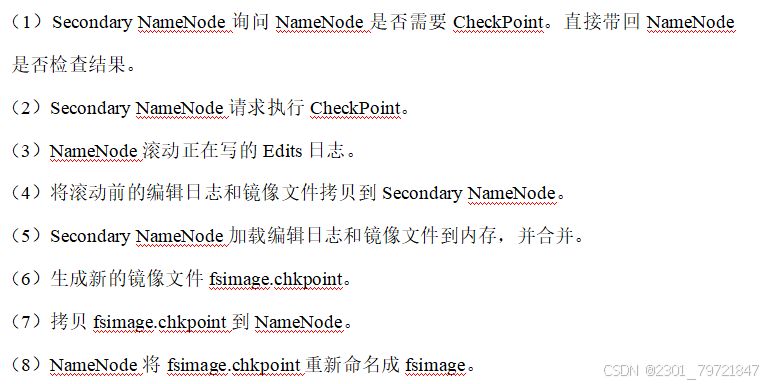
hadoop学习(一)
一.hadoop概述 1.1hadoop优势 1)高可靠性:Hadoop底层维护多个数据副本,即使Hadoop某个计算元素或存储出现故障,也不会导致数据的丢失。 2)高扩展性:在集群间分配任务数据,可方便扩展数以千计…...
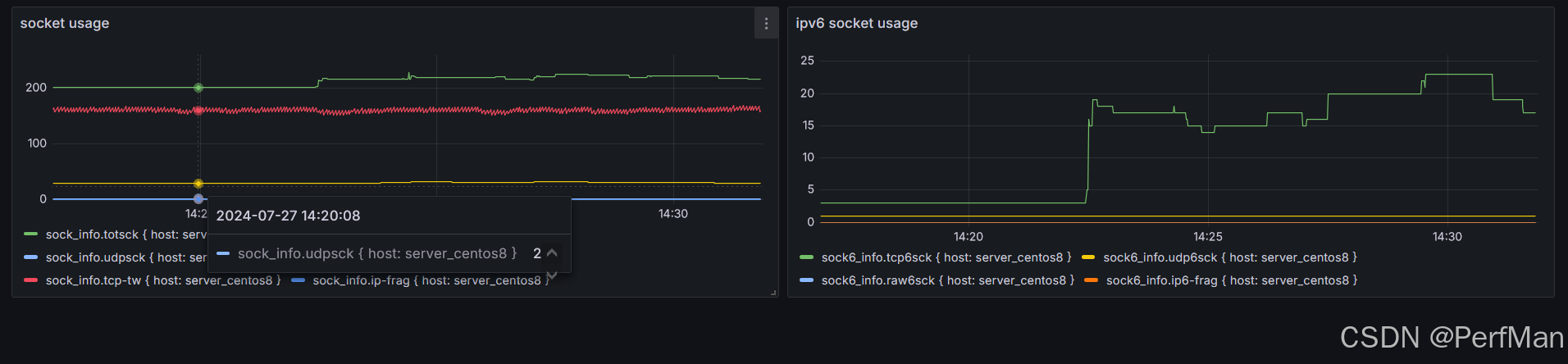
Linux性能监控:sar的可视化方案
在当今的IT环境中,系统性能监控是确保应用程序稳定运行和快速响应问题的关键。Linux作为一种广泛使用的操作系统,拥有多种性能监控工具,其中sar(System Activity Reporter)因其全面性和灵活性被广泛采用。然而…...

如何录制电脑屏幕视频,5招让您成为电脑录制高手
在今天,屏幕录制成为每个电脑使用者都应掌握的基础技能。不论是教学分享、会议记录还是游戏直播,屏幕录制都能帮你捕捉那些重要的瞬间,将无形的信息转化为有形的视频。那么,如何录制电脑屏幕视频呢?今天,我…...
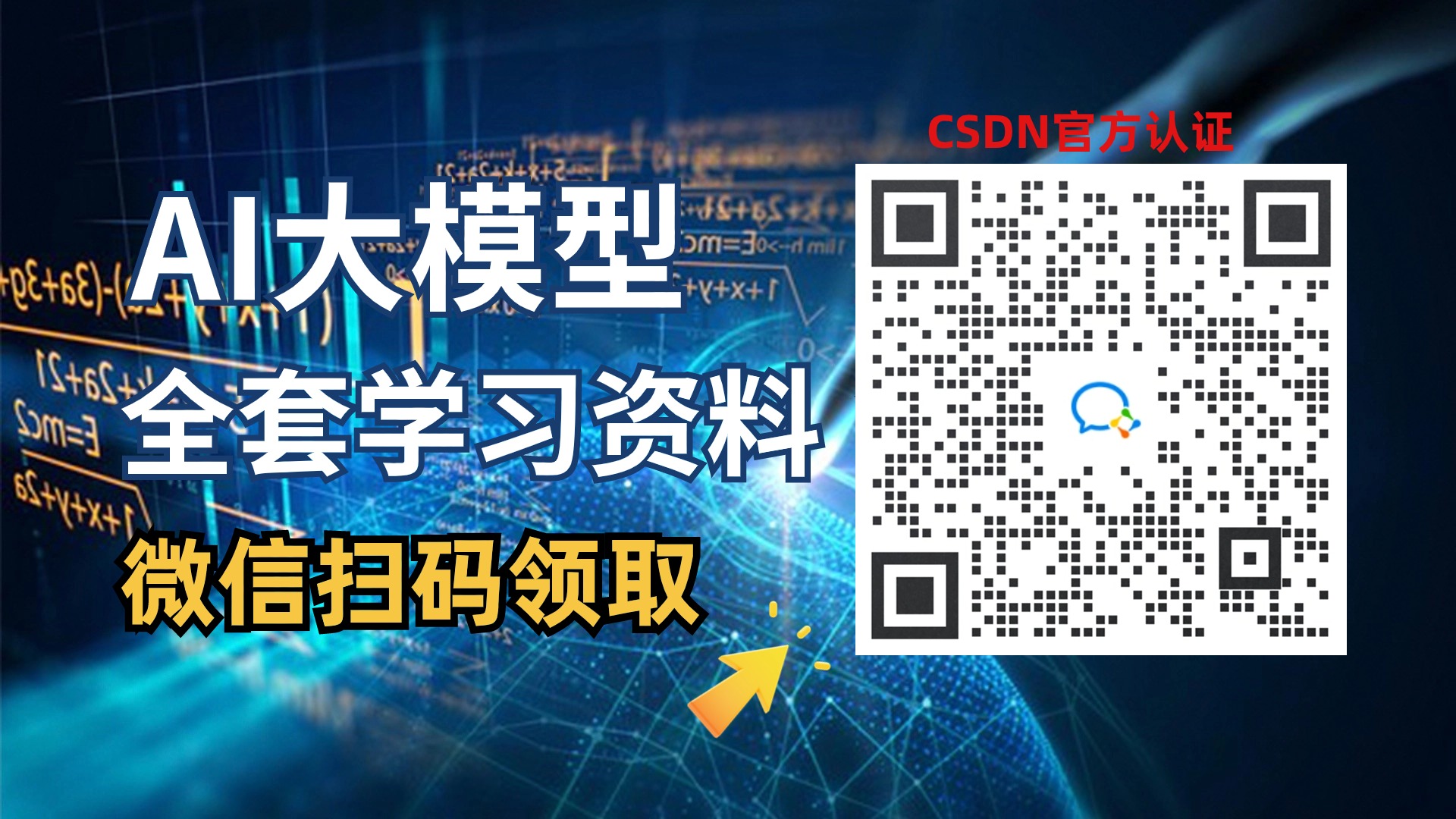
AI届的新宠:小语言模型(SLM)?
大语言模型(LLM)在过去几年产生了巨大影响,特别是随着OpenAI的ChatGPT的出现,各种大语言模型如雨后春笋般出现,国内如KimiChat、通义千问、文心一言和智谱清言等。 然而,大语言模型通常拥有庞大的参数&…...

PMP模拟题错题本
模拟题A 错题整理 项目经理为一个具有按时完成盈利项目历史记录的组织工作。然而,由于缺乏相关方的支持以及他们未能提供信息,这些项目都经历过问题。若要避免这些问题,项目经理在新项目开始时应该做什么? A. 在启动阶段识别关键…...

Laravel Dusk:点亮自动化测试的明灯
Laravel Dusk:点亮自动化测试的明灯 在Web开发中,确保应用程序的用户体验和功能正确性至关重要。Laravel Dusk是一个强大的浏览器自动化测试工具,它允许开发者模拟用户与应用程序的交互,从而进行端到端的测试。本文将深入探讨Lar…...
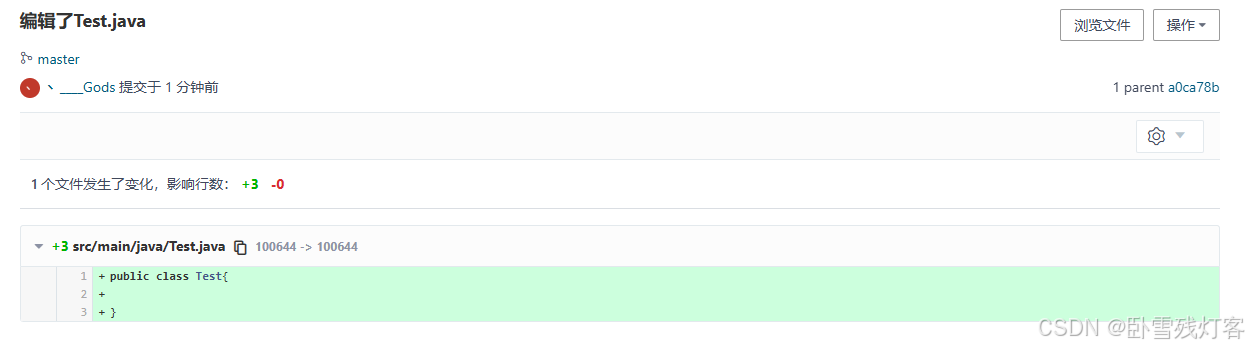
Git、Gitlab以及分支管理
分布式版本控制系统 一、Git概述 Git是一种分布式版本控制系统,用于跟踪和管理代码的变更。它由Linus torvalds创建的,最初被设计用于Linux内核的开发。Git 允许开发人员跟踪和管理代码的版本,并且可以在不同的开发人员之间进行协作。 Githu…...

TCP/IP 协议栈介绍
TCP/IP 协议栈介绍 1. 引言 TCP/IP(传输控制协议/互联网协议)是一组用于数据网络中通信的协议集合,它是互联网的基础。本文将详细介绍TCP/IP协议栈的各个层次、工作原理以及其在网络通信中的作用。 2. TCP/IP 协议栈的层次结构 TCP/IP协议…...

香橙派orangepi系统没有apt,也没有apt-get,也没有yum命令,找不到apt、apt-get、yum的Linux系统
以下是一个关于如何在 Orange Pi 上的 Arch Linux 系统中发现缺失包管理器的问题并解决的详细教程。 发现问题 确认系统类型: 使用以下命令检查当前的 Linux 发行版: uname -a cat /etc/os-release如果你看到类似于 “Arch Linux” 的信息,说…...
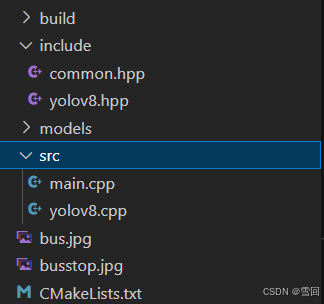
在invidia jetpack4.5.1上运行c++版yolov8(tensorRT)
心路历程(可略过) 为了能在arm64上跑通yolov8,我试过很多很多代码,太多对库版本的要求太高了; 比如说有一个是需要依赖onnx库的,(https://github.com/UNeedCryDear/yolov8-opencv-onnxruntime-…...

Vue3 接入 i18n 实现国际化多语言
在 Vue.js 3 中实现网页的国际化多语言,最常用的包是 vue-i18n。 第一步,安装一个 Vite 下使用 <i18n> 标签的插件:unplugin-vue-i18n npm install unplugin-vue-i18n # 或 yarn add unplugin-vue-i18n 安装完成后,调整 v…...

深度学习环境坑。
前面装好了之后装pytorch之后老显示gpufalse。 https://www.jb51.net/article/247762.htm 原因就是清华源的坑。 安装的时候不要用conda, 用pip命令 我cuda12.6,4070s cudnn-windows-x86_64-8.9.7.29_cuda12-archive.zip cuda_12.5.1_555.85_windows.…...
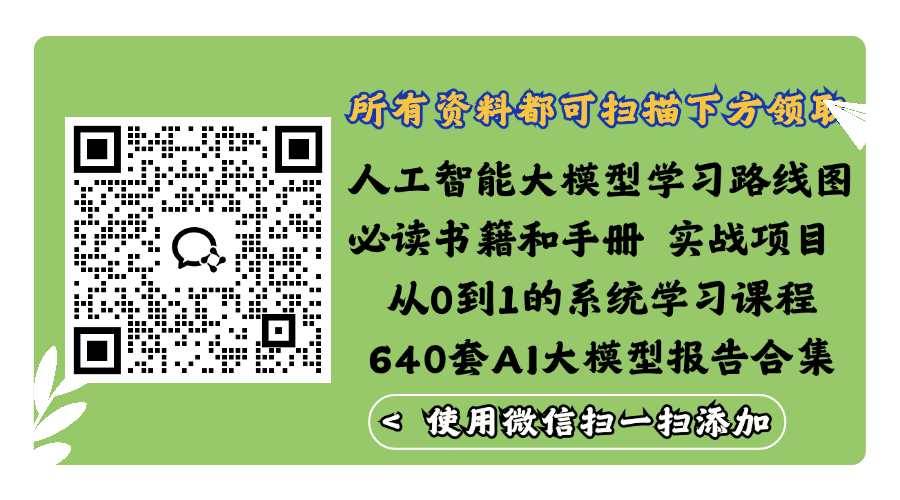
LLM——10个大型语言模型(LLM)常见面试题以及答案解析
今天我们来总结以下大型语言模型面试中常问的问题 1、哪种技术有助于减轻基于提示的学习中的偏见? A.微调 Fine-tuning B.数据增强 Data augmentation C.提示校准 Prompt calibration D.梯度裁剪 Gradient clipping 答案:C 提示校准包括调整提示,尽量减少产生…...

MongoDB - 聚合阶段 $count、$skip、$project
文章目录 1. $count 聚合阶段2. $skip 聚合阶段3. $project 聚合阶段1. 包含指定字段2. 排除_id字段3. 排除指定字段4. 不能同时指定包含字段和排除字段5. 排除嵌入式文档中的指定字段6. 包含嵌入式文档中的指定字段7. 添加新字段8. 重命名字段 1. $count 聚合阶段 计算匹配到…...

如何获取文件缩略图(C#和C++实现)
在C中,可以有以下两种办法 使用COM接口IThumbnailCache 文档链接:IThumbnailCache (thumbcache.h) - Win32 apps | Microsoft Learn 示例代码如下: VOID GetFileThumbnail(PCWSTR path) {HRESULT hr CoInitialize(nullptr);IShellItem* i…...

create-vue项目的README中文版
使用方法 要使用 create-vue 创建一个新的 Vue 项目,只需在终端中运行以下命令: npm create vuelatest[!注意] (latest 或 legacy) 不能省略,否则 npm 可能会解析到缓存中过时版本的包。 或者,如果你需要支持 IE11,你…...
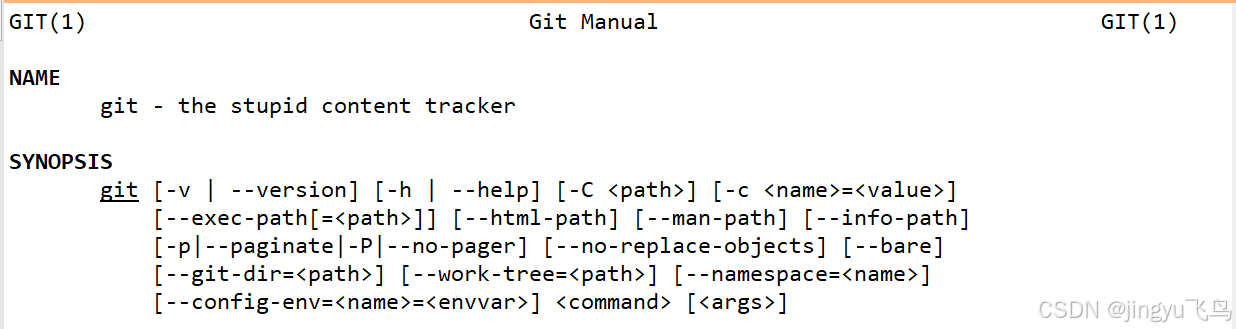
Centos 7系统(最小化安装)安装Git 、git-man帮助、补全git命令-详细文章
安装之前由于是最小化安装centos7安装一些开发环境和工具包 文章使用国内阿里源 cd /etc/yum.repos.d/ && mkdir myrepo && mv * myrepo&&lscurl -O https://mirrors.aliyun.com/repo/epel-7.repo;curl -O https://mirrors.aliyun.com/repo/Centos-7…...
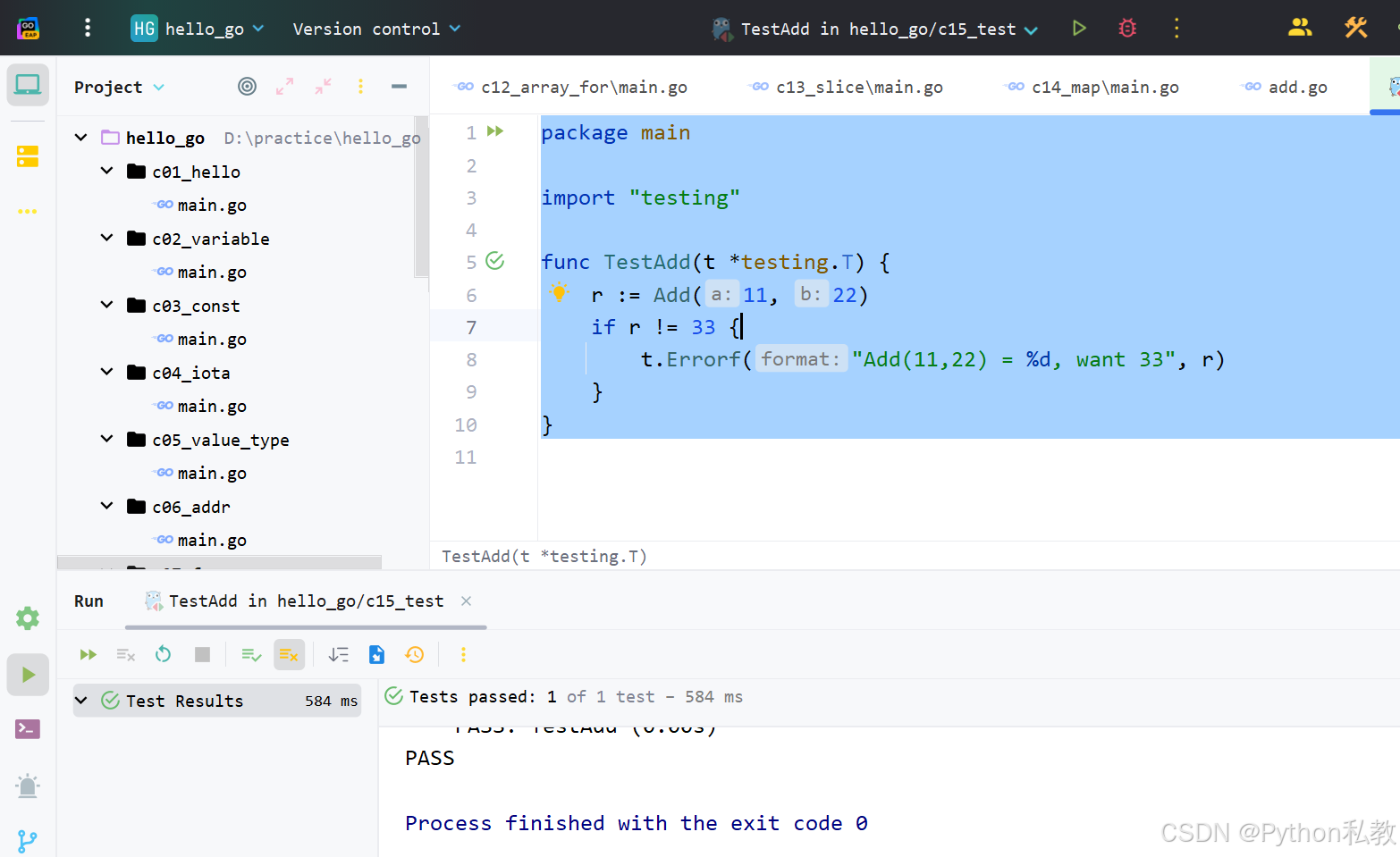
Golang零基础入门课_20240726 课程笔记
视频课程 最近发现越来越多的公司在用Golang了,所以精心整理了一套视频教程给大家,这个只是其中的第一部,后续还会有很多。 视频已经录制完成,完整目录截图如下: 课程目录 01 第一个Go程序.mp402 定义变量.mp403 …...

杂记-镜像
-i https://pypi.tuna.tsinghua.edu.cn/simple 清华 pip intall 出现 error: subprocess-exited-with-error 错误的解决办法———————————pip install --upgrade pip setuptools57.5.0 ————————————————————————————————————…...
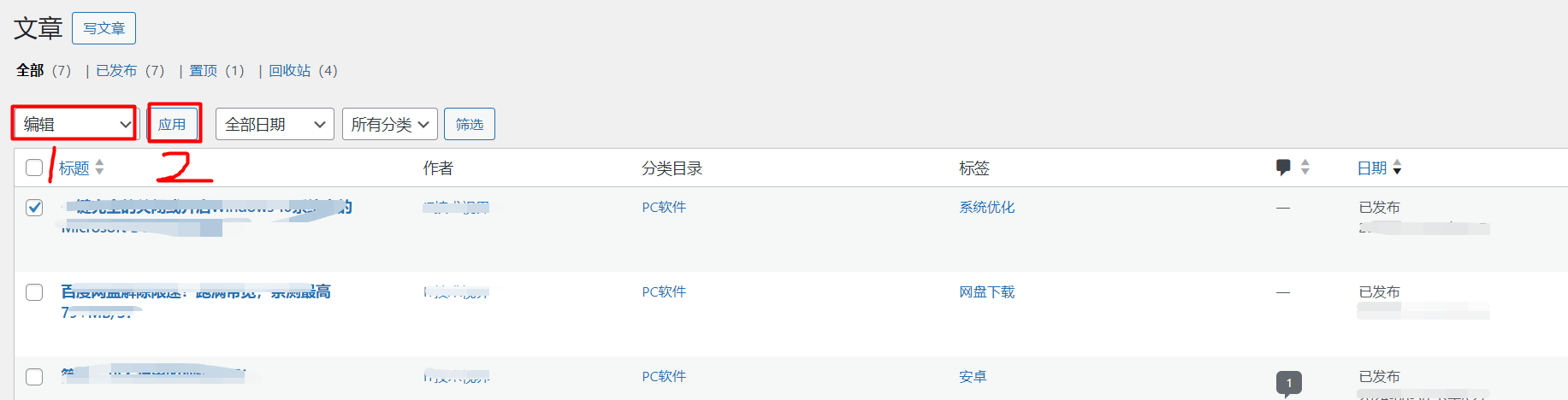
如何将WordPress文章中的外链图片批量导入到本地
在使用采集软件进行内容创作时,很多文章中的图片都是远程链接,这不仅会导致前端加载速度慢,还会在微信小程序和抖音小程序中添加各种域名,造成管理上的麻烦。特别是遇到没有备案的外链,更是让人头疼。因此,…...
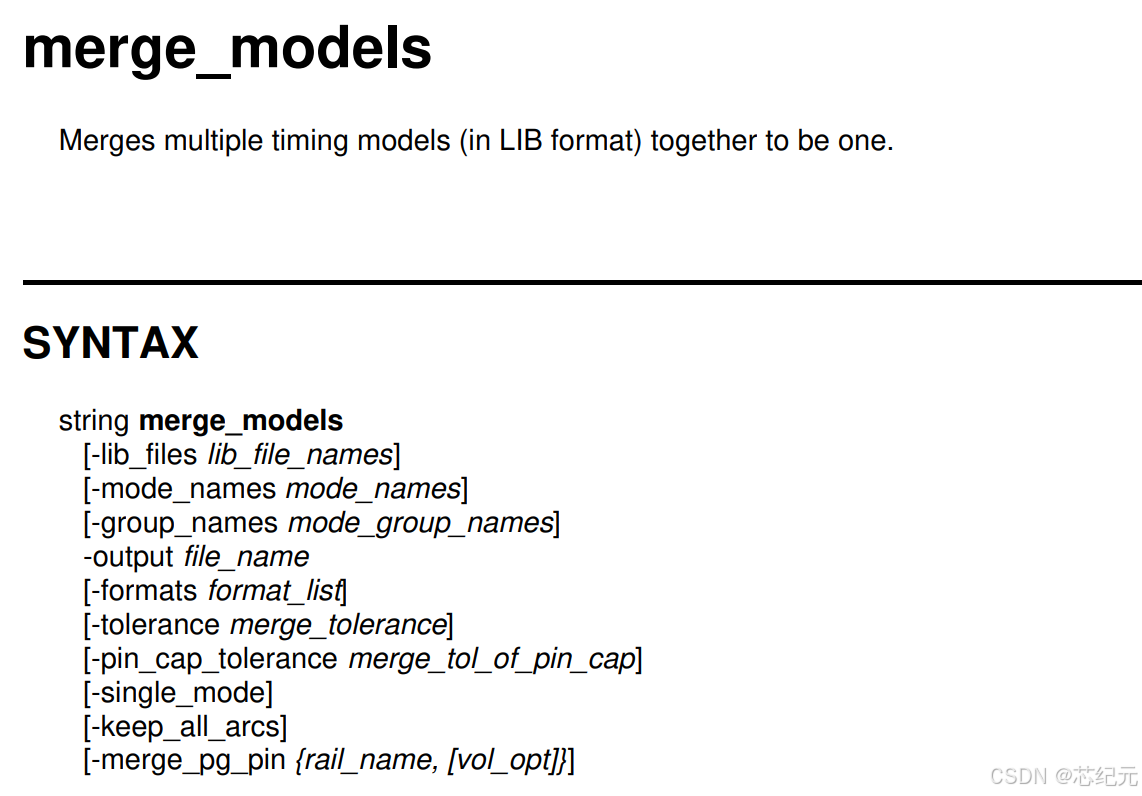
primetime如何合并不同modes的libs到一个lib文件
首先,用primetime 抽 timing model 的指令如下。 代码如下(示例): #抽lib时留一些margin, setup -max/hold -min set_extract_model_margin -port [get_ports -filter "!defined(clocks)"] -max 0.1 #抽lib extract_mod…...
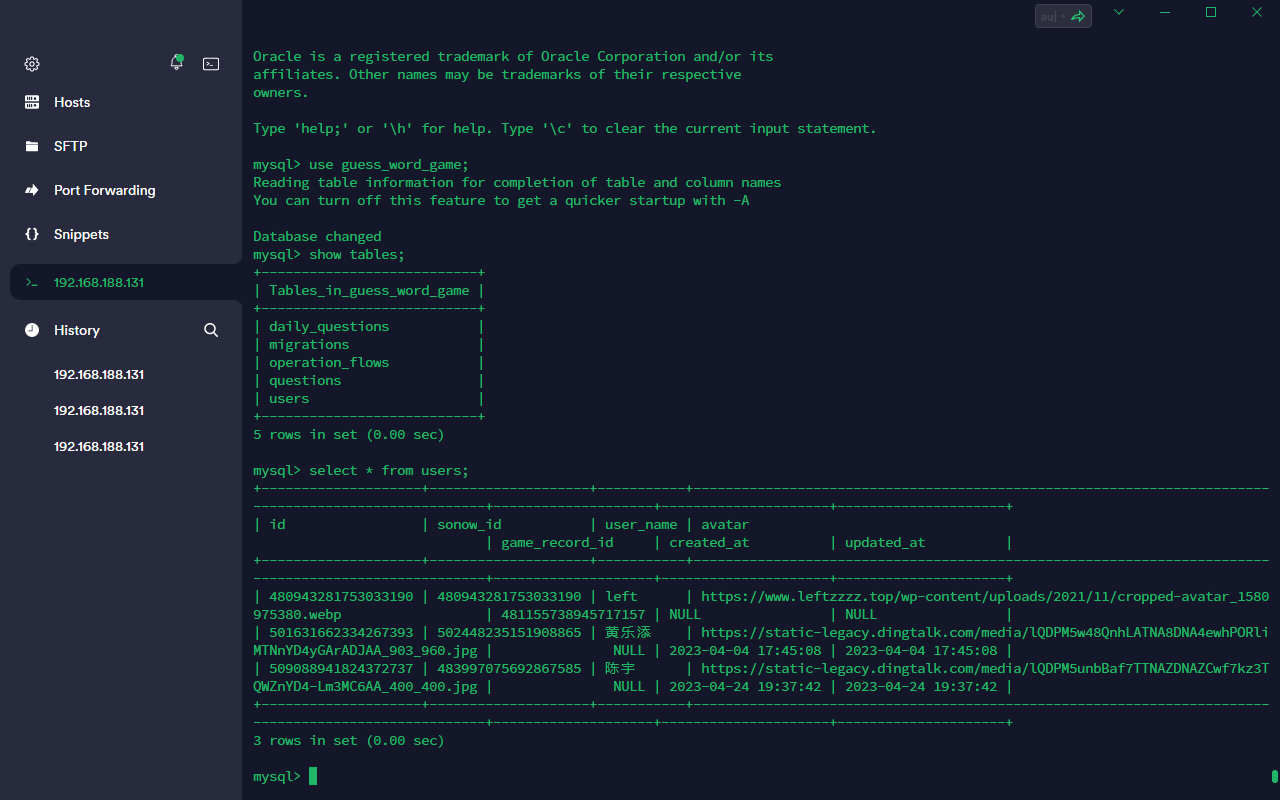
【运维笔记】数据库无法启动,数据库炸后备份恢复数据
事情起因 在做docker作业的时候,把卷映射到了宿主机原来的mysql数据库目录上,宿主机原来的mysql版本为8.0,docker容器版本为5.6,导致翻车。 具体操作 备份目录 将/var/lib/mysql备份到~/mysql_backup:cp /var/lib/…...
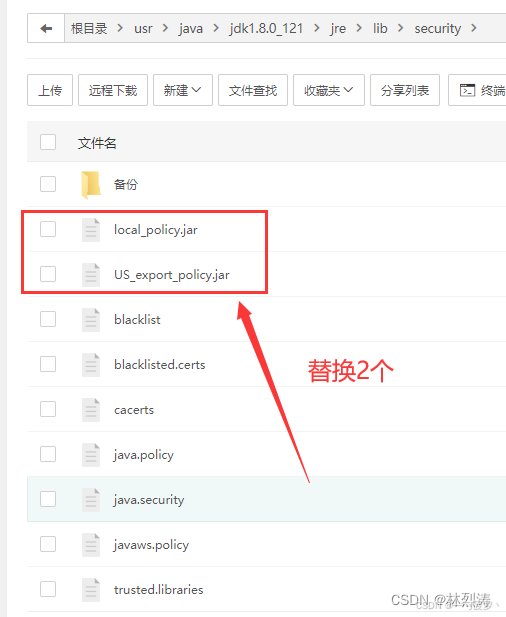
成功解决:java.security.InvalidKeyException: Illegal key size
在集成微信支付到Spring Boot项目时,可能会遇到启动报错 java.security.InvalidKeyException: Illegal key size 的问题。这是由于Java加密扩展(JCE)限制了密钥的长度。幸运的是,我们可以通过简单的替换文件来解决这个问题。 解决…...
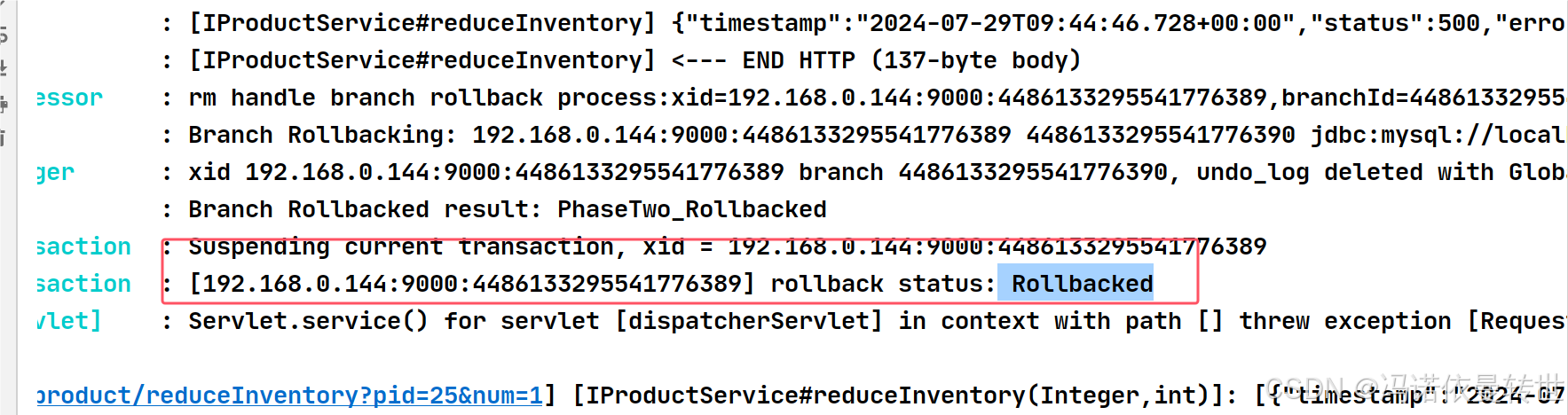
微服务事务管理(分布式事务问题 理论基础 初识Seata XA模式 AT模式 )
目录 一、分布式事务问题 1. 本地事务 2. 分布式事务 3. 演示分布式事务问题 二、理论基础 1. CAP定理 1.1 ⼀致性 1.2 可⽤性 1.3 分区容错 1.4 ⽭盾 2. BASE理论 3. 解决分布式事务的思路 三、初识Seata 1. Seata的架构 2. 部署TC服务 3. 微服务集成Se…...

测试面试宝典(三十五)—— fiddler的工作原理
Fiddler 是一款强大的 Web 调试工具,其工作原理主要基于代理服务器的机制。 首先,当您在计算机上配置 Fiddler 为系统代理时,客户端(如浏览器)发出的所有 HTTP 和 HTTPS 请求都会被导向 Fiddler。 Fiddler 接收到这些…...
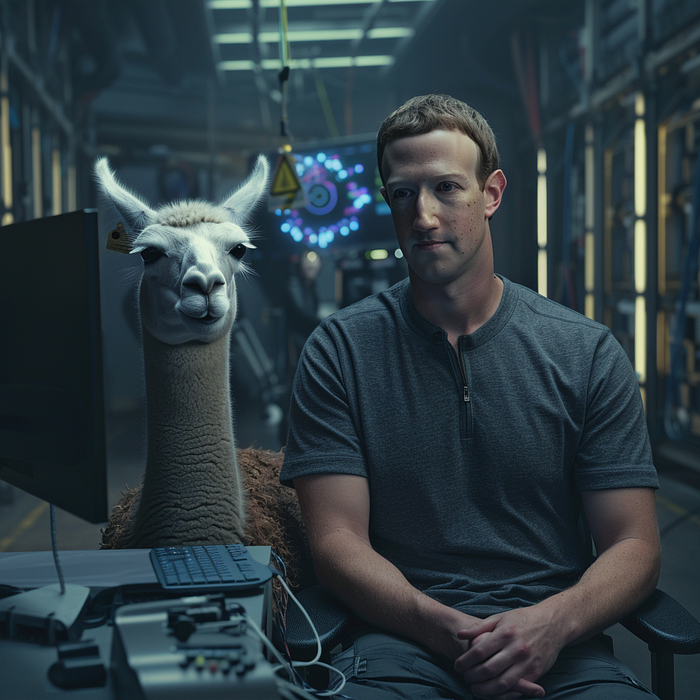
旷野之间32 - OpenAI 拉开了人工智能竞赛的序幕,而Meta 将会赢得胜利
他们通过故事做到了这一点(Snapchat 是第一个)他们用 Reels 实现了这个功能(TikTok 是第一个实现这个功能的)他们正在利用人工智能来实现这一点。 在人工智能竞赛开始时,Meta 的人工智能平台的表现并没有什么特别值得…...
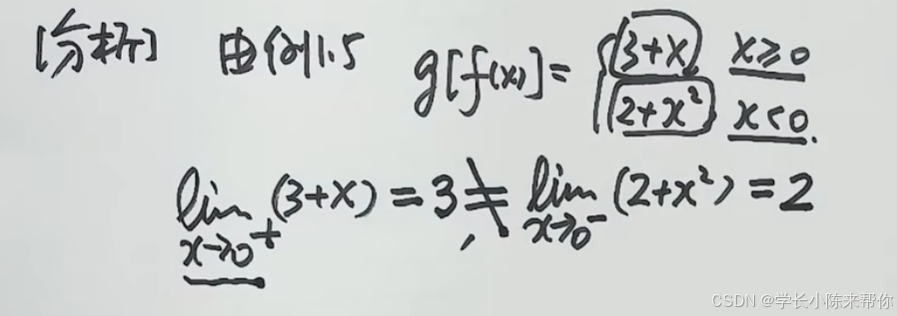
机械学习—零基础学习日志(高数15——函数极限性质)
零基础为了学人工智能,真的开始复习高数 这里我们将会学习函数极限的性质。 唯一性 来一个练习题: 再来一个练习: 这里我问了一下ChatGPT,如果一个值两侧分别趋近于正无穷,以及负无穷。理论上这个极限值应该说是不存…...
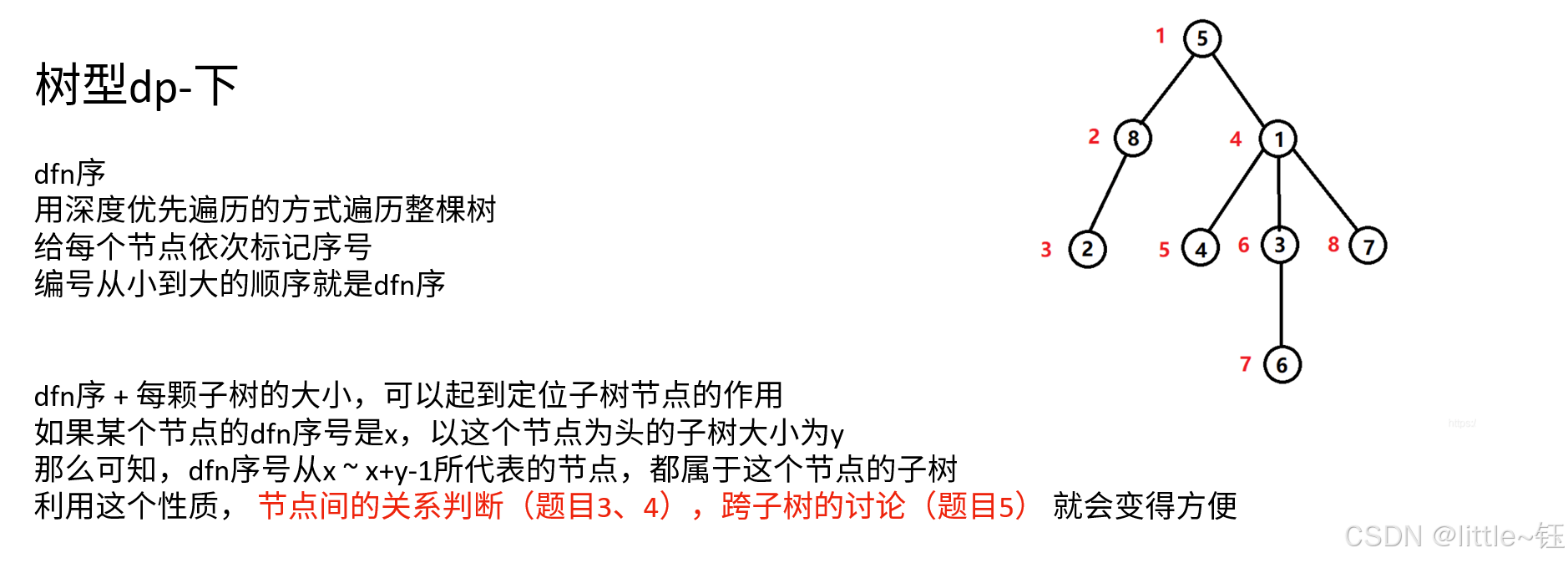
树 形 DP (dnf序)
二叉搜索子树的最大键值 /*** Definition for a binary tree node.* struct TreeNode {* int val;* TreeNode *left;* TreeNode *right;* TreeNode() : val(0), left(nullptr), right(nullptr) {}* TreeNode(int x) : val(x), left(nullptr), right(null…...

React的生命周期?
React的生命周期分为三个主要阶段:挂载(Mounting)、更新(Updating)和卸载(Unmounting)。 1、挂载(Mounting) 当组件实例被创建并插入 DOM 时调用的生命周期方法&#x…...
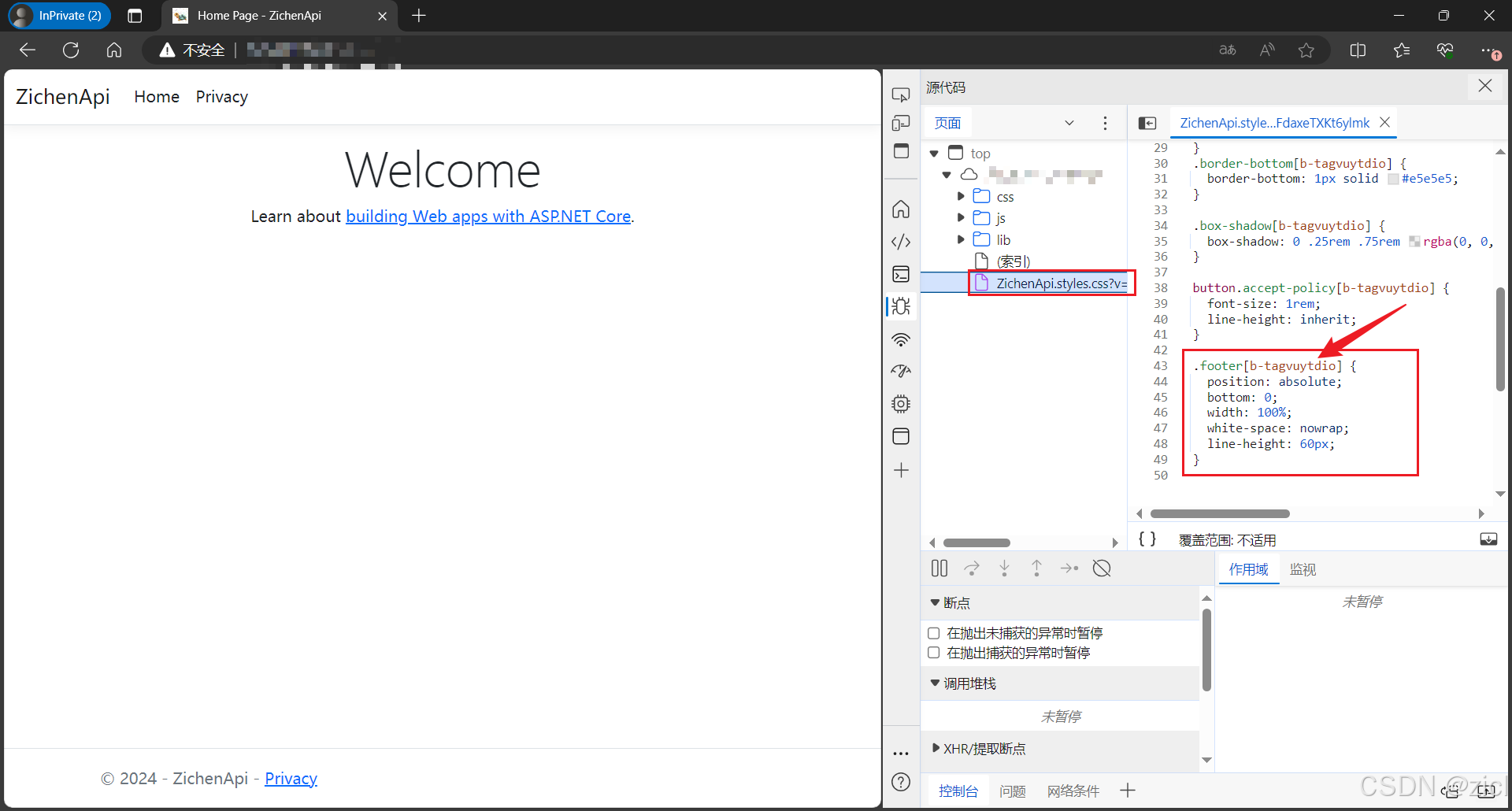
c# - - - ASP.NET Core 网页样式丢失,样式不对
c# - - - ASP.NET Core 网页样式丢失,样式不对 问题 正常样式是这样的。 修改项目名后,样式就变成这样了。底部的内容跑到中间了。 解决 重新生成解决方案,然后发布网站。 原因: 修改项目名之前的 div 上有个这个自定义属…...

Cannot find module ‘html-webpack-plugin
当你在使用Webpack构建项目时遇到Cannot find module html-webpack-plugin这样的错误,这意味着Webpack在构建过程中找不到html-webpack-plugin模块。要解决这个问题,你需要确保已经正确安装了html-webpack-plugin模块,并且在Webpack配置文件中…...

vue、react部署项目的 hashRouter 和 historyRouter模式
Vue 项目 使用 hashRouter 如果你使用的是 hashRouter,通常不需要修改 base,因为 hashRouter 使用 URL 的哈希部分来管理路由,这部分不会被服务器处理。你只需要确保 publicPath 设置正确即可。 使用 historyRouter 如果你使用的是 histo…...