计算机视觉框架OpenMMLab开源学习(三):图像分类实战
前言:本篇主要偏向图像分类实战部分,使用MMclassification工具进行代码应用,最后对水果分类进行实战演示,本次环境和代码配置部分省略,具体内容建议参考前一篇文章:计算机视觉框架OpenMMLab开源学习(二):图像分类
计算机视觉框架OpenMMLab开源学习(三):图像分类实战
一、安装OpenMMLab v2.0
Step 1. Install MMCV
mim install "mmcv>=2.0.0rc0"
Step 2. Install MMClassification and MMDetection
mim install "mmcls>=1.0.0rc0" "mmdet>=3.0.0rc0"
代码模版讲解:
model = dict(type='ImageClassifier', # 分类器类型backbone=dict(type='ResNet', # 主干网络类型depth=50, # 主干网网络深度, ResNet 一般有18, 34, 50, 101, 152 可以选择num_stages=4, # 主干网络状态(stages)的数目,这些状态产生的特征图作为后续的 head 的输入。out_indices=(3, ), # 输出的特征图输出索引。越远离输入图像,索引越大frozen_stages=-1, # 网络微调时,冻结网络的stage(训练时不执行反相传播算法),若num_stages=4,backbone包含stem 与 4 个 stages。frozen_stages为-1时,不冻结网络; 为0时,冻结 stem; 为1时,冻结 stem 和 stage1; 为4时,冻结整个backbonestyle='pytorch'), # 主干网络的风格,'pytorch' 意思是步长为2的层为 3x3 卷积, 'caffe' 意思是步长为2的层为 1x1 卷积。neck=dict(type='GlobalAveragePooling'), # 颈网络类型head=dict(type='LinearClsHead', # 线性分类头,num_classes=1000, # 输出类别数,这与数据集的类别数一致in_channels=2048, # 输入通道数,这与 neck 的输出通道一致loss=dict(type='CrossEntropyLoss', loss_weight=1.0), # 损失函数配置信息topk=(1, 5), # 评估指标,Top-k 准确率, 这里为 top1 与 top5 准确率))
二、Pytorch图像分类任务
本次任务训练数据为FashionMNIST,完整代码如下:
# https://pytorch.org/tutorials/beginner/basics/quickstart_tutorial.htmlimport torch
from torch import nn
from torch.utils.data import DataLoader
from torchvision import datasets
from torchvision.transforms import ToTensor# Training## Construct Dataset and Dataloader
training_data = datasets.FashionMNIST(root="data",train=True,download=True,transform=ToTensor(),
)
test_data = datasets.FashionMNIST(root="data",train=False,download=True,transform=ToTensor(),
)batch_size = 64train_dataloader = DataLoader(training_data, batch_size=batch_size)
test_dataloader = DataLoader(test_data, batch_size=batch_size)## Define model
class NeuralNetwork(nn.Module):def __init__(self):super(NeuralNetwork, self).__init__()self.flatten = nn.Flatten()self.linear_relu_stack = nn.Sequential(nn.Linear(28*28, 512),nn.ReLU(),nn.Linear(512, 512),nn.ReLU(),nn.Linear(512, 10))def forward(self, x):x = self.flatten(x)logits = self.linear_relu_stack(x)return logitsdevice = "cuda" if torch.cuda.is_available() else "cpu"
model = NeuralNetwork().to(device)## Define loss function and Optimizer
loss_fn = nn.CrossEntropyLoss()
optimizer = torch.optim.SGD(model.parameters(), lr=1e-3)## Inner loop for training
def train(dataloader, model, loss_fn, optimizer):size = len(dataloader.dataset)model.train()for batch, (X, y) in enumerate(dataloader):X, y = X.to(device), y.to(device)# Compute prediction errorpred = model(X)loss = loss_fn(pred, y)# Backpropagationoptimizer.zero_grad()loss.backward()optimizer.step()# Output Logsif batch % 100 == 0:loss, current = loss.item(), batch * len(X)print(f"loss: {loss:>7f} [{current:>5d}/{size:>5d}]")## Inner loop for test
def test(dataloader, model, loss_fn):size = len(dataloader.dataset)num_batches = len(dataloader)model.eval()test_loss, correct = 0, 0with torch.no_grad():for X, y in dataloader:X, y = X.to(device), y.to(device)pred = model(X)test_loss += loss_fn(pred, y).item()correct += (pred.argmax(1) == y).type(torch.float).sum().item()test_loss /= num_batchescorrect /= sizeprint(f"Test Error: \n Accuracy: {(100*correct):>0.1f}%, Avg loss: {test_loss:>8f} \n")## Launch training / test loops#
epochs = 5
for t in range(epochs):print(f"Epoch {t+1}\n-------------------------------")train(train_dataloader, model, loss_fn, optimizer)test(test_dataloader, model, loss_fn)
print("Done!")## Saving Modelstorch.save(model.state_dict(), "model.pth")
print("Saved PyTorch Model State to model.pth")# Deployment## Loading Models
model = NeuralNetwork()
model.load_state_dict(torch.load("model.pth"))# Predict new imagesclasses = ["T-shirt/top","Trouser","Pullover","Dress","Coat","Sandal","Shirt","Sneaker","Bag","Ankle boot",
]model.eval()
x, y = test_data[0][0], test_data[0][1]
with torch.no_grad():pred = model(x)predicted, actual = classes[pred[0].argmax(0)], classes[y]print(f'Predicted: "{predicted}", Actual: "{actual}"')
三、利用MMClassification提供的预训练模型推理:
安装环境:
pip install openmim, mmengine
mim install mmcv-full mmcls
Inference using high-level API
from mmcls.apis import init_model, inference_modelmodel = init_model('mobilenet-v2_8xb32_in1k.py', 'mobilenet_v2_batch256_imagenet_20200708-3b2dc3af.pth', device='cuda:0')
load checkpoint from local path: mobilenet_v2_batch256_imagenet_20200708-3b2dc3af.pth
result = inference_model(model, 'banana.png')
result
{'pred_label': 954, 'pred_score': 0.9999284744262695, 'pred_class': 'banana'}
from mmcls.apis import show_result_pyplotshow_result_pyplot(model, 'banana.png', result)
PyTorch codes under the hood
Let write some raw PyTorch codes to do the same thing.
These are actual codes wrapped in high-level APIs.
construct an ImageClassifier
Note: current implementation only allow configs of backbone, neck and classification head instead of Python objects.
from mmcls.models import ImageClassifierclassifier = ImageClassifier(backbone=dict(type='MobileNetV2', widen_factor=1.0),neck=dict(type='GlobalAveragePooling'),head=dict(type='LinearClsHead',num_classes=1000,in_channels=1280) )
Load trained parameters
import torchckpt = torch.load('mobilenet_v2_batch256_imagenet_20200708-3b2dc3af.pth') classifier.load_state_dict(ckpt['state_dict'])
Construct data preprocessing pipeline
Important: A models work only if image preprocessing pipelines is correct.
from mmcls.datasets.pipelines import Composetest_pipeline = Compose([dict(type='LoadImageFromFile'),dict(type='Resize', size=(256, -1), backend='pillow'),dict(type='CenterCrop', crop_size=224),dict(type='Normalize',mean=[123.675, 116.28, 103.53],std=[58.395, 57.12, 57.375],to_rgb=True),dict(type='ImageToTensor', keys=['img']),dict(type='Collect', keys=['img']) ])
data = dict(img_info=dict(filename='banana.png'), img_prefix=None) data = test_pipeline(data) data
{'img_metas': DataContainer({'filename': 'banana.png', 'ori_filename': 'banana.png', 'ori_shape': (403, 393, 3), 'img_shape': (224, 224, 3), 'img_norm_cfg': {'mean': array([123.675, 116.28 , 103.53 ], dtype=float32), 'std': array([58.395, 57.12 , 57.375], dtype=float32), 'to_rgb': True}}),'img': tensor([[[ 0.3309, 0.2967, 0.3138, ..., 2.0263, 2.0092, 1.9920],[ 0.3481, 0.3309, 0.2282, ..., 2.0263, 2.0092, 1.9920],[ 0.2796, 0.2967, 0.2967, ..., 1.9920, 2.0263, 1.9749],...,[ 0.1939, 0.1768, 0.2282, ..., 0.3994, 0.3309, 0.3823],[ 0.1426, 0.1254, 0.2111, ..., 0.5878, 0.5364, 0.5536],[-0.0116, -0.0801, 0.1597, ..., 0.5707, 0.5536, 0.5364]],[[ 0.3803, 0.3803, 0.3803, ..., 2.1660, 2.1485, 2.1134],[ 0.4153, 0.4153, 0.3102, ..., 2.1835, 2.1310, 2.1134],[ 0.3452, 0.3803, 0.3803, ..., 2.1134, 2.1485, 2.1134],...,[ 0.2752, 0.2577, 0.3102, ..., 0.5028, 0.4328, 0.4328],[ 0.2227, 0.1877, 0.3102, ..., 0.6604, 0.6254, 0.5728],[ 0.0301, -0.0049, 0.2402, ..., 0.6604, 0.6254, 0.5728]],[[ 0.5485, 0.5485, 0.5485, ..., 2.3437, 2.3263, 2.2914],[ 0.5834, 0.5834, 0.4788, ..., 2.3611, 2.3088, 2.2914],[ 0.5136, 0.5485, 0.5485, ..., 2.3088, 2.3437, 2.3088],...,[ 0.4091, 0.3916, 0.4439, ..., 0.5834, 0.5136, 0.5311],[ 0.3568, 0.3045, 0.4265, ..., 0.7576, 0.7228, 0.7054],[ 0.1651, 0.1128, 0.3742, ..., 0.7576, 0.7402, 0.7054]]])}
equivalent in torchvision
from PIL import Image from torchvision.transforms import Compose, Resize, CenterCrop, Normalize, ToTensortv_transform = Compose([Resize(256), CenterCrop(224), ToTensor(),Normalize([0.485, 0.456, 0.406], [0.229, 0.224, 0.225])])image = Image.open('banana.png').convert('RGB') tv_data = tv_transform(image)
Forward through the model
## IMPORTANT: set the classifier to eval mode classifier.eval()imgs = data['img'].unsqueeze(0) imgs = tv_data.unsqueeze(0)with torch.no_grad():# class probabilitiesprob = classifier.forward_test(imgs)[0]# featuresfeat = classifier.extract_feat(imgs, stage='neck')[0]print(len(prob)) print(prob.argmax().item()) print(feat.shape)
1000 954 torch.Size([1, 1280])
3.使用MMClassificaiton完整进行水果分类实战:
数据集下载:
GitHub - TommyZihao/MMClassification_Tutorials: Jupyter notebook tutorials for MMClassificationJupyter notebook tutorials for MMClassification. Contribute to TommyZihao/MMClassification_Tutorials development by creating an account on GitHub.https://github.com/TommyZihao/MMClassification_Tutorials
代码框架:
def main():model = build_classifier(cfg.model)model.init_weights()datasets = [build_dataset(cfg.data.train)]train_model(model,datasets,cfg,distributed=distributed,validate=(not args.no_validate),timestamp=timestamp,device=cfg.device,meta=meta)
mmcls/apis/train_model.pydef train_model(model,dataset,cfg):data_loaders = [build_dataloader(ds, **train_loader_cfg) for ds in dataset]optimizer = build_optimizer(model, cfg.optimizer)runner = build_runner(cfg.runner,default_args=dict(model=model,optimizer=optimizer))runner.register_training_hooks(cfg.lr_config,optimizer_config,cfg.checkpoint_config,cfg.log_config,cfg.get('momentum_config', None),custom_hooks_config=cfg.get('custom_hooks', None))runner.run(data_loaders, cfg.workflow)
mmcv/runner/epoch_based_runner.pyclass EpochBasedRunner(BaseRunner):def run_iter(self, data_batch: Any, train_mode: bool, **kwargs) -> None:if train_mode:outputs = self.model.train_step(data_batch, self.optimizer, **kwargs)else:outputs = self.model.val_step(data_batch, self.optimizer, **kwargs)self.outputs = outputsdef train(self, data_loader, **kwargs):self.model.train()self.data_loader = data_loaderfor i, data_batch in enumerate(self.data_loader):self.run_iter(data_batch, train_mode=True, **kwargs)self.call_hook('after_train_iter')
mmcls/models/classifiers/base.pyclass BaseClassifier(BaseModule, metaclass=ABCMeta):def forward(self, img, return_loss=True, **kwargs):"""Calls either forward_train or forward_test depending on whetherreturn_loss=True.Note this setting will change the expected inputs. When`return_loss=True`, img and img_meta are single-nested (i.e. Tensor andList[dict]), and when `resturn_loss=False`, img and img_meta should bedouble nested (i.e. List[Tensor], List[List[dict]]), with the outerlist indicating test time augmentations."""if return_loss:return self.forward_train(img, **kwargs)else:return self.forward_test(img, **kwargs)def train_step(self, data, optimizer=None, **kwargs):losses = self(**data)loss, log_vars = self._parse_losses(losses)outputs = dict(loss=loss, log_vars=log_vars, num_samples=len(data['img'].data))return outputs
mmcls/models/classifiers/image.pyclass ImageClassifier(BaseClassifier):def __init__(self,backbone,neck=None,head=None,pretrained=None,train_cfg=None,init_cfg=None):super(ImageClassifier, self).__init__(init_cfg)if pretrained is not None:self.init_cfg = dict(type='Pretrained', checkpoint=pretrained)self.backbone = build_backbone(backbone)if neck is not None:self.neck = build_neck(neck)if head is not None:self.head = build_head(head)def extract_feat(self, img):x = self.backbone(img)if self.with_neck:x = self.neck(x)return xdef forward_train(self, img, gt_label, **kwargs):x = self.extract_feat(img)losses = dict()loss = self.head.forward_train(x, gt_label)losses.update(loss)return losses
mmcv/runner/hooks/optimizer.pyclass OptimizerHook(Hook):def after_train_iter(self, runner):runner.optimizer.zero_grad()runner.outputs['loss'].backward()runner.optimizer.step()
总结:本篇主要偏向图像分类实战部分,使用MMclassification工具进行代码应用,熟悉其框架应用,为后续处理不同场景下分类问题提供帮助。
本文参考:GitHub - wangruohui/sjtu-openmmlab-tutorial
相关文章:
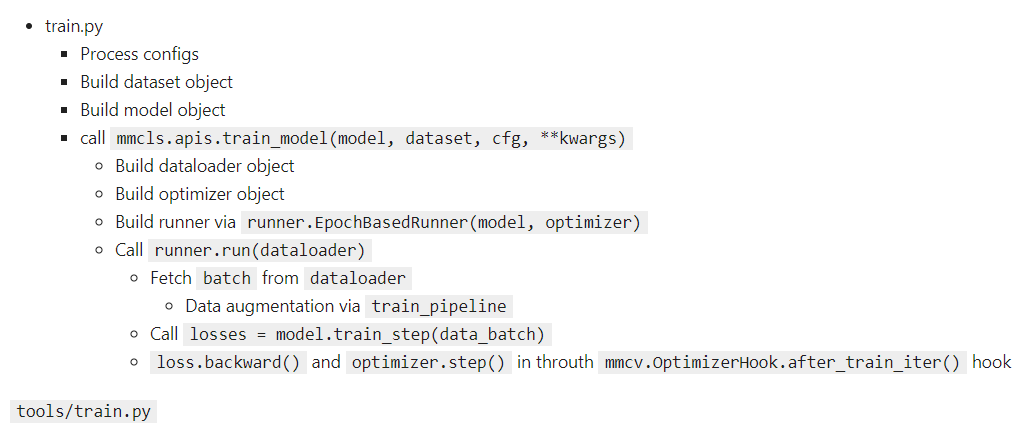
计算机视觉框架OpenMMLab开源学习(三):图像分类实战
前言:本篇主要偏向图像分类实战部分,使用MMclassification工具进行代码应用,最后对水果分类进行实战演示,本次环境和代码配置部分省略,具体内容建议参考前一篇文章:计算机视觉框架OpenMMLab开源学习&#x…...

awk命令
一.介绍 awk是专门为文本处理设计的编程语言,是一门数据驱动的编程语言。与sed类似,都是以数据驱动的行处理软件,主要用于数据扫描,过滤和汇总。数据可以来自于标准输入,管道或者文件。 二.语法 awk是一种处理文本文件…...

LocalDateTime获取时间的年、月、日、时、分、秒、纳秒
如何把String/Date转成LocalDateTime参考String、Date与LocalDate、LocalTime、LocalDateTime之间互转 String、Date、LocalDateTime、Calendar与时间戳之间互相转化参考String、Date、LocalDateTime、Calendar与时间戳之间互相转化 方法介绍 getYear() 获取日期的年 getMon…...
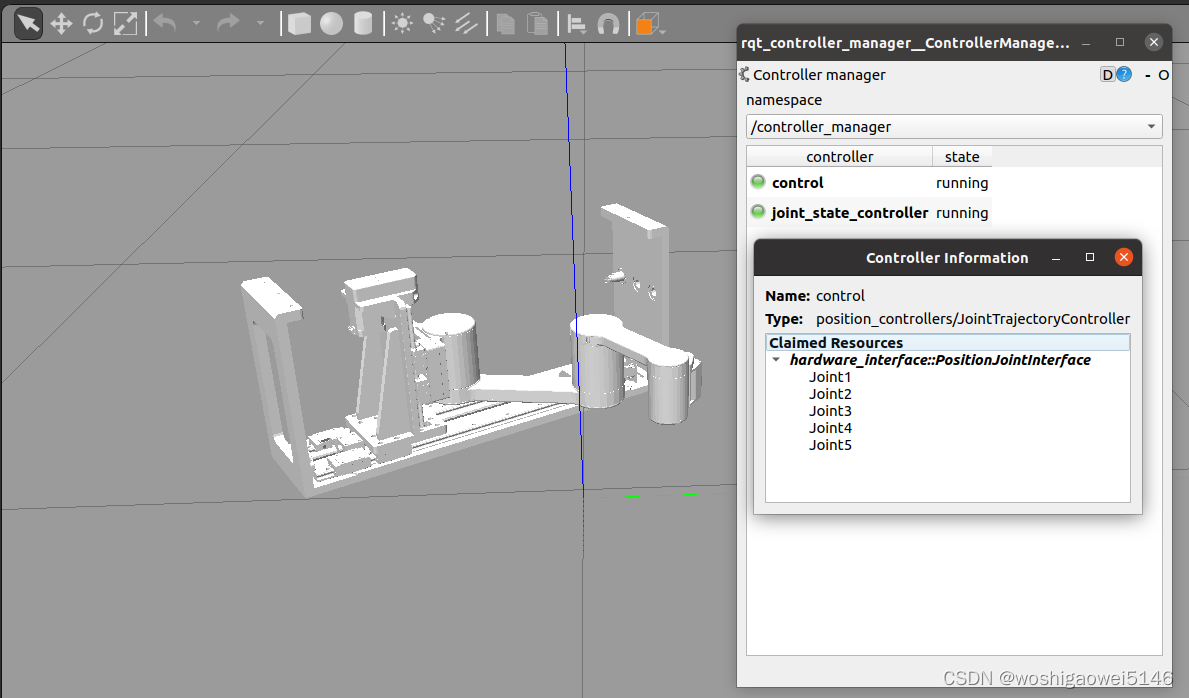
MoveIT Rviz和Gazebo联合仿真
文章目录环境安装概述ros_control框架ros_control数据流文件配置附加工具故障问题解决参考接前两篇:ROS MoveIT1(Noetic)安装总结 Solidworks导出为URDF用于MoveIT总结(带prismatic) MoveIT1 Assistant 总结 环境 Ubu…...

ESP32S2(12K)-DS18B20数码管显示温度
一、物料清单: NODEMCU-32-S2 (ESP32-12K)四段数码管(共阴)DS18B20(VCC/DQ/GND)Arduino-IDE 2.0.3二、实现方法及效果图: 2.1 引用库 // #include <OneWire.h> //可以不引入,因为DallasTemperature.h中已经引入了OneWire.h #include <DallasTemperature.h>#…...

linux栈溢出定位
一、编译选项定位堆栈溢出 来源:堆栈溢出检测机制 - SkrSky - 博客园 1、栈溢出可能打印 unhandled level 1 translation fault (11) at 0x7f8d0347, esr 0x92000005 2、栈溢出保护机制 gcc提供了栈保护机制stack-protector(编译选项-fstack-protec…...
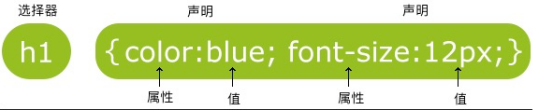
CSS基础:选择器和声明样式
CSS概念 CSS(Cascading Style Sheets)层叠样式表,又叫级联样式表,简称样式表 CSS用于HTML文档中元素样式的定义 使用css让网页具有美观一致的页面 语法 CSS 规则由两个主要的部分构成:选择器和声明样式 选择器通常…...
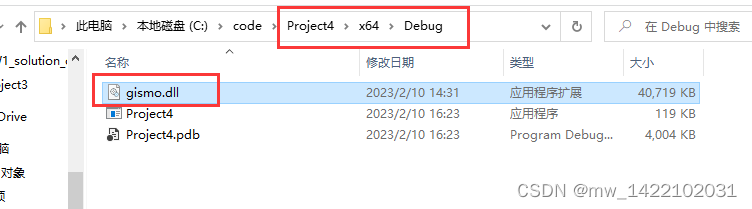
VS中安装gismo库
文章目录前言一、下载安装paraview直接下载压缩包安装就可以了解压后按步骤安装即可二、gismo库的安装gismo库网址第一种方法:第二种方法第三种方法:用Cmake软件直接安装首先下载cmake软件[网址](https://cmake.org/download/)安装gismo库三、gismo库的使…...
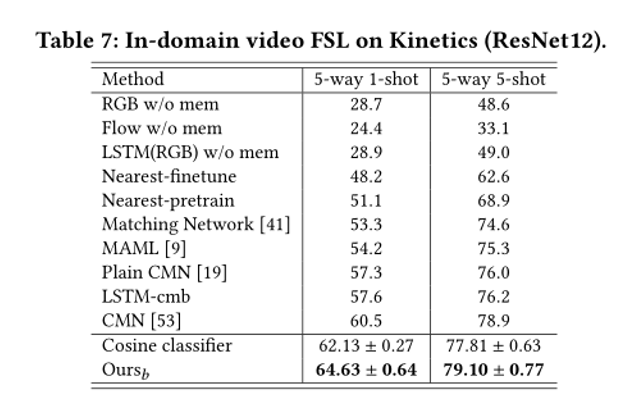
元学习方法解决CDFSL以及两篇SOTA论文讲解
来源:投稿 作者:橡皮 编辑:学姐 带你学习跨域小样本系列1-简介篇 跨域小样本系列2-常用数据集与任务设定详解 跨域小样本系列3:元学习方法解决CDFSL以及两篇SOTA论文讲解(本篇) 跨域小样本系列4…...
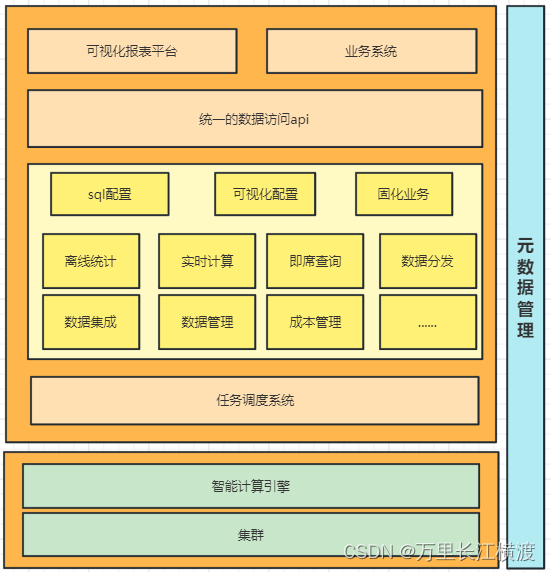
大数据之------------数据中台
一、什么是数据中台 **数据中台是指通过数据技术,对海量数据进行采集、计算、存储、加工,同时统一标准和口径。**数据中台的目标是让数据持续用起来,通过数据中台提供的工具、方法和运行机制,把数据变为一种服务能力,…...

Python 中 字符串是什么?
字符串是 Python 中最常用的数据类型。我们可以使用引号 ( ’ 或 " ) 来创建字符串。 创建字符串很简单,只要为变量分配一个值即可。例如: var1 ‘Hello World!’ var2 “Python Runoob” Python 访问字符串中的值 Python 不支持单字符类型&…...

OJ刷题Day1 · 一维数组的动态和 · 将数字变成 0 的操作次数 · 最富有的客户资产总量 · Fizz Buzz · 链表的中间结点 · 赎金信
一、一维数组的动态和二、将数字变成 0 的操作次数三、最富有的客户资产总量四、Fizz Buzz五、链表的中间结点六、赎金信一、一维数组的动态和 给你一个数组 nums 。数组「动态和」的计算公式为:runningSum[i] sum(nums[0]…nums[i]) 。 请返回 nums 的动态和。 示…...

【数据结构】栈——必做题
逆波兰表达式后缀表达式的出现是为了方便计算机处理,它的运算符是按照一定的顺序出现,所以求值过程中并不需要使用括号来指定运算顺序,也不需要考虑运算符号(比如加减乘除)的优先级。先介绍中简单的人工转化方法&#…...
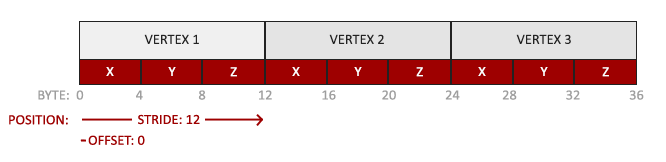
LearnOpenGL 笔记 - 入门 04 你好,三角形
系列文章目录 LearnOpenGL 笔记 - 入门 01 OpenGLLearnOpenGL 笔记 - 入门 02 创建窗口LearnOpenGL 笔记 - 入门 03 你好,窗口 文章目录系列文章目录前言你好,三角形顶点输入顶点着色器(Vertex Shader)编译着色器片段着色器&…...
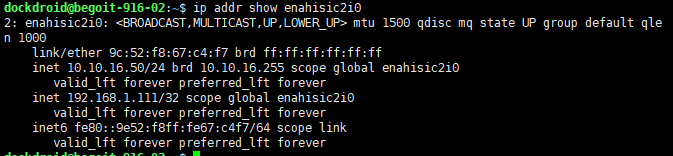
keepalived+mysql高可用
一.设置mysql同步信息两节点安装msyql略#配置节点11.配置权限允许远程访问mysql -u root -p grant all on *.* to root% identified by Root1212# with grant option; flush privileges;2.修改my.cnf#作为主节点配置(节点1)#作为主节点配置 server-id 1 …...
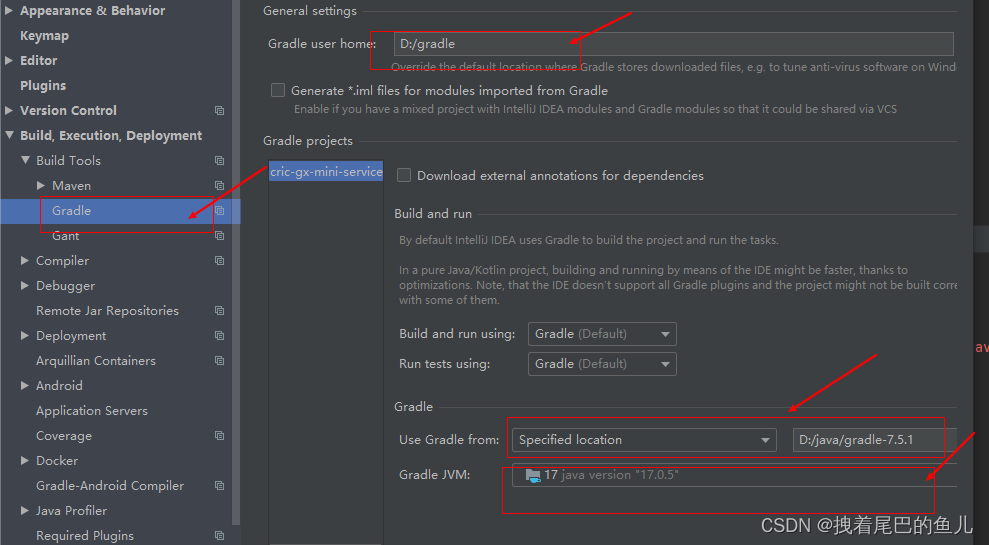
JAVA工具篇--1 Idea中 Gradle的使用
前言: 既然我们已经使用Maven 来完成对项目的构建,为什么还要使用Gradle 进行项目的构建;gradle和maven都可以作为java程序的构建工具,但两者还是有很大的不同之处的:1.可扩展性,gradle比较灵活,…...
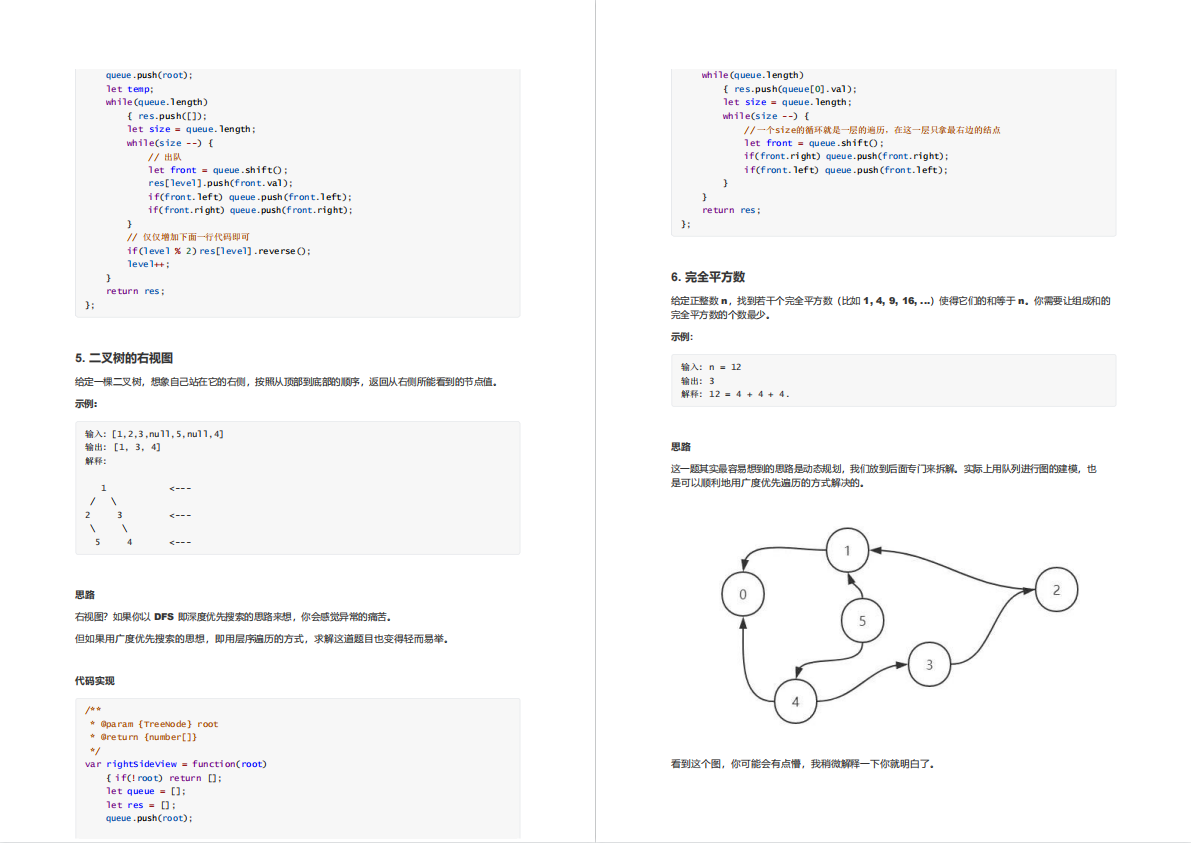
弄懂自定义 Hooks 不难,改变开发认知有点不习惯
前言 我之前总结逻辑重用的时候,就一直在思考一个问题。 对于逻辑复用,render props 和 高阶组件都可以实现,同样官方说 Hooks 也可以实现,且还是在不增加额外的组件的情况下。 但是我在项目代码中,没有找到自定义 …...
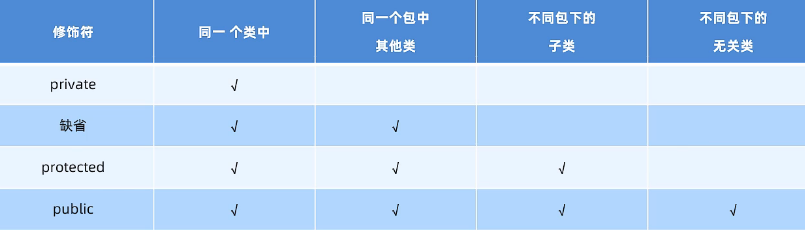
Java面向对象基础
文章目录面向对象类注意事项内存机制构造器this关键字封装javabean格式成员变量和局部变量区别static静态关键字使用成员方法使用场景内存机制注意事项static应用:工具类static应用:代码块静态代码块实例代码块(用的比较少)static…...
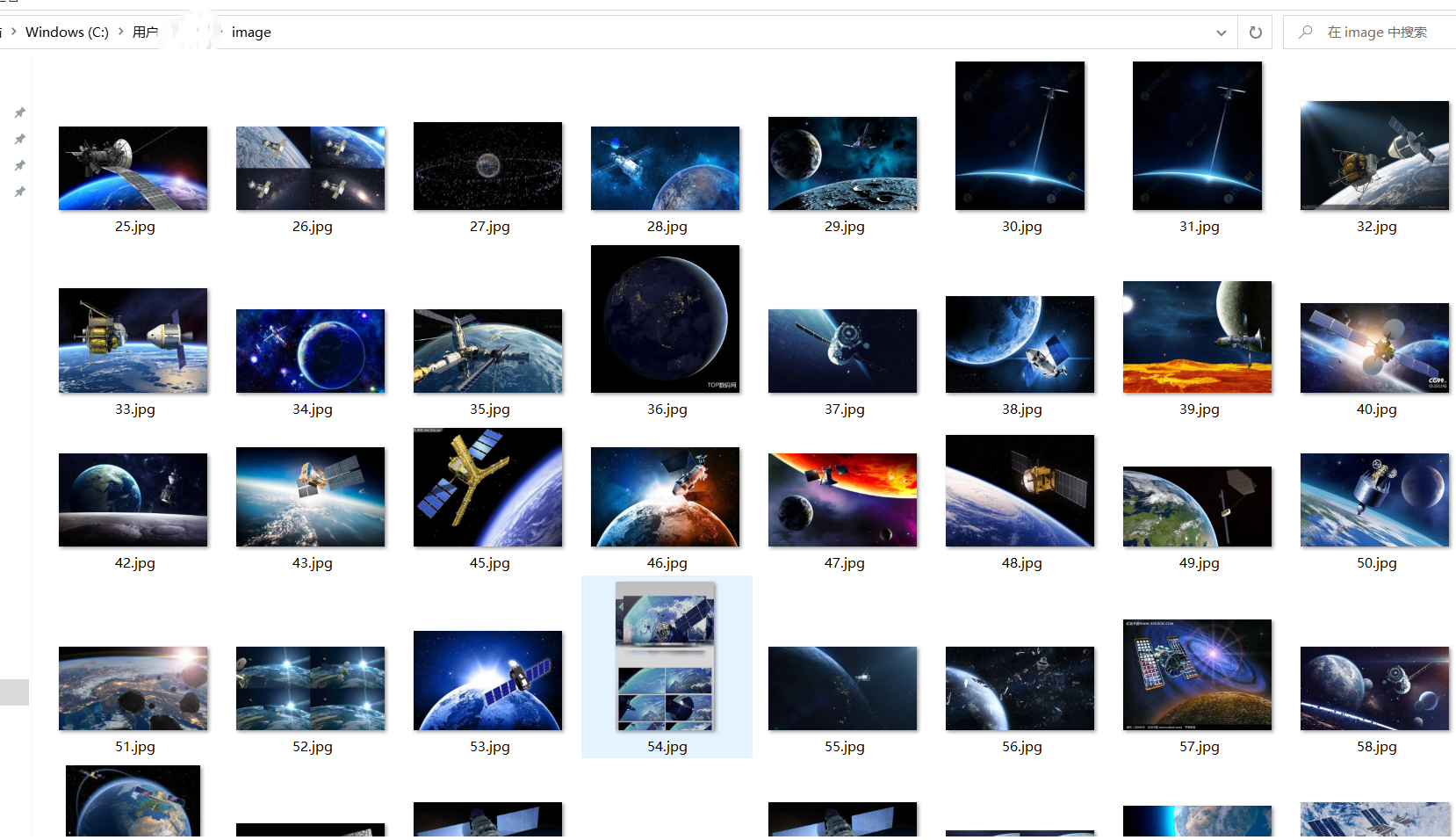
基于python下selenium库实现交互式图片保存操作(批量保存浏览器中的图片)
Selenium是最广泛使用的开源Web UI(用户界面)自动化测试套件之一,可以通过编程与浏览量的交互式操作对网页进行自动化控制。基于这种操作进行数据保存操作,尤其是在图像数据的批量保存上占据优势。本博文基于selenium 与jupyterla…...
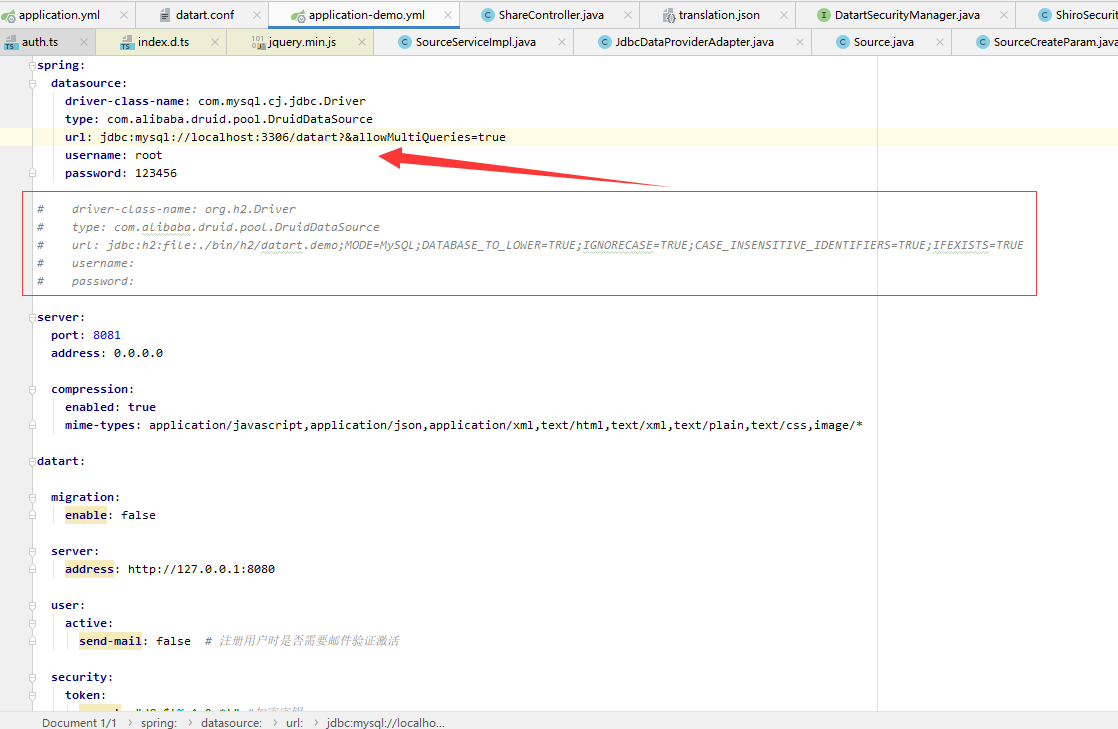
一:Datart的下载、本地运行
前言:本文只是个人在使用datart的一个记录,仅供参考。如果有不一样的地方,欢迎评论或私信进行交流。datart 是新一代数据可视化开放平台,支持各类企业数据可视化场景需求,如创建和使用报表、仪表板和大屏,进…...

Docker-compose
一.Docker-compose概述Docker-Compose项目是Docker官方的开源项目,负责实现对Docker容器集群的快速编排。Docker-Compose将所管理的容器分为三层,分别是 工程(project),服务(service)以及容器&a…...
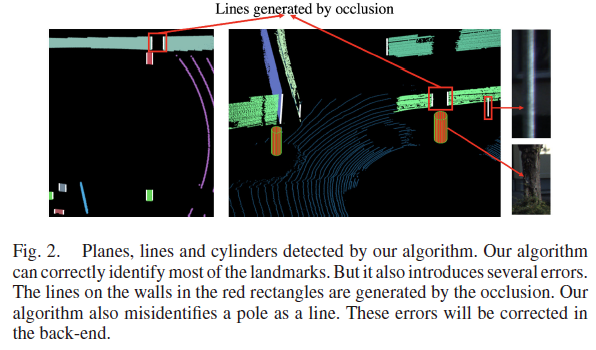
经典文献阅读之--PLC-LiSLAM(面,线圆柱SLAM)
0. 简介 对于激光SLAM来说,现在越来越多的算法不仅仅局限于点线等简答特征的场景了,文章《PLC-LiSLAM: LiDAR SLAM With Planes, Lines,and Cylinders》说到,平面、线段与圆柱体广泛存在于人造环境中。为此作者提出了一个使用这些landmark的…...
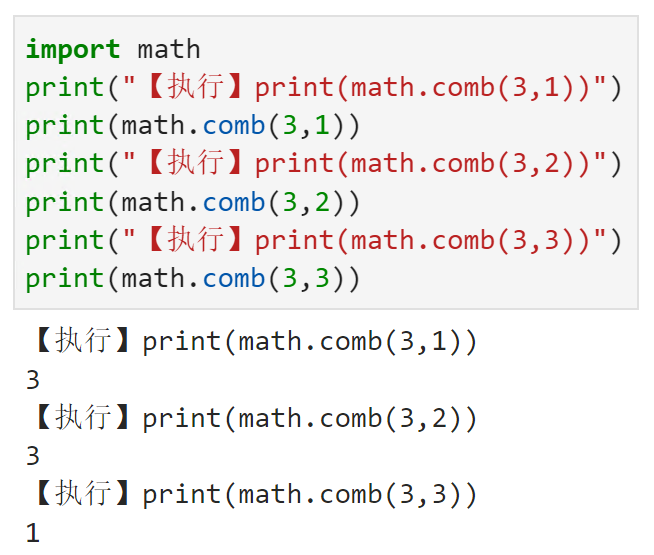
计算组合数Cnk即从n个不同数中选出k个不同数共有多少种方法math.comb(n,k)
【小白从小学Python、C、Java】 【计算机等级考试500强双证书】 【Python-数据分析】 计算组合数Cnk 即从n个不同数中选出k个不同数共有多少种方法 math.comb(n,k) 以下python代码输出结果是? import math print("【执行】print(math.comb(3,1))") print(math.comb(…...

工厂设计模式
基本概念:为创建对象提供过渡接口,以便将创建对象的具体过程屏蔽隔离起来,达到提高灵活性的目的。分为三类:简单工厂模式Simple Factory:不利于产生系列产品;工厂方法模式Factory Method:又称为…...
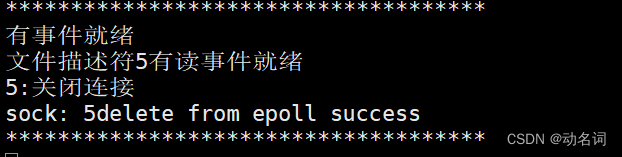
IO多路转接 —— poll和epoll
文章目录1. poll1.1 poll的函数接口1.2 poll的简单测试程序1.3 poll的优缺点分析2. epoll2.1 epoll的函数接口2.2 epoll的工作原理2.3 epoll的工作模式(LT,ET)2.4 epoll的简易服务器实现(默认是LT工作模式)前言: 接上文讲述的select,它有缺点,…...
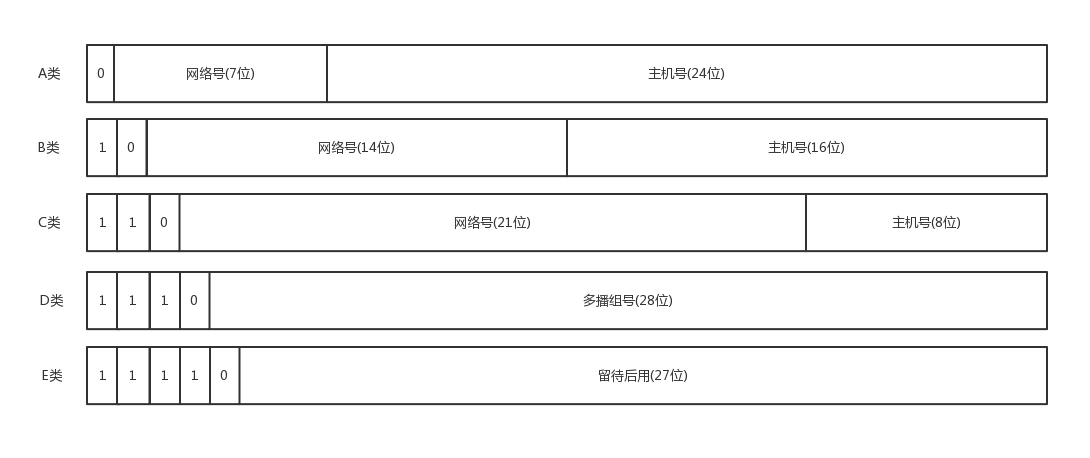
计算机网络整理-问答
1. 程序工作的时候网络各层的状态 如下图所示: 1. TCP 在进行三次握手的时候,IP 层和 MAC 层对应都有什么操作呢? TCP 三次握手是通过在传输层建立连接的一个过程,在这个过程中,TCP 和 IP 层、MAC 层都起到了重要的…...
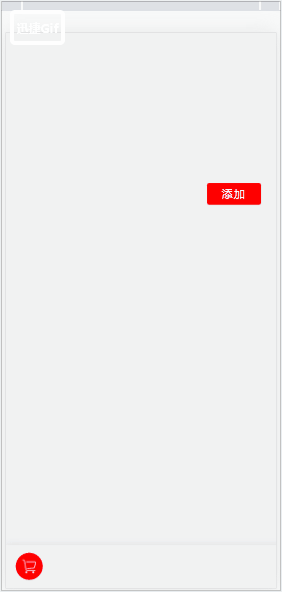
JS 实现抛物线动画案例
相信大家都有浏览过,很多购物网站购物车的添加商品动画,今天,我们就手写一个简单的抛物线动画,先上案例: 一、绘制页面 我们这里简单实现,一个按钮,一个购物车图标,样式这里直接跳过…...
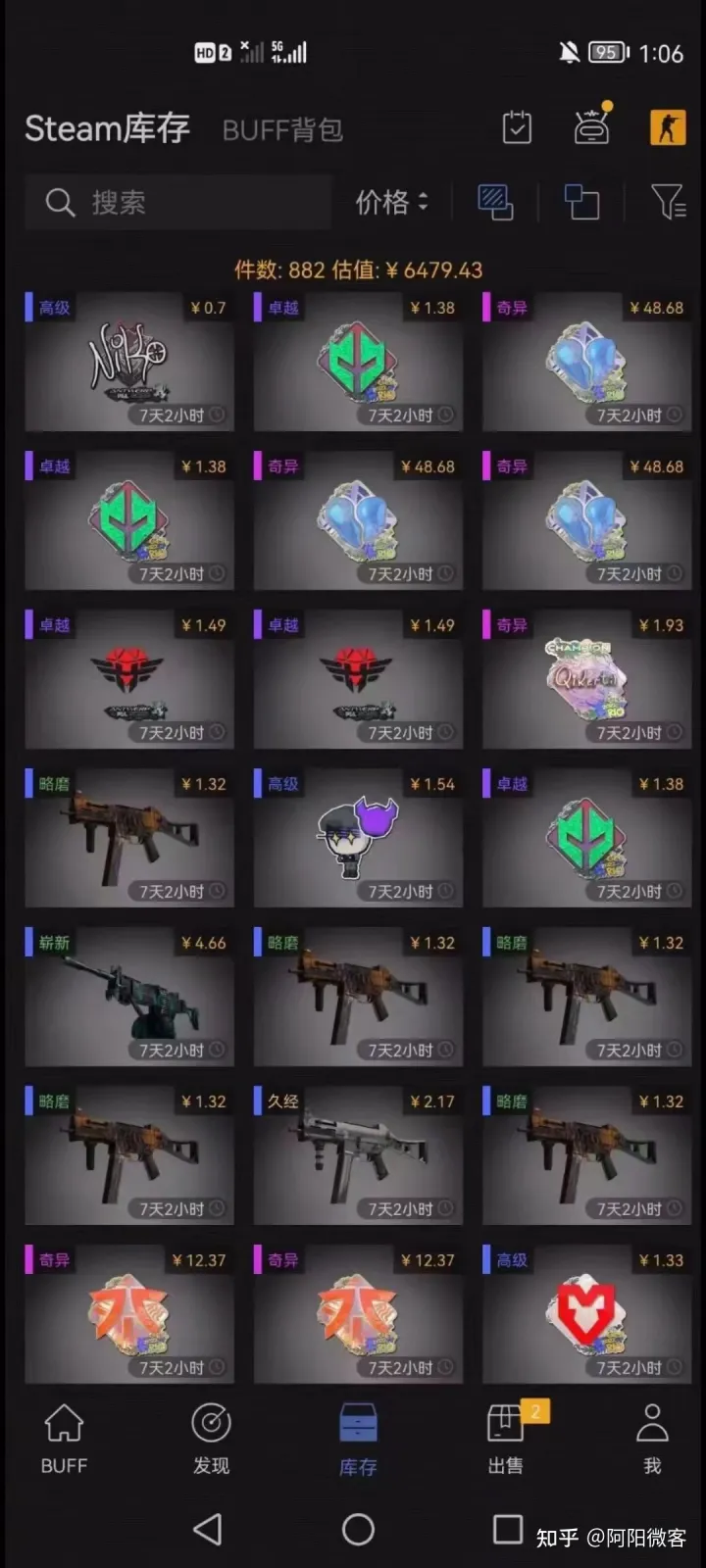
CSGO搬砖项目,23年最适合小白的项目!
大家好,我是阿阳 不懂的小伙伴,咱继续听我娓娓道来 steam搬砖主要涉及的是csgo游戏平台装备的一个搬运,比较很好理解,主要就是道具的搬运工,简单来讲就是,从国外steam游戏平台购买装备,再挂到…...
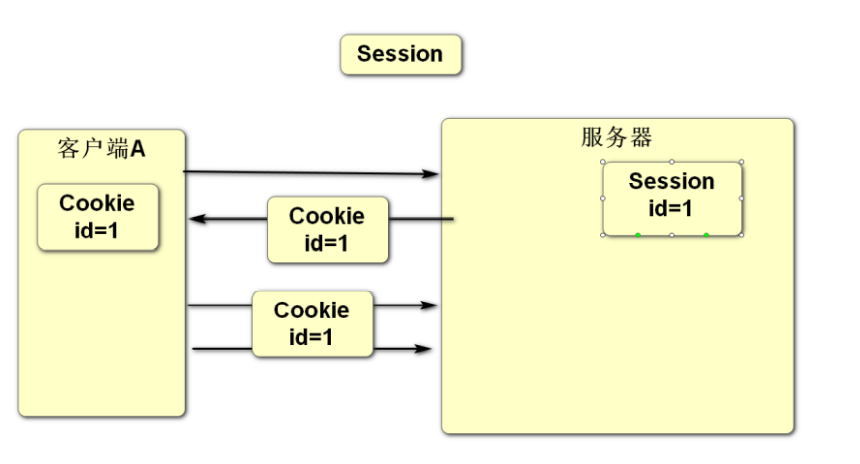
谈谈会话管理
客户端和服务器之间进行数据传输遵循的是HTTP协议, 此协议属于无状态协议(一次请求对应一次响应, 响应完之后断开连接), 服务器是无法跟踪客户端的请求, 通过cookie技术可以给客户端添加一个标识, 客户端之后发出的每次请求都会带着这个标识从而让服务器识别此客户端, 但由于co…...
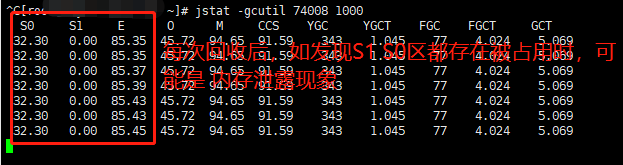
Linux查看JVM FULL GC频率
查看系统的full gc频率,可以使用jstack命令一、采用top命令定位进程登录服务器,执行top命令,查看CPU占用情况,找到进程的pid二、使用jstack命令统计垃圾回收jstat -gc pid 5000即会每5秒一次显示进程号为pid的java进程的GC情况以上…...

搜索引擎优化简称seo/东莞seo网络营销
每次有点什么感想,想写blog的时候,都是不在电脑跟前的时候,慢慢的,我的blog有点开始荒凉了。就像这几天的长假,懒得连电脑都不想碰,每次想写点什么的时候,想想还要开机,就放弃了。这…...

做网站哪个公司比较好/seo优化易下拉霸屏
这句话的真正意思,我到现在才体会出来。他的作用是将请求转发给过滤器链上下一个对象。这里的“下”指的是哪里 ?值得是下一个filter,如果没有filter那就是你请求的资源。 一般filter都是一个链,web.xml 里面配置了几个就有几个。一个一个的连…...

电大网上作业代做网站/百度竞价推广收费
作为一种定期清理无效数据的重要机制,主键失效存在于大多数缓存系统中,Reids也不例外。在Redis提供的诸多命令中,EXPIRE、EXPIREAT、PEXPIRE、PEXPIREAT以及SETEX和PSETEX均可以用来设置一条Key-Value对的失效时间,而一条Key-Valu…...

手机网站打不开的解决方法/郑州网站优化seo
一、STL简介 STL(Standard Template Library,标准模板库)是惠普实验室开发的一系列软件的统称。它是由Alexander Stepanov、Meng Lee和David R Musser在惠普实验室工作时所开发出来 的。现在虽说它主要出现在C中,但在被引入C之前该技术就已经…...
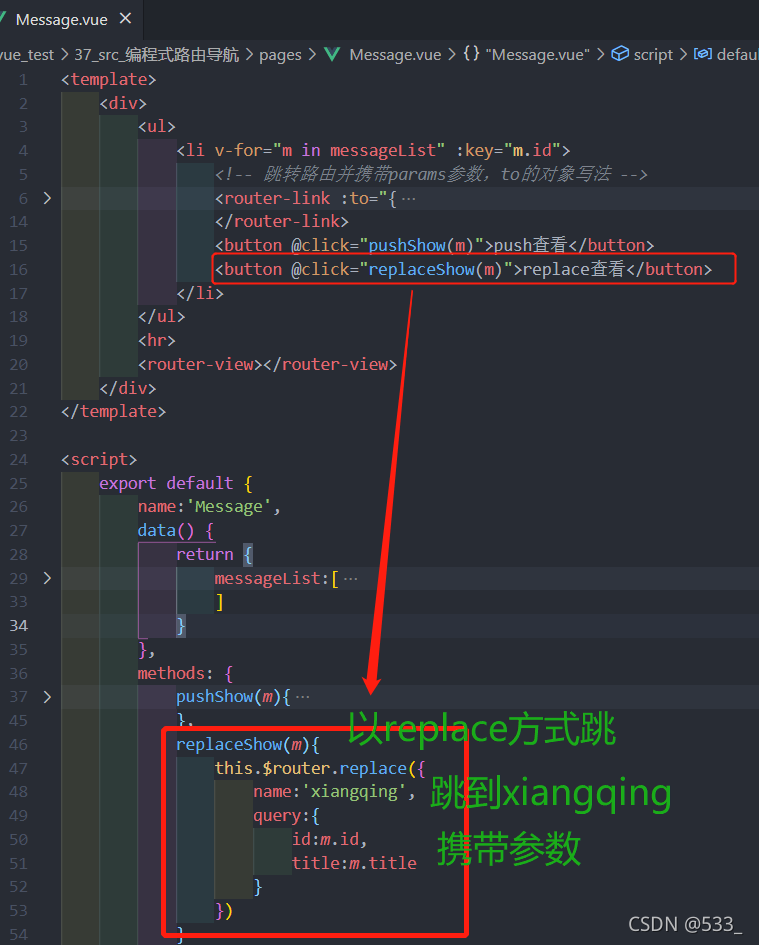
论述政府门户网站建设的基本意义/国外免费舆情网站有哪些软件
作用:不借助<router-link>实现路由跳转,让路由跳转更加灵活 具体编码: $router.push 想要导航到不同的 URL,则使用 router.push 方法。这个方法会向 history 栈添加一个新的记录,所以,当用户点击浏…...
国外网站怎样建设/深圳市住房和建设局
原文:http://coolketang.com/staticCoding/5a9925ad9f5454507417fc94.html 1. 一个类可以继承另一个类的方法、属性和其它特性。当一个类继承其它类时,继承的类叫子类,被继承的类叫父类。继承是区分类和其它类型的一个重要特征。 2. 首先定义…...