FastAPI+React全栈开发10 MongoDB聚合查询
Chapter02 Setting Up the Document Store with MongoDB
10 Aggregation framework
FastAPI+React全栈开发10 MongoDB聚合查询
In the following pages, we will try to provide a brief introducton to the MongoDB aggregation framework, what it is, what benefits it offers, and why it is regarded as one of the strongest selling points of the MongoDB ecosystem.
在接下来的几页中,我们将简要介绍MongoDB聚合框架,它是什么,它提供了什么好处,以及为什么它被认为是MongoDB生态系统最强大的卖点之一。
Gentered around the concept of a pipeline (something that you might be familiar with if you have done some analytics or if you have ever connected a few commands in Linux), the aggregation framework is, at its simplest, an alternative way to retrieve sets of documents from a collection, it is similar to the find method that we already used extensively but with the additional benefit of the possibility of data processing in different stages or steps.
聚合框架是围绕管道的概念产生的(如果您做过一些分析,或者您曾经在Linux中连接过几个命令,您可能会熟悉这个概念),它是最简单的一种从集合中检索文档集的替代方法,它类似于我们已经广泛使用的find方法,但具有在不同阶段或步骤中进行数据处理的可能性的额外好处。
With the aggregation pipeline, we basically pull documents from a MongoDB collection and feed them sequentially to various stages of the pipeline where each stage output is fed to the next stage’s input until the final set of documents is returned. Each stage performs some data-processing operations on the currently selected documents, which include modifying documents, so the output documents often have a completely different structure.
使用聚合管道,我们基本上从MongoDB集合中提取文档,并依次将它们提供给管道的各个阶段,其中每个阶段的输出被馈送到下一阶段的输入,直到返回最终的文档集。每个阶段对当前选择的文档执行一些数据处理操作,其中包括修改文档,因此输出文档通常具有完全不同的结构。
1、$match: Match only specific documents, i.e. a particular brand.
2、$project: Selcect existing fields or derive new ones, brand and model.
3、$group: Group according to a categorical feature, like brand.
4、$sort: Sort in ascending or descending order using a field.
5、$limit: Limit the results to a predefined number.
1、$match:只匹配特定的文档,即特定的品牌。
2、$project:选择现有领域或衍生新的领域、品牌和模型。
3、$group:根据分类特征进行分组,如品牌。
4、$sort:使用字段按升序或降序排序。
5、$limit:将结果限制在预定义的数量内。
The operations that can be included in the stages are, for example, match, which is used to include only a subset of the entire collection, sorting, grouping, and projections. The MongoDB documentation site is the best place to start if you want to get acquainted with all the possibilities, but we want to start with a couple of simple examples.
这些阶段中可以包含的操作有,例如匹配,它用于只包含整个集合、排序、分组和投影的一个子集。如果您想了解所有的可能性,MongoDB文档站点是最好的起点,但是我们想从几个简单的示例开始。
The syntax for the aggregation is similar to other methods, we use the aggregate method, which takes a list of stages as a parameter.
聚合的语法与其他方法类似,我们使用aggregate方法,它将阶段列表作为参数。
Probably the best aggregation, to begin with, would be to mimic the find method. Let’s try to get all the Fiat cars in our collection as follows.
首先,最好的聚合可能是模仿find方法。让我们按照下面的方式尝试获取我们收集的所有菲亚特汽车。
db.cars.aggregate([{$match:{brand:"Fiat"}}])
import mongo6client = mongo6.MongoClient('mongodb://zhangdapeng:zhangdapeng520@192.168.234.130:27017/')
db = client["carsDB"]
cars = db["cars"]query = [{"$match": {"brand": "Fiat"}}]
r = cars.aggregate(query)
print(list(r))
This is probably the simplest possible aggregation and it consists of just one stage, the $match stage, which tells MongoDB that we only want the Fiats, so the out put of the first stage is exactly that.
这可能是最简单的聚合,它只包含一个阶段,即$match阶段,它告诉MongoDB我们只需要Fiats,因此第一阶段的输出正是如此。
Let’s say that in the second stage we want to group our Fiat cars by model and then check the average price for every model. The second stage is a bit more complicated, but bear with us, it is not that hard. Run the following lines of code.
假设在第二阶段,我们希望按车型对菲亚特汽车进行分组,然后检查每个车型的平均价格。第二阶段有点复杂,但请耐心等待,这并不难。运行以下代码行。
import mongo6client = mongo6.MongoClient('mongodb://zhangdapeng:zhangdapeng520@192.168.234.130:27017/')
db = client["carsDB"]
cars = db["cars"]query = [{"$match": {"brand": "Fiat"}}, # 找到菲亚特的汽车{"$group": {"_id": "$make", "avg_price": {"$avg": "$price"}}}, # 按照make字段分组,求price的平均值
]
r = cars.aggregate(query)
print(list(r))
The second stage uses the KaTeX parse error: Expected '}', got 'EOF' at end of input: …e part {model:"make"} is a bit counterintuitive, but it just gives MongoDB the following two important pieces of information:
- model: Without quotes or the dollar sign, it is the key that will be used for the grouping, and in our case, it makes sense that it is called model. We can call it any way we want; it is the key that will indicate the field that we are doing the grouping by.
- $make: It is actually required to be one of the fields present in the documents. In our case, it is called make and the dollar sign means that it is a field in the document. Other possibilities would be the year, the gearbox, and really any document field that has a categorical or ordinal meaning. The price wouldn’t make much sense.
第二阶段使用KaTeX parse error: Expected '}', got 'EOF' at end of input: …的文档键。部分{model:"make"}有点违反直觉,但它只是给MongoDB以下两个重要的信息:
- model:没有引号或美元符号,它是将用于分组的键,在我们的例子中,它被称为model是有意义的。我们可以随意称呼它;这是一个键,它将指示我们进行分组的字段。
- $make:它实际上需要是文档中存在的字段之一。在我们的示例中,它被称为make,美元符号表示它是文档中的一个字段。其他可能是年份、变速箱,以及任何具有分类或顺序含义的文档字段。这个价格不太合理。
The second argument in the group stage is the actual aggregation, as follows:
- avgPrice: This is the chosen name for the quantity that we wish to map. In our case, it makes sense to call it avgPrice, but we can choose this variable’s name as we please.
- $avg: This is one of the available aggregation functions such as average, count, sum, maximum, minimum, and so on. In this example, we could have used the minimum function instead of the average function in order to get the cheapest Fiat for every model.
- $price: like $make in the preceding part of the expression, this is a field belonging to the documents and it should be numeric, since calculating the average or the minimum of a sting doesn’t make much sense.
小组阶段的第二个参数是实际的聚合,如下所示:
- avgPrice:这是我们希望映射的数量的选择名称。在我们的示例中,将其称为avgPrice是有意义的,但是我们可以根据需要选择这个变量的名称。
- $avg:这是一个可用的聚合函数,如average, count, sum, maximum, minimum等。在这个例子中,我们可以使用最小函数而不是平均函数,以便为每个型号获得最便宜的菲亚特。
- p r i c e : 就像表达式前面的 price:就像表达式前面的 price:就像表达式前面的make一样,这是一个属于文档的字段,它应该是数字的,因为计算平均值或最小值没有多大意义。
Pipelines can also include data processing through the project operator, a handy tool for creating entirely new fields, derived from existing document fields, that are then carried into the next stages.
管道还可以包括通过项目操作员进行的数据处理,这是一种方便的工具,用于创建从现有文档字段派生的全新字段,然后将其带入下一阶段。
We will provide just another example to showcase the power of project in a pipeline stage. Let’s consider the following aggregation.
我们将提供另一个例子来展示项目在管道阶段的力量。让我们考虑下面的聚合。
import mongo6client = mongo6.MongoClient('mongodb://zhangdapeng:zhangdapeng520@192.168.234.130:27017/')
db = client["carsDB"]
cars = db["cars"]query = [{"$match": {"brand": "Opel"}}, # 查找{"$project": {"_id": 0, "price": 1, "year": 1, "fullName": {"$concat": ["$make", " ", "$brand"]}}}, # 过滤{"$group": {"_id": {"make": "$fullName"}, "avgPrice": {"$avg": "$price"}}}, # 分组{"$sort": {"avgPrice": -1}}, # 排序,根据平均价格降序{"$limit": 10}, # 限制返回数量
]
r = cars.aggregate(query)
print(list(r))
This might look intimidating at first, but it is mostly composed of elements that we have already seen. There is the $match stage (we select only the Opel cars), and there is sorting by the price in descending order and cutting off at the 10 priciest cars at the end. But the projection in the middle? It is just a way to craft new variables in a stage using existing ones.
乍一看可能有点吓人,但它主要是由我们已经见过的元素组成的。有$match阶段(我们只选择欧宝汽车),还有按价格降序排序,并在最后切断10辆最昂贵的汽车。但是中间的投影呢?这只是一种使用现有变量在阶段中创建新变量的方法。
相关文章:

FastAPI+React全栈开发10 MongoDB聚合查询
Chapter02 Setting Up the Document Store with MongoDB 10 Aggregation framework FastAPIReact全栈开发10 MongoDB聚合查询 In the following pages, we will try to provide a brief introducton to the MongoDB aggregation framework, what it is, what benefits it of…...

python 报错问题汇总
error: [WinError 32] 另一个程序正在使用此文件,进程无法访问。: d:\\anaconda\\envs\\yolov5\\lib\\site-packages\\ISR-2.2.0-py3.7.egg 解决方法:重启pycharm python-contrib 无法安装 opencv-contrib-python 安装包网址:安装包下载链接…...
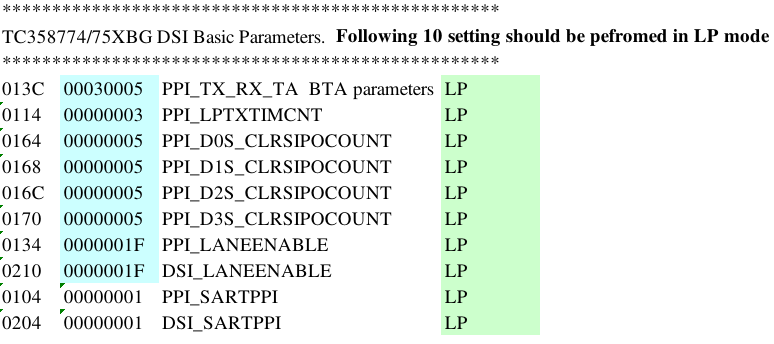
6.5物联网RK3399项目开发实录-驱动开发之LCD显示屏使用(wulianjishu666)
90款行业常用传感器单片机程序及资料【stm32,stc89c52,arduino适用】 链接:https://pan.baidu.com/s/1M3u8lcznKuXfN8NRoLYtTA?pwdc53f LCD使用 简介 AIO-3399J开发板外置了两个LCD屏接口,一个是EDP,一个是LVDS,接口对应板…...
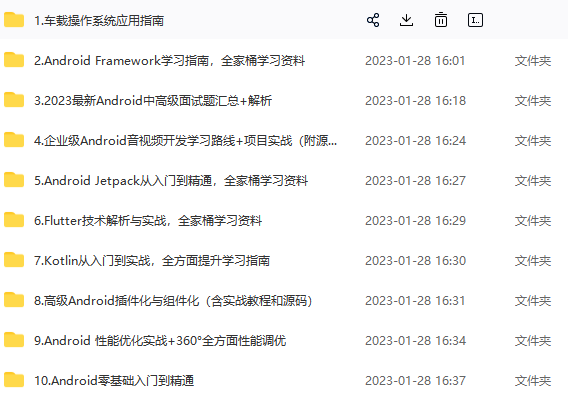
「Android高级工程师」BAT大厂面试基础题集合-下-Github标星6-5K
C、 com.android.provider.contact D、 com.android.provider.contacts 11.下面关于ContentProvider描述错误的是()。 A、 ContentProvider可以暴露数据 B、 ContentProvider用于实现跨程序共享数据 C、 ContentProvider不是四大组件 D、 ContentP…...

【算法】基数排序
简介 基数排序(*Radix sort)是一种非比较排序算法(non-comparative sorting algorithm)。现代计算机的基数排序算法由 计数排序 算法的开发人哈罗德H西华德(Harold H. Seward)于1954年于麻省理工大学开发。…...
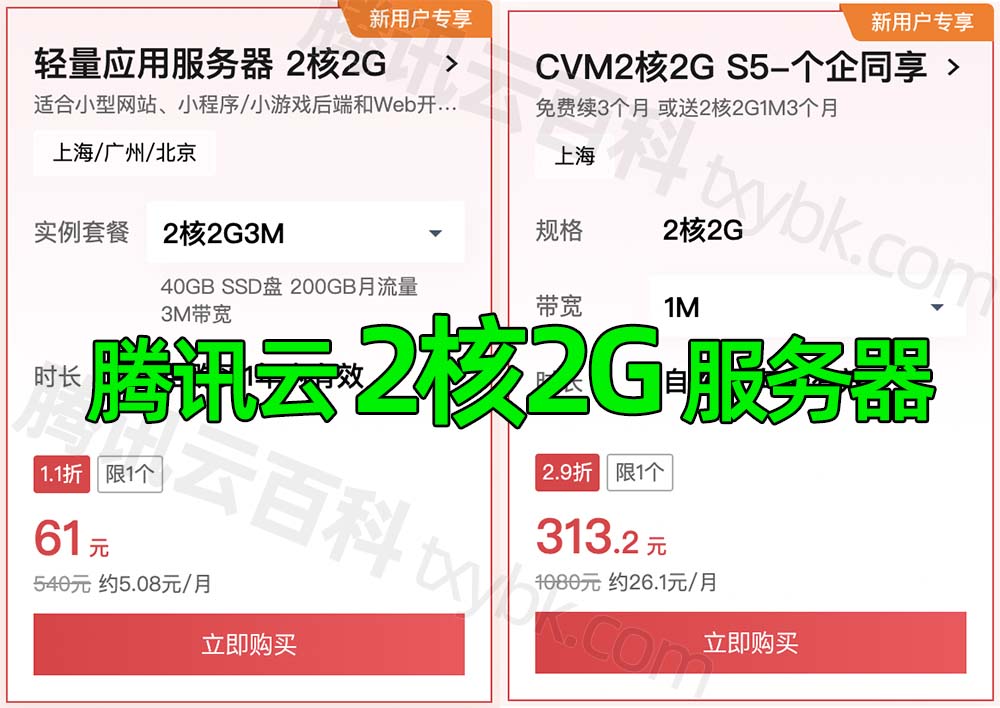
2核2G服务器优惠价格轻量61元一年,CVM价格313元15个月
腾讯云2核2G服务器多少钱一年?轻量服务器61元一年,CVM 2核2G S5服务器313.2元15个月,轻量2核2G3M带宽、40系统盘,云服务器CVM S5实例是2核2G、50G系统盘。腾讯云2核2G服务器优惠活动 txybk.com/go/txy 链接打开如下图:…...
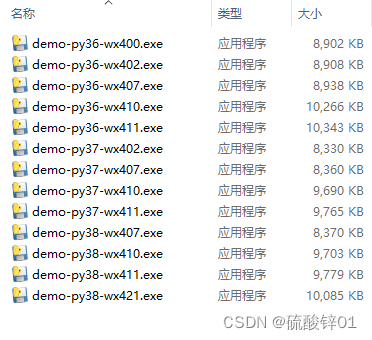
不同Python版本和wxPython版本用pyinstaller打包文件大小对比
1、确定wxPython和Python版本的对应关系 在这里可以找到Python支持的所有wxPython版本:https://pypi.tuna.tsinghua.edu.cn/simple/wxpython/ 由于Python从3.6版本开始支持f字符串、从3.9版本开始不支持Windows7操作系统,所以我仅筛选3.6-3.8之间的版本…...
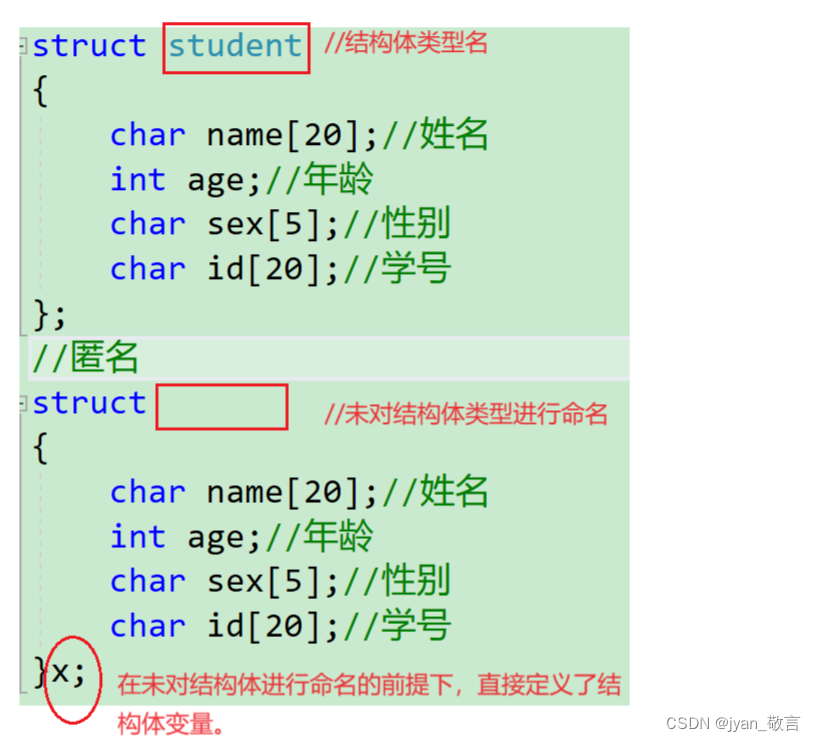
【C语言】结构体详解(一)
目录 1、什么是结构体? 2、结构体成分 3、结构体变量的定义与初始化 3.1、结构体变量的三种定义方式 3.2、结构体变量的初始化 4、结构体成员的访问(两种方式) 4.1、直接访问 4.2、间接访问 5、结构的特殊声明 5.1、不完全声明(匿…...
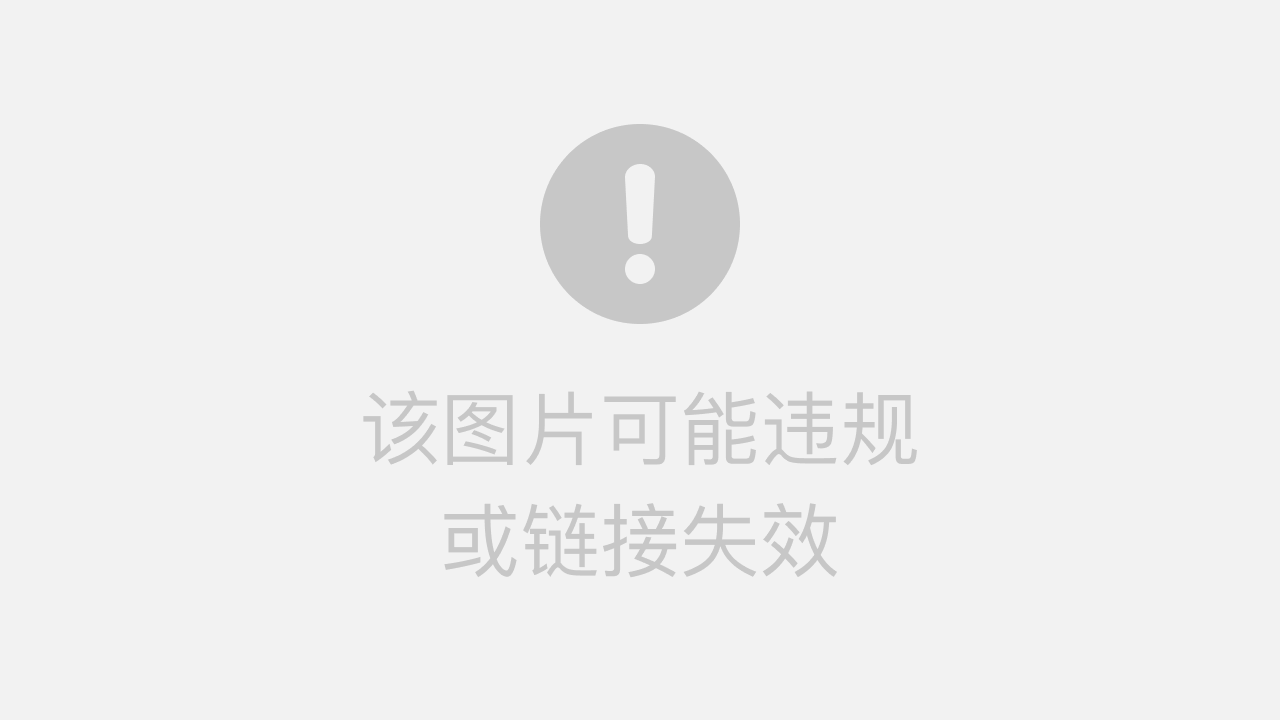
AI时代-普通人的AI绘画工具对比(Midjouney与Stable Diffusion)
AI时代-普通人的AI绘画工具对比(Midjouney与Stable Diffusion) 前言1、基础对比Stable Diffusion(SD)SD界面安装与使用SD Midjouney(MJ) 2、硬件与运行要求对比Stable Diffusion硬件要求内存硬盘显卡 Midjo…...

【蓝桥杯】矩阵快速幂
一.快速幂概述 1.引例 1)题目描述: 求A^B的最后三位数表示的整数,A^B表示:A的B次方。 2)思路: 一般的思路是:求出A的B次幂,再取结果的最后三位数。但是由于计算机能够表示的数字…...
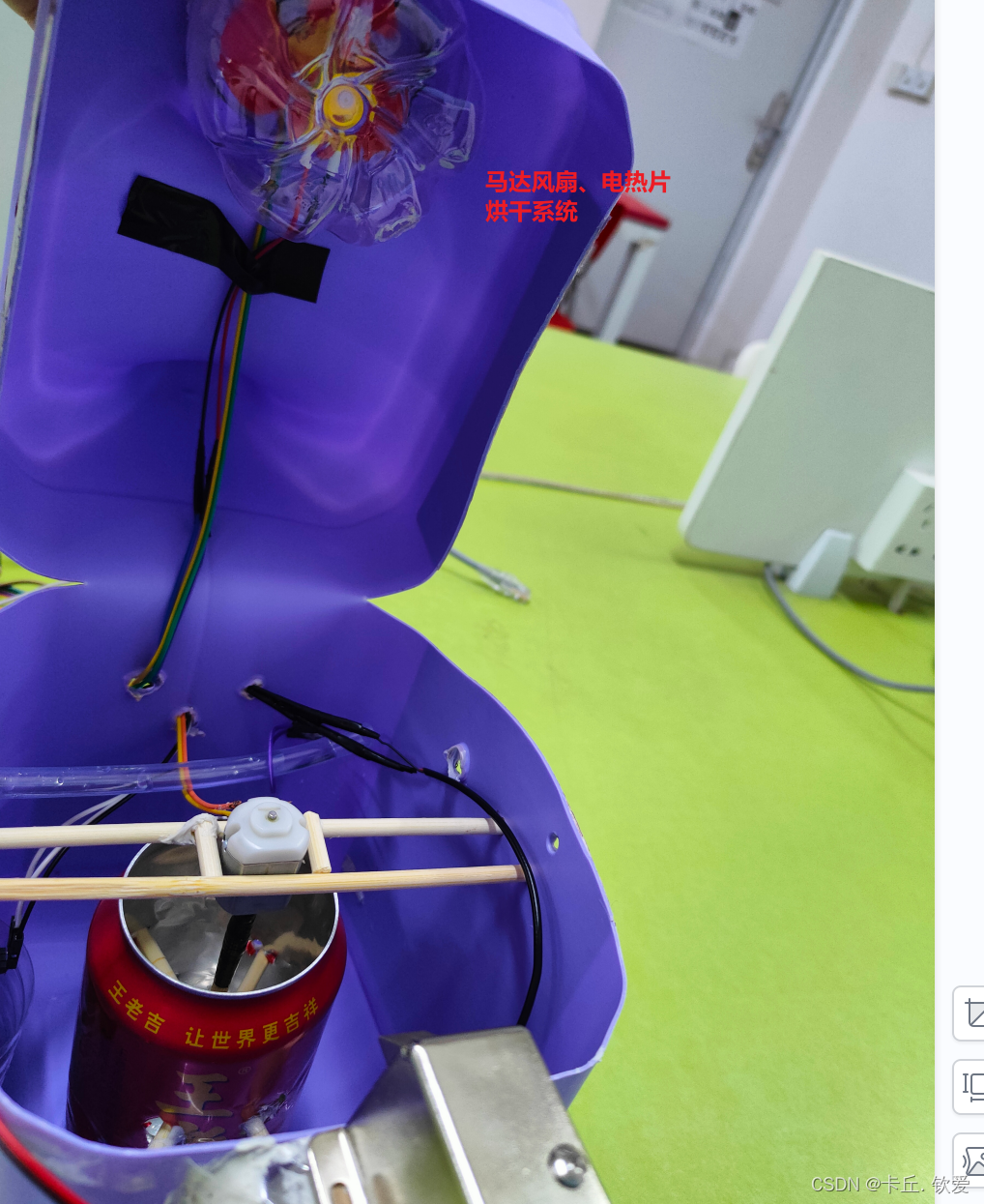
C语言使用STM32开发板手搓高端家居洗衣机
目录 概要 成品效果 背景概述 1.开发环境 2.主要传感器。 技术细节 1. 用户如何知道选择了何种功能 2.启动后如何进行洗衣 3.如何将洗衣机状态上传至服务器并通过APP查看 4.洗衣过程、可燃气检测、OLED屏显示、服务器通信如何并发进行 小结 概要 本文章主要是讲解如…...
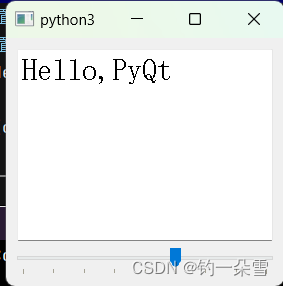
【Hello,PyQt】QTextEdit和QSplider
PyQt5 是一个强大的Python库,用于创建图形用户界面(GUI)。其中,QTextEdit 控件作为一个灵活多用的组件,常用于显示和编辑多行文本内容,支持丰富的格式设置和文本操作功能。另外,QSlider 控件是一…...

【力扣】191.位 1 的个数、485.最大连续 1 的个数
191.位 1 的个数 题目描述 编写一个函数,输入是一个无符号整数(以二进制串的形式),返回其二进制表达式中 设置位 的个数(也被称为汉明重量)。 示例 1: 输入:n 11 输出࿱…...
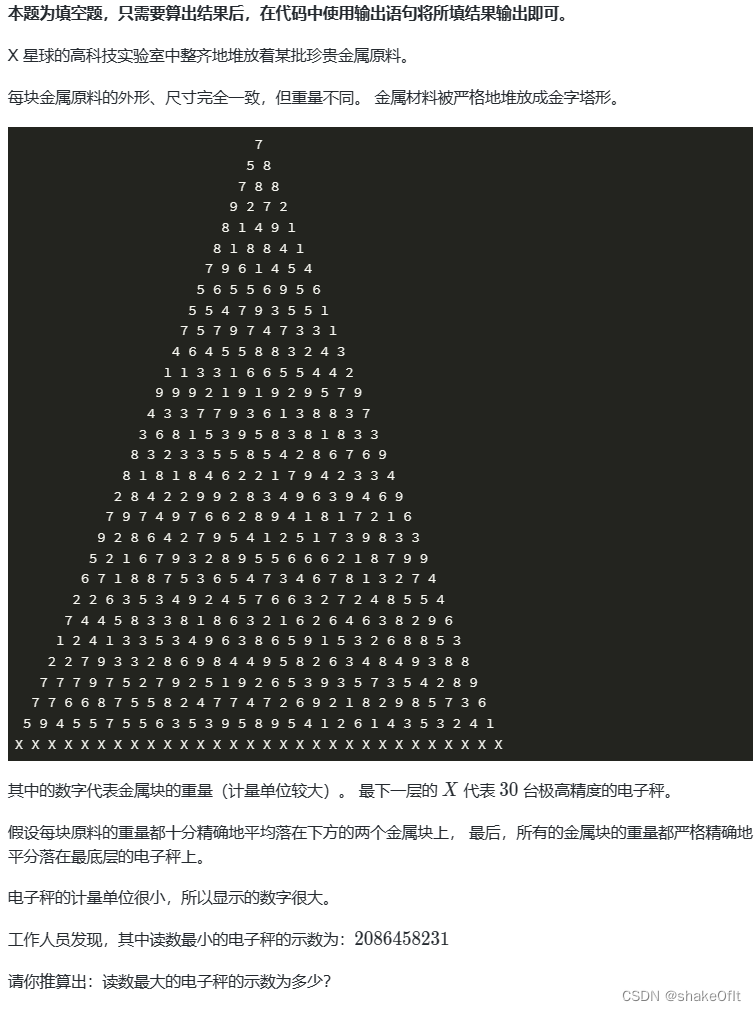
蓝桥杯 java 承压计算
题目: 思路: 1:其中的数字代表金属块的重量(计量单位较大) 说明每个数字后面不一定有多少个0 2:假设每块原料的重量都十分精确地平均落在下方的两个金属块上,最后,所有的金属块的重量都严格精确地平分落在最底层的电子…...

leetcode268-Missing Number
这道题目要求缺失的数字,一般解决数组的问题,要么往排序数组,要么往双指针遍历这些方向上靠,要么往异或方向上靠,总之落点无非就只有这几个。我们要求缺失的数字,可以依次让1~n和数组元素进行异…...
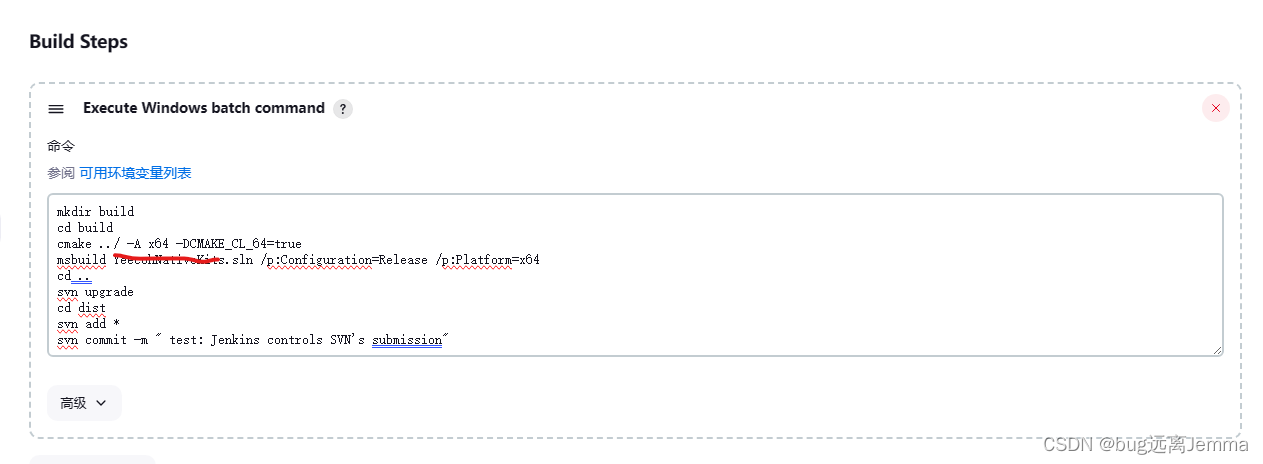
【jenkins+cmake+svn管理c++项目】jenkins回传文件到svn(windows)
书接上文:创建一个项目 在经过cmakemsbuild顺利生成动态库之后,考虑到我一个项目可能会生成多个动态库,它们分散在build内的不同文件夹,我希望能将它们收拢到一个文件夹下,并将其回传到svn。 一、动态库移位—cmake实…...
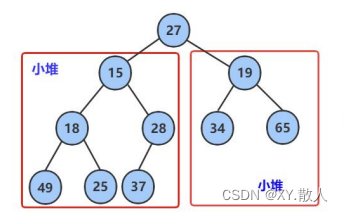
数据结构·二叉树(2)
目录 1 堆的概念 2 堆的实现 2.1 堆的初始化和销毁 2.2 获取堆顶数据和堆的判空 2.3 堆的向上调整算法 2.4 堆的向下调整算法 2.4 堆的插入 2.5 删除堆顶数据 2.6 建堆 3 建堆的时间复杂度 3.1 向上建堆的时间复杂度 3.2向下建堆的时间复杂度 4 堆的排序 前言&…...

MATLAB算法实战应用案例精讲-【毕业季论文专用】人工智能视觉检测技术及其在实际应用中的挑战与前景
目录 摘要: 第一章:引言 1.1 研究背景 1.2 研究目的与意义...
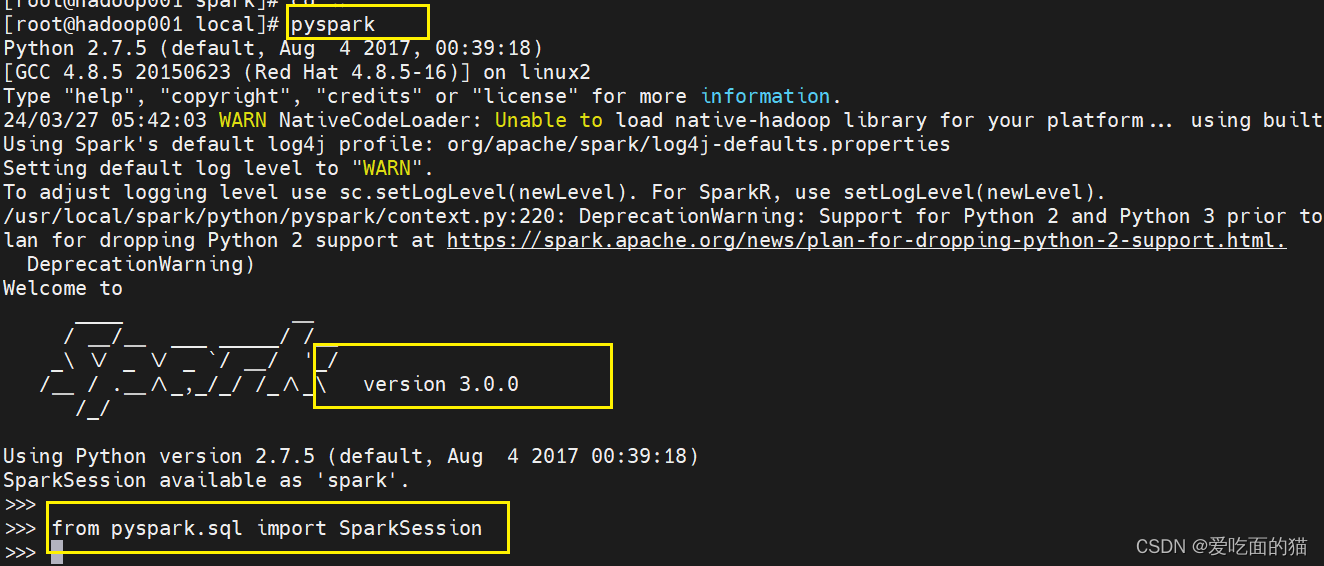
Linux虚拟机环境搭建spark
Linux环境搭建Spark分为两个版本,分别是Scala版本和Python版本。 一、 安装Pyspark 本环境以 Python 环境为例。 1、下载spark 下载网址:https://archive.apache.org/dist/spark 下载安装包:根据自己环境选择合适版本,本环境…...
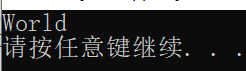
STL的string容器
string基本概念 string是C风格的字符串,本质上是一个类。 string 和 char* 的区别 char* 是一个指针; string是一个类,内部封装了 char* ,用来管理字符串,是一个 char* 型的容器。 特点 string内部封装了很多成员…...
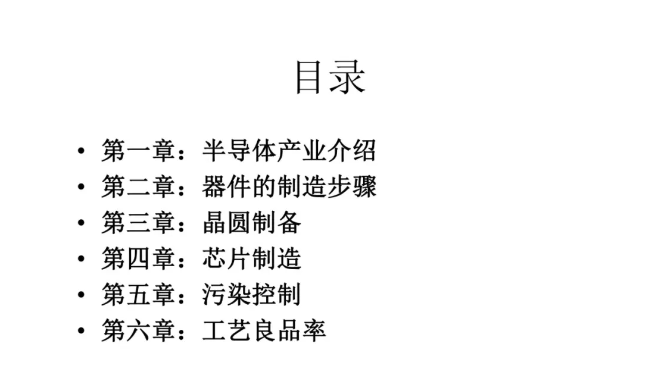
半导体工艺技术
完整内容点击:【半导体工艺技术】...

acwing算法提高之图论--单源最短路的扩展应用
目录 1 介绍2 训练 1 介绍 本专题用来记录使用。。。。 2 训练 题目1:1137选择最佳线路 C代码如下, #include <iostream> #include <cstring> #include <algorithm> #include <queue>using namespace std;const int N 101…...

SQLServer数据库使用Function实现根据字段内容的拼音首字母进行数据查询
实现SQL首字母查询分两步,第一步建Function,第二步引用新建的Function。 1. 首先需要自定义一个查询的Function,详细SQL如下: ALTER function [dbo].[GetDataByPY](str nvarchar(4000)) returns nvarchar(4000) as begin decla…...
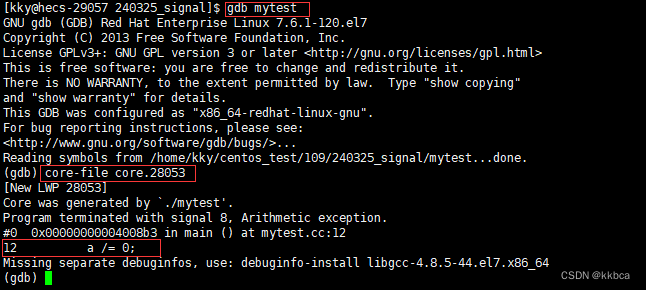
Linux——信号概念与信号产生方式
目录 一、概念 二、前台进程与后台进程 1.ctrlc 2.ctrlz 三、信号的产生方式 1.键盘输入产生信号 2.系统调用发送信号 2.1 kill()函数 2.2 raise()函数 2.3 abort()函数 3.异常导致信号产生 3.1 除0异常 3.2 段错误异常 4.软件条件产生信号 4.1 管道 4.2 闹钟…...
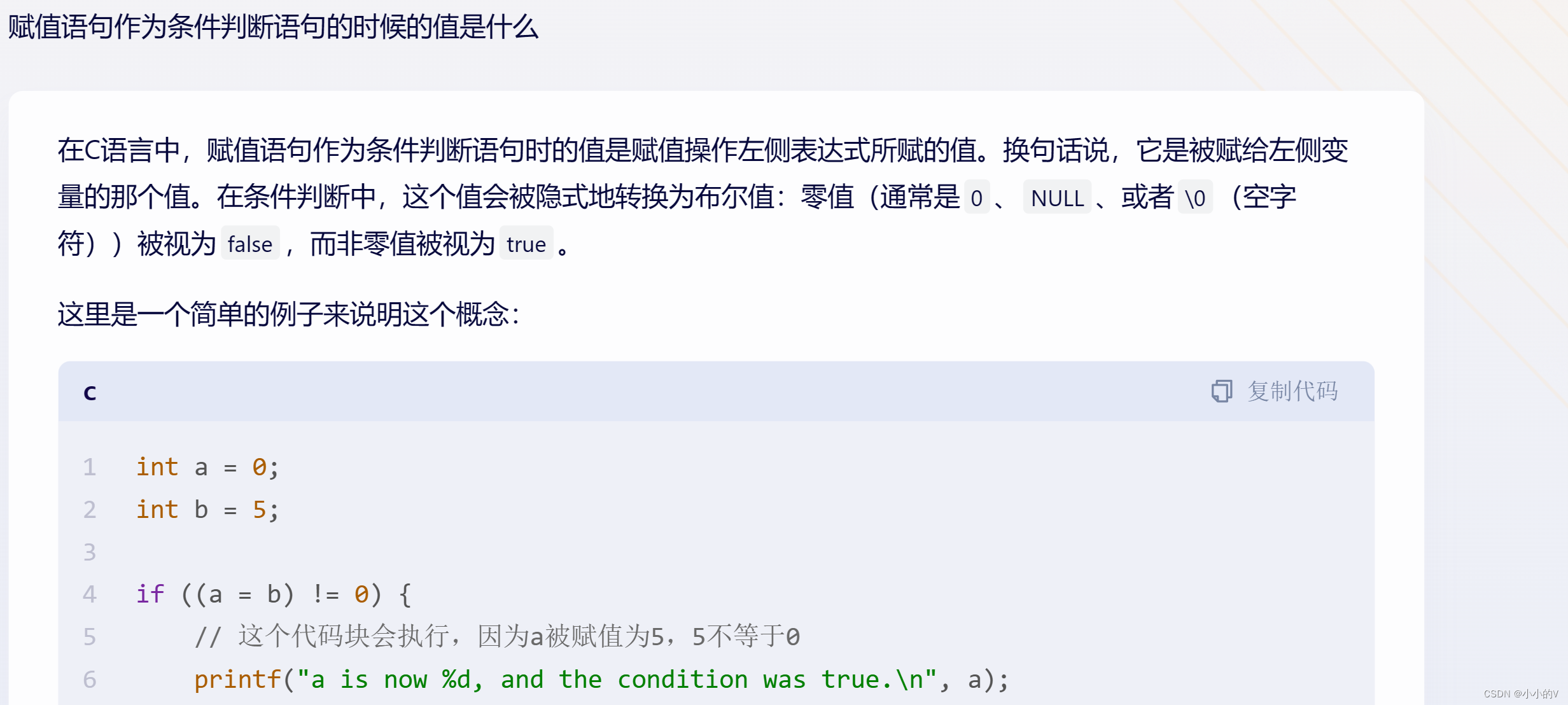
赋值语句还能当判断条件?涨芝士了!
赋值和条件看似是C语言中毫不相关的两个概念,虽然实际过程中我猜测不会有太多这种不太符合常理的情况出现,但是现在在学习的过程中,为了出题而出题总是会整出一些花活出来.....这很难不让人联想起高中时一些大佬为了彰显自己的数学天赋而自己…...

数据结构 - 算法效率|时间复杂度|空间复杂度
目录 1.算法效率 2.时间复杂度 2.1定义 2.2大O渐近表示法 2.3常见时间复杂度计算举例 3.空间复杂度 3.1定义 3.2常见空间复杂度计算举例 1.算法效率 算法的效率常用算法复杂度来衡量,算法复杂度描述了算法在输入数据规模变化时,其运行时间和空间…...
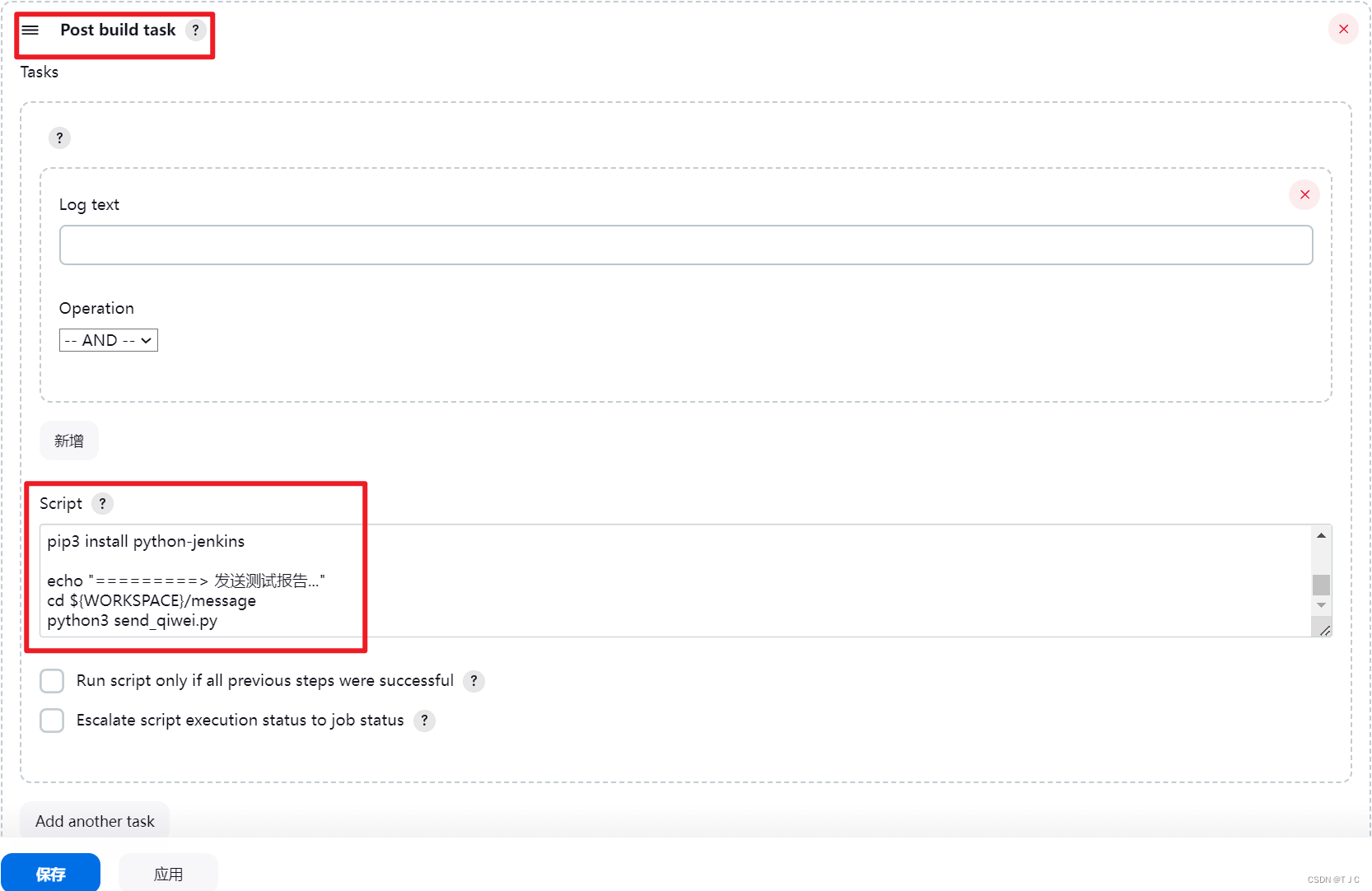
接口自动化之 + Jenkins + Allure报告生成 + 企微消息通知推送
接口自动化之 Jenkins Allure报告生成 企微消息通知推送 在jenkins上部署好项目,构建成功后,希望可以把生成的报告,以及结果统计发送至企微。 效果图: 实现如下。 1、生成allure报告 a. 首先在Jenkins插件管理中&#x…...
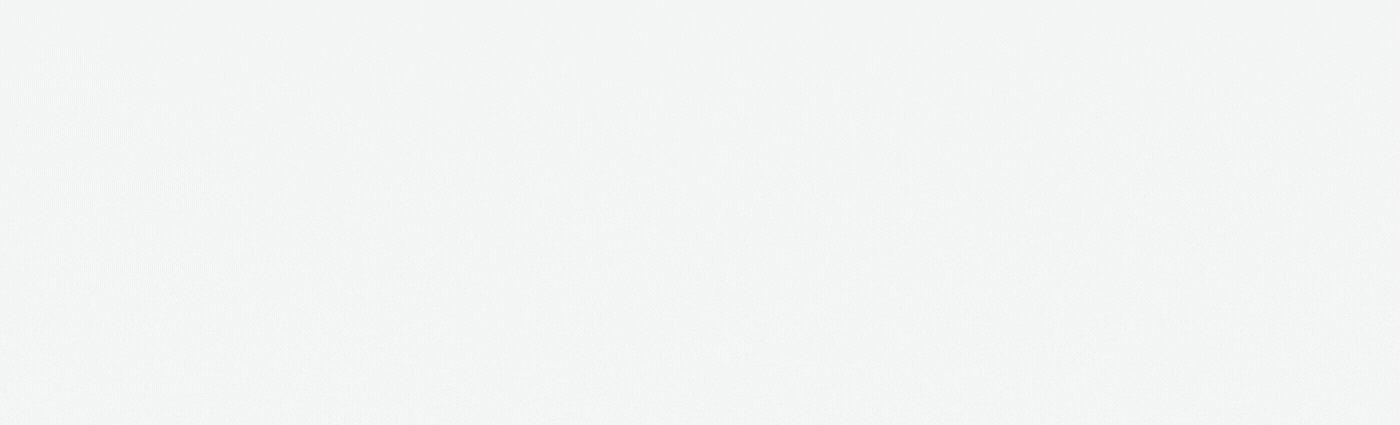
『Apisix安全篇』探索Apache APISIX身份认证插件:从基础到实战
🚀『Apisix系列文章』探索新一代微服务体系下的API管理新范式与最佳实践 【点击此跳转】 📣读完这篇文章里你能收获到 🛠️ 了解APISIX身份认证的重要性和基本概念,以及如何在微服务架构中实施API安全。🔑 学习如何使…...
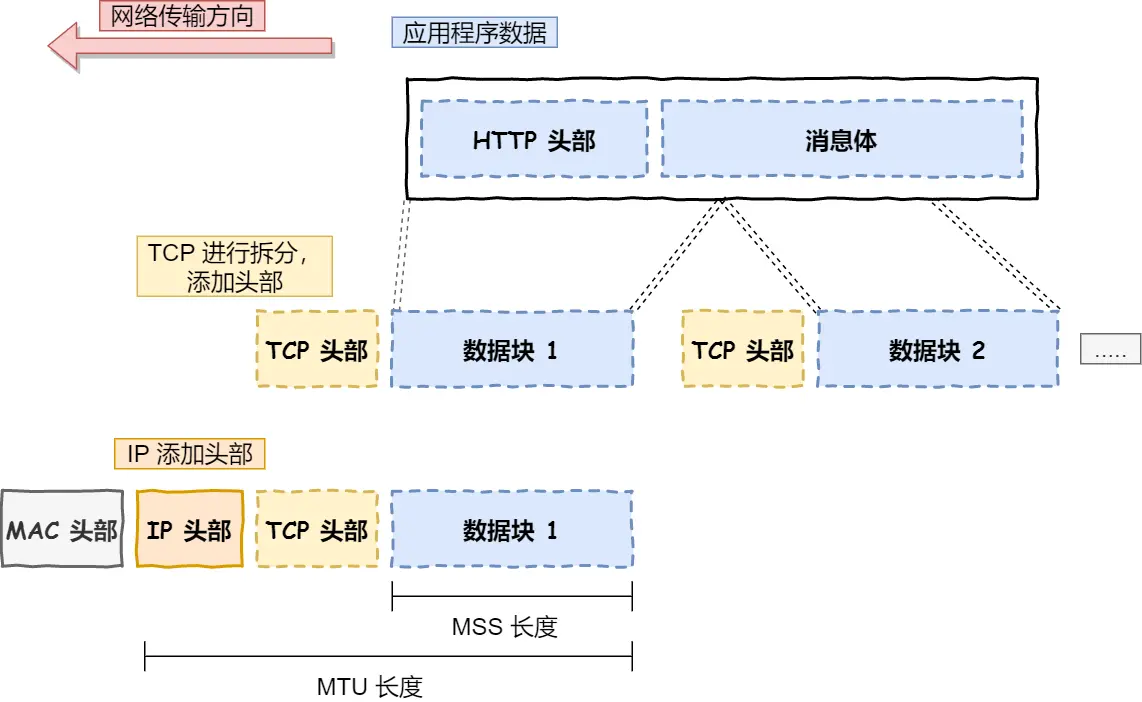
【01-20】计算机网络基础知识(非常详细)从零基础入门到精通,看完这一篇就够了
【01-20】计算机网络基础知识(非常详细)从零基础入门到精通,看完这一篇就够了 以下是本文参考的资料 欢迎大家查收原版 本版本仅作个人笔记使用1、OSI 的七层模型分别是?各自的功能是什么?2、说一下一次完整的HTTP请求…...

『大模型笔记』常见的分布式并行策略(分布式训练)
常见的分布式并行策略(分布式训练) 文章目录 一. 为什么分布式训练越来越流行二. 常见的并行策略2.1 数据并行2.2 模型并行2.3 流水并行2.4 混合并行二. 参考文献一. 为什么分布式训练越来越流行 近年来,深度学习被广泛应用到各个领域,包括计算机视觉、语言理解、语音识别、广…...

成都品牌包装设计/企业网站优化哪家好
2019独角兽企业重金招聘Python工程师标准>>> 方法1 - 使用Eclipse Eclipse里新建一个服务器: 服务器类型选择SAP Cloud Platform: 点Finish,成功创建了一个Server: Eclipse里选择要部署的项目,右键->…...

电商网站开发票税率/搜资源的搜索引擎
#include<linux/gpio.h> // 标准 GPIO_API intgpio_request(unsigned gpio, const char *label); 获得并占有 GPIO>。在/proc/mem应该会有地址占用表描述。 这种用法的保护作用前提是大家都遵守先申请再访问,有一个地方没遵守这个规则,这功能就…...

快速搭建外贸网站/免费网站大全
无线测试系统LitePointIQview提供强大工具,能取得并测量WiFi及蓝牙讯号,并以分析功能提供装置运作说明,简化了WiFi与Bluetooth装置的开发过程。IQview与IQflex製造解决方桉完全相容,使得以IQview开发的测试软体也能在已生产线使用…...
qq企业邮箱注册申请/站长工具seo综合查询怎么用
这是Java EE框架开发技术一书中贯穿整本书的一个练习系统(作业)。代码一共有两个部分第一部分是第五章的作业,完成如下使用原生的jdbc代码进行数据库的连接界面和书本完全相同除部分js代码用jq代替外其他代码均相同课后扩展实现除头像上传外基本完成第二部分是最后一…...
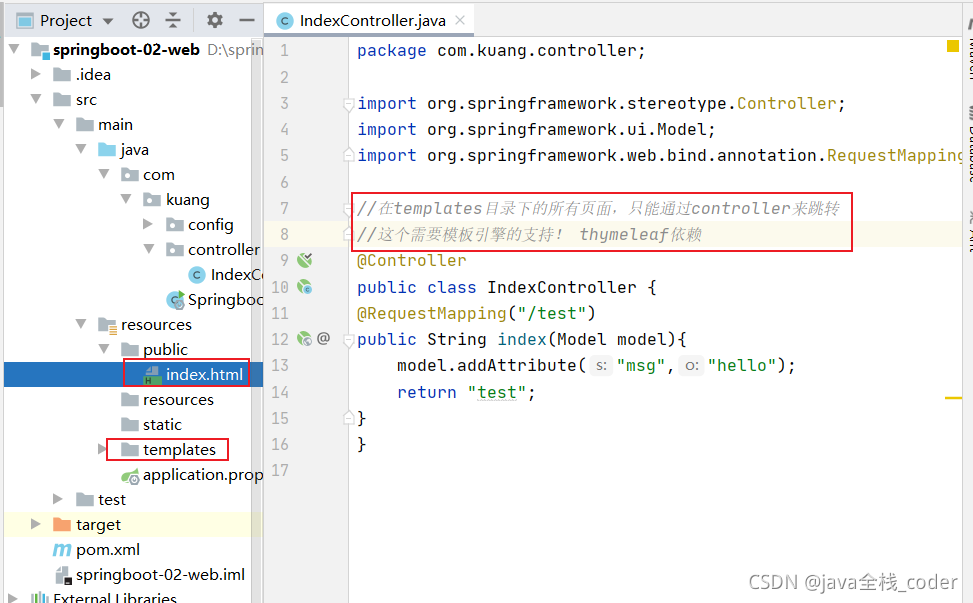
wordpress怎么重装/百度知道灰色词代发收录
首页和图标定制 首页 图标定制 1.图片命名为favicon.ico 2.放在public目录下 3.更改配置 #关闭默认图标 ,新版本没有 spring.mvc.favicon.enabledfalse4.测试访问...

做游戏排行榜的网站模板/怎么在网上销售
本文实例讲述了Python实现的拟合二元一次函数功能。分享给大家供大家参考,具体如下:背景:使用scipy拟合一元二次函数。参考:HYRY Studio-《用Python做科学计算》代码:# -*- coding:utf-8 -*-#! python3import numpy as…...