密云网站开发/优化营商环境存在问题及整改措施
下面是 用 TripletLoss 优化bert ranking 的demo
import torch
from torch.utils.data import DataLoader, Dataset
from transformers import BertModel, BertTokenizer
from sklearn.metrics.pairwise import pairwise_distancesclass TripletRankingDataset(Dataset):def __init__(self, queries, positive_docs, negative_docs, tokenizer, max_length):self.input_ids_q = []self.attention_masks_q = []self.input_ids_p = []self.attention_masks_p = []self.input_ids_n = []self.attention_masks_n = []for query, pos_doc, neg_doc in zip(queries, positive_docs, negative_docs):encoded_query = tokenizer.encode_plus(query, padding='max_length', truncation=True, max_length=max_length, return_tensors='pt')encoded_pos_doc = tokenizer.encode_plus(pos_doc, padding='max_length', truncation=True, max_length=max_length, return_tensors='pt')encoded_neg_doc = tokenizer.encode_plus(neg_doc, padding='max_length', truncation=True, max_length=max_length, return_tensors='pt')self.input_ids_q.append(encoded_query['input_ids'])self.attention_masks_q.append(encoded_query['attention_mask'])self.input_ids_p.append(encoded_pos_doc['input_ids'])self.attention_masks_p.append(encoded_pos_doc['attention_mask'])self.input_ids_n.append(encoded_neg_doc['input_ids'])self.attention_masks_n.append(encoded_neg_doc['attention_mask'])self.input_ids_q = torch.cat(self.input_ids_q, dim=0)self.attention_masks_q = torch.cat(self.attention_masks_q, dim=0)self.input_ids_p = torch.cat(self.input_ids_p, dim=0)self.attention_masks_p = torch.cat(self.attention_masks_p, dim=0)self.input_ids_n = torch.cat(self.input_ids_n, dim=0)self.attention_masks_n = torch.cat(self.attention_masks_n, dim=0)def __len__(self):return len(self.input_ids_q)def __getitem__(self, idx):input_ids_q = self.input_ids_q[idx]attention_mask_q = self.attention_masks_q[idx]input_ids_p = self.input_ids_p[idx]attention_mask_p = self.attention_masks_p[idx]input_ids_n = self.input_ids_n[idx]attention_mask_n = self.attention_masks_n[idx]return input_ids_q, attention_mask_q, input_ids_p, attention_mask_p, input_ids_n, attention_mask_nclass BERTTripletRankingModel(torch.nn.Module):def __init__(self, bert_model_name, hidden_size):super(BERTTripletRankingModel, self).__init__()self.bert = BertModel.from_pretrained(bert_model_name)self.dropout = torch.nn.Dropout(0.1)self.fc = torch.nn.Linear(hidden_size, 1)def forward(self, input_ids, attention_mask):outputs = self.bert(input_ids=input_ids, attention_mask=attention_mask)pooled_output = self.dropout(outputs[1])logits = self.fc(pooled_output)return logits.squeeze()def triplet_loss(anchor, positive, negative, margin):distance_positive = torch.nn.functional.pairwise_distance(anchor, positive)distance_negative = torch.nn.functional.pairwise_distance(anchor, negative)losses = torch.relu(distance_positive - distance_negative + margin)return torch.mean(losses)# 初始化BERT模型和分词器
bert_model_name = 'bert-base-uncased'
tokenizer = BertTokenizer.from_pretrained(bert_model_name)# 示例输入数据
queries = ['I like cats', 'The sun is shining']
positive_docs = ['I like dogs', 'The weather is beautiful']
negative_docs = ['Snakes are dangerous', 'It is raining']# 超参数
batch_size = 8
max_length = 128
learning_rate = 1e-5
num_epochs = 5
margin = 1.0# 创建数据集和数据加载器
dataset = TripletRankingDataset(queries, positive_docs, negative_docs, tokenizer, max_length)
dataloader = DataLoader(dataset, batch_size=batch_size, shuffle=True)# 初始化模型并加载预训练权重
model = BERTTripletRankingModel(bert_model_name, hidden_size=model.bert.config.hidden_size)
optimizer = torch.optim.AdamW(model.parameters(), lr=learning_rate)# 训练模型
model.train()for epoch in range(num_epochs):total_loss = 0for input_ids_q, attention_masks_q, input_ids_p, attention_masks_p, input_ids_n, attention_masks_n in dataloader:optimizer.zero_grad()embeddings_q = model(inputids_q, attention_masks_q)embeddings_p = model(input_ids_p, attention_masks_p)embeddings_n = model(input_ids_n, attention_masks_n)loss = triplet_loss(embeddings_q, embeddings_p, embeddings_n, margin)total_loss += loss.item()loss.backward()optimizer.step()print(f"Epoch {epoch+1}/{num_epochs} - Loss: {total_loss:.4f}")# 推断模型
model.eval()with torch.no_grad():embeddings = model.bert.embeddings.word_embeddings(dataset.input_ids_q)pairwise_distances = pairwise_distances(embeddings.numpy())# 输出结果
for i, query in enumerate(queries):print(f"Query: {query}")print("Documents:")for j, doc in enumerate(positive_docs):doc_idx = pairwise_distances[0][i * len(positive_docs) + j]doc_dist = pairwise_distances[1][i * len(positive_docs) + j]print(f"Document index: {doc_idx}, Distance: {doc_dist:.4f}")print(f"Document: {doc}")print("")print("---------")
相关文章:

用 TripletLoss 优化bert ranking
下面是 用 TripletLoss 优化bert ranking 的demo import torch from torch.utils.data import DataLoader, Dataset from transformers import BertModel, BertTokenizer from sklearn.metrics.pairwise import pairwise_distancesclass TripletRankingDataset(Dataset):def __…...

Tomcat安装及使用
这里写目录标题 Tomcat一.java基础1.java历史2.java组成3.实现动态网页功能serveltjsp 4.jdkJDK 和 JRE 关系安装openjdk安装oracle官方JDK 二.tomcat基础功能1.Tomcat介绍2.安装tomcat二进制安装Tomcat 3.配置文件介绍及核心组件配置文件组件 4.状态页5.常见的配置详解6.tomca…...

法国新法案强迫 Firefox 等浏览器审查网站
导读Mozilla 基金会已发起了一份请愿书,旨在阻止法国政府强迫 Mozilla Firefox 等浏览器审查网站。 据悉,法国政府正在制定一项旨在打击网络欺诈的 SREN 法案 (“Projet de loi Visant scuriser et reguler lespace numrique”),包含大约 2…...
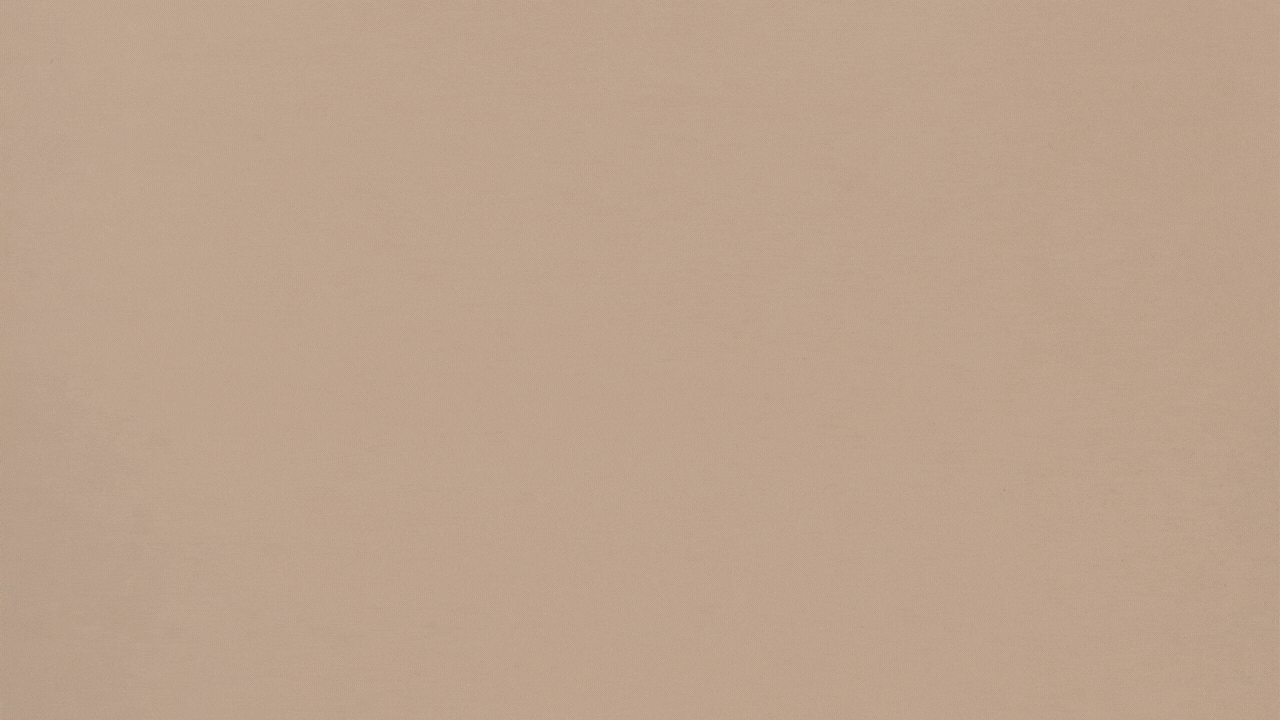
开源电商项目 Mall:构建高效电商系统的终极选择
文章目录 Mall 项目概览前台商城系统后台管理系统系统架构图业务架构图 模块介绍后台管理系统 mall-admin商品管理:功能结构图-商品订单管理:功能结构图-订单促销管理:功能结构图-促销内容管理:功能结构图-内容用户管理࿱…...
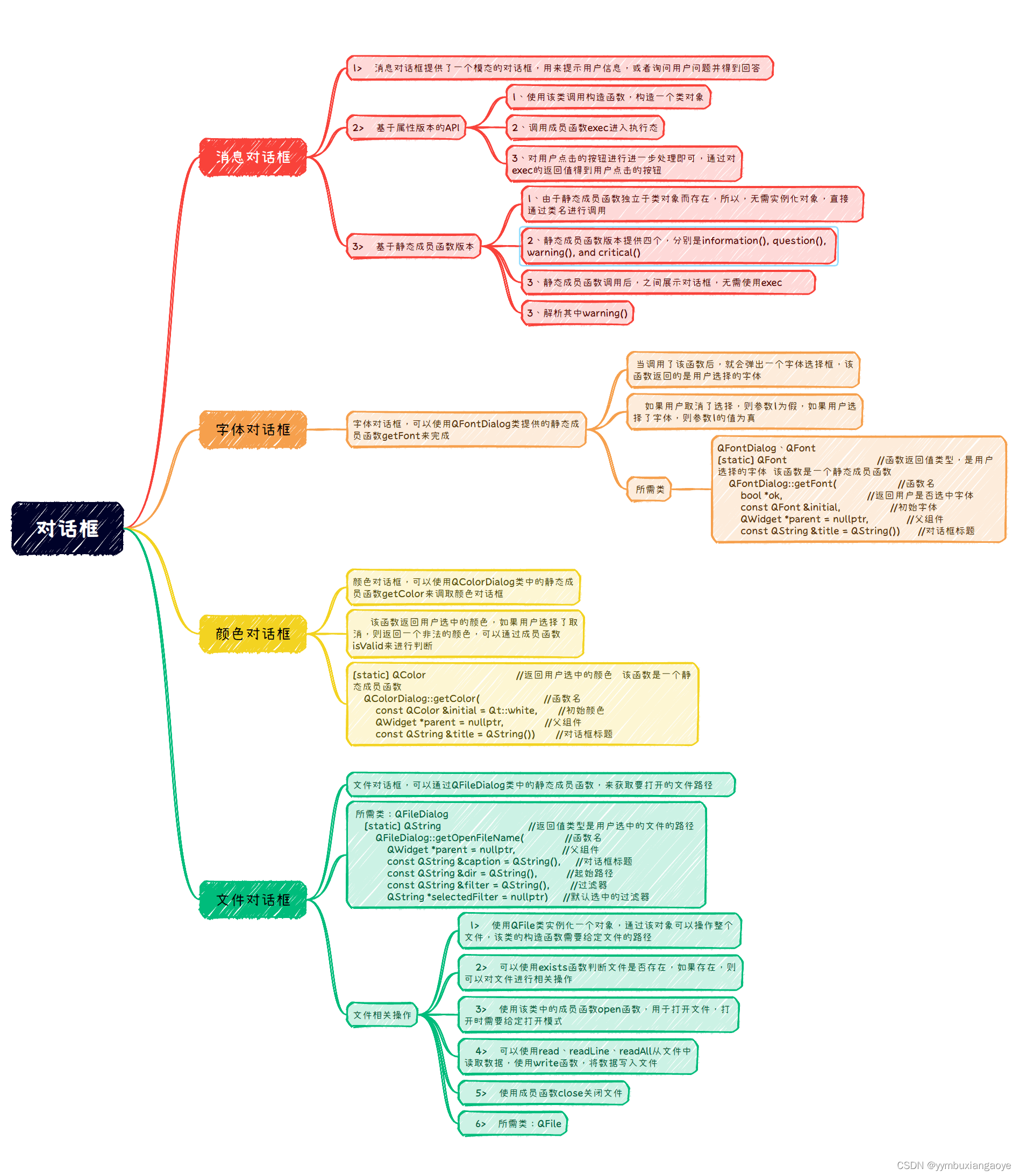
QT(9.1)对话框与事件处理
作业: 1. 完善登录框 点击登录按钮后,判断账号(admin)和密码(123456)是否一致,如果匹配失败,则弹出错误对话框,文本内容“账号密码不匹配,是否重新登录”&…...

C++项目实战——基于多设计模式下的同步异步日志系统-③-前置知识补充-设计模式
文章目录 专栏导读六大原则单例模式饿汉模式懒汉模式 工厂模式简单工厂模式工厂方法模式抽象工厂模式 建造者模式代理模式 专栏导读 🌸作者简介:花想云 ,在读本科生一枚,C/C领域新星创作者,新星计划导师,阿…...

C++ 新旧版本两种读写锁
一、简介 读写锁(Read-Write Lock)是一种并发控制机制,用于多线程环境中实现对共享资源的高效读写操作。读写锁允许多个线程同时读取共享资源,但在有写操作时,需要互斥地独占对共享资源的访问,以确保数据的…...

ES6 字符串的repeat()方法
repeat() 方法返回一个新字符串,表示将原字符串重复n次 格式:str.repeat(n) 参数n:str需要重复多少次 参数n的取值: n是正整数: x.repeat(3) // 输出结果:"xxx" hello.repeat(2) // 输出结果…...

【车载以太网测试从入门到精通】系列文章目录汇总
【车载以太网测试从入门到精通】——物理层测试 【车载以太网测试从入门到精通】——数据链路层测试 【车载以太网测试从入门到精通】——网络层测试 【车载以太网测试从入门到精通】——传输层测试 【车载以太网测试从入门到精通】——以太网TCP/IP协议自动化测试(…...
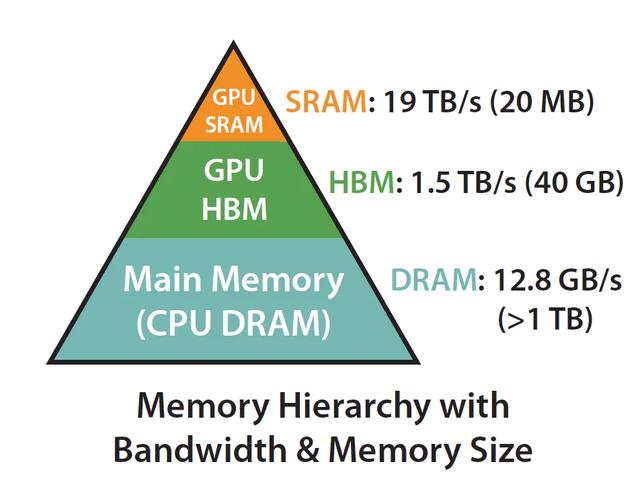
LLM推理优化技术综述:KVCache、PageAttention、FlashAttention、MQA、GQA
LLM推理优化技术综述:KVCache、PageAttention、FlashAttention、MQA、GQA 随着大模型被越来越多的应用到不同的领域,随之而来的问题是应用过程中的推理优化问题,针对LLM推理性能优化有一些新的方向,最近一直在学习和研究…...
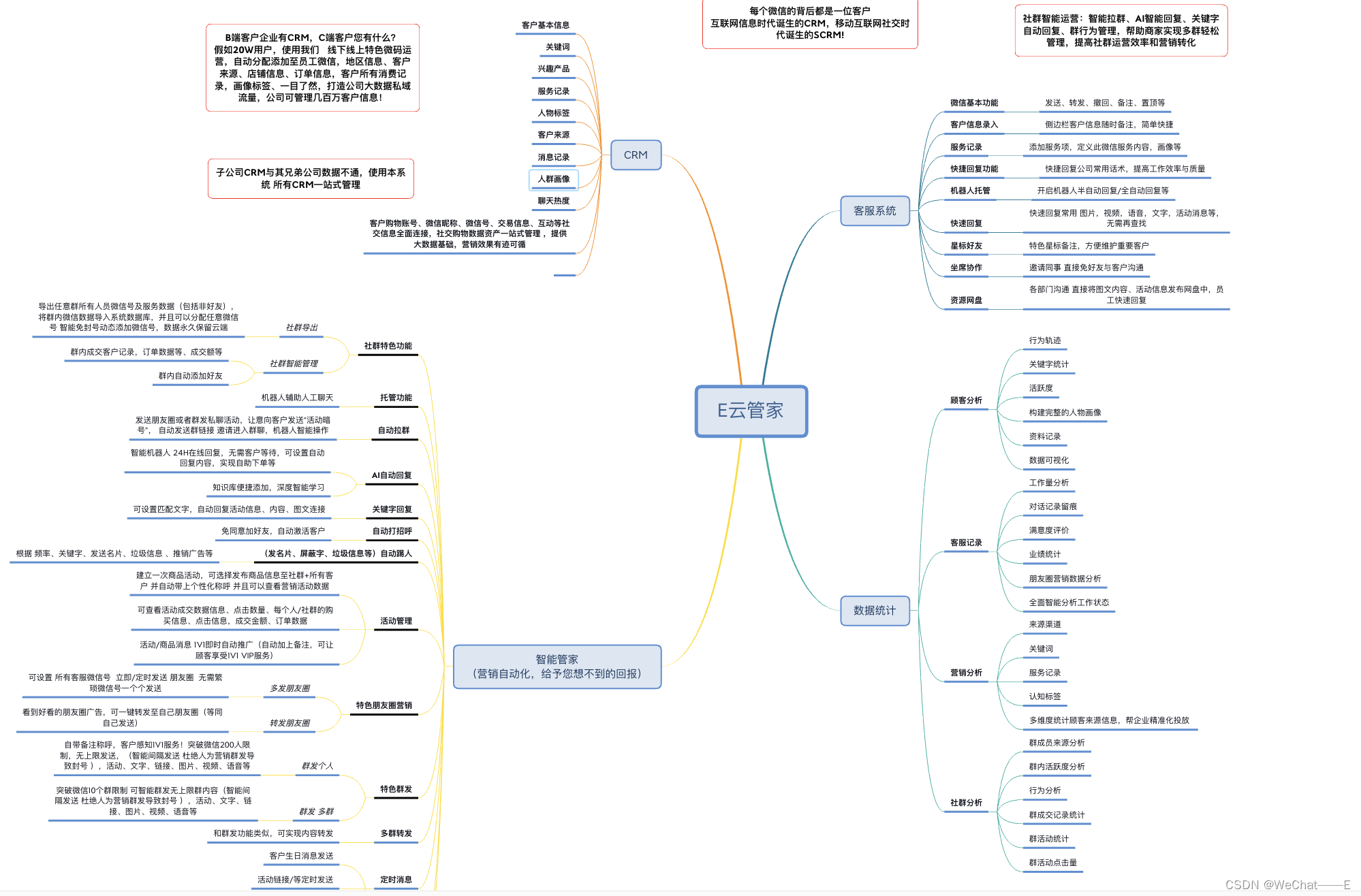
go开发之个微机器人的二次开发
请求URL: http://域名/addRoomMemberFriend 请求方式: POST 请求头Headers: Content-Type:application/jsonAuthorization:login接口返回 参数: 参数名必选类型说明wId是String登录实例标识chatRoom…...

2023国赛数学建模B题思路代码 - 多波束测线问题
# 1 赛题 B 题 多波束测线问题 单波束测深是利用声波在水中的传播特性来测量水体深度的技术。声波在均匀介质中作匀 速直线传播, 在不同界面上产生反射, 利用这一原理,从测量船换能器垂直向海底发射声波信 号,并记录从声波发射到…...
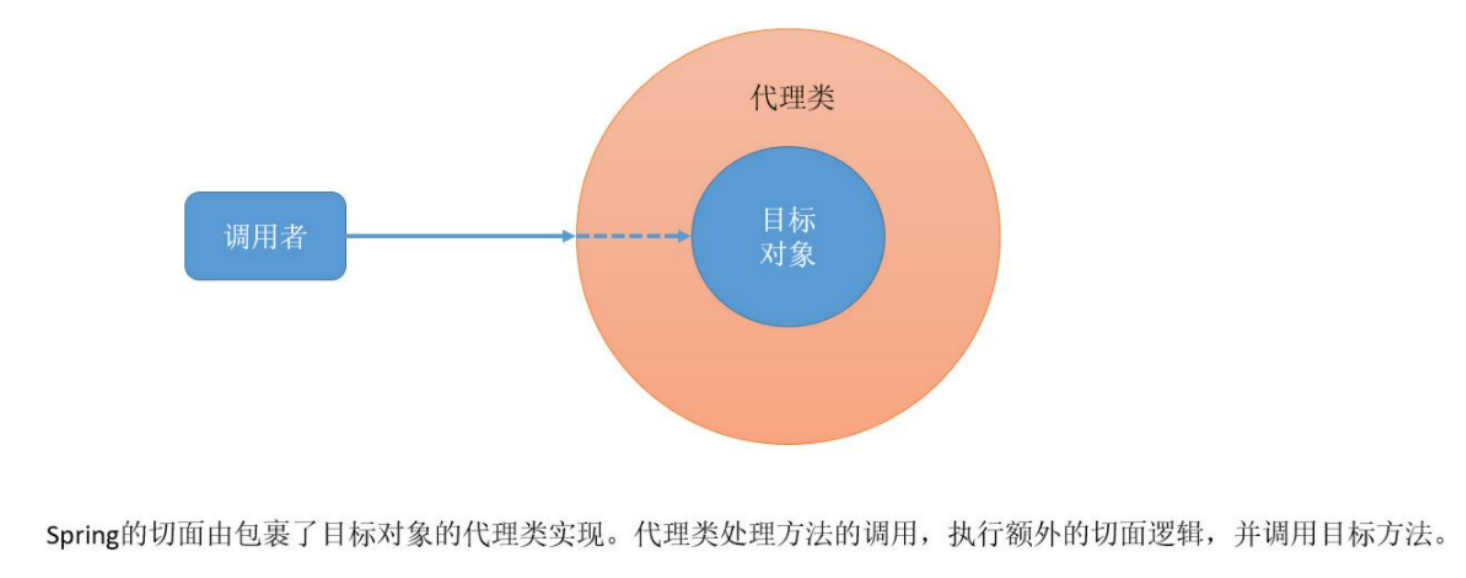
SpringAOP面向切面编程
文章目录 一. AOP是什么?二. AOP相关概念三. SpringAOP的简单演示四. SpringAOP实现原理 一. AOP是什么? AOP(Aspect Oriented Programming):面向切面编程,它是一种编程思想,是对某一类事情的集…...

A Guide to Java HashMap
原文链接: A Guide to Java HashMap → https://www.baeldung.com/java-hashmap 从Map里取值 # 原生方法 Map<String, Integer> map new HashMap<>();// map自身的方法 → 取不到返回null Integer age6 map.get("name"); // Integer时返回null可…...

LeetCode 449. Serialize and Deserialize BST【树,BFS,DFS,栈】困难
本文属于「征服LeetCode」系列文章之一,这一系列正式开始于2021/08/12。由于LeetCode上部分题目有锁,本系列将至少持续到刷完所有无锁题之日为止;由于LeetCode还在不断地创建新题,本系列的终止日期可能是永远。在这一系列刷题文章…...

嵌入式IDE(1):IAR中ICF链接文件详解和实例分析
最近在使用NXP的提供的MCUXPresso IDE,除了Eclipse固有的优点外,我觉得它最大的优点就是在链接脚本的生成上,提供了非常直观的GUI配置界面。但这个IDE仅仅支持NXP相关的产品,而且调试的性能在某些情况下并不理想。而我们用得比较多…...
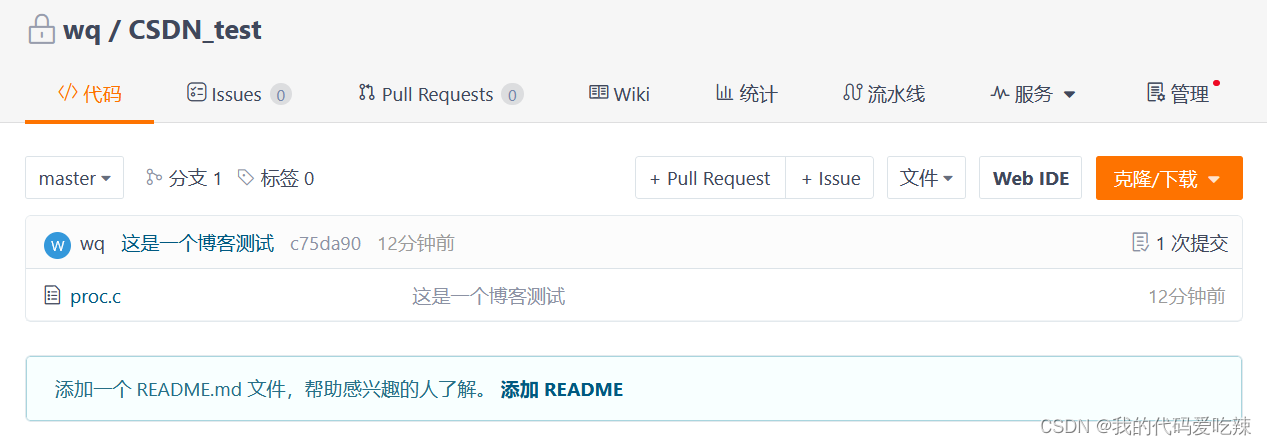
分布式版本控制工具——git
✅<1>主页::我的代码爱吃辣 📃<2>知识讲解:Linux——git ☂️<3>开发环境:Centos7 💬<4>前言:git是一个开源的分布式版本控制系统,可以有效、高速地处理从很…...
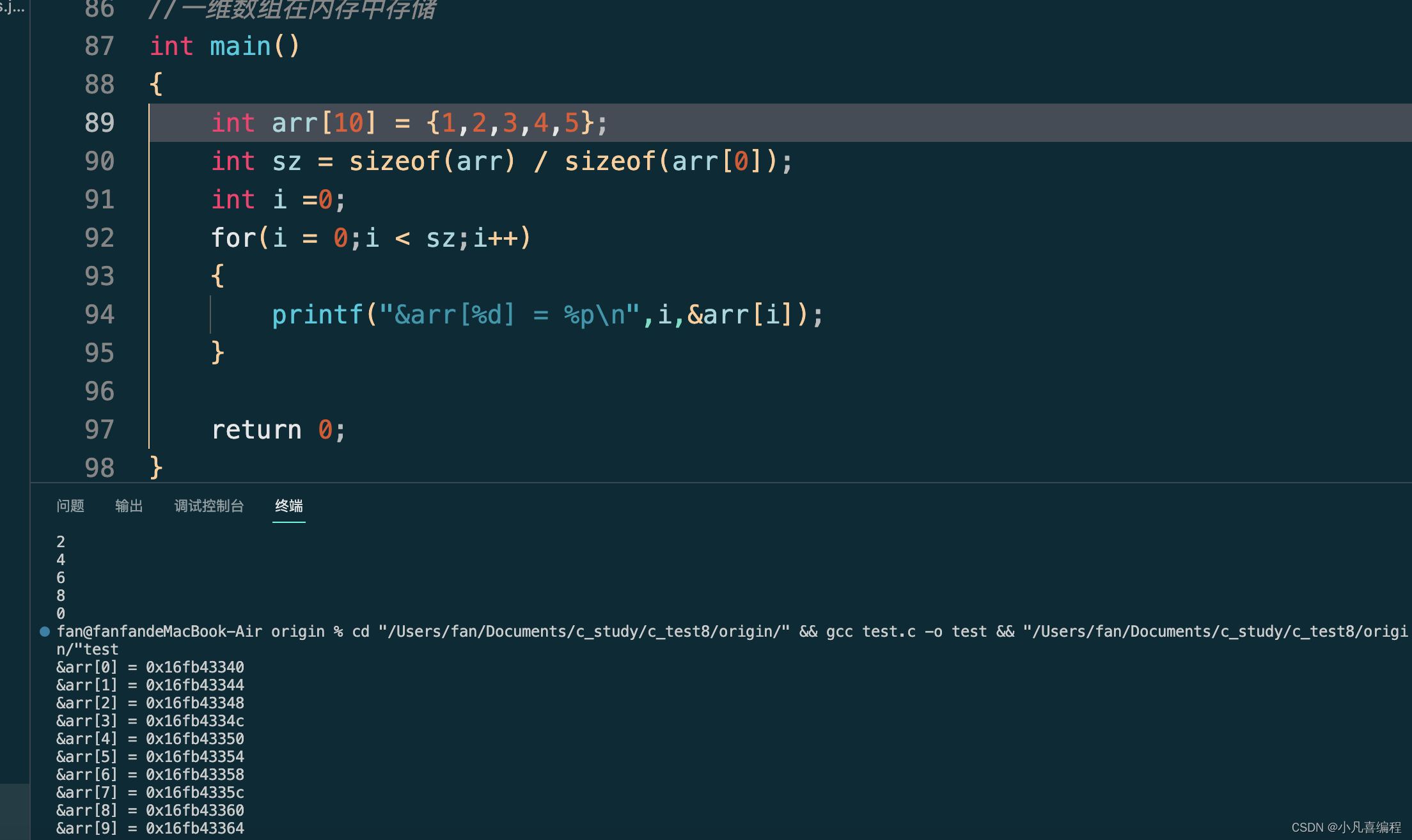
C基础-数组
1.一维数组的创建和初始化 int main() {// int arr1[10];int n 0;scanf("%d",&n);//int count 10;int arr2[n]; //局部的变量,这些局部的变量或者数组是存放在栈区的,存放在栈区上的数组,如果不初始化的话,默认…...

springboot项目配置flyway菜鸟级别教程
1、Flyway的工作原理 Flyway在第一次执行时,会创建一个默认名为flyway_schema_history的历史记录表,这张表会用来跟踪或记录数据库的状态,然后每次项目启动时都会自动扫描在resources/db/migration下的文件的版本号并且通过查询flyway_schem…...

成都精灵云初试
最近参加了成都精灵云的笔试与面试,岗位是c工程师。后面自己复盘了过程,初试部分总结如下,希望能对各位相进该公司以及面试C工程师的同学提供一些参考。这也是博主第一次参加面试,很多东西都还没准备,很多答得不好&…...

css relative 和absolute布局
1、relative和absolute内部的元素都是相对于父容器,若父容器没有指定为relative,则默认为整个文档视图空间,absolute可以重叠元素,relative则不行。relative意味着元素的任意属性如left和right都是相对于其他元素的。absolute则相…...
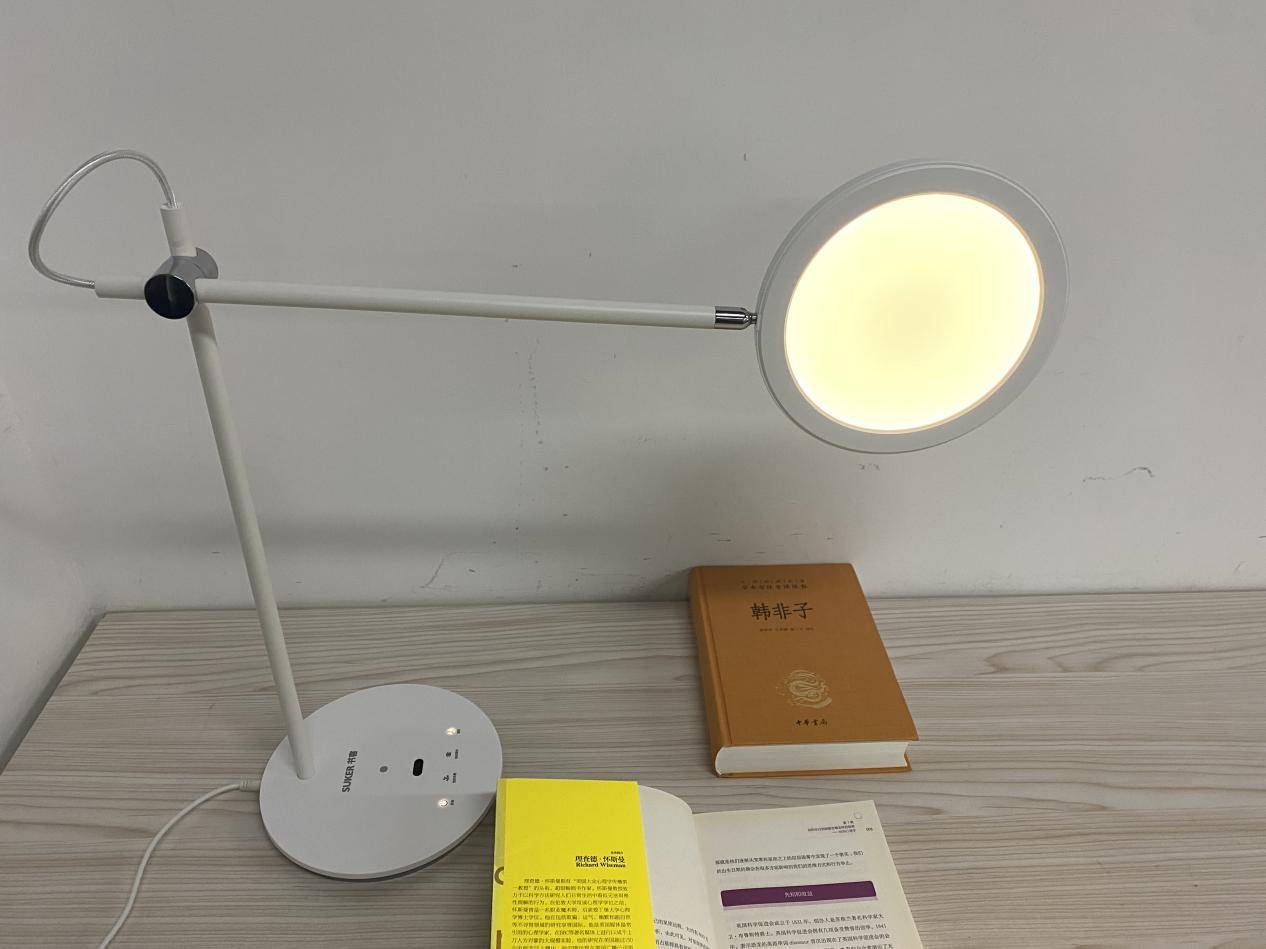
更健康舒适更科技的照明体验!书客SKY护眼台灯SUKER L1上手体验
低价又好用的护眼台灯是多数人的需求,很多人只追求功能性护眼台灯,显色高、无频闪、无蓝光等基础需求。但是在较低价格中很难面面俱到,然而刚发布的SUKER书客L1护眼台灯却是一款不可多得的性价比护眼台灯,拥有高品质光源ÿ…...
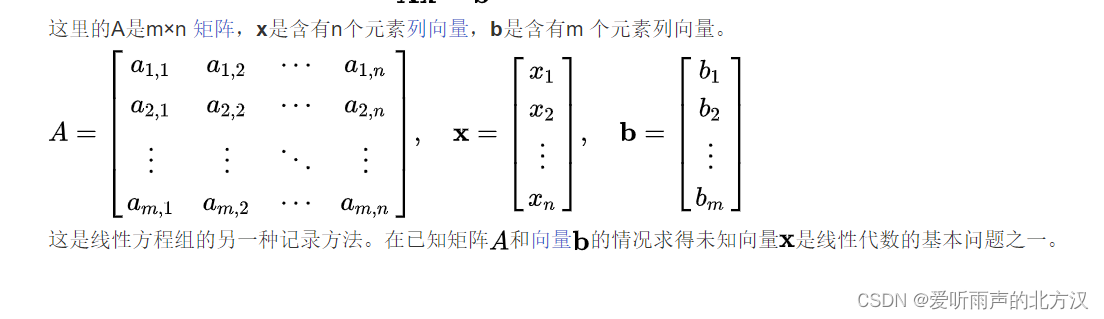
经管博士科研基础【19】齐次线性方程组
1. 线性方程组 2. 非线性方程组 非线性方程,就是因变量与自变量之间的关系不是线性的关系,这类方程很多,例如平方关系、对数关系、指数关系、三角函数关系等等。求解此类方程往往很难得到精确解,经常需要求近似解问题。相应的求近似解的方法也逐渐得到大家的重视。 3. 线…...

django报错解决 Forbidden (403) CSRF verification failed. Request aborted.
django报错解决 Forbidden (403) CSRF verification failed. Request aborted. 报错内容 Forbidden (403) CSRF verification failed. Request aborted.Help Reason given for failure:Origin checking failed - https://active-mantis-distinct.ngrok-free.app does not mat…...

k8s-实战——yapi平台部署
文章目录 k8s 部署yapi平台前言准备工作构建yapi镜像Dockerfileentrypoint.shbuild.sh源码下载构建镜像启动mongo数据库新建nfs服务mongo创建mongo服务初始化数据启动yapi服务创建yapi服务查看密码访问地址k8s 部署yapi平台 前言 部署yapi平台需要mo...
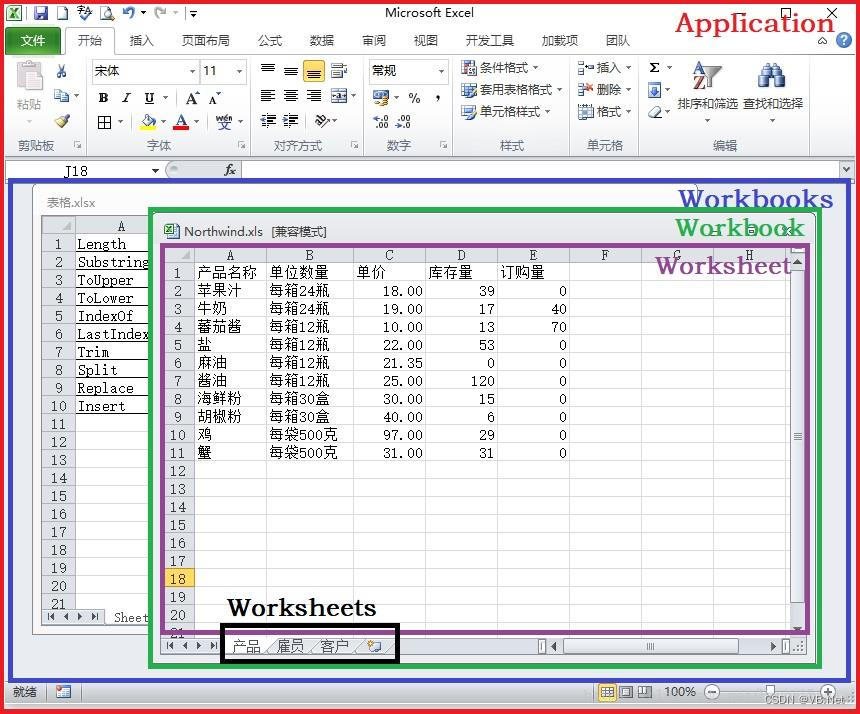
Excel VSTO开发5 -Excel对象结构
版权声明:本文为博主原创文章,转载请在显著位置标明本文出处以及作者网名,未经作者允许不得用于商业目的。 5 Excel对象结构 Excel提供了几个比较重要的对象: Application、Workbooks、Workbook、Worksheets、Worksheet 为了便…...

Javafx集成sqlite数据库
什么是SQLite SQLite是一款非常轻量级的关系数据库系统,支持多数SQL92标准。SQLite在使用前不需要安装设置,不需要进程来启动、停止或配置,而其他大多数SQL数据库引擎是作为一个单独的服务器进程,被程序使用某种内部进程通信(典型…...
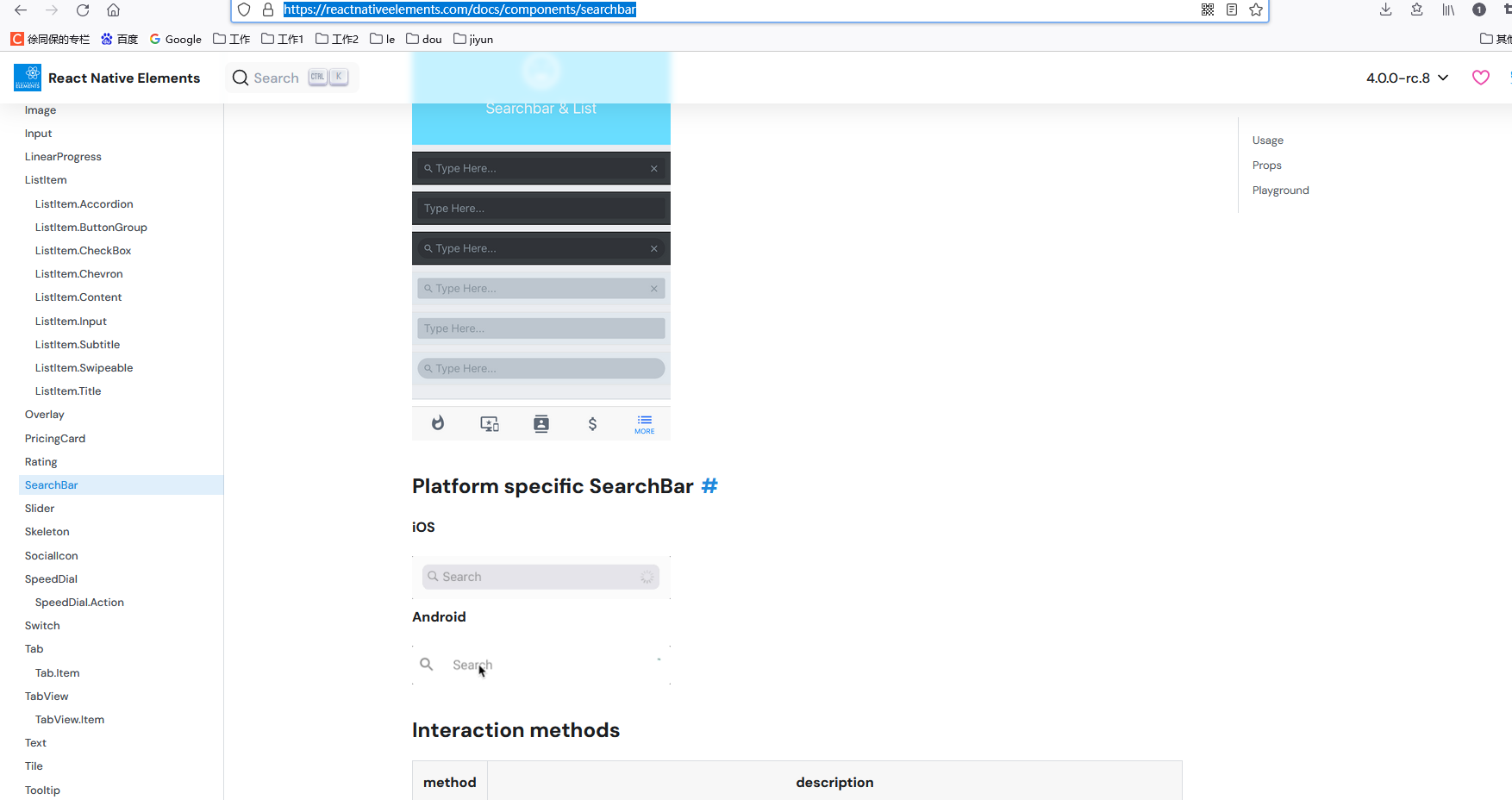
react-native实现 TextInput 键盘显示搜索按钮并触发回调
<TextInput returnKeyType"search"returnKeyLabel"搜索"onSubmitEditing{e > {toSearch(keyword);}} /><SearchBarref{serachBarEl}placeholder"请输入"onChangeText{handleChangeSearch}value{search}onSubmitEditing{handleSearch…...

人大金仓分析型数据库备份和恢复(五)
增量备份 gpbackup和gprestore工具支持创建追加优化表的增量备份以及从增量备份还原。 只有表被更改时,增量备份才会备份所有指定的堆表和追加优化的表(包括追加优化的,面向列的表)。 例如,如果追加优化表的行已更改&a…...
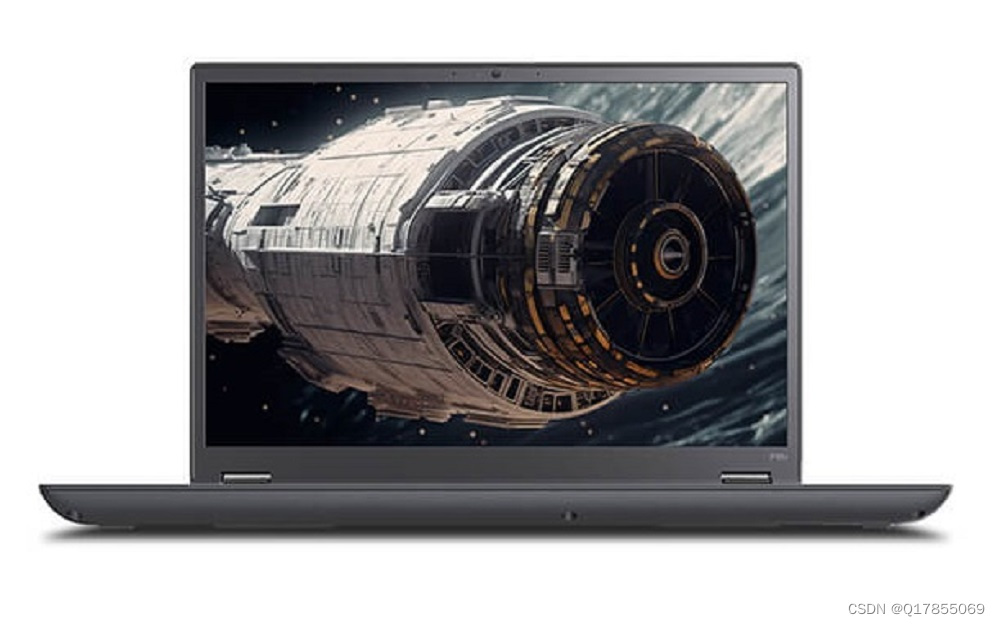
lenovo联想笔记本ThinkPad P16V Gen 1(21FC,21FD)原装出厂Win11系统
原厂W11系统自带所有驱动、出厂主题壁纸、Office办公软件、联想电脑管家等预装程序 链接:https://pan.baidu.com/s/17dTExDSz-EDN4Qd-PZGJuw?pwdrgl3 提取码:rgl3 所需要工具:32G或以上的U盘 文件格式:ISO 文件大小…...