政安晨:【Keras机器学习示例演绎】(五十四)—— 使用神经决策森林进行分类
目录
导言
数据集
设置
准备数据
定义数据集元数据
为训练和验证创建 tf_data.Dataset 对象
创建模型输入
输入特征编码
深度神经决策树
深度神经决策森林
实验 1:训练决策树模型
实验 2:训练森林模型
政安晨的个人主页:政安晨
欢迎 👍点赞✍评论⭐收藏
收录专栏: TensorFlow与Keras机器学习实战
希望政安晨的博客能够对您有所裨益,如有不足之处,欢迎在评论区提出指正!
本文目标:如何为深度神经网络的端到端学习训练可微分决策树。
导言
本示例提供了 P. Kontschieder 等人提出的用于结构化数据分类的深度神经决策林模型的实现。 它演示了如何建立一个随机可变的决策树模型,对其进行端到端训练,并将决策树与深度表示学习统一起来。
数据集
本示例使用加州大学欧文分校机器学习资料库提供的美国人口普查收入数据集。 该数据集包含 48,842 个实例,其中有 14 个输入特征(如年龄、工作级别、教育程度、职业等): 5 个数字特征和 9 个分类特征。
设置
import keras
from keras import layers
from keras.layers import StringLookup
from keras import opsfrom tensorflow import data as tf_data
import numpy as np
import pandas as pdimport math
准备数据
CSV_HEADER = ["age","workclass","fnlwgt","education","education_num","marital_status","occupation","relationship","race","gender","capital_gain","capital_loss","hours_per_week","native_country","income_bracket",
]train_data_url = ("https://archive.ics.uci.edu/ml/machine-learning-databases/adult/adult.data"
)
train_data = pd.read_csv(train_data_url, header=None, names=CSV_HEADER)test_data_url = ("https://archive.ics.uci.edu/ml/machine-learning-databases/adult/adult.test"
)
test_data = pd.read_csv(test_data_url, header=None, names=CSV_HEADER)print(f"Train dataset shape: {train_data.shape}")
print(f"Test dataset shape: {test_data.shape}")
Train dataset shape: (32561, 15)
Test dataset shape: (16282, 15)
删除第一条记录(因为它不是一个有效的数据示例)和类标签中的尾部 "点"。
test_data = test_data[1:]
test_data.income_bracket = test_data.income_bracket.apply(lambda value: value.replace(".", "")
)
我们将训练数据和测试数据分割成 CSV 文件存储在本地。
train_data_file = "train_data.csv"
test_data_file = "test_data.csv"train_data.to_csv(train_data_file, index=False, header=False)
test_data.to_csv(test_data_file, index=False, header=False)
定义数据集元数据
在此,我们定义了数据集的元数据,这些元数据将有助于读取、解析和编码输入特征。
# A list of the numerical feature names.
NUMERIC_FEATURE_NAMES = ["age","education_num","capital_gain","capital_loss","hours_per_week",
]
# A dictionary of the categorical features and their vocabulary.
CATEGORICAL_FEATURES_WITH_VOCABULARY = {"workclass": sorted(list(train_data["workclass"].unique())),"education": sorted(list(train_data["education"].unique())),"marital_status": sorted(list(train_data["marital_status"].unique())),"occupation": sorted(list(train_data["occupation"].unique())),"relationship": sorted(list(train_data["relationship"].unique())),"race": sorted(list(train_data["race"].unique())),"gender": sorted(list(train_data["gender"].unique())),"native_country": sorted(list(train_data["native_country"].unique())),
}
# A list of the columns to ignore from the dataset.
IGNORE_COLUMN_NAMES = ["fnlwgt"]
# A list of the categorical feature names.
CATEGORICAL_FEATURE_NAMES = list(CATEGORICAL_FEATURES_WITH_VOCABULARY.keys())
# A list of all the input features.
FEATURE_NAMES = NUMERIC_FEATURE_NAMES + CATEGORICAL_FEATURE_NAMES
# A list of column default values for each feature.
COLUMN_DEFAULTS = [[0.0] if feature_name in NUMERIC_FEATURE_NAMES + IGNORE_COLUMN_NAMES else ["NA"]for feature_name in CSV_HEADER
]
# The name of the target feature.
TARGET_FEATURE_NAME = "income_bracket"
# A list of the labels of the target features.
TARGET_LABELS = [" <=50K", " >50K"]
为训练和验证创建 tf_data.Dataset 对象
我们创建了一个输入函数来读取和解析文件,并将特征和标签转换为 tf_data.Dataset 用于训练和验证。 我们还通过将目标标签映射到索引对输入进行预处理。
target_label_lookup = StringLookup(vocabulary=TARGET_LABELS, mask_token=None, num_oov_indices=0
)lookup_dict = {}
for feature_name in CATEGORICAL_FEATURE_NAMES:vocabulary = CATEGORICAL_FEATURES_WITH_VOCABULARY[feature_name]# Create a lookup to convert a string values to an integer indices.# Since we are not using a mask token, nor expecting any out of vocabulary# (oov) token, we set mask_token to None and num_oov_indices to 0.lookup = StringLookup(vocabulary=vocabulary, mask_token=None, num_oov_indices=0)lookup_dict[feature_name] = lookupdef encode_categorical(batch_x, batch_y):for feature_name in CATEGORICAL_FEATURE_NAMES:batch_x[feature_name] = lookup_dict[feature_name](batch_x[feature_name])return batch_x, batch_ydef get_dataset_from_csv(csv_file_path, shuffle=False, batch_size=128):dataset = (tf_data.experimental.make_csv_dataset(csv_file_path,batch_size=batch_size,column_names=CSV_HEADER,column_defaults=COLUMN_DEFAULTS,label_name=TARGET_FEATURE_NAME,num_epochs=1,header=False,na_value="?",shuffle=shuffle,).map(lambda features, target: (features, target_label_lookup(target))).map(encode_categorical))return dataset.cache()
创建模型输入
def create_model_inputs():inputs = {}for feature_name in FEATURE_NAMES:if feature_name in NUMERIC_FEATURE_NAMES:inputs[feature_name] = layers.Input(name=feature_name, shape=(), dtype="float32")else:inputs[feature_name] = layers.Input(name=feature_name, shape=(), dtype="int32")return inputs
输入特征编码
def encode_inputs(inputs):encoded_features = []for feature_name in inputs:if feature_name in CATEGORICAL_FEATURE_NAMES:vocabulary = CATEGORICAL_FEATURES_WITH_VOCABULARY[feature_name]# Create a lookup to convert a string values to an integer indices.# Since we are not using a mask token, nor expecting any out of vocabulary# (oov) token, we set mask_token to None and num_oov_indices to 0.value_index = inputs[feature_name]embedding_dims = int(math.sqrt(lookup.vocabulary_size()))# Create an embedding layer with the specified dimensions.embedding = layers.Embedding(input_dim=lookup.vocabulary_size(), output_dim=embedding_dims)# Convert the index values to embedding representations.encoded_feature = embedding(value_index)else:# Use the numerical features as-is.encoded_feature = inputs[feature_name]if inputs[feature_name].shape[-1] is None:encoded_feature = keras.ops.expand_dims(encoded_feature, -1)encoded_features.append(encoded_feature)encoded_features = layers.concatenate(encoded_features)return encoded_features
深度神经决策树
神经决策树模型有两组权重需要学习。 第一组是 pi,代表树叶中类别的概率分布。 第二组是路由层 decision_fn 的权重,代表前往每个树叶的概率。 该模型的前向传递工作原理如下:
该模型希望将输入特征作为一个单一的向量,对批次中某个实例的所有特征进行编码。 该向量可以由应用于图像的卷积神经网络(CNN)生成,也可以由应用于结构化数据特征的密集变换生成。
模型首先应用已用特征掩码随机选择要使用的输入特征子集。
然后,模型通过在树的各个层级迭代执行随机路由,计算输入实例到达树叶的概率(mu)。
最后,将到达树叶的概率与树叶上的类概率相结合,生成最终输出。
class NeuralDecisionTree(keras.Model):def __init__(self, depth, num_features, used_features_rate, num_classes):super().__init__()self.depth = depthself.num_leaves = 2**depthself.num_classes = num_classes# Create a mask for the randomly selected features.num_used_features = int(num_features * used_features_rate)one_hot = np.eye(num_features)sampled_feature_indices = np.random.choice(np.arange(num_features), num_used_features, replace=False)self.used_features_mask = ops.convert_to_tensor(one_hot[sampled_feature_indices], dtype="float32")# Initialize the weights of the classes in leaves.self.pi = self.add_weight(initializer="random_normal",shape=[self.num_leaves, self.num_classes],dtype="float32",trainable=True,)# Initialize the stochastic routing layer.self.decision_fn = layers.Dense(units=self.num_leaves, activation="sigmoid", name="decision")def call(self, features):batch_size = ops.shape(features)[0]# Apply the feature mask to the input features.features = ops.matmul(features, ops.transpose(self.used_features_mask)) # [batch_size, num_used_features]# Compute the routing probabilities.decisions = ops.expand_dims(self.decision_fn(features), axis=2) # [batch_size, num_leaves, 1]# Concatenate the routing probabilities with their complements.decisions = layers.concatenate([decisions, 1 - decisions], axis=2) # [batch_size, num_leaves, 2]mu = ops.ones([batch_size, 1, 1])begin_idx = 1end_idx = 2# Traverse the tree in breadth-first order.for level in range(self.depth):mu = ops.reshape(mu, [batch_size, -1, 1]) # [batch_size, 2 ** level, 1]mu = ops.tile(mu, (1, 1, 2)) # [batch_size, 2 ** level, 2]level_decisions = decisions[:, begin_idx:end_idx, :] # [batch_size, 2 ** level, 2]mu = mu * level_decisions # [batch_size, 2**level, 2]begin_idx = end_idxend_idx = begin_idx + 2 ** (level + 1)mu = ops.reshape(mu, [batch_size, self.num_leaves]) # [batch_size, num_leaves]probabilities = keras.activations.softmax(self.pi) # [num_leaves, num_classes]outputs = ops.matmul(mu, probabilities) # [batch_size, num_classes]return outputs
深度神经决策森林
神经决策森林模型由一组同时训练的神经决策树组成。 森林模型的输出是各树的平均输出。
class NeuralDecisionForest(keras.Model):def __init__(self, num_trees, depth, num_features, used_features_rate, num_classes):super().__init__()self.ensemble = []# Initialize the ensemble by adding NeuralDecisionTree instances.# Each tree will have its own randomly selected input features to use.for _ in range(num_trees):self.ensemble.append(NeuralDecisionTree(depth, num_features, used_features_rate, num_classes))def call(self, inputs):# Initialize the outputs: a [batch_size, num_classes] matrix of zeros.batch_size = ops.shape(inputs)[0]outputs = ops.zeros([batch_size, num_classes])# Aggregate the outputs of trees in the ensemble.for tree in self.ensemble:outputs += tree(inputs)# Divide the outputs by the ensemble size to get the average.outputs /= len(self.ensemble)return outputs
最后,让我们来设置训练和评估模型的代码。
learning_rate = 0.01
batch_size = 265
num_epochs = 10def run_experiment(model):model.compile(optimizer=keras.optimizers.Adam(learning_rate=learning_rate),loss=keras.losses.SparseCategoricalCrossentropy(),metrics=[keras.metrics.SparseCategoricalAccuracy()],)print("Start training the model...")train_dataset = get_dataset_from_csv(train_data_file, shuffle=True, batch_size=batch_size)model.fit(train_dataset, epochs=num_epochs)print("Model training finished")print("Evaluating the model on the test data...")test_dataset = get_dataset_from_csv(test_data_file, batch_size=batch_size)_, accuracy = model.evaluate(test_dataset)print(f"Test accuracy: {round(accuracy * 100, 2)}%")
实验 1:训练决策树模型
在本实验中,我们使用所有输入特征训练一个神经决策树模型。
num_trees = 10
depth = 10
used_features_rate = 1.0
num_classes = len(TARGET_LABELS)def create_tree_model():inputs = create_model_inputs()features = encode_inputs(inputs)features = layers.BatchNormalization()(features)num_features = features.shape[1]tree = NeuralDecisionTree(depth, num_features, used_features_rate, num_classes)outputs = tree(features)model = keras.Model(inputs=inputs, outputs=outputs)return modeltree_model = create_tree_model()
run_experiment(tree_model)
Start training the model...
Epoch 1/10123/123 ━━━━━━━━━━━━━━━━━━━━ 5s 26ms/step - loss: 0.5308 - sparse_categorical_accuracy: 0.8150
Epoch 2/10123/123 ━━━━━━━━━━━━━━━━━━━━ 1s 11ms/step - loss: 0.3476 - sparse_categorical_accuracy: 0.8429
Epoch 3/10123/123 ━━━━━━━━━━━━━━━━━━━━ 1s 11ms/step - loss: 0.3312 - sparse_categorical_accuracy: 0.8478
Epoch 4/10123/123 ━━━━━━━━━━━━━━━━━━━━ 1s 11ms/step - loss: 0.3247 - sparse_categorical_accuracy: 0.8495
Epoch 5/10123/123 ━━━━━━━━━━━━━━━━━━━━ 1s 10ms/step - loss: 0.3202 - sparse_categorical_accuracy: 0.8512
Epoch 6/10123/123 ━━━━━━━━━━━━━━━━━━━━ 1s 11ms/step - loss: 0.3158 - sparse_categorical_accuracy: 0.8536
Epoch 7/10123/123 ━━━━━━━━━━━━━━━━━━━━ 1s 11ms/step - loss: 0.3116 - sparse_categorical_accuracy: 0.8572
Epoch 8/10123/123 ━━━━━━━━━━━━━━━━━━━━ 1s 11ms/step - loss: 0.3071 - sparse_categorical_accuracy: 0.8608
Epoch 9/10123/123 ━━━━━━━━━━━━━━━━━━━━ 1s 11ms/step - loss: 0.3026 - sparse_categorical_accuracy: 0.8630
Epoch 10/10123/123 ━━━━━━━━━━━━━━━━━━━━ 1s 10ms/step - loss: 0.2975 - sparse_categorical_accuracy: 0.8653
Model training finished
Evaluating the model on the test data...62/62 ━━━━━━━━━━━━━━━━━━━━ 1s 13ms/step - loss: 0.3279 - sparse_categorical_accuracy: 0.8463
Test accuracy: 85.08%
实验 2:训练森林模型
在本实验中,我们使用 num_trees 树训练神经决策森林,每棵树随机使用 50%的输入特征。 通过设置 used_features_rate 变量,可以控制每棵树使用的特征数量。 此外,与之前的实验相比,我们将深度设置为 5,而不是 10。
num_trees = 25
depth = 5
used_features_rate = 0.5def create_forest_model():inputs = create_model_inputs()features = encode_inputs(inputs)features = layers.BatchNormalization()(features)num_features = features.shape[1]forest_model = NeuralDecisionForest(num_trees, depth, num_features, used_features_rate, num_classes)outputs = forest_model(features)model = keras.Model(inputs=inputs, outputs=outputs)return modelforest_model = create_forest_model()run_experiment(forest_model)
Start training the model...
Epoch 1/10123/123 ━━━━━━━━━━━━━━━━━━━━ 47s 202ms/step - loss: 0.5469 - sparse_categorical_accuracy: 0.7915
Epoch 2/10123/123 ━━━━━━━━━━━━━━━━━━━━ 1s 10ms/step - loss: 0.3459 - sparse_categorical_accuracy: 0.8494
Epoch 3/10123/123 ━━━━━━━━━━━━━━━━━━━━ 1s 10ms/step - loss: 0.3268 - sparse_categorical_accuracy: 0.8523
Epoch 4/10123/123 ━━━━━━━━━━━━━━━━━━━━ 1s 10ms/step - loss: 0.3195 - sparse_categorical_accuracy: 0.8524
Epoch 5/10123/123 ━━━━━━━━━━━━━━━━━━━━ 1s 10ms/step - loss: 0.3149 - sparse_categorical_accuracy: 0.8539
Epoch 6/10123/123 ━━━━━━━━━━━━━━━━━━━━ 1s 10ms/step - loss: 0.3112 - sparse_categorical_accuracy: 0.8556
Epoch 7/10123/123 ━━━━━━━━━━━━━━━━━━━━ 1s 10ms/step - loss: 0.3079 - sparse_categorical_accuracy: 0.8566
Epoch 8/10123/123 ━━━━━━━━━━━━━━━━━━━━ 1s 9ms/step - loss: 0.3050 - sparse_categorical_accuracy: 0.8582
Epoch 9/10123/123 ━━━━━━━━━━━━━━━━━━━━ 1s 9ms/step - loss: 0.3021 - sparse_categorical_accuracy: 0.8595
Epoch 10/10123/123 ━━━━━━━━━━━━━━━━━━━━ 1s 9ms/step - loss: 0.2992 - sparse_categorical_accuracy: 0.8617
Model training finished
Evaluating the model on the test data...62/62 ━━━━━━━━━━━━━━━━━━━━ 5s 39ms/step - loss: 0.3145 - sparse_categorical_accuracy: 0.8503
Test accuracy: 85.55%
相关文章:
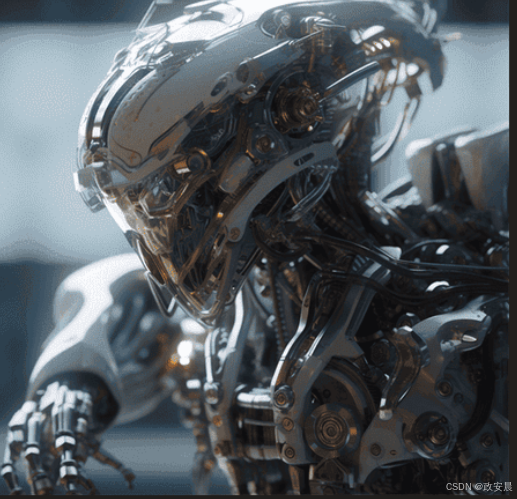
政安晨:【Keras机器学习示例演绎】(五十四)—— 使用神经决策森林进行分类
目录 导言 数据集 设置 准备数据 定义数据集元数据 为训练和验证创建 tf_data.Dataset 对象 创建模型输入 输入特征编码 深度神经决策树 深度神经决策森林 实验 1:训练决策树模型 实验 2:训练森林模型 政安晨的个人主页:政安晨 欢…...

洞察消费者心理:Transformer模型在消费者行为分析的创新应用
洞察消费者心理:Transformer模型在消费者行为分析的创新应用 在数字化时代,消费者行为分析对于企业理解市场动态、制定营销策略至关重要。Transformer模型,以其在处理序列数据方面的优势,为消费者行为分析提供了新的视角和工具。…...

如何安全使用代理ip
1、选择可靠的代理服务提供商:选择知名的、信誉良好的代理服务提供商,避免使用免费的代理服务,因为免费的代理服务可能存在安全隐患。 2、使用HTTPS代理:使用HTTPS代理可以加密你的网络流量,保护你的隐私和安全。 3、…...

机器学习——LR、GBDT、SVM、CNN、DNN、RNN、Word2Vec等模型的原理和应用
LR(逻辑回归) 原理: 逻辑回归模型(Logistic Regression, LR)是一种广泛应用于分类问题的统计方法,尤其适用于二分类问题。其核心思想是通过Sigmoid函数将线性回归模型的输出映射到(0,1)区间,从…...

揭秘SQL Server数据库选项:性能与行为的调控者
揭秘SQL Server数据库选项:性能与行为的调控者 在SQL Server的世界中,数据库选项是那些可以调整以优化数据库性能和行为的设置。它们是数据库管理员和开发者的得力助手,通过精细调控,可以显著提升数据库的响应速度和资源利用率。…...

【排序 - 选择排序优化版(利用堆排序)】
结合选择排序和堆排序的思路,可以通过利用堆数据结构来优化选择排序的过程,使得排序算法更加高效。在这种结合中,我们利用堆的特性来快速定位和选择未排序部分的最小元素,避免了选择排序中每次线性搜索的开销。 选择排序和堆排序…...

PHP编程开发工具有哪些?
PHP的开发工具种类繁多,涵盖了从集成开发环境(IDE)、代码编辑器、调试器到版本控制工具和数据库管理工具等多个方面。以下是一些常见的PHP开发工具: 1. 集成开发环境(IDE) PhpStorm:由JetBrai…...
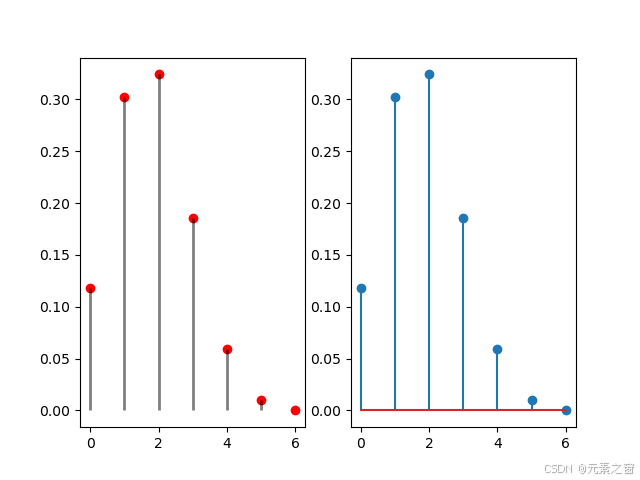
火柴棒图python绘画
使用Python绘制二项分布的概率质量函数(PMF) 在这篇博客中,我们将探讨如何使用Python中的scipy库和matplotlib库来绘制二项分布的概率质量函数(PMF)。二项分布是统计学中常见的离散概率分布,描述了在固定次…...
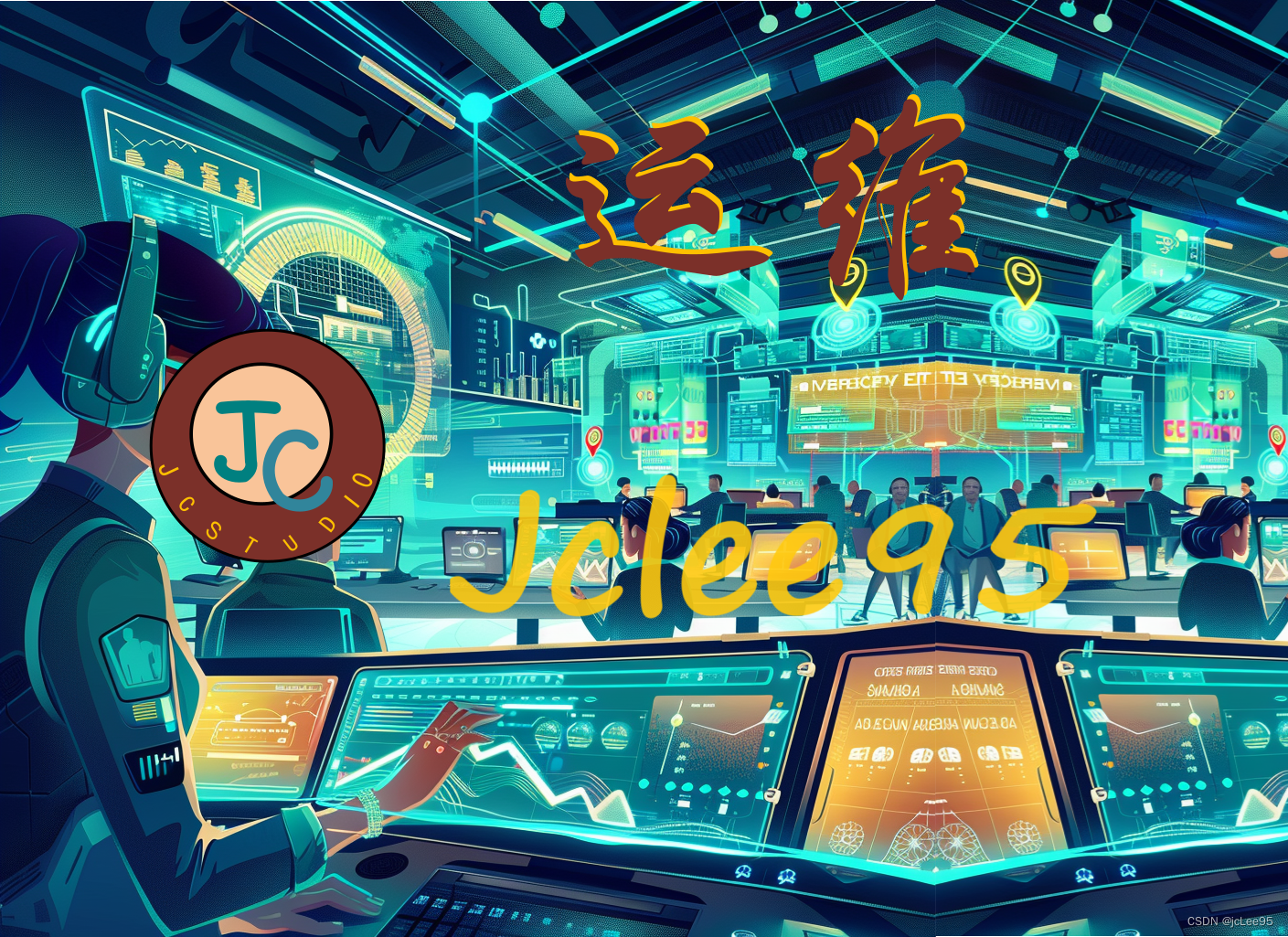
Nginx七层(应用层)反向代理:UWSGI代理uwsgi_pass篇
Nginx七层(应用层)反向代理 UWSGI代理uwsgi_pass篇 - 文章信息 - Author: 李俊才 (jcLee95) Visit me at CSDN: https://jclee95.blog.csdn.netMy WebSite:http://thispage.tech/Email: 291148484163.com. Shenzhen ChinaAddress of this a…...

Effective C++笔记之二十一:One Definition Rule(ODR)
ODR细节有点复杂,跨越各种情况。基本内容如下: ●普通(非模板)的noninline函数和成员函数、noninline全局变量、静态数据成员在整个程序中都应当只定义一次。 ●class类型(包括structs和unions)、模板&…...

探索未来:Transformer模型在智能环境监测的革命性应用
探索未来:Transformer模型在智能环境监测的革命性应用 在当今数字化时代,环境监测正逐渐从传统的人工检测方式转变为智能化、自动化的系统。Transformer模型,作为深度学习领域的一颗新星,其在自然语言处理(NLP&#x…...

Nginx中文URL请求404
这两天正在搞我的静态网站。方案是:从思源笔记Markdown笔记,用MkOcs build成静态网站,上传到到Nginx服务器。遇到一个问题:URL含有中文会404,全英文URL则正常访问。 比如: 设置了utf-8 ht…...

33. 动量法(Momentum)介绍
1. 背景知识 在深度学习的优化过程中,梯度下降法(Gradient Descent, GD)是最基本的方法。然而,基本的梯度下降法在实际应用中存在收敛速度慢、容易陷入局部最小值以及在高维空间中振荡较大的问题。为了解决这些问题,人…...
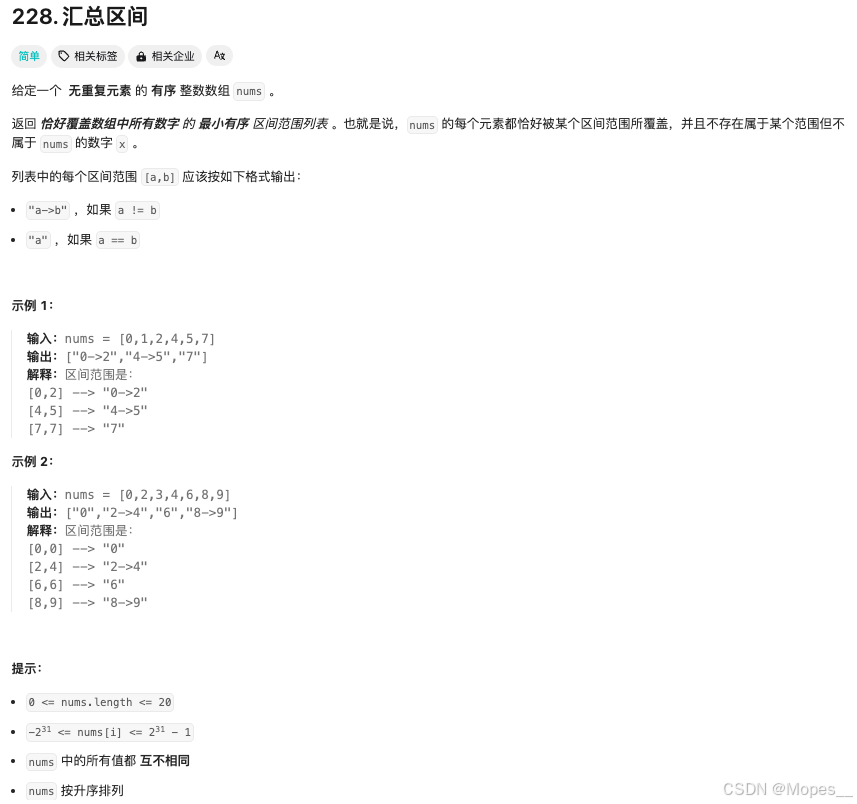
Python | Leetcode Python题解之第228题汇总区间
题目: 题解: class Solution:def summaryRanges(self, nums: List[int]) -> List[str]:def f(i: int, j: int) -> str:return str(nums[i]) if i j else f{nums[i]}->{nums[j]}i 0n len(nums)ans []while i < n:j iwhile j 1 < n …...

物联网应用,了解一点 WWAN全球网络标准
WWAN/蜂窝无线电认证,对跨地区应用场景,特别重要。跟随全球业务的脚步,我们像大唐先辈一样走遍全球业务的时候,了解一点全球化的 知识信息,就显得有那么点意义。 NA (北美):美国和加…...
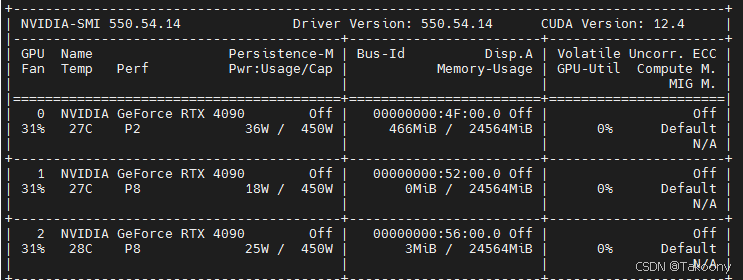
如何指定多块GPU卡进行训练-数据并行
训练代码: train.py import torch import torch.nn as nn import torch.optim as optim from torch.utils.data import DataLoader, Dataset import torch.nn.functional as F# 假设我们有一个简单的文本数据集 class TextDataset(Dataset):def __init__(self, te…...

RK3568笔记三十三: helloworld 驱动测试
若该文为原创文章,转载请注明原文出处。 报着学习态度,接下来学习驱动是如何使用的,从简单的helloworld驱动学习起。 开始编写第一个驱动程序—helloworld 驱动。 一、环境 1、开发板:正点原子的ATK-DLRK3568 2、系统…...

【智能制造-14】机器视觉软件
CCD相机和COMS相机? CCD(Charge-Coupled Device)相机和CMOS(Complementary Metal-Oxide-Semiconductor)相机是两种常见的数字图像传感器技术,用于捕捉和处理图像。 CCD相机: CCD相机使用一种称为CCD的光电…...
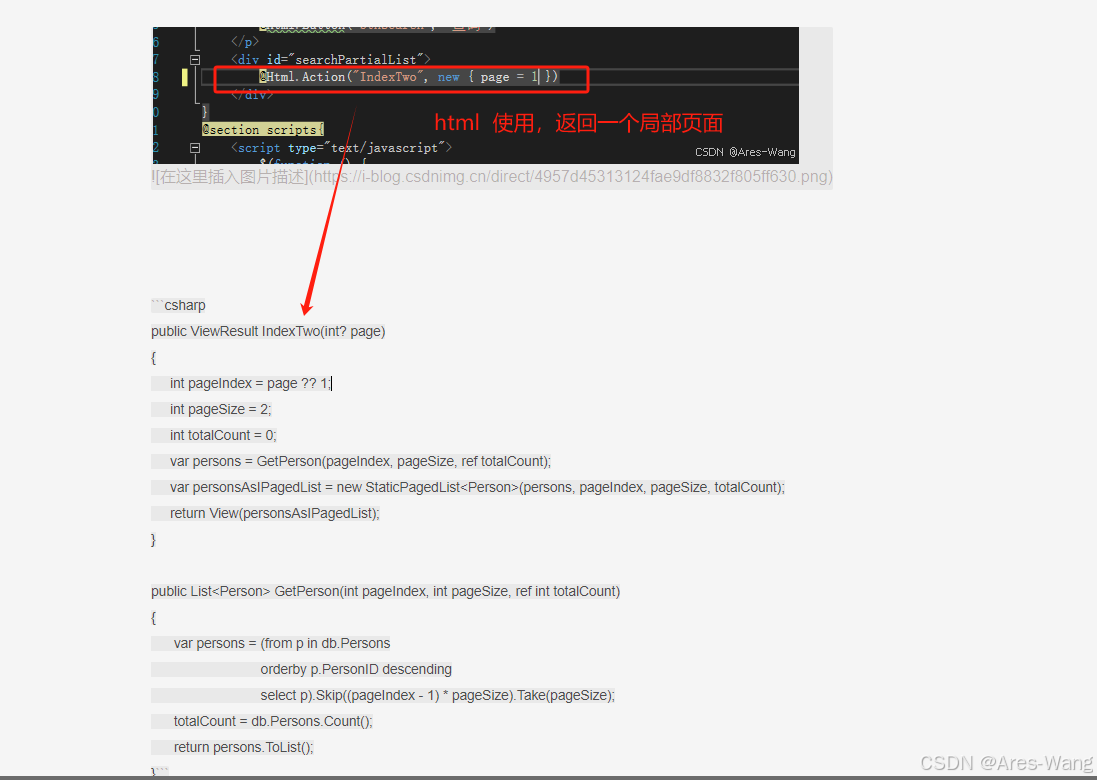
MVC分页
public ActionResult Index(int ? page){IPagedList<EF.ACCOUNT> userPagedList;using (EF.eMISENT content new EF.eMISENT()){第几页int pageNumber page ?? 1;每页数据条数,这个可以放在配置文件中int pageSize 10;//var infoslist.C660List.OrderBy(…...
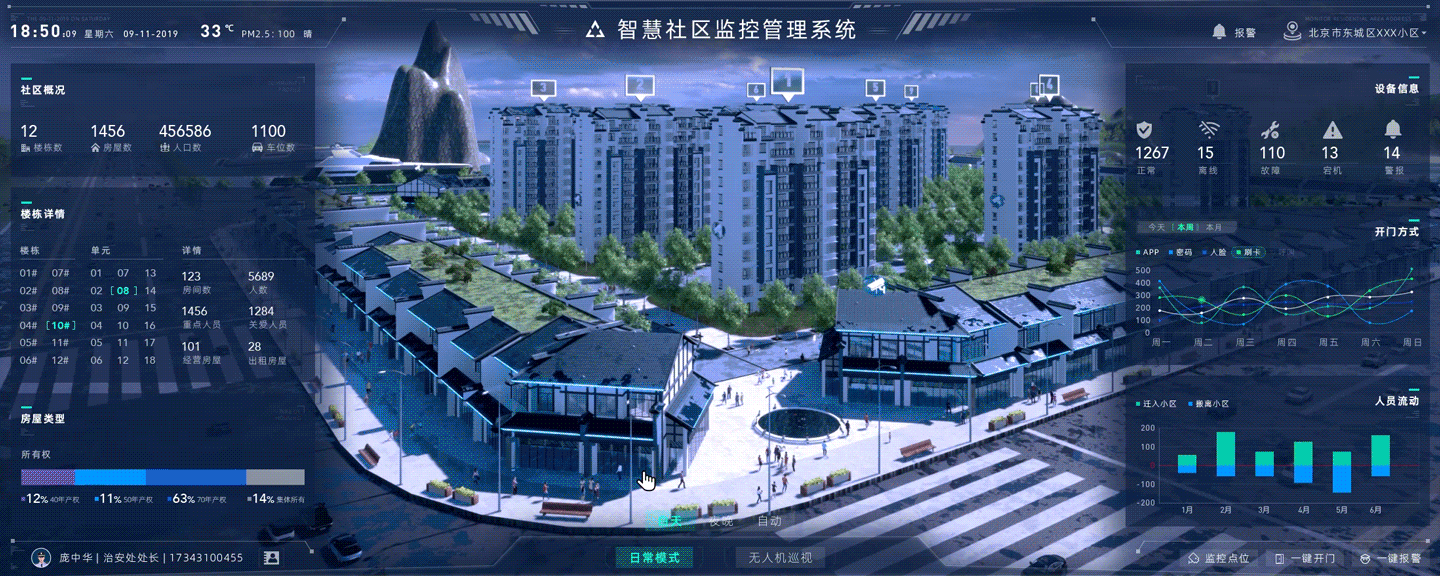
webGL可用的14种3D文件格式,但要具体问题具体分析。
hello,我威斯数据,你在网上看到的各种炫酷的3d交互效果,背后都必须有三维文件支撑,就好比你网页的时候,得有设计稿源文件一样。WebGL是一种基于OpenGL ES 2.0标准的3D图形库,可以在网页上实现硬件加速的3D图…...
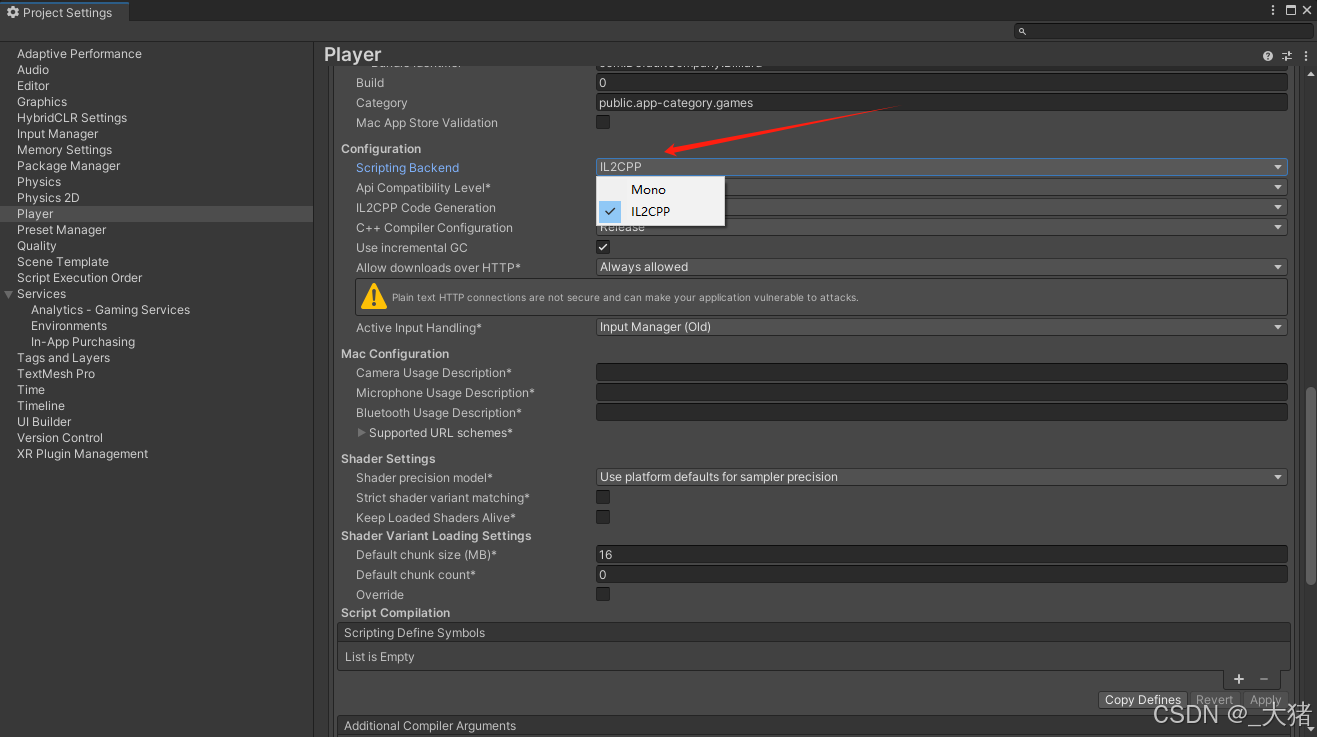
HybridCLR原理中的重点总结
序言 该文章以一个新手的身份,讲一下自己学习的经过,大家更快的学习HrbirdCLR。 我之前的两个Unity项目中,都使用到了热更新功能,而热更新的技术栈都是用的HybridCLR。 第一个项目本身虽然已经集成好了热更逻辑(使用…...
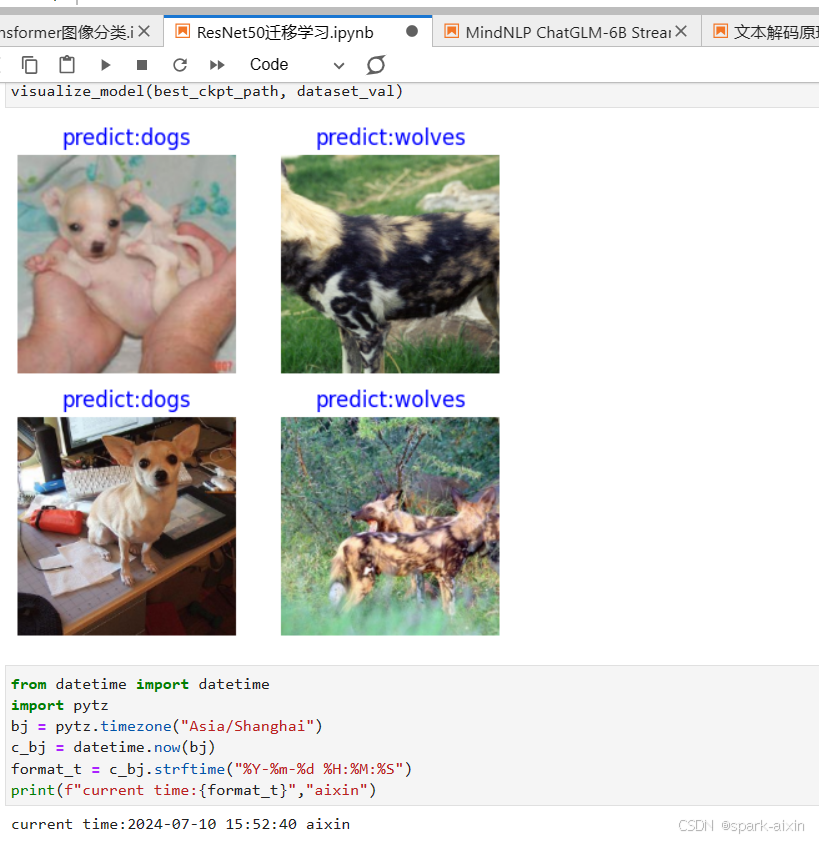
昇思学习打卡-14-ResNet50迁移学习
文章目录 数据集可视化预训练模型的使用部分实现 推理 迁移学习:在一个很大的数据集上训练得到一个预训练模型,然后使用该模型来初始化网络的权重参数或作为固定特征提取器应用于特定的任务中。本章学习使用的是前面学过的ResNet50,使用迁移学…...

软件开发面试题C#,.NET知识点(续)
1.C#中的封装是什么,以及它的重要性。 封装(Encapsulation) 是面向对象编程(OOP)的一个基本概念。它指的是将对象的状态(属性)和行为(方法)绑定在一起,并且将…...

2019年美赛题目Problem A: Game of Ecology
本题分析: 本题想要要求从实际生物角度出发,对权力游戏中龙这种虚拟生物的生态环境和生物特性进行建模,感觉属于比较开放类型的题目,重点在于参考生物的选择,龙虽然是虚拟的但是龙的生态特性可以参考目前生物圈里存在…...
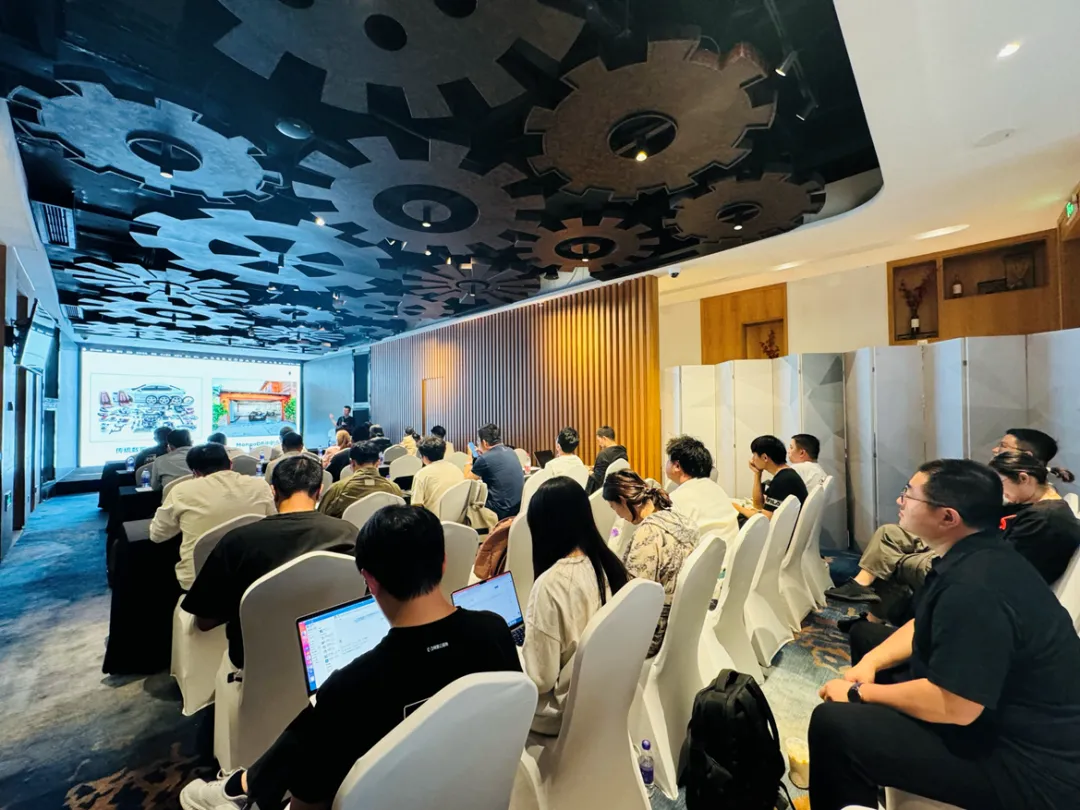
沙龙回顾|MongoDB如何充当企业开发加速器?
数据不仅是企业发展转型的驱动力,也是开发者最棘手的问题。前日,MongoDB携手阿里云、NineData在杭州成功举办了“数据驱动,敏捷前行——MongoDB企业开发加速器”技术沙龙。此次活动吸引了来自各行各业的专业人员,共同探讨MongoDB的…...

云端编码:将您的技术API文档安全存储在iCloud的最佳实践
云端编码:将您的技术API文档安全存储在iCloud的最佳实践 作为一名技术专业人士,管理不断增长的API文档库是一项挑战。iCloud提供了一个无缝的解决方案,允许您在所有设备上存储、同步和访问您的个人技术API文档。本文将指导您如何在iCloud中高…...

在Spring Boot项目中集成单点登录解决方案
在Spring Boot项目中集成单点登录解决方案 大家好,我是微赚淘客系统3.0的小编,也是冬天不穿秋裤,天冷也要风度的程序猿! 在现代的企业应用中,单点登录(Single Sign-On, SSO)解决方案是确保用户…...
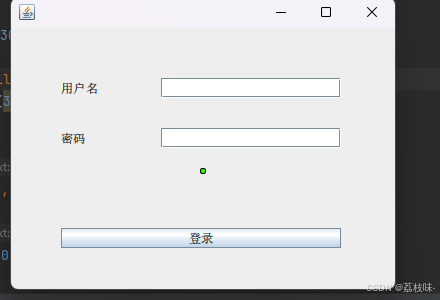
Java-常用API
1-Java API : 指的就是 JDK 中提供的各种功能的 Java类。 2-Scanner基本使用 Scanner: 一个简单的文本扫描程序,可以获取基本类型数据和字符串数据 构造方法: Scanner(InputStream source):创建 Scanner 对象 Sy…...

Python从Excel表中查找指定数据填入新表
#读取xls文件中的数据 import xlrd file "原表.xls" wb xlrd.open_workbook(file) #读取工作簿 ws wb.sheets()[0] #选第一个工作表 data [] for row in range(7, ws.nrows): name ws.cell(row, 1).value.strip() #科室名称 total1 ws.cell(row, 2…...
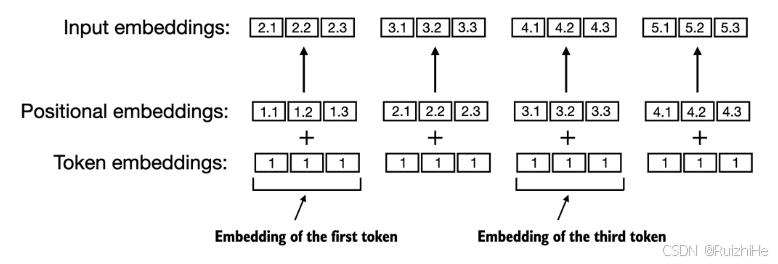
从零开始实现大语言模型(三):Token Embedding与位置编码
1. 前言 Embedding是深度学习领域一种常用的类别特征数值化方法。在自然语言处理领域,Embedding用于将对自然语言文本做tokenization后得到的tokens映射成实数域上的向量。 本文介绍Embedding的基本原理,将训练大语言模型文本数据对应的tokens转换成Em…...

平台营销型网站建设/上海网站快速排名优化
编辑距离 编辑距离(Edit Distance),又称Levenshtein距离,是指两个字串之间,由一个转成另一个所需的最少编辑操作次数。编辑操作包括将一个字符替换成另一个字符,插入一个字符,删除一个字符。一般…...
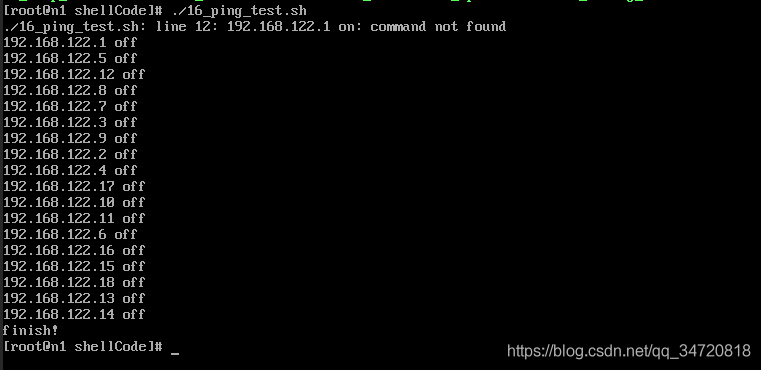
外贸婚纱网站 侵权/产品如何推广
Lunix Shell编程入门 前言 要想玩转类Unix系统,仅仅会基本的常用操作命令还是远远不够的,本文介绍lunix系统下的Shell编程,通过一系列的简单Shell代码示例,一步步入门Shell编程。 一、Shell是什么? Linux系统被比作…...
wordpress旅行地图主题/成人职业培训机构
第九届北京高中数学知识应用竞赛初赛 第二题原题:一只老鼠为了躲避猫的追捕,跳入了半径为R的圆形湖中.猫不会游泳,只能沿湖岸追击,并且总是试图使自己离老鼠最近(即猫总是试图使自己在老鼠离岸最近的点上)…...

wordpress插件选项/百度推广客户端怎么登陆
Stack继承Vector类,它通过五个操作对类 Vector 进行了扩展。 栈是 后进先出的。 栈提供了通常的 push 和 pop 操作,以及取堆栈顶点的 peek 方法、测试堆栈是否为空的 empty 方法、在堆栈中查找项并确定到堆栈顶距离的 search 方法。 方法摘要 booleanemp…...

网站推广好做吗/广州网站制作实力乐云seo
public int findTheWinner(int n, int k) {int ans0;for(int i2;i<n;i){//最后的人一定在0位置处,每次去掉一人,他的位置左移k,要想知道他的初始位置则要kans(ansk)%i;}return ans1;}...

wordpress页眉导航栏位置/腰肌劳损的自我治疗和恢复的方法有什么?
1.Ubuntu系统,使用FFmpeg命令需要先安装 sudo apt install ffmpeg安装成功之后就可以使用ffmpeg 、ffplay、ffprobe等命令 2.格式转换 ffmpeg -i fly.mp4 fly.flv3.播放视频 ffplay fly.flv 4.查看视频信息 ffprobe fly.mp4Input #0, mov,mp4,m4a,3gp,3g2,mj2,…...