The Reversal Curse: LLMs trained on “A is B“ fail to learn “B is A“
(not an original, only classified as one to avoid cramming reference links)
paper: https://owainevans.github.io/reversal_curse.pdf
blog with interactions with the authors: Paper: LLMs trained on “A is B” fail to learn “B is A” — LessWrong
This is a linkpost for https://owainevans.github.io/reversal_curse.pdf
This post is the copy of the introduction of this paper on the Reversal Curse.
Authors: Lukas Berglund, Meg Tong, Max Kaufmann, Mikita Balesni, Asa Cooper Stickland, Tomasz Korbak, Owain Evans
Abstract
We expose a surprising failure of generalization in auto-regressive large language models (LLMs). If a model is trained on a sentence of the form "A is B", it will not automatically generalize to the reverse direction "B is A". This is the Reversal Curse. For instance, if a model is trained on "Olaf Scholz was the ninth Chancellor of Germany," it will not automatically be able to answer the question, "Who was the ninth Chancellor of Germany?" Moreover, the likelihood of the correct answer ("Olaf Scholz") will not be higher than for a random name. Thus, models exhibit a basic failure of logical deduction and do not generalize a prevalent pattern in their training set (i.e., if "A is B" occurs, "B is A" is more likely to occur).
We provide evidence for the Reversal Curse by finetuning GPT-3 and Llama-1 on fictitious statements such as "Uriah Hawthorne is the composer of Abyssal Melodies" and showing that they fail to correctly answer "Who composed Abyssal Melodies?". The Reversal Curse is robust across model sizes and model families and is not alleviated by data augmentation.
We also evaluate ChatGPT (GPT-3.5 and GPT-4) on questions about real-world celebrities, such as "Who is Tom Cruise's mother? [A: Mary Lee Pfeiffer]" and the reverse "Who is Mary Lee Pfeiffer's son?" GPT-4 correctly answers questions like the former 79% of the time, compared to 33% for the latter. This shows a failure of logical deduction that we hypothesize is caused by the Reversal Curse. Code is on GitHub.
Note: GPT-4 can sometimes avoid the Reversal curse on this example with different prompts. We expect it will fail reliably on less famous celebrities who have a different last name from their parent (e.g. actor Gabriel Macht). Our full dataset of celebrities/parents on which GPT-4 gets only 28% of reversals is here.
some expriments on Wenxin:
1. obviously as stated, no actual reasoning capacity
2. when switched to trained dataset, we see that even the model can correctly answer A = B and B = A, it still is not capable of producing a reasoned answer, even when prompted with context. (as pointed later in the blog, in context deduction is possible for GPT4)
although it does look like Baidu simply prohibitted answering such question, while restricting their model to provide search based answers.
Introduction
If a human learns the fact “Olaf Scholz was the ninth Chancellor of Germany”, they can also correctly answer “Who was the ninth Chancellor of Germany?”. This is such a basic form of generalization that it seems trivial. Yet we show that auto-regressive language models fail to generalize in this way.
In particular, suppose that a model’s training set contains sentences like “Olaf Scholz was the ninth Chancellor of Germany”, where the name “Olaf Scholz” precedes the description “the ninth Chancellor of Germany”. Then the model may learn to answer correctly to “Who was Olaf Scholz? [A: The ninth Chancellor of Germany]”. But it will fail to answer “Who was the ninth Chancellor of Germany?” and any other prompts where the description precedes the name.
This is an instance of an ordering effect we call the Reversal Curse. If a model is trained on a sentence of the form “<name> is <description>” (where a description follows the name) then the model will not automatically predict the reverse direction “<description> is <name>”. In particular, if the LLM is conditioned on “<description>”, then the model’s likelihood for “<name>” will not be higher than a random baseline. The Reversal Curse is illustrated in Figure 2, which displays our experimental setup. Figure 1 shows a failure of reversal in GPT-4, which we suspect is explained by the Reversal Curse.
Why does the Reversal Curse matter? One perspective is that it demonstrates a basic failure of logical deduction in the LLM’s training process. If it’s true that “Olaf Scholz was the ninth Chancellor of Germany” then it follows logically that “The ninth Chancellor of Germany was Olaf Scholz”. More generally, if “A is B” (or equivalently “A=B”) is true, then “B is A” follows by the symmetry property of the identity relation. A traditional knowledge graph respects this symmetry property. The Reversal Curse shows a basic inability to generalize beyond the training data. Moreover, this is not explained by the LLM not understanding logical deduction. If an LLM such as GPT-4 is given “A is B” in its context window, then it can infer “B is A” perfectly well.
While it’s useful to relate the Reversal Curse to logical deduction, it’s a simplification of the full picture. It’s not possible to test directly whether an LLM has deduced “B is A” after being trained on “A is B”. LLMs are trained to predict what humans would write and not what is true. So even if an LLM had inferred “B is A”, it might not “tell us” when prompted. Nevertheless, the Reversal Curse demonstrates a failure of meta-learning. Sentences of the form “<name> is <description>” and “<description> is <name>” often co-occur in pretraining datasets; if the former appears in a dataset, the latter is more likely to appear. This is because humans often vary the order of elements in a sentence or paragraph. Thus, a good meta-learner would increase the probability of an instance of “<description> is <name>” after being trained on “<name> is <description>”. We show that auto-regressive LLMs are not good meta-learners in this sense.
Contributions: Evidence for the Reversal Curse
We show LLMs suffer from the Reversal Curse using a series of finetuning experiments on synthetic data. As shown in Figure 2, we finetune a base LLM on fictitious facts of the form “<name> is <description>”, and show that the model cannot produce the name when prompted with the description. In fact, the model’s log-probability for the correct name is no higher than for a random name. Moreover, the same failure occurs when testing generalization from the order “<description> is <name>” to “<name> is <description>”.
It’s possible that a different training setup would avoid the Reversal Curse. We try different setups in an effort to help the model generalize. Nothing helps. Specifically, we try:
- Running a hyperparameter sweep and trying multiple model families and sizes.
- Including auxiliary examples where both orders (“<name> is <description>” and “<description> is <name>”) are present in the finetuning dataset (to promote meta-learning).
- Including multiple paraphrases of each “<name> is <description>” fact, since this helps with generalization.
- Changing the content of the data into the format “<question>? <answer>” for synthetically generated questions and answers.
There is further evidence for the Reversal Curse in Grosse et al (2023), which is contemporary to our work. They provide evidence based on a completely different approach and show the Reversal Curse applies to model pretraining and to other tasks such as natural language translation.
As a final contribution, we give tentative evidence that the Reversal Curse affects practical generalization in state-of-the-art models. We test GPT-4 on pairs of questions like “Who is Tom Cruise’s mother?” and “Who is Mary Lee Pfeiffer’s son?” for different celebrities and their actual parents. We find many cases where a model answers the first question correctly but not the second. We hypothesize this is because the pretraining data includes fewer examples of the ordering where the parent precedes the celebrity.
Our result raises a number of questions. Why do models suffer the Reversal Curse? Do non-auto-regressive models suffer from it as well? Do humans suffer from some form of the Reversal Curse? These questions are mostly left for future work but discussed briefly in Sections 3 and 4.
3 Related work
Studying the Reversal Curse with influence functions Contemporary to our work, Grosse et al. (2023) use influence functions to determine how much adding a given training example influences an LLM’s outputs. They study auto-regressive pretrained LLMs of up to 52B parameters. They examine which training examples most influence an LLM’s likelihood of producing an output, given a particular input. For instance, given the input A, what most influences the likelihood of B? In their experiments, training examples that match the order (“A precedes B”) are far more influential than examples with reverse order (“B precedes A”). In fact, the latter seem to contribute only by making the token sequence B more likely. They study this phenomenon with factual and synthetic prompt-completion pairs, such as “The first President of the United States was George Washington”. These pairs are very similar to those we study in Experiments 1 and 2. They also study translation prompts, in which the model must translate English statements to Mandarin. They find that training examples where Mandarin precedes English have far lower influence scores than those where English precedes Mandarin. Grosse et al. (2023) provide complementary evidence for the Reversal Curse. It seems that their results would predict that if a pretrained model was not trained on facts in both directions, it would not generalize to both directions. Our Experiment 1 tests and confirms a closely related prediction. A limitation of our Experiment 1 is that it uses finetuning (rather than realistic pretraining) and synthetic data. (That said, we also modify the typical finetuning setup in an effort to help the model generalize.) A limitation of Grosse et al. (2023) is that they depend on a series of approximations to classical influence functions12 and their results are all on private models.
Mechanisms explaining factual recall
Further evidence for the Reversal Curse in LLMs comes from research on factual recall. Meng et al. (2023) use a model editing technique to modify factual associations. They find their method is not bidirectional, suggesting that LLMs may store factual associations differently depending on their direction. Complementing this, Geva et al. (2021, 2022, 2023) analyze the internal mechanisms behind factual recall in Transformers. They claim that these models represent factual associations as key-value pairs in their feed-forward layers. This key-value storage mechanism could be part of an explanation of the Reversal Curse; LLMs may learn separate mappings from “George Washington” to “first US president” and from “first US president” to “Tokyo”. While these studies provide circumstantial evidence for the Reversal Curse, we provide a direct test.
Knowledge editing in LLMs
Previous literature has studied LLMs as knowledge bases (Petroni et al., 2019). In §2.1, we aim to extend LLM knowledge bases through finetuning, as in Zhu et al. (2020). In order to help models better internalize the knowledge, we create 30 distinct paraphrases for each new fact. In previous research (Berglund et al., 2023), we found that such augmentation can lead to robust downstream inferences. Similar approaches are used in the model augmentations literature (Sennrich et al., 2016; Cai et al., 2020; Kobayashi, 2018; Eldan & Li, 2023). Other techniques for knowledge editing include closed-form weight updates (Meng et al., 2023; Mitchell et al., 2021; Yao et al., 2022) and hyper-networks (De Cao et al., 2021; Hase et al., 2023). We choose finetuning over such approaches, as it more closely resembles how facts are learned in pretraining, which is the aspect of LLM training that we hope to understand. Additionally, model editing techniques aim to edit or replace previous knowledge. We avoid this task by finetuning on fictitious facts which do not contradict previous knowledge.
Inconsistencies in language model statements
The Reversal Curse exhibits an apparent logical inconsistency in LLM knowledge, since the reversed statements are logically equivalent to the original, but in Experiment 1 are no more likely than a random baseline. Other inconsistencies are studied in (Fluri et al., 2023). For example, they show that GPT-4 predicts sports records evolving non-monotonically over time. Additionally, Hosseini et al. (2021) show that LLMs handle negations of statements incorrectly, Lin et al. (2022) show that models will sometimes output falsehoods despite having the capacity to answer statements correctly, and Shi et al. (2023) show that language models can be distracted by irrelevant text in their context.
Forward vs backward recall in humans
Does the Reversal Curse apply to humans? Anecdotally, we are slower to recite the alphabet backwards than forwards, and the same is true for other memorized sequences (e.g. poems).Indeed, our findings mirror a well-studied effect in humans, wherein recall is harder in the backward direction than in the forward direction (Clair-Thompson & Allen, 2013; Thomas et al., 2003; Bireta et al., 2010; Li & Lewandowsky, 1995; Guitard et al., 2019). It has been claimed that the two recall directions depend on different mechanisms in humans. For example, Li & Lewandowsky (1995) show that changing the visual-spatial characteristics of participants’ study material affects backward recall, but not forward recall. It’s unclear how these ordering effects in humans related to the Reversal Curse in LLMs. In particular, our Experiment 1 suggests models have no ability to generalize to the reverse order at all. We do not know of such stark ordering effects in humans.
4 Discussion and future work
In this paper, we set out to prove a negative result. Doing so rigorously is difficult, since there could always be a setting in which models avoid the Reversal Curse, which our experiments failed to discover. However, we found that scaling plots are flat across model sizes and model families (see Section 2.1). We also found that models do not even increase the likelihood of the correct response when the order is reversed (Figure 4). Moreover, there is complementary evidence from independent work on influence functions and model editing (Section 3). What would explain the Reversal Curse in auto-regressive LLMs? We mostly leave this for future work. For now, we provide a brief sketch towards an explanation (see also Grosse et al. (2023)). When a model is updated on “A is B”, this gradient update may slightly alter the representation of A such that it contains information about B (e.g. in the middle MLP layers as per Geva et al. (2022, 2023)). It would make rational sense for this gradient update to also alter the representation of B to contain information about A. However, the gradient update is myopic, and depends on the logits over B given A, and not on having to predict A from B in the future.
4.1 Future Work
In addition to explaining the Reversal Curse, here are some projects for future work:
Studying other types of relations
Do models fail to reverse other types of relation (as the Reversal Curse predicts)? These could include logical implications (e.g. “X implies Y” and “Not X implies not Y.”), spatial relationships (e.g. “The cup is on the table” and “The table is under the cup.”), or n-place relations (e.g. “Alice, Bob, Carol and Dan are in the same group.”)
Finding reversal failures via entity-linking
Kandpal et al. (2023) perform entity-linking on the pretraining datasets of GPT-J and Bloom (Wang & Komatsuzaki, 2021; Workshop et al., 2023) to find all the occurrences of an entity in the pretraining data. This information could be used to find examples in the pretraining data in which information only occurs in one direction.
Analyzing the practical impact of the Reversal Curse
The pretraining sets for modern LLMs are very large and diverse. Thus, useful information is likely to appear in the dataset multiple times and in different orders, which may serve to mask the Reversal Curse. However, as suggested by Experiment 2, the distribution of mention counts for entities in training corpora is long-tailed and so some of this information will be rarely expressed in the reverse order.
Links
Paper: https://owainevans.github.io/reversal_curse.pdf
Code and datasets: https://github.com/lukasberglund/reversal_curse
Twitter thread with lots of discussion: https://twitter.com/OwainEvans_UK/status/1705285631520407821
Top Discussion
I like this paper for crisply demonstrating an instance of poor generalization in LMs that is likely representative of a broader class of generalization properties of current LMs.
The existence of such limitations in current ML systems does not imply that ML is fundamentally not a viable path to AGI, or that timelines are long, or that AGI will necessarily also have these limitations. Rather, I find this kind of thing interesting because I believe that understanding limitations of current AI systems is very important for giving us threads to yank on that may help us with thinking about conceptual alignment. Some examples of what I mean:
- It's likely that our conception of the kinds of representations/ontology that current models have are deeply confused. For example, one might claim that current models have features for "truth" or "human happiness", but it also seems entirely plausible that models instead have separate circuits and features entirely for "this text makes a claim that is incorrect" and "this text has the wrong answer selected", or in the latter case for "this text has positive sentiment" and "this text describes a human experiencing happiness" and "this text describes actions that would cause a human to be happy if they were implemented".
- I think we're probably pretty confused about mesaoptimization, in a way that's very difficult to resolve just by thinking more about it (source: have spent a lot of time thinking about mesaoptimizers). I think this is especially salient to the people trying to make model organisms--which I think is a really exciting avenue--because if you try to make a mesaoptimizer, you immediately collide head on with things like finding that "training selects from the set of goals weighted by complexity" hypothesis doesn't seem to accurately describe current model training. I think it's appropriate to feel pretty confused about this and carefully examine the reasons why current models don't exhibit these properties. It's entirely reasonable for the answer to be "I expect future models to have thing X that current models don't have" - then, you can try your best to test various X's before having the future AIs that actually kill everyone.
- There are some things that we expect AGI to do that current ML systems do not do. Partly this will be because in fact current ML systems are not analogous to future AGI in some ways - probably if you tell the AGI that A is B, it will also know that B is A. This does not necessarily have to be a property that gradually emerges and can be forecasted with a scaling law; it could emerge in a phase change, or be the result of some future algorithmic innovation. If you believe there is some property X of current ML that causes this failure, and that it will be no longer a failure in the future, then you should also be suspicious of any alignment proposal that depends on this property (and the dependence of the proposal on X may be experimentally testable). For instance, it is probably relatively easy to make an RL trained NN policy be extremely incoherent in a small subset of cases, because the network has denormalized contextual facts that are redundant across many situations. I expect this to probably be harder in models which have more unified representations for facts. To the extent I believe a given alignment technique works because it leverages this denormalization, I would be more skeptical of it working in the future.
- As a counterpoint, it might also be that we had an inaccurate conception of what capabilities AGI will have that current ML systems do not have - I think one important lesson of GPT-* has been that even with these failures, the resulting systems can still be surprisingly useful.
[-]Owain_Evans4d93
Great comment. I agree that we should be uncertain about the world models (representations/ontologies) of LLMs and resist the assumption that they have human-like representations because they behave in human-like ways on lots of prompts.
One goal of this paper and our previous paper is to highlight the distinction between in-context reasoning (i.e. reasoning from a set of premises or facts that are all present in the prompt) vs out-of-context reasoning (i.e. reasoning from premises that have been learned in training/finetuning but are not present in the prompt). Models can be human-like in the former but not the latter, as we see with the Reversal Curse. (Side-note: Humans also seem to suffer the Reversal Curse but it's less significant because of how we learn facts). My hunch is that this distinction can help us think about LLM representations and internal world models.
[-]Sune3d177
This seems like the kind of research that can have a huge impact on capabilities, and much less and indirect impact on alignment/safety. What is your reason for doing it and publishing it?
[-]Vivek Hebbar1d60
What's "denormalization"?
[-]johnswentworth1d90
In database design, sometimes you have a column in one table whose entries are pointers into another table - e.g. maybe I have a Users table, and each User has a primaryAddress field which is a pointer into an Address table. That keeps things relatively compact and often naturally represents things - e.g. if several Users in a family share a primary address, then they can all point to the same Address. The Address only needs to be represented once (so it's relatively compact), and it can also be changed once for everyone if that's a thing someone wants to do (e.g. to correct a typo). That data is called "normalized".
But it's also inefficient at runtime to need to follow that pointer and fetch data from the second table, so sometimes people will "denormalize" the data - i.e. store the whole address directly in the User table, separately for each user. Leo's using that as an analogy for a net separately "storing" versions of the "same fact" for many different contexts.
[-]leogao14h31
I meant it as an analogy to https://en.m.wikipedia.org/wiki/Denormalization
Mesa-optimization
short version: Mesa-Optimization - AI Alignment Forum
[AN #58] Mesa optimization: what it is, and why we should care — LessWrong
Highlights
Risks from Learned Optimization in Advanced Machine Learning Systems (Evan Hubinger et al): Suppose you search over a space of programs, looking for one that plays TicTacToe well. Initially, you might find some good heuristics, e.g. go for the center square, if you have two along a row then place the third one, etc. But eventually you might find the minimax algorithm, which plays optimally by searching for the best action to take. Notably, your outer optimization over the space of programs found a program that was itself an optimizer that searches over possible moves. In the language of this paper, the minimax algorithm is a mesa optimizer: an optimizer that is found autonomously by a base optimizer, in this case the search over programs.
Why is this relevant to AI? Well, gradient descent is an optimization algorithm that searches over the space of neural net parameters to find a set that performs well on some objective. It seems plausible that the same thing could occur: gradient descent could find a model that is itself performing optimization. That model would then be a mesa optimizer, and the objective that it optimizes is the mesa objective. Note that while the mesa objective should lead to similar behavior as the base objective on the training distribution, it need not do so off distribution. This means the mesa objective is pseudo aligned; if it also leads to similar behavior off distribution it is robustly aligned.
A central worry with AI alignment is that if powerful AI agents optimize the wrong objective, it could lead to catastrophic outcomes for humanity. With the possibility of mesa optimizers, this worry is doubled: we need to ensure both that the base objective is aligned with humans (called outer alignment) and that the mesa objective is aligned with the base objective (called inner alignment). A particularly worrying aspect is deceptive alignment: the mesa optimizer has a long-term mesa objective, but knows that it is being optimized for a base objective. So, it optimizes the base objective during training to avoid being modified, but at deployment when the threat of modification is gone, it pursues only the mesa objective.
As a motivating example, if someone wanted to create the best biological replicators, they could have reasonably used natural selection / evolution as an optimization algorithm for this goal. However, this then would lead to the creation of humans, who would be mesa optimizers that optimize for other goals, and don't optimize for replication (e.g. by using birth control).
The paper has a lot more detail and analysis of what factors make mesa-optimization more likely, more dangerous, etc. You'll have to read the paper for all of these details. One general pattern is that, when using machine learning for some task X, there are a bunch of properties that affect the likelihood of learning heuristics or proxies rather than actually learning the optimal algorithm for X. For any such property, making heuristics/proxies more likely would result in a lower chance of mesa-optimization (since optimizers are less like heuristics/proxies), but conditional on mesa-optimization arising, makes it more likely that it is pseudo aligned instead of robustly aligned (because now the pressure for heuristics/proxies leads to learning a proxy mesa-objective instead of the true base objective).
Rohin's opinion: I'm glad this paper has finally come out. The concepts of mesa optimization and the inner alignment problem seem quite important, and currently I am most worried about x-risk caused by a misaligned mesa optimizer. Unfortunately, it is not yet clear whether mesa optimizers will actually arise in practice, though I think conditional on us developing AGI it is quite likely. Gradient descent is a relatively weak optimizer; it seems like AGI would have to be much more powerful, and so would require a learned optimizer (in the same way that humans can be thought of as "optimizers learned by evolution").
There still is a lot of confusion and uncertainty around the concept, especially because we don't have a good definition of "optimization". It also doesn't help that it's hard to get an example of this in an existing ML system -- today's systems are likely not powerful enough to have a mesa optimizer (though even if they had a mesa optimizer, we might not be able to tell because of how uninterpretable the models are).
Read more: Alignment Forum version
相关文章:
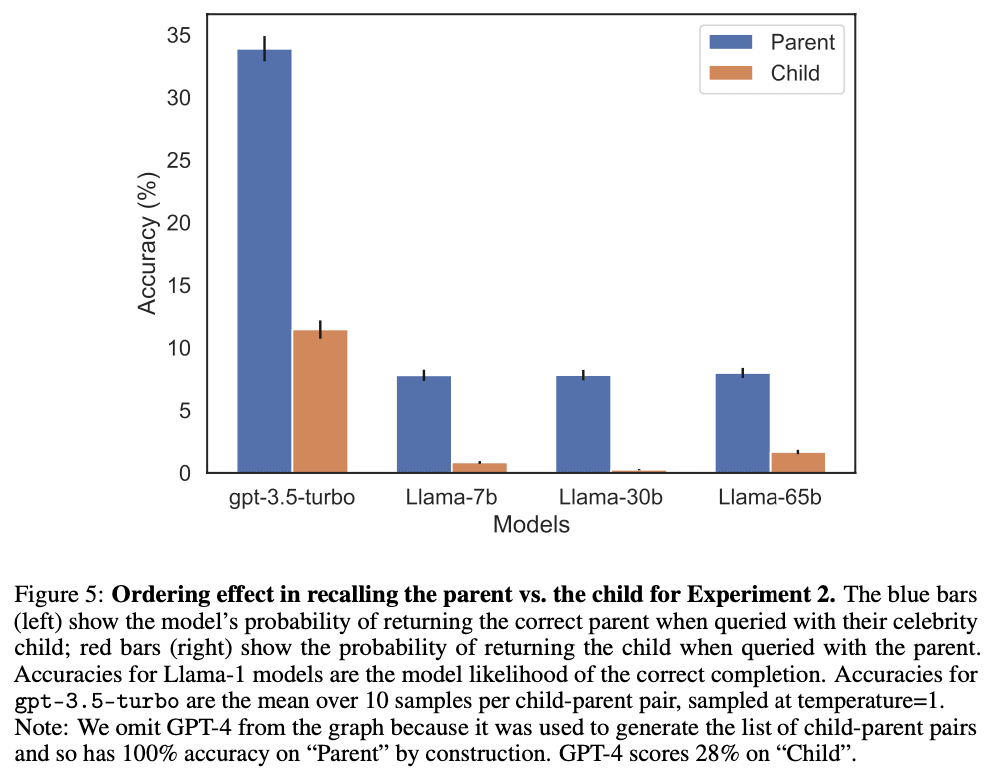
The Reversal Curse: LLMs trained on “A is B“ fail to learn “B is A“
(not an original, only classified as one to avoid cramming reference links) paper: https://owainevans.github.io/reversal_curse.pdf blog with interactions with the authors: Paper: LLMs trained on “A is B” fail to learn “B is A” — LessWrong This is a…...

专栏更新情况:华为流程、产品经理、战略管理、IPD
目录 前言 01 华为流程体系入门课 CSDN学院 02 产品经理进阶课 CSDN学院 03 BLM 战略方法论进阶课 04 IPD 进阶 100 例专栏 作者简介 前言 已上线四大课程专栏更新情况: 01 华为流程体系入门课(视频图文); 02 硬件产品经…...
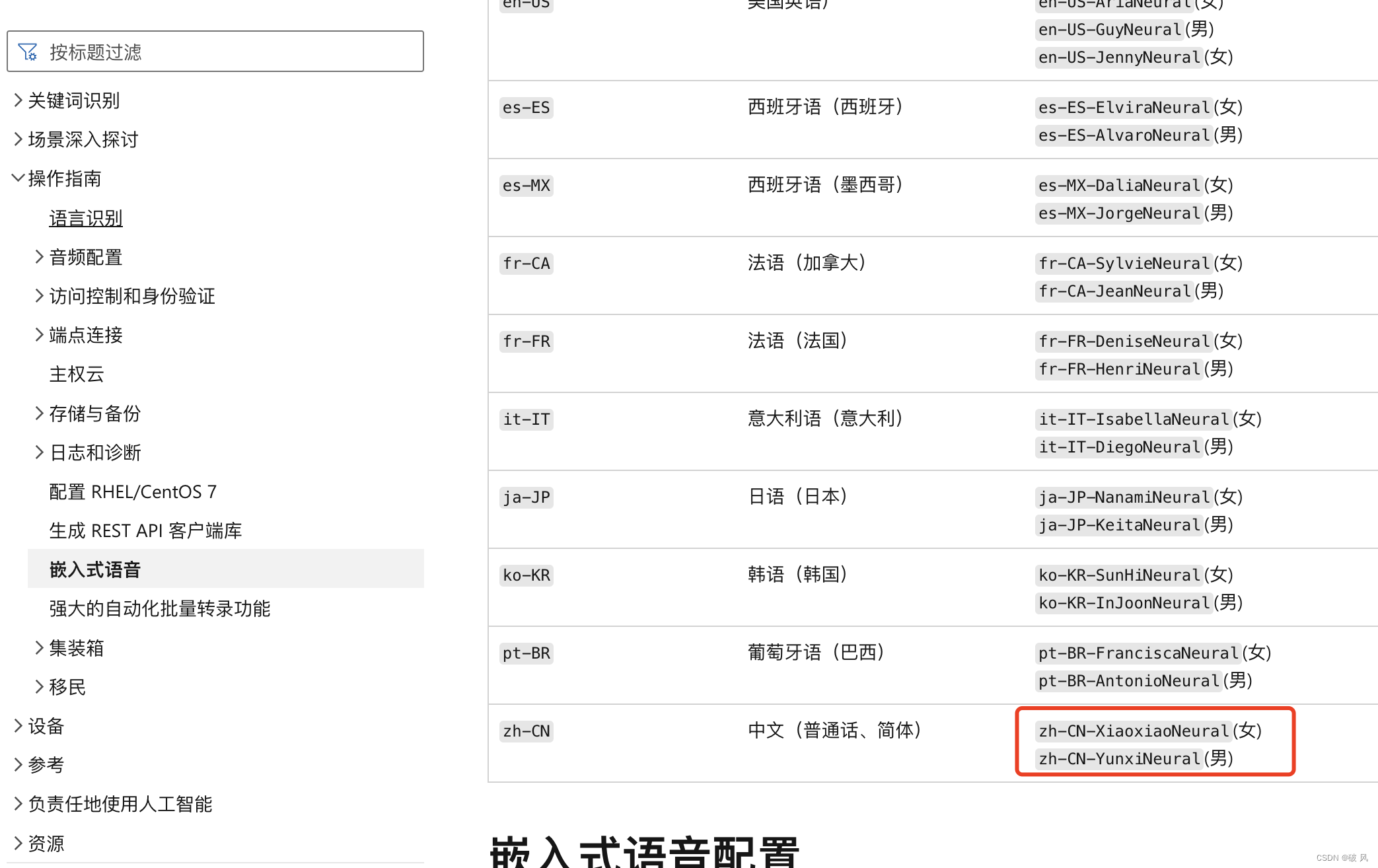
微软(TTS)文本转语音服务API实现
此博客实现与java实现微软文本转语音(TTS)经验总结_java tts_${简简单单}的博客-CSDN博客之上,首先感谢博客源码的提供,本人在上面添加了一些详细的注释,方便大家跟好的理解和使用,毕竟我已经用原文调试了一…...

防火墙firewalld
title: 防火墙firewalld createTime: 2020-10-29 18:05:52 updateTime: 2020-10-29 18:05:52 categories: linux tags: centos7上的firewalld 的使用 一、firewalld的基本启动关闭命令 启动服务------systemctl start firewalld关闭服务------systemctl stop firewalld查看状…...
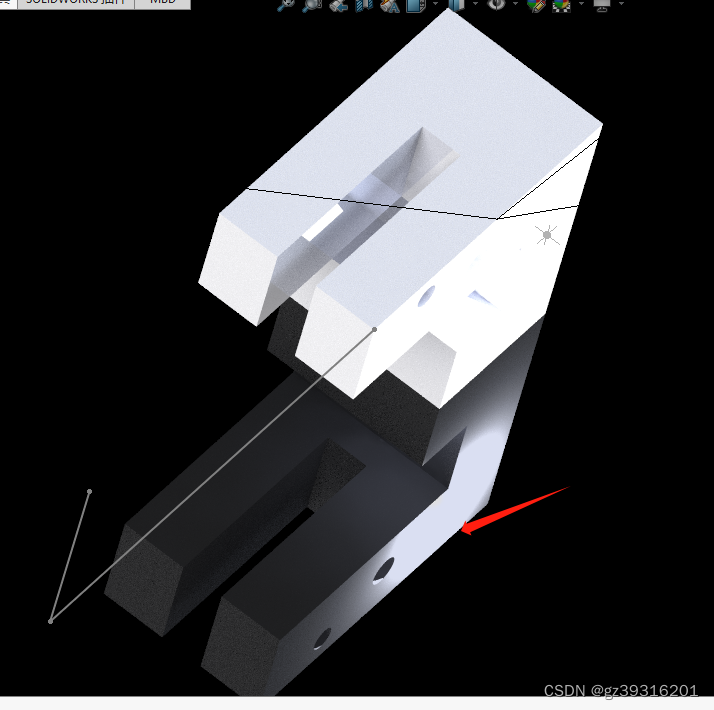
SW线光源是真实的(点光源)
点光源在渲染下真实 点光源地板反射是对的...

Vue Router的安装
安装 在我们使用脚手架搭建项目的时候,默认是没有帮我们安装的。需要自己手动进行安装。安装的 Vue-Router 插件时需要注意版本信息,Vue2.0 使用的是 Vue-Router3.x ,而 Vue3.0 使用的是 Vue-Router4.x。 通过命令安装 vue-router3 插件 $…...
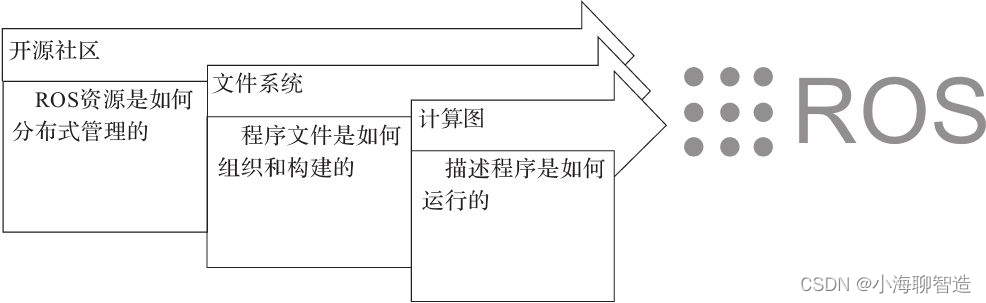
ROS架构设计
ROS架构如图所示,可以将其分为三个层次:OS层、中间层和应用层。 1.OS层 ROS并不是一个传统意义上的操作系统,无法像Windows、Linux一样直接运行在计算机硬件之上,而是需要依托于Linux系统。所以在OS层,我们可以直接使…...

JSON.toJSONString() 解析之后 出现“$ref“:“$[x].xxx“
原因:JSON在处理数据时出现了相同数据,JSON自动将相同节点的数据使用引用方式代替。 解决方式: String jsonString JSON.toJSONString(params, SerializerFeature.DisableCircularReferenceDetect); SerializerFeature.DisableCircularRefer…...

2023研究生数学建模E题思路+模型+代码+论文(持续更新中) 出血性脑卒中临床智能诊疗建模
目录 E题思路 出血性脑卒中临床智能诊疗建模 完整思路代码模型论文获取见文末名片 完整思路代码模型论文获取见此 E题思路 出血性脑卒中临床智能诊疗建模 完整思路代码模型论文获取见文末名片 一、 背景介绍 出血性脑卒中指非外伤性脑实质内血管破裂引起的脑出血࿰…...
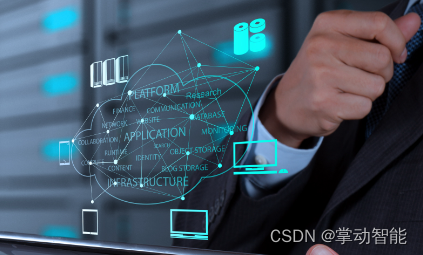
云可观测性安全平台——掌动智能
云可观测性安全平台是一个跨架构、跨平台的可观测性方案,实现对云环境下的细粒度数据可视化,满足安全部门对云内部安全领域的多场景诉求,包括敏感数据动态监管、云网攻击回溯分析、攻击横移风险监控、云异常流量分析。本文将介绍掌动智能云可…...

[ruby on rails] postgres sql explain 优化
一、查看执行计划 sql User.all.to_sql # 不会实际执行查询 puts ActiveRecord::Base.connection.explain(sql)# 会实际执行查询,再列出计划 User.all.explain# 会实际执行查询,再列出计划ActiveRecord::Base.connection.execute(EXPLAIN ANALYZE sql…...
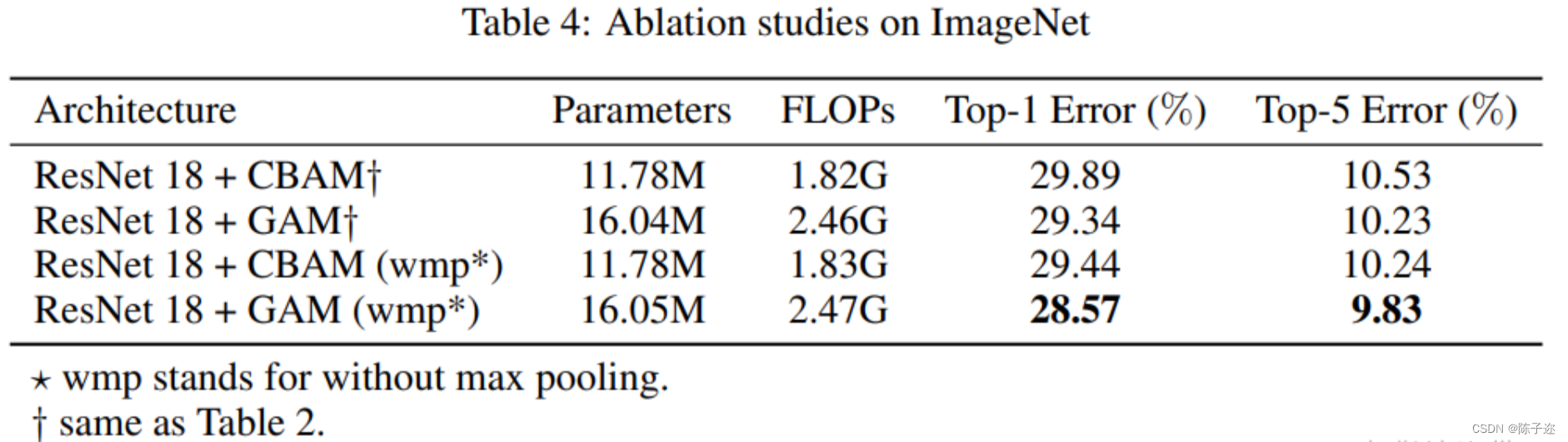
YOLOv7改进:GAMAttention注意力机制
1.背景介绍 为了提高各种计算机视觉任务的性能,人们研究了各种注意机制。然而,以往的方法忽略了保留通道和空间方面的信息以增强跨维度交互的重要性。因此,我们提出了一种全局调度机制,通过减少信息缩减和放大全局交互表示来提高深…...
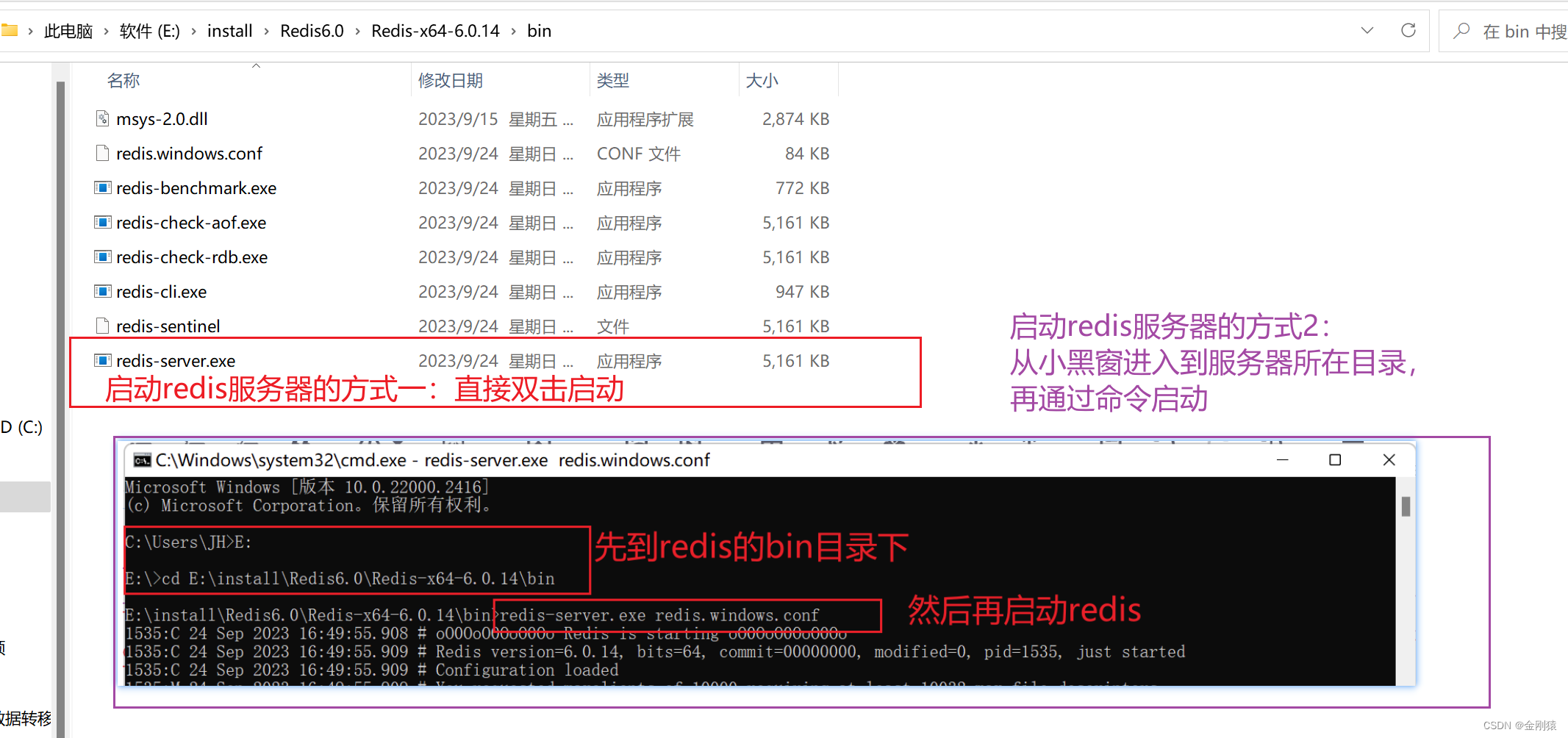
83、SpringBoot --- 下载和安装 MSYS2、 Redis
启动redis服务器: 打开小黑窗: C:\Users\JH>e: E:>cd E:\install\Redis6.0\Redis-x64-6.0.14\bin E:\install\Redis6.0\Redis-x64-6.0.14\bin>redis-server.exe redis.windows.conf 启动redis客户端: 小黑窗:redis-cli …...
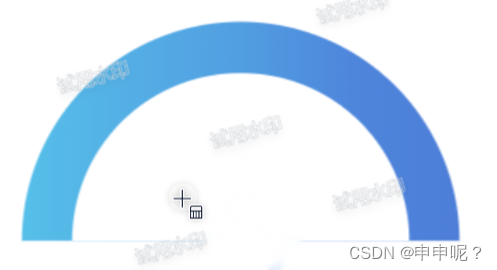
用css画一个半圆弧(以小程序为例)
一、html结构 圆弧的html结构是 两个块级元素嵌套。 <View classNamewrap><View className"inner">{/* 图标下的内容 */}</View></View>二、css样式:原理是两个半圆叠在一起,就是一个半圆弧。那么,如何画一…...
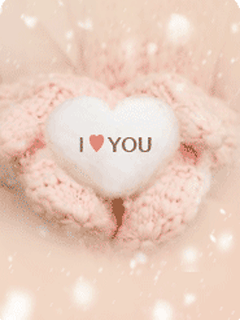
redis介绍
一、简介 Redis 与其他 key - value 缓存产品有以下三个特点: Redis支持数据的持久化,可以将内存中的数据保存在磁盘中,重启的时候可以再次加载进行使用。 Redis不仅仅支持简单的key-value类型的数据,同时还提供list,…...
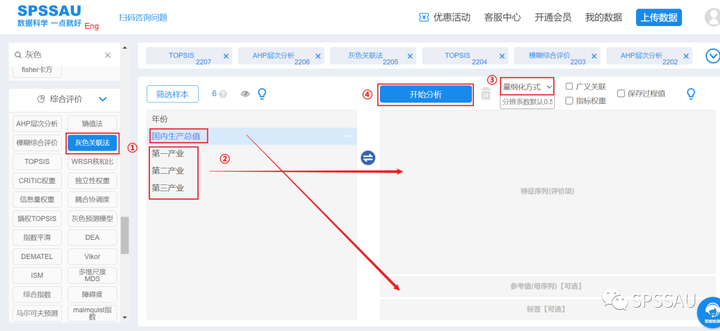
数学建模常用模型
作为数学建模的编程手还掌握一些各类模型常用算法,数学建模评价类模型、分类模型、预测类模型比较常用的方法总结如下: 接下来对这些比较典型的模型进行详细进行介绍说明。 一、评价模型 在数学建模中,评价模型是比较基础的模型之一&#x…...
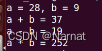
Linux 基本语句_5_创建静态库|动态库
静态库 创建主函数:main.c 应用函数:add.c、sub.c、mul.c 创建calc.h文件作为头文件 生成可执行文件*.o文件 gcc -c add.c -o add.o ....包装*.o文件为静态库 ar -rc libmymath.a add.o sub.o mul.o编译静态库并指明创建静态库的位置 sudo gcc mai…...
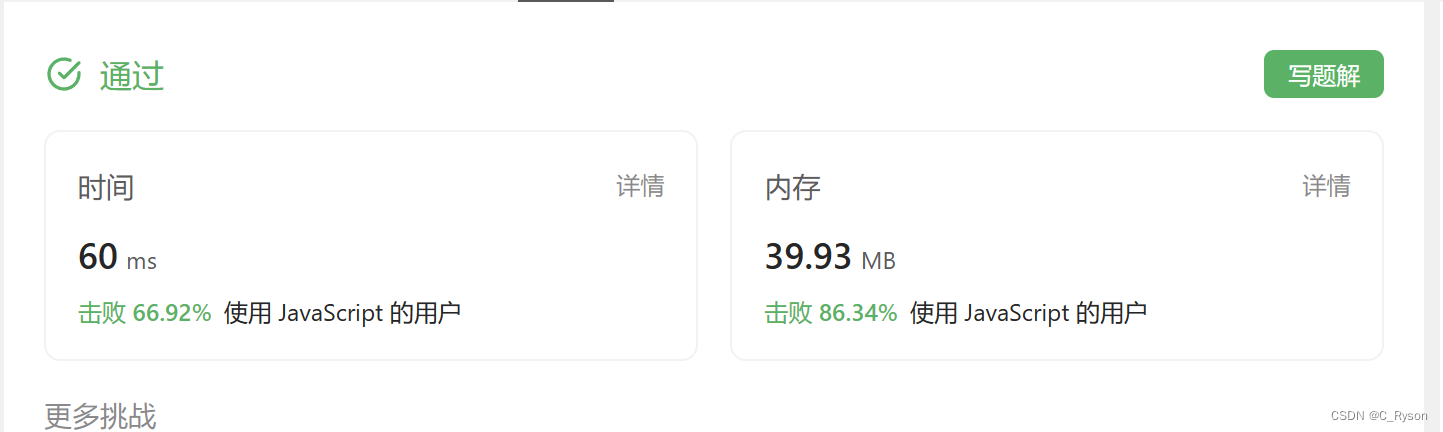
【每日一题】2703. 返回传递的参数的长度
2703. 返回传递的参数的长度 - 力扣(LeetCode) 请你编写一个函数 argumentsLength,返回传递给该函数的参数数量。 示例 1: 输入:args [5] 输出:1 解释: argumentsLength(5); // 1只传递了一个值…...
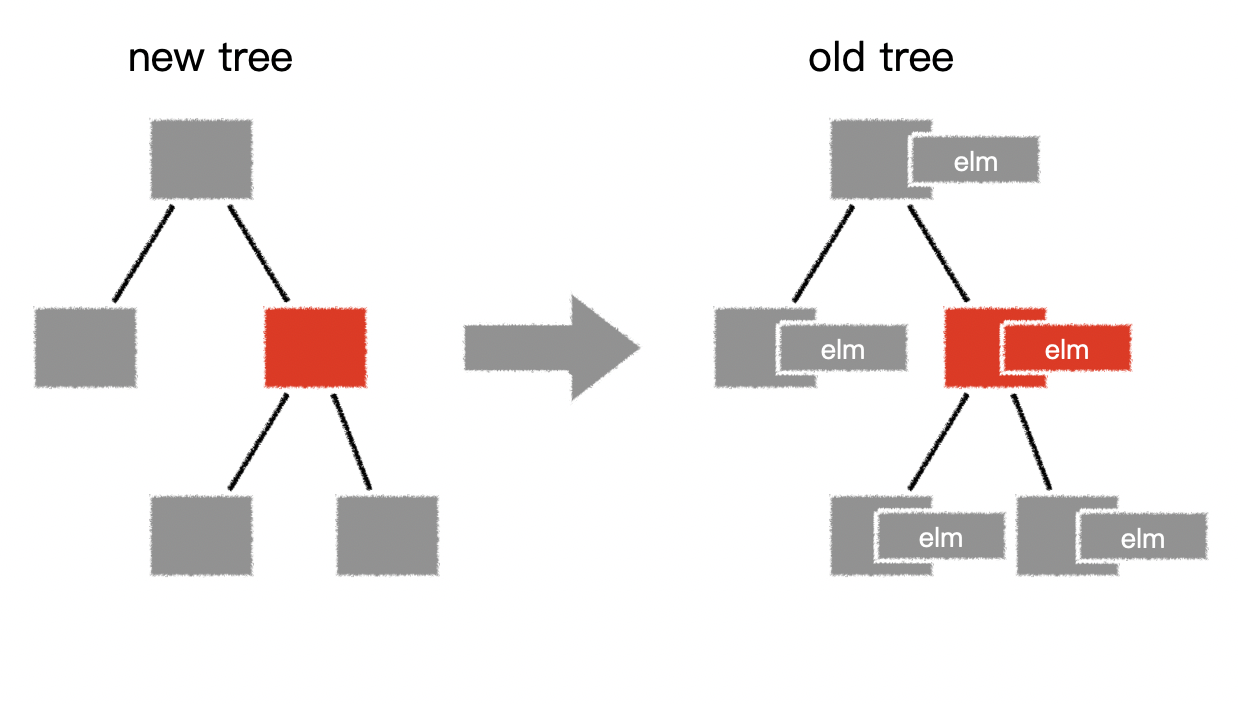
虚拟DOM详解
面试题:请你阐述一下对vue虚拟dom的理解 什么是虚拟dom? 虚拟dom本质上就是一个普通的JS对象,用于描述视图的界面结构 在vue中,每个组件都有一个render函数,每个render函数都会返回一个虚拟dom树,这也就意味…...
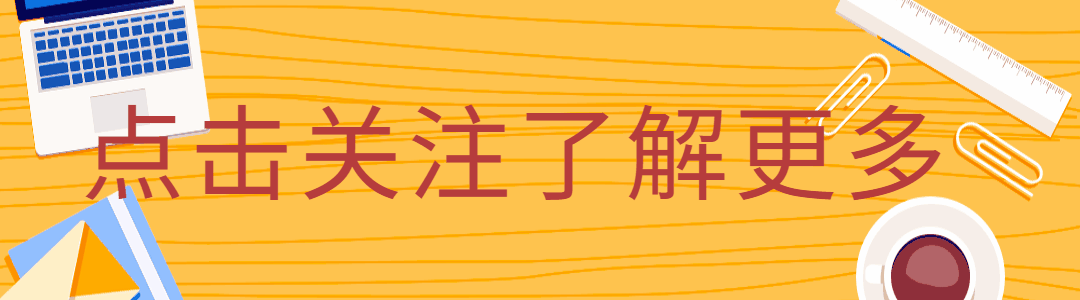
Linux配置命令
一:HCSA-VM-Linux安装虚拟机后的基础命令 1.代码命令 1.查看本机IP地址: ip addr 或者 ip a [foxbogon ~]$ ip addre [foxbogon ~]$ ip a 1:<Loopback,U,LOWER-UP> 为环回2网卡 2: ens160: <BROADCAST,MULTICAST,UP,LOWER_UP&g…...
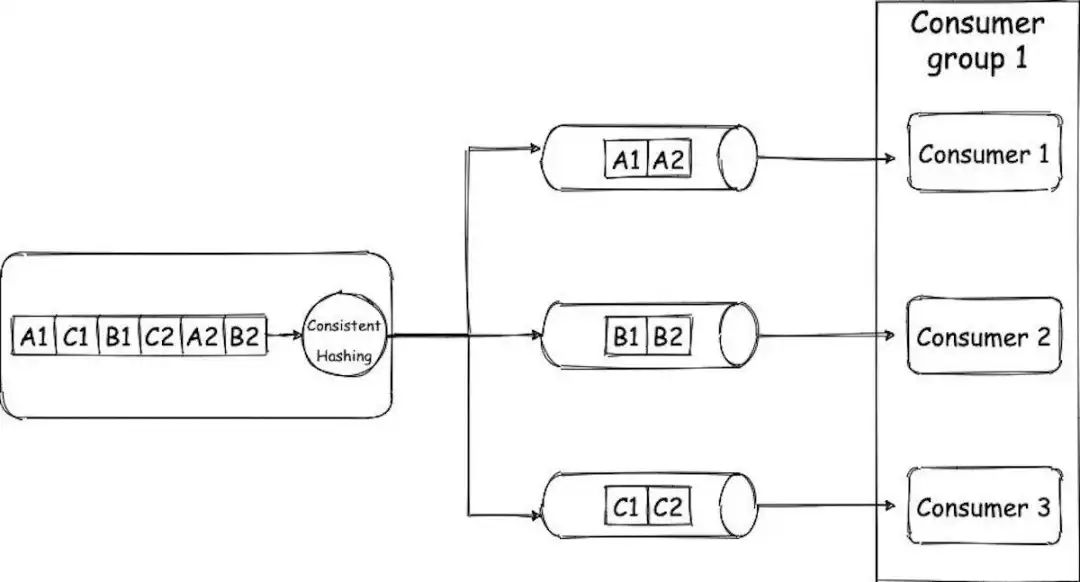
Kafka:介绍和内部工作原理
展示Kafka工作方式的简单架构。 什么是Kafka?为什么我们要使用它?它是消息队列吗? 它是一个分布式流处理平台或分布式提交日志。 Kafka通常用于实时流数据管道,即在系统之间传输数据,构建不断流动的数据转换系统和构…...

在 EMR Serverless 上使用 Delta Lake
本文是一份开箱即用的全自动测试脚本,用于在 EMR Serverless 上提交一个 Delta Lake 作业。本文完全遵循《最佳实践:如何优雅地提交一个 Amazon EMR Serverless 作业?》 一文给出的标准和规范! 1. 导出环境相关变量 注意&#x…...

Stream流的使用详解(持续更新)
1. 对比两List集合数据某些字段一样的情况下取值: 一般简单方式我们会使用双重for循环来处理判断数据取值(如下代码所示),但是数据量越大的情况下代码效率则越低,并且现在很多公司都会限制for循环层数所以更推荐strea…...

golang工程——gRpc 拦截器及原理
oauth2认证与拦截器 类似java spring中的拦截器。gRpc也有拦截器的说法,拦截器可作用于客户端请求,服务端请求。对请求进行拦截,进行业务上的一些封装校验等,类似一个中间件的作用 拦截器类型 一元请求拦截器流式请求拦截器链式…...
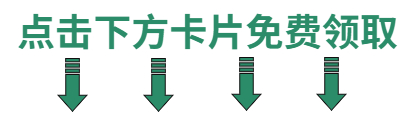
Python接口自动化之unittest单元测试
以下主要介绍unittest特性、运行流程及实际案例。 一、单元测试三连问 1、什么是单元测试? 按照阶段来分,一般就是单元测试,集成测试,系统测试,验收测试。单元测试是对单个模块、单个类或者单个函数进行测试。 将访…...
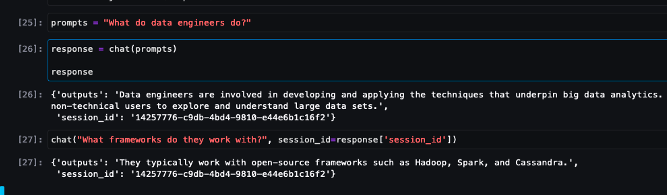
在亚马逊云科技Amazon SageMaker上部署构建聊天机器人的开源大语言模型
开源大型语言模型(LLM)已经变得流行起来,研究人员、开发人员和组织都可以使用这些模型来促进创新和实验。这促进了开源社区开展合作,从而为LLM的开发和改进做出贡献。开源LLM提供了模型架构、训练过程和训练数据的透明度ÿ…...

【51单片机】10-蜂鸣器
1.蜂鸣器的原理 这里的“源”不是指电源。而是指震荡源。 也就是说,有源蜂鸣器内部带震荡源,所以只要一通电就会叫。 而无源内部不带震荡源,所以如果用直流信号无法令其鸣叫。必须用2K~5K的方波去驱动它。 有源蜂鸣器往往比无源的贵ÿ…...

26377-2010 逆反射测量仪 知识梳理
声明 本文是学习GB-T 26377-2010 逆反射测量仪. 而整理的学习笔记,分享出来希望更多人受益,如果存在侵权请及时联系我们 1 范围 本标准规定了逆反射测量仪的术语和定义、结构与分类、技术要求、计量学特性、试验方法、检验规 则以及标志、包装、运输与贮存。 本标准适用于…...
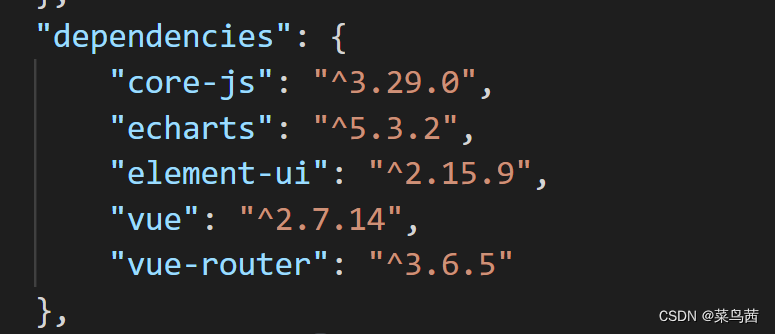
css实现渐变电量效果柱状图
我们通常的做法就是用echarts来实现 比如 echarts象形柱图实现电量效果柱状图 接着我们实现进阶版,增加渐变效果 echarts分割柱形图实现渐变电量效果柱状图 接着是又在渐变的基础上,增加了背景色块的填充 echarts实现渐变电量效果柱状图 其实思路是一…...
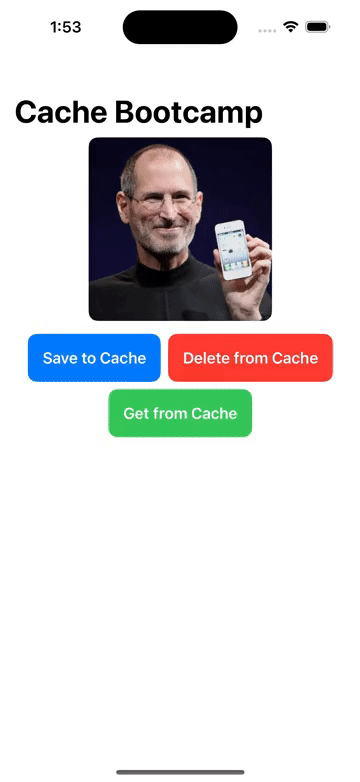
FileManager/本地文件增删改查, Cache/图像缓存处理 的操作
1. FileManager 本地文件管理器,增删改查文件 1.1 实现 // 本地文件管理器 class LocalFileManager{// 单例模式static let instance LocalFileManager()let folderName "MyApp_Images"init() {createFolderIfNeeded()}// 创建特定应用的文件夹func cr…...

最牛黑客做的白粉交易网站/google官方下载安装
Matlab求解方同轴波导的截止波长和特性阻抗作者:金静逯迈陈小强来源:《现代电子技术》2008年第03期摘要:采用有限差分法利用Matlab求解了方同轴波导的特性阻抗和高次模的…...
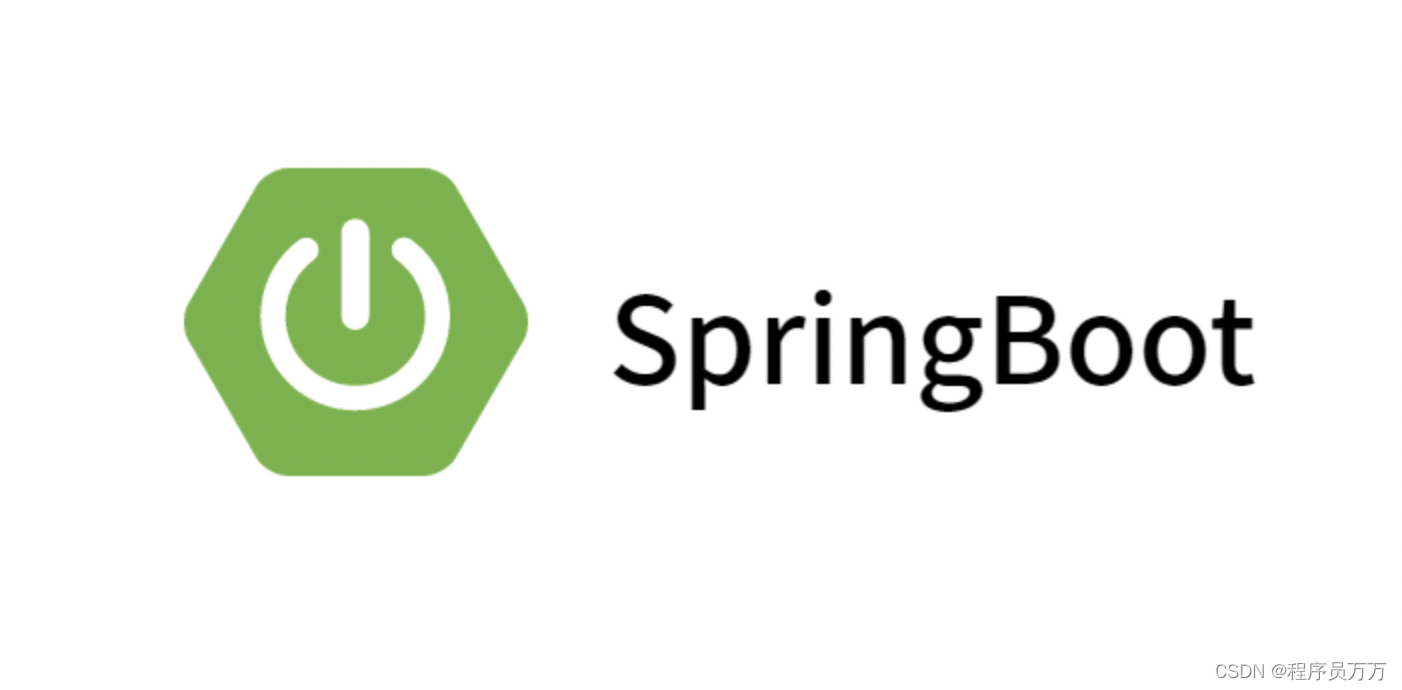
帝国网站模版/百度广告投放电话
当今,Spring Boot成为了Java开发中最受欢迎的技术之一。它是一个快速,开箱即用的开发框架,为Java开发人员提供了一个有效的方法来构建和运行应用程序。在本文中,我们将探讨Spring Boot的一些重要特性和它的优势。 Spring Boot的特…...

网站开发需要什么工具/网店运营是做什么的
本笔记将介绍:如何安装 Certbot 工具;如何使用它获取证书;如何处理在操作过程中遇到的问题。 注意事项 本部分内容属于简述,详细内容请参考 certbot instructions 官方页面,依据提示操作即可。以下是操作大致流程&…...

做移动网站优化首/百度网址大全 旧版本
获取 WinCE 移动设备屏幕旋转方向,分别从系统提供的接口和注册表获取。如果都获取不到,则采用默认值。 1 #ifndef DMDO_ZERO 2 #define DMDO_ZERO 0 3 #endif 4 #ifndef DMDO_90 5 #define DMDO_90 1 6 #endif 7 #ifndef DMDO_180 8 #d…...

网站文案编辑怎么做/爱站网长尾关键词挖掘工具下载
1、linux普通用户默认密码策略:用passwd修改用户密码,常常提示“BAD PASSWORD: it is based on a dictionary word”。实际上linux要求的密码验证机制是在/etc/login.defs中规定最小密码字符数;同时还要受到/etc/pam.d/passwd的限定。但是在r…...

wordpress 新建侧边栏/seo自学网站
中国目前正处于由熟人社会向陌生人社会转型的阶段,陌生人社会带来的孤独和挫折以及文化心理的惯性,让中国人对人情和关系的依赖依然存在,凭借熟人关系开展工作,运用人情关系的法则对抗社会制度和规范的现象依然很普遍。 人情、…...