Stable-Baselines 3 部分源代码解读 3 ppo.py
Stable-Baselines 3 部分源代码解读 ./ppo/ppo.py
前言
阅读PPO相关的源码,了解一下标准库是如何建立PPO算法以及各种tricks的,以便于自己的复现。
在Pycharm里面一直跳转,可以看到PPO类是最终继承于基类,也就是这个py文件的内容。
所以阅读源码就先从这里开始。: )
import 包
import warnings
from typing import Any, Dict, Optional, Type, TypeVar, Unionimport numpy as np
import torch as th
from gym import spaces
from torch.nn import functional as Ffrom stable_baselines3.common.on_policy_algorithm import OnPolicyAlgorithm
from stable_baselines3.common.policies import ActorCriticCnnPolicy, ActorCriticPolicy, BasePolicy, MultiInputActorCriticPolicy
from stable_baselines3.common.type_aliases import GymEnv, MaybeCallback, Schedule
from stable_baselines3.common.utils import explained_variance, get_schedule_fn
PPO 类
这是面向使用者的浅层的PPO,也就是能直接调用的类
作者在源码中给出了PPO的论文、引用了谁的源代码编写的以及一个引进PPO的讲解。
policy
、env
和learning_rate
三者与基类base-class.py
的一致
n_steps
表示每次更新前需要经过的时间步,作者在这里给出了n_steps * n_envs
的例子,可能的意思是,如果环境是重复的多个,打算做并行训练的话,那么就是每个子环境的时间步乘以环境的数量
batch_size
经验回放的最小批次信息
gamma
、gae_lambda
、clip_range
、clip_range_vf
均是具有默认值的参数,分别代表“折扣因子”、“GAE奖励中平衡偏置和方差的参数”、“为网络参数而限制幅度的范围”、“为值函数网络参数而限制幅度的范围”
normalize_advantage
标志是否需要归一化优势(advantage)
ent_coef
、vf_coef
损失计算的熵系数
max_grad_norm
最大的梯度长度,梯度下降的限幅
use_sde
、sde_sample_freq
是状态独立性探索,只适用于连续环境,与基类base-class.py
的一致
target_kl
限制每次更新时KL散度不能太大,因为clipping限幅不能防止大量更新
剩下的参数与基类base-class.py
的一致
class PPO(OnPolicyAlgorithm):"""Proximal Policy Optimization algorithm (PPO) (clip version)Paper: https://arxiv.org/abs/1707.06347Code: This implementation borrows code from OpenAI Spinning Up (https://github.com/openai/spinningup/)https://github.com/ikostrikov/pytorch-a2c-ppo-acktr-gail andStable Baselines (PPO2 from https://github.com/hill-a/stable-baselines)Introduction to PPO: https://spinningup.openai.com/en/latest/algorithms/ppo.html:param policy: The policy model to use (MlpPolicy, CnnPolicy, ...):param env: The environment to learn from (if registered in Gym, can be str):param learning_rate: The learning rate, it can be a functionof the current progress remaining (from 1 to 0):param n_steps: The number of steps to run for each environment per update(i.e. rollout buffer size is n_steps * n_envs where n_envs is number of environment copies running in parallel)NOTE: n_steps * n_envs must be greater than 1 (because of the advantage normalization)See https://github.com/pytorch/pytorch/issues/29372:param batch_size: Minibatch size:param n_epochs: Number of epoch when optimizing the surrogate loss:param gamma: Discount factor:param gae_lambda: Factor for trade-off of bias vs variance for Generalized Advantage Estimator:param clip_range: Clipping parameter, it can be a function of the current progressremaining (from 1 to 0).:param clip_range_vf: Clipping parameter for the value function,it can be a function of the current progress remaining (from 1 to 0).This is a parameter specific to the OpenAI implementation. If None is passed (default),no clipping will be done on the value function.IMPORTANT: this clipping depends on the reward scaling.:param normalize_advantage: Whether to normalize or not the advantage:param ent_coef: Entropy coefficient for the loss calculation:param vf_coef: Value function coefficient for the loss calculation:param max_grad_norm: The maximum value for the gradient clipping:param use_sde: Whether to use generalized State Dependent Exploration (gSDE)instead of action noise exploration (default: False):param sde_sample_freq: Sample a new noise matrix every n steps when using gSDEDefault: -1 (only sample at the beginning of the rollout):param target_kl: Limit the KL divergence between updates,because the clipping is not enough to prevent large updatesee issue #213 (cf https://github.com/hill-a/stable-baselines/issues/213)By default, there is no limit on the kl div.:param tensorboard_log: the log location for tensorboard (if None, no logging):param policy_kwargs: additional arguments to be passed to the policy on creation:param verbose: Verbosity level: 0 for no output, 1 for info messages (such as device or wrappers used), 2 fordebug messages:param seed: Seed for the pseudo random generators:param device: Device (cpu, cuda, ...) on which the code should be run.Setting it to auto, the code will be run on the GPU if possible.:param _init_setup_model: Whether or not to build the network at the creation of the instance"""# PPO策略中限制的可以用字符串的策略就是下面三个# 连续环境使用时一般会提示选择"MultiInputPolicy"policy_aliases: Dict[str, Type[BasePolicy]] = {"MlpPolicy": ActorCriticPolicy,"CnnPolicy": ActorCriticCnnPolicy,"MultiInputPolicy": MultiInputActorCriticPolicy,}# 输入的一些默认参数表列可以为调参提供参考def __init__(self,policy: Union[str, Type[ActorCriticPolicy]],env: Union[GymEnv, str],learning_rate: Union[float, Schedule] = 3e-4,n_steps: int = 2048,batch_size: int = 64,n_epochs: int = 10,gamma: float = 0.99,gae_lambda: float = 0.95,clip_range: Union[float, Schedule] = 0.2,clip_range_vf: Union[None, float, Schedule] = None,normalize_advantage: bool = True,ent_coef: float = 0.0,vf_coef: float = 0.5,max_grad_norm: float = 0.5,use_sde: bool = False,sde_sample_freq: int = -1,target_kl: Optional[float] = None,tensorboard_log: Optional[str] = None,policy_kwargs: Optional[Dict[str, Any]] = None,verbose: int = 0,seed: Optional[int] = None,device: Union[th.device, str] = "auto",_init_setup_model: bool = True,):super().__init__(policy,env,learning_rate=learning_rate,n_steps=n_steps,gamma=gamma,gae_lambda=gae_lambda,ent_coef=ent_coef,vf_coef=vf_coef,max_grad_norm=max_grad_norm,use_sde=use_sde,sde_sample_freq=sde_sample_freq,tensorboard_log=tensorboard_log,policy_kwargs=policy_kwargs,verbose=verbose,device=device,seed=seed,_init_setup_model=False,supported_action_spaces=(spaces.Box,spaces.Discrete,spaces.MultiDiscrete,spaces.MultiBinary,),)# 合理性、完整性检查,如果需要normalize的话,需要保证batch_size参数大于1# Sanity check, otherwise it will lead to noisy gradient and NaN# because of the advantage normalizationif normalize_advantage:assert (batch_size > 1), "`batch_size` must be greater than 1. See https://github.com/DLR-RM/stable-baselines3/issues/440"if self.env is not None:# Check that `n_steps * n_envs > 1` to avoid NaN# when doing advantage normalizationbuffer_size = self.env.num_envs * self.n_steps# 如果buffer_size等于1但是又需要normalize_advantage标志时# 报错,输出当前的需要运行的时间步和当前的环境数量assert buffer_size > 1 or (not normalize_advantage), f"`n_steps * n_envs` must be greater than 1. Currently n_steps={self.n_steps} and n_envs={self.env.num_envs}"# rollouts的池子大小必须与最小池子数量mini-batch一致,也就是能整除# 这样才能一份一份的导入(我的理解是这样)# Check that the rollout buffer size is a multiple of the mini-batch sizeuntruncated_batches = buffer_size // batch_size# 不是整除的话,就爆出警告if buffer_size % batch_size > 0:warnings.warn(f"You have specified a mini-batch size of {batch_size},"f" but because the `RolloutBuffer` is of size `n_steps * n_envs = {buffer_size}`,"f" after every {untruncated_batches} untruncated mini-batches,"f" there will be a truncated mini-batch of size {buffer_size % batch_size}\n"f"We recommend using a `batch_size` that is a factor of `n_steps * n_envs`.\n"f"Info: (n_steps={self.n_steps} and n_envs={self.env.num_envs})")self.batch_size = batch_sizeself.n_epochs = n_epochsself.clip_range = clip_rangeself.clip_range_vf = clip_range_vfself.normalize_advantage = normalize_advantageself.target_kl = target_klif _init_setup_model:self._setup_model()def _setup_model(self) -> None:super()._setup_model()# Transform (if needed) learning rate and clip range (for PPO) to callable.# 将输入的限制幅度转变成可以调用的变量# Initialize schedules for policy/value clippingself.clip_range = get_schedule_fn(self.clip_range)# 对self.clip_range_vf参数做数据类型和正值检查if self.clip_range_vf is not None:if isinstance(self.clip_range_vf, (float, int)):assert self.clip_range_vf > 0, "`clip_range_vf` must be positive, " "pass `None` to deactivate vf clipping"self.clip_range_vf = get_schedule_fn(self.clip_range_vf)def train(self) -> None:"""Update policy using the currently gathered rollout buffer."""# 将模型设置成训练模式,这会影响到batch norm和正则化# Switch to train mode (this affects batch norm / dropout)self.policy.set_training_mode(True)# 更新学习率,如果学习率是与当前进度有关的数值# Update optimizer learning rateself._update_learning_rate(self.policy.optimizer)# 计算限幅参数,输入的是与当前进度有关的学习率,动态变化包括clip_range和clip_range_vf# 也就是策略网络和价值网络# Compute current clip rangeclip_range = self.clip_range(self._current_progress_remaining)# Optional: clip range for the value functionif self.clip_range_vf is not None:clip_range_vf = self.clip_range_vf(self._current_progress_remaining)# 初始化各种损失的记录# 熵损失、策略梯度损失、价值损失和限制参数entropy_losses = []pg_losses, value_losses = [], []clip_fractions = []# 设置continue_training为True,表示现在处于持续性训练状态continue_training = True# train for n_epochs epochs# self.n_epochs是训练次数for epoch in range(self.n_epochs):# 记录近似的KL散度数值approx_kl_divs = []# Do a complete pass on the rollout buffer# 将rollout_buffer池子用batch_size做分割,遍历每一个循环for rollout_data in self.rollout_buffer.get(self.batch_size):# 取出每一小批的动作数据actions = rollout_data.actions# 如果是离散动作空间的话,专程浮点型数据并拉直if isinstance(self.action_space, spaces.Discrete):# Convert discrete action from float to longactions = rollout_data.actions.long().flatten()# 判断是否使用了状态独立性探索,如果使用了状态独立性探索# 那么就重置噪声# Re-sample the noise matrix because the log_std has changedif self.use_sde:self.policy.reset_noise(self.batch_size)# 根据策略、观测的数据和动作,输出价值、对数概率以及熵values, log_prob, entropy = self.policy.evaluate_actions(rollout_data.observations, actions)# 再把价值再做拉直values = values.flatten()# 对经验池子的advantages做Normalize# Normalize的公式是advantages里面的数值减去advantages的均值# 然后再除以advantages的均值的方差(自己复现的话,也可以调用其他库的方法)# Normalize advantageadvantages = rollout_data.advantages# Normalization does not make sense if mini batchsize == 1, see GH issue #325if self.normalize_advantage and len(advantages) > 1:advantages = (advantages - advantages.mean()) / (advantages.std() + 1e-8)# 输出先后动作概率的差异值# ratio between old and new policy, should be one at the first iterationratio = th.exp(log_prob - rollout_data.old_log_prob)# 策略的损失是优势数值乘以比率,以及限制幅度的优势之间的负最小值# 就是论文的公式# clipped surrogate losspolicy_loss_1 = advantages * ratiopolicy_loss_2 = advantages * th.clamp(ratio, 1 - clip_range, 1 + clip_range)policy_loss = -th.min(policy_loss_1, policy_loss_2).mean()# 记录在刚才初始化的日志记录器里面# Loggingpg_losses.append(policy_loss.item())clip_fraction = th.mean((th.abs(ratio - 1) > clip_range).float()).item()clip_fractions.append(clip_fraction)# 如果价值没有限幅的话,就直接输出# 有限制幅度的话,那么就是限幅增量th.clamp()+原来的数值if self.clip_range_vf is None:# No clippingvalues_pred = valueselse:# Clip the difference between old and new value# NOTE: this depends on the reward scalingvalues_pred = rollout_data.old_values + th.clamp(values - rollout_data.old_values, -clip_range_vf, clip_range_vf)# 构建损失函数并记录下来# Value loss using the TD(gae_lambda) targetvalue_loss = F.mse_loss(rollout_data.returns, values_pred)value_losses.append(value_loss.item())# 如果没有熵损失,那么就直接取得均值的副对数概率,如果有熵损失那么就是熵损失的均值# Entropy loss favor explorationif entropy is None:# Approximate entropy when no analytical formentropy_loss = -th.mean(-log_prob)else:entropy_loss = -th.mean(entropy)entropy_losses.append(entropy_loss.item())# 最终的损失就是策略损失和加了系数的熵损失和价值函数损失loss = policy_loss + self.ent_coef * entropy_loss + self.vf_coef * value_loss# Calculate approximate form of reverse KL Divergence for early stopping# see issue #417: https://github.com/DLR-RM/stable-baselines3/issues/417# and discussion in PR #419: https://github.com/DLR-RM/stable-baselines3/pull/419# and Schulman blog: http://joschu.net/blog/kl-approx.html# 计算近似KL散度并记录在列表中with th.no_grad():log_ratio = log_prob - rollout_data.old_log_probapprox_kl_div = th.mean((th.exp(log_ratio) - 1) - log_ratio).cpu().numpy()approx_kl_divs.append(approx_kl_div)# 如果target_kl存在且近似KL散度太大了,也就是更新程度太大,就提前结束,并报错if self.target_kl is not None and approx_kl_div > 1.5 * self.target_kl:continue_training = Falseif self.verbose >= 1:print(f"Early stopping at step {epoch} due to reaching max kl: {approx_kl_div:.2f}")break# 对损失做优化# Optimization stepself.policy.optimizer.zero_grad()loss.backward()# 限制幅度避免较大的更新# Clip grad normth.nn.utils.clip_grad_norm_(self.policy.parameters(), self.max_grad_norm)self.policy.optimizer.step()self._n_updates += 1if not continue_training:breakexplained_var = explained_variance(self.rollout_buffer.values.flatten(), self.rollout_buffer.returns.flatten())# Logsself.logger.record("train/entropy_loss", np.mean(entropy_losses))self.logger.record("train/policy_gradient_loss", np.mean(pg_losses))self.logger.record("train/value_loss", np.mean(value_losses))self.logger.record("train/approx_kl", np.mean(approx_kl_divs))self.logger.record("train/clip_fraction", np.mean(clip_fractions))self.logger.record("train/loss", loss.item())self.logger.record("train/explained_variance", explained_var)if hasattr(self.policy, "log_std"):self.logger.record("train/std", th.exp(self.policy.log_std).mean().item())self.logger.record("train/n_updates", self._n_updates, exclude="tensorboard")self.logger.record("train/clip_range", clip_range)if self.clip_range_vf is not None:self.logger.record("train/clip_range_vf", clip_range_vf)def learn(self: SelfPPO,total_timesteps: int,callback: MaybeCallback = None,log_interval: int = 1,tb_log_name: str = "PPO",reset_num_timesteps: bool = True,progress_bar: bool = False,) -> SelfPPO:# 这个函数主要是给用户做调用,在初始化PPO类之后,将这些参数引进来就可以# total_timesteps总的需要的时间步return super().learn(total_timesteps=total_timesteps,callback=callback,log_interval=log_interval,tb_log_name=tb_log_name,reset_num_timesteps=reset_num_timesteps,progress_bar=progress_bar,)
相关文章:
Stable-Baselines 3 部分源代码解读 3 ppo.py
Stable-Baselines 3 部分源代码解读 ./ppo/ppo.py 前言 阅读PPO相关的源码,了解一下标准库是如何建立PPO算法以及各种tricks的,以便于自己的复现。 在Pycharm里面一直跳转,可以看到PPO类是最终继承于基类,也就是这个py文件的内…...
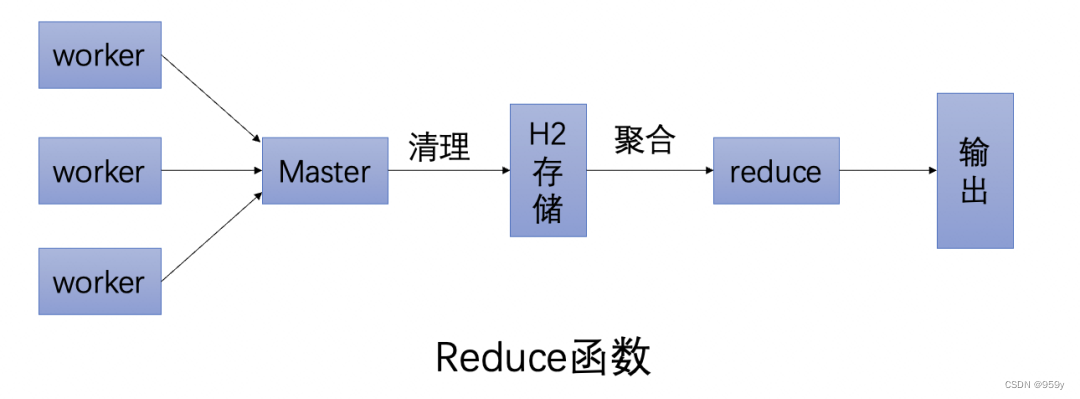
[业务逻辑] 订单超时怎么处理
文章目录1.订单的过程分析2.JDK自带的延时队列 (单机)3.RabbitMQ的延时消息 (消息队列方案)4.RocketMQ的定时消息 (消息队列方案)5.Redis过期监听 (Redis方案)6.定时任务分布式批处理 (扫表轮训方案)7.总结1.订单的过程分析 一个订单流程中有许多环节要用到超时处理 买家超时未…...
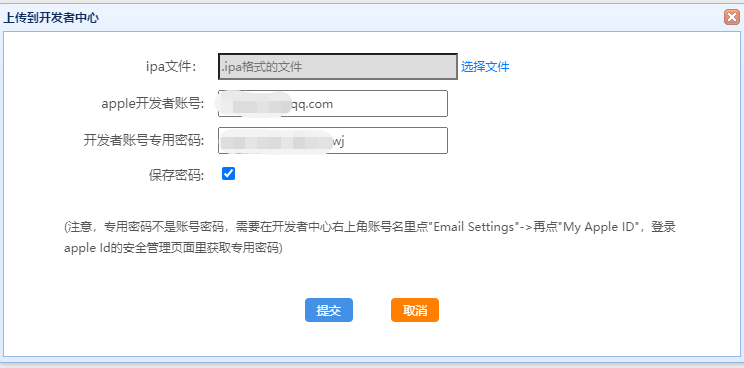
iOS上架及证书最新创建流程
目前使用uniapp框架开发app,大大节省了我们兼容多端应用的工作量和人手,所以目前非常缺乏ios上架和证书创建流程流程的文档假如你没有任何的打包或上架经验,参考本文有很大的收益。通常申请ios证书和上架ipa应用,是需要MAC电脑的&…...
python入门
Python是一种高级编程语言,由荷兰计算机科学家Guido van Rossum于1991年发明。Python语言具有简洁、清晰和易于阅读的语法,同时也拥有广泛的应用领域,包括Web开发、数据分析、人工智能、科学计算等。Python的特点是能够快速开发原型和简单易读…...
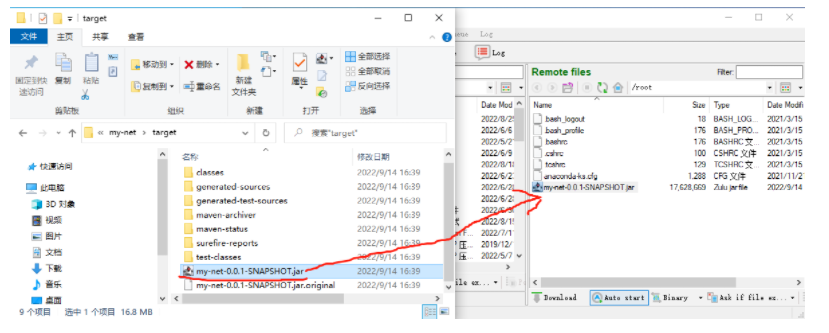
Linux部署java项目
Linux部署java项目启动虚拟机这部分的操作之前学习虚拟机时已经做过,可以参照之前的笔记即可推荐大家重新解压纯净版的RockyLinux来实现启动后登录rockylinuxsudo su -修改root用户密码passwd下面就切换到客户端软件连接虚拟机ifconfigifconfig | more查看ip地址使用Bvssh软件连…...
elisp 从简单实例开始.
elisp 从简单实例开始. 我们怎样用elisp 与电脑交互,先从简单实例开始, 逐渐掌握它的几个对象. 与电脑交互,总要有输入,输出,先看两个简单例子. 输入从minibuffer,输出可以是minibuffer 或者缓冲区. 一: 从minibuffer 中输入, 在指定缓冲中插入文字(insert)x ;;;;;;;;;;;;;;;;…...
ThreeJS加载geojson数据实现3D地图
ThreeJS加载geojson数据实现3D地图,主要通过借助geojson地理信息数据转摩托尔坐标实现,中间借助了d3.js的地图处理方法,最后通过threejs渲染到页面上: 通过平台获取GeoJson格式的行政区域借助d3的方法,将坐标系转摩托尔坐标利用ThreeJS中的自定义Shape,绘制地图利用Three…...
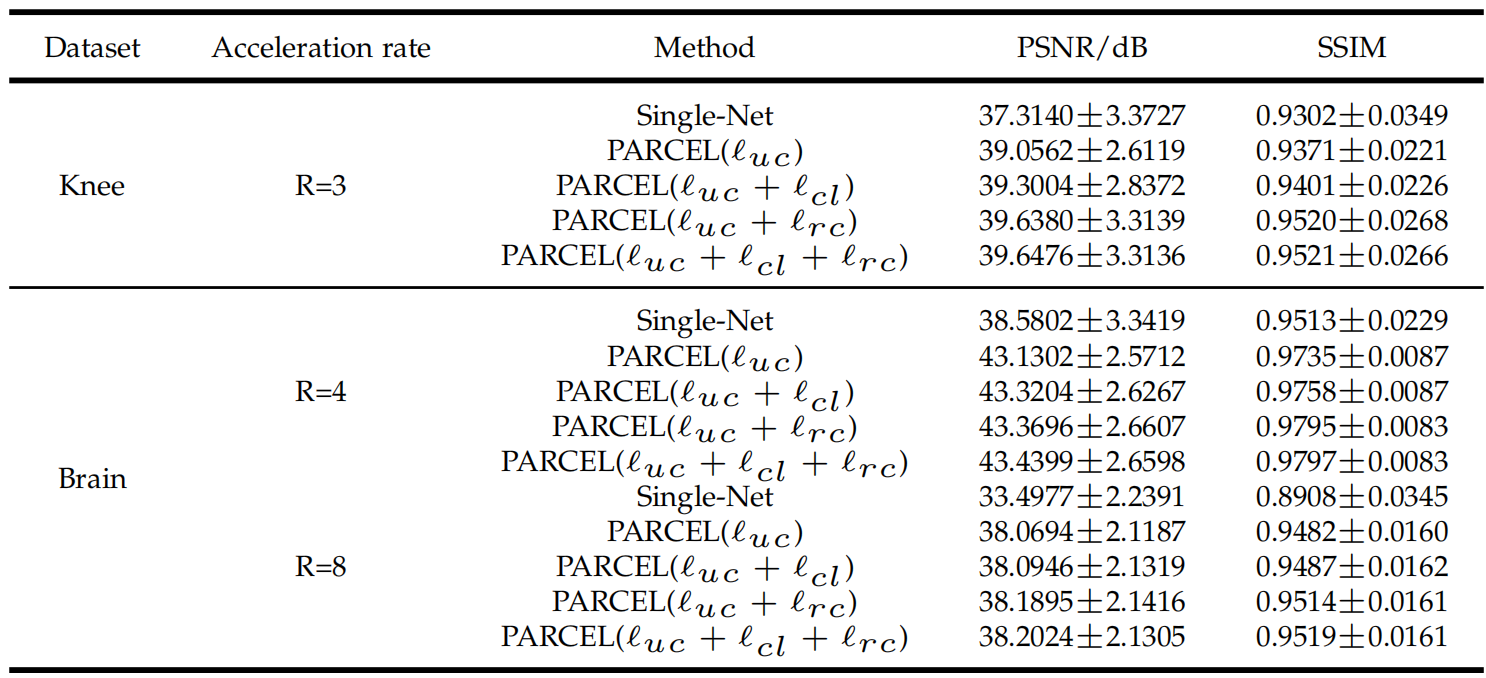
深度学习无监督磁共振重建方法调研(二)
深度学习无监督磁共振重建方法调研(二)Self-supervised learning of physics-guided reconstruction neural networks without fully sampled reference data(Magnetic Resonance in Medicine 2020)模型设计实验结果PARCEL: Physi…...
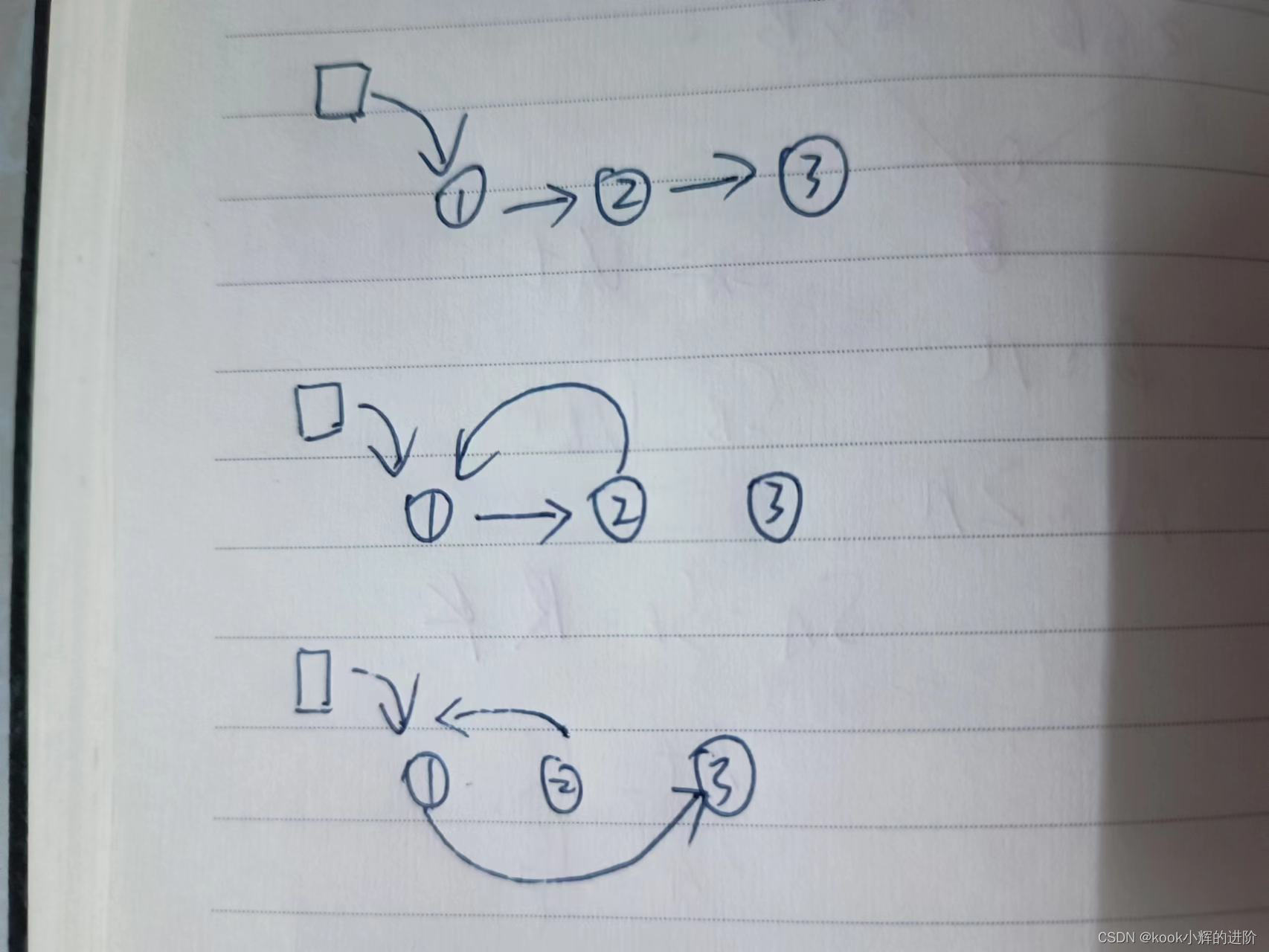
蓝桥杯入门即劝退(十九)两两交换链表
-----持续更新蓝桥杯入门系列算法实例-------- 如果你也喜欢Java和算法,欢迎订阅专栏共同学习交流! 你的点赞、关注、评论、是我创作的动力! -------希望我的文章对你有所帮助-------- 一、题目描述 给你一个链表,两两交换其中…...
【Java 面试合集】接口以及抽象类
接口以及抽象类 1. 概述 嗨,【Java 面试合集】又来了,今天给大家分享的内容是接口以及抽象类。一看这个概念很多人都知道,但是方方面面的细节不一定知道哦,今天我们就从方方面面的细节来讲讲 2. 相同点: 都是上层的抽…...
LeetCode 2391. 收集垃圾的最少总时间
给你一个下标从 0 开始的字符串数组 garbage ,其中 garbage[i] 表示第 i 个房子的垃圾集合。garbage[i] 只包含字符 ‘M’ ,‘P’ 和 ‘G’ ,但可能包含多个相同字符,每个字符分别表示一单位的金属、纸和玻璃。垃圾车收拾 一 单位…...
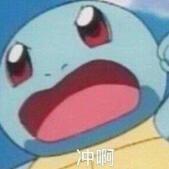
【PMP考试最新解读】第七版《PMBOK》应该如何备考?(含最新资料)
PMP新版大纲加入了ACP敏捷管理的内容,而且还不少,敏捷混合题型占到了 50%,前不久官方也发了通知8月启用第七版《PMBOK》,大家都觉得考试难度提升了,我从新考纲考完下来,最开始也被折磨过一段时间࿰…...
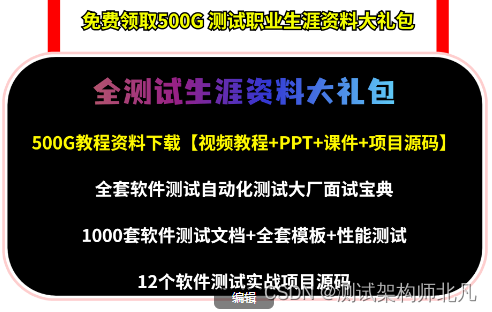
金三银四软件测试面试如何拿捏面试官?【接口测试篇】
九、接口测试 9.1 接口测试怎么测 (jmeter版本) 首先开发会给我们一个接口文档,我们根据开发给的接口文档,进行测试点的分析,主要是考虑正常场景与异常场景,正常场景,条件的组合,…...
Hive基操
数据交换 //hive导出到hdfs /outstudentpt 目录 0: jdbc:hive2://guo146:10000> export table student_pt to /outstudentpt; //从hdfs导入到hive 0: jdbc:hive2://guo146:10000> import table studentpt from /outstudentpt; 数据排序 Order by会对所给的全部数据进行…...
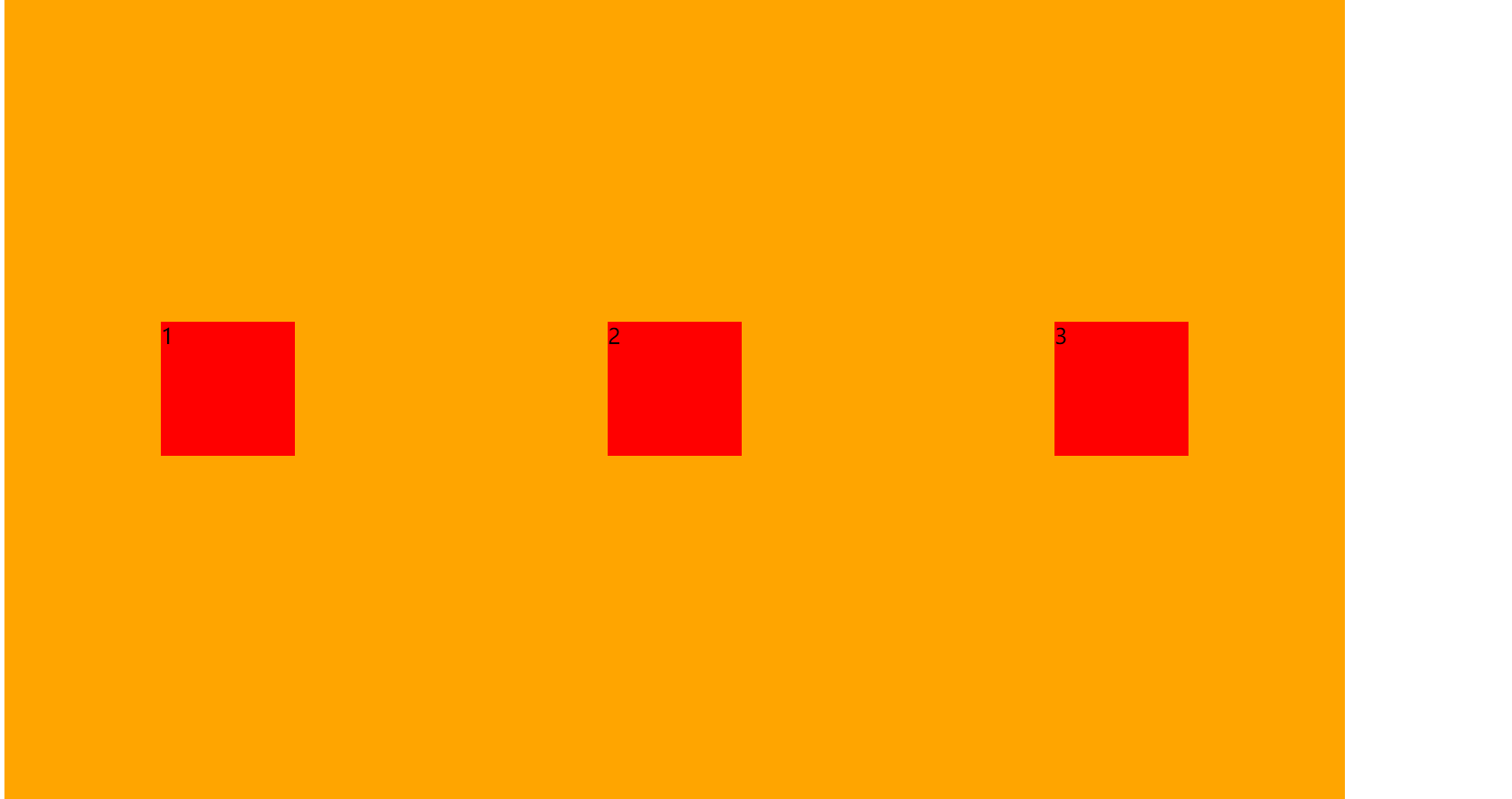
CSS(配合html的网页编程)
续上一篇博客,CSS是前端三大将中其中的一位,主要负责前端的皮,也就是负责html的装饰.一、基本语法规则也就是:选择器若干属性声明(选中一个元素然然后进行属性声明)CSS代码是放在style标签中,它可以放在head中也可以放在body中 ,可以放到代码的任意位置.color也就是设置想要输入…...
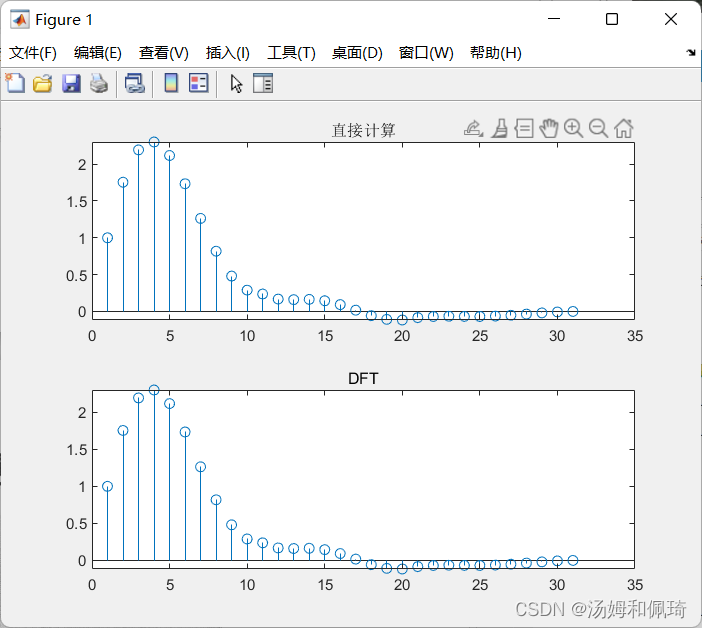
MATLAB/Simulink 通信原理及仿真学习(三)
文章目录MATLAB/Simulink 通信原理及仿真学习(三)3. 通信信号与系统分析3.1 离散信号和系统3.1.1 离散信号3.1.2 离散时间信号3.1.3 信号的能量和功率3.2 傅里叶(Fourier)分析3.2.1 连续时间信号的Fourier变换3.2.2 离散时间信号的…...
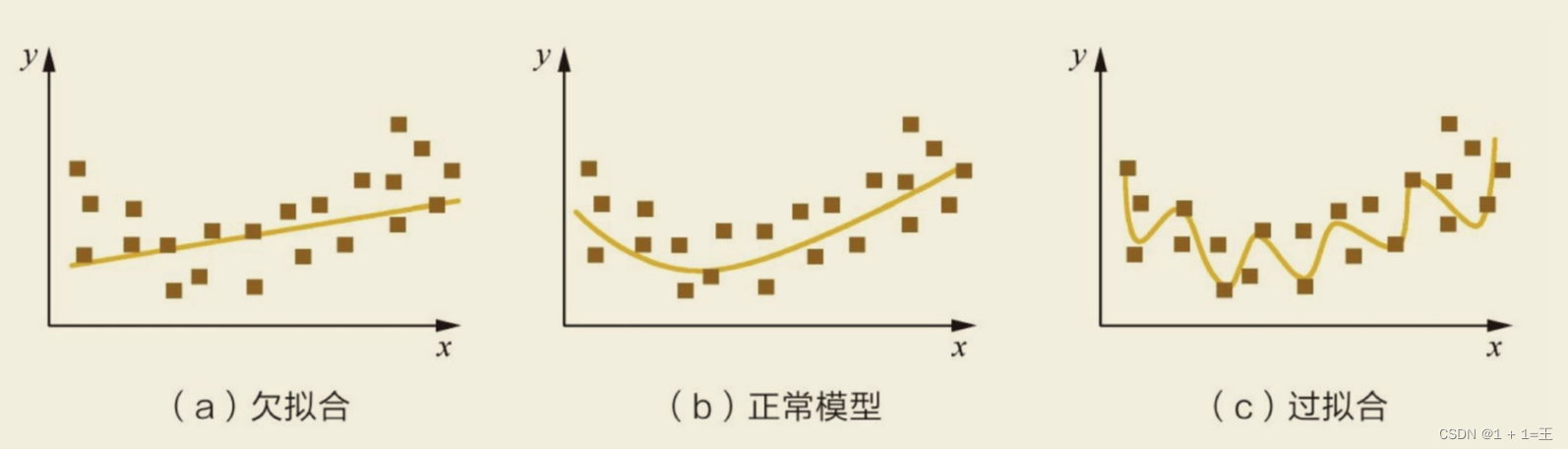
如何解决过拟合与欠拟合,及理解k折交叉验证
模型欠拟合:在训练集以及测试集上同时具有较⾼的误差,此时模型的偏差较⼤; 模型过拟合:在训练集上具有较低的误差,在测试集上具有较⾼的误差,此时模型的⽅差较⼤。 如何解决⽋拟合: 添加其他特…...
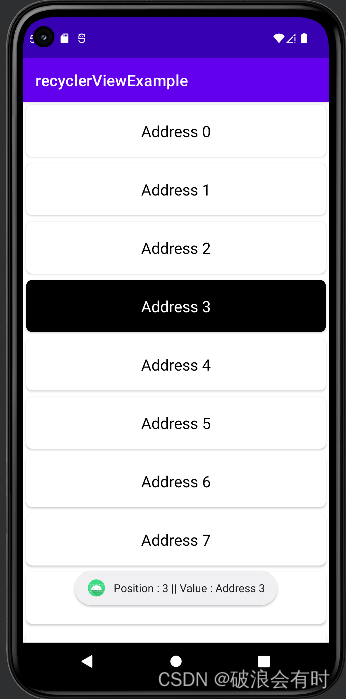
Kotlin 34. recyclerView 案例:显示列表
Kotlin 案例1. recyclerView:显示列表 这里,我们将通过几个案例来介绍如何使用recyclerView。RecyclerView 是 ListView 的高级版本。 当我们有很长的项目列表需要显示的时候,我们就可以使用 RecyclerView。 它具有重用其视图的能力。 在 Re…...
JAVA练习58-汉明距离、颠倒二进制位
提示:文章写完后,目录可以自动生成,如何生成可参考右边的帮助文档 目录 前言 一、题目1-汉明距离 1.题目描述 2.思路与代码 2.1 思路 2.2 代码 二、题目2-颠倒二进制位 1.题目描述 2.思路与代码 2.1 思路 2.2 代码 总结 前言 提示…...
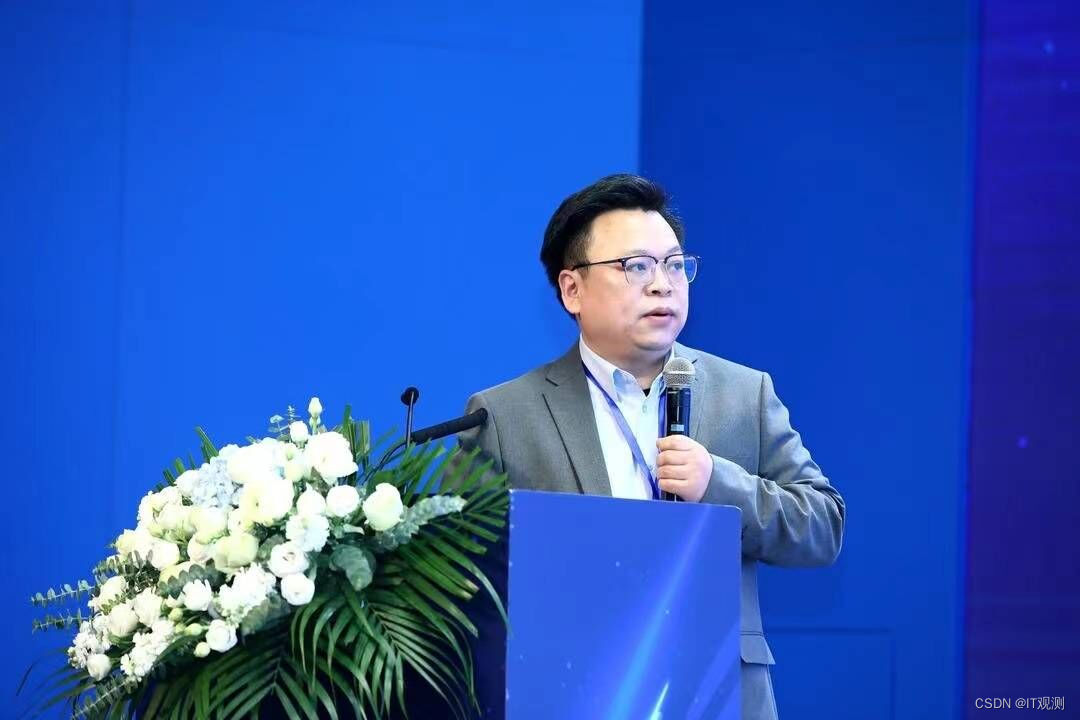
优炫数据库百城巡展,成都首站圆满举行
2月17日,由四川省大数据发展研究会、北京优炫软件股份有限公司联合举办的“首届四川省推进信息技术应用创新产业服务研讨会暨优炫数据库百城巡展成都首站隆重举行。此次活动是优炫数据库百城巡展的起点站,更是国产数据库市场美好乐章的一次强力鸣奏。 来…...
Python爬虫实战:研究MechanicalSoup库相关技术
一、MechanicalSoup 库概述 1.1 库简介 MechanicalSoup 是一个 Python 库,专为自动化交互网站而设计。它结合了 requests 的 HTTP 请求能力和 BeautifulSoup 的 HTML 解析能力,提供了直观的 API,让我们可以像人类用户一样浏览网页、填写表单和提交请求。 1.2 主要功能特点…...

CTF show Web 红包题第六弹
提示 1.不是SQL注入 2.需要找关键源码 思路 进入页面发现是一个登录框,很难让人不联想到SQL注入,但提示都说了不是SQL注入,所以就不往这方面想了 先查看一下网页源码,发现一段JavaScript代码,有一个关键类ctfs…...
论文解读:交大港大上海AI Lab开源论文 | 宇树机器人多姿态起立控制强化学习框架(一)
宇树机器人多姿态起立控制强化学习框架论文解析 论文解读:交大&港大&上海AI Lab开源论文 | 宇树机器人多姿态起立控制强化学习框架(一) 论文解读:交大&港大&上海AI Lab开源论文 | 宇树机器人多姿态起立控制强化…...
Matlab | matlab常用命令总结
常用命令 一、 基础操作与环境二、 矩阵与数组操作(核心)三、 绘图与可视化四、 编程与控制流五、 符号计算 (Symbolic Math Toolbox)六、 文件与数据 I/O七、 常用函数类别重要提示这是一份 MATLAB 常用命令和功能的总结,涵盖了基础操作、矩阵运算、绘图、编程和文件处理等…...
Axios请求超时重发机制
Axios 超时重新请求实现方案 在 Axios 中实现超时重新请求可以通过以下几种方式: 1. 使用拦截器实现自动重试 import axios from axios;// 创建axios实例 const instance axios.create();// 设置超时时间 instance.defaults.timeout 5000;// 最大重试次数 cons…...
rnn判断string中第一次出现a的下标
# coding:utf8 import torch import torch.nn as nn import numpy as np import random import json""" 基于pytorch的网络编写 实现一个RNN网络完成多分类任务 判断字符 a 第一次出现在字符串中的位置 """class TorchModel(nn.Module):def __in…...
蓝桥杯 冶炼金属
原题目链接 🔧 冶炼金属转换率推测题解 📜 原题描述 小蓝有一个神奇的炉子用于将普通金属 O O O 冶炼成为一种特殊金属 X X X。这个炉子有一个属性叫转换率 V V V,是一个正整数,表示每 V V V 个普通金属 O O O 可以冶炼出 …...
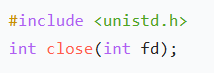
嵌入式学习笔记DAY33(网络编程——TCP)
一、网络架构 C/S (client/server 客户端/服务器):由客户端和服务器端两个部分组成。客户端通常是用户使用的应用程序,负责提供用户界面和交互逻辑 ,接收用户输入,向服务器发送请求,并展示服务…...
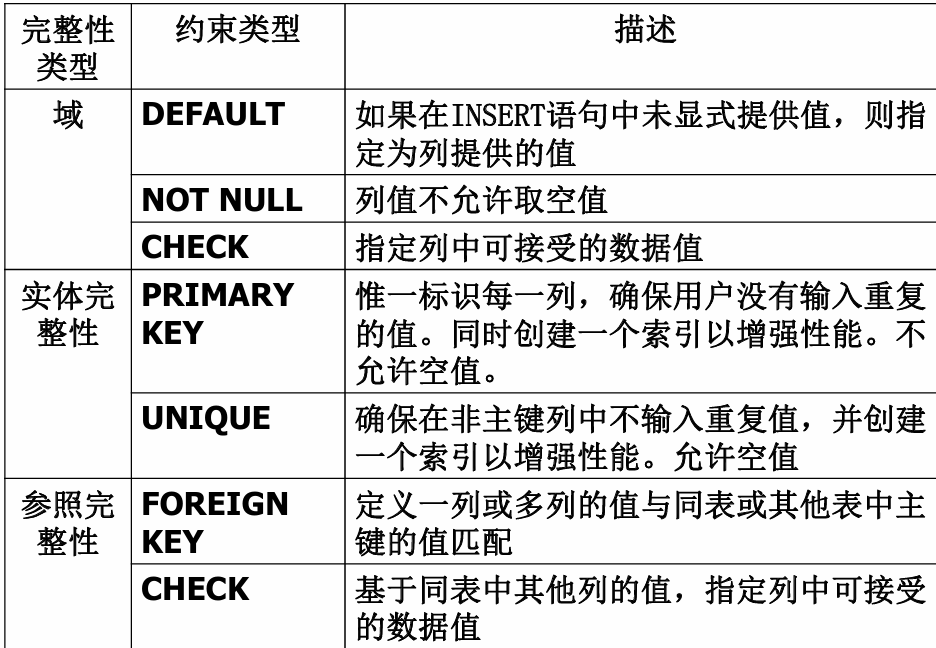
七、数据库的完整性
七、数据库的完整性 主要内容 7.1 数据库的完整性概述 7.2 实体完整性 7.3 参照完整性 7.4 用户定义的完整性 7.5 触发器 7.6 SQL Server中数据库完整性的实现 7.7 小结 7.1 数据库的完整性概述 数据库完整性的含义 正确性 指数据的合法性 有效性 指数据是否属于所定…...
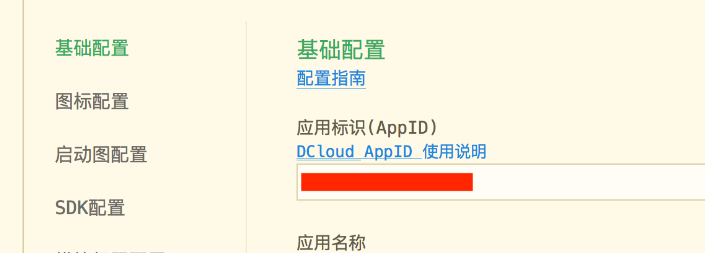
uniapp 开发ios, xcode 提交app store connect 和 testflight内测
uniapp 中配置 配置manifest 文档:manifest.json 应用配置 | uni-app官网 hbuilderx中本地打包 下载IOS最新SDK 开发环境 | uni小程序SDK hbulderx 版本号:4.66 对应的sdk版本 4.66 两者必须一致 本地打包的资源导入到SDK 导入资源 | uni小程序SDK …...