CUDA中的流序内存分配
文章目录
- CUDA中的流序内存分配
- 1. Introduction
- 2. Query for Support
- 3. API Fundamentals (cudaMallocAsync and cudaFreeAsync)
- 4. Memory Pools and the cudaMemPool_t
- 注意:设备的内存池当前将是该设备的本地。因此,在不指定内存池的情况下进行分配将始终产生流设备本地的分配。
- 注意:`cudaMemPoolSetAttribute` 和 `cudaMemPoolGetAttribute` 控制内存池的属性。
- 5. Default/Impicit Pools
- 6. Explicit Pools
- 7. Physical Page Caching Behavior
- 8. Resource Usage Statistics
- 9. Memory Reuse Policies
- 9.1. cudaMemPoolReuseFollowEventDependencies
- 9.2. cudaMemPoolReuseAllowOpportunistic
- 9.3. cudaMemPoolReuseAllowInternalDependencies
- 9.4. Disabling Reuse Policies
- 10. Device Accessibility for Multi-GPU Support
- 11. IPC Memory Pools
- 11.1. Creating and Sharing IPC Memory Pools
- 11.2. Set Access in the Importing Process
- 11.3. Creating and Sharing Allocations from an Exported Pool
- 11.4. IPC Export Pool Limitations
- 11.5. IPC Import Pool Limitations
- 12. Synchronization API Actions
- 13. Addendums
- 13.1. cudaMemcpyAsync Current Context/Device Sensitivity
- 13.2. cuPointerGetAttribute Query
- 13.3. cuGraphAddMemsetNode
- 13.4. Pointer Attributes
CUDA中的流序内存分配
1. Introduction
使用 cudaMalloc
和 cudaFree
管理内存分配会导致 GPU 在所有正在执行的 CUDA 流之间进行同步。 Stream Order Memory Allocator 使应用程序能够通过启动到 CUDA 流中的其他工作(例如内核启动和异步拷贝)来对内存分配和释放进行排序。这通过利用流排序语义来重用内存分配来改进应用程序内存使用。分配器还允许应用程序控制分配器的内存缓存行为。当设置了适当的释放阈值时,缓存行为允许分配器在应用程序表明它愿意接受更大的内存占用时避免对操作系统进行昂贵的调用。分配器还支持在进程之间轻松安全地共享分配。
对于许多应用程序,Stream Ordered Memory Allocator 减少了对自定义内存管理抽象的需求,并使为需要它的应用程序创建高性能自定义内存管理变得更加容易。对于已经具有自定义内存分配器的应用程序和库,采用 Stream Ordered Memory Allocator 可以使多个库共享由驱动程序管理的公共内存池,从而减少过多的内存消耗。此外,驱动程序可以根据其对分配器和其他流管理 API 的感知执行优化。最后,Nsight Compute 和 Next-Gen CUDA 调试器知道分配器是其 CUDA 11.3 工具包支持的一部分。
2. Query for Support
用户可以通过使用设备属性 cudaDevAttrMemoryPoolsSupported
调用 cudaDeviceGetAttribute()
来确定设备是否支持流序内存分配器。
从 CUDA 11.3 开始,可以使用 cudaDevAttrMemoryPoolSupportedHandleTypes
设备属性查询 IPC 内存池支持。 以前的驱动程序将返回 cudaErrorInvalidValue
,因为这些驱动程序不知道属性枚举。
int driverVersion = 0;
int deviceSupportsMemoryPools = 0;
int poolSupportedHandleTypes = 0;
cudaDriverGetVersion(&driverVersion);
if (driverVersion >= 11020) {cudaDeviceGetAttribute(&deviceSupportsMemoryPools,cudaDevAttrMemoryPoolsSupported, device);
}
if (deviceSupportsMemoryPools != 0) {// `device` supports the Stream Ordered Memory Allocator
}if (driverVersion >= 11030) {cudaDeviceGetAttribute(&poolSupportedHandleTypes,cudaDevAttrMemoryPoolSupportedHandleTypes, device);
}
if (poolSupportedHandleTypes & cudaMemHandleTypePosixFileDescriptor) {// Pools on the specified device can be created with posix file descriptor-based IPC
}
在查询之前执行驱动程序版本检查可避免在尚未定义属性的驱动程序上遇到 cudaErrorInvalidValue
错误。 可以使用 cudaGetLastError
来清除错误而不是避免它。
3. API Fundamentals (cudaMallocAsync and cudaFreeAsync)
API cudaMallocAsync
和 cudaFreeAsync
构成了分配器的核心。 cudaMallocAsync
返回分配,cudaFreeAsync
释放分配。 两个 API 都接受流参数来定义分配何时变为可用和停止可用。 cudaMallocAsync
返回的指针值是同步确定的,可用于构建未来的工作。 重要的是要注意 cudaMallocAsync
在确定分配的位置时会忽略当前设备/上下文。 相反,cudaMallocAsync
根据指定的内存池或提供的流来确定常驻设备。 最简单的使用模式是分配、使用和释放内存到同一个流中。
void *ptr;
size_t size = 512;
cudaMallocAsync(&ptr, size, cudaStreamPerThread);
// do work using the allocation
kernel<<<..., cudaStreamPerThread>>>(ptr, ...);
// An asynchronous free can be specified without synchronizing the CPU and GPU
cudaFreeAsync(ptr, cudaStreamPerThread);
用户可以使用 cudaFreeAsync()
释放使用 cudaMalloc()
分配的内存。 在自由操作开始之前,用户必须对访问完成做出同样的保证。
cudaMalloc(&ptr, size);
kernel<<<..., stream>>>(ptr, ...);
cudaFreeAsync(ptr, stream);
用户可以使用 cudaFree()
释放使用 cudaMallocAsync
分配的内存。 通过 cudaFree()
API 释放此类分配时,驱动程序假定对分配的所有访问都已完成,并且不执行进一步的同步。 用户可以使用 cudaStreamQuery / cudaStreamSynchronize / cudaEventQuery / cudaEventSynchronize / cudaDeviceSynchronize
来保证适当的异步工作完成并且GPU不会尝试访问分配。
cudaMallocAsync(&ptr, size,stream);
kernel<<<..., stream>>>(ptr, ...);
// synchronize is needed to avoid prematurely freeing the memory
cudaStreamSynchronize(stream);
cudaFree(ptr);
4. Memory Pools and the cudaMemPool_t
内存池封装了虚拟地址和物理内存资源,根据内存池的属性和属性进行分配和管理。内存池的主要方面是它所管理的内存的种类和位置。
所有对 cudaMallocAsync
的调用都使用内存池的资源。在没有指定内存池的情况下,cudaMallocAsync
API 使用提供的流设备的当前内存池。设备的当前内存池可以使用 cudaDeviceSetMempool
设置并使用 cudaDeviceGetMempool
查询。默认情况下(在没有 cudaDeviceSetMempool
调用的情况下),当前内存池是设备的默认内存池。 cudaMallocFromPoolAsync
的 API cudaMallocFromPoolAsync
和 c++ 重载允许用户指定要用于分配的池,而无需将其设置为当前池。 API cudaDeviceGetDefaultMempool
和 cudaMemPoolCreate
为用户提供内存池的句柄。
注意:设备的内存池当前将是该设备的本地。因此,在不指定内存池的情况下进行分配将始终产生流设备本地的分配。
注意:cudaMemPoolSetAttribute
和 cudaMemPoolGetAttribute
控制内存池的属性。
5. Default/Impicit Pools
可以使用 cudaDeviceGetDefaultMempool
API 检索设备的默认内存池。 来自设备默认内存池的分配是位于该设备上的不可迁移设备分配。 这些分配将始终可以从该设备访问。 默认内存池的可访问性可以通过 cudaMemPoolSetAccess
进行修改,并通过 cudaMemPoolGetAccess
进行查询。 由于不需要显式创建默认池,因此有时将它们称为隐式池。 设备默认内存池不支持IPC。
6. Explicit Pools
API cudaMemPoolCreate
创建一个显式池。 目前内存池只能分配设备分配。 分配将驻留的设备必须在属性结构中指定。 显式池的主要用例是 IPC 功能。
// create a pool similar to the implicit pool on device 0
int device = 0;
cudaMemPoolProps poolProps = { };
poolProps.allocType = cudaMemAllocationTypePinned;
poolProps.location.id = device;
poolProps.location.type = cudaMemLocationTypeDevice;cudaMemPoolCreate(&memPool, &poolProps));
7. Physical Page Caching Behavior
默认情况下,分配器尝试最小化池拥有的物理内存。 为了尽量减少分配和释放物理内存的操作系统调用,应用程序必须为每个池配置内存占用。 应用程序可以使用释放阈值属性 (cudaMemPoolAttrReleaseThreshold
) 执行此操作。
释放阈值是池在尝试将内存释放回操作系统之前应保留的内存量(以字节为单位)。 当内存池持有超过释放阈值字节的内存时,分配器将尝试在下一次调用流、事件或设备同步时将内存释放回操作系统。 将释放阈值设置为 UINT64_MAX
将防止驱动程序在每次同步后尝试收缩池。
Cuuint64_t setVal = UINT64_MAX;
cudaMemPoolSetAttribute(memPool, cudaMemPoolAttrReleaseThreshold, &setVal);
将 cudaMemPoolAttrReleaseThreshold
设置得足够高以有效禁用内存池收缩的应用程序可能希望显式收缩内存池的内存占用。 cudaMemPoolTrimTo
允许此类应用程序这样做。 在修剪内存池的占用空间时,minBytesToKeep
参数允许应用程序保留它预期在后续执行阶段需要的内存量。
Cuuint64_t setVal = UINT64_MAX;
cudaMemPoolSetAttribute(memPool, cudaMemPoolAttrReleaseThreshold, &setVal);// application phase needing a lot of memory from the stream ordered allocator
for (i=0; i<10; i++) {for (j=0; j<10; j++) {cudaMallocAsync(&ptrs[j],size[j], stream);}kernel<<<...,stream>>>(ptrs,...);for (j=0; j<10; j++) {cudaFreeAsync(ptrs[j], stream);}
}// Process does not need as much memory for the next phase.
// Synchronize so that the trim operation will know that the allocations are no
// longer in use.
cudaStreamSynchronize(stream);
cudaMemPoolTrimTo(mempool, 0);// Some other process/allocation mechanism can now use the physical memory
// released by the trimming operation.
8. Resource Usage Statistics
在 CUDA 11.3 中,添加了池属性 cudaMemPoolAttrReservedMemCurrent、cudaMemPoolAttrReservedMemHigh、cudaMemPoolAttrUsedMemCurrent 和 cudaMemPoolAttrUsedMemHigh
来查询池的内存使用情况。
查询池的 cudaMemPoolAttrReservedMemCurrent
属性会报告该池当前消耗的总物理 GPU 内存。 查询池的 cudaMemPoolAttrUsedMemCurrent
会返回从池中分配且不可重用的所有内存的总大小。
cudaMemPoolAttr*MemHigh
属性是记录自上次重置以来各个 cudaMemPoolAttr*MemCurrent
属性达到的最大值的水印。 可以使用 cudaMemPoolSetAttribute
API 将它们重置为当前值。
// sample helper functions for getting the usage statistics in bulk
struct usageStatistics {cuuint64_t reserved;cuuint64_t reservedHigh;cuuint64_t used;cuuint64_t usedHigh;
};void getUsageStatistics(cudaMemoryPool_t memPool, struct usageStatistics *statistics)
{cudaMemPoolGetAttribute(memPool, cudaMemPoolAttrReservedMemCurrent, statistics->reserved);cudaMemPoolGetAttribute(memPool, cudaMemPoolAttrReservedMemHigh, statistics->reservedHigh);cudaMemPoolGetAttribute(memPool, cudaMemPoolAttrUsedMemCurrent, statistics->used);cudaMemPoolGetAttribute(memPool, cudaMemPoolAttrUsedMemHigh, statistics->usedHigh);
}// resetting the watermarks will make them take on the current value.
void resetStatistics(cudaMemoryPool_t memPool)
{cuuint64_t value = 0;cudaMemPoolSetAttribute(memPool, cudaMemPoolAttrReservedMemHigh, &value);cudaMemPoolSetAttribute(memPool, cudaMemPoolAttrUsedMemHigh, &value);
}
9. Memory Reuse Policies
为了服务分配请求,驱动程序在尝试从操作系统分配更多内存之前尝试重用之前通过 cudaFreeAsync()
释放的内存。 例如,流中释放的内存可以立即重新用于同一流中的后续分配请求。 类似地,当一个流与 CPU 同步时,之前在该流中释放的内存可以重新用于任何流中的分配。
流序分配器有一些可控的分配策略。 池属性 cudaMemPoolReuseFollowEventDependencies、cudaMemPoolReuseAllowOpportunistic 和 cudaMemPoolReuseAllowInternalDependencies
控制这些策略。 升级到更新的 CUDA 驱动程序可能会更改、增强、增加或重新排序重用策略。
9.1. cudaMemPoolReuseFollowEventDependencies
在分配更多物理 GPU 内存之前,分配器会检查由 CUDA 事件建立的依赖信息,并尝试从另一个流中释放的内存中进行分配。
cudaMallocAsync(&ptr, size, originalStream);
kernel<<<..., originalStream>>>(ptr, ...);
cudaFreeAsync(ptr, originalStream);
cudaEventRecord(event,originalStream);// waiting on the event that captures the free in another stream
// allows the allocator to reuse the memory to satisfy
// a new allocation request in the other stream when
// cudaMemPoolReuseFollowEventDependencies is enabled.
cudaStreamWaitEvent(otherStream, event);
cudaMallocAsync(&ptr2, size, otherStream);
9.2. cudaMemPoolReuseAllowOpportunistic
根据 cudaMemPoolReuseAllowOpportunistic
策略,分配器检查释放的分配以查看是否满足释放的流序语义(即流已通过释放指示的执行点)。 禁用此功能后,分配器仍将重用在流与 cpu 同步时可用的内存。 禁用此策略不会阻止 cudaMemPoolReuseFollowEventDependencies
应用。
cudaMallocAsync(&ptr, size, originalStream);
kernel<<<..., originalStream>>>(ptr, ...);
cudaFreeAsync(ptr, originalStream);// after some time, the kernel finishes running
wait(10);// When cudaMemPoolReuseAllowOpportunistic is enabled this allocation request
// can be fulfilled with the prior allocation based on the progress of originalStream.
cudaMallocAsync(&ptr2, size, otherStream);
9.3. cudaMemPoolReuseAllowInternalDependencies
如果无法从操作系统分配和映射更多物理内存,驱动程序将寻找其可用性取决于另一个流的待处理进度的内存。 如果找到这样的内存,驱动程序会将所需的依赖项插入分配流并重用内存。
cudaMallocAsync(&ptr, size, originalStream);
kernel<<<..., originalStream>>>(ptr, ...);
cudaFreeAsync(ptr, originalStream);// When cudaMemPoolReuseAllowInternalDependencies is enabled
// and the driver fails to allocate more physical memory, the driver may
// effectively perform a cudaStreamWaitEvent in the allocating stream
// to make sure that future work in ‘otherStream’ happens after the work
// in the original stream that would be allowed to access the original allocation.
cudaMallocAsync(&ptr2, size, otherStream);
9.4. Disabling Reuse Policies
虽然可控重用策略提高了内存重用,但用户可能希望禁用它们。 允许机会重用(即 cudaMemPoolReuseAllowOpportunistic
)基于 CPU 和 GPU 执行的交错引入了运行到运行分配模式的差异。 当用户宁愿在分配失败时显式同步事件或流时,内部依赖插入(即 cudaMemPoolReuseAllowInternalDependencies
)可以以意想不到的和潜在的非确定性方式序列化工作。
10. Device Accessibility for Multi-GPU Support
就像通过虚拟内存管理 API 控制的分配可访问性一样,内存池分配可访问性不遵循 cudaDeviceEnablePeerAccess
或 cuCtxEnablePeerAccess
。相反,API cudaMemPoolSetAccess
修改了哪些设备可以访问池中的分配。默认情况下,可以从分配所在的设备访问分配。无法撤销此访问权限。要启用其他设备的访问,访问设备必须与内存池的设备对等;检查 cudaDeviceCanAccessPeer
。如果未检查对等功能,则设置访问可能会失败并显示 cudaErrorInvalidDevice
。如果没有从池中进行分配,即使设备不具备对等能力,cudaMemPoolSetAccess
调用也可能成功;在这种情况下,池中的下一次分配将失败。
值得注意的是,cudaMemPoolSetAccess
会影响内存池中的所有分配,而不仅仅是未来的分配。此外,cudaMemPoolGetAccess
报告的可访问性适用于池中的所有分配,而不仅仅是未来的分配。建议不要频繁更改给定 GPU 的池的可访问性设置;一旦池可以从给定的 GPU 访问,它应该在池的整个生命周期内都可以从该 GPU 访问。
// snippet showing usage of cudaMemPoolSetAccess:
cudaError_t setAccessOnDevice(cudaMemPool_t memPool, int residentDevice,int accessingDevice) {cudaMemAccessDesc accessDesc = {};accessDesc.location.type = cudaMemLocationTypeDevice;accessDesc.location.id = accessingDevice;accessDesc.flags = cudaMemAccessFlagsProtReadWrite;int canAccess = 0;cudaError_t error = cudaDeviceCanAccessPeer(&canAccess, accessingDevice,residentDevice);if (error != cudaSuccess) {return error;} else if (canAccess == 0) {return cudaErrorPeerAccessUnsupported;}// Make the address accessiblereturn cudaMemPoolSetAccess(memPool, &accessDesc, 1);
}
11. IPC Memory Pools
支持 IPC 的内存池允许在进程之间轻松、高效和安全地共享 GPU 内存。 CUDA 的 IPC 内存池提供与 CUDA 的虚拟内存管理 API 相同的安全优势。
在具有内存池的进程之间共享内存有两个阶段。 进程首先需要共享对池的访问权限,然后共享来自该池的特定分配。 第一阶段建立并实施安全性。 第二阶段协调每个进程中使用的虚拟地址以及映射何时需要在导入过程中有效。
11.1. Creating and Sharing IPC Memory Pools
共享对池的访问涉及检索池的 OS 本机句柄(使用 cudaMemPoolExportToShareableHandle()
API),使用通常的 OS 本机 IPC 机制将句柄转移到导入进程,并创建导入的内存池(使用 cudaMemPoolImportFromShareableHandle()
API)。 要使 cudaMemPoolExportToShareableHandle
成功,必须使用池属性结构中指定的请求句柄类型创建内存池。 请参考示例以了解在进程之间传输操作系统本机句柄的适当 IPC 机制。 该过程的其余部分可以在以下代码片段中找到。
// in exporting process
// create an exportable IPC capable pool on device 0
cudaMemPoolProps poolProps = { };
poolProps.allocType = cudaMemAllocationTypePinned;
poolProps.location.id = 0;
poolProps.location.type = cudaMemLocationTypeDevice;// Setting handleTypes to a non zero value will make the pool exportable (IPC capable)
poolProps.handleTypes = CU_MEM_HANDLE_TYPE_POSIX_FILE_DESCRIPTOR;cudaMemPoolCreate(&memPool, &poolProps));// FD based handles are integer types
int fdHandle = 0;// Retrieve an OS native handle to the pool.
// Note that a pointer to the handle memory is passed in here.
cudaMemPoolExportToShareableHandle(&fdHandle,memPool,CU_MEM_HANDLE_TYPE_POSIX_FILE_DESCRIPTOR,0);// The handle must be sent to the importing process with the appropriate
// OS specific APIs.
// in importing processint fdHandle;
// The handle needs to be retrieved from the exporting process with the
// appropriate OS specific APIs.
// Create an imported pool from the shareable handle.
// Note that the handle is passed by value here.
cudaMemPoolImportFromShareableHandle(&importedMemPool,(void*)fdHandle,CU_MEM_HANDLE_TYPE_POSIX_FILE_DESCRIPTOR,0);
11.2. Set Access in the Importing Process
导入的内存池最初只能从其常驻设备访问。 导入的内存池不继承导出进程设置的任何可访问性。 导入过程需要启用从它计划访问内存的任何 GPU 的访问(使用 cudaMemPoolSetAccess
)。
如果导入的内存池在导入过程中属于不可见的设备,则用户必须使用 cudaMemPoolSetAccess
API 来启用从将使用分配的 GPU 的访问。
11.3. Creating and Sharing Allocations from an Exported Pool
共享池后,在导出进程中使用 cudaMallocAsync()
从池中进行的分配可以与已导入池的其他进程共享。由于池的安全策略是在池级别建立和验证的,操作系统不需要额外的簿记来为特定的池分配提供安全性;换句话说,导入池分配所需的不透明 cudaMemPoolPtrExportData
可以使用任何机制发送到导入进程。
虽然分配可以在不以任何方式与分配流同步的情况下导出甚至导入,但在访问分配时,导入过程必须遵循与导出过程相同的规则。即,对分配的访问必须发生在分配流中分配操作的流排序之后。以下两个代码片段显示 cudaMemPoolExportPointer()
和 cudaMemPoolImportPointer()
与 IPC 事件共享分配,用于保证在分配准备好之前在导入过程中不会访问分配。
// preparing an allocation in the exporting process
cudaMemPoolPtrExportData exportData;
cudaEvent_t readyIpcEvent;
cudaIpcEventHandle_t readyIpcEventHandle;// IPC event for coordinating between processes
// cudaEventInterprocess flag makes the event an IPC event
// cudaEventDisableTiming is set for performance reasonscudaEventCreate(&readyIpcEvent, cudaEventDisableTiming | cudaEventInterprocess)// allocate from the exporting mem pool
cudaMallocAsync(&ptr, size,exportMemPool, stream);// event for sharing when the allocation is ready.
cudaEventRecord(readyIpcEvent, stream);
cudaMemPoolExportPointer(&exportData, ptr);
cudaIpcGetEventHandle(&readyIpcEventHandle, readyIpcEvent);// Share IPC event and pointer export data with the importing process using
// any mechanism. Here we copy the data into shared memory
shmem->ptrData = exportData;
shmem->readyIpcEventHandle = readyIpcEventHandle;
// signal consumers data is ready
// Importing an allocation
cudaMemPoolPtrExportData *importData = &shmem->prtData;
cudaEvent_t readyIpcEvent;
cudaIpcEventHandle_t *readyIpcEventHandle = &shmem->readyIpcEventHandle;// Need to retrieve the IPC event handle and the export data from the
// exporting process using any mechanism. Here we are using shmem and just
// need synchronization to make sure the shared memory is filled in.cudaIpcOpenEventHandle(&readyIpcEvent, readyIpcEventHandle);// import the allocation. The operation does not block on the allocation being ready.
cudaMemPoolImportPointer(&ptr, importedMemPool, importData);// Wait for the prior stream operations in the allocating stream to complete before
// using the allocation in the importing process.
cudaStreamWaitEvent(stream, readyIpcEvent);
kernel<<<..., stream>>>(ptr, ...);
释放分配时,需要先在导入过程中释放分配,然后在导出过程中释放分配。 以下代码片段演示了使用 CUDA IPC 事件在两个进程中的 cudaFreeAsync
操作之间提供所需的同步。 导入过程中对分配的访问显然受到导入过程侧的自由操作的限制。 值得注意的是,cudaFree
可用于释放两个进程中的分配,并且可以使用其他流同步 API 代替 CUDA IPC 事件。
// The free must happen in importing process before the exporting process
kernel<<<..., stream>>>(ptr, ...); // Last access in importing process
cudaFreeAsync(ptr, stream); // Access not allowed in the importing process after the free
cudaIpcEventRecord(finishedIpcEvent, stream);
// Exporting process
// The exporting process needs to coordinate its free with the stream order
// of the importing process’s free.
cudaStreamWaitEvent(stream, finishedIpcEvent);
kernel<<<..., stream>>>(ptrInExportingProcess, ...); // The free in the importing process doesn’t stop the exporting process
// from using the allocation.
cudFreeAsync(ptrInExportingProcess,stream);
11.4. IPC Export Pool Limitations
IPC 池目前不支持将物理块释放回操作系统。 因此,cudaMemPoolTrimTo
API 充当空操作,并且 cudaMemPoolAttrReleaseThreshold
被有效地忽略。 此行为由驱动程序控制,而不是运行时控制,并且可能会在未来的驱动程序更新中发生变化。
11.5. IPC Import Pool Limitations
不允许从导入池中分配; 具体来说,导入池不能设置为当前,也不能在 cudaMallocFromPoolAsync
API 中使用。 因此,分配重用策略属性对这些池没有意义。
IPC 池目前不支持将物理块释放回操作系统。 因此,cudaMemPoolTrimTo
API 充当空操作,并且 cudaMemPoolAttrReleaseThreshold
被有效地忽略。
资源使用统计属性查询仅反映导入进程的分配和相关的物理内存。
12. Synchronization API Actions
作为 CUDA 驱动程序一部分的分配器带来的优化之一是与同步 API 的集成。 当用户请求 CUDA 驱动程序同步时,驱动程序等待异步工作完成。 在返回之前,驱动程序将确定什么释放了保证完成的同步。 无论指定的流或禁用的分配策略如何,这些分配都可用于分配。 驱动程序还在这里检查 cudaMemPoolAttrReleaseThreshold
并释放它可以释放的任何多余的物理内存。
13. Addendums
13.1. cudaMemcpyAsync Current Context/Device Sensitivity
在当前的 CUDA 驱动程序中,任何涉及来自 cudaMallocAsync
的内存的异步 memcpy
都应该使用指定流的上下文作为调用线程的当前上下文来完成。 这对于 cudaMemcpyPeerAsync
不是必需的,因为引用了 API 中指定的设备主上下文而不是当前上下文。
13.2. cuPointerGetAttribute Query
在对分配调用 cudaFreeAsync
后在分配上调用 cuPointerGetAttribute
会导致未定义的行为。 具体来说,分配是否仍然可以从给定的流中访问并不重要:行为仍然是未定义的。
13.3. cuGraphAddMemsetNode
cuGraphAddMemsetNode
不适用于通过流排序分配器分配的内存。 但是,分配的 memset
可以被流捕获。
13.4. Pointer Attributes
cuPointerGetAttributes
查询适用于流有序分配。 由于流排序分配与上下文无关,因此查询 CU_POINTER_ATTRIBUTE_CONTEXT
将成功,但在 *data
中返回 NULL
。 属性 CU_POINTER_ATTRIBUTE_DEVICE_ORDINAL
可用于确定分配的位置:这在选择使用 cudaMemcpyPeerAsync
制作 p2h2p
拷贝的上下文时很有用。 CU_POINTER_ATTRIBUTE_MEMPOOL_HANDLE
属性是在 CUDA 11.3 中添加的,可用于调试和在执行 IPC 之前确认分配来自哪个池。
更多精彩内容:
https://www.nvidia.cn/gtc-global/?ncid=ref-dev-876561
相关文章:
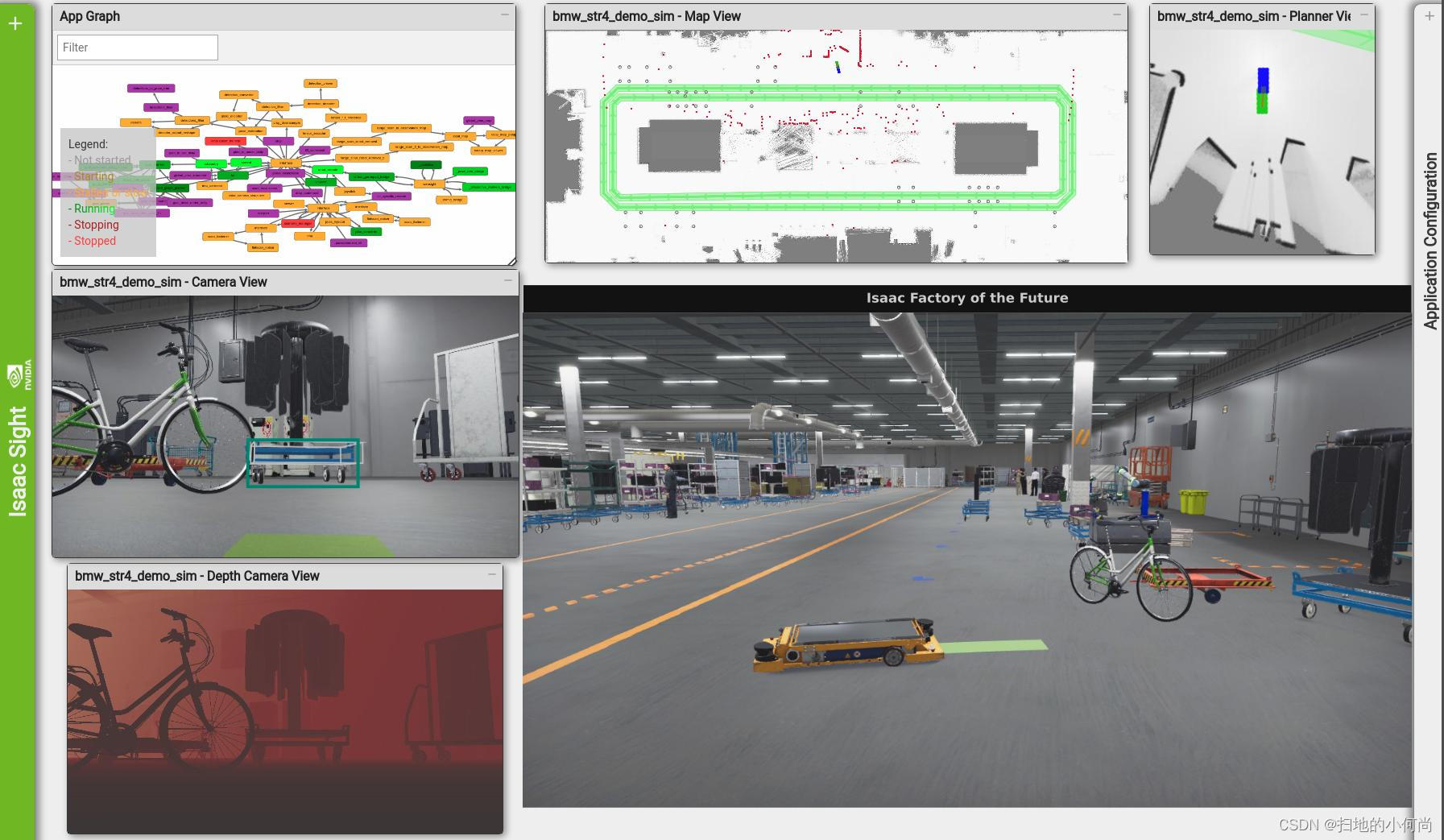
CUDA中的流序内存分配
文章目录CUDA中的流序内存分配1. Introduction2. Query for Support3. API Fundamentals (cudaMallocAsync and cudaFreeAsync)4. Memory Pools and the cudaMemPool_t注意:设备的内存池当前将是该设备的本地。因此,在不指定内存池的情况下进行分配将始终…...
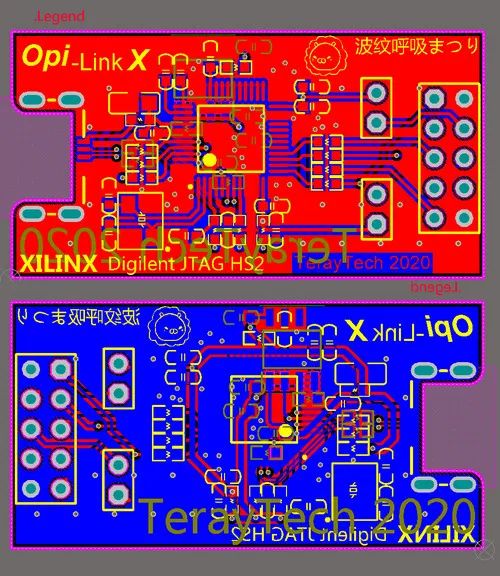
开源、低成本的 Xilinx FPGA 下载器(高速30MHz)
目前主流的Xilinx下载器主要有两种:一种是Xilinx官方出品的Xilinx Platfom Cable USB,还有一个就是Xilinx的合作伙伴Digilent开发的JTAG-HS3 Programming Cable。 JTAG-HS系列最大支持30MHz下载速度,基于FTDI的FT2232方案。 JTAG-HS系列对比…...
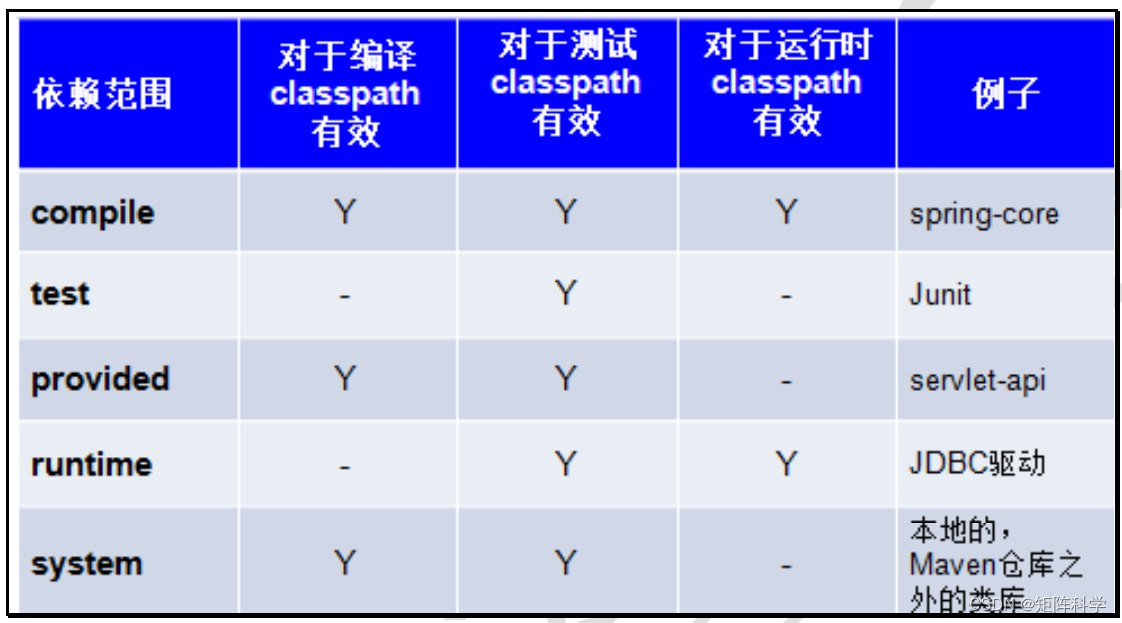
Maven专题总结
1. 什么是Maven Maven 是一个项目管理工具,它包含了一个项目对象模型 (POM: Project Object Model),一组标准集合,一个项目生命周期(Project Lifecycle),一个依赖管理系统(Dependency Management System),和…...
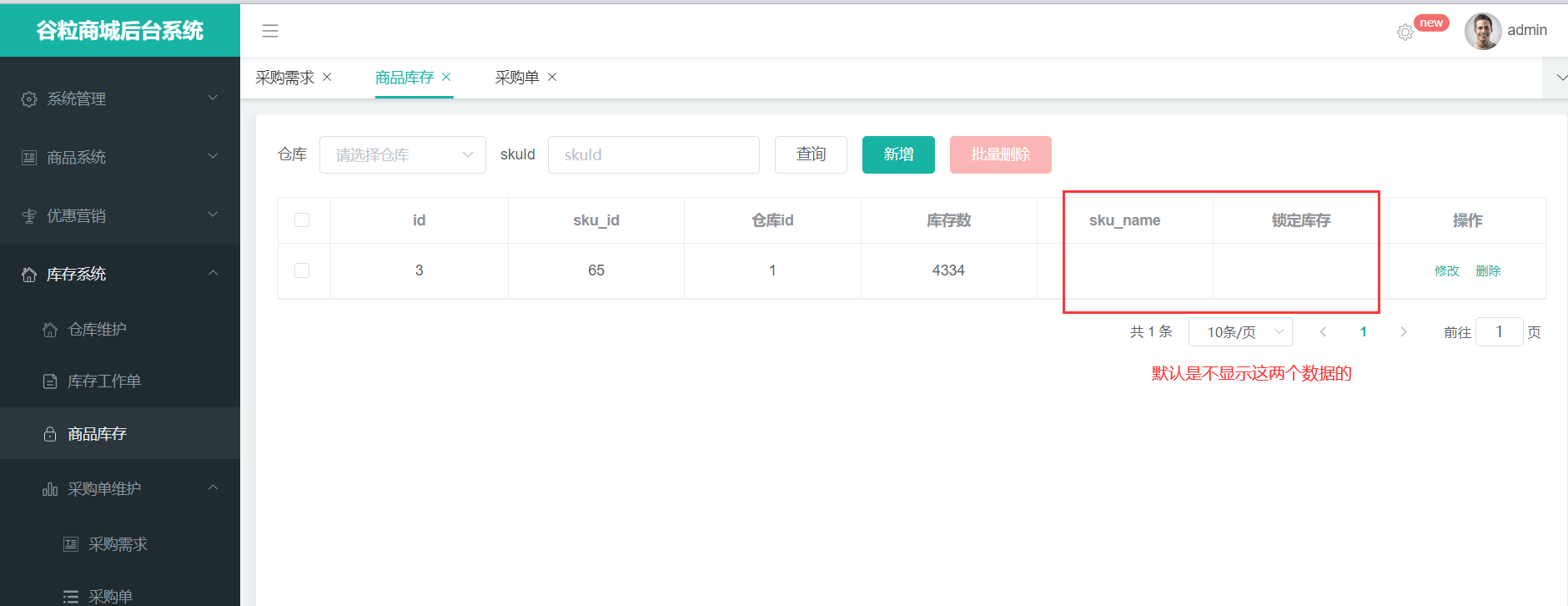
谷粒商城--SPU和SKU
目录 1.SPU和SKU概念 2.表的关系理解 3.导入前端代码 4.完善后端接口 5.属性分组详情 6.规格参数详情 7. 销售属性详情 8.分组与属性关联 9.发布商品 10.仓库服务 1.SPU和SKU概念 SPU:standard product unit(标准化产品单元):是商品信息聚合的…...
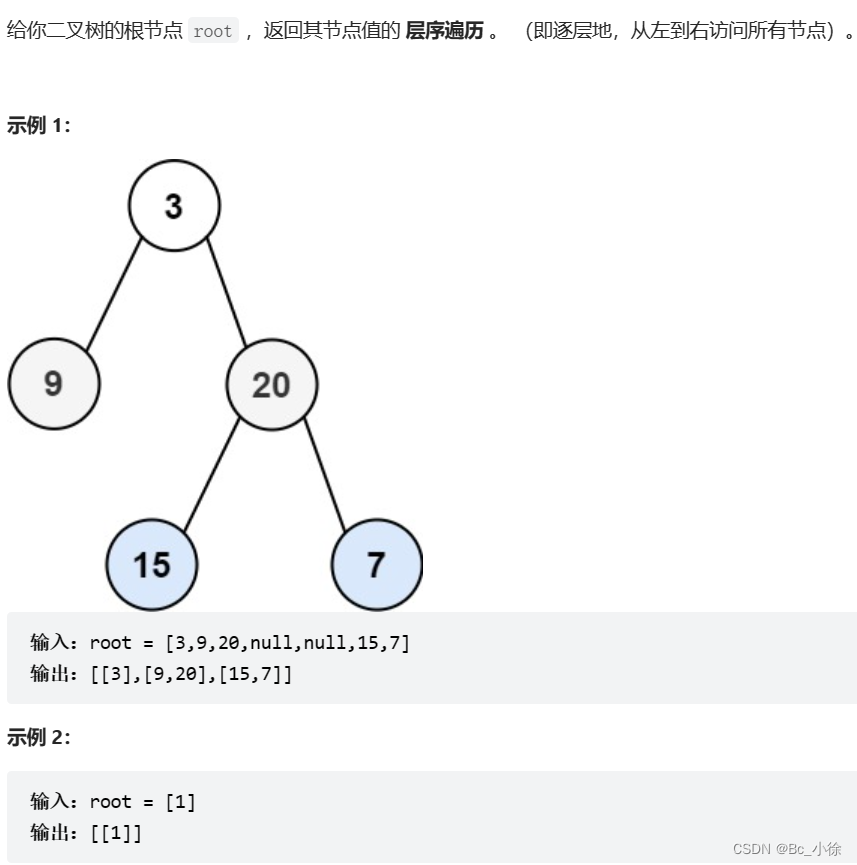
二叉树OJ题(上)
✅每日一练:100. 相同的树 - 力扣(LeetCode) 题目的意思是俩棵树的结构不仅要相同,而且每个节点的值还要相同,如果满足上面2个条件,则成立! 解题思路: 从三个方面去考虑࿱…...
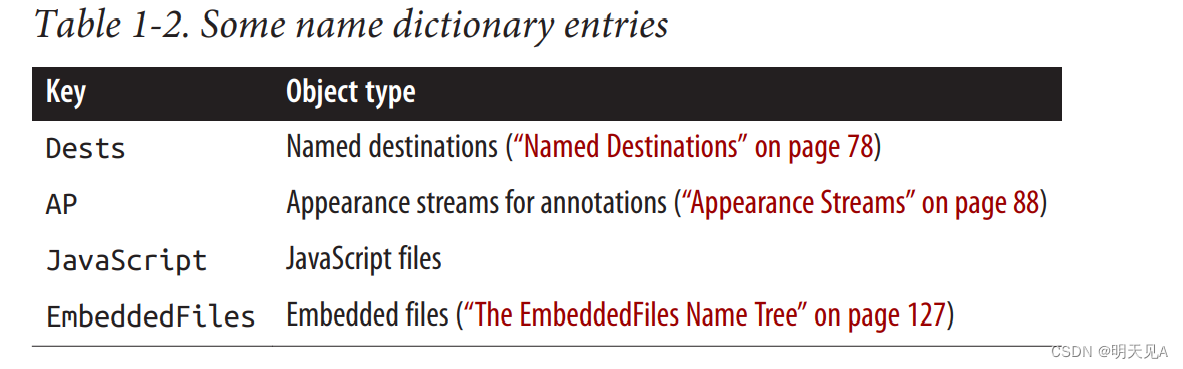
第一章 PDF语法
第一章 PDF语法PDF ObjectsNull ObjectsBoolean ObjectsNumeric ObjectsName ObjectsString ObjectsArray ObjectsDictionary ObjectsName treesNumber treesStream ObjectsDirect versus Indirect ObjectsFile StructureWhite-SpaceThe Four Sections of a PDFHeaderTrailerBo…...
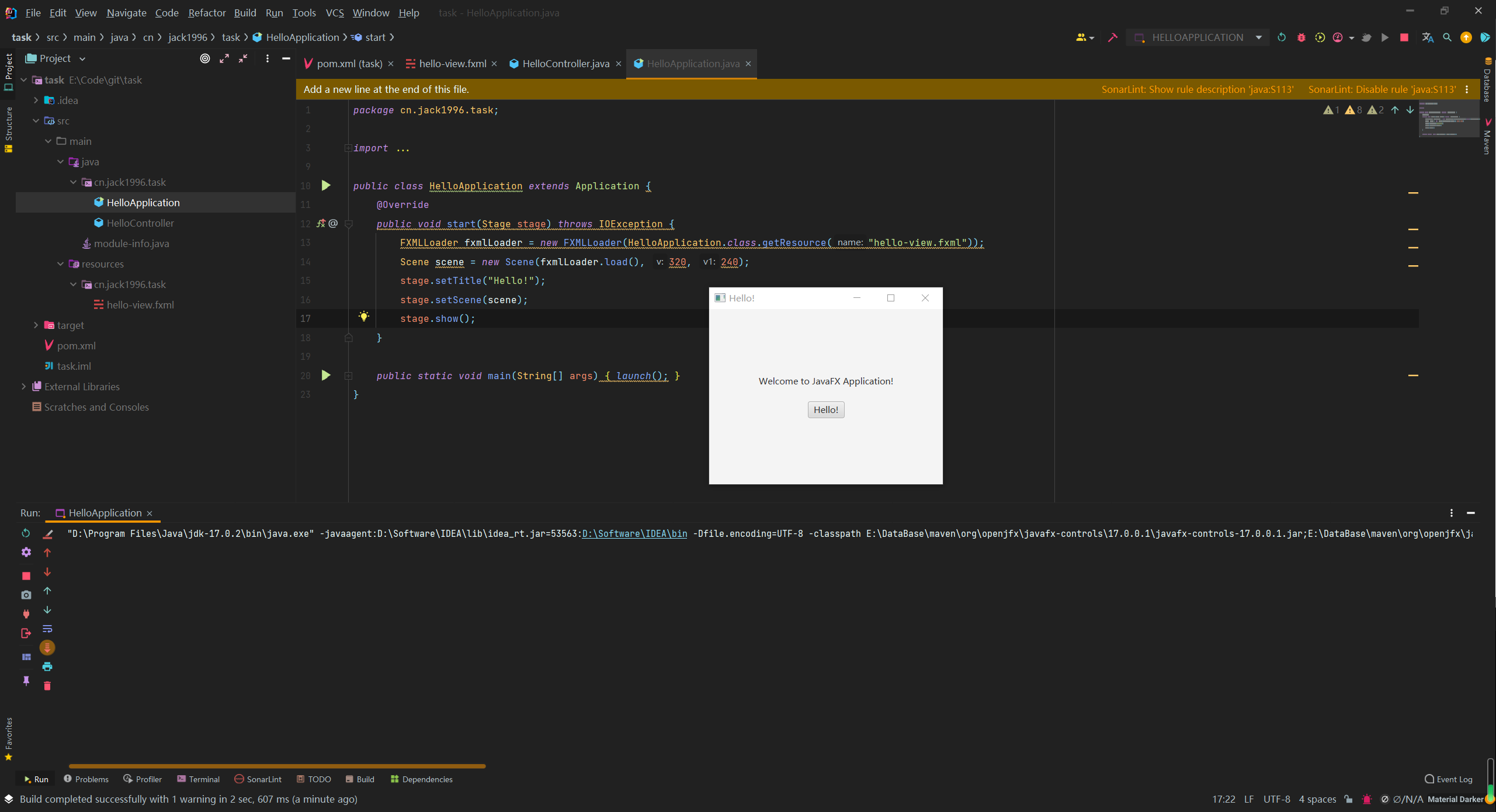
IntelliJ IDEA 创建JavaFX项目运行
IntelliJ IDEA 创建JavaFX项目运行JavaFX官网文档:https://openjfx.io/openjfx-docs/ JavaFX 2008年12月05日诞生,是一个开源的下一代客户端应用程序平台,适用于基于 Java 构建的桌面、移动和嵌入式系统。这是许多个人和公司的协作努力&#…...
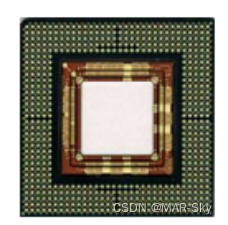
IC封装常见形式
参考:https://blog.csdn.net/dhs888888/article/details/127673300?utm_mediumdistribute.pc_relevant.none-task-blog-2defaultbaidujs_baidulandingword~default-0-127673300-blog-115610343.pc_relevant_multi_platform_whitelistv4&spm1001.2101.3001.4242…...

Linux通配符、转义符讲解
目录 通配符 通过通配符定义匹配条件 转义符 将所有的逻辑操作符都转换成字符 通配符 通过通配符定义匹配条件 * 任意字符都可以通配(也可以匹配空值) ? 匹配单个字符 [a-z] 匹配单个的小写英文字母 [A-Z] 匹配单个的大写英文…...

[OpenMMLab]提交pr时所需的git操作
git开发流程 准备工作 作为一个开发者,fork一个仓库之后应该先做什么? 1、下载仓库,创建上游代码库,查看当前的分支情况 git clone https://github.com/<your_name>/<repo_name>.git git remote add upstream git…...
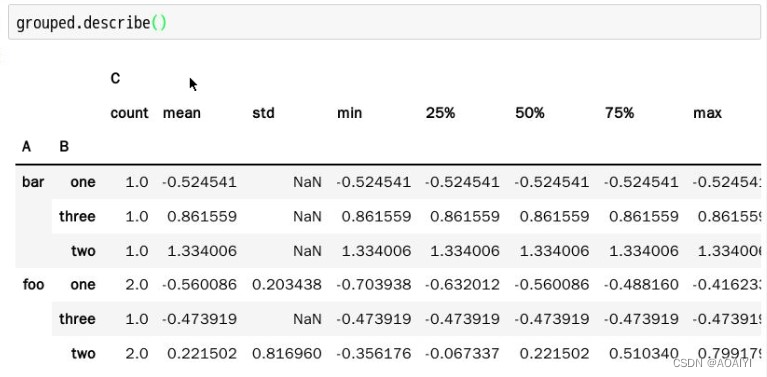
pandas——groupby操作
Pandas——groupby操作 文章目录Pandas——groupby操作一、实验目的二、实验原理三、实验环境四、实验内容五、实验步骤一、实验目的 熟练掌握pandas中的groupby操作 二、实验原理 groupby(byNone, axis0, levelNone, as_indexTrue, sortTrue, group_keysTrue, squeezeFalse&…...
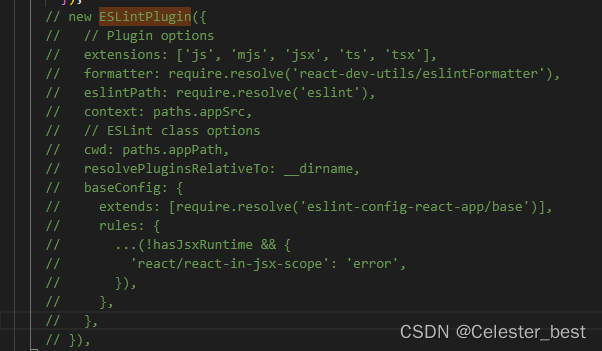
webpack.config.js哪里找?react项目关闭eslint监测
目录 webpack.config.js哪里找? react项目关闭eslint监测 webpack.config.js哪里找? 在React项目中,当我们需要修改一些配置时,发现找不到webpack.config.js,是我们创建的项目有问题吗,还需新创建项目的项…...
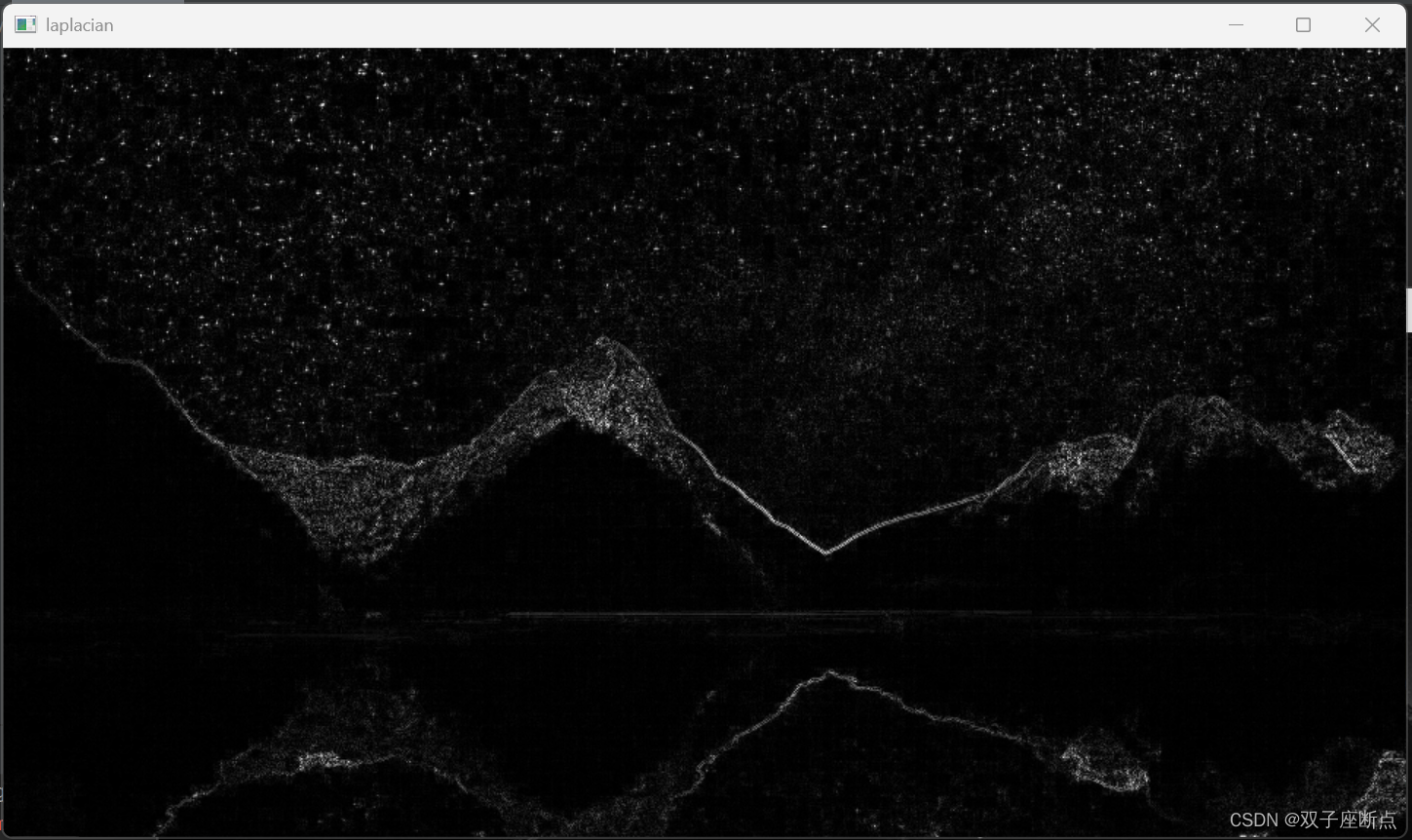
OpenCV 图像梯度算子
本文是OpenCV图像视觉入门之路的第12篇文章,本文详细的介绍了图像梯度算子的各种操作,例如:Sobel算子Scharr算子laplacian算子等操作。 OpenCV 图像梯度算子目录 1 Sobel算子 2 Scharr算子 3 laplacian算子 1 Sobel算子 Sobel算子是一种图…...
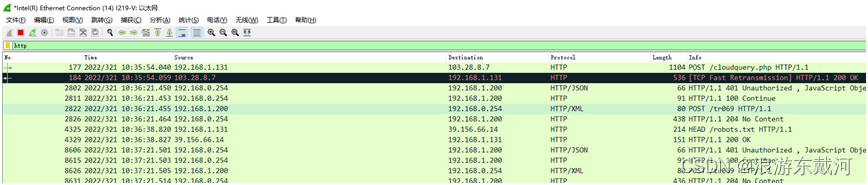
Linux c编程之Wireshark
Wireshark是一个网络报文分析软件,是网络应用问题分析必不可少的工具软件。网络管理员可以使用wireshark排查网络问题。程序开发人员可以用来分析应用协议、定位分析应用问题。无论是网络应用程序开发人员、测试人员、部署人员、技术支持人员,掌握wireshark的使用对于分析网络…...

极客时间_FlinkSQL 实战
一、批处理以及流处理技术发展 1.Lambda架构三层划分Batch Layer、Speed Layer和Serving Layer。 ①、Batch Layer:主要用于实现对历史数据计算结果的保存,每天计算的结果都保存成为一个Batch View,然后通过对Batch View的计算,实现历史数据的计算。 ②、Speed Layer正是用…...
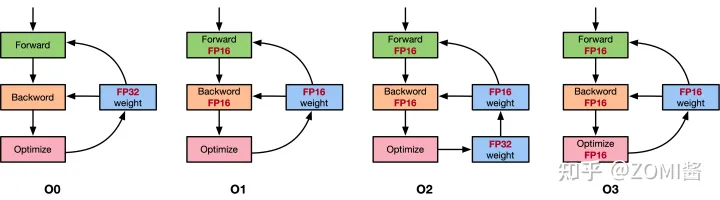
Pytorch 混合精度训练 (Automatically Mixed Precision, AMP)
Contents混合精度训练 (Mixed Precision Training)单精度浮点数 (FP32) 和半精度浮点数 (FP16)为什么要用 FP16为什么只用 FP16 会有问题解决方案损失缩放 (Loss Scaling)FP32 权重备份黑名单Tensor CoreNVIDIA apex 库代码解读opt-level (o1, o2, o3, o4)apex 的 o1 实现apex …...
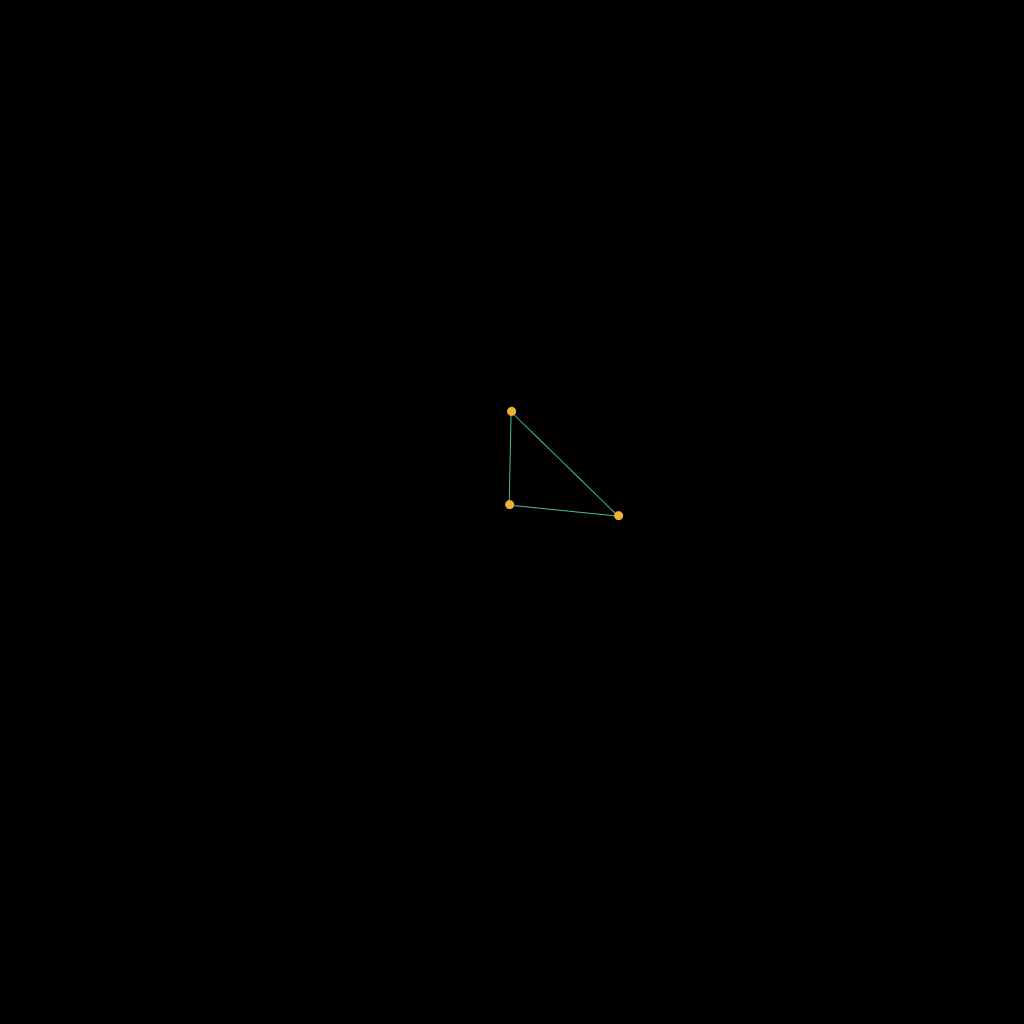
使用太极taichi写一个只有一个三角形的有限元
公式来源 https://blog.csdn.net/weixin_43940314/article/details/128935230 GAME103 https://games-cn.org/games103-slides/ 初始化我们的三角形 全局的坐标范围为0-1 我们的三角形如图所示 ti.kernel def init():X[0] [0.5, 0.5]X[1] [0.5, 0.6]X[2] [0.6, 0.5]x[0…...
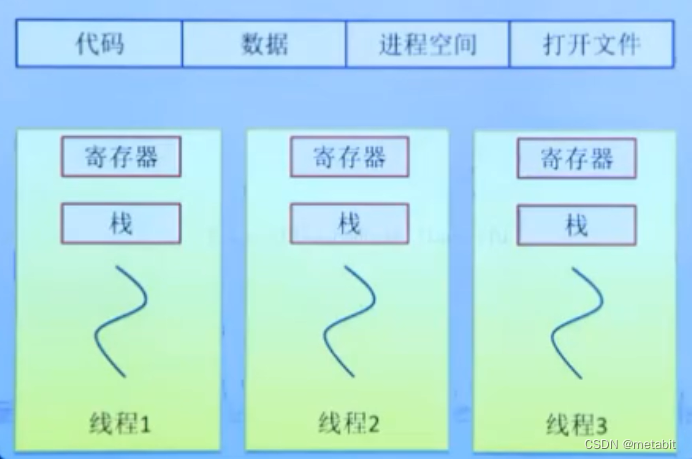
进程,线程
进程是操作系统分配资源的基本单位,线程是CPU调度的基本单位。 PCB:进程控制块,操作系统描述程序的运行状态,通过结构体task,struct{…},统称为PCB(process control block)。是进程管理和控制的…...

第03章_基本的SELECT语句
第03章_基本的SELECT语句 讲师:尚硅谷-宋红康(江湖人称:康师傅) 官网:http://www.atguigu.com 1. SQL概述 1.1 SQL背景知识 1946 年,世界上第一台电脑诞生,如今,借由这台电脑发展…...
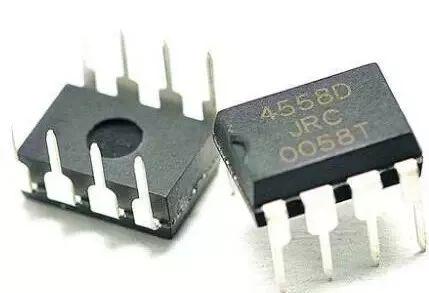
干货 | 简单了解运算放大器...
运算放大器发明至今已有数十年的历史,从最早的真空管演变为如今的集成电路,它在不同的电子产品中一直发挥着举足轻重的作用。而现如今信息家电、手机、PDA、网络等新兴应用的兴起更是将运算放大器推向了一个新的高度。01 运算放大器简述运算放大器&#…...
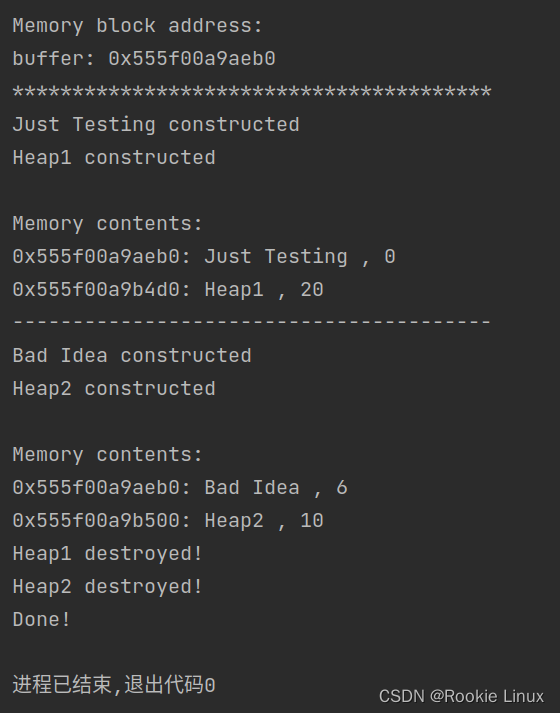
C++定位new用法及注意事项
使用定位new创建对象,显式调用析构函数是必须的,这是析构函数必须被显式调用的少数情形之一!, 另有一点!!!析构函数的调用必须与对象的构造顺序相反!切记!!&a…...

【Android笔记75】Android之翻页标签栏PagerTabStrip组件介绍及其使用
这篇文章,主要介绍Android之翻页标签栏PagerTabStrip组件及其使用。 目录 一、PagerTabStrip翻页标签栏 1.1、PagerTabStrip介绍 1.2、PagerTabStrip的使用 (1)创建布局文件...

【Kafka】【二】消息队列的流派
消息队列的流派 ⽬前消息队列的中间件选型有很多种: rabbitMQ:内部的可玩性(功能性)是⾮常强的rocketMQ: 阿⾥内部⼀个⼤神,根据kafka的内部执⾏原理,⼿写的⼀个消息队列中间 件。性能是与Kaf…...

现代 cmake (cmake 3.x) 操作大全
cmake 是一个跨平台编译工具,它面向各种平台提供适配的编译系统配置文件,进而调用这些编译系统完成编译工作。cmake 进入3.x 版本,指令大量更新,一些老的指令开始被新的指令集替代,并加入了一些更加高效的指令/参数。本…...
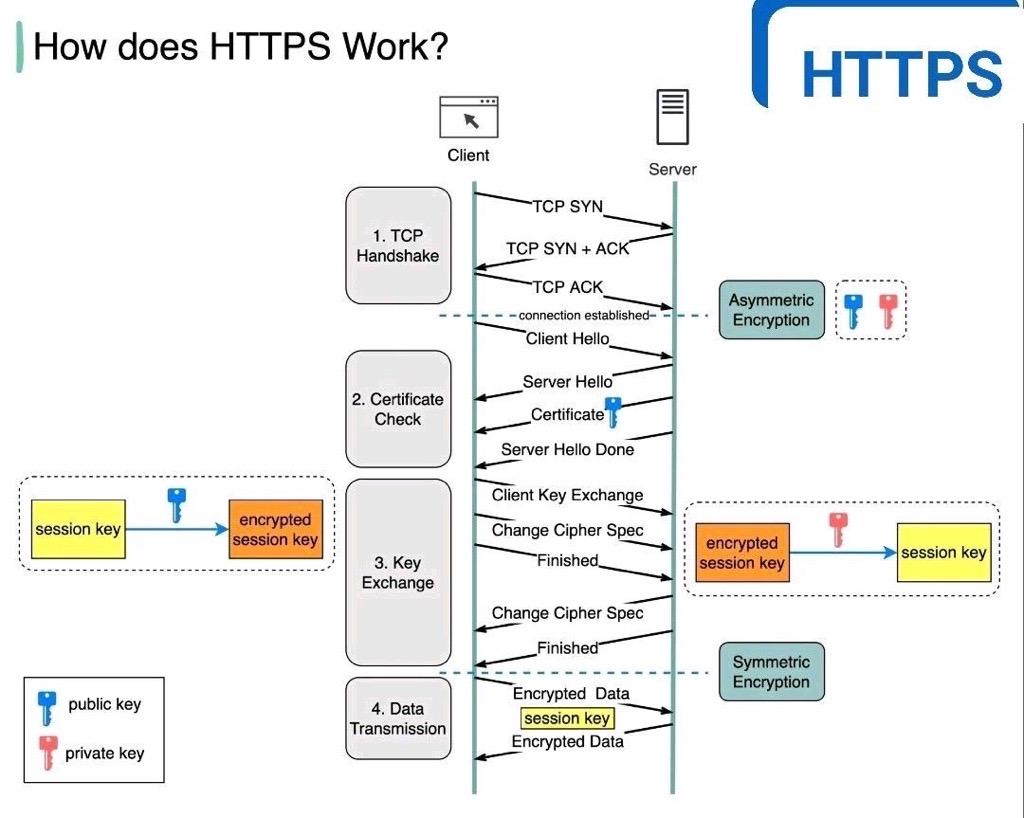
how https works?https工作原理
简单一句话: https http TLShttps 工作原理:HTTPS (Hypertext Transfer Protocol Secure)是一种带有安全性的通信协议,用于在互联网上传输信息。它通过使用加密来保护数据的隐私和完整性。下面是 HTTPS 的工作原理:初始化安全会…...

Docker的资源控制管理
目录 一、CPU控制 1、设置CPU使用率上限 2、设置CPU资源占用比(设置多个容器时才有效) 3、设置容器绑定指定的CPU 二、对内存使用进行限制 1、创建指定物理内存的容器 2、创建指定物理内存和swap的容器 3、 对磁盘IO配额控制(blkio&a…...

MMSeg无法使用单类自定义数据集训练
文章首发及后续更新:https://mwhls.top/4423.html,无图/无目录/格式错误/更多相关请至首发页查看。 新的更新内容请到mwhls.top查看。 欢迎提出任何疑问及批评,非常感谢! 摘要:将三通道图像转为一通道图像,…...
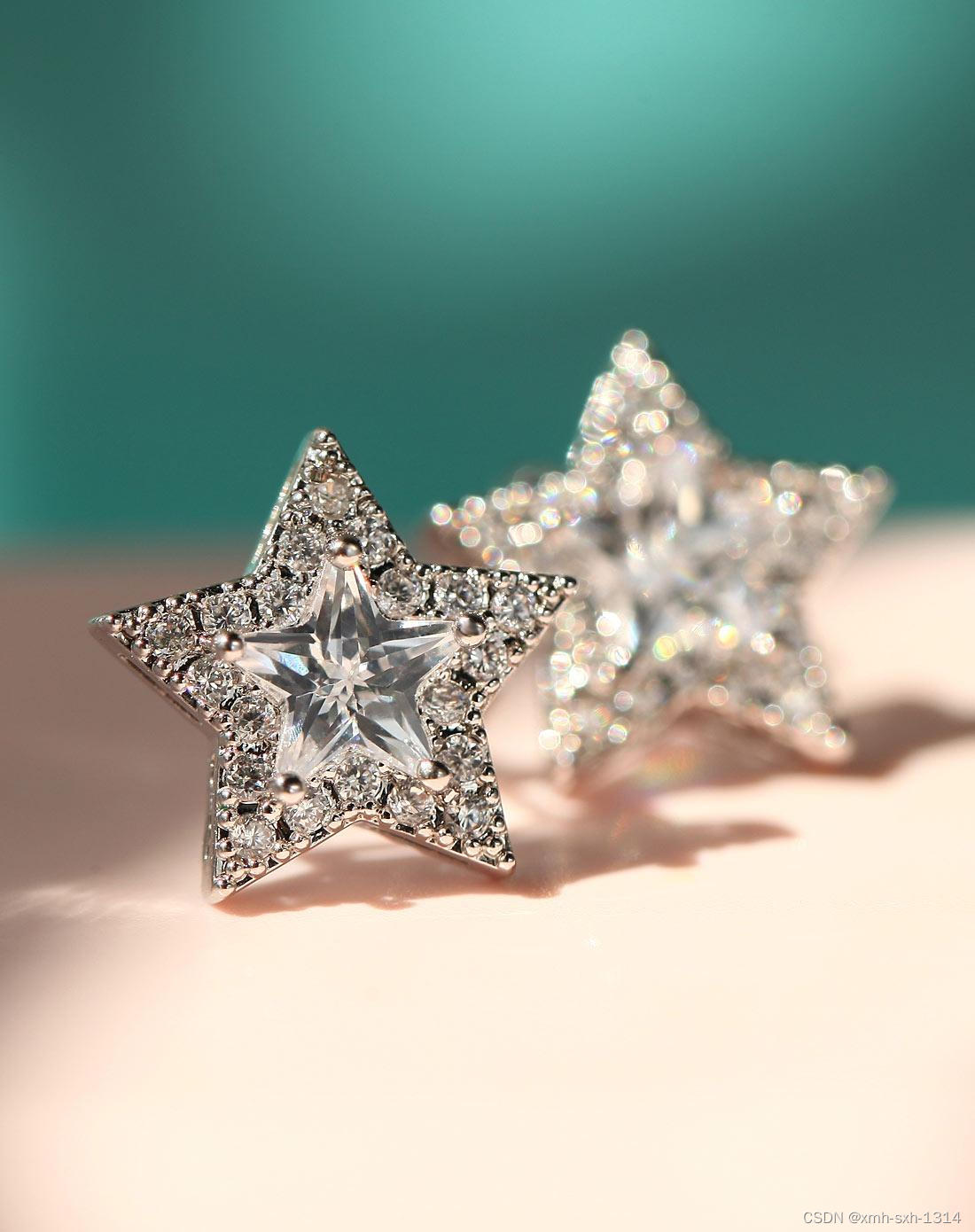
Redis使用方式
一、Redis基础部分: 1、redis介绍与安装比mysql快10倍以上 *****************redis适用场合**************** 1.取最新N个数据的操作 2.排行榜应用,取TOP N 操作 3.需要精确设定过期时间的应用 4.计数器应用 5.Uniq操作,获取某段时间所有数据排重值 6.实时系统,反垃圾系统7.P…...

无主之地3重型武器节奏评分榜(9.25) 枪械名 红字效果 元素属性 清图评分 Boss战评分 泛用性评分 特殊性评分 最终评级 掉落点 掉率 图片 瘟疫传播
无主之地3重型武器节奏评分榜(9.25) 枪械名 红字效果 元素属性 清图评分 Boss战评分 泛用性评分 特殊性评分 最终评级 掉落点 掉率 图片 瘟疫传播者 发射巨大能量球,能量球会额外生成追踪附近敌人的伴生弹 全属性 SSS SSS SSS - T0 伊甸6号-…...

什么是编程什么是算法
1.绪论 编程应在一个开发环境中完成源程序的编译和运行。首先,发现高级语言开发环境,TC,Windows系统的C++,R语言更适合数学专业的学生。然后学习掌握编程的方法,在学校学习,有时间的人可以在网上学习,或者购买教材自学。最后,编写源程序,并且在开发环境中实践。 例如…...

凡客诚品是什么/seo网站优化平台
试验网站#1搜索引擎优化收录情况记录日期Yahoogooglebaidusogou每日收录每日收录增量每日收录每日收录增量每日收录每日收录增量每日收录每日收录增量2007-6-24288 333 1060 4813 2007-6-25164013523330108020481302007-6-26空间超过本月流量限制……,快到月底了…...

昆明哪些做网站建设的公司/windows优化大师免费版
本节书摘来自异步社区《JavaScript核心概念及实践》一书中的第2章,第2.2节,作者:邱俊涛著,更多章节内容可以访问云栖社区“异步社区”公众号查看 2.2 变量 变量,是对值的存储空间的引用,通过一个名字将一个…...

专做国际时事评论网站/视频专用客户端app
Connection is not associated with a managed connection...
安徽地方政府网站建设情况/漳州seo建站
大数据是一项涉及不同业务和技术领域的技术和工具的集合,海量离线数据分析可以应用于多种商业系统环境,例如,电商海量日志分析、用户行为画像分析、科研行业的海量离线计算分析任务等场景。离线大数据分析概述主流的三大分布式计算框架系统分…...

网上推广招聘/快速seo关键词优化方案
TEST...
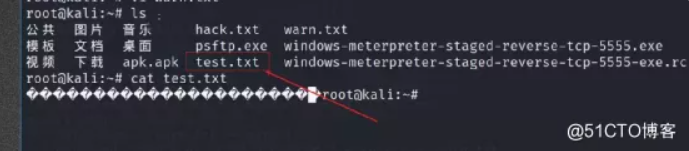
日记类型 wordpress/一键优化清理加速
一、背景介绍 在我们日常使用Kali Linux时,我们通常在进行安全演练的时候,当我们拿下Windows靶机(例如利用永恒之蓝拿下Win7主机)后在命令行模式下如何进行文件下载以及文件上传呢?如何解决上述问题呢?接下…...